- 1Centre for Environmental Genomics Applications, eDNAtec Inc., St. John's, NL, Canada
- 2Department of Integrative Biology, Centre for Biodiversity Genomics, University of Guelph, Guelph, ON, Canada
Global biodiversity loss is unprecedented, and threats to existing biodiversity are growing. Given pervasive global change, a major challenge facing resource managers is a lack of scalable tools to rapidly and consistently measure Earth's biodiversity. Environmental genomic tools provide some hope in the face of this crisis, and DNA metabarcoding, in particular, is a powerful approach for biodiversity assessment at large spatial scales. However, metabarcoding studies are variable in their taxonomic, temporal, or spatial scope, investigating individual species, specific taxonomic groups, or targeted communities at local or regional scales. With the advent of modern, ultra-high throughput sequencing platforms, conducting deep sequencing metabarcoding surveys with multiple DNA markers will enhance the breadth of biodiversity coverage, enabling comprehensive, rapid bioassessment of all the organisms in a sample. Here, we report on a systematic literature review of 1,563 articles published about DNA metabarcoding and summarize how this approach is rapidly revolutionizing global bioassessment efforts. Specifically, we quantify the stakeholders using DNA metabarcoding, the dominant applications of this technology, and the taxonomic groups assessed in these studies. We show that while DNA metabarcoding has reached global coverage, few studies deliver on its promise of near-comprehensive biodiversity assessment. We then outline how DNA metabarcoding can help us move toward real-time, global bioassessment, illustrating how different stakeholders could benefit from DNA metabarcoding. Next, we address barriers to widespread adoption of DNA metabarcoding, highlighting the need for standardized sampling protocols, experts and computational resources to handle the deluge of genomic data, and standardized, open-source bioinformatic pipelines. Finally, we explore how technological and scientific advances will realize the promise of total biodiversity assessment in a sample—from microbes to mammals—and unlock the rich information genomics exposes, opening new possibilities for merging whole-system DNA metabarcoding with (1) abundance and biomass quantification, (2) advanced modeling, such as species occupancy models, to improve species detection, (3) population genetics, (4) phylogenetics, and (5) food web and functional gene analysis. While many challenges need to be addressed to facilitate widespread adoption of environmental genomic approaches, concurrent scientific and technological advances will usher in methods to supplement existing bioassessment tools reliant on morphological and abiotic data. This expanded toolbox will help ensure that the best tool is used for the job and enable exciting integrative techniques that capitalize on multiple tools. Collectively, these new approaches will aid in addressing the global biodiversity crisis we now face.
Environmental Genomics Integration as a Natural Progression for Biodiversity Assessments
Current rates of biodiversity loss are at an all-time high and we are observing rapid global change (IPBES, 2019), making it essential to monitor biodiversity and ecosystems as they respond to these changes. Traditional methods of biodiversity assessment relying on direct observations of taxa are widely used (Friberg et al., 2011), and while they generate important data, they face many challenges. One of these problems is that obtaining biodiversity data is very slow; one study, for example, demonstrated that a typical environmental impact assessment takes 3.4 years to complete (range: 51–6,708 days) (deWitt and deWitt, 2008). Additionally, the cost of traditional monitoring is often very high, especially for whole ecosystems and, consequently, often focuses on target groups or indicator taxa (Pearson and Hawksworth, 1994; Souza et al., 2016; Kissling et al., 2018). Another issue with traditional biomonitoring approaches is that the methods are variable and inconsistent (Birk et al., 2012), in part because they target different taxonomic groups at different levels of taxonomic organization (Friberg et al., 2011). Failure to standardize methods represents a missed opportunity to generate regionally and globally comparable datasets, leading to gaps in large-scale datasets and the over-generalization of local findings to larger scales (Araújo and Guisan, 2006; Wilkes et al., 2017). Because of these difficulties, increasing the efficiency and scale with which bioassessment is conducted has not substantially improved since its advent, and some efforts to improve biomonitoring have complicated programs more than simplified them (Hering et al., 2003). Given these challenges, it is not surprising that little progress has been made to transform biomonitoring into a predictive science in a way analogous to other scientific fields (Purvis et al., 2018). Environmental managers are often left playing catch-up, treating the symptoms of environmental problems instead of addressing the sources, especially where old methods are being used to monitor novel stressors (Woodward, 2009), and these problems will only be exacerbated as climate change and other global disturbances begin to alter ecological baselines, undermining the control-reference systems that are at the core of many contemporary bioassessment programs (Moss et al., 2009; Woodward, 2009).
Despite the difficulties inherent in scaling any type of monitoring up to the global level, real-time data acquisition tools exist for assessing other types of information at national or global scales, including weather (National Center for Atmospheric Research, National Weather Service), hydrology (USGS), seismic activity (USGS), land and water imagery (NASA), and atmospheric constituents for climate change modeling (NOAA Earth System Research Laboratory). These tools have allowed for great strides in modeling and predictive science with direct application to human livelihood (Alley et al., 2019). There is no equivalent unified effort for collection and assessment of global or continental biodiversity data in real or near-real time, despite promising regional, national, and international efforts (e.g., U.S. National Ecological Observatory Network; E.U. Water Framework Directive; Group on Earth Observations Biodiversity Observation Network; but see the Global Biodiversity Information Facility and the International Barcode of Life BIOSCAN project as examples of long-term efforts to amass global biodiversity information). To understand and mitigate biodiversity loss, we must be able to measure it at the temporal and spatial scales that it is changing, which is even more important given rapid global change.
Here, we argue that the adoption of environmental genomic tools to facilitate rapid, large-scale biomonitoring efforts represents a natural progression in bioassessment and ecosystem surveillance. DNA metabarcoding can easily be included in routine biomonitoring programs to provide biodiversity data across ecosystems. As sequencing technologies progress and commercial labs become more prevalent, this will enable new possibilities for creating networks of practitioners and labs that will be able to consistently produce environmental genomic data at regional, national, and global scales. The added value that the adoption of DNA metabarcoding will provide includes (1) streamlined field collection efforts and rapid generation of results, (2) opportunities for developing global, near real-time biodiversity models, (3) spatially and temporally large datasets of consistently observed, whole-system biodiversity data for testing large-scale metacommunity hypotheses, and (4) opportunities for communities to collect biological samples to enhance engagement with environmental stewardship. Additionally, deep sequencing technologies will enable the coupling of DNA metabarcoding with other analytical possibilities, including phylogenetic, functional genomic, and food web analyses. These advances will set the stage for a new era of global, whole-ecosystem bioassessment.
DNA Metabarcoding as a Tool for Rapid, Whole-Ecosystem, Global Bioassessment
DNA metabarcoding provides a rapid means of biodiversity assessment by identifying multiple taxa simultaneously in a sample using DNA sequencing. These may include samples of bulk tissue from whole organisms or environmental samples of water or soil with residual or bound DNA. Environmental DNA, or “eDNA,” is simply any DNA that is found in the environment, which is present in both living cells and dead cells from sloughed-off skin, mucous, or feces, as well as fragments of DNA that are either suspended or bound to organic particles (Creer et al., 2016). Environmental DNA includes both intracellular DNA (Zhang et al., 2013), which is the component of eDNA found within the cells and organelles of living or recently dead organisms, and extracellular DNA, which is the component of eDNA found outside of cells, including both adsorbed DNA and free DNA. Both intracellular DNA and extracellular DNA can be captured and analyzed from an environmental sample. In practice, however, different types of eDNA are not separated for downstream genomic analysis. Consumed DNA consists of DNA recovered from the digestive tract of an organism found under various stages of digestion. Further, bulk sample DNA is often used to distinguish eDNA from DNA recovered from mixtures of organisms that have been separated from environmental samples (Deiner et al., 2017). Environmental DNA is commonly analyzed using one of two molecular methods: metabarcoding or qPCR. The qPCR approach targets small sets of species of interest and does not rely on DNA sequencing (Rees et al., 2014) while the metabarcoding approach takes advantage of high throughput, massively parallel DNA sequencing instruments to identify organisms across various taxonomic groups simultaneously (Creer et al., 2016). In this paper, we focus on metabarcoding methods that can be applied to eDNA and bulk samples.
Metabarcoding uses a marker-based approach to biodiversity assessment, where short strands of DNA that initiate DNA synthesis of specific regions of the genome are used to amplify fragments of DNA from a range of taxa in a sample. Additionally, environmental RNA metabarcoding, which targets complementary DNA through reverse-transcription PCR, can be performed in a similar way (reviewed in Zaiko et al., 2018), providing a measure of the active or viable community (Mengoni et al., 2005); however, this approach needs more development to be widely applicable and is not further considered in this review. DNA metabarcoding assays can be designed to target specific groups of organisms (i.e., using targeted primers) or large swaths of biodiversity across multiple taxonomic groups (i.e., using general primers to target all eukaryotes in a sample). However, in practice PCR amplification can have many sources of bias, including variation in primer binding capacity, especially when used on environmental samples with many DNA templates of different concentrations (Hajibabaei et al., 2012). Metabarcoding assays targeting multiple gene regions can be run for each sample to increase the range of taxa detected from each sample (Gibson et al., 2014). Different metabarcoding assays across samples can be multiplexed into a single sequencing library, and with the advent of sequencers with very high sequencing capacities, the number of samples that can be sequenced simultaneously—while achieving high sequencing depths—is huge (e.g., hundreds to thousands in a single sequencing run). Raw sequence data are processed through several bioinformatic steps (e.g., quality filtering, denoising) and then the recovered sequence reads are assigned taxonomy by comparing them against known sequences in a reference database. The resulting data consist of taxonomic lists of all taxa detected in a given sample. Taxonomy-free approaches are also available to study biodiversity patterns directly from the recovered sequences.
There has been an exponential rise in the use of DNA metabarcoding as a bioassessment tool (Figure 1), with growth driven primarily by the academic and government sectors (Figure 1A) and applications of this technology generally becoming more diverse through time (Figure 1B). Advances in technology and decreases in cost continue to make this methodology more accessible (Slatko et al., 2018). As DNA sequencing technology has advanced, the amount of data generated from environmental samples has increased. Current high capacity sequencing platforms (e.g., Illumina NovaSeq) enable taxonomically comprehensive, rapid bioassessment of virtually all organisms in a sample using a uniform approach. This technology is superior in both sequencing depth and enhanced instrument sensitivity (Singer et al., 2019). To complement the increase in data generated, advancements in computing power and bioinformatic pipelines allow raw sequences to become taxonomic lists at an equally rapid pace (Langmead and Nellore, 2018). Coupled with ultra-deep sequencing and new bioinformatic tools, DNA metabarcoding can generate a taxonomic list of species present in a sample across taxonomic groups in a matter of days.
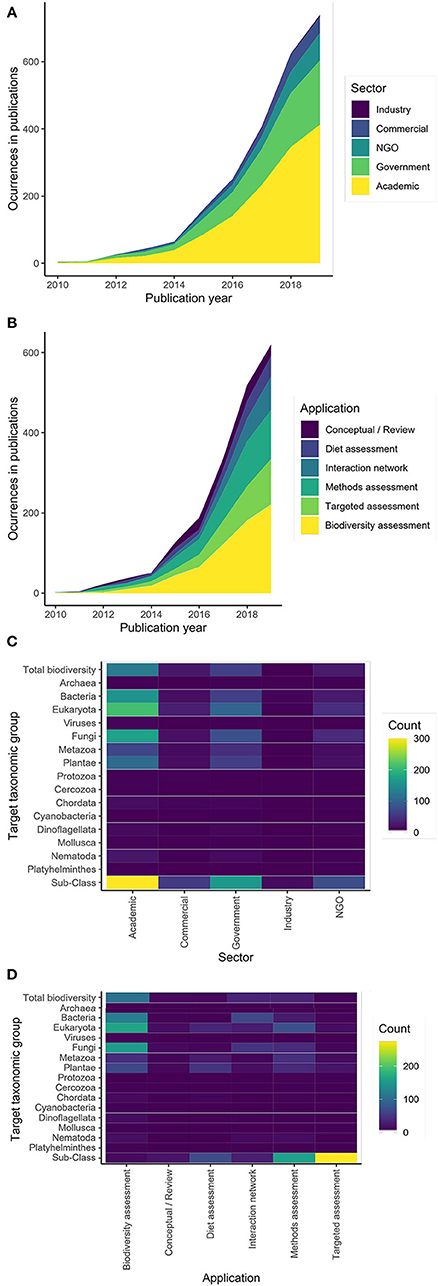
Figure 1. Occurrences of (A) sectors and (B) applications in publications through time. Across all publications, the target taxonomy of each study is broken up by (C) sector and (D) application. Data come from a systematic literature search of 1,563 published articles on DNA metabarcoding (Supplementary Material 1); for methodological details, see Supplementary Materials 2, 3.
Despite near global coverage of sampling efforts across a wide range of taxonomic groups (Figure 2), most of the applications of DNA metabarcoding have been limited in scope (Figures 1C,D) and tend to focus on: (1) targeted species detection (e.g., invasive or endangered species); (2) specific taxonomic groups or target communities (e.g., fish or microbes); (3) local and regional scales; and (4) diet assessment studies linking a target consumer to its prey items, as inferred by sequencing consumer gut contents, blood meals, or feces. While these examples certainly illustrate the breadth of use cases for DNA metabarcoding, they are limited in spatiotemporal scope and the range of biodiversity targeted, throttling the potential information available from eDNA. Indeed, one of the strongest promises of eDNA metabarcoding is that its samples contain DNA from the entire complement of biodiversity in a system, meaning that existing metabarcoding techniques can easily be leveraged for larger scale studies. The inclusion of multiple metabarcoding assays targeting different marker genes can expand the taxonomic coverage of results from samples, greatly expanding the applications of a dataset (Ritter et al., 2019; Silva et al., 2019; Zhang et al., 2020). For example, if a researcher is interested in detecting a target species at risk, an additional general assay can be added to the workflow in addition to the targeted assay, such that the same samples used for targeted assessment could also be used in a more broad biodiversity campaign targeting all of the biodiversity in a system. Additionally, this added information on all the cooccurring biodiversity in a system would provide more context for the targeted assessment, illuminating the biological context in which endangered species persist or non-native species invade an ecosystem. What is more, even if a set of eDNA samples are run for a particular application (i.e., initially run with a targeted primer to detect an invasive species), the DNA extract can easily be archived and re-sequenced using additional assays for future applications, greatly extending the value of these samples.
