- 1Department of Biological Sciences, Idaho State University, Pocatello, ID, United States
- 2Department of Biology, Duke University, Durham, NC, United States
- 3Economic Herbarium of Oakes Ames, Department of Organismic and Evolutionary Biology, Harvard University, Cambridge, MA, United States
- 4Department of Botany, Biodiversity Research Centre, University of British Columbia, Vancouver, BC, Canada
Invasive species represent excellent opportunities to study the evolutionary potential of traits important to success in novel environments. Although some ecologically important traits have been identified in invasive species, little is typically known about the genetic mechanisms that underlie invasion success in non-model species. Here, we use a genome-wide association (GWAS) approach to identify the genetic basis of trait variation in the non-model, invasive, diffuse knapweed [Centaurea diffusa Lam. (Asteraceae)]. To assist with this analysis, we have assembled the first draft genome reference and fully annotated plastome assembly for this species, and one of the first from this large, weedy, genus, which is of major ecological and economic importance. We collected phenotype data from 372 individuals from four native and four invasive populations of C. diffusa grown in a common environment. Using these individuals, we produced reduced-representation genotype-by-sequencing (GBS) libraries and identified 7,058 SNPs. We identify two SNPs associated with leaf width in these populations, a trait which significantly varies between native and invasive populations. In this rosette forming species, increased leaf width is a major component of increased biomass, a common trait in invasive plants correlated with increased fitness. Finally, we use annotations from Arabidopsis thaliana to identify 98 candidate genes that are near the associated SNPs and highlight several good candidates for leaf width variation.
Introduction
Research on rapid phenotypic change in contemporary time is at the forefront of modern investigations of evolution (Stapley et al., 2015; Hendry et al., 2017; Rodríguez-Verdugo et al., 2017). A detailed understanding of ecological and evolutionary responses to novel environments will improve predictions of how organisms are likely to respond to change in many contexts, including climate change, invasion, or agriculture (Rodríguez-Verdugo et al., 2017). Anthropogenically induced environmental change (such as climate, land use, and species introductions) may expand the opportunities for rapid evolution of increased invasiveness (Ricciardi et al., 2017). Both observational and experimental studies have documented adaptive changes in plants from the invaded range relative to conspecific native populations (Dlugosch and Parker, 2008; Felker-Quinn et al., 2013). Rates of adaptive phenotypic change are high in human-disturbed contexts (Hendry et al., 2008; Hufbauer et al., 2012), such as invasion, and more common in introduced relative to native species in the same environment (Buswell et al., 2011). Increased growth rate or reproductive capacity is frequently reported from field observations and common garden experiments of introduced populations (Elton, 1958; Hodgins and Rieseberg, 2011; Felker-Quinn et al., 2013; Kumschick et al., 2013; Parker et al., 2013). This improved fecundity could contribute to rapid spread and population growth in the invaded range, in other words, increased invasiveness.
Plant phenotypic traits determine how plants respond to abiotic and biotic environmental factors and reflect the outcome of evolutionary and ecological processes (Violle et al., 2007). Individual plant traits affect fitness in a given environment, and particularly in the case of invasive species, can have major impacts on ecosystem properties (Kattge et al., 2020). Which functional traits are important for invasion success may be context dependent and vary among invasive species. Important functional traits may change over the progression of the invasion (Dietz and Edwards, 2006; Catford et al., 2019; Galland et al., 2019) and may depend on the habitats invaded (Lachmuth et al., 2011), the traits of species in the invaded community (Roscher et al., 2018), or the trait diversity of other conspecific invaders (Turner et al., 2020a). Most traits relevant for predictive ecological or evolutionary modeling of invasions require data on continuous intraspecific variation and trait-environmental relationships; these traits have to be measured on individual plants in their respective environments (Kattge et al., 2020), and thus are likely to be correlated with individual variation at the genetic level. The complexity of the context dependency in invasion success thus highlights the need to incorporate molecular data into our ecological understanding of invasions (Galland et al., 2019).
Although some ecologically important traits have been identified in invasive species (for example specific leaf area (SLA) or carbon capture strategy, see Catford et al., 2019), little is known about the possible genomic mechanisms that underlie invasion success. With a genomic trait mapping approach, we may be able to understand the genetic mechanisms underlying a species’ capacity for the evolution of complex phenotypes, such as increased invasiveness, in “wild” populations (Santure and Garant, 2018). By correlating marker variants with trait variation using association analyses, large-scale genotyping and phenotyping of individuals from native and invasive populations can allow us to identify genomic regions that contribute to phenotypic differences among individuals and between ranges. This approach may enable us to better understand the sources of adaptive genetic variation that fuel invasions and predict the ability of populations to adapt to challenges, such as novel environments. This understanding may both contribute to our ability to mitigate or prevent invasions, and to our understanding of rapid evolution in other contexts, such as adaptive potential in the face of climate change. Much recent work has gone into understanding the processes and impacts of rapid evolution in novel environments. Understanding these processes is important in many contexts, including invasion, adaptation to climate change, and agricultural breeding for drought tolerance. Our work investigates the genetic mechanisms underlying traits that vary between the native and invasive range of a wide-spread, problematic invasive species. The source of variation between native and invasive populations of the same species is a standing question. Do species rapidly adapt (in this case, in about ∼100 generations) to be more invasive? Does trait variation spring from novel mutation in the new range, or standing genetic variation?
To identify the genetic mechanisms underlying the rapid evolution of invasiveness, genomic tools and analyses are necessary (Stewart et al., 2009). Genome-scale transcriptional profiling has been used to identify loci that are differentially expressed between native and invasive genotypes for a handful of weedy or invasive species, and suggest hypotheses to explain phenotypic variation, physiological trade-offs, and the origin of diversity, biological novelty, and adaptation (Whitehead and Crawford, 2006; Lai et al., 2008; Guggisberg et al., 2013; Hodgins et al., 2013). When an adaptive phenotype has been identified, trait mapping is the most straightforward way to identify the loci underlying adaptation (Flood and Hancock, 2017). Here, we seek to identify genetic variation underlying trait evolution that may explain invasion success in the non-model, invasive, diffuse knapweed [Centaurea diffusa Lam. (Asteraceae)]. We use a genome-wide association (GWAS) approach to identify the genetic basis of traits that vary between the native and invasive ranges of this species. To assist with this analysis, we have assembled the first draft genome reference and annotated plastome assembly for this species. These are some of the first assemblies for Centaurea, which is a large and weedy genus of major ecological and economic importance (see also C. stoebe subsp. micranthos plastome; Park et al., 2019). We then use these resources to identify genes associated with traits that vary among individuals after being raised in a common environment for two generations to reduce maternal effects, with particular focus on traits that vary between the native and invasive ranges. We further investigate trait associations with genes that have been previously identified as being differentially expressed between the ranges, and thus may contribute to the complex phenotype of increased invasiveness.
Materials and Methods
Study System
The Asteraceae is one of the largest angiosperm families and includes many well-known weedy and invasive species, such as thistles, dandelions, and ragweed. The Centaurea genus (knapweeds, star thistles), containing approximately 250 species (Susanna and Garcia-Jacas, 2009), comprises the most abundant noxious weed genus in the western United States, and is one of only 15 plant genera in the United States that is significantly more likely to contain weedy species than expected by chance (Lejeune and Seastedt, 2001; Kuester et al., 2014). The five invasive Centaurea species with the greatest impact, including C. diffusa, have invaded millions of hectares of grassland, making it the most abundant noxious weed genus in the western United States (Lejeune and Seastedt, 2001). Knapweed invasions can form dense monocultures that reduce quality of forage for livestock and wildlife and alter resource availability (Sheley and Larson, 1996).
Centaurea diffusa is a monocarpic annual or biennial which grows as a rosette, and bolts before flowering (Thompson and Stout, 1991). This species is diploid with a moderately sized genome (2n = 16, 1C = 882 Mbp; Bancheva and Greilhuber, 2006). Native to parts of eastern Europe and western Asia, C. diffusa is considered a naturalized alien throughout western Europe (Greuter, 2009). In the century since C. diffusa was first reported in North America, it has invaded rangeland habitats to form dense monocultures, reduced forage quality, and altered soil and water resource availability in invaded grasslands (Lejeune and Seastedt, 2001). Previous work (Turner et al., 2014) has demonstrated the rapid evolution of C. diffusa in the invaded range under an array of benign and stressful conditions, including drought. Invasive individuals grew larger, tolerated stressful conditions as well or better, or matured later than native individuals across treatment conditions. Additionally, invasive individuals may have been released from a trade-off between growth and drought tolerance apparent in the native range (Turner et al., 2015).
Draft Reference Genome Assembly
Seed was collected from an individual in the native range of C. diffusa (TR001-1; Supplementary Table S1). Seed from this collection was grown in a greenhouse at the University of British Columbia in 2009. Young leaf tissue was sampled from one progeny (TR001-1L) and stored at −80°C to be used for draft reference genome assembly. DNA was extracted from frozen tissue using a modified Qiagen DNeasy column-less protocol (Qiagen, Valencia, CA, United States). Concentration and quality were verified by Nanodrop, Qubit 2.0 Fluorometer (Thermo Fisher Scientific, Waltham, MA, United States), and gel electrophoresis. A whole genome shotgun library for this individual was produced and sequenced using Illumina HiSeq 2000 100 bp paired-end sequencing (Genome Quebec). This produced ∼69 million reads and ∼14 Gbp, for a sequencing depth of ∼16X. Raw reads were quality trimmed and screened for sequencing artifacts using Trimmomatic (Bolger et al., 2014).
