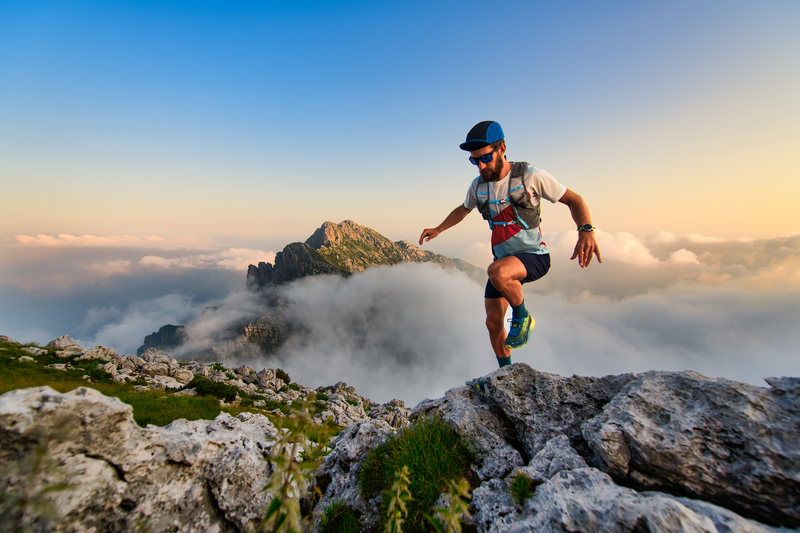
95% of researchers rate our articles as excellent or good
Learn more about the work of our research integrity team to safeguard the quality of each article we publish.
Find out more
PERSPECTIVE article
Front. Ecol. Evol. , 21 December 2020
Sec. Evolutionary Ecology of Social Behaviour
Volume 8 - 2020 | https://doi.org/10.3389/fevo.2020.586058
Recent findings indicate that the utilization of social information, produced inadvertently by other individuals through their spatial location and/or interaction with the environment, may be ubiquitous in the animal kingdom. If so, social information-mediated effects on population growth and interspecies interactions may be more prevalent than previously thought. However, little is known about how social information may spread among non-grouping individuals, i.e., in animals that do not form cohesive groups and therefore social attraction among group-mates does not facilitate information diffusion. Are there any perception-related, temporal, and/or spatial parameters that may facilitate or limit the spread of social information in temporary aggregations or among dispersed individuals in a population? We argue that living in cohesive groups is not necessarily required for the diffusion of social information and for social information-mediated effects to emerge in a population. We propose that while learning complex problem-solving techniques socially is less likely to occur in non-grouping animals, the spread of adaptive responses to social stimuli, especially to non-visual cues, can be common and may affect population, and/or community dynamics in a wide range of taxa. We also argue that network-based diffusion analysis could be a suitable analytical method for studying information diffusion in future investigations, providing comparable estimations of social effects on information spread to previous studies on group-living animals. We conclude that more studies are warranted to verify what intrinsic and extrinsic factors influence information propagation among incidentally and/or indirectly interacting individuals if we are to better understand the role of social information in animal populations and how the social and ecological characteristics of species are related to information spread in natural communities.
There is a great diversity of animal assemblies in nature, which can vary in composition, structure, and function (Krause and Ruxton, 2002). Same-species groups can be open, so individuals are free to join or leave, like in the foraging, and/or wintering flocks of many bird species. In others, entry to the group is restricted and group membership is more or less consistent over time, as in various reproductive family groups of primates. In some species, conspecific individuals with similar physiological characteristics and motivation assemble in aggregations and display some temporary synchrony in their patterns of activity (e.g., some fish shoals or locust swarms), while in other organisms, like eusocial hymenopterans, they form highly structured, and stable societies (Ward and Webster, 2016). Animals often aggregate with heterospecifics and create mixed-species groups as well, especially when they share resources or are subject to the same threats (Goodale et al., 2017, 2020). Because of the apparent diversity in animal grouping behavior, however, the dichotomous categorization of animals into group-living and solitary species can be misleading. Species commonly regarded as solitary may aggregate periodically when resources (such as food, water, mates, or shelter) become patchily distributed (e.g., Laidre, 2010), individuals may regularly interact with conspecifics in neighboring territories (e.g., Giuggioli et al., 2011), or live in a closed family unit while receiving parental care at an early life stage (e.g., Elbroch et al., 2017). Within the same species, grouping behavior can also change with age, between seasons, or differ between males and females (Schradin et al., 2020; Shizuka and Johnson, 2020). Animals that form groups are not necessarily social, and many species that are referred to as being social exhibit a wide distribution of group sizes including many singletons, so not all individuals are part of a social group at a given time (Krause and Ruxton, 2002). In the present work, we use the term “cohesive group” to refer to those permanent or periodical group formations, in which animals exhibit social attraction toward other group-members, thus actively seek and maintain close spatial proximity (Figure 1). On the other end of the grouping spectrum, we define non-grouping animals as those individuals that may aggregate or form temporary groups under specific abiotic environmental conditions, but do not show attraction to conspecifics. We collected evidence that social information use occurs in non-grouping animals that do not form cohesive groups, describe those circumstances under which one might expect social information to diffuse among individuals, and suggest a suitable analytical approach to study such information diffusion in non-grouping animals.
Figure 1. General concept of the adopted distinction between animals living in cohesive groups and non-grouping animals. Arrows represent the movement vectors of individuals, solid circles denote the maximum distances within which animals maintain spatial proximity with their group-mates, dashed circles show their perception ranges. In (A), members of a cohesive group exhibit social attraction toward group-mates and, as a result, maintain close spatial proximity. Due to such short inter-individual distances, perception ranges of group-members greatly overlap; social attraction often leads to synchronized movements as well. In (B), there is no social cohesion among individuals, but through the observation of spatially distributed others within their perception range (or coming across remnants/scent marks at a different time) non-grouping animals have the possibility to detect social cues. Such cues are not limited to visual ones, but they can also be acoustic, chemical, and vibration-related, etc. For simplicity, perception ranges are only a function of distance.