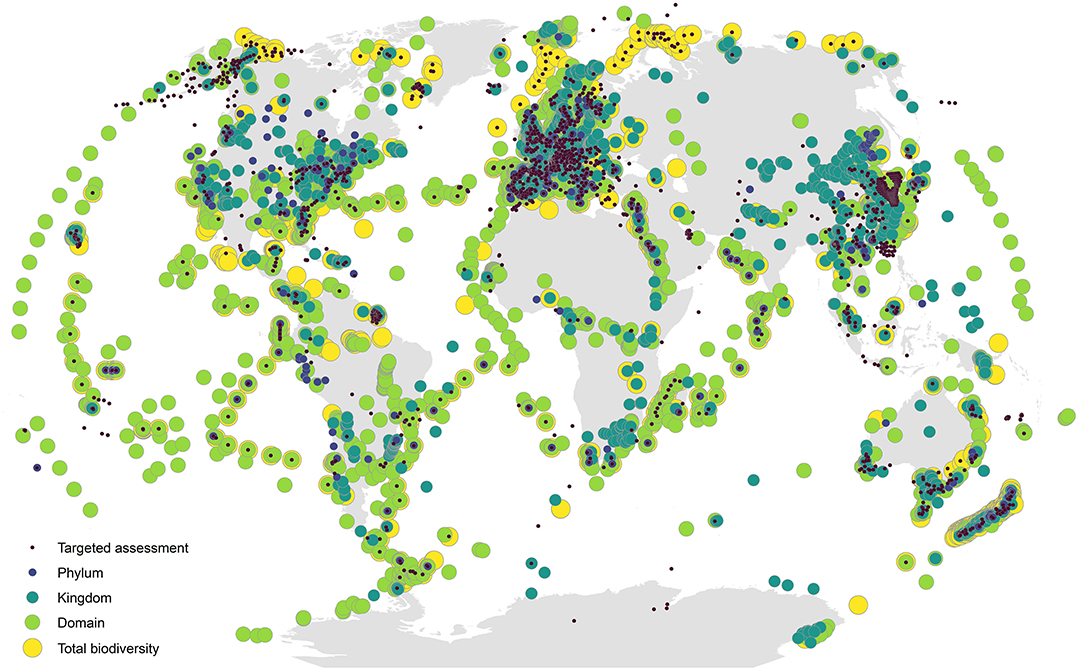
Figure 2. Global map of metabarcoding sampling locations. Points are scaled and colored by the breadth of biodiversity covered, ranging from targeted assessment (class level or below) to total biodiversity assessment (samples using broad-coverage primers covering two or more domains). Data come from a systematic literature search of 1,563 published articles on DNA metabarcoding (Supplementary Material 1); for methodological details, see Supplementary Materials 2, 3.
Beyond the utility of DNA metabarcoding to greatly expand the breadth of taxonomic coverage for total biodiversity assessment, this approach is conducive to expanding sampling efforts to unprecedented spatiotemporal scales (Creer et al., 2016; Bush et al., 2019), allowing for more samples to be collected more quickly, more easily, and in more remote locations. This promise will be further realized as eDNA metabarcoding is met with efforts toward automating sample collection [e.g., using drones and Autonomous Underwater Vehicles (AUVs)] and as ultra-deep sequencing technologies allow for greater read depth, meaning that more biodiversity information can be extracted from the same sample, potentially leading to less sample volume required for adequate biodiversity assessment.
Who Could Benefit From DNA Metabarcoding: Outlining the Stakeholders Across Sectors
While the users of DNA metabarcoding techniques have primarily been academics, other stakeholders from various sectors have also started taking advantage of this technology (Figure 1A). The benefits of the time and cost-savings provided by DNA metabarcoding are applicable across sectors, however the impacts and challenges associated with adopting these techniques are unique to each. Resistance to adoption of DNA metabarcoding by many stakeholders is similar to the methodological conservativism that slows adoption of other technologies, but adoption of guiding virtues—or attitudes toward engagement with environmental genomic technologies—will serve to assuage concerns, facilitate positive inter-agency collaboration, and guide positive interactions between end users and these technologies, hopefully leading wise management decisions (Darling, 2019).
Academic
DNA metabarcoding was pioneered by the academic sector, which has since been using the rich biodiversity information generated through DNA sequences to address a range of ecological questions. The academic sector has benefitted by improvements in the processing time and decreased cost. The promise of rapid assessment of total ecosystem diversity from DNA metabarcoding will equip academics lacking taxonomic expertise with the ability to assess any organism group at any level of taxonomic resolution, greatly expanding the pool of scientists able to address biodiversity questions and allowing for more rapid scientific advancement. Additionally, it will allow for the concurrent examination of many different groups of taxa, from microbes to mammals, which is often not possible due to financial constraints or the narrow taxonomic expertise of many academic researchers. An increasing user base for biodiversity data will facilitate synergies between academics specializing in taxonomy and genomics, as the increased demand for rapid, whole system bioassessment will require these taxonomists to do the hard work of describing species, linking these species to genomic databases, and describing their life histories so that traits information can be obtained.
Challenges to widespread adoption in academic sectors come from accessibility to academic or commercial labs with the capacity for whole-community DNA metabarcoding. Barriers also result from misconceptions relating to the cost and perceived technical difficulty of DNA extraction, amplification, and sequencing methods, as well as bioinformatic processing of data. These limitations will likely be overcome once additional public and commercial facilities emerge (see Synergies below).
Government
Like the academic sector, government agencies will benefit from the rich biodiversity information provided by DNA metabarcoding. However, where academic stakeholders are often more interested in utilizing DNA metabarcoding as a tool for specific case studies, local, and especially federal, governments have greater capacity to employ DNA metabarcoding for widespread application to mandated biomonitoring programs. In this sense, the consistency, reproducibility, and DNA archival aspects (i.e., DNA extract can be stored long-term at −80°C and used again in the future) of DNA metabarcoding are invaluable. For example, DNA metabarcoding has the potential to eliminate the biases related to different sample processing methodologies and the different taxonomic biases associated with morphological identifications (Nerbonne et al., 2008). While DNA metabarcoding on a national or global scale would require a standardized field sampling methodology, collecting and filtering a water sample of known volume or obtaining a bulk sediment sample is much easier to standardize and affords much more consistency than traditional sampling methods, which are often more complicated. This would be a natural progression and a great improvement on existing efforts that remain localized and fragmented.
A key challenge to adoption of genomic approaches, like DNA metabarcoding, by government agencies is overcoming socioeconomic constraints and legal and historical precedents mandating how biomonitoring is performed (Leese et al., 2018). While some promising signs exist on the frontier of federal biomonitoring programs utilizing DNA metabarcoding (e.g., NEON: https://www.neonscience.org/data-collection/dna-sequences; ECCC: https://www.canada.ca/en/environment-climate-change/services/canadian-aquatic-biomonitoring-network/news-events.html#assessing), these efforts have taken time to realize, largely due to the confines of how sampling was traditionally done and a general resistance to change (Ford et al., 2008). Some of this resistance likely comes from the unwillingness to abandon invaluable, long-term morphological datasets by those involved in regional biomonitoring programs. Fortunately, DNA metabarcoding could be conducted in a way to supplement existing programs, with minimal added effort (e.g., using a subsample from a sediment sample; collecting a water sample for eDNA at existing monitoring sites). This issue, however, is complicated by local and national bioassessment mandates, which are often broad legislative guidelines that do not require a specific methodology or technology for these assessments. The prospect of adopting DNA metabarcoding for developing countries, which are not encumbered by historical precedents and legislation related to biomonitoring, is even more immediately plausible, as DNA metabarcoding will facilitate generating biodiversity data for bioassessment program development, and allow for biodiversity data to be connected to other datasets across large spatial scales, a possibility that will become more realized as the archival of DNA extracts and raw sequence data (collected under standardized protocols) become more common and legally mandated.
NGOs/Citizen Scientists
While key benefits of DNA metabarcoding to academic and government sectors relate to standardization and streamlining of biodiversity data acquisition, the benefits to NGOs and citizen scientists are much broader and indirect. The technical skills required to perform the lab and bioinformatic steps of DNA metabarcoding are often too complex for direct adoption by these stakeholders. Nevertheless, NGOs and citizen scientists will certainly become an important component of field sampling for DNA metabarcoding, particularly for terrestrial and freshwater ecosystems. For marine systems, which often require large vessels with a lot of on-board equipment to access and sample, especially at depth, citizen scientists will be unlikely to play key roles in sampling; however, in the long term, the emergence of automated sampling technologies may enable passive sampling from commercial or private ships (see Technological and Scientific Advances section, below; Figure 3). Since DNA metabarcoding can be conducted using water or soil samples and requires less training than traditional sampling, standardized procedures and sampling kits mean these groups would not only have new tools for studying their local ecosystems, but they could become key players in scaling up DNA metabarcoding, both spatially and temporally. National efforts are already emerging in many countries, including New Zealand (the Environmental Protection Authority's Open Waters Aotearoa: https://www.wilderlab.co.nz/explore), the U.S. (CaleDNA Initiative: https://ucedna.com/), and Canada (Sequencing the Rivers for Environmental Assessment and Monitoring, STREAM: https://stream-dna.com/), that leverage community groups to collect DNA metabarcoding samples across large spatial scales. These types of efforts will engage stakeholders by involving them in the processes of metabarcoding-based biomonitoring, giving them exposure to cutting-edge, forward-thinking biomonitoring approaches and providing important exposure to STEM education and outreach through hands-on programs. The indirect benefits of this and similar programs will be even more broad and impactful as these programs mature, and citizen scientists involved or interested in these programs will be able to access online databases of taxonomic lists for the taxa found in their local ecosystems.
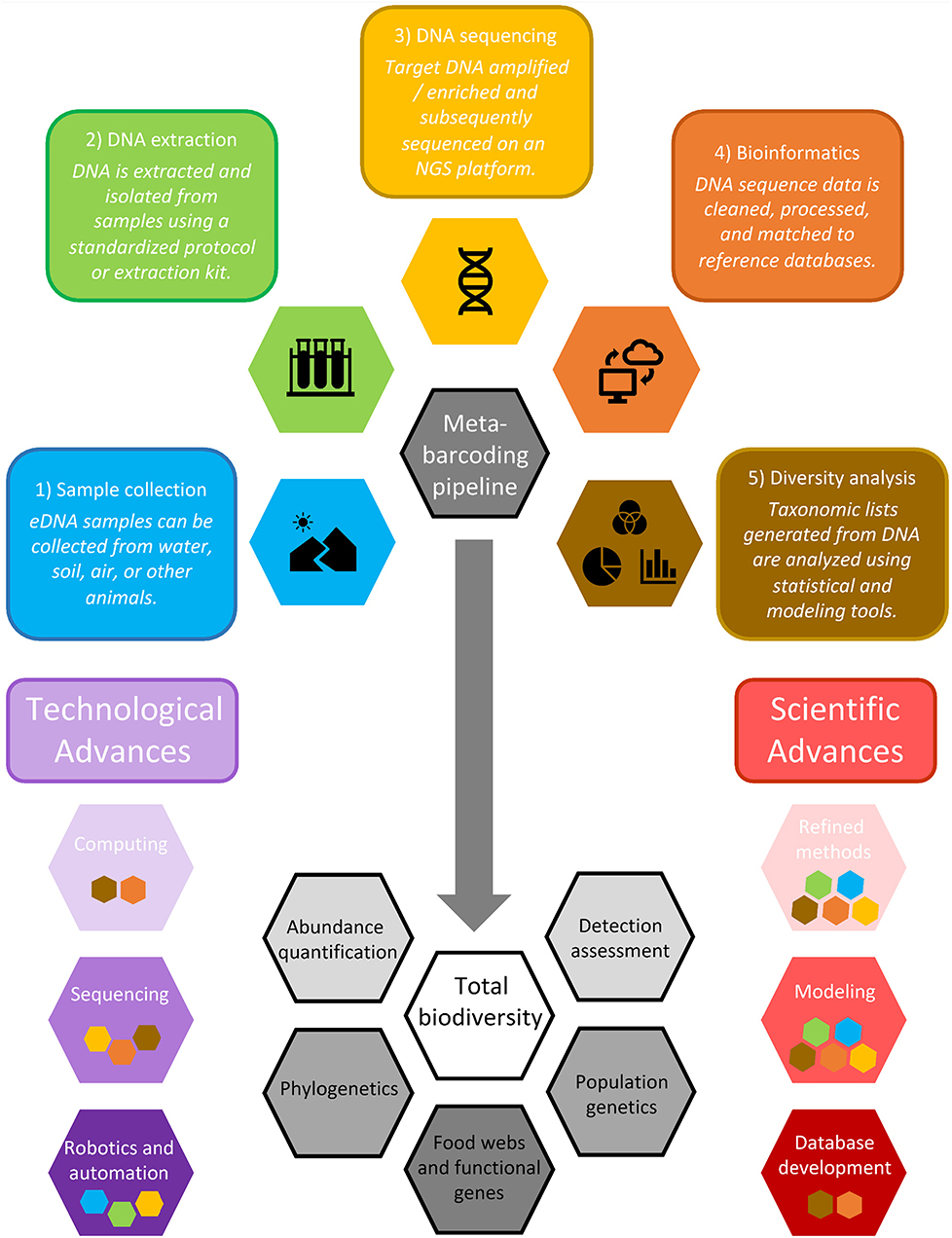
Figure 3. Conceptual model outlining the DNA metabarcoding pipeline, including (1) sample collection, (2) DNA isolation and extraction, (3) high throughput sequencing, (4) bioinformatics, and (5) biodiversity analysis. Purple and red hexagons depict technological and scientific advances that will improve this workflow, with inset colored symbols reflecting the steps of the pipeline that will most benefit from these advances. The gray arrow indicates progress toward total biodiversity assessment (white) and other related advances (gray). Shape shading depicts advances and applications that range from those that are currently receiving a lot of attention and are achievable in the short term (light colors) to those that will require more research and development to achieve in the long-term (dark colors).
