- 1Centre for Functional Ecology - Science for People & the Planet, Instituto Politécnico de Coimbra, Escola Superior Agrária de Coimbra, Coimbra, Portugal
- 2Centro de Investigação em Biodiversidade e Recursos Genéticos (CIBIO/InBIO), Universidade do Porto, Porto, Portugal
- 3Centre for Functional Ecology - Science for People & the Planet, Department of Life Sciences, University of Coimbra, Coimbra, Portugal
- 4Institute of Environmental Sciences, Leiden University, Leiden, Netherlands
Biological invasions are among the major contemporary threats to biodiversity. Biocontrol has a long history as a safe and effective strategy for the control of invasive species in several world regions, yet the life history and ecological requirements of biocontrol agents are often poorly understood. Species distribution models and assessments of niche overlap and dynamics constitute a way to quantify and compare ecological niches and are widely used for predicting biological invasions. While these tools can also be valuable to predict the effectiveness of biocontrol programs and anticipate whether biocontrol agents can establish on areas targeted for control, they remain underutilized for this purpose. In this work, species distribution models and comparisons of niche dynamics are used to predict the success of the ongoing biocontrol program for Acacia longifolia in Portugal, and potentially along the Mediterranean Basin, using the Australian gall-forming wasp Trichilogaster acaciaelongifoliae, previously released in South Africa. Niches of both the invasive plant and the biocontrol agent were found to be highly similar and to remain conserved through the introduction process. Distribution models identify suitable climatic areas for A. longifolia in 19% of the Mediterranean Basin and predict successful establishment of T. acaciaelongifoliae in 41% of the suitable area for A. longifolia, despite excluding a few locations where very recent establishment occurred. These results allow us to quantify the risk of future A. longifolia invasion and potential success of biocontrol, as well as establish a comparative framework for similar programs being considered in other regions of the world dealing with A. longifolia invasions.
Introduction
Biological invasions are among the major contemporary threats to biodiversity (IPBES, 2019). Specifically, invasive plants cause changes in ecosystem services and functionality, with negative impacts at different levels, e.g., at the level of plant and soil communities and nutrient dynamics (Marchante et al., 2008a, b; Le Maitre et al., 2011; Vilà et al., 2011; Simberloff et al., 2013; Zhang et al., 2019) which are often difficult to reverse or mitigate without active restoration efforts (Le Maitre et al., 2011). Control of invasive species is frequently prohibitively expensive and labor-intensive (Marais et al., 2004). Understanding biological invasion processes is essential for informing decision-making and optimizing the allocation of limited resources. Correlative approaches based on geographic occurrence data (such as species distribution models and multivariate environmental assessments of niche overlap) are valuable to quantify and compare ecological niches and are widely used to predict and improve management of biological invasions (e.g., Broennimann et al., 2007; Vicente et al., 2010, 2011, 2016; Petitpierre et al., 2012; Guisan et al., 2014).
Biological control (hereafter biocontrol) has a long history (over a century) as a safe and effective strategy for the control of invasive plant species (Clewley et al., 2012) in several regions of the world, such as South Africa, Australia, or United States. Yet, the biocontrol of invasive plants was only recently (less than a decade ago) initiated in Europe (Shaw et al., 2018). Classical biocontrol consists on the deliberate introduction of a natural enemy of the target invasive species into a new region invaded by the latter with the objective of mitigating its competitive advantage. Biocontrol agents should be highly specific in order to minimize interactions with native biodiversity in the regions where they are introduced. They should also be able to establish viable populations in the invaded areas. Considering this, it follows that the chances of success of a biocontrol agent are likely to be improved when the ecological requirements for its successful establishment and long-term persistence are similar to those of its target. These requirements, due to their explicitly geographically-oriented and scenopoetic nature, fall within the Grinnellian definition of the ecological niche (sensu Peterson et al., 2011). Consequently, the similarity in ecological requirements between species can be characterized using the related concept of niche overlap (sensu Warren et al., 2008). Despite this, very few studies so far have explicitly compared biocontrol agents and their respective targets in terms of niche overlap (but see Sun et al., 2017).