We use “social information use” as a broad term to denote all cases when social stimuli influence decision options among individuals. This term thus also refers to those incidences when the presence, routine behavior, or the product of the behavior of other individuals convey information to the observers about the environment or themselves. In that sense, unless “social learning” has occurred (i.e., the presence of a conditioning phase; Leadbeater, 2015), most natural occurrences of eavesdropping (Lea et al., 2008; Martínez et al., 2018), mate copying (Mennill et al., 2002; Witte and Noltemeier, 2002; White, 2004), habitat copying (Nocera et al., 2006; Parejo et al., 2007; Battesti et al., 2012), choosing among foraging alternatives (Templeton and Giraldeau, 1995; Coolen et al., 2003; Danchin et al., 2004), or responding to the predator avoidance behavior of others (Chivers and Smith, 1998; Magrath et al., 2015) can be regarded as examples of social information use. The exploitation of social cues is often considered adaptive, because it reduces the time, and/or energy required for gaining updated information about the surrounding environment (Dall et al., 2005; Grüter and Leadbeater, 2014; Duboscq et al., 2016; but see Sigaud et al., 2017). The advantages of living in cohesive groups are often thought to include the opportunity to access social information by observing the actions of others or the product of those actions (Krause and Ruxton, 2002; Ward and Webster, 2016; Goodale et al., 2017). Individuals may also follow various rules like when to copy group-mates or alter behavior based on personal information (Kendal et al., 2009, 2018). However, most animals encounter conspecifics and heterospecifics at specific times, and may routinely encounter others indirectly through scent marks, excretions or food remnants, all of which can provide relevant information about prevalent environmental conditions (Webster and Laland, 2017). Therefore, there is a high likelihood that most species use social information when such information is available and its use is beneficial (Heyes and Pearce, 2015; Heyes, 2016).
A number of studies have provided experimental evidence that social information use is present in non-grouping animals. Whiting and Greeff (1999) showed that flat lizards (Platysaurus broadleyi) use bird activity as a social cue during foraging to locate Namaqua fig trees (Ficus cordata cordata) with ripe fruits. Bearded dragons (Pogona vitticeps) and red-footed tortoises (Geochelone carbonaria) were found to be able to overcome physical obstacles to get access to food when they had the opportunity to observe the actions of others, but not in the absence of a conspecific demonstrator (Wilkinson et al., 2010; Kis et al., 2015). Similarly, juvenile Port Jackson sharks (Heterodontus portusjacksoni) are more successful in a novel foraging task in the presence of a trained conspecific compared to individual foragers or those paired with sham demonstrators (Pouca et al., 2020). Wild-caught wood crickets (Nemobius sylvestris) utilize and transmit information on predation threat represented by wolf spiders, and naïve individuals change their behavior after having observed the predator avoidance behavior of knowledgeable conspecifics (Coolen et al., 2005). These changes last even after the removal of demonstrators and can modify the behavior of other naïve crickets, too. Individuals of four non-grouping and two shoaling fish species originating from natural populations were shown to similarly exploit social cues that were provided by groups of shoal-forming heterospecifics during a foraging task (Webster and Laland, 2017). Male Betta splendens acquire information on the relative fighting ability of others through the observation of aggressive interactions between neighboring conspecifics (Oliveira et al., 1998). In North-American red squirrels (Tamiasciurus hudsonicus), Dantzer et al. (2013) showed that females use conspecifics’ territorial vocalization as a social cue to estimate population density and adjust their offspring growth accordingly through a hormone-mediated maternal effect. Male American green tree frogs (Hyla cinerea) eavesdrop on competing males to estimate their competitive environment and adjust their behavior based on their own body size and the call characteristics of surrounding competitors (Garcia et al., 2019). The collared pika (Ochotona collaris), a small alpine lagomorph, is able to exploit both conspecific and heterospecific alarm calls in their acoustic environment during behavioral decisions on foraging and predator avoidance (Trefry and Hik, 2009). Terrestrial hermit crabs (Coenobita compressus) use social cues produced by conspecific competitors to find food and shelter (Laidre, 2010). These results support the idea that responding adaptively to social stimuli may be taxonomically widespread, advantageous in various ecological scenarios, and, similarly to the utilization of other biotic or abiotic cues in the environment, not strongly associated with sociality or permanent group-living.
As the above examples suggest, social information may originate not only from conspecifics, heterospecific individuals are also likely to be an important, and for a long time overlooked, source of social information (Seppänen et al., 2007; Webster and Laland, 2017; Sridhar and Guttal, 2018). Using social information produced by heterospecifics might be preferable when territoriality or competition among conspecific individuals limit the frequency of direct interactions (Goodale et al., 2020); in line with that, foraging in mixed-species groups has been predicted to be more beneficial under several ecological scenarios than foraging in single species groups (Gil et al., 2017). Non-grouping animals may encounter heterospecific social cues more frequently than cues originating from conspecifics; on the other hand, such cues can be less reliable or relevant as well. Some benefits of social information use is likely to be obtainable only from conspecifics (e.g., those related to reproduction), others only from dissimilar heterospecifics (e.g., when social cue production is related to an ability that is lacking in the other species), while there is a range of benefits that can, in principle, come either from conspecifics or heterospecifics (Sridhar and Guttal, 2018). Potential difference in the production, strength and reliability of social information between conspecific and heterospecific sources is an exciting aspect of social information use, but its detailed discussion is beyond the scope of this study. Instead, we focus on scrutinizing what conditions may favor the spread of social information in non-grouping animals when reliable social cues – originating either from conspecific or heterospecific individuals – are present.