Challenges to adoption of DNA metabarcoding by NGOs and citizen scientists will be less logistical, given that these groups will not be responsible for lab processing, bioinformatics, or analysis; rather, these groups will need to be educated about DNA metabarcoding and convinced of the benefits this and other environmental genomic tools will have for their local community. Further, specific education programs will need to be developed to train members of these groups how to sample in a standardized way, using DNA-compatible sterilization techniques, in order to obtain quality data.
Commercial/Consulting
Because DNA metabarcoding involves technical equipment and expertise in the lab (e.g., DNA extraction, library prep, high-throughput sequencing) and computing (e.g., denoising, chimera removal, database matching) steps in the workflow, this and other metagenomic approaches are still beyond the reach of many practitioners who have, until recently, had to partner with academic or government labs to achieve their metabarcoding goals. This limitation has likely been a barrier to more rapid, widespread adoption of DNA metabarcoding for routine biomonitoring, even when there is desire or curiosity to explore these new methods. This gap represents an unoccupied niche that is quickly being filled by commercial genomic labs across the globe. As of November 2019, we identified 26 commercial labs globally—primarily concentrated in North America, Europe, and Australia—that provide some form of eDNA metabarcoding services. These labs are emerging as important partners for members of other sectors, and this was seen in our literature search, which revealed that while members of these companies seldom lead papers, they are becoming increasingly involved in research with other sectors.
Industry
Benefits of DNA metabarcoding to industry will largely overlap with benefits to other sectors, but this sector may stand to benefit the most from the rapid and cost-efficient DNA metabarcoding approach. Legal mandates requiring natural resource companies to perform environmental impact assessments often lead to long, expensive processes that pit governments and NGOs against industry and can lead to lawsuits ensnaring all parties in litigation for years and sometimes decades (Macintosh et al., 2018; Estes, 2019). While not all of these problems will be solved by DNA metabarcoding, this revolutionary technology certainly will provide a much faster, cost-effective way of performing environmental impact assessments, leading to a more efficient process of data acquisition. Further, given the capacity of DNA metabarcoding to unlock the full complement of biodiversity information—from microbes to mammals—this will lead to more holistic decisions based on a more comprehensive assessment of impacts to biodiversity and ecosystem function compared to existing assessment efforts that focus on indicator taxa or target species of interest (e.g., charismatic megafauna). Additionally, with the full complement of biodiversity at the disposal of bioassessment practitioners, the opportunity for novel metric development will arise, potentially leading to more predictive metrics for ecosystem change and stability. Further, deeper sequencing approaches should allow for more complete functional gene assessment, enabling practitioners to connect the dots between ecosystem structure and function. Collectively, this new suite of tools will provide industries with a more complete picture of ecosystem impacts resulting from natural resource development and alteration, equipping industries—ranging from oil and gas to commercial enterprises—with unprecedented tools for rapidly conducting environmental impact assessments and, ultimately, expediting project approvals while assuring that they are done in an ecologically responsible and sustainable manner.
As communities and stakeholders gain a heightened awareness of climate change and other ways humans can alter global processes, environmental disasters arising from corporate neglect or abuse of the environment (e.g., Deep Water Horizon, Union Carbide in India) have been met with public outcry, leading many large corporations to reevaluate their role in these societal issues. This has resulted in a trend where many companies—including some of the largest oil and gas companies—have established environmental stewardship initiatives to ascertain good corporate responsibility through various programs and pro-environment campaigns. Together with the promises of environmental genomic tools, this desire within industry will provide exciting opportunities for partnership and collaboration among other sectors and ultimately help resource development industries become more environmentally conscious entities (Graham, 2017). Additionally, other industries will emerge from DNA metabarcoding and environmental genomics, which we are already seeing, including the personal genomics industry (Contreras and Deshmukh, 2017; Geiger and Gross, 2019).
Challenges to the adoption of DNA metabarcoding by industrial sectors will parallel those that have kept academic, government, NGO, and industrial sectors separated for so long: namely, a failure to see the synergistic capacity of collaboration among these sectors. For the part of industry, there are legitimate concerns relating to proprietary information and the legal ownership of generated products and data, but the benefits to collaboration will likely outweigh any difficulty in working out these agreements. Additionally, regulatory agencies must be willing to accept genomic data for impact assessments and monitoring. Again, this can benefit from collaboration between government, industry, and academia to establish the reliability of metabarcoding as a method of environmental monitoring. Currently, several government agencies are evaluating the applications of eDNA for impact assessment and monitoring (Baillie et al., 2019).
Synergy Among Sectors
While stakeholders from each of these sectors will benefit from adopting DNA metabarcoding for bioassessment purposes, these sectors will need to become much more integrated than they currently are to facilitate widespread adoption of DNA metabarcoding. Currently, most of the research that is being conducted is coming out of the academic sector (Figure 1A), but these estimates are biased by the fact that a lot of research and development that occurs in other sectors (especially industry) is not published in peer-reviewed journals. Despite the popularity of DNA metabarcoding research in academia, this research has been limited in scale and application. For example, most academic DNA metabarcoding studies focus on local scales and detection of a target taxon (e.g., for detection of threatened species or species at risk) or one group of taxa (e.g., fish, frogs, or macroinvertebrates) (Figures 1C,D, 2).
Because most academic labs have their own internal laboratory protocols and bioinformatic methods, results from these studies cannot be broadly compared across labs. This is one key area where collaboration among sectors could yield great advances in adoption and application of DNA metabarcoding. For example, federal programs could be used to create large national networks using established, universal methodologies. Large, federated data portals could be created to house this information, which would provide academic labs with a database and infrastructure to both connect their research campaigns with, and give them access to, high-resolution, long-term datasets collected at national and global scales, facilitating the advancement of macroecology, including concepts proposed—but seldom tested—by metacommunity theory (Leibold and Chase, 2017). These programs will facilitate transferring genomic tools to industry, such that they can more quickly, more cost-effectively achieve environmental compliance. However, these efforts must be synchronized with rapid advances in genomics and bioinformatics. A balance between the evolution of technologies and their adoption will be necessary and can be achieved through focused multi-stakeholder workshops and discussions. Beyond their role in adopting these tools for rapid compliance, industry—with its growing capacity of global infrastructure (Liu and Dunford, 2016)—will be well-positioned to aid in advancement of global-scale biomonitoring efforts (e.g., through shipping networks or commercial fishing systems), and natural collaborations will emerge, particularly between industry and academic or government sectors. The efficiency and utility of metabarcoding can be maximized by collaborations between industries (e.g., oil and gas and fisheries). When multiple industries are active in the same region, they can pool resources to perform comprehensive biodiversity surveys of those regions that will yield data relevant for various applications.
Another aspect of environmental genomics that invites collaborative relationships among sectors is the Convention on Biological Diversity's (CBD) mandate of asset and benefit sharing (ABS) of genomic resources derived from national and international biomonitoring efforts. The CBD mandates equitable access to genetic resources and their benefits [Article 5, Nagoya Protocol: (Secretariat of the Convention on Biological Diversity, 2011)], with direct implications for researchers and practitioners using environmental genomic tools, such as DNA metabarcoding, that characterize biodiversity (Davis and Borisenko, 2017). Specifically, Article 5 of the Nagoya Protocol states that all parties should take legislative, administrative, or policy measures to ensure that “benefits arising from the utilization of genetic resources that are held by indigenous and local communities, in accordance with domestic legislation regarding the established rights of these indigenous and local communities over these genetic resources, are shared in a fair and equitable way with the communities concerned, based on mutually agreed terms.” The intent of this mandate is to provide safeguards to governing bodies that house biodiversity resources, such that access to these genetic resources comes with an exchange of shared benefits derived from their use. Developing countries often house these resources, and so partnerships within these regions can raise awareness of environmental genomic tools among the public, including policy makers, applied practitioners, and government regulatory bodies (Davis and Borisenko, 2017). One of the advantages of eDNA metabarcoding is that it does not use animal or plant tissues for identification and does not require DNA derived directly from tissue; rather, eDNA is from environmental samples and only small gene fragments, barcodes that are approved by the CBD for DNA identification, are sequenced. Because this process uses barcodes that cannot produce functional transcripts, the legislative ABS mandates are less restrictive than for whole genome resources, which means that eDNA metabarcoding is a strong candidate for global biomonitoring programs that cross international jurisdictions and involve diverse stakeholder groups. Moreover, rather than fearing environmental genomics as a means of exploitation of genetic resources, biodiversity-rich countries should embrace the technology as a means of providing an inventory of their resources so that they can more easily be tracked and protected (Vernooy et al., 2010).
Building the necessary partnerships among different stakeholders and sectors will be key to scaling up DNA metabarcoding and next-generation bioassessment, but these types of synergistic efforts have traditionally been difficult due to lack of communication among sectors. While governments have created many incentivization programs to foster cross-sector partnerships (e.g., academic-industry, public-private), these programs have not been very effective (Veletanlić and Sá, 2019), and adoption of novel technologies and methodologies has traditionally been slow (Hering et al., 2018). Yet, the promise of next-generation sequencing and DNA metabarcoding represents a leap forward in bioassessment capacity and, consequently, has the potential to unite traditionally siloed sectors. There is an emerging ontology for global biomonitoring (e.g., Global Infrastructures for Supporting Biodiversity Research), and concerted efforts are arising to aggregate and archive linked genomic (e.g., Global Biodiversity Information Facility) and trait databases (e.g., Global Biotic Interactions). There have even been efforts to formulate global policy for biomonitoring [e.g., Global Earth Observation System of Systems, the EU COST Action DNAqua-Net, European Territorial Cooperation (Interreg)] (Hering et al., 2018; Lefrançois et al., 2018).
Overcoming Limitations to Widespread Adoption of Next-Generation Bioassessment: A Path Forward
Even as interest in DNA metabarcoding has risen (Figure 1A), there has been resistance toward its adoption, a phenomenon seen with other revolutionary technologies (Ram and Sheth, 1989; Claudy et al., 2015; König and Neumayr, 2017). Some of the concerns about DNA metabarcoding are warranted, while others are baseless and motivated by patch protection and fear of change—including the notion that DNA metabarcoding will threaten the livelihood of, for example, existing biomonitoring programs or the taxonomists upon which they rely. The view that DNA metabarcoding will be in direct opposition to traditional biomonitoring programs and bioassessment approaches, however, is a false dichotomy: these two approaches provide unique and complementary information. While DNA metabarcoding affords greatly enhanced taxonomic resolution (Gibson et al., 2015), rapid results, non-invasive sampling methods, and the ability to identify hidden trophic linkages (Paula et al., 2016; Pornon et al., 2017; Casey et al., 2019), traditional taxonomy is more suitable for measures that rely on individual specimens, such as abundance, size, sex, or age (Lamb et al., 2019; Piñol et al., 2019). These measures are currently used in many monitoring programs and quantification approaches, though recent studies have suggested that presence-absence data obtained from DNA metabarcoding can provide similar trends in ecological assessment compared to measures that incorporate abundance (Aylagas et al., 2016; Sard et al., 2019). Similar to how multiple sampling methods are used in traditional surveys to obtain comprehensive data (e.g., gill netting, fyke netting, and electrofishing for fish surveys), metabarcoding can represent another approach to enhance the results of bioassessments. If anything, we foresee the rise of DNA metabarcoding generating added interest in partnerships with morphological taxonomists (e.g., to expand sequence libraries like BOLD and GenBank) and practitioners conducting existing biomonitoring programs (e.g., to incorporate DNA metabarcoding into existing sampling efforts in order to expedite adoption and expansion of scale). Here, we outline some of the key barriers to widespread adoption of DNA metabarcoding and illuminate how these barriers could be overcome in the short and long term (Figure 3).
Developing Standardized Field Sampling and Lab Methods
Field sampling often represents the highest cost in any biomonitoring effort, and this remains the case in biomonitoring with DNA metabarcoding, where sample collection is typically the most expensive part of the sample processing pipeline for fish, invertebrates, and algae (Stein et al., 2014). Consequently, optimizing field sampling methods remains a major challenge in ecology, especially in difficult to sample regions (Hajibabaei et al., 2016). While considerable effort has been undertaken to compare DNA metabarcoding with traditional morphological methods of bioassessment (Stein et al., 2014), it is important to remember that these two approaches are very different, and so practitioners should not necessarily be trying to sample for DNA in the same way as they traditionally have for morphological samples. DNA can be collected to obtain biodiversity information from a multitude of environmental samples, including water (Ficetola et al., 2008), soil (Fahner et al., 2016), and homogenized bulk tissue (Gibson et al., 2015), and sample volumes can be adjusted based on the total DNA content in a sample. Further, because eDNA does not require whole organisms for identification, samples can also be collected from partially digested gut contents, feces, blood meal (Sousa et al., 2019), and even from sample preservatives (Zizka et al., 2019). Beyond the wide range of samples from which eDNA can be collected that differentiate it from traditional approaches, DNA metabarcoding provides a huge volume of data, which means greater statistical power even with fewer samples, likely making it a more efficient approach than conventional sampling methods. These differences from traditional morphology require novel sampling methods for eDNA that consider (a) how to most efficiently capture eDNA, (b) where variability occurs during the sampling process and how best to replicate sampling for optimal statistical and modeling power, and (c) how to standardize pre-processing steps (e.g., filtering, preservation, fixation) such that they can be reliably used in the field.