Acacia longifolia is a Leguminosae native to southeastern Australia which has become highly invasive in several exotic locations. Currently, invasive populations of A. longifolia are established beyond its native range in south-western Australia (Costello et al., 2000; Impson et al., 2011), as well as in New Zealand, South Africa, Chile, United States (California), Spain (Galicia), and Portugal (EFSA Panel on Plant Health, 2015). Large long-lived seed banks and the ability to germinate following disturbances (particularly fire) are common traits in the Acacia genus which account for its high invasive ability (Richardson and Kluge, 2008; Passos et al., 2017). Invasions by A. longifolia are associated to changes in soil communities and nutrient cycles (Marchante et al., 2008a, b), disturbance of plant (Costello et al., 2000; Marchante et al., 2003, 2015), and plant-gall communities (López-Núñez et al., 2017), nutrient and water cycling (Werner et al., 2010), and fire regimes. Many of these effects tend to persist after removal, hindering restoration efforts (Marchante et al., 2009, 2011a). Mechanical and chemical control of invasive Acacia species is often highly demanding in terms of resources, time and labor (Marais et al., 2004). Reduction of seed banks is particularly problematic, yet essential for the long-term management of invasive plants with high seed production (Richardson and Kluge, 2008). Biological control is consequently often proposed as a valuable and indispensable asset for the management of Acacia invasions (Marais et al., 2004; Richardson and Kluge, 2008; Wilson et al., 2011).
Trichilogaster acaciaelongifoliae is a gall-forming wasp endemic to Australia. Like all gall-forming insects, it is a highly specific endoparasite which requires successful interaction with its host to complete the life cycle, making it an ideal candidate for biocontrol programs due to the extremely low risk of detrimental interactions with species other than its preferred host, and its inability to expand beyond the distribution of its host (Dennill et al., 1993; Marchante et al., 2011b). Currently, only two programs using T. acaciaelongifoliae to control A. longifolia exist in the world. It was first introduced in South Africa in 1982 and 1983 (Dennill, 1985, 1988, 1990; Dennill and Donnelly, 1991; Dennill et al., 1993) where it quickly became established through most of the range of A. longifolia, successfully decreasing the reproductive potential (89–95%) and vegetative growth (53%) of its target and causing A. longifolia mortality in stressful situations (Dennill, 1985, 1988; Dennill and Gordon, 1990). Owing to the success of A. longifolia biocontrol in South Africa, a similar program was initiated in Portugal (Marchante et al., 2011b), with the first release of T. acaciaelongifoliae in the wild taking place recently, in 2015 (Marchante et al., 2017). As of 2018, four populations of T. acaciaelongifoliae established in Portugal, and it is expected that they will maintain the current trend of population growth and expansion, eventually reaching all areas of the country invaded by A. longifolia (Marchante et al., 2017). While the program was initiated in Portugal, the agent may potentially spread to other regions of the Mediterranean Basin where adequate environmental conditions are available.
Understanding how the niches of a biocontrol agent and its target species relate in their native distribution may be insufficient to predict the success of biocontrol. The possibility of niche shifts during introduction into new environments (Guisan et al., 2014) may promote niche divergence between the two species, creating a mismatch in environmental space which, when transported to geographic space, can result in inability of the biocontrol agent to establish in areas targeted for control.
This work aims to characterize the realized niches of A. longifolia and T. acaciaelongifoliae and test the adequacy of T. acaciaelongifoliae as a biocontrol agent for A. longifolia in Portugal as a function of niche overlap. To accomplish this, we characterize and compare the realized niches of the host plant and of the biocontrol agent in their native and introduced ranges. We also test the hypothesis of niche shifts by comparing realized niches between native and introduced ranges for both species. We then project the environmental affinities of A. longifolia and T. acaciaelongifoliae into geographical space using species distribution models, in order to predict the success of the biocontrol program primarily in Portugal but ultimately in the geographically and climatically proximate Mediterranean Basin.