To produce the plastome assembly, clean reads were aligned to the Lactuca sativa plastome (Timme et al., 2007) using BWA (Li and Durbin, 2009). Pairs in which both reads aligned to the L. sativa plastome were extracted from the SAM files with Picard Tools SamToFastq.jar (Broad Institute, 2009). ALLPATHS-LG (Gnerre et al., 2011) was used to merge overlapping pairs and error-correct the data, which was then assembled with Ray (Boisvert et al., 2010). Ray contigs were aligned to the L. sativa plastome with BLAST (Altschul et al., 1990) and scaffolded based on synteny using OSLay (Richter et al., 2007). Gaps were filled with GapFiller (Boetzer and Pirovano, 2012) resulting in a sequence containing a single “N.” Visual inspection indicated that the “N” separated an erroneous tandem duplication, which was corrected by hand with Vim. The boundaries of the inverted repeat (IR) region were confirmed with aTRAM (Allen et al., 2015) assemblies of flanking regions. Reads were aligned to the assembly with BWA and sorted with SAMTOOLS (Li et al., 2009). Visual inspection of the alignment revealed a few small indel and substitution errors, which were hand-corrected with Vim. A final alignment and inspection revealed no errors. The plastome was annotated using DOGMA (Wyman et al., 2004) and validated for NCBI GenBank submission using Sequin 13.05. We used the blastn program (Altschul et al., 1997) to compare the gene content and sequence identity of the C. diffusa and Cynara cardunculus (GenBank: KP842711.1; Curci et al., 2016) plastid genomes. In order to calculate dN, dS, and dN/dS in protein coding regions, we made multiple alignments of the amino acid sequences using Muscle v3.8.31 (Edgar, 2004) and back translated them to codons (Grassa and Kulathinal, 2011). Lactuca sativa plastid genes (GenBank: NC_007578.1; Kanamoto et al., 2004) were also included in the analysis of protein-coding regions. We used the yn00 method (Yang and Nielsen, 2000) in PAML v4.8a (Yang, 2007) to calculate dN, dS, and dN/dS values on the aligned codons.
An additional assembly was made to target the nuclear genome albeit at low depth. Unfiltered trimmed reads were assembled with Megahit (Li et al., 2015). Megahit contigs were repeat masked using Red (Girgis, 2015) and aligned to the Globe Artichoke reference genome (Scaglione et al., 2016) using Blastn (Altschul et al., 1990). These alignments were used as input to Chromosomer (Tamazian et al., 2016), which scaffolds contigs based on the order of homologous regions in a more completely assembled reference genome. Unplaced Megahit contigs were added to the output of Chromosomer to make a reference nuclear sequence. Finally, we polished the pseudomolecules with six iterations of Pilon (Walker et al., 2014) to fix local errors.
The reference nuclear sequence was annotated for putative protein-coding genes using three types of evidence: ab initio Hidden Markov Model-based predictions, protein homology, and expressed sequence tags. Ab initio predictions were made using Augustus (Stanke et al., 2006) with the gene model trained for tomato (the phylogenetically closest model available at the time of analysis). Viridiplantae proteins were downloaded from RefSeq (O’Leary et al., 2016) and clustered at 90% identity with CD-HIT (Fu et al., 2012). Representative peptide sequences were first aligned to the nuclear reference with Diamond (Buchfink et al., 2015) (in sensitive mode) to quickly identify candidate regions with sequence homology to known proteins. Peptides were realigned to the nuclear sequence at candidate loci using the more sensitive, but longer running, AAT (Huang et al., 1997). Assembled ESTs from a native (TR001-1L; the same individual used for this reference assembly) and invasive (US022-31E) genotype (Lai et al., 2012) were aligned with GMAP (Wu et al., 2016). The three lines of evidence were combined using Evidence Modeler (Haas et al., 2008) to generate the gene coordinates.
Genome-Wide Association Study
Seeds were collected as part of a broad collaborative effort from four native range and four invaded range C. diffusa populations (Figure 1 and Supplementary Table S1). Field collection took place between 2006 and 2008. Because maternal environmental effects can have strong, even adaptive, impacts on offspring phenotype in some systems (Galloway, 2005), we used individuals grown for two generations in a common environment to reduce maternal effects in this study. Specifically, field collected seed from these populations were raised in a greenhouse and crossed within populations (as previously reported in Turner et al., 2014). The seeds produced by those crosses were raised in a benign control environment, provided with ample resources, and phenotyped (as reported in Turner et al., 2014). Briefly, the 381 C. diffusa individuals included in this study were phenotyped as follows: Basal leaf number and length and width of the longest basal leaf for each individual were assessed three times during the experiment: beginning 7 weeks after germination (at the mean 15 leaf stage), 10 weeks after germination, and at harvest, and the maximum value was noted. At the end of the experiment, 19–20 weeks after germination, the diameter at the interface between root and stem was assessed. In addition, two or three young leaves were sampled for DNA extraction and stored at −80°C, and one mature leaf was harvested to measure SLA. Leaves for SLA were scanned and area measured using ImageJ 1.45s (Rasband, 2011) then dried at 29°C and weighed. The rest of the above ground biomass was harvested, dried, and weighed. These phenotypes were summarized into the following values for use in the GWAS analysis: (1) maximum leaf count, (2) maximum leaf width, (3) maximum leaf length, (4) root crown diameter, (5) shoot mass, and (6) SLA.
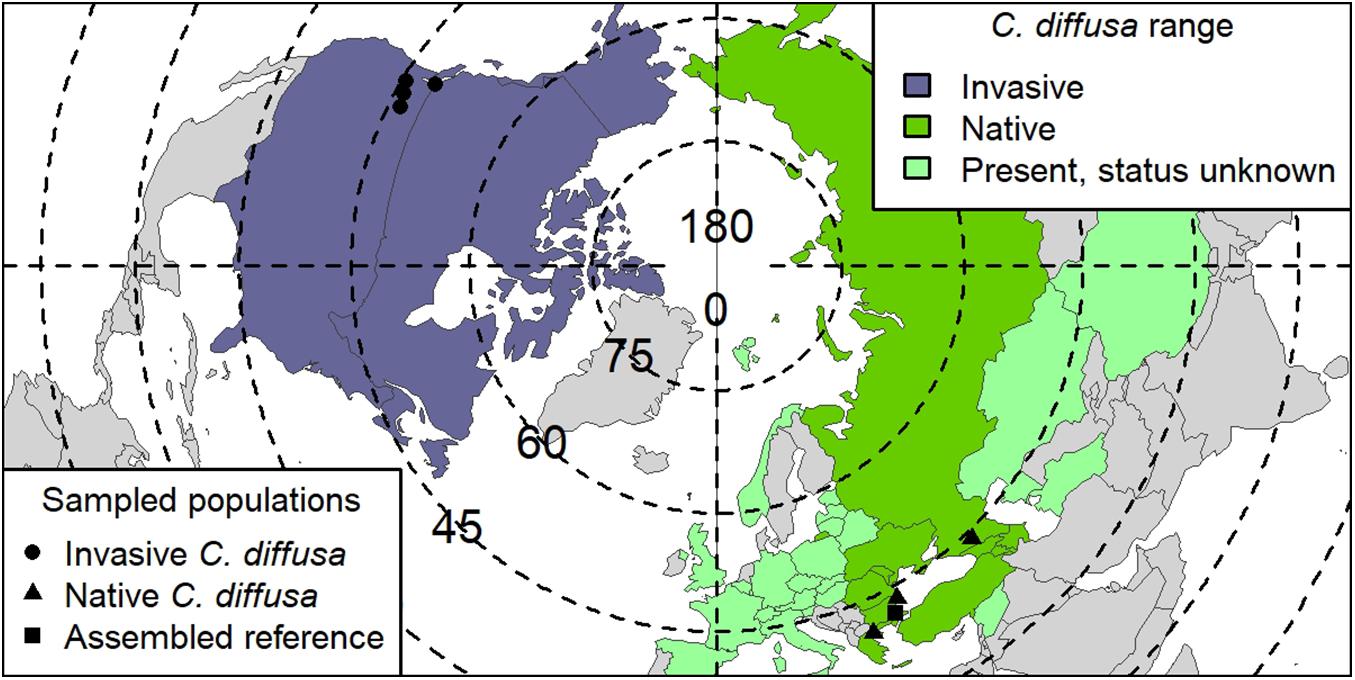
Figure 1. Centaurea diffusa range map. Global range of C. diffusa, by country (modified from Turner et al., 2014). Range status in a particular country is indicated by color. “Present, status unknown” also includes countries where C. diffusa is considered naturalized. Points indicate seed collection locations used in GWAS study. One native population (TR001, Turkey; Supplementary Table S1) was used for both the reference assembly and as a population in GWAS. Degrees of latitude are indicated on dotted lines, and degrees of longitude, solid lines.
To genotype individuals, DNA was extracted from young leaves using a Qiagen DNeasy 96 Plant Kit (Qiagen, Valencia, CA, United States) and assessed for quantity using a Qubit 2.0 Fluorometer (Thermo Fisher Scientific, Waltham, MA, United States). High quality DNA from 381 individuals was used to produce PstI-MspI genotype-by-sequencing (GBS) libraries using a modified version of the protocol described in Poland et al. (2012). Briefly, we treated genomic DNA with HF-PstI and MspI at 37°C for 5 h. We ligated barcoded adaptors and barcoded common Y-shaped adaptors (Poland et al., 2012) to digested DNA at 22°C for 3 h. We pooled groups of 96 ligated samples, which were then purified using the Agencourt AMPure XP system and amplified by PCR using KAPPA HiFi Hotstart master mix. Finally, we selected DNA fragments between 300 and 500 bp using the Agencourt AMPure XP system for paired-end 100 bp sequencing on an Illumina HiSeq 2000 platform. Sequencing runs resulted in ∼402 million reads passing initial quality control at the sequencing facility, with an average quality score of 36.