When social information spreads among individuals, social cues generate behavioral responses beyond their original detection range, as preceding observers become potential demonstrators themselves. For instance, if the detection of a predator induces reduced activity, the behavioral response of the first individual sensing predation risk may serve as a social cue for nearby conspecifics. If so, a second animal may detect this behavioral response, and adjusts its own behavior accordingly. This social cue, in turn, may also elicit a similar behavioral response in a third individual (and so on), so the information about predation threat may spread further away from the location of the predator as long as there are demonstrators within the perception range of potential observers. This phenomenon can occur irrespective of the presence of social attraction between individuals. In line with the terminology used by Hoppitt and Laland (2013), we refer to the spread of social information associated with a detectable trait or behavior of other individuals (including routine behavior or the product of that behavior) in a population or community as diffusion of social information. This is a more general term than social transmission, which occurs when first the acquisition of a (most often novel) trait takes place by an individual (Hoppitt and Laland, 2013), so its spread increases the skill/trait set characteristic to a given animal collective. From the above examples, for instance, one may also expect that the diffusion of social information about predation risk may occur in wood crickets, but information about conspecific density will not spread in North-American red squirrels as in the latter species observers do not become demonstrators, i.e., social cue producers, themselves.
There are several intriguing questions related to the diffusion of social information in a population of non-grouping animals. How far can social information spread in space and how long will it persist? What type of perception modalities may play a role in its diffusion? What kind of information (in terms of complexity) can disperse among individuals? We argue that being a member of a cohesive group is not essential for information spread itself, but the diffusion of social information is likely to differ between non-grouping animals and those living in cohesive groups. Such differences may exist either in the perception of the social cues, in the motivation to react to such cues, and/or in the complexity of behavioral adjustment to the perceived cues. In cohesive groups, social attraction maintains a local density that generates opportunity for the acquisition of social information, so the per unit time acquisition of information is expected to be high in such groups. In non-grouping animals, the frequency of direct interactions between individuals can be incidental, and maintaining spatial proximity to conspecifics may incur high costs (i.e., in the form of increased probability of aggressive interactions). However, social diffusion may take place if social cue producers emerge within the perception ranges of potential observers without the necessity of direct interactions between them. Individuals may interact not only via direct encounters, but also indirectly in a variety of ways (e.g., auditory, chemosensory, and electro-sensory, etc.), all of which may have important implications for the acquisition and spread of social information. Chemical social cues may last for an extended period and remain detectable, in which case short inter-individual distances between observers and social cue producers are not necessarily required. On the other hand, such social cues may also need to reach a threshold concentration to elicit behavioral responses from others (e.g., Mirza and Chivers, 2003).
From a motivational perspective, individuals may rely more frequently on personal information, i.e., gather information by directly interacting with the environment, and social information use may not take place when the already acquired personal information is equally relevant in a given situation (Laland, 2004; Galef and Laland, 2005). In specific contexts, however, non-grouping species might be paying more attention to the potential for social cues, and therefore the per unit of opportunity rate of information diffusion could be similarly high or higher than in cohesive groups. In terms of behavioral adjustment, one may expect that the spread of social information related to complex problem-solving techniques or novel motor skills may be rare outside of cohesive groups as it is often restricted to visual observations and would require risky, direct encounters with demonstrators (e.g., Aplin et al., 2015). On the other hand, cues associated with the routine behavior of other individuals can commonly elicit similar responses in naïve observers and may diffuse among multiple individuals in non-grouping animals as well.
The ecological significance of social information lies in the possibility of finding strong correlations in the behaviors and space use of many individuals (Gil et al., 2018). Living in cohesive groups may shape those perceptual, attentional or motivational processes that play important roles in the acquisition and utilization of social information (Heyes, 2012), but, as seen above, non-grouping animals can also benefit from exploiting social cues in certain contexts. Individuals can experience selection for exploiting social cues whenever they are exposed to potential information producers as long as this action yields fitness advantages that exceeds associated costs (Goodale et al., 2010; Rieucau and Giraldeau, 2011). According to the predictions of mathematical models on social information use, such advantageous cost-benefit ratios may occur across various contexts (e.g., in foraging and predator avoidance scenarios; Gil et al., 2017, 2018). When social information indeed disperses among individuals, information-mediated effects (see below) may be substantial in a population (although diffusion is not necessarily a prerequisite for a population-level effect to emerge; e.g., see Dantzer et al., 2013). Gil et al. (2018) summarized evidence indicating that social information may mediate positive density dependence in a wide range of systems, also without the necessity of forming cohesive groups. They proposed that the benefit of enhanced access to social information could outweigh competition especially at low local densities, leading to a temporary positive feedback loop between social information availability and population growth. Moreover, when social information affects predator evasion, the population size at equilibrium can be higher than without social information use and the prey population may persist under higher baseline predation rate relative to the per capita growth rate (Gil et al., 2018). At the community level, even if two species compete for the same resource, social information on predation risk shared by heterospecific individuals can result in the stable coexistence of prey populations (Parejo and Avilés, 2016; Gil et al., 2018, 2019). These model predictions suggest that social information may more frequently mediate population growth, population stability and interspecific interactions than previously considered.
If social cues are as any other predictive environmental cues, all observers present within the range of the relevant sensory perception can have the opportunity to exploit those particular cues (although they will not necessarily do that). Then, variation in information use is most likely caused by being present or absent when a demonstration event occurs, or by being present or absent within the detection range of the social cue if it has an extended lifespan (i.e., in the case of non-volatile chemical cues). The key criterion is to know which individuals are within the relevant sensory perception range of a given social cue, while that range depends on both the cue itself (i.e., whether it is visual, auditory, and chemical, etc.) and the organism in question. However, defining cue detection through some perception modes can be challenging. For instance, chemical cues are known to play substantial roles in various life processes in both terrestrial and aquatic organisms, but modeling their detection requires quantifying and considering all physical and biological parameters (e.g., velocity, flow regime, and individual movements) that affect the transportation of the released chemicals through the medium in question (Webster and Weissburg, 2009).