In addition to designing sampling approaches for maximizing eDNA capture, considerations for sampling eDNA in different types of ecosystems also need to be made. Developing sampling approaches that allow for consistent observation across different environments and ecosystems is necessary to maximize the power of DNA metabarcoding data, especially since different sampling approaches can yield very different results. For example, recent efforts have examined how well eDNA can be recovered from different sample types (Macher et al., 2018; Erdozain et al., 2019; Hajibabaei et al., 2019a; Koziol et al., 2019). Currently, the most common approach for eDNA metabarcoding is sampling filtered water, which is frequently conducted in both freshwater and marine systems [e.g., BC Ministry of Environment: (Hobbs et al., 2017); USGS: (Laramie et al., 2015)]. While other systems (e.g., forests, frozen tundra) might not be as amenable to water sampling, collecting other eDNA sample types nevertheless involves only a few additional steps. For example, bulk samples of freshwater benthos (Gibson et al., 2015; Bush et al., 2020) and soil or sediments (Fahner et al., 2016; Porter et al., 2016) have been used in numerous publications and have been integrated in large-scale DNA metabarcoding programs (e.g., Canadian Aquatic Biomonitoring Network Field Manual: Wadeable Streams, 2012). The simplicity of eDNA sampling likely provides a path forward for standardized sampling approaches across ecosystem boundaries, and novel approaches for in situ eDNA sampling are being considered (Dickie et al., 2018; Ruppert et al., 2019).
One common challenge to practitioners interested in using eDNA metabarcoding as a bioassessment tool is how to implement sterilization and DNA-compatible techniques to avoid cross-contamination during sample collection in the field. This can be particularly intimidating for practitioners who have limited lab experience or who have never worked with DNA; however, this should not be a barrier to adoption by practitioners or citizen scientists. There are several programs, for example, that provide formalized training for DNA metabarcoding field sampling (e.g., CABIN, NEON). For eDNA water sampling in particular, field sampling using DNA-compatible techniques is relatively easy. In cases where filtering is not required in the field, sample collection can be expedited with short-term storage on ice or dry ice, and immediate transfer to the lab, where samples are stored at −80°C and then sent to a lab specializing in DNA metabarcoding. With the rise of many regional commercial genomic labs, practitioners will have increasing accessibility to these environmental genomic resources, underscoring the importance of these procedures and programs. Another exciting prospect that could overcome the challenge of sterile field sampling, at least in aquatic environments, is autonomous samplers for eDNA, which can be deployed in large water bodies to collect and preserve many samples across large spatial and temporal scales (Yamahara et al., 2019).
Compared to field methods, standardizing lab protocols represents a larger challenge, as there are a lot more steps with variable methods in the lab. Despite the many field factors that influence DNA metabarcoding results [e.g., water volume (Mächler et al., 2016), filter size (Deiner et al., 2018), and preservation method (Robinson et al., 2020)], the laboratory side of eDNA metabarcoding has more variables to control for (e.g., DNA extraction kits, primer choice, the number of PCR replicates performed, sequencing depth, etc.). These laboratory methods tend to be well-documented, as they often use commercially available standardized DNA extraction kits and existing, published primer sets. Indeed, work is already underway to determine how these factors influence the DNA metabarcoding pipeline (Deiner et al., 2018; Majaneva et al., 2018; Piñol et al., 2019). What is more, because DNA extract can be frozen and archived, these samples could be retroactively processed for new primers even after samples are run, enabling comparison to other datasets that used the same methodology.
Preservation and Storage of eDNA Samples
Sample preservation and storage of eDNA samples has been relatively standardized compared to other parts of the sampling pipeline, and these methods seem to follow the conventions established for DNA sampling by microbial ecologists despite the fact that eDNA targets a wider group of organisms (i.e., not just microbes). Yet variation in this step of the sampling pipeline exists, and it is important to remember that no amount of back-end bioinformatics can recover a sample that has been poorly collected, stored, or assayed (Murray et al., 2015). Bulk samples are typically preserved with 95% DNA-grade ethanol, and then transferred on dry ice to the lab where they are stored at −80°C (Jarman et al., 2018). While it is certainly wise to use the most conservative approach, this level of stringency might not be necessary for DNA metabarcoding of macroorganisms, as even samples stored at room temperature have been shown to yield adequate quantities of eDNA (Renshaw et al., 2015). Currently, a major research focus is to ascertain the effectiveness of preservation methods for eDNA recovery (Williams et al., 2016; Marquina et al., 2019; Robinson et al., 2020). While we underscore the importance of consistency in sample preservation for project development and recommend using the most conservative and common approach, as it will potentially facilitate inclusion of more citizen scientists and enable more cross-study comparisons, there may be instances or applications where this is not possible (e.g., recovering DNA from museum samples, sampling in remote locations, samples susceptible to degradation from a high microbial load), and these limitations should not preclude researchers from exploring the use of DNA metabarcoding for their particular application. Indeed, while we aspire toward consistency and standardization in preserving and processing eDNA samples, we must be cautious to generalize one sampling approach as superior to others, given the many different types of eDNA samples, preservation techniques, and confounding variables.
Consistent, Open-Source Bioinformatic Pipelines
As the advancement of genomic tools and other technologies facilitate rapid data generation, we will be confronted with the enormous task of dealing with this deluge of information (Hey and Trefethen, 2003; Bell et al., 2009). Thus, vital to advancements in next-generation biomonitoring will be concurrent advancements in computing and bioinformatics that will enable us to maximize the use of these rich genomic and biodiversity datasets. Recent sequencing technologies have enabled the generation of billions of short reads in a matter of days, and open-source bioinformatic tools for genomic data processing are maturing [e.g., DADA2: (Callahan et al., 2016); CutAdapt: (Martin, 2011); QIIME2: (Bolyen et al., 2019); Mothur: (Schloss et al., 2009)]; however, progress needs to be made in terms of data accessibility, data discoverability, and standards development, as advancement in these areas will likely emerge from the recent advent of consortia related to standards for genomics and associated data (Levy and Myers, 2016). Specifically, we need to develop quality control tools to test for and correct errors that inherently emerge from these bioinformatic approaches (Olson et al., 2019), as the scientific community currently has no systematic approach for developing, peer-reviewing, and publishing open-source tools that go into existing bioinformatic pipelines (Mangul et al., 2019). Archival and versioning of these tools and pipelines is also inconsistent, reducing their utility and widespread adoption. Collectively, these issues present great challenges in terms of data comparison between studies using different bioinformatic pipelines, different versions of a pipeline, or different pipeline parameters (e.g., filtering and mismatch thresholds), which themselves are often not documented in published literature or publicly available. Fortunately, practical models for improving the discoverability, usability, and archival of these tools and pipelines already exist for digital data archival, and tools for sharing, archiving, and simplifying this process already exist through open-source archival services (e.g., GitHub), package managers [e.g., Bioconda: (Grüning et al., 2018; Mangul et al., 2019)], or containers [e.g., Docker: (Merkel, 2014)].
Reference Databases
A key barrier to adoption of next generation bioassessment tools is incomplete reference databases. Databases like GenBank (Sayers et al., 2019), a general sequence data repository, and Barcode of Life Data Systems (BOLD) (Ratnasingham and Hebert, 2007), a taxonomically oriented sequence data repository, are some of the world's most widely used sequence databases for DNA metabarcoding analysis, yet their coverage is both incomplete and uneven, with extreme variation based on taxonomic group and geographic region (Porter and Hajibabaei, 2018b), as well as gene coverage [e.g., cytochrome c oxidase I (COI), 16S, 18S] (Meiklejohn et al., 2019). For example, taxa that have traditionally been used for bioassessment are generally well-represented in these databases, with COI sequence records for 61% of North American freshwater invertebrates (based on taxa in public databases) represented; specific taxonomic groups within invertebrates, however, range widely, with COI sequence records for 74% of mollusks but only 15% of Nematodes (Curry et al., 2018). In contrast, database coverage for marine species is less complete, with only 30% of operational taxonomic units having species-level matches at a 97% identity threshold (López-Escardó et al., 2018) and only half of the reads identifiable to even the phylum level (Bakker et al., 2019). Again, taxonomic coverage varies widely, with much better coverage for fish and marine mammals. The problem of database incompleteness is compounded by the fact that methods for assigning higher taxonomic levels to unknowns have traditionally been inaccurate (Koski and Golding, 2001), so better methods [e.g., Bayesian classifiers: (Porter and Hajibabaei, 2018a)] need to be developed that can assign some level of identification to unknowns when an exact match in the reference database is not available (Berger et al., 2011). Still, DNA metabarcoding represents a full step forward for rapid, accurate species detection and identification, and is especially useful in diverse systems including species with challenging taxonomies. Further, many ecological analyses (e.g., diversity analyses, ordinations) do not require taxonomic identities, and in these cases use of unique sequences, such as operational taxonomic units (OTUs) or amplicon sequence variants (ASVs), can be powerful tools for resolving environmental differences (Gibson et al., 2015; Cahill et al., 2018).
Dynamics of eDNA in the Environment
While the collection, extraction, and amplification of eDNA from the environment is relatively well-studied, the dynamics of eDNA in the environment are less well-known. This includes the abundance, persistence, and movement of eDNA within the environment, all of which are impacted by a variety of abiotic (e.g., temperature, sunlight, oxygen) (Pilliod et al., 2014; Eichmiller et al., 2016) and biotic factors (e.g., species richness, relative species biomass, individual behavior) (Freeland, 2017; Stoeckle et al., 2017; Hansen et al., 2018). This may be especially relevant in large, highly dynamic systems, such as the marine environment. While there is some evidence that DNA persistence varies across different environments (Barnes et al., 2014), most of the information on DNA shedding and decay rates (Sansom and Sassoubre, 2017) and movement of DNA (Shogren et al., 2017; Wood et al., 2020) comes from studies of freshwater systems, often using experimental mesocosms with a small number of species. As more research is done to reveal these dynamics, conclusions from metabarcoding studies will become more robust.
Technological and Scientific Advances Will Usher in a New ERA of Whole Ecosystem Assessment
We foresee synergistic advancements arising from extracting and utilizing additional layers of information coded in DNA that will emerge as sequencing technologies advance, particularly as greater sequencing depth and genome coverage are achieved. Concurrent with efforts to overcome the limitations to widespread adoption of DNA metabarcoding and other environmental genomic tools, there are several promising technological and scientific advances that will facilitate a new era of near real-time, whole ecosystem assessment. These advances include those that are relatively easy and achievable in the short-term (e.g., advances in computing, methods refinement and standardization), those that will require more research and investment to develop (e.g., improved sequencing technologies, more sophisticated physical and ecological model development), and those that will require long-term, global, collaborative efforts that will revolutionize environmental genomics (e.g., autonomous robotics, genomic database development; Figure 3). Collectively, these technological and scientific advances will unlock novel possibilities for data exploration by merging DNA metabarcoding results with other genomic and ecological information, facilitating the exploration of (1) abundance quantification, (2) advanced physical and ecological modeling of eDNA and associated taxa, (3) exploration of phylogenetic patterns; (4) intraspecific genetic assessment of whole communities, enabling population genetics applications across a range of species; and (5) ecological network, food web, and functional gene analysis (Figure 3).
Abundance and Biomass Quantification
A primary criticism lodged against using genomic approaches for bioassessment is that the relative read abundances of sequenced DNA do not often correlate strongly with the abundance or biomass of species in the environment. Further, these patterns appear to be highly context dependent. For example, a recent meta-analysis of qPCR and ddPCR results showed that eDNA concentration is much more strongly correlated to abundance in controlled laboratory studies (82% of variation explained) compared to field experiments (57% of variation explained) (Yates et al., 2019). Another meta-analysis of single-species qPCR assays showed similar patterns, where there was a weak and highly variable correlation between biomass and eDNA sequences (global model: slope = −0.52 ± 0.34) (Lamb et al., 2019). Yet another recent study found excellent correlation (R2 = 0.79) between eDNA copy numbers and biomass from trawl catches in commercially important Atlantic cod (Salter et al., 2019). The reason for the high degree of variation in these relationships is not fully understood, though it could be due to taxon-specific affinities to different primers. For example, a DNA metabarcoding study using a mock community of aquatic macroinvertebrates found four orders of magnitude differences in sequence abundances of 52 taxa despite standardization of biomass prior to PCR amplification and sequencing (Elbrecht and Leese, 2015). These issues are compounded by the fact that different DNA metabarcoding primers and mixture templates can perform differently (Hajibabaei et al., 2019b). Further, different organisms have different copy numbers of mitochondrial DNA, chloroplast DNA, ribosomal RNA, and internal transcribed spacer genes, and there can even be variation in the copy number of some genes in tissues within the same organism (Guryev et al., 2008; Henrichsen et al., 2009). These complications could mean that individual assays need to be done for each taxa and primer combination in order to get reliable abundance estimates from eDNA sequence data, and even then individual taxa patterns are likely dependent both upon the choice of primers and the properties of the taxa mixtures (Piñol et al., 2019). Despite these challenges, quantification of abundance from eDNA samples remains one of the most desired and broadly applicable advancements that will emerge from the field of environmental genomics. We anticipate that promising results will emerge from hierarchical multispecies occupancy models, for example, which would allow—through replicated presence-absence data—calculation of occupancy probabilities that can reveal patterns of relative abundance. Further, more direct approaches could be undertaken in the lab, such as using internal standards or individual barcodes for each molecule in a PCR reaction. Both approaches would generate even larger datasets and require additional bioinformatic steps, and thus would be contingent upon other technological and scientific advancements (Figure 3).
Advanced Modeling to Improve Species Detection
While the genomic and technological advancements we have outlined up to this point will impact the field of biodiversity assessment broadly, advances in modeling will specifically help end-users, including regulators and resource managers who are commissioned with making use of the high-resolution biodiversity information emerging from next-generation biomonitoring approaches. As technological advances bring down the costs of sample and bioinformatic processing, more sophisticated community occupancy models will be able to make use of repeated samples in order to provide vital information about taxon-specific detection probabilities and inform practitioners about the sampling effort required to answer specific questions in specific systems, which will greatly enhance the efficiency of next-generation bioassessment compared to traditional bioassessment.