Raw sequencing reads were de-multiplexed and data for each individual from all lanes were concatenated. Single nucleotide polymorphisms were called against the assembled reference sequence using dDocent v2.6.0 (Puritz et al., 2014). dDocent combines several existing software packages into a single pipeline designed specifically for paired-end GBS/RAD data and takes advantage of both forward and reverse reads for SNP discovery. SNPs were called based on a larger set of Centaurea individuals, including the 381 GWAS set and 54 individuals from additional populations. Then, using the individuals in this GWAS study only, SNPs were filtered for quality and missing data. The resulting variant call file (VCF) was filtered using vcftools v0.1.15 (Danecek et al., 2011) to a minimum quality score of 30 and a minor allele frequency (MAF) of 5%, retaining individuals with no more than 40% missing data, and sites missing in no more than 10% of individuals with a minimum mean depth value greater than 20. Complex variants (multinucleotide polymorphisms and composite insertions and substitutions) were decomposed into SNP and indel representation following Puritz et al. (2014), retaining only one biallelic SNP per locus. Further filtering steps were performed to remove SNPs likely to be the result of sequencing errors, paralogs, multicopy loci or artifacts of library preparation (Supplementary Methods S1). We concatenated SNPs found on unplaced scaffolds onto a single contig for analysis, and, because most GWAS analyses do not tolerate missing data, we replaced unknown genotypes with heterozygous calls. Using only SNPs without any missing data substantially decreases the number of SNPs that we could test but did not affect our results. The final data set used in downstream analyses consisted of 7,058 SNPs found in 372 individuals from eight populations represented by 6–169 individuals (Figure 1 and Supplementary Table S1).
We used compressed mixed linear models (Zhang et al., 2010) implemented in GAPIT (Lipka et al., 2012) to test for associations between SNPs and our phenotypes. To avoid spurious associations and account for relatedness between our individuals, our models included a kinship matrix, which was calculated using the default settings for the VanRaden (2008) algorithm. This kinship matrix was validated against the known pedigree for these individuals from the greenhouse crossing design, and our results were not affected by using alternative ways to account for relatedness. To further account for population structure in our population, we used the Bayesian information criterion (BIC)-based model selection procedure implemented in GAPIT to determine the optimal number of principal components to include in our models (up to a maximum of 30) in addition to the calculated kinship matrix.
We searched for genes near significant GWAS hits to gain insight as to what the genetic basis of these traits might be. For each significant GWAS hit we focused on genes located within 500 kb in both the upstream and downstream direction; this marked the boundaries for the gene search. Genes identified in this way were functionally annotated based on sequence similarity to Arabidopsis thaliana using all-against-all BLASTP reciprocal best hits to ESTs in the TAIR 10 database (Berardini et al., 2015; TAIR, 2020). These TAIR 10 loci and associated Gene Ontology terms are reported.
Finally, we put these results in the context of previous work on invasive candidate traits in this system. We determined whether significant GWAS hits were associated with traits that significantly varied between individuals from the native and invasive ranges in previous studies (Turner et al., 2014) and may be candidate invasiveness traits. We used linear mixed effect models in the R package lme4 (R version 3.5; Bates et al., 2014; R Core Team, 2018) and Fisher’s exact tests to interrogate the relationships between range (native or invasive), genotypes, and traits. We further assessed overlap between SNPs and genes identified here and previous transcriptomic and gene expression work in this system (Lai et al., 2012; Hodgins et al., 2015; Turner et al., 2017).
Results
Draft Genome Reference Assembly
The fully annotated C. diffusa plastid genome is 152,559 bp in length (Figure 2, produced using OGDraw, Lohse et al., 2013; see also Supplementary Figures S1–S3 and Supplementary Table S2; GenBank: KJ690264). It contains a pair of inverted repeats 25,238 bp in length that differ by 2 substitutions. The repeats are separated by a large single copy region 83,596 bp in length and a small single copy region 18,487 bp in length. The G + C content of the whole genome is 38%. There are 82 unique protein-coding genes, including two open reading frames of unknown function. Nine full-length protein coding genes are duplicated within the IR, as is the second exon of rps12. There are 29 unique tRNA genes, seven of which are duplicated in the IR. All four rRNA are duplicated within the IR. We compared the C. diffusa plastid genome to that of Cynara cardunculus (GenBank: KP842711.1; Curci et al., 2016) and found it very similar (Length: 152,462, LSC: 83,541, IRa&IRb: 25,155, SSC:18,611), except that the gene orders are reversed within the SSC. The sequence identities for the two genomes across the LSC, IRa, SSC, and IRb are: 97.69, 99.24, 96.44, and 99.25%, respectively. Gene content is nearly identical. Both genomes share all of their protein coding genes. The C. diffusa plastid genome is missing a trnE-UUC that is present in the Cyn. cardunculus genome. When possible, we calculated pairwise dN, dS, and dN/dS between Cyn. cardunculus and C. diffusa for 83 plastid genes (Supplementary Table S3). Eighteen genes had zero synonymous changes and 30 genes had zero non-synonymous changes. Mean values (and standard deviations) for dN, dS, and dN/dS are: 0.03 (0.23), 0.05 (0.26), and 0.25 (0.29), respectively. As expected, the very low dN/dS suggests very strong purifying selection throughout most of the plastid genome. Three genes have 0.9 > dN/dS > 1.1: accD, and both copies of ycf2. One gene has dN/dS > 1.1: petA. A simple molecular clock estimate (Wolfe et al., 1987) suggests the Cynara and Centaurea lineages diverged 35.4 ± 17.7 MYA.
The draft nuclear assembly is made up of 431,654 contigs spanning 432,640,212 bp with an N50 of 1,482 bp. Minimum contig size is 200 bp, the maximum is 38,144 bp, and the mean is 1,002 bp. This represents approximately 49% of the genome size based on a previous estimate (1C = 880 Mbp, using Feulgen DNA image densitometry; Bancheva and Greilhuber, 2006). 44% of our assembly is estimated to be repetitive, as modeled by Red (Girgis, 2015). We annotated 47,402 putative protein coding genes based on multiple lines of evidence. 33,957 of these start with a methionine and end with a stop codon (counted as complete). Mean protein length is 346 amino acids. Mean exon number is 4.45 with 13,736 genes made up of a single exon. We scaffolded 226,408 contigs and 224,691,925 bp of the draft assembly against the globe artichoke genome (Cynara cardunculus var. scolymus, Asteraceae; Scaglione et al., 2016) based on homology to build pseudomolecules. The pseudomolecule sequence includes 55% of the non-repetitive portion of the genome assembly and 24,342 annotated genes.
Genome-Wide Association Study
The BIC-model selection procedure implemented in GAPIT showed that including principal components in any of our models did not significantly improve fit. Therefore, we present results based on models using only kinship as a covariate but note that including the first three principle components in our model fit does not change any of our results. These models did not find any significant associations with the following traits in this dataset: shoot mass, root crown diameter, maximum leaf count and SLA (Supplementary Figure S4). Using less stringent parameters and thresholds during SNP filtering does not change these results.
However, we did find a single region, made up of two markers, that was significantly associated with maximum leaf width. These two markers are clear outliers in Manhattan and Q-Q plots (Figure 3 and Table 1). As in previous analyses of this phenotypic data (Turner et al., 2014), range (native or invasive) has a significant effect on maximum leaf width (F1 = 15.1, p < 0.001, Figure 4). As expected, genotype at the marker with the highest association to maximum leaf width in our GWAS (TR001.Ccrd12_11392699) has a significant effect on maximum leaf width (F2 = 33.3, p < 0.0001). There are significantly more alleles associated with wider leaves in the invasive populations (Fisher’s exact test, p < 0.0001). Both of these significant SNPs align uniquely to the reference and both align to the same putative chromosome and are within 150 base pairs of each other (TR001.Ccrd12_11392699, TR001.Ccrd12_11392552; Table 2). Ninety-eight genes were identified within the region surrounding the GWAS hits, bordered by non-significant SNPs, and are functionally annotated based on similarity to Arabidopsis thaliana (Supplementary Table S4).
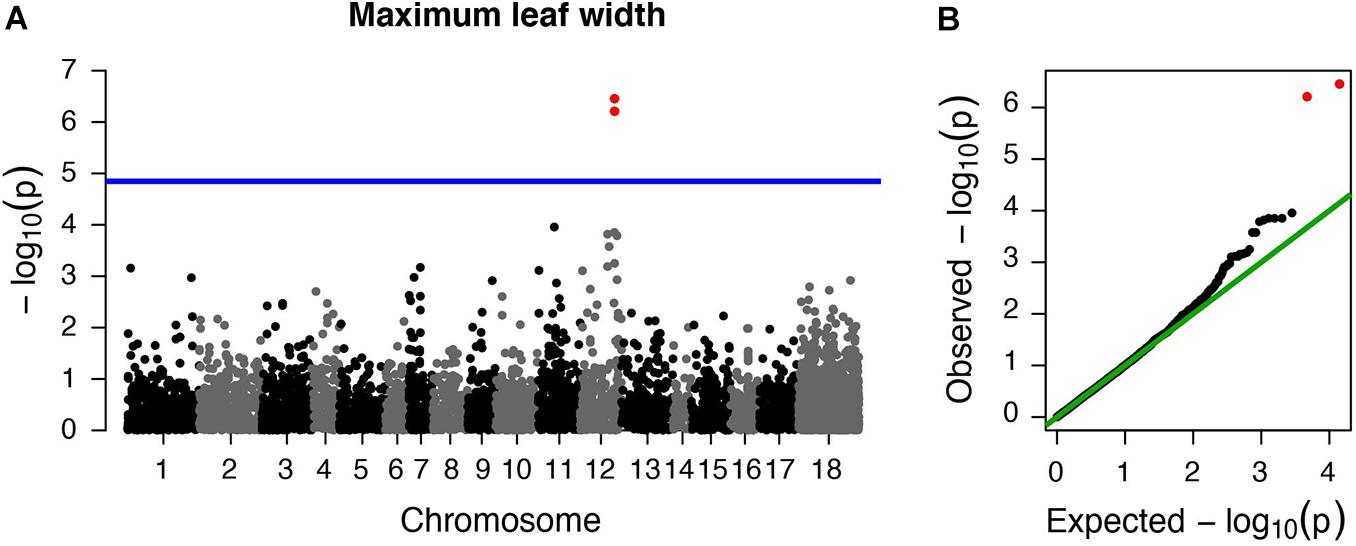
Figure 3. Centaurea diffusa GWAS Manhattan and QQ plots for one trait with significant associations. Manhattan and QQ plots for maximum leaf width. (A) The horizontal blue line represents a p-value threshold of 0.05 after accounting for Bonferroni multiple test correction. (B) QQ plot showing the expected –log10 p-values plotted against the observed –log10 p-values. Red points denote the two markers that exceed our significance threshold.