Alternatively, one can use spatial proximity networks when sensory networks are not possible or logistically challenging to construct. In both cases, individual location should be recorded together with the time and duration of their behavioral response to a detectable social cue, so social cue producers (i.e., demonstrators) and potential observers can be identified and monitored together with their sensory range or spatial position among individuals. The resulting “demonstration networks” incorporate data about when a particular behavior was performed, by whom, and who observed (potentially) each event (e.g., as in Hobaiter et al., 2014). In such networks, the diffusion of social information can be detected if the incidental observation of others performing the target behavior predicts its diffusion through the network using network-based diffusion analysis (NBDA; Franz and Nunn, 2009; Hoppitt et al., 2010; Hoppitt and Laland, 2011; Krause et al., 2015; Hoppitt, 2017). NBDA quantifies the effect of social transmission on individuals’ acquisition of a new trait or information based on their connections with other individuals in a network. If social transmission is present, acquisition rate is expected to accelerate when connected individuals demonstrate the target trait (either a routine behavior or a new skill). During model fitting, parameter s, the rate of social transmission per unit connection relative to the rate of asocial learning, is estimated. This can be used then to calculate the estimated proportion of learning events that occurred by social transmission (as opposed to asocial learning; Hoppitt, 2017), which is a more comprehendible measure of social transmission than parameter s in itself, and being independent of the scale of the network it allows direct comparison between studies. While most social network methods require specialized statistical techniques for hypothesis testing, NBDA has an inherent null hypothesis (i.e., there is no social transmission) and thus it is not necessary to combine it with randomizations/permutations (Farine and Whitehead, 2015). For a comprehensive guide on how to use NBDA with worked examples and potential issues regarding dynamic or time-aggregated networks, see Hasenjager et al., 2020 (demonstration networks are equivalent to “observation networks” in this paper).
In a demonstration network, connections represent the direct or indirect interactions with other individuals [in the example of a chemical social cue, this would be when individual A leaves a cue at a specific location (as a result of its adjusted behavior) and then a “social” connection in the network would exist if individual B encounters this cue; Figure 2]. Then, the data of the time of acquisition for all individuals is incorporated with this network data, and NBDA determines if “social” connections from the network, expressed as standardized rates, indeed shorten the latency to perform the behavior under study. NBDA can be an efficient alternative method for examining how non-grouping animals use social information. For instance, previous studies typically used an observer-demonstrator setup to examine the behavioral response of non-grouping animals to social cues, when a single or multiple demonstrators performed a specific behavior within the perception range of an observer (for an exception, see Laidre, 2010). Whether information use may also trigger the propagation of social information in a collective of individuals, however, cannot be investigated with that approach. With the proposed application of NBDA on demonstration networks, we believe that one may conduct meaningful comparisons between species regarding the diffusion of social information, and infer possible relationships between the efficiency and rate of information spread and the ecological and social characteristics of the studied species.
Figure 2. Potential application of a “demonstration network.” Nodes represent individuals (circular arrangement of the nodes do not reflect individual spatial positions); directed weighted links between nodes denote the frequency or duration of getting within the perception range of another individual (i.e., potential observation opportunities). The square symbol indicates the individual that responds to an environmental cue, circles denote all other individuals in the study area. Color of the nodes denote whether or not an individual altered its behavior within given period (green versus blue). At t1, ID1 detect a relevant environmental cue and adjusts its behavior accordingly. By doing so, ID1 produces a social cue that is detectable to others. Thus, t1 represents the first exhibition of the target behavior by ID1. At t2, ID2, and ID3 also exhibit this behavioral response and become social information producers themselves. At t3, ID4 also shows the relevant behavioral adjustment. Each of these sets of connections (observed in the 0-t1, 0-t2, and 0-t3 periods, respectively) is characteristic for the individual(s) that performed the target behavior at ti. All connections together represent a demonstration network, which can be split into a series of time-aggregated networks using the time points ti as threshold values. With the application of NBDA on these series, one may investigate whether observation opportunities, expressed as standardized rates, accelerate the diffusion of the studied behavioral response among individuals. In order to do that, NBDA has to be modified to take the series of time-aggregated networks (e.g., as a list object) as input data. Factors that may influence information exchange through such connections are discussed in the “Criteria for the diffusion of social information” section. We used the igraph (Csardi and Nepusz, 2006) and tnet (Opsahl, 2009). R packages to draw the figure.
From a constructed demonstration network, initiation success of each demonstrator can also be calculated and used to investigate what conditions and/or individual characteristics are important for information provision to others. Some phenotypic traits can ultimately affect individuals’ capacity to produce social cues, so phenotypic variation between individuals (e.g., related to age or developmental stage) may affect information diffusion in non-grouping animals as well (Farine et al., 2015; Gil et al., 2018). At the community level, functional traits that transcend species (e.g., similarity in body size may lead to shared predators) can similarly affect information propagation (Hua et al., 2016; Martínez et al., 2016; Zhang et al., 2020). Heterogeneity in group phenotypic composition may either facilitate (variation in knowledge or motivation to copy) or hinder (variation in locomotor activity) information spread; Grüter and Leadbeater (2014) and Kendal et al. (2018) provide excellent overviews about relevant individual characteristics and contexts found previously in group-living species. Adoption of more specific models (e.g., Arganda et al., 2012; Berdahl et al., 2013; Rosenthal et al., 2015) may also help to explore whether common rules for decision making in cohesive groups also apply to non-grouping animals.