Materials and Methods
Occurrence Records
A database of A. longifolia and T. acaciaelongifoliae occurrence records was compiled from the Global Biodiversity Information Facility1, Atlas of Living Australia2, South Australian Museum3 provided upon request by Doctor Peter Hudson, Australian National Insect Collection4 provided upon request by Doctor Juanita Rodriguez, Invasive Plants in Portugal – Invasoras.pt5 (Marchante et al., 2017), Southern Africa Plant Invaders Atlas (Henderson, 1999), literature references (Dennill, 1985, 1987; Prinsloo and Neser, 2007; Lado, 2008; Henriksen et al., 2017, 2019); and data provided by authors and collaborators (see section “acknowledgments”). Data collection for A. longifolia and T. acaciaelongifoliae was independent and no records were shared among the two datasets.
Occurrence data were considered until June 2018. A. longifolia records were included taking into account the species sensu lato (i.e., including subspecies A. longifolia longifolia, A. longifolia floribunda and A. longifolia sophorae, all considered hosts of T. acaciaelongifoliae). In June 2018, T. acaciaelongifoliae was still narrowly distributed in Portugal, and thus occurrence records in this area were not considered for subsequent analyses. Records outside the native area (southeastern Australia) and the introduced areas of interest (South Africa and Portugal) were excluded. Uncertain or duplicate records were also excluded. Records with less positional accuracy than 10 km were excluded to maximize geographic coverage while minimizing the loss of spatial precision (Gutiérrez-Rodríguez et al., 2017). To reduce potential sampling bias and spatial autocorrelation, a declustering approach (e.g., Vale et al., 2016; Dinis et al., 2019) was performed using the R package ecospat (Di Cola et al., 2017), which consisted of delimiting a 10 km buffer around each record and, when two or more buffers overlapped, removing one of the records randomly. This process was repeated until all remaining