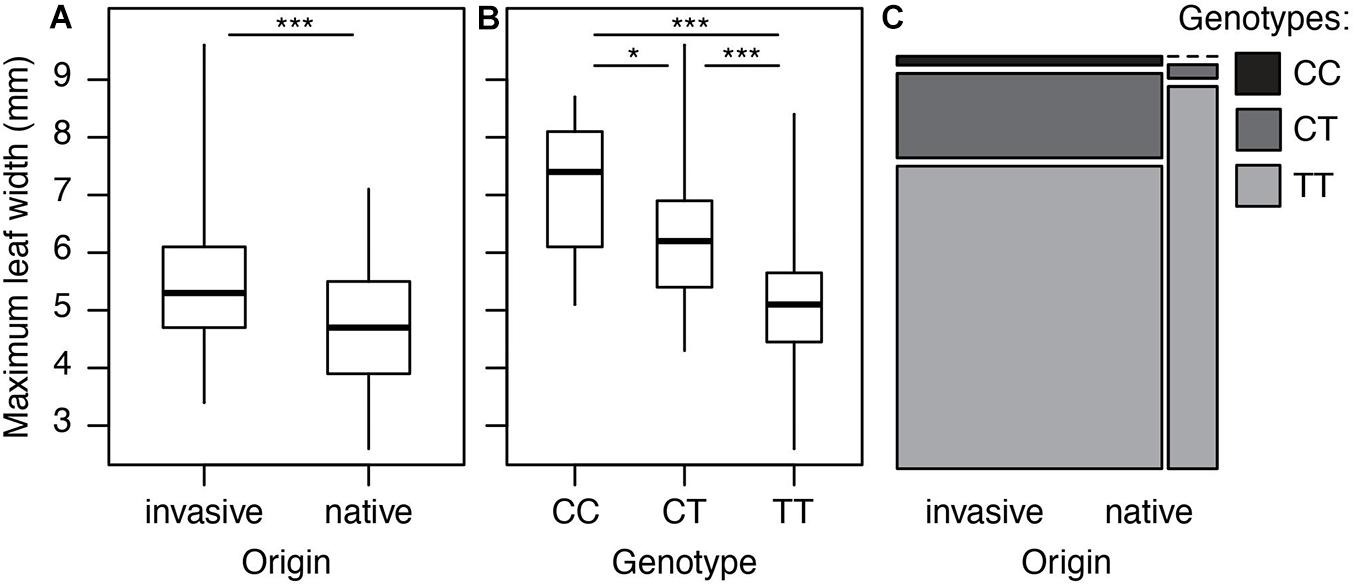
Figure 4. Leaf width and associated genotypes in native and invasive populations of Centaurea diffusa. Distribution of leaf width and associated allele across native and invasive populations. (A) Maximum leaf width of native and invasive plants. (B) The effect of genotype at position TR001.Ccrd12_11392699 on maximum leaf width. All pairwise differences are significant according to a Tukey test (CC vs. CT: p = 0.03, CC vs. TT: p < 0.0001, CT vs. TT: p < 0.0001). (C) Mosaic plot showing the frequency of genotypes at position TR001.Ccrd12_11392699 in native and invasive populations. The width of each bar is proportional to the sample size. *p < 0.05, ***p < 0.00.
Neither of the two significant SNPs identified in this study mapped directly to a previously produced transcriptome of the same individual we used for our reference assembly (Lai et al., 2012). Because of this, they could not be directly identified in a previous analysis as rapidly evolving in invasive C. diffusa (Hodgins et al., 2015). Nor could they be directly identified as differentially expressed among nearly the same set of native and invasive populations in benign or drought stressed environments using a transcriptome-based microarray (Turner et al., 2017). However, other segments of the gene containing the significant GWAS SNPs (TR001.Ccrd12.1184) were included in the ESTs used in these previous studies and so that gene was included in these previous analyses. Additionally, 42 other sites included in the 114 gene genomic region associated with maximum leaf width in this study were identified in these previous studies (see Supplementary Table S4 for full list). Of the 114 genes annotated in this region, 1 gene had a marginal effect of native/invasive range on expression, 19 genes were differentially expressed between control and drought treatments, and 20 were not differentially expressed (Turner et al., 2017). Two additional genes were identified by Hodgins et al. (2015) as rapidly evolving among weedy species of the Carduoideae subfamily (which includes C. diffusa) using stochastic branch-site models for positive selection.
Discussion
This study provides one of the first chloroplast genomes and the first draft whole genome reference for this large and economically important genus. It also represents the first efforts at mapping the genes underlying phenotypic differences between native and invasive populations in this species, such as leaf and root characteristics, and above ground biomass. The assembly work presented here demonstrates the utility of even imperfect reference assemblies in functional studies of non-model organisms. The plastome assembly is complete, annotated, and has already been used in several studies of related species (e.g., Rius et al., 2015; Salih et al., 2017; Lee-Yaw et al., 2019; pre-print published as Turner and Grassa, 2014a). Though the nuclear assembly represents only about 49% of the genome, it was boot strapped from relatively little data, and contigs assembled from only about ∼16X coverage with short reads. We were then able to scaffold these contigs against a well-studied agricultural relative, globe artichoke (Scaglione et al., 2016). The chromosomal positions reported here, should, however, be interpreted with caution due to the high evolutionary rate of structural change known to exist in the Asteraceae (Burke et al., 2004; Badouin et al., 2017; Ostevik et al., 2020). Even given these caveats, we were able to anchor 51% of genes and 75% of GBS markers to pseudomolecules. This allowed us to synthesize these GWAS results with those of previous transcriptome-based studies (Supplementary Discussion S1).
Relative to cross-based mapping strategies, GWAS provides greater resolution due to increased opportunity for recombination. However, population structure and relatedness can confound causative variation (Korte and Farlow, 2013). To address this, we included a kinship matrix in our models. We also interrogated whether including principal components would be helpful, which it was not. This was surprising given that there is clear structure in our samples (Supplementary Figure S5). However, relatedness and population structure are highly correlated across these individuals, likely accounting for the lack of additional explanatory power when including population structure in the model. Due to the uncertainty in disentangling true associations from spurious ones, it would be best to validate the association between position TR001.Ccrd12_11392699 and maximum leaf width in an independent study despite its robustness. On the other hand, GWAS may also fail to detect true associations. This can be especially problematic in cases like this where correcting for extensive relatedness and population structure can mute the signal of associations. Furthermore, associations due to complex genetic interactions will also likely be missed (for example, epistasis; Rowe et al., 2008). Using a reduced representation approach for genotyping also means that we are very unlikely to capture all the associated loci.
Variation in leaf width, which we successfully mapped using this GWAS approach, has been previously identified as varying between the native and invasive ranges, suggesting it might be an important target of selection in the novel habitat. The alleles at the two identified GWAS hits confer larger leaves and are more often found in invasive populations, at least among those sampled here. This implies the evolution of increased leaf width in invasive C. diffusa populations relied mainly on standing genetic variation, which adds to a growing body of studies suggesting that adaptation in introduced populations is largely fueled by pre-existing variation rather than de novo mutations (reviewed in Bock et al., 2015). A caveat is that we cannot rule out the possibility that new mutations contributed as well, but that we failed to detect them.
Leaf width is an important component of biomass in this rosette forming species and is one of the main axes upon which selection can act to increase biomass. Although biomass could not be directly associated with any underlying genetic mechanisms in this study, increased biomass is associated with invasive populations of this, and many other species (e.g., Blumenthal and Hufbauer, 2007). Under benign common conditions, across several common gardens, invasive C. diffusa individuals have demonstrated greater growth rate (including leaf size, basal leaf number, and shoot biomass) relative to native individuals (Turner et al., 2014, 2015). This previous work also indicated a positive relationship between size and seed production/fitness, a common pattern found in other monocarpic species (reviewed in Metcalf et al., 2003). However, biomass and growth rate are hugely polygenic traits (de Lima et al., 2017; D’Esposito et al., 2019; Wieters et al., 2020). GWAS often fail to identify markers for very polygenic traits like biomass even with whole genome sequence data (Korte and Farlow, 2013). It may therefore be more likely for analyses such as GWAS to identify genes associated with traits which contribute to size but may have simpler underlying genetic mechanisms, like leaf width. The genetic basis underlying leaf shape variation has been investigated in only a small number of species [including grape (Chitwood et al., 2014), tomato (Chitwood et al., 2013), and sweet potato (Gupta et al., 2020)], but most extensively in Arabidopsis thaliana. In Arabidopsis, leaf length and width are controlled independently; one major pathway, ANGUSTIFOLIA3 (AN3)—GROWTH REGULATING FACTOR5 (GRF5), has been identified to control width via regulation of cell shape or number (Tsukaya, 2018). Though more work is necessary, relatively few genes have been associated with leaf width variation in dicots (19 genes in dicots associated with “leaf size”, 2 genes associated with “leaf width”; UniProtKB, 2019).