Using specific experimental setups, demonstration networks of social cue producers and potential observers can be constructed in a number of non-grouping species in the laboratory or even in the wild. For instance, wood crickets studied by Coolen et al. (2005) can reach a natural density of 120 individuals per m2, corresponding to the estimation of nearest neighbor distances to be less than 8 cm (with 95% probability and assuming pure random patterns). Because of such a short characteristic inter-individual distance, one may expect that there is opportunity for social information-mediated predator avoidance behavior to spread among individuals in their natural habitat. However, it is not known how far (in space) such information may disperse and for how long (in time) it may persist. To address these questions in the laboratory, one may use a large arena, into which a physically separated compartment (e.g., via plexiglass) is installed. Into this compartment, treated (initial) demonstrators can be placed; these individuals will produce social cues for nearby conspecifics. Naïve observers can be put into a starting compartment with multiple exits (e.g., in the opposite corner of the arena) at the beginning of the observation trial, then their exploration of the arena and their potential response to social stimuli near the demonstrators can be recorded. In this setup, the experimenter may manipulate the size of the demonstrator compartment (to estimate perception range using double walls between demonstrators and potential observers) or the mode of sensory detection through the walls bordering the demonstrator compartment (to determine which perception modality is relevant) between trials as well. Network connections between individuals can be established based on a threshold value of inter-individual distance (a proxy for detection range) or by compartment membership if the inner area of the arena is further structured by walls. The complete and immediate cessation of movement in response to the detection of a social cue is not expected, only a significant reduction in activity and most likely as a result of repeated and/or prolonged exposure to individuals exhibiting the target behavior (reduced activity was found to last approximately for 45 min following perturbation in wood crickets). Thus, naïve individuals can encounter social cue producing individuals multiple times and in different parts of the arena. Then, NBDA can be applied to investigate if connections based on these encounters indeed facilitated the acquisition of the target behavior. For example, in an arena divided into nine adjacent compartments, followings between compartments influenced the discovery rate of a novel food patch in temporary aggregations of common frog (Rana temporaria) tadpoles (Tóth, Z. and Jaloveczki, B., unpublished data). Such an influence of one another’s movement in a foraging context was also described in the cohesive groups of threespined sticklebacks (Gasterosteous aculeatus), and termed as an “untransmitted social effect” on learning (Atton et al., 2012, 2014).
In the wild, analogous setups can be applied if social stimuli can be confined to a specific location (e.g., an artificial resource patch) and spatial movement of resident individuals can be tracked in the surrounding area simultaneously. Alternatively, field studies may be conducted in species where inter-individual distances (with overlapping perception ranges) remain more or less stable, e.g., in species that use natural or previously installed artificial constructs for dwelling or nesting. In a collective of nearby burrows (or neighboring territories), for instance, individuals can be exposed remotely to an experimental cue and their behavioral response (acoustic or chemical) to that specific cue may serve as social cues for naïve observers.
Previous research on social information use provided important insights about how differentiated social connections between group-members facilitate the spread of information about food location (Webster et al., 2013; Atton et al., 2014; Tóth et al., 2017), novel song elements (Garland et al., 2017; Owen et al., 2019), foraging techniques (Allen et al., 2013; Claidière et al., 2013; Aplin et al., 2015), or tool use (Hobaiter et al., 2014) within animal collectives or in a population. In this paper, we argued that similar studies on non-grouping animals could further expand our knowledge about the adaptive functions and consequences of the use and diffusion of social information in nature. We also proposed that the construction of demonstration networks based on perception range or spatial position, and follow-up application of NBDA can be a potential avenue for statistical inference. In many non-grouping species, data with sufficient resolution to construct demonstration networks could be obtained if demonstrations are performed only in specific locations that can be monitored closely (as also suggested in Hoppitt, 2017). Mathematical models predict that social information use is likely to be prevalent in species that form temporal aggregations at feeding sites or other resource locations (Gil et al., 2017, 2018), and may also be present in those where individuals occupy small (relative to their perception range) neighboring territories. Forthcoming studies on such non-grouping animals will certainly help to better understand how the routine behavior of individuals may elicit behavioral adjustments in observer conspecifics and may shed light on how different perception modalities contribute to the emergence of social information-mediated effects in natural populations and communities.
The original contributions presented in the study are included in the article/supplementary material, further inquiries can be directed to the corresponding author/s.
All authors listed have made a substantial, direct and intellectual contribution to the work, and approved it for publication.
ZT was supported by the Prémium Postdoctoral Research Program of the Hungarian Academy of Sciences (MTA, PREMIUM-2018-198) and BJ by the Young Researcher Program of the Hungarian Academy of Sciences (MTA, Mv-33/2019).
The authors declare that the research was conducted in the absence of any commercial or financial relationships that could be construed as a potential conflict of interest.
Allen, J., Weinrich, M., Hoppitt, W., and Rendell, L. (2013). Network-based diffusion analysis reveals cultural transmission of lobtail feeding in humpback whales. Science 340, 485–488. doi: 10.1126/science.1231976
Aplin, L. M., Farine, D. R., Morand-Ferron, J., Cockburn, A., Thornton, A., and Sheldon, B. C. (2015). Experimentally induced innovations lead to persistent culture via conformity in wild birds. Nature 518, 538–541. doi: 10.1038/nature13998
Arganda, S., Pérez-Escudero, A., and de Polavieja, G. G. (2012). A common rule for decision making in animal collectives across species. Proc. Natl. Acad. Sci. U S A. 109, 20508–20513. doi: 10.1073/pnas.1210664109
Atton, N., Galef, B. J., Hoppitt, W., Webster, M. M., and Laland, K. N. (2014). Familiarity affects social network structure and discovery of prey patch locations in foraging stickleback shoals. Proc. R. Soc. B 281:20140579. doi: 10.1098/rspb.2014.0579
Atton, N., Hoppitt, W., Webster, M. M., Galef, B. G., and Laland, K. N. (2012). Information flow through threespine stickleback networks without social transmission. Proc. R. Soc. B 279, 4272–4278. doi: 10.1098/rspb.2012.1462
Battesti, M., Moreno, C., Joly, D., and Mery, F. (2012). Spread of social information and dynamics of social transmission within Drosophila groups. Curr. Biol. 22, 309–313. doi: 10.1016/j.cub.2011.12.050
Berdahl, A., Torney, C. J., Ioannou, C. C., Faria, J. J., and Couzin, I. D. (2013). Emergent sensing of complex environments by mobile animal groups. Science 339, 574–576. doi: 10.1126/science.1225883
Chivers, D. P., and Smith, R. J. F. (1998). Chemical alarm signalling in aquatic predator-prey systems: a review and prospectus. Ecoscience 5, 338–352. doi: 10.1080/11956860.1998.11682471
Claidière, N., Messer, E. J., Hoppitt, W., and Whiten, A. (2013). Diffusion dynamics of socially learned foraging techniques in squirrel monkeys. Curr. Biol. 23, 1251–1255. doi: 10.1016/j.cub.2013.05.036
Coolen, I., Bergen, Y. V., Day, R. L., and Laland, K. N. (2003). Species difference in adaptive use of public information in sticklebacks. Proc. R. Soc. B 270, 2413–2419. doi: 10.1098/rspb.2003.2525
Coolen, I., Dangles, O., and Casas, J. (2005). Social learning in noncolonial insects? Curr. Biol. 15, 1931–1935. doi: 10.1016/j.cub.2005.09.015
Csardi, G., and Nepusz, T. (2006). The igraph software package for complex network research. Inter. J. Complex Syst. 1695, 1–9.