Currently, applied hierarchical modeling can be used to build occupancy models to address questions related to species' detection and abundance (Kery and Royle, 2015). However, studies utilizing DNA metabarcoding information are often limited in scope (i.e., limited environmental gradients), scale (i.e., the spatial extent of the study), or taxonomic coverage (i.e., covering only a single taxon or narrow taxonomic group) (Schmidt et al., 2013; Doi et al., 2019), and advancement will be made by expanding these models to include datasets covering broader ranges and along more gradients of environmental change, as well as incorporating variation from more of the steps along the biodiversity-generation pathway (i.e., from sample collection to sequencing and bioinformatics). For example, while current models have focused on detection in the context of sampling effort, more sophisticated Bayesian hierarchical models could be built to also include detection probabilities associated with different primers, sequencing depths, and other steps along the sampling-to-sequencing pathway (Doi et al., 2019), which would provide valuable information at all steps of the process, allowing next-generation bioassessment practitioners to better design experiments that more efficiently make use of resources (Lugg et al., 2018). Applying multi-species occupancy modeling to the large datasets generated from metabarcoding can reveal whole-community responses to environmental variables while accounting for variation in sampling and processing.
Phylogenetics
Greater sequencing depth and long-read applications (where DNA preservation allows) will enable construction of phylogenetic trees for entire communities, potentially leading to a new field of metaphylogeography research (Turon et al., 2020). Most efforts to combine DNA metabarcoding with phylogenetic reconstruction have been limited to targeted groups of known and closely related organisms (e.g., Andújar et al., 2015; Gómez-Rodríguez et al., 2015), but recent efforts have been made to assess intraspecific genetic diversity from communities in bulk metabarcoding samples (Elbrecht et al., 2018), and this diversity has been used to construct phylogenies from metabarcoding data in order to explore metaphylogeographic patterns (Turon et al., 2020). This approach is limited by current DNA metabarcoding methods that rely on short sequence read lengths (i.e., normally ~150–300 bases), leading to coarse and unresolved phylogenetic trees. In theory, this problem could be partially overcome by using multiple primers covering multiple loci, but there is currently no way of determining which sequences come from the same organism across markers, and thus bioinformatic developments are required to provide ways of merging metabarcoding data across multiple primers. However, with much greater sequencing capacity afforded by state-of-the-art platforms, some of these limitations can be quickly overcome, as more of the genome can be covered. Combining DNA metabarcoding with shotgun sequencing [e.g., mitochondrial metagenomics: (Crampton-Platt et al., 2016)] and taking advantage of greater sequencing depths will further resolve the problems associated with short read lengths, but assembly problems will remain, especially for poorly studied or closely related species, as well as rare or under-represented taxa. Since these trees will likely be difficult to create for all taxa at the species level (i.e., because metabarcoding markers are from a limited number of linkage groups), this approach may not be able to resolve phylogenies of closely related taxa or address community assembly patterns, which require an understanding of species' evolutionary histories (Cavender-Bares et al., 2009). Constructing metaphylogenies from DNA metabarcoding data will nevertheless lead to exciting possibilities for advancing biodiversity research, allowing practitioners to address an array of questions ranging from basic (e.g., biogeography, connectivity, dispersal) to applied (e.g., conservation genetics, invasion genetics, determining protected areas) research (Turon et al., 2020), and improving the current standards of biodiversity assessment by allowing for a more holistic exploration of rare or unknown taxa in hyper-diverse, poorly studied ecosystems (Papadopoulou et al., 2015).
Population Genetics
Modern next-generation platforms are so highly parallelized that genome coverage for each taxon in the sample allows for assessment of intraspecific genetic variation, enabling researchers to address questions about population genetics from eDNA (Sigsgaard et al., 2016; Parsons et al., 2018; Marshall and Stepien, 2019). While most population genetic approaches now use thousands of single nucleotide polymorphisms (SNPs) (Adams et al., 2019), recent application of ancient bulk bone DNA metabarcoding has successfully characterized mitochondrial DNA haplotypes of extinct lineages of frogs and birds (Seersholm et al., 2018). Since eDNA sampling is non-invasive and easier than directly sampling tissue from organisms, population genetics applications can potentially be done on much larger scales. While assessment of intraspecific variation of the entire community assemblage of a sample has been limited to a couple case studies (Elbrecht et al., 2018; Turon et al., 2020), this would revolutionize bioassessment, as the benefits of getting population genetic data from environmental samples are numerous (e.g., conservation genetics, invasion biology, protecting endangered species) and have diverse applications affecting many stakeholder groups and environmental disciplines (Figure 3).
Currently, population genetics has been mostly limited by primer development for target organisms, focusing on no more than a few taxa at a time to answer very specific questions related to these species. Improved read quality afforded by state-of-the-art sequencers (e.g., Illumina NovaSeq), however, will rapidly improve the quality and coverage of the genome of all taxa recovered from DNA metabarcoding, further advancing this environmental genomic application. Additionally, mitochondrial metagenomic approaches that combine shotgun sequencing and DNA metabarcoding will allow for read mapping that may provide the quantitative information on intraspecific genetic variation needed to assess population genetic structure (Crampton-Platt et al., 2016); combining these approaches [sensu (Elbrecht et al., 2018)] would then make it possible to assess the genetic structure of any taxon of interest in the community, or even ask larger questions about the entire metacommunity.
Food Webs and Functional Genes: Connecting Biostructure to Ecosystem Function
Recently, interest in utilizing the rich biodiversity data from DNA metabarcoding for ecological network analysis has surged. These tools promise to provide rapid assessment of structural attributes of a system (Bohan et al., 2017). In their simplest form, networks created from DNA metabarcoding taxonomic lists are co-occurrence networks, and like any co-occurrence network they do not measure actual connections among species; rather, they depict the possible set of interacting species in graphical form. Co-occurrence networks can be refined through filtering based on mathematical rules [e.g., probability of interaction (Morales-Castilla et al., 2015)], known phylogenetic relationships (Morales-Castilla et al., 2015), or trait matching (Compson et al., 2018, 2019). By automating construction of networks and food webs, they can be linked to large biomonitoring datasets, providing new opportunities for complex, diagnostic development that will hopefully be more targeted and predictive than traditional bioindicators (Makiola et al., 2020) and, because networks provide taxa-free biodiversity estimates, will provide the potential for global indicator development [e.g., Essential Biodiversity Variables (Kissling et al., 2018)]. Furthermore, synergistic advancements will arise from merging occupancy modeling and machine learning approaches to create more robust ecological networks. For example, hierarchical models could incorporate relative read abundance information, and outputs from these models could be integrated into ecological networks and heuristic food webs to estimate interaction strengths and calculate probabilities of interaction [sensu (Vázquez et al., 2007; Morales-Castilla et al., 2015)], which will make these models immediately more quantitative. As more data are generated that capitalize on these approaches, there will also be increasing volumes of high-quality information to feed into machine learning algorithms, leading to more predictive modeling of diverse ecosystems and an unprecedented opportunity for practitioners to anticipate change and prevent ecosystem impairment in real time.
Enhanced sequencing depth from newer next-generation, deep-sequencing platforms will also allow for the exploration of functional genes associated with environmental samples (Carradec et al., 2018), facilitating exciting new applications for connecting aspects of biostructure with measures of ecosystem function. Until recently, research into functional genes in eukaryotes has been restricted to laborious efforts to document taxon-specific functions for addressing a narrow range of questions across a limited spectrum of environmental conditions. In much the same way that deep sequencing will provide taxon-specific genetic variation associated with all the organisms in a sample, this approach will enable rapid assessment of biodiversity and associated functions in a sample, giving ecologists the power to address metacommunity questions at unprecedented spatial and temporal scales. Currently, functional gene analysis can be performed in two ways. First, bioinformatic software packages can be used to query databases of reference genomes to link known taxa to their functions. In these cases, marker gene analysis is first done to identify taxa, which are then matched to reference databases of taxa genomes. For example, machine learning has been leveraged to link genus-level predictions of function in microbial communities using Phylogenetic Investigation of Communities by Reconstruction of Unobserved States [PICRUSt: (Langille et al., 2013)]. While this approach is limited to inferential assessments of function, pattern exploration, and hypothesis generation (Douglas et al., 2018), advances in machine learning, coupled with more data to feed into machine learning algorithms (Jordan and Mitchell, 2015), will improve this type of approach moving forward. A second method of functional gene analysis involves shotgun sequencing of functional genes directly from samples and then linking these sequenced genes to annotated functional gene databases. While this approach does not involve marker gene analysis to identify taxa, DNA metabarcoding can be done to supplement shotgun sequencing results, but this approach will not create a direct linkage between specific taxa and the functions they perform. Currently, efforts are being made to expand and annotate functional gene databases [e.g., the Kyoto Encyclopedia of Genes and Genomes (KEGG), https://www.genome.jp/kegg/], which facilitate the mapping of genes to functions across a wide-range of biodiversity, bringing incredible added value to projects that leverage the greater sequencing depth afforded by newer sequencing platforms. As machine learning is adopted for advanced data discovery applications (e.g., Gonzalez et al., 2016; Liu et al., 2016), these tools will help to rapidly improve both functional and trait databases.
Collectively, these advancements will equip resource managers with powerful bioassessment tools for rapid, whole ecosystem assessments, as functional profiles can have much greater discriminatory power compared to taxonomic profiles, especially in cases where taxonomic profiles are highly variable (Turnbaugh et al., 2009; Huttenhower et al., 2012; Xu et al., 2014). Further, these advances will facilitate bioindicator development (Makiola et al., 2020), as tools capitalizing on the biostructure in a system—such ecological networks and heuristic food webs—can potentially provide higher resolution information that is more sensitive to environmental change and has a much wider breadth of application [e.g., estimating trophic position of heuristic food web members: (Compson et al., 2019)]. Finally, by linking DNA-based food webs to functional gene assessments, it will be possible to relate community and food web structure to ecosystem function at unprecedented spatial and temporal scales, enabling scientists to test global hypotheses arising from metacommunity theory (Leibold and Chase, 2017).
Outlook: The Future is Now
DNA metabarcoding generates whole-community biodiversity data rapidly from relatively easy to collect and non-invasive samples. With advances in laboratory processing, sequencing technologies, bioinformatic processing, and analytical methods, the amount of data that can be recovered from a sample is huge and applications of the resulting data are widespread and transformative (Figure 3). While some limitations to DNA metabarcoding remain, we have highlighted some of the efforts to refine and enhance this approach, and extremely useful, high-resolution biodiversity data can be generated right now. Furthermore, due to the nature of these methods, in the future raw sequence data can be re-analyzed with improved bioinformatic pipelines and reference databases, and archived DNA extracts can be re-analyzed, providing us with a way of storing a snap-shot in time of biodiversity data that could become important—and extremely rare (Downs et al., 2011)—baseline data for future bioassessment work. The applications of metabarcoding are diverse and can serve a range of users, from those interested in assessment of targeted taxa to those interested in total biodiversity assessment, from microbes to mammals. With concurrent technological (i.e., improved computing and sequencing platforms; robotics and automation) and scientific advances (i.e., refined methods and modeling approaches; improved genomic, trait, and functional databases), exciting possibilities for extracting even more information from environmental sequences will usher in a new era of bioassessment, where quantifying community abundance and occupancy, exploring population genetic and phylogenetic relationships, and linking whole-system biostructure with measurements of ecosystem function will not only be possible, but become routine, transforming the speed and depth with which we can explore, model, predict—and therefore protect—global ecosystems.
Data Availability Statement
The original contributions presented in the study are included in the article/Supplementary Materials, further inquiries can be directed to the corresponding author.
Author Contributions
ZC, GS, and MH conceived the idea for the manuscript. ZC, BM, and MH developed the approach for the systematic literature review. ZC and BM gathered the literature and extracted the data from the papers. ZC performed all analyses and wrote the first draft of the manuscript. All authors provided substantial feedback aiding in subsequent revisions of the manuscript.
Funding
This work was partly funded through an award from the Atlantic Canada Opportunities Agency's Atlantic Innovation Fund (project number: 781-37749-207993), and a grant from Petroleum Research Newfoundland and Labrador. Any opinions, findings, conclusions, or recommendations expressed in this publication are those of the authors and do not necessarily reflect the views of Petroleum Research or its members.
Conflict of Interest
ZC, BM, GS, and NF were employed by the company eDNAtec Inc. MH was the founder and CSO of eDNAtec Inc.
Acknowledgments
We thank staff at the Centre for Environmental Genomics Applications (CEGA), including Hoda Rajabi, Emily Porter, Kerry Hobrecker, Avery McCarthy, and Shahrokh Shekarriz, who provided valuable insights into the development of this study. Additionally, Bruce Hungate provided feedback on an early draft of the manuscript, and Wendy Monk provided important assistance in generating and refining the global map.