records per species had a distance of at least 10 km among them. Clustering was quantified before and after the declustering treatment to ensure an effective decrease in the level of clustering (e.g., Dinis et al., 2019). This was done by calculating the Nearest Neighbor Index in ArcMap 10.1 (Environmental Systems Research Institute, 2012). The final database consisted of 1493 and 140 occurrences for A. longifolia and T. acaciaelongifoliae, respectively (Supplementary Table 1). Occurrences were then further subdivided by geographical areas of interest (southeastern Australia, South Africa, and Portugal; Figure 1).
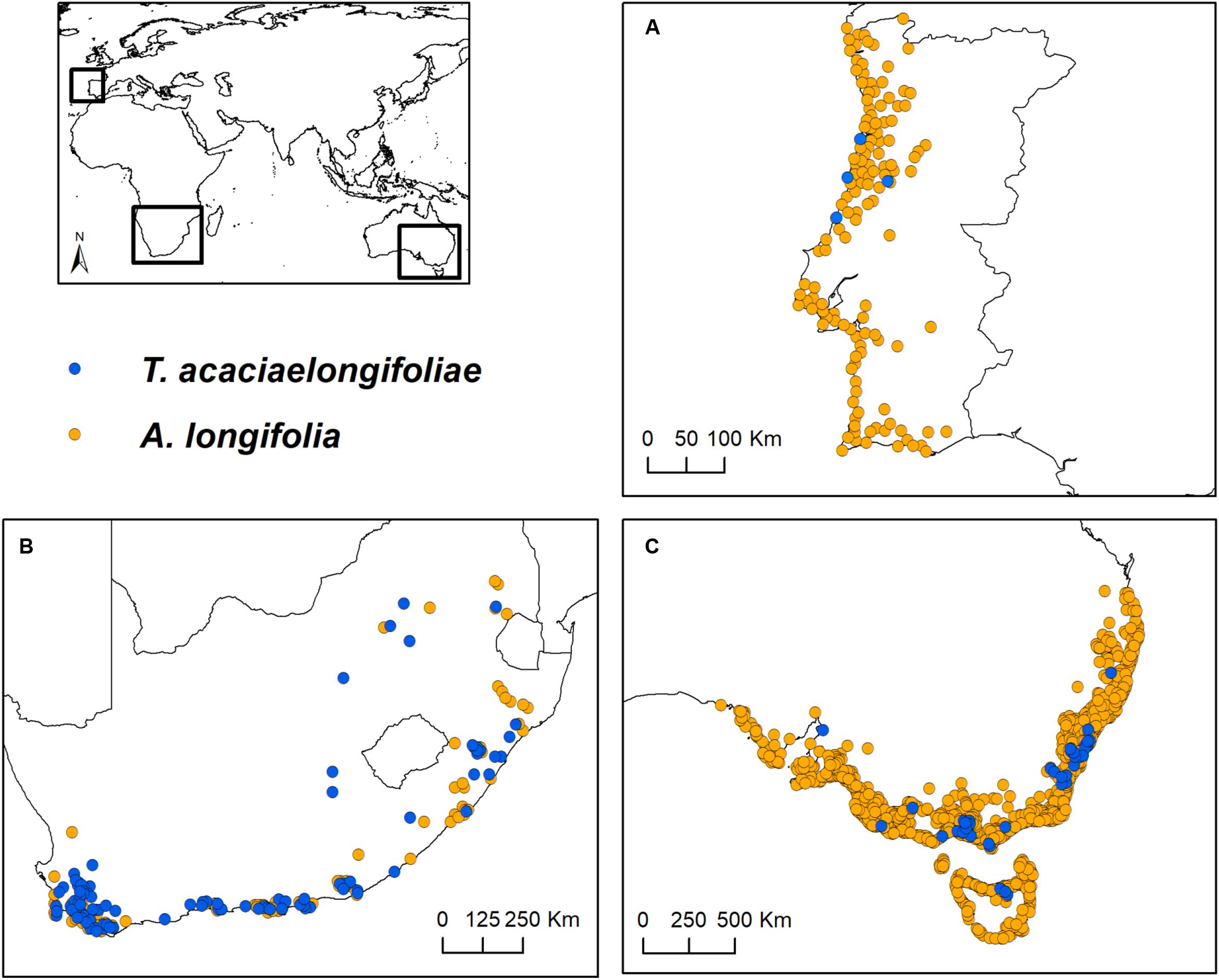
Figure 1. Study areas and occurrence records of Acacia longifolia and Trichilogaster acaciaelongifoliae used in this work. (A; top right): Portugal; (B; bottom left): South Africa; and (C; bottom right): southeastern Australia. Trichilogaster acaciaelongifoliae records from Portugal are merely indicative and were not included in any analyses due to their low number and recent establishment.
Bioclimatic Variables and Study Area
The native distributions of A. longifolia and T. acaciaelongifoliae were represented by southeastern Australia. South Africa and Portugal were selected to represent areas of A. longifolia invasion because they are the areas where biocontrol programs with T. acaciaelongifoliae are ongoing. The Mediterranean Basin (slightly expanded to include also northern Spain) was selected for model projections for two reasons. First, it is the geographical area of which Portugal is part and A. longifolia occurs with invasive potential in several countries in the region (Spain, France, Italy, Lorenzo et al., 2010); at the same time, if A. longifolia is present, this creates the possibility of natural dispersion of T. acaciaelongifoliae. Second, climatic similarity with the global distribution of A. longifolia and T. acaciaelongifoliae (predominately in areas of Mediterranean climate) is expected to maximize analogy of predictors.