Dall, S. R., Giraldeau, L. A., Olsson, O., McNamara, J. M., and Stephens, D. W. (2005). Information and its use by animals in evolutionary ecology. Trends Ecol. Evol. 20, 187–193. doi: 10.1016/j.tree.2005.01.010
Danchin, É, Giraldeau, L.-A., Valone, T. J., and Wagner, R. H. (2004). Public information: from nosy neighbors to cultural evolution. Science 305, 487–491. doi: 10.1126/science.1098254
Dantzer, B., Newman, A. E., Boonstra, R., Palme, R., Boutin, S., Humphries, M. M., et al. (2013). Density triggers maternal hormones that increase adaptive offspring growth in a wild mammal. Science 340, 1215–1217. doi: 10.1126/science.1235765
Duboscq, J., Romano, V., MacIntosh, A., and Sueur, C. (2016). Social Information Transmission in Animals: Lessons from Studies of Diffusion. Front. Psychol. 7:1147. doi: 10.3389/fpsyg.2016.01147
Elbroch, L. M., Levy, M., Lubell, M., Quigley, H., and Caragiulo, A. (2017). Adaptive social strategies in a solitary carnivore. Sci. Adv. 3:e1701218. doi: 10.1126/sciadv.1701218
Farine, D. R., and Whitehead, H. (2015). Constructing, conducting and interpreting animal social network analysis. J. Anim. Ecol. 84, 1144–1163. doi: 10.1111/1365-2656.12418
Farine, D. R., Montiglio, P. O., and Spiegel, O. (2015). From individuals to groups and back: the evolutionary implications of group phenotypic composition. Trends Ecol. Evol. 30, 609–621. doi: 10.1016/j.tree.2015.07.005
Franz, M., and Nunn, C. L. (2009). Network-based diffusion analysis: a new method for detecting social learning. Proc. R. Soc. B 276, 1829–1836. doi: 10.1098/rspb.2008.1824
Galef, B. G., and Laland, K. N. (2005). Social learning in animals: empirical studies and theoretical models. Bioscience 55, 489–499. doi: 10.1641/0006-35682005055
Garcia, M. J., Cronin, A., Bowling, T., Bushera, H., Hunter, K. L., and Taylor, R. C. (2019). Dueling frogs: do male green tree frogs (Hyla cinerea) eavesdrop on and assess nearby calling competitors? Behav. Ecol. Sociobiol. 73:21. doi: 10.1007/s00265-018-2632-1
Garland, E. C., Rendell, L., Lamoni, L., Poole, M. M., and Noad, M. J. (2017). Song hybridization events during revolutionary song change provide insights into cultural transmission in humpback whales. Proc. Natl. Acad. Sci. U S A. 114, 7822–7829. doi: 10.1073/pnas.1621072114
Gil, M. A., Baskett, M. L., and Schreiber, S. J. (2019). Social information drives ecological outcomes among competing species. Ecology 100:e02835. doi: 10.1002/ecy.2835
Gil, M. A., Emberts, Z., Jones, H., and St Mary, C. M. (2017). Social information on fear and food drives animal grouping and fitness. Am. Nat. 189, 227–241. doi: 10.1086/690055
Gil, M. A., Hein, A. M., Spiegel, O., Baskett, M. L., and Sih, A. (2018). Social information links individual behavior to population and community dynamics. Trends Ecol. Evol. 33, 535–548. doi: 10.1016/j.tree.2018.04.010
Giuggioli, L., Potts, J. R., and Harris, S. (2011). Animal interactions and the emergence of territoriality. PLoS Comp. Biol. 7:e1002008. doi: 10.1371/journal.pcbi.1002008
Goodale, E., Beauchamp, G., and Ruxton, G. D. (2017). Mixed-species groups of animals: behavior, community structure, and conservation. London: Academic Press.