Supplementary Material
The Supplementary Material for this article can be found online at: https://www.frontiersin.org/articles/10.3389/fevo.2020.581835/full#supplementary-material
References
Adams, C. I. M., Knapp, M., Gemmell, N. J., Jeunen, G.-J., Bunce, M., Lamare, M. D., et al. (2019). Beyond biodiversity: can environmental DNA (eDNA) cut it as a population genetics tool? Genes 10:192. doi: 10.3390/genes10030192
Alley, R. B., Emanuel, K. A., and Zhang, F. (2019). Advances in weather prediction. Science 363, 342–344. doi: 10.1126/science.aav7274
Andújar, C., Arribas, P., Ruzicka, F., Crampton-Platt, A., Timmermans, M. J. T. N., and Vogler, A. P. (2015). Phylogenetic community ecology of soil biodiversity using mitochondrial metagenomics. Mol. Ecol. 24, 3603–3617. doi: 10.1111/mec.13195
Araújo, M. B., and Guisan, A. (2006). Five (or so) challenges for species distribution modelling. J. Biogeogr. 33, 1677–1688. doi: 10.1111/j.1365-2699.2006.01584.x
Aylagas, E., Borja, Á., Irigoien, X., and Rodríguez-Ezpeleta, N. (2016). Benchmarking DNA metabarcoding for biodiversity-based monitoring and assessment. Front. Mar. Sci. 3:96. doi: 10.3389/fmars.2016.00096
Baillie, S. M., McGowan, C., May-McNally, S., Leggatt, R., Sutherland, B. J. G., and Robinson, S. (2019). Environmental DNA and Its Applications to Fisheries and Oceans Canada: National Needs and Priorities. Canada Department of Fisheries and Oceans Canada; National Capital Region; Ecosystems and Oceans Science Sector. Available online at: http://epe.lac-bac.gc.ca/100/201/301/weekly_acquisitions_list-ef/2019/19-33/publications.gc.ca/collections/collection_2019/mpo-dfo/Fs97-6-3329-eng.pdf (accessed April 23, 2020)
Bakker, J., Wangensteen, O. S., Baillie, C., Buddo, D., Chapman, D. D., Gallagher, A. J., et al. (2019). Biodiversity assessment of tropical shelf eukaryotic communities via pelagic eDNA metabarcoding. Ecol. Evol. 9, 14341–14355. doi: 10.1002/ece3.5871
Barnes, M. A., Turner, C. R., Jerde, C. L., Renshaw, M. A., Chadderton, W. L., and Lodge, D. M. (2014). Environmental conditions influence eDNA persistence in aquatic systems. Environ. Sci. Technol. 48, 1819–1827. doi: 10.1021/es404734p
Bell, G., Hey, T., and Szalay, A. (2009). Computer science: beyond the data deluge. Science 323, 1297–1298. doi: 10.1126/science.1170411
Berger, S. A., Krompass, D., and Stamatakis, A. (2011). Performance, accuracy, and web server for evolutionary placement of short sequence reads under maximum likelihood. Syst. Biol. 60, 291–302. doi: 10.1093/sysbio/syr010
Birk, S., Bonne, W., Borja, A., Brucet, S., Courrat, A., Poikane, S., et al. (2012). Three hundred ways to assess Europe's surface waters: an almost complete overview of biological methods to implement the Water Framework Directive. Ecol. Indic. 18, 31–41. doi: 10.1016/j.ecolind.2011.10.009
Bohan, D. A., Vacher, C., Tamaddoni-Nezhad, A., Raybould, A., Dumbrell, A. J., and Woodward, G. (2017). Next-generation global biomonitoring: large-scale, automated reconstruction of ecological networks. Trends Ecol. Evol. 32, 477–487. doi: 10.1016/j.tree.2017.03.001
Bolyen, E., Rideout, J. R., Dillon, M. R., Bokulich, N. A., Abnet, C. C., Al-Ghalith, G. A., et al. (2019). Reproducible, interactive, scalable and extensible microbiome data science using QIIME 2. Nat. Biotechnol. 37, 852–857. doi: 10.1038/s41587-019-0209-9
Bush, A., Compson, Z. G., Monk, W. A., Porter, T. M., Steeves, R., Emilson, E., et al. (2019). Studying ecosystems with DNA metabarcoding: lessons from biomonitoring of aquatic macroinvertebrates. Front. Ecol. Evol. 7:434. doi: 10.3389/fevo.2019.00434
Bush, A., Monk, W. A., Compson, Z. G., Peters, D. L., Porter, T. M., Shokralla, S., et al. (2020). DNA metabarcoding reveals metacommunity dynamics in a threatened boreal wetland wilderness. Proc. Natl. Acad. Sci. U.S.A. 117, 8539–8545. doi: 10.1073/pnas.1918741117
Cahill, A. E., Pearman, J. K., Borja, A., Carugati, L., Carvalho, S., Danovaro, R., et al. (2018). A comparative analysis of metabarcoding and morphology-based identification of benthic communities across different regional seas. Ecol. Evol. 8, 8908–8920. doi: 10.1002/ece3.4283
Callahan, B. J., McMurdie, P. J., Rosen, M. J., Han, A. W., Johnson, A. J. A., and Holmes, S. P. (2016). DADA2: High-resolution sample inference from Illumina amplicon data. Nat. Methods 13, 581–583. doi: 10.1038/nmeth.3869
Canadian Aquatic Biomonitoring Network Field Manual: Wadeable Streams (2012). Available online at: http://publications.gc.ca/collections/collection_2012/ec/En84-87-2012-eng.pdf (accessed October 20, 2020).
Carradec, Q., Pelletier, E., Da Silva, C., Alberti, A., Seeleuthner, Y., Blanc-Mathieu, R., et al. (2018). A global ocean atlas of eukaryotic genes. Nat. Commun. 9, 1–13. doi: 10.1038/s41467-017-02342-1
Casey, J. M., Meyer, C. P., Morat, F., Brandl, S. J., Planes, S., and Parravicini, V. (2019). Reconstructing hyperdiverse food webs: gut content metabarcoding as a tool to disentangle trophic interactions on coral reefs. Methods Ecol. Evol. 10, 1157–1170. doi: 10.1111/2041-210X.13206
Cavender-Bares, J., Kozak, K. H., Fine, P. V. A., and Kembel, S. W. (2009). The merging of community ecology and phylogenetic biology. Ecol. Lett. 12, 693–715. doi: 10.1111/j.1461-0248.2009.01314.x
Claudy, M. C., Garcia, R., and O'Driscoll, A. (2015). Consumer resistance to innovation—a behavioral reasoning perspective. J. Acad. Mark. Sci. 43, 528–544. doi: 10.1007/s11747-014-0399-0
Compson, Z. G., Monk, W. A., Curry, C. J., Gravel, D., Bush, A., Baker, C. J. O., et al. (2018). “Linking DNA metabarcoding and text mining to create network-based biomonitoring tools: a case study on boreal wetland macroinvertebrate communities,” in Advances in Ecological Research: Next Generation Biomonitoring: Part 2, eds D. A. Bohan, A. J. Dumbrell, G. Woodward, and M. Jackson (London: Elsevier), 33–74.
Compson, Z. G., Monk, W. A., Hayden, B., Bush, A., O'Malley, Z., Hajibabaei, M., et al. (2019). Network-based biomonitoring: exploring freshwater food webs with stable isotope analysis and DNA metabarcoding. Front. Ecol. Evol. 7:395. doi: 10.3389/fevo.2019.00395
Contreras, J. L., and Deshmukh, V. (2017). Development of the Personal Genomics Industry. Rochester, NY: Social Science Research Network. Available online at: https://papers.ssrn.com/abstract=3092313 (accessed April 23, 2020).
Crampton-Platt, A., Yu, D. W., Zhou, X., and Vogler, A. P. (2016). Mitochondrial metagenomics: letting the genes out of the bottle. GigaScience 5:15. doi: 10.1186/s13742-016-0120-y
Creer, S., Deiner, K., Frey, S., Porazinska, D., Taberlet, P., Thomas, W. K., et al. (2016). The ecologist's field guide to sequence-based identification of biodiversity. Methods Ecol. Evol. 7, 1008–1018. doi: 10.1111/2041-210X.12574
Curry, C. J., Gibson, J. F., Shokralla, S., Hajibabaei, M., and Baird, D. J. (2018). Identifying North American freshwater invertebrates using DNA barcodes: are existing COI sequence libraries fit for purpose? Freshw. Sci. 37, 178–189. doi: 10.1086/696613
Darling, J. A. (2019). How to learn to stop worrying and love environmental DNA monitoring. Aquat. Ecosyst. Health Manag. 22, 440–451. doi: 10.1080/14634988.2019.1682912
Davis, K., and Borisenko, A. (2017). Introduction to access and benefit-sharing and the nagoya protocol: what DNA barcoding researchers need to know. Adv. Books 1:e22579. doi: 10.3897/ab.e22579
Deiner, K., Bik, H. M., Mächler, E., Seymour, M., Lacoursière-Roussel, A., Altermatt, F., et al. (2017). Environmental DNA metabarcoding: transforming how we survey animal and plant communities. Mol. Ecol. 26, 5872–5895. doi: 10.1111/mec.14350
Deiner, K., Lopez, J., Bourne, S., Holman, L., Seymour, M., Grey, E. K., et al. (2018). Optimising the detection of marine taxonomic richness using environmental DNA metabarcoding: the effects of filter material, pore size and extraction method. Metabarcoding Metagenomics 2:e28963. doi: 10.3897/mbmg.2.28963
deWitt, P., and deWitt, C. A. (2008). Research article: how long does it take to prepare an environmental impact statement? Environ. Pract. 10, 164–174. doi: 10.1017/S146604660808037X
Dickie, I. A., Boyer, S., Buckley, H. L., Duncan, R. P., Gardner, P. P., Hogg, I. D., et al. (2018). Towards robust and repeatable sampling methods in eDNA-based studies. Mol. Ecol. Resour. 18, 940–952. doi: 10.1111/1755-0998.12907
Doi, H., Fukaya, K., Oka, S., Sato, K., Kondoh, M., and Miya, M. (2019). Evaluation of detection probabilities at the water-filtering and initial PCR steps in environmental DNA metabarcoding using a multispecies site occupancy model. Sci. Rep. 9:3581. doi: 10.1038/s41598-019-40233-1
Douglas, G. M., Beiko, R. G., and Langille, M. G. I. (2018). “Predicting the Functional Potential of the Microbiome from Marker Genes Using PICRUSt,” in Microbiome Analysis: Methods and Protocols Methods in Molecular Biology, eds R. G. Beiko, W. Hsiao, and J. Parkinson (New York, NY: Springer), 169–177.
Downs, P. W., Singer, M. S., Orr, B. K., Diggory, Z. E., and Church, T. C. (2011). Restoring ecological integrity in highly regulated rivers: the role of baseline data and analytical references. Environ. Manage. 48, 847–864. doi: 10.1007/s00267-011-9736-y
Eichmiller, J. J., Best, S. E., and Sorensen, P. W. (2016). Effects of temperature and trophic state on degradation of environmental DNA in lake water. Environ. Sci. Technol. 50, 1859–1867. doi: 10.1021/acs.est.5b05672
Elbrecht, V., and Leese, F. (2015). Can DNA-based ecosystem assessments quantify species abundance? Testing primer bias and biomass—sequence relationships with an innovative metabarcoding protocol. PLoS ONE 10:e0130324. doi: 10.1371/journal.pone.0130324
Elbrecht, V., Vamos, E. E., Steinke, D., and Leese, F. (2018). Estimating intraspecific genetic diversity from community DNA metabarcoding data. PeerJ 6:e4644. doi: 10.7717/peerj.4644
Erdozain, M., Thompson, D. G., Porter, T. M., Kidd, K. A., Kreutzweiser, D. P., Sibley, P. K., et al. (2019). Metabarcoding of storage ethanol vs. conventional morphometric identification in relation to the use of stream macroinvertebrates as ecological indicators in forest management. Ecol. Indic. 101, 173–184. doi: 10.1016/j.ecolind.2019.01.014
Estes, N. (2019). Our History Is the Future: Standing Rock Versus the Dakota Access Pipeline, and the Long Tradition of Indigenous Resistance. Verso Books.
Fahner, N. A., Shokralla, S., Baird, D. J., and Hajibabaei, M. (2016). Large-scale monitoring of plants through environmental DNA metabarcoding of soil: recovery, resolution, and annotation of four DNA markers. PLoS ONE 11:e0157505. doi: 10.1371/journal.pone.0157505
Ficetola, G. F., Miaud, C., Pompanon, F., and Taberlet, P. (2008). Species detection using environmental DNA from water samples. Biol. Lett. 4, 423–425. doi: 10.1098/rsbl.2008.0118
Ford, J. D., Ford, L. W., and D'Amelio, A. (2008). Resistance to change: the rest of the story. Acad. Manage. Rev. 33, 362–377. doi: 10.5465/amr.2008.31193235
Freeland, J. R. (2017). The importance of molecular markers and primer design when characterizing biodiversity from environmental DNA. Genome 60, 358–374. doi: 10.1139/gen-2016-0100
Friberg, N., Bonada, N., Bradley, D. C., Dunbar, M. J., Edwards, F. K., Grey, J., et al. (2011). “Biomonitoring of human impacts in freshwater ecosystems: the good, the bad and the ugly,” in Advances in Ecological Research: Ecosystems in a Human-Modified Landscape: A European Perspective, ed G. Woodward (Amsterdam: Elsevier), 1–68.
Geiger, S., and Gross, N. (2019). A tidal wave of inevitable data? Assetization in the consumer genomics testing industry. Bus. Soc. 1–36. doi: 10.1177/0007650319826307
Gibson, J., Shokralla, S., Curry, C., Baird, D. J., Monk, W. A., King, I., et al. (2015). Large-scale biomonitoring of remote and threatened ecosystems via high-throughput sequencing. PLoS ONE 10:e0138432. doi: 10.1371/journal.pone.0138432
Gibson, J., Shokralla, S., Porter, T. M., King, I., Konynenburg, S., van Janzen, D. H., et al. (2014). Simultaneous assessment of the macrobiome and microbiome in a bulk sample of tropical arthropods through DNA metasystematics. Proc. Natl. Acad. Sci. U.S.A. 111, 8007–8012. doi: 10.1073/pnas.1406468111
Gómez-Rodríguez, C., Crampton-Platt, A., Timmermans, M. J. T. N., Baselga, A., and Vogler, A. P. (2015). Validating the power of mitochondrial metagenomics for community ecology and phylogenetics of complex assemblages. Methods Ecol. Evol. 6, 883–894. doi: 10.1111/2041-210X.12376
Gonzalez, G. H., Tahsin, T., Goodale, B. C., Greene, A. C., and Greene, C. S. (2016). Recent advances and emerging applications in text and data mining for biomedical discovery. Brief. Bioinform. 17, 33–42. doi: 10.1093/bib/bbv087
Graham, C. (2017). The future is not what it used to be: oil and gas strategies for a carbon-conscious world. APPEA J. 57, 459–461. doi: 10.1071/AJ17017
Grüning, B., Dale, R., Sjödin, A., Rowe, J., Chapman, B. A., Tomkins-Tinch, C. H., et al. (2018). Bioconda: sustainable and comprehensive software distribution for the life sciences. Nat. Methods 15, 475–476. doi: 10.1038/s41592-018-0046-7
Guryev, V., Saar, K., Adamovic, T., Verheul, M., van Heesch, S. A. A. C., Cook, S., et al. (2008). Distribution and functional impact of DNA copy number variation in the rat. Nat. Genet. 40, 538–545. doi: 10.1038/ng.141
Hajibabaei, M., Baird, D. J., Fahner, N. A., Beiko, R., and Golding, G. B. (2016). A new way to contemplate darwin's tangled bank: how DNA barcodes are reconnecting biodiversity science and biomonitoring. Philos. Trans. R. Soc. B Biol. Sci. 371:20150330. doi: 10.1098/rstb.2015.0330
Hajibabaei, M., Porter, T. M., Robinson, C. V., Baird, D. J., Shokralla, S., and Wright, M. T. G. (2019a). Watered-down biodiversity? A comparison of metabarcoding results from DNA extracted from matched water and bulk tissue biomonitoring samples. PLoS ONE 14:e0225409. doi: 10.1371/journal.pone.0225409
Hajibabaei, M., Porter, T. M., Wright, M., and Rudar, J. (2019b). COI metabarcoding primer choice affects richness and recovery of indicator taxa in freshwater systems. PLoS ONE 14:e0220953. doi: 10.1371/journal.pone.0220953
Hajibabaei, M., Spall, J. L., Shokralla, S., and van Konynenburg, S. (2012). Assessing biodiversity of a freshwater benthic macroinvertebrate community through non-destructive environmental barcoding of DNA from preservative ethanol. BMC Ecol. 12:28. doi: 10.1186/1472-6785-12-28
Hansen, B. K., Bekkevold, D., Clausen, L. W., and Nielsen, E. E. (2018). The sceptical optimist: challenges and perspectives for the application of environmental DNA in marine fisheries. Fish Fish. 19, 751–768. doi: 10.1111/faf.12286
Henrichsen, C. N., Vinckenbosch, N., Zöllner, S., Chaignat, E., Pradervand, S., Schütz, F., et al. (2009). Segmental copy number variation shapes tissue transcriptomes. Nat. Genet. 41, 424–429. doi: 10.1038/ng.345
Hering, D., Borja, A., Jones, J. I., Pont, D., Boets, P., Bouchez, A., et al. (2018). Implementation options for DNA-based identification into ecological status assessment under the European water framework directive. Water Res. 138, 192–205. doi: 10.1016/j.watres.2018.03.003
Hering, D., Buffagni, A., Moog, O., Sandin, L., Sommerhäuser, M., Stubauer, I., et al. (2003). The development of a system to assess the ecological quality of streams based on macroinvertebrates – design of the sampling programme within the AQEM project. Int. Rev. Hydrobiol. 88, 345–361. doi: 10.1002/iroh.200390030
Hey, T., and Trefethen, A. (2003). “The data deluge: an e-science perspective,” in Wiley Series in Communications Networking & Distributed Systems, eds F. Berman, G. Fox, and T. Hey (Chichester: John Wiley & Sons, Ltd), 809–824.