Nineteen bioclimatic variables representing average contemporary conditions (1970–2000) were obtained from Worldclim v2.06 (Hijmans et al., 2005) at a spatial resolution of 10 × 10 km, to ensure consistency with the minimum positional accuracy of the occurrence data. Other scenopoetic candidate variables, such as topography, were considered but ultimately excluded under the assumption that they would not be informative at this spatial resolution, while variables related to soil composition and vegetation cover/structure were excluded due to being dynamically linked to the presence of the target species, consequently falling outside the scope of the Grinnellian niche (Peterson et al., 2011). The temporal extent of the Worldclim dataset overlaps with the collection dates for most occurrence records used in this work, and was therefore selected as an adequate representation of climatic conditions available to the target species upon the time of observation.
Background and training areas were defined by clipping bioclimatic layers to a 200 km buffer surrounding the minimum convex polygon which includes all occurrence records. Buffer size was selected taking into account coarse distributional patterns, topography, vegetation zones, and the limits of climatic regions according to the Köppen-Geiger climate classification (Köppen, 1900) and aims to represent the habitats and areas which the species may have reasonably sampled in each region (Barve et al., 2011) and prevent biases in the sampling of background data, which are known to occur when using overly broad or restrictive training areas (VanDerWal et al., 2009; Anderson and Raza, 2010). This was done separately for each species in each relevant area of distribution, i.e.: for A. longifolia in its native range in southeastern Australia as well as the invasive ranges in South Africa and Portugal; and for T. acaciaelongifoliae in its native and introduced ranges in southeastern Australia and South Africa, respectively. Analyses were also performed combining the multiple native and introduced/invaded ranges for each species (Australia, South Africa and Portugal for A. longifolia, Australia and South Africa for T. acaciaelongifoliae, Figure 2). All operations were performed in ArcMap 10.1 (Environmental Systems Research Institute, 2012).