Goodale, E., Beauchamp, G., Magrath, R. D., Nieh, J. C., and Ruxton, G. D. (2010). Interspecific information transfer influences animal community structure. Trends Ecol. Evol. 25, 354–361. doi: 10.1016/j.tree.2010.01.002
Goodale, E., Sridhar, H., Sieving, K. E., Bangal, P., Colorado, Z. G. J., Farine, D. R., et al. (2020). Mixed company: a framework for understanding the composition and organization of mixed−species animal groups. Biol. Rev. 95, 889–910. doi: 10.1111/brv.12591
Grüter, C., and Leadbeater, E. (2014). Insights from insects about adaptive social information use. Trends Ecol Evol. 29, 177–184. doi: 10.1016/j.tree.2014.01.004
Hasenjager, M. J., Leadbeater, E., and Hoppitt, W. (2020). Detecting and quantifying social transmission using network−based diffusion analysis. J. Anim. Ecol. 00, 1–9. doi: 10.1111/1365-2656.13307
Heyes, C. (2012). What’s social about social learning? J. Comp. Psychol. 126:193. doi: 10.1037/a0025180
Heyes, C. (2016). Who knows? Metacognitive social learning strategies. Trends Cogn. Sci. 20, 204–213. doi: 10.1016/j.tics.2015.12.007
Heyes, C., and Pearce, J. M. (2015). Not-so-social learning strategies. Proc. R. Soc. B 282:20141709. doi: 10.1098/rspb.2014.1709
Hobaiter, C., Poisot, T., Zuberbühler, K., Hoppitt, W., and Gruber, T. (2014). Social network analysis shows direct evidence for social transmission of tool use in wild chimpanzees. PLoS Biol. 12:e1001960. doi: 10.1371/journal.pbio.1001960
Hoppitt, W. (2017). The conceptual foundations of network-based diffusion analysis: choosing networks and interpreting results. Philos. Trans. R. Soc. B 372:20160418. doi: 10.1098/rstb.2016.0418
Hoppitt, W., and Laland, K. N. (2011). Detecting social learning using networks: a user’s guide. Am. J. Primatol. 73, 834–844. doi: 10.1002/ajp.20920
Hoppitt, W., and Laland, K. N. (2013). Social Learning: An Introduction to Mechanisms, Methods, and Models. Princeton: Princeton University Press.
Hoppitt, W., Boogert, N. J., and Laland, K. N. (2010). Detecting social transmission in networks. J. Theor. Biol. 263, 544–555. doi: 10.1016/j.jtbi.2010.01.004
Hua, F., Yong, D. L., Janra, M. N., Fitri, L. M., Prawiradilaga, D., and Sieving, K. E. (2016). Functional traits determine heterospecific use of risk−related social information in forest birds of tropical South−East Asia. Ecol. Evol. 6, 8485–8494. doi: 10.1002/ece3.2545
Kendal, J., Giraldeau, L.-A., and Laland, K. (2009). The evolution of social learning rules: payoff-biased and frequency-dependent biased transmission. J. Theor. Biol. 260, 210–219. doi: 10.1016/j.jtbi.2009.05.029
Kendal, R. L., Boogert, N. J., Rendell, L., Laland, K. N., Webster, M., and Jones, P. L. (2018). Social learning strategies: Bridge-building between fields. Trends Cogn. Sci. 22, 651–665. doi: 10.1016/j.tics.2018.04.003
Kis, A., Huber, L., and Wilkinson, A. (2015). Social learning by imitation in a reptile (Pogona vitticeps). Anim. Cogn. 18, 325–331. doi: 10.1007/s10071-014-0803-7
Krause, J., James, R., Franks, D. W., and Croft, D. P. (eds) (2015). Animal social networks. New York: Oxford University Press.
Laidre, M. E. (2010). How rugged individualists enable one another to find food and shelter: field experiments with tropical hermit crabs. Proc. R. Soc. B 277, 1361–1369. doi: 10.1098/rspb.2009.1580
Laland, K. N. (2004). Social learning strategies. Anim. Learn. Behav. 32, 4–14. doi: 10.3758/BF03196002
Lea, A. J., Barrera, J. P., Tom, L. M., and Blumstein, D. T. (2008). Heterospecific eavesdropping in a nonsocial species. Behav. Ecol. 19, 1041–1046. doi: 10.1093/beheco/arn064
Leadbeater, E. (2015). What evolves in the evolution of social learning? J. Zool. 295, 4–11. doi: 10.1111/jzo.12197
Magrath, R. D., Haff, T. M., Fallow, P. M., and Radford, A. N. (2015). Eavesdropping on heterospecific alarm calls: from mechanisms to consequences. Biol. Rev. 90, 560–586. doi: 10.1111/brv.12122
Martínez, A. E., Gomez, J. P., Ponciano, J. M., and Robinson, S. K. (2016). Functional traits, flocking propensity, and perceived predation risk in an Amazonian understory bird community. Am. Nat. 187, 607–619. doi: 10.1086/685894
Martínez, A. E., Pollock, H. S., Kelley, J. P., and Tarwater, C. E. (2018). Social information cascades influence the formation of mixed-species foraging aggregations of ant-following birds in the Neotropics. Anim. Behav. 135, 25–35. doi: 10.1016/j.anbehav.2017.10.024
Mennill, D. J., Ratcliffe, L. M., and Boag, P. T. (2002). Female eavesdropping on male song contests in songbirds. Science 296, 873–873. doi: 10.1126/science.296.5569.873
Mirza, R. S., and Chivers, D. P. (2003). Response of juvenile rainbow trout to varying concentrations of chemical alarm cue: response thresholds and survival during encounters with predators. Can. J. Zool. 81, 88–95. doi: 10.1139/Z02-216
Nocera, J. J., Forbes, G. J., and Giraldeau, L. A. (2006). Inadvertent social information in breeding site selection of natal dispersing birds. Proc. R. Soc. B 273, 349–355. doi: 10.1098/rspb.2005.3318
Oliveira, R. F., McGregor, P. K., and Latruffe, C. (1998). Know thine enemy: fighting fish gather information from observing conspecific interactions. Proc. R. Soc. B 265, 1045–1049. doi: 10.1098/rspb.1998.0397
Opsahl, T. (2009). Structure and Evolution of Weighted Networks. PhD thesis. London, UK: University of London, 104–122.