GWAS identified two SNPs that are associated with leaf width in C. diffusa after controlling for kinship and population structure and correcting for multiple comparisons. Alleles associated with wider maximum leaf widths are more common in invasive populations in this study. Interestingly both SNPs map to a single genomic region in our reference assembly, and that region is functionally annotated as the gene APS reductase 1 [APR1 (AT4G04610); Table 3]. APR1 is involved in the oxidation-reduction process (Bick et al., 1998), sulfate assimilation and reduction (Setya et al., 1996), and has inferred involvement with cell redox homeostasis and the cystine biosynthetic process (Lamesch et al., 2012; TAIR, 2020). Native and invasive populations vary in drought tolerance (Turner et al., 2014), and APR1 was also found to be differentially expressed between control and drought treatments of this species (Table 3; Turner et al., 2017). However, this gene has not been previously associated with variation in leaf width.
Expanding potential gene candidates to the genomic region within 500 kb of SNPs significant after FDR correction identified 113 additional genes which may contribute to variation in leaf width (Table 3, Supplementary Table S4, and Supplementary Discussion S1). This includes genes involved with cell wall biogenesis [LRX5 (AT4G18670), ANAC073 (AT4G28500)], intra-cellular transport and polar growth [COG5 (AT1G67930), ATVPS54 (AT4G19490)], and meristem growth [MGP (AT1G03840); Berardini et al., 2015; TAIR, 2020]. This region also contains a homeodomain-like superfamily protein (AT2G40260). Genes containing homeobox domains have been associated with leaf dissection in several species [for example SHOOT MERISTEMLESS (STM) in Arabidopsis (Piazza et al., 2010) and other examples discussed in Gupta et al., 2020]. Additionally, the gene KNOTTED-LIKE FROM ARABIDOPSIS THALIANA2 (KNAT2) (AT1G70510) is involved with both meristem function and response to ethylene.
This region includes two particularly promising gene candidates underlying variation in leaf width in C. diffusa. bHLH30 (AT1G68810) is a basic helix-loop-helix DNA-binding transcription factor involved in transcription regulation (Table 3; Berardini et al., 2015; TAIR, 2020). Other transcription factors in this family are involved in many biological processes, including embryo growth, root development, stomatal development, iron uptake, modulating responses to absisic and gibberellic acid, and epidermal cell fate specification in leaves (UniProtKB, 2019). In particular, bHLH transcription factor SPATULA can act as a leaf size regulator by restricting the size of the meristematic region in leaf primordia independent of the leaf size regulating pathway AN3-GRF5 (Ichihashi et al., 2010). It may be that bHLH30 has a similar function in C. diffusa. bHLH30 was differentially expressed under drought vs. control condition is previous work in C. diffusa (Turner et al., 2017). Another promising candidate gene in this region is LIGHT SENSITIVE HYPOCOTYLS 10 (LSH10 [AT2G42610]), a probable transcription regulator that acts as a developmental regulator by promoting cell growth in response to light, and is involved in mRNA transcription, post-embryonic plant morphogenesis, and response to light stimulus (Berardini et al., 2015; UniProtKB, 2019; TAIR, 2020). LSH genes have been shown to affect the expression of genes, such as BLADE-ON-PETIOLE (BOP), that regulate KNOTTED-LIKE HOMEOBOX (KNOX) gene activity, and thus leaf shape complexity, in tomato (Ichihashi et al., 2014). This suggests a role for LSH genes in regulating leaf width and leaf shape complexity in C. diffusa. Differential expression of LSH10 in particular is associated with leaf shape differences due to broadness and leaf dissection in sweet potato (Gupta et al., 2020).
Genetics alone may not be sufficient to understand some phenotypic dynamics; thus genetic data must be incorporated into an environmental context. Previous analyses of the phenotypes of these individuals found a significant interaction of range (native or invasive) by latitude of population collection site in basal leaf count, area of longest leaf, and other phenotypic traits not assessed here (Turner et al., 2014). Further investigation found that abiotic environmental variation of collection site had more explanatory power than latitude in a field common garden including the populations studied here (Turner et al., 2015). Native populations demonstrated a significant relationship with abiotic environmental variation for several size and life history traits, which was typically non-significant in invasive populations, suggesting a difference in the extent of local adaptation in each range. An analysis of the climate of 662 occurrence locations of this species in both the native and invasive ranges suggests a shift in the realized niche of C. diffusa in the invaded range, which may coincide with the evolution of increased physiological tolerance; the invasive realized niche appears to have shifted into more arid climates and expanded into climates with a broader range of precipitation (Turner et al., 2015). A shift in the realized niched between ranges may have changed selection on traits, such as leaf width, and the genes that underlie those traits.
This work has integrated and advanced multiple efforts to understand the genetic mechanisms underlying traits associated with invasiveness in C. diffusa. This work contributes the first draft assembly of weedy member of a large and weedy genus, and the first non-domesticated member of the Carduoideae subfamily. These tools will be useful to future studies of other weedy and economically important relatives. We have further associated variation in leaf width with alleles at the two SNPs which confer larger leaves and are more often found in invasive populations, and we have identified likely candidate genes underlying this trait. In this rosette forming species, increased leaf width is a major component of increased biomass, a common trait in invasive plants correlated with increased fitness, and yet may be less polygenic and therefore more likely than biomass to be identified by GWAS. Variation in plant size between the ranges suggest selection for increased fitness, and therefore invasiveness, among North American populations. Thus, this study represents an important step in functionally identifying the underlying causes of invasion success in this species over the last ∼100 years.
Data Availability Statement
Raw data used to produce the draft reference genome is available at the NCBI SRA at https://www.ncbi.nlm.nih.gov/sra/SRX1355843[accn] (Turner, 2015). Raw reads used in GWAS are available at https://www.ncbi.nlm.nih.gov/bioproject/PRJNA681918/ (Turner et al., 2020b). The annotated plastome is available at the NCBI GenBank, accession number KJ690264 (Turner and Grassa, 2014b). Other data and scripts used for cleaning, assembly, and annotation of the plastome are available at http://dx.doi.org/10.6084/m9.figshare.1044306 (Supplementary Figures S1–3, Supplementary Table S2; Turner and Grassa, 2014c). Draft reference assembly and all other data, scripts, and supplementary materials available at Dryad repository at https://datadryad.org/stash/dataset/doi:10.5061/dryad.bvq83bk79 (Turner et al., 2020c).
Author Contributions
KT and LR designed the experiment. KT collected the phenotypic data. KT and KO collected genomic data. KT, KO, and CG performed genomic analyses. All authors contributed to and approved the manuscript.
Funding
This work was supported by the National Science Foundation grants (award 1523842, and NSF Idaho EPSCoR Program award OIA-1757324) to KT, a Natural Sciences and Engineering Research Council (NSERC) Postdoctoral Fellowship (516658) to KO, and NSERC grants (327475 and 353026) to LR.
Conflict of Interest
The authors declare that the research was conducted in the absence of any commercial or financial relationships that could be construed as a potential conflict of interest.
Acknowledgments
We thank A. Guggisberg, A. Shipunov, A. Stephens, and M. King for seed collection, K. Nurkowski for plant care, D. Huang for assistance with error checking the plastome assembly, and R. Timme for help with finalizing the plastome annotation.
Supplementary Material
The Supplementary Material for this article can be found online at: https://www.frontiersin.org/articles/10.3389/fevo.2020.577635/full#supplementary-material
References
Allen, J. M., Huang, D. I., Cronk, Q. C., and Johnson, K. P. (2015). aTRAM - automated target restricted assembly method: a fast method for assembling loci across divergent taxa from next-generation sequencing data. BMC Bioinform. 16:98. doi: 10.1186/s12859-015-0515-2
Altschul, S. F., Gish, W., Miller, W., Myers, E. W., and Lipman, D. J. (1990). Basic local alignment search tool. J. Mol. Biol. 215, 403–410. doi: 10.1016/S0022-2836(05)80360-2
Altschul, S. F., Madden, T. L., Schäffer, A. A., Zhang, J., Zhang, Z., Miller, W., et al. (1997). Gapped BLAST and PSI-BLAST: a new generation of protein database search programs. Nucl. Acids Res. 25, 3389–3402. doi: 10.1093/nar/25.17.3389
Badouin, H., Gouzy, J., Grassa, C. J., Murat, F., Staton, S. E., Cottret, L., et al. (2017). The sunflower genome provides insights into oil metabolism, flowering and Asterid evolution. Nature 546, 148–152. doi: 10.1038/nature22380
Bancheva, S., and Greilhuber, J. (2006). Genome size in Bulgarian Centaurea s.l. (Asteraceae). Plant Syst. Evol. 257, 95–117. doi: 10.1007/s00606-005-0384-7
Bates, D., Mächler, M., Bolker, B., and Walker, S. (2014). Fitting linear mixed-effects models using lme4. ArXiv[Preprint]. doi: 10.18637/jss.v067.i01
Berardini, T. Z., Reiser, L., Li, D., Mezheritsky, Y., Muller, R., Strait, E., et al. (2015). The arabidopsis information resource: Making and mining the “gold standard” annotated reference plant genome. Genesis 53, 474–485. doi: 10.1002/dvg.22877
Bick, J.-A., Åslund, F., Chen, Y., and Leustek, T. (1998). Glutaredoxin function for the carboxyl-terminal domain of the plant-type 5’-adenylylsulfate reductase. Proc. Natl. Acad. Sci. U.S.A. 95, 8404–8409. doi: 10.1073/pnas.95.14.8404
Blumenthal, D. M., and Hufbauer, R. A. (2007). Increased plant size in exotic populations: a common-garden test with 14 invasive species. Ecology 88, 2758–2765. doi: 10.1890/06-2115.1
Bock, D. G., Caseys, C., Cousens, R. D., Hahn, M. A., Heredia, S. M., Hübner, S., et al. (2015). What we still don’t know about invasion genetics. Mol. Ecol. 24, 2277–2297. doi: 10.1111/mec.13032
Boetzer, M., and Pirovano, W. (2012). Toward almost closed genomes with GapFiller. Genome Biol. 13:R56. doi: 10.1186/gb-2012-13-6-r56
Boisvert, S., Laviolette, F., and Corbeil, J. (2010). Ray: simultaneous assembly of reads from a mix of high-throughput sequencing technologies. J. Comp. Biol. 17, 1519–1533. doi: 10.1089/cmb.2009.0238
Bolger, A. M., Lohse, M., and Usadel, B. (2014). Trimmomatic: a flexible trimmer for Illumina sequence data. Bioinformatics 30, 2114-2120. doi: 10.1093/bioinformatics/btu170
Broad Institute (2009). Picard Toolkit. Available at: http://broadinstitute.github.io/picard/ (accessed on 29 April 2014).