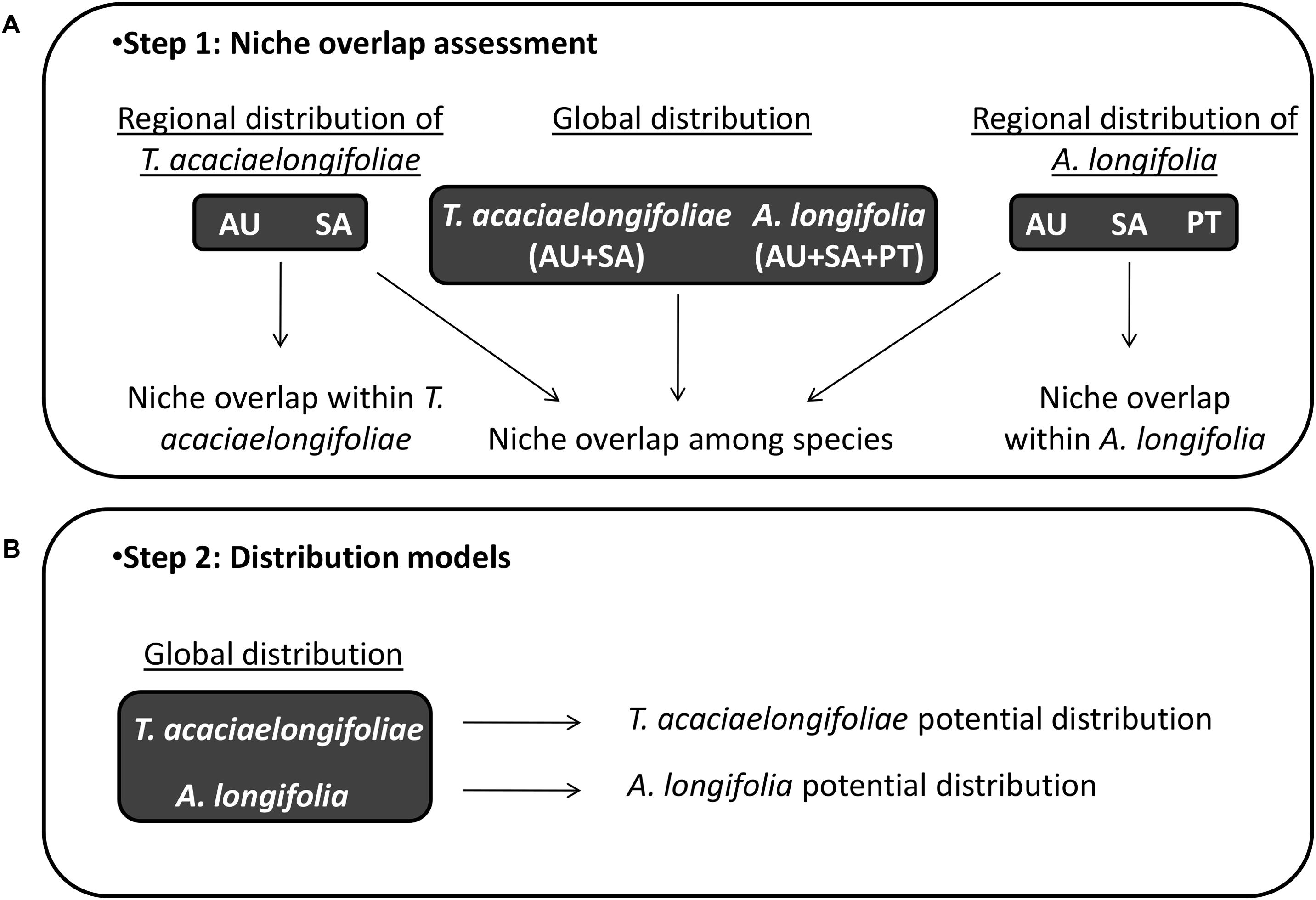
Figure 2. Workflow for niche overlap assessments and distribution modeling. Panel (A; top): Regional species distribution datasets were compared to assess niche conservatism for Acacia longifolia and Trichilogaster acaciaelongifoliae. Niche overlap among species was quantified (overlap), tested (equivalency and similarity), and characterized (stability, expansion, and unfilling) to assess ecological overlap in environmental space. Panel (B; bottom): Distribution modeling was used to project ecological overlap into geographic space and predict the potential ability of T. acaciaelongifoliae to establish in areas susceptible to A. longifolia invasion in the Mediterranean basin. Acronyms: AU: Australia, SA: South Africa, and PT: Portugal.
Niche Overlap
Ecological niches were compared between A. longifolia and T. acaciaelongifoliae, as well as between different native and introduced areas of each species’ distribution (Figure 2). For A. longifolia, niches were compared between the native range in Australia and the two invaded ranges in South Africa and Portugal. For T. acaciaelongifoliae, comparisons took into account only the native and introduced distributions in Australia and South Africa, respectively. All three distribution areas for A. longifolia were compared, to avoid assumptions about introduction pathways. Niches were compared in multivariate environmental space using the PCA-env technique (Broennimann et al., 2012). This approach allows for pairwise comparisons of niches between groups, and consists of three steps: (1) calculation of density of occurrences along a multivariate environmental space for both target groups; (2) measurement of the overlap between density distributions of the two groups in environmental space (niche overlap), as defined by Schoener’s D metric (Schoener, 1970); and (3) statistical tests of niche equivalency (whether niche overlap is constant when randomizing occurrences among both groups) and niche similarity (whether the niche of one group is more similar to the other than would be expected by chance given the available environmental background), using a permutation-based framework (Warren et al., 2008; Broennimann et al., 2012).