Hobbs, J., Goldberg, C., Helbing, C., and Veldhoen, N. (2017). Environmental DNA Protocol for Freshwater Aquatic Ecosystems: Version 2.2. Available online at: https://www.hemmera.com/wp-content/uploads/2018/08/171115-eDNA-protocol-V2.2.pdf (accessed October 20, 2020).
Huttenhower, C., Gevers, D., Knight, R., Abubucker, S., Badger, J. H., Chinwalla, A. T., et al. (2012). Structure, function and diversity of the healthy human microbiome. Nature 486, 207–214. doi: 10.1038/nature11234
IPBES (2019). Global Assessment Report on Biodiversity and Ecosystem Services of the Intergovernmental Science-Policy Platform on Biodiversity and Ecosystem Services. IPBES Secretariat, Bonn.
Jarman, S. N., Berry, O., and Bunce, M. (2018). The value of environmental DNA biobanking for long-term biomonitoring. Nat. Ecol. Evol. 2, 1192–1193. doi: 10.1038/s41559-018-0614-3
Jordan, M. I., and Mitchell, T. M. (2015). Machine learning: trends, perspectives, and prospects. Science 349, 255–260. doi: 10.1126/science.aaa8415
Kery, M., and Royle, J. A. (2015). Applied Hierarchical Modeling in Ecology: Analysis of Distribution, Abundance and Species Richness in R and BUGS: Volume 1: Prelude and Static Models. London: Elsevier.
Kissling, W. D., Walls, R., Bowser, A., Jones, M. O., Kattge, J., Agosti, D., et al. (2018). Towards global data products of essential biodiversity variables on species traits. Nat. Ecol. Evol. 2, 1531–1540. doi: 10.1038/s41559-018-0667-3
König, M., and Neumayr, L. (2017). Users' resistance towards radical innovations: the case of the self-driving car. Transp. Res. Part F Traffic Psychol. Behav. 44, 42–52. doi: 10.1016/j.trf.2016.10.013
Koski, L. B., and Golding, G. B. (2001). The closest BLAST hit is often not the nearest neighbor. J. Mol. Evol. 52, 540–542. doi: 10.1007/s002390010184
Koziol, A., Stat, M., Simpson, T., Jarman, S., DiBattista, J. D., Harvey, E. S., et al. (2019). Environmental DNA metabarcoding studies are critically affected by substrate selection. Mol. Ecol. Resour. 19, 366–376. doi: 10.1111/1755-0998.12971
Lamb, P. D., Hunter, E., Pinnegar, J. K., Creer, S., Davies, R. G., and Taylor, M. I. (2019). How quantitative is metabarcoding: a meta-analytical approach. Mol. Ecol. 28, 420–430. doi: 10.1111/mec.14920
Langille, M. G. I., Zaneveld, J., Caporaso, J. G., McDonald, D., Knights, D., Reyes, J. A., et al. (2013). Predictive functional profiling of microbial communities using 16S rRNA marker gene sequences. Nat. Biotechnol. 31, 814–821. doi: 10.1038/nbt.2676
Langmead, B., and Nellore, A. (2018). Cloud computing for genomic data analysis and collaboration. Nat. Rev. Genet. 19, 208–219. doi: 10.1038/nrg.2017.113
Laramie, M., Pilliod, D., Goldberg, C., and Strickler, K. (2015). Environmental DNA Sampling Protocol—Filtering Water to Capture DNA From Aquatic Organisms: U. S. Geological Survey Techniques and Methods: Book 2, Chapter A13. Reston, VA: U.S. Geological Survey. P. 15 Available online at: https://pubs.usgs.gov/tm/02/a13/tm2a13.pdf
Leese, F., Bouchez, A., Abarenkov, K., Altermatt, F., Borja, Á., Bruce, K., et al. (2018). “Why we need sustainable networks bridging countries, disciplines, cultures and generations for aquatic biomonitoring 2.0: a perspective derived from the DNAqua-Net COST action,” in Advances in Ecological Research: Next Generation Biomonitoring: Part 1, eds D. A. Bohan, A. J. Dumbrell, G. Woodward, and M. Jackson (Amsterdam: Elsevier), 63–99.
Lefrançois, E., Apothéloz-Perret-Gentil, L., Blancher, P., Botreau, S., Chardon, C., Crepin, L., et al. (2018). Development and implementation of eco-genomic tools for aquatic ecosystem biomonitoring: the SYNAQUA French-Swiss program. Environ. Sci. Pollut. Res. 25, 33858–33866. doi: 10.1007/s11356-018-2172-2
Leibold, M. A., and Chase, J. M. (2017). Metacommunity Ecology, Vol. 59. Princeton, NJ: Princeton University Press.
Levy, S. E., and Myers, R. M. (2016). Advancements in next-generation sequencing. Annu. Rev. Genomics Hum. Genet. 17, 95–115. doi: 10.1146/annurev-genom-083115-022413
Liu, F., Chen, J., Jagannatha, A., and Yu, H. (2016). Learning for biomedical information extraction: methodological review of recent advances. arXiv arXiv: 160607993 Cs. Available online at: http://arxiv.org/abs/1606.07993 (accessed April 24, 2020).
Liu, W., and Dunford, M. (2016). Inclusive globalization: unpacking China's Belt and Road Initiative. Area Dev. Policy 1, 323–340. doi: 10.1080/23792949.2016.1232598
López-Escardó, D., Paps, J., de Vargas, C., Massana, R., Ruiz-Trillo, I., and del Campo, J. (2018). Metabarcoding analysis on European coastal samples reveals new molecular metazoan diversity. Sci. Rep. 8:9106. doi: 10.1038/s41598-018-27509-8
Lugg, W. H., Griffiths, J., Rooyen, A. R., van Weeks, A. R., and Tingley, R. (2018). Optimal survey designs for environmental DNA sampling. Methods Ecol. Evol. 9, 1049–1059. doi: 10.1111/2041-210X.12951
Macher, J.-N., Vivancos, A., Piggott, J. J., Centeno, F. C., Matthaei, C. D., and Leese, F. (2018). Comparison of environmental DNA and bulk-sample metabarcoding using highly degenerate cytochrome c oxidase I primers. Mol. Ecol. Resour. 18, 1456–1468. doi: 10.1111/1755-0998.12940
Mächler, E., Deiner, K., Spahn, F., and Altermatt, F. (2016). Fishing in the water: effect of sampled water volume on environmental DNA-based detection of macroinvertebrates. Environ. Sci. Technol. 50, 305–312. doi: 10.1021/acs.est.5b04188
Macintosh, A., Gibbons, P., Jones, J., Constable, A., and Wilkinson, D. (2018). Delays, stoppages and appeals: an empirical evaluation of the adverse impacts of environmental citizen suits in the New South Wales land and environment court. Environ. Impact Assess. Rev. 69, 94–103. doi: 10.1016/j.eiar.2018.01.001
Majaneva, M., Diserud, O. H., Eagle, S. H. C., Boström, E., Hajibabaei, M., and Ekrem, T. (2018). Environmental DNA filtration techniques affect recovered biodiversity. Sci. Rep. 8:4682. doi: 10.1038/s41598-018-23052-8
Makiola, A., Compson, Z. G., Baird, D. J., Barnes, M. A., Boerlijst, S. P., Bouchez, A., et al. (2020). Key questions for next-generation biomonitoring. Front. Environ. Sci. 7:197. doi: 10.3389/fenvs.2019.00197
Mangul, S., Martin, L. S., Eskin, E., and Blekhman, R. (2019). Improving the usability and archival stability of bioinformatics software. Genome Biol. 20:47. doi: 10.1186/s13059-019-1649-8
Marquina, D., Esparza-Salas, R., Roslin, T., and Ronquist, F. (2019). Establishing arthropod community composition using metabarcoding: surprising inconsistencies between soil samples and preservative ethanol and homogenate from Malaise trap catches. Mol. Ecol. Resour. 19, 1516–1530. doi: 10.1111/1755-0998.13071
Marshall, N. T., and Stepien, C. A. (2019). Invasion genetics from eDNA and thousands of larvae: a targeted metabarcoding assay that distinguishes species and population variation of zebra and quagga mussels. Ecol. Evol. 9, 3515–3538. doi: 10.1002/ece3.4985
Martin, M. (2011). Cutadapt removes adapter sequences from high-throughput sequencing reads. EMBnet. J. 17:10. doi: 10.14806/ej.17.1.200
Meiklejohn, K. A., Damaso, N., and Robertson, J. M. (2019). Assessment of BOLD and GenBank – Their accuracy and reliability for the identification of biological materials. PLoS ONE 14:e0217084. doi: 10.1371/journal.pone.0217084
Mengoni, A., Tatti, E., Decorosi, F., Viti, C., Bazzicalupo, M., and Giovannetti, L. (2005). Comparison of 16S rRNA and 16S rDNA T-RFLP approaches to study bacterial communities in soil microcosms treated with chromate as perturbing agent. Microb. Ecol. 50, 375–384. doi: 10.1007/s00248-004-0222-4
Merkel, D. (2014). Docker: lightweight linux containers for consistent development and deployment. Linex J. 239:1–5.
Morales-Castilla, I., Matias, M. G., Gravel, D., and Araújo, M. B. (2015). Inferring biotic interactions from proxies. Trends Ecol. Evol. 30, 347–356. doi: 10.1016/j.tree.2015.03.014
Moss, B., Hering, D., Green, A. J., Aidoud, A., Becares, E., Beklioglu, M., et al. (2009). Climate change and the future of freshwater biodiversity in Europe: a primer for policy-makers. Freshw. Rev. 2, 103–130. doi: 10.1608/FRJ-2.2.1
Murray, D. C., Coghlan, M. L., and Bunce, M. (2015). From benchtop to desktop: important considerations when designing amplicon sequencing workflows. PLoS ONE 10:e0124671. doi: 10.1371/journal.pone.0124671
Nerbonne, J. F., Ward, B., Ollila, A., Williams, M., and Vondracek, B. (2008). Effect of sampling protocol and volunteer bias when sampling for macroinvertebrates. J. North Am. Benthol. Soc. 27, 640–646. doi: 10.1899/07-101.1
Olson, N. D., Treangen, T. J., Hill, C. M., Cepeda-Espinoza, V., Ghurye, J., Koren, S., et al. (2019). Metagenomic assembly through the lens of validation: recent advances in assessing and improving the quality of genomes assembled from metagenomes. Brief. Bioinform. 20, 1140–1150. doi: 10.1093/bib/bbx098
Papadopoulou, A., Taberlet, P., and Zinger, L. (2015). Metagenome skimming for phylogenetic community ecology: a new era in biodiversity research. Mol. Ecol. 24, 3515–3517. doi: 10.1111/mec.13263
Parsons, K. M., Everett, M., Dahlheim, M., and Park, L. (2018). Water, water everywhere: environmental DNA can unlock population structure in elusive marine species. R. Soc. Open Sci. 5:180537. doi: 10.1098/rsos.180537
Paula, D. P., Linard, B., Crampton-Platt, A., Srivathsan, A., Timmermans, M. J. T. N., Sujii, E. R., et al. (2016). uncovering trophic interactions in arthropod predators through dna shotgun-sequencing of gut contents. PLoS ONE 11:e0161841. doi: 10.1371/journal.pone.0161841
Pearson, D. L., and Hawksworth, D. L. (1994). Selecting indicator taxa for the quantitative assessment of biodiversity. Philos. Trans. R. Soc. Lond. B. Biol. Sci. 345, 75–79. doi: 10.1098/rstb.1994.0088
Pilliod, D. S., Goldberg, C. S., Arkle, R. S., and Waits, L. P. (2014). Factors influencing detection of eDNA from a stream-dwelling amphibian. Mol. Ecol. Resour. 14, 109–116. doi: 10.1111/1755-0998.12159
Piñol, J., Senar, M. A., and Symondson, W. O. C. (2019). The choice of universal primers and the characteristics of the species mixture determine when DNA metabarcoding can be quantitative. Mol. Ecol. 28, 407–419. doi: 10.1111/mec.14776
Pornon, A., Andalo, C., Burrus, M., and Escaravage, N. (2017). DNA metabarcoding data unveils invisible pollination networks. Sci. Rep. 7:16828. doi: 10.1038/s41598-017-16785-5
Porter, T. M., and Hajibabaei, M. (2018a). Automated high throughput animal CO1 metabarcode classification. Sci. Rep. 8:4226. doi: 10.1038/s41598-018-22505-4
Porter, T. M., and Hajibabaei, M. (2018b). Over 2.5 million COI sequences in GenBank and growing. PLoS ONE 13:e0200177. doi: 10.1371/journal.pone.0200177
Porter, T. M., Shokralla, S., Baird, D., Golding, G. B., and Hajibabaei, M. (2016). Ribosomal DNA and plastid markers used to sample fungal and plant communities from wetland soils reveals complementary biotas. PLoS ONE 11:e0142759. doi: 10.1371/journal.pone.0142759
Purvis, A., Newbold, T., De Palma, A., Contu, S., Hill, S. L. L., Sanchez-Ortiz, K., et al. (2018). “Chapter five - modelling and projecting the response of local terrestrial biodiversity worldwide to land use and related pressures: the PREDICTS project,” in Advances in Ecological Research: Next Generation Biomonitoring: Part 1, eds D. A. Bohan, A. J. Dumbrell, G. Woodward, and M. Jackson (Amsterdam: Elsevier), 201–241.