Buchfink, B., Xie, C., and Huson, D. H. (2015). Fast and sensitive protein alignment using DIAMOND. Nat. Methods 12, 59–60. doi: 10.1038/nmeth.3176
Burke, J. M., Lai, Z., Salmaso, M., Nakazato, T., Tang, S., Heesacker, A., et al. (2004). Comparative mapping and rapid karyotypic evolution in the genus Helianthus. Genetics 167, 449–457. doi: 10.1534/genetics.167.1.449
Buswell, J. M., Moles, A. T., and Hartley, S. (2011). Is rapid evolution common in introduced plant species? J. Ecol. 99, 214–224. doi: 10.1111/j.1365-2745.2010.01759.x
Catford, J. A., Smith, A. L., Wragg, P. D., Clark, A. T., Kosmala, M., Cavender-Bares, J., et al. (2019). Traits linked with species invasiveness and community invasibility vary with time, stage and indicator of invasion in a long-term grassland experiment. Ecol. Lett. 22, 593–604. doi: 10.1111/ele.13220
Chitwood, D. H., Kumar, R., Headland, L. R., Ranjan, A., Covington, M. F., Ichihashi, Y., et al. (2013). A quantitative genetic basis for leaf morphology in a set of precisely defined tomato introgression lines. Plant Cell 25, 2465–2481. doi: 10.1105/tpc.113.112391
Chitwood, D. H., Ranjan, A., Martinez, C. C., Headland, L. R., Thiem, T., Kumar, R., et al. (2014). A modern ampelography: a genetic basis for leaf shape and venation patterning in grape. Plant Physiol. 164, 259–272. doi: 10.1104/pp.113.229708
Curci, P. L., De Paola, D., and Sonnante, G. (2016). Development of chloroplast genomic resources for Cynara. Mol. Ecol. Resour. 16, 562–573. doi: 10.1111/1755-0998.12457
Danecek, P., Auton, A., Abecasis, G., Albers, C. A., Banks, E., DePristo, M. A., et al. (2011). The variant call format and VCFtools. Bioinformatics 27, 2156–2158. doi: 10.1093/bioinformatics/btr330
de Lima, M. F., Eloy, N. B., de Siqueira, J. A. B., Inzé, D., Hemerly, A. S., and Ferreira, P. C. G. (2017). Molecular mechanisms of biomass increase in plants. Biotechnol. Res. Innov. 1, 14–25. doi: 10.1016/j.biori.2017.08.001
D’Esposito, D., Cappetta, E., Andolfo, G., Ferriello, F., Borgonuovo, C., Caruso, G., et al. (2019). Deciphering the biological processes underlying tomato biomass production and composition. Plant Physiol. Biochem. 143, 50–60. doi: 10.1016/j.plaphy.2019.08.010
Dietz, H., and Edwards, P. J. (2006). Recognition that causal processes change during plant invasion helps explain conflicts in evidence. Ecology 87, 1359–1367. doi: 10.1890/0012-9658(2006)87[1359:rtcpcd]2.0.co;2
Dlugosch, K. M., and Parker, I. M. (2008). Invading populations of an ornamental shrub show rapid life history evolution despite genetic bottlenecks. Ecol. Lett. 11, 701–709. doi: 10.1111/j.1461-0248.2008.01181.x
Edgar, R. C. (2004). MUSCLE: multiple sequence alignment with high accuracy and high throughput. Nucl. Acids Res. 32, 1792–1797. doi: 10.1093/nar/gkh340
Felker-Quinn, E., Schweitzer, J. A., and Bailey, J. K. (2013). Meta-analysis reveals evolution in invasive plant species but little support for Evolution of Increased Competitive Ability (EICA). Ecol. Evol. 3, 739–751. doi: 10.1002/ece3.488
Flood, P. J., and Hancock, A. M. (2017). The genomic basis of adaptation in plants. Curr. Opin. Plant Biol. 36, 88–94. doi: 10.1016/j.pbi.2017.02.003
Fu, L., Niu, B., Zhu, Z., Wu, S., and Li, W. (2012). CD-HIT: accelerated for clustering the next-generation sequencing data. Bioinformatics 28, 3150–3152. doi: 10.1093/bioinformatics/bts565
Galland, T., Adeux, G., Dvořáková, H., E-Vojtkó, A., Orbán, I., Lussu, M., et al. (2019). Colonization resistance and establishment success along gradients of functional and phylogenetic diversity in experimental plant communities. J. Ecol. 107, 2090–2104. doi: 10.1111/1365-2745.13246
Galloway, L. F. (2005). Maternal effects provide phenotypic adaptation to local environmental conditions. New Phytol. 166, 93–100. doi: 10.1111/j.1469-8137.2004.01314.x
Girgis, H. Z. (2015). Red: an intelligent, rapid, accurate tool for detecting repeats de-novo on the genomic scale. BMC Bioinform. 16:227. doi: 10.1186/s12859-015-0654-5
Gnerre, S., MacCallum, I., Przybylski, D., Ribeiro, F. J., Burton, J. N., Walker, B. J., et al. (2011). High-quality draft assemblies of mammalian genomes from massively parallel sequence data. Proc. Natl. Acad. Sci. U.S.A. 108, 1513–1518. doi: 10.1073/pnas.1017351108
Grassa, C. J., and Kulathinal, R. J. (2011). Elevated evolutionary rates among functionally diverged reproductive genes across deep vertebrate lineages. Int. J. Evol. Biol. 2011:e274975. doi: 10.4061/2011/274975
Greuter, W. (2009). Compositae (pro parte majore). In: Greuter, W. & Raab-Straube, E. von (Ed.): Compositae. Euro+Med Plantbase - the Information Resource for Euro-Mediterranean Plant Diversity. Available at: http://ww2.bgbm.org/EuroPlusMed/. (accessed October18, 2020).