Owen, C., Rendell, L., Constantine, R., Noad, M. J., Allen, J., Andrews, O., et al. (2019). Migratory convergence facilitates cultural transmission of humpback whale song. R. Soc. Open Sci. 6:190337. doi: 10.1098/rsos.190337
Parejo, D., and Avilés, J. M. (2016). Social information use by competitors: Resolving the enigma of species coexistence in animals? Ecosphere 7:e01295. doi: 10.1002/ecs2.1295
Parejo, D., White, J., and Danchin, E. (2007). Settlement decisions in blue tits: difference in the use of social information according to age and individual success. Sci. Nat. 94, 749–757. doi: 10.1007/s00114-007-0253-z
Pouca, C. V., Heinrich, D., Huveneers, C., and Brown, C. (2020). Social learning in s olitary juvenile sharks. Anim. Behav. 159, 21–27. doi: 10.1016/j.anbehav.2019.10.017
Rieucau, G., and Giraldeau, L.-A. (2011). Exploring the costs and benefits of social information use: an appraisal of current experimental evidence. Philos. Trans. R. Soc. B 366, 949–957. doi: 10.1098/rstb.2010.0325
Rosenthal, S. B., Twomey, C. R., Hartnett, A. T., Wu, H. S., and Couzin, I. D. (2015). Revealing the hidden networks of interaction in mobile animal groups allows prediction of complex behavioral contagion. Proc. Natl. Acad. Sci. U S A. 112, 4690–4695. doi: 10.1073/pnas.1420068112
Schradin, C., Drouard, F., Lemonnier, G., Askew, R., Olivier, C. A., and Pillay, N. (2020). Geographic intra-specific variation in social organization is driven by population density. Behav. Ecol. Sociobiol. 74, 1–9. doi: 10.1007/s00265-020-02896-z
Seppänen, J. T., Forsman, J. T., Mönkkönen, M., and Thomson, R. L. (2007). Social information use is a process across time, space, and ecology, reaching heterospecifics. Ecology 88, 1622–1633. doi: 10.1890/06-1757.1
Shizuka, D., and Johnson, A. E. (2020). How demographic processes shape animal social networks. Behav. Ecol. 31, 1–11. doi: 10.1093/beheco/arz083
Sigaud, M., Merkle, J. A., Cherry, S. G., Fryxell, J. M., Berdahl, A., and Fortin, D. (2017). Collective decision-making promotes fitness loss in a fusion−fission society. Ecol. Lett. 20, 33–40. doi: 10.1111/ele.12698
Sridhar, H., and Guttal, V. (2018). Friendship across species borders: factors that facilitate and constrain heterospecific sociality. Phil. Trans. R. Soc. B 373:20170014. doi: 10.1098/rstb.2017.0014
Templeton, J. J., and Giraldeau, L. A. (1995). Patch assessment in foraging flocks of European starlings: evidence for the use of public information. Behav. Ecol. 6, 65–72. doi: 10.1093/beheco/6.1.65
Tóth, Z., Tuliozi, B., Baldan, D., Hoi, H., and Griggio, M. (2017). The effect of social connections on the discovery of multiple hidden food patches in a bird species. Sci. Rep. 7:816. doi: 10.1038/s41598-017-00929-8
Trefry, S. A., and Hik, D. S. (2009). Eavesdropping on the neighbourhood: collared pika (Ochotona collaris) responses to playback calls of conspecifics and heterospecifics. Ethology 115, 928–938. doi: 10.1111/j.1439-0310.2009.01675.x
Ward, A., and Webster, M. (2016). Sociality: The Behaviour of Group-Living Animals. New York: Springer International Publishing.
Webster, D. R., and Weissburg, M. J. (2009). The hydrodynamics of chemical cues among aquatic organisms. Annu. Rev. Fluid Mech. 41, 73–90. doi: 10.1146/annurev.fluid.010908.165240
Webster, M. M., and Laland, K. N. (2017). Social information use and social learning in non-grouping fishes. Behav. Ecol. 28, 1547–1552. doi: 10.1093/beheco/arx121
Webster, M. M., Atton, N., Hoppitt, W. J., and Laland, K. N. (2013). Environmental complexity influences association network structure and network-based diffusion of foraging information in fish shoals. Am. Nat. 181, 235–244. doi: 10.1086/668825
White, D. J. (2004). Influences of social learning on mate-choice decisions. Anim. Learn. Behav. 32, 105–113. doi: 10.3758/BF03196011
Whiting, M. J., and Greeff, J. M. (1999). Use of heterospecific cues by the lizard Platysaurus broadleyi for food location. Behav. Ecol. Sociobiol. 45, 420–423. doi: 10.1007/s002650050579
Wilkinson, A., Kuenstner, K., Mueller, J., and Huber, L. (2010). Social learning in a non-social reptile (Geochelone carbonaria). Biol. Lett. 6, 614–616. doi: 10.1098/rsbl.2010.0092
Witte, K., and Noltemeier, B. (2002). The role of information in mate-choice copying in female sailfin mollies (Poecilia latipinna). Behav. Ecol. Sociobiol. 52, 194–202. doi: 10.1007/s00265-002-0503-1
Keywords: social information use, behavioral adjustment, demonstration network, network-based diffusion analysis, grouping behavior
Citation: Tóth Z, Jaloveczki B and Tarján G (2020) Diffusion of Social Information in Non-grouping Animals. Front. Ecol. Evol. 8:586058. doi: 10.3389/fevo.2020.586058
Received: 23 July 2020; Accepted: 30 November 2020;
Published: 21 December 2020.
Edited by:
Eric L. Walters, Old Dominion University, United StatesReviewed by:
Teri Jones, University of Liverpool, United KingdomCopyright © 2020 Tóth, Jaloveczki and Tarján. This is an open-access article distributed under the terms of the Creative Commons Attribution License (CC BY). The use, distribution or reproduction in other forums is permitted, provided the original author(s) and the copyright owner(s) are credited and that the original publication in this journal is cited, in accordance with accepted academic practice. No use, distribution or reproduction is permitted which does not comply with these terms.
*Correspondence: Zoltán Tóth, dG90aC56b2x0YW5AYXRrLmh1
Disclaimer: All claims expressed in this article are solely those of the authors and do not necessarily represent those of their affiliated organizations, or those of the publisher, the editors and the reviewers. Any product that may be evaluated in this article or claim that may be made by its manufacturer is not guaranteed or endorsed by the publisher.
Research integrity at Frontiers
Learn more about the work of our research integrity team to safeguard the quality of each article we publish.