Ram, S., and Sheth, J. (1989). Consumer resistance to innovations: the marketing problem and its solutions. J. Consum. Mark.6, 5–14. doi: 10.1108/EUM0000000002542
Ratnasingham, S., and Hebert, P. D. N. (2007). Bold: the barcode of life data system (http://www.barcodinglife.org). Mol. Ecol. Notes 7, 355–364. doi: 10.1111/j.1471-8286.2007.01678.x
Rees, H. C., Maddison, B. C., Middleditch, D. J., Patmore, J. R. M., and Gough, K. C. (2014). Review: the detection of aquatic animal species using environmental DNA – a review of eDNA as a survey tool in ecology. J. Appl. Ecol. 51, 1450–1459. doi: 10.1111/1365-2664.12306
Renshaw, M. A., Olds, B. P., Jerde, C. L., McVeigh, M. M., and Lodge, D. M. (2015). The room temperature preservation of filtered environmental DNA samples and assimilation into a phenol–chloroform–isoamyl alcohol DNA extraction. Mol. Ecol. Resour. 15, 168–176. doi: 10.1111/1755-0998.12281
Ritter, C. D., Häggqvist, S., Karlsson, D., Sääksjärvi, I. E., Muasya, A. M., Nilsson, R. H., et al. (2019). Biodiversity assessments in the 21st century: the potential of insect traps to complement environmental samples for estimating eukaryotic and prokaryotic diversity using high-throughput DNA metabarcoding. Genome 62, 147–159. doi: 10.1139/gen-2018-0096
Robinson, C. V., Porter, T. M., Wright, M. T. G., and Hajibabaei, M. (2020). Propylene glycol-based antifreeze as an effective preservative for DNA metabarcoding of benthic arthropods. Genomics. doi: 10.1101/2020.02.28.970475
Ruppert, K. M., Kline, R. J., and Rahman, M. S. (2019). Past, present, and future perspectives of environmental DNA (eDNA) metabarcoding: A systematic review in methods, monitoring, and applications of global eDNA. Glob. Ecol. Conserv. 17:e00547. doi: 10.1016/j.gecco.2019.e00547
Salter, I., Joensen, M., Kristiansen, R., Steingrund, P., and Vestergaard, P. (2019). Environmental DNA concentrations are correlated with regional biomass of Atlantic cod in oceanic waters. Commun. Biol. 2:461. doi: 10.1038/s42003-019-0696-8
Sansom, B. J., and Sassoubre, L. M. (2017). Environmental DNA (eDNA) shedding and decay rates to model freshwater mussel eDNA transport in a river. Environ. Sci. Technol. 51, 14244–14253. doi: 10.1021/acs.est.7b05199
Sard, N. M., Herbst, S. J., Nathan, L., Uhrig, G., Kanefsky, J., Robinson, J. D., et al. (2019). Comparison of fish detections, community diversity, and relative abundance using environmental DNA metabarcoding and traditional gears. Environ. DNA 1, 368–384. doi: 10.1002/edn3.38
Sayers, E. W., Cavanaugh, M., Clark, K., Ostell, J., Pruitt, K. D., and Karsch-Mizrachi, I. (2019). GenBank. Nucl. Acids Res. 47, D94–D99. doi: 10.1093/nar/gkz956
Schloss, P. D., Westcott, S. L., Ryabin, T., Hall, J. R., Hartmann, M., Hollister, E. B., et al. (2009). Introducing mothur: open-source, platform-independent, community-supported software for describing and comparing microbial communities. Appl. Environ. Microbiol. 75, 7537–7541. doi: 10.1128/AEM.01541-09
Schmidt, P.-A., Bálint, M., Greshake, B., Bandow, C., Römbke, J., and Schmitt, I. (2013). Illumina metabarcoding of a soil fungal community. Soil Biol. Biochem. 65, 128–132. doi: 10.1016/j.soilbio.2013.05.014
Secretariat of the Convention on Biological Diversity (2011). Nagoya Protocol on Access to Genetic Resources and the Fair and Equitable Sharing of Benefits Arising From Their Utilization to the Convention on Biological Diversity. Available online at: https://www.cbd.int/abs/doc/protocol/nagoya-protocol-en.pdf (accessed October 20, 2020).
Seersholm, F. V., Cole, T. L., Grealy, A., Rawlence, N. J., Greig, K., Knapp, M., et al. (2018). Subsistence practices, past biodiversity, and anthropogenic impacts revealed by New Zealand-wide ancient DNA survey. Proc. Natl. Acad. Sci. U.S.A. 115, 7771–7776. doi: 10.1073/pnas.1803573115
Shogren, A. J., Tank, J. L., Andruszkiewicz, E., Olds, B., Mahon, A. R., Jerde, C. L., et al. (2017). Controls on eDNA movement in streams: transport, retention, and resuspension. Sci. Rep. 7, 1–11. doi: 10.1038/s41598-017-05223-1
Sigsgaard, E. E., Nielsen, I. B., Bach, S. S., Lorenzen, E. D., Robinson, D. P., Knudsen, S. W., et al. (2016). Population characteristics of a large whale shark aggregation inferred from seawater environmental DNA. Nat. Ecol. Evol. 1, 1–5. doi: 10.1038/s41559-016-0004
Silva, L. P., Mata, V. A., Lopes, P. B., Pereira, P., Jarman, S. N., Lopes, R. J., et al. (2019). Advancing the integration of multi-marker metabarcoding data in dietary analysis of trophic generalists. Mol. Ecol. Resour. 19, 1420–1432. doi: 10.1111/1755-0998.13060
Singer, G., a, C, Fahner, N. A., Barnes, J. G., McCarthy, A., and Hajibabaei, M. (2019). Comprehensive biodiversity analysis via ultra-deep patterned flow cell technology: a case study of eDNA metabarcoding seawater. Sci. Rep. 9, 1–12. doi: 10.1038/s41598-019-42455-9
Slatko, B. E., Gardner, A. F., and Ausubel, F. M. (2018). Overview of next-generation sequencing technologies. Curr. Protoc. Mol. Biol. 122:e59. doi: 10.1002/cpmb.59
Sousa, L. L., de Silva, S. M., and Xavier, R. (2019). DNA metabarcoding in diet studies: unveiling ecological aspects in aquatic and terrestrial ecosystems. Environ. DNA 1, 199–214. doi: 10.1002/edn3.27
Souza, J. L. P., Baccaro, F. B., Landeiro, V. L., Franklin, E., Magnusson, W. E., Pequeno, P. A. C. L., et al. (2016). Taxonomic sufficiency and indicator taxa reduce sampling costs and increase monitoring effectiveness for ants. Divers. Distrib. 22, 111–122. doi: 10.1111/ddi.12371
Stein, E. D., Martinez, M. C., Stiles, S., Miller, P. E., and Zakharov, E. V. (2014). Is DNA Barcoding actually cheaper and faster than traditional morphological methods: results from a survey of freshwater bioassessment efforts in the United States? PLoS ONE 9:e095525. doi: 10.1371/journal.pone.0095525
Stoeckle, M. Y., Soboleva, L., and Charlop-Powers, Z. (2017). Aquatic environmental DNA detects seasonal fish abundance and habitat preference in an urban estuary. PLoS ONE 12:e0175186. doi: 10.1371/journal.pone.0175186
Turnbaugh, P. J., Hamady, M., Yatsunenko, T., Cantarel, B. L., Duncan, A., Ley, R. E., et al. (2009). A core gut microbiome in obese and lean twins. Nature 457, 480–484. doi: 10.1038/nature07540
Turon, X., Antich, A., Palacín, C., Præbel, K., and Wangensteen, O. S. (2020). From metabarcoding to metaphylogeography: separating the wheat from the chaff. Ecol. Appl. 30:e02036. doi: 10.1002/eap.2036
Vázquez, D. P., Melián, C. J., Williams, N. M., Blüthgen, N., Krasnov, B. R., and Poulin, R. (2007). Species abundance and asymmetric interaction strength in ecological networks. Oikos 116, 1120–1127. doi: 10.1111/j.0030-1299.2007.15828.x
Veletanlić, E., and Sá, C. (2019). Government programs for university–industry partnerships: logics, design, and implications for academic science. Res. Eval. 28, 109–122. doi: 10.1093/reseval/rvy034
Vernooy, R., Haribabu, E., Muller, M. R., Vogel, J. H., Hebert, P. D. N., Schindel, D. E., et al. (2010). Barcoding life to conserve biological diversity: beyond the taxonomic imperative. PLoS Biol. 8:e1000417. doi: 10.1371/journal.pbio.1000417
Wilkes, P., Lau, A., Disney, M., Calders, K., Burt, A., Gonzalez de Tanago, J., et al. (2017). Data acquisition considerations for terrestrial laser scanning of forest plots. Remote Sens. Environ. 196, 140–153. doi: 10.1016/j.rse.2017.04.030
Williams, K. E., Huyvaert, K. P., and Piaggio, A. J. (2016). No filters, no fridges: a method for preservation of water samples for eDNA analysis. BMC Res. Notes 9:298. doi: 10.1186/s13104-016-2104-5
Wood, S. A., Biessy, L., Latchford, J. L., Zaiko, A., von Ammon, U., Audrezet, F., et al. (2020). Release and degradation of environmental DNA and RNA in a marine system. Sci. Total Environ. 704:135314. doi: 10.1016/j.scitotenv.2019.135314
Woodward, G. (2009). Biodiversity, ecosystem functioning and food webs in fresh waters: assembling the jigsaw puzzle. Freshw. Biol. 54, 2171–2187. doi: 10.1111/j.1365-2427.2008.02081.x
Xu, Z., Malmer, D., Langille, M. G. I., Way, S. F., and Knight, R. (2014). Which is more important for classifying microbial communities: who's there or what they can do? ISME J. 8, 2357–2359. doi: 10.1038/ismej.2014.157
Yamahara, K. M., Preston, C. M., Birch, J., Walz, K., Marin, R. I., Jensen, S., et al. (2019). In situ autonomous acquisition and preservation of marine environmental dna using an autonomous underwater vehicle. Front. Mar. Sci. 6:373. doi: 10.3389/fmars.2019.00373
Yates, M. C., Fraser, D. J., and Derry, A. M. (2019). Meta-analysis supports further refinement of eDNA for monitoring aquatic species-specific abundance in nature. Environ. DNA 1, 5–13. doi: 10.1002/edn3.7
Zaiko, A., Pochon, X., Garcia-Vazquez, E., Olenin, S., and Wood, S. A. (2018). Advantages and limitations of environmental DNA/RNA tools for marine biosecurity: management and surveillance of non-indigenous species. Front. Mar. Sci. 5:322. doi: 10.3389/fmars.2018.00322
Zhang, J., Kapli, P., Pavlidis, P., and Stamatakis, A. (2013). A general species delimitation method with applications to phylogenetic placements. Bioinformatics 29, 2869–2876. doi: 10.1093/bioinformatics/btt499
Zhang, Y., Pavlovska, M., Stoica, E., Prekrasna, I., Yang, J., Slobodnik, J., et al. (2020). Holistic pelagic biodiversity monitoring of the black sea via eDNA metabarcoding approach: from bacteria to marine mammals. Environ. Int. 135:105307. doi: 10.1016/j.envint.2019.105307
Keywords: biodiversity, bioassessment, DNA metabarcoding, environmental genomics, high throughput sequencing, next generation sequencing
Citation: Compson ZG, McClenaghan B, Singer GAC, Fahner NA and Hajibabaei M (2020) Metabarcoding From Microbes to Mammals: Comprehensive Bioassessment on a Global Scale. Front. Ecol. Evol. 8:581835. doi: 10.3389/fevo.2020.581835
Received: 13 July 2020; Accepted: 07 October 2020;
Published: 30 November 2020.
Edited by:
Muniyandi Nagarajan, Central University of Kerala, IndiaReviewed by:
Michael Bunce, Curtin University, AustraliaChandika Damesh Gamage, University of Peradeniya, Sri Lanka
Copyright © 2020 Compson, McClenaghan, Singer, Fahner and Hajibabaei. This is an open-access article distributed under the terms of the Creative Commons Attribution License (CC BY). The use, distribution or reproduction in other forums is permitted, provided the original author(s) and the copyright owner(s) are credited and that the original publication in this journal is cited, in accordance with accepted academic practice. No use, distribution or reproduction is permitted which does not comply with these terms.
*Correspondence: Mehrdad Hajibabaei, aGFqaWJhYmFlaUBnbWFpbC5jb20=