Guggisberg, A., Lai, Z., Huang, J., and Rieseberg, L. H. (2013). Transcriptome divergence between introduced and native populations of Canada thistle. Cirsium arvense. New Phytol. 199, 595–608. doi: 10.1111/nph.12258
Gupta, S., Rosenthal, D. M., Stinchcombe, J. R., and Baucom, R. S. (2020). The remarkable morphological diversity of leaf shape in sweet potato (Ipomoea batatas): the influence of genetics, environment, and G×E. New Phytol. 225, 2183–2195. doi: 10.1111/nph.16286
Haas, B. J., Salzberg, S. L., Zhu, W., Pertea, M., Allen, J. E., Orvis, J., et al. (2008). Automated eukaryotic gene structure annotation using EVidenceModeler and the Program to Assemble Spliced Alignments. Genome Biol. 9:R7. doi: 10.1186/gb-2008-9-1-r7
Hendry, A., Farrugia, T., and Kinnison, M. (2008). Human influences on rates of phenotypic change in wild animal populations. Mol. Ecol. 17, 20–29. doi: 10.1111/j.1365-294x.2007.03428.x
Hendry, A. P., Gotanda, K. M., and Svensson, E. I. (2017). Human influences on evolution, and the ecological and societal consequences. Philos. Trans. Royal Soc. B Biol. Sci. 372:20160028. doi: 10.1098/rstb.2016.0028
Hodgins, K. A., Bock, D. G., Hahn, M. A., Heredia, S. M., Turner, K. G., and Rieseberg, L. H. (2015). Comparative genomics in the Asteraceae reveals little evidence for parallel evolutionary change in invasive taxa. Mol. Ecol. 24, 2226–2240. doi: 10.1111/mec.13026
Hodgins, K. A., Lai, Z., Nurkowski, K., Huang, J., and Rieseberg, L. H. (2013). The molecular basis of invasiveness: differences in gene expression of native and introduced common ragweed (Ambrosia artemisiifolia) in stressful and benign environments. Mol. Ecol. 22, 2496–2510. doi: 10.1111/mec.12179
Hodgins, K. A., and Rieseberg, L. (2011). Genetic differentiation in life-history traits of introduced and native common ragweed (Ambrosia artemisiifolia) populations. J. Evol. Biol. 24, 2731–2749. doi: 10.1111/j.1420-9101.2011.02404.x
Huang, X., Adams, M. D., Zhou, H., and Kerlavage, A. R. (1997). A Tool for Analyzing and Annotating Genomic Sequences. Genomics 46, 37–45. doi: 10.1006/geno.1997.4984
Hufbauer, R. A., Facon, B., Ravigné, V., Turgeon, J., Foucaud, J., Lee, C. E., et al. (2012). Anthropogenically induced adaptation to invade (AIAI): Contemporary adaptation to human-altered habitats within the native range can promote invasions. Evol. Appl. 5, 89–101. doi: 10.1111/j.1752-4571.2011.00211.x
Ichihashi, Y., Aguilar-Martínez, J. A., Farhi, M., Chitwood, D. H., Kumar, R., Millon, L. V., et al. (2014). Evolutionary developmental transcriptomics reveals a gene network module regulating interspecific diversity in plant leaf shape. Proc. Natl. Acad. Sci. U.S.A. 111, E2616–E2621. doi: 10.1073/pnas.1402835111
Ichihashi, Y., Horiguchi, G., Gleissberg, S., and Tsukaya, H. (2010). The bHLH transcription factor SPATULA controls final leaf size in Arabidopsis thaliana. Plant Cell Physiol. 51, 252–261. doi: 10.1093/pcp/pcp184
Kanamoto, H., Yamashita, A., Okumura, S., Hattori, M., and Tomizawa, K. I. (2004). The complete genome sequence of the Lactuca sativa (lettuce) chloroplast. Jap. Soc. Plant Physiol. 45, 3031–3032. doi: 10.1080/23802359.2020.1778553
Kattge, J., Bönisch, G., Díaz, S., Lavorel, S., Prentice, I. C., Leadley, P., et al. (2020). TRY plant trait database – enhanced coverage and open access. Glob. Change Biol. 26, 119–188. doi: 10.1111/gcb.14904
Korte, A., and Farlow, A. (2013). The advantages and limitations of trait analysis with GWAS: a review. Plant Methods 9:29. doi: 10.1186/1746-4811-9-29
Kuester, A., Conner, J. K., Culley, T., and Baucom, R. S. (2014). How weeds emerge: a taxonomic and trait-based examination using United States data. New Phytol. 202, 1055–1068. doi: 10.1111/nph.12698
Kumschick, S., Hufbauer, R. A., Alba, C., and Blumenthal, D. M. (2013). Evolution of fast-growing and more resistant phenotypes in introduced common mullein (Verbascum thapsus). J. Ecol. 101, 378–387. doi: 10.1111/1365-2745.12044
Lachmuth, S., Durka, W., and Schurr, F. M. (2011). Differentiation of reproductive and competitive ability in the invaded range of Senecio inaequidens: the role of genetic Allee effects, adaptive and nonadaptive evolution. New Phytol. 192, 529–541. doi: 10.1111/j.1469-8137.2011.03808.x
Lai, Z., Kane, N. C., Kozik, A., Hodgins, K. A., Dlugosch, K. M., Barker, M. S., et al. (2012). Genomics of Compositae weeds: EST libraries, microarrays, and evidence of introgression. Am. J. Bot. 99, 209–218. doi: 10.3732/ajb.1100313
Lai, Z., Kane, N. C., Zou, Y., and Rieseberg, L. H. (2008). Natural variation in gene expression between wild and weedy populations of Helianthus annuus. Genetics 179:1881. doi: 10.1534/genetics.108.091041
Lamesch, P., Berardini, T. Z., Li, D., Swarbreck, D., Wilks, C., Sasidharan, R., et al. (2012). The arabidopsis information resource (TAIR): improved gene annotation and new tools. Nucleic Acids Res. 40, D1202–D1210. doi: 10.1093/nar/gkr1090
Lee-Yaw, J. A., Grassa, C. J., Joly, S., Andrew, R. L., and Rieseberg, L. H. (2019). An evaluation of alternative explanations for widespread cytonuclear discordance in annual sunflowers (Helianthus). New Phytol. 221, 515–526. doi: 10.1111/nph.15386
Lejeune, K. D., and Seastedt, T. R. (2001). Centaurea species: the forb that won the west. Conserv. Biol. 15, 1568–1574. doi: 10.1046/j.1523-1739.2001.00242.x
Li, D., Liu, C.-M., Luo, R., Sadakane, K., and Lam, T.-W. (2015). MEGAHIT: an ultra-fast single-node solution for large and complex metagenomics assembly via succinct de Bruijn graph. Bioinformatics 31, 1674–1676. doi: 10.1093/bioinformatics/btv033
Li, H., and Durbin, R. (2009). Fast and accurate short read alignment with Burrows–Wheeler transform. Bioinformatics 25, 1754–1760. doi: 10.1093/bioinformatics/btp324
Li, H., Handsaker, B., Wysoker, A., Fennell, T., Ruan, J., Homer, N., et al. (2009). The Sequence Alignment/Map format and SAMtools. Bioinformatics 25, 2078–2079. doi: 10.1093/bioinformatics/btp352
Lipka, A. E., Tian, F., Wang, Q., Peiffer, J., Li, M., Bradbury, P. J., et al. (2012). GAPIT: genome association and prediction integrated tool. Bioinformatics 28, 2397–2399. doi: 10.1093/bioinformatics/bts444
Lohse, M., Drechsel, O., Kahlau, S., and Bock, R. (2013). OrganellarGenomeDRAW–a suite of tools for generating physical maps of plastid and mitochondrial genomes and visualizing expression data sets. Nucl. Acids Res. 41, W575–W581. doi: 10.1093/nar/gkt289
Metcalf, J. C., Rose, K. E., and Rees, M. (2003). Evolutionary demography of monocarpic perennials. Trends Ecol. Evol. 18, 471–480. doi: 10.1016/S0169-5347(03)00162-9
O’Leary, N. A., Wright, M. W., Brister, J. R., Ciufo, S., Haddad, D., McVeigh, R., et al. (2016). Reference sequence (RefSeq) database at NCBI: current status, taxonomic expansion, and functional annotation. Nucl. Acids Res. 44, D733–D745. doi: 10.1093/nar/gkv1189
Ostevik, K. L., Samuk, K., and Rieseberg, L. H. (2020). Ancestral reconstruction of karyotypes reveals an exceptional rate of nonrandom chromosomal evolution in sunflower. Genetics 214, 1031–1045. doi: 10.1534/genetics.120.303026
Park, K. T., Park, L., Kim, J.-H., and Park, S. (2019). Characterization of the complete chloroplast genome of Centaurea maculosa (Asteraceae). Mitochondr. DNA Part B 4, 3929–3930. doi: 10.1080/23802359.2019.1687342
Parker, J. D., Torchin, M. E., Hufbauer, R. A., Lemoine, N. P., Alba, C., Blumenthal, D. M., et al. (2013). Do invasive species perform better in their new ranges? Ecology 94, 985–994. doi: 10.1890/12-1810.1
Piazza, P., Bailey, C. D., Cartolano, M., Krieger, J., Cao, J., Ossowski, S., et al. (2010). Arabidopsis thaliana leaf form evolved via loss of KNOX expression in leaves in association with a selective wweep. Curr. Biol. 20, 2223–2228. doi: 10.1016/j.cub.2010.11.037
Poland, J. A., Brown, P. J., Sorrells, M. E., and Jannink, J.-L. (2012). Development of high-density genetic maps for barley and wheat using a novel two-enzyme genotyping-by-sequencing approach. PLoS One 7:e32253. doi: 10.1371/journal.pone.0032253
Puritz, J. B., Hollenbeck, C. M., and Gold, J. R. (2014). dDocent: a RADseq, variant-calling pipeline designed for population genomics of non-model organisms. PeerJ 2:e431. doi: 10.7717/peerj.431
R Core Team (2018). R: A Language and Environment for Statistical Computing. Vienna: R Foundation for Statiscial Computing.
Rasband, W. (2011). ImageJ. Available online at: http://imagej.nih.gov/ij/ (accessed August 15, 2011).
Ricciardi, A., Blackburn, T. M., Carlton, J. T., Dick, J. T. A., Hulme, P. E., Iacarella, J. C., et al. (2017). Invasion science: a horizon scan of emerging challenges and opportunities. Trends Ecol. Evol. 32, 464–474. doi: 10.1016/j.tree.2017.03.007
Richter, D. C., Schuster, S. C., and Huson, D. H. (2007). OSLay: optimal syntenic layout of unfinished assemblies. Bioinformatics 23, 1573–1579. doi: 10.1093/bioinformatics/btm153
Rius, M., Bourne, S., Hornsby, H. G., and Chapman, M. A. (2015). Applications of next-generation sequencing to the study of biological invasions. Curr. Zool. 61, 488–504. doi: 10.1093/czoolo/61.3.488
Rodríguez-Verdugo, A., Buckley, J., and Stapley, J. (2017). The genomic basis of eco-evolutionary dynamics. Mol. Ecol. 26, 1456–1464. doi: 10.1111/mec.14045
Roscher, C., Gubsch, M., Lipowsky, A., Schumacher, J., Weigelt, A., Buchmann, N., et al. (2018). Trait means, trait plasticity and trait differences to other species jointly explain species performances in grasslands of varying diversity. Oikos 127, 865–865. doi: 10.1111/oik.04815
Rowe, H. C., Hansen, B. G., Halkier, B. A., and Kliebenstein, D. J. (2008). Biochemical networks and epistasis shape the Arabidopsis thaliana metabolome. Plant Cell 20, 1199–1216. doi: 10.1105/tpc.108.058131
Salih, R. H. M., Majeský, L’, Schwarzacher, T., Gornall, R., and Heslop-Harrison, P. (2017). Complete chloroplast genomes from apomictic Taraxacum (Asteraceae): identity and variation between three microspecies. PLoS One 12:e0168008. doi: 10.1371/journal.pone.0168008
Santure, A. W., and Garant, D. (2018). Wild GWAS—association mapping in natural populations. Mol. Ecol. Resour. 18, 729–738. doi: 10.1111/1755-0998.12901
Scaglione, D., Reyes-Chin-Wo, S., Acquadro, A., Froenicke, L., Portis, E., Beitel, C., et al. (2016). The genome sequence of the outbreeding globe artichoke constructed de novo incorporating a phase-aware low-pass sequencing strategy of F1 progeny. Sci. Rep. 6:19427. doi: 10.1038/srep19427
Setya, A., Murillo, M., and Leustek, T. (1996). Sulfate reduction in higher plants: Molecular evidence for a novel 5’-adenylylsulfate reductase. Proc. Natl. Acad. Sci. U.S.A. 93, 13383–13388. doi: 10.1073/pnas.93.23.13383
Sheley, R. L., and Larson, L. L. (1996). Emergence date effects on resource partitioning between diffuse knapweed seedlings. J. Range Manag. 49, 241–244. doi: 10.2307/4002885
Stanke, M., Keller, O., Gunduz, I., Hayes, A., Waack, S., and Morgenstern, B. (2006). AUGUSTUS: ab initio prediction of alternative transcripts. Nucl. Acids Res. 34(Suppl._2), W435–W439. doi: 10.1093/nar/gkl200
Stapley, J., Santure, A. W., and Dennis, S. R. (2015). Transposable elements as agents of rapid adaptation may explain the genetic paradox of invasive species. Mol. Ecol. 24, 2241–2252. doi: 10.1111/mec.13089
Stewart, C. N., Tranel, P. J., Horvath, D. P., Anderson, J. V., Rieseberg, L. H., Westwood, J. H., et al. (2009). Evolution of weediness and invasiveness: charting the course for weed genomics. Weed Sci. 57, 451–462. doi: 10.1614/WS-09-011.1
Susanna, A., and Garcia-Jacas, N. (2009). “Cardueae (Carduoideae),” in Systematics, evolution, and biogeography of Compositae, eds V. A. Funk, T. Stuessy, and R. Bayer (Vienna: International Association for Plant Taxonomy), 293–313.
TAIR (2020). The Arabidopsis Information Resource. Available at: https://www.arabidopsis.org (accessed on 23 April 2020).
Tamazian, G., Dobrynin, P., Krasheninnikova, K., Komissarov, A., Koepfli, K.-P., and O’Brien, S. J. (2016). Chromosomer: a reference-based genome arrangement tool for producing draft chromosome sequences. GigaScience 5:38. doi: 10.1186/s13742-016-0141-6
Thompson, D. J., and Stout, D. G. (1991). Duration of the juvenile period in diffuse knapweed (Centaurea diffusa). Can. J. Bot. 69, 368–371. doi: 10.1139/b91-050
Timme, R. E., Kuehl, J. V., Boore, J. L., and Jansen, R. K. (2007). A comparative analysis of the Lactuca and Helianthus (Asteraceae) plastid genomes: identification of divergent regions and categorization of shared repeats. Am. J. Bot. 94, 302–312. doi: 10.3732/ajb.94.3.302
Tsukaya, H. (2018). “A Consideration of leaf shape evolution in the context of the primary function of the leaf as a photosynthetic organ,” in The Leaf: A Platform for Performing Photosynthesis, eds W. W. Adams, III and I. Terashima (New York, NY: Springer), 1–26. doi: 10.1007/978-3-319-93594-2_1
Turner, K. G. (2015). [DATA] Whole genome sequencing of Centaurea diffusa: native individual from Turkey. 1 Illumina HiSeq 2000 run: 69.6M spots, 13.9G bases, 8Gb downloads. Accession: SRX1355843. https://www.ncbi.nlm.nih.gov/sra/SRX1355843[accn] (accessed October 24, 2015).
Turner, K. G., Fréville, H., and Rieseberg, L. H. (2015). Adaptive plasticity and niche expansion in an invasive thistle. Ecol. Evol. 5, 3183–3197. doi: 10.1002/ece3.1599
Turner, K. G., and Grassa, C. J. (2014a). [PRE-PRINT] Complete plastid genome assembly of invasive plant, Centaurea diffusa. bioRxiv[Preprint]. doi: 10.1101/005900
Turner, K. G., and Grassa, C. J. (2014b). [DATA] Centaurea diffusa chloroplast, complete genome. NCBI GenBank. Accession: KJ690264.1. Available online at: https://www.ncbi.nlm.nih.gov/nuccore/KJ690264 (accessed April 10, 2014).
Turner, K. G., and Grassa, C. J. (2014c). [DATA] Complete plastid genome assembly of invasive plant, Centaurea diffusa, Supplementary Files. figshare. Available online at: http://dx.doi.org/10.6084/m9.figshare.1044306 (accessed June 3, 2014).
Turner, K. G., Hufbauer, R. A., and Rieseberg, L. H. (2014). Rapid evolution of an invasive weed. New Phytol. 202, 309–321. doi: 10.1111/nph.12634
Turner, K. G., Lorts, C. M., Haile, A. T., and Lasky, J. R. (2020a). Effects of genomic and functional diversity on stand-level productivity and performance of non-native Arabidopsis. Proc. Royal Soc. B Biol. Sci. 287:20202041. doi: 10.1098/rspb.2020.2041
Turner, K. G., Nurkowski, K. A., and Rieseberg, L. H. (2017). Gene expression and drought response in an invasive thistle. Biol. Invas. 19, 875–893. doi: 10.1007/s10530-016-1308-x
Turner, K. G., Ostevik, K. L., Grassa, C. J., and Rieseberg, L. H. (2020b). [DATA] Reduced Representation Sequence Data From Centaurea Diffusa Individuals – BioProject. Accession: PRJNA681918. Available online at: https://www.ncbi.nlm.nih.gov/bioproject/PRJNA681918/ (accessed December 1, 2020).
Turner, K. G., Ostevik, K. L., Grassa, C. J., and Rieseberg, L. H. (2020c). [DATA] Genomic Analyses of Phenotypic Differences Between Native and Invasive Populations of Diffuse Knapweed (Centaurea diffusa), Dryad, Dataset. Available online at: https://doi.org/10.5061/dryad.bvq83bk79 (accessed December 4, 2020).
UniProtKB. (2019). UniProt: a worldwide hub of protein knowledge. Nucl. Acids Res. 47, D506–D515. doi: 10.1093/nar/gky1049
VanRaden, P. M. (2008). Efficient methods to compute genomic predictions. J. Dairy Sci. 91, 4414–4423. doi: 10.3168/jds.2007-0980
Violle, C., Navas, M.-L., Vile, D., Kazakou, E., Fortunel, C., Hummel, I., et al. (2007). Let the concept of trait be functional! Oikos 116, 882–892. doi: 10.1111/j.0030-1299.2007.15559.x
Walker, B. J., Abeel, T., Shea, T., Priest, M., Abouelliel, A., Sakthikumar, S., et al. (2014). Pilon: an integrated tool for comprehensive microbial variant detection and genome assembly improvement. PLoS One 9:e112963. doi: 10.1371/journal.pone.0112963
Whitehead, A., and Crawford, D. L. (2006). Variation within and among species in gene expression: raw material for evolution. Mol. Ecol. 15, 1197–1211. doi: 10.1111/j.1365-294x.2006.02868.x
Wieters, B., Steige, K. A., He, F., Koch, E. M., Ramos-Onsins, S. E., Gu, H., et al. (2020). Polygenic adaptation of rosette growth variation in Arabidopsis thaliana populations. bioRxiv[Preprint]. doi: 10.1101/2020.03.31.018341
Wolfe, K. H., Li, W. H., and Sharp, P. M. (1987). Rates of nucleotide substitution vary greatly among plant mitochondrial, chloroplast, and nuclear DNAs. Proc. Natl. Acad. Sci. U.S.A. 84, 9054–9058. doi: 10.1073/pnas.84.24.9054
Wu, T. D., Reeder, J., Lawrence, M., Becker, G., and Brauer, M. J. (2016). “GMAP and GSNAP for Genomic Sequence Alignment: Enhancements to Speed, Accuracy, and Functionality,” in Statistical Genomics: Methods and Protocols, eds E. Mathé and S. Davis (New York, NY: Springer), 283–334. doi: 10.1007/978-1-4939-3578-9_15
Wyman, S. K., Jansen, R. K., and Boore, J. L. (2004). Automatic annotation of organellar genomes with DOGMA. Bioinformatics 20, 3252–3255. doi: 10.1093/bioinformatics/bth352
Yang, Z. (2007). PAML 4: phylogenetic analysis by maximum likelihood. Mol. Biol. Evol. 24, 1586–1591. doi: 10.1093/molbev/msm088
Yang, Z., and Nielsen, R. (2000). Estimating synonymous and nonsynonymous substitution rates under realistic evolutionary models. Mol. Biol. Evol. 17, 32–43. doi: 10.1093/oxfordjournals.molbev.a026236
Keywords: invasive species, rapid evolution, adaptive genetic variation, novel environments, leaf width, diffuse knapweed (Centaura diffusa), GWAS, draft genome assembly
Citation: Turner KG, Ostevik KL, Grassa CJ and Rieseberg LH (2021) Genomic Analyses of Phenotypic Differences Between Native and Invasive Populations of Diffuse Knapweed (Centaurea diffusa). Front. Ecol. Evol. 8:577635. doi: 10.3389/fevo.2020.577635
Received: 29 June 2020; Accepted: 23 October 2020;
Published: 08 January 2021.
Edited by:
Emiliano Mori, University of Siena, ItalyReviewed by:
Michele Lussu, Istituto Regionale per la Floricoltura (IRF), ItalyFabio Bozzeda, Universidad Austral de Chile, Chile
Copyright © 2021 Turner, Ostevik, Grassa and Rieseberg. This is an open-access article distributed under the terms of the Creative Commons Attribution License (CC BY). The use, distribution or reproduction in other forums is permitted, provided the original author(s) and the copyright owner(s) are credited and that the original publication in this journal is cited, in accordance with accepted academic practice. No use, distribution or reproduction is permitted which does not comply with these terms.
*Correspondence: Kathryn G. Turner, dHVybmthdDJAaXN1LmVkdQ==