Representation of niches as densities along environmental space allows the quantification of differences in the densities of groups, which can be expressed as niche stability (proportion of niche B overlapping with niche A), niche expansion (proportion of niche B non-overlapping with niche A), and niche unfilling [unique proportion of niche A (i.e., non-overlapping with niche B); Guisan et al., 2014]. These metrics were developed to compare native and exotic niches, and represent the proportion (0–1) of the realized niche that remains constant (stability), is gained (expansion), or is lost (unfilling) during introduction/invasion. Application outside of an explicit comparison of native vs introduced ranges (such as when comparing two unrelated taxa like A. longifolia and T. acaciaelongifoliae) requires an arbitrary assignment of each group to either niche A or B, which will alter the order in which niche expansion and unfilling are calculated but will not affect the final results or interpretation. Background areas for each group were spatially delimited using the same areas used for calibration of niche models. For each pairwise comparison, background areas of the two groups under comparison were merged. Environmental space was defined by the 19 bioclimatic variables available from Worldclim v2.0 (Hijmans et al., 2005), at a resolution of 10 × 10 km. Though several of these variables are correlated in geographic space, the PCA-based approach addresses this by creating an orthogonal linear combination of the original variables. This approach allows the inclusion of all available bioclimatic variables, maximizing the environmental variance under consideration for niche assessments and minimizing assumptions about variable importance. Given what little is known about the ecology of T. acaciaelongifoliae, we opted to minimize such assumptions. Niche overlap was calculated and niche equivalency and similarity tests were performed among regions for each species, and across species. We also calculated metrics of niche stability, expansion and unfilling using the same framework. For all tests among species, A. longifolia was considered as group A and T. acaciaelongifoliae as group B. All analyses were performed in the R package ecospat (Di Cola et al., 2017).
Species Distribution Models
Based on the evidence for niche conservatism for both A. longifolia and T. acaciaelongifoliae (see Results section), species distribution models were calibrated using all available records for southeastern Australia, South Africa and (for A. longifolia) Portugal. This allows maximization of the number of occurrence records used to train the models and a better sampling of relevant environmental gradients. To avoid overparametrization, distribution models were developed using a subset of the bioclimatic variables used for niche overlap analysis. This approach precludes direct comparison between the two methods. Consequently, we treat the distribution models strictly as niche-based tools for predicting distributions rather than accurate representations of the niches, relying solely on the niche overlap tests for any inferences regarding the dynamics and relationships of niches. Spatial correlation between bioclimatic variables was assessed in ArcMap 10.1 (Environmental Systems Research Institute, 2012), and multicollinearity was assessed by calculating the Variance Inflation Factor (VIF) using the usdm package in R (Naimi et al., 2014). When two or more variables were highly correlated, preference was given to the one with the most biological sense. A set of five and six slightly correlated (Pearson’s r < 0.7) and non-collinear variables (VIF < 5; e.g., Vicente et al., 2013) were selected to create models for T. acaciaelongifoliae and A. longifolia, respectively, (Table 1). An ensemble modeling approach was performed in the R package biomod2 (Thuiller et al., 2009) using a combination of seven modeling techniques: Generalized Linear Models (GLM), Generalized Boosting Models (GBM), Flexible Discriminant Analysis (FDA), Classification Tree Analysis (CTA), Multivariate Adaptive Regression Splines (MARS), Random Forests (RF), and Maximum Entropy (MAXENT). Models were created using 5 datasets of 1000 pseudoabsences randomly distributed through the training areas, and 8 replicates were performed for each combination of pseudoabsence dataset and modeling algorithm, for a total of 280 models per species. Each model was calibrated setting aside 30% of available presence records for model evaluation. The resulting testing datasets were used to evaluate model discrimination by calculating the area under the curve of the receiver operating characteristic curve (AUC), and ensemble models across all modeling techniques were created for each species (e.g., Petitpierre et al., 2017), using the mean of all models where AUC > 0.7. Individual models were projected for the Mediterranean Basin and binary ensemble models were created using the Maximized Sum Threshold criteria (MST; Cantor et al., 1999), implemented in biomod2 under the “binary.meth = ‘ROC”’ argument (Thuiller et al., 2009). This method selects the threshold which maximizes the sum of sensitivity and specificity. We opted to use this approach due to its good general performance in comparative studies and particularly for its superior performance with low-prevalence datasets and ability to minimize omission errors, which are generally more costly in conservation applications such as identifying areas under risk of biological invasion (Liu et al., 2005; Jiménez-Valverde and Lobo, 2007). The importance of environmental variables for each model was determined by average permutation importance (Phillips et al., 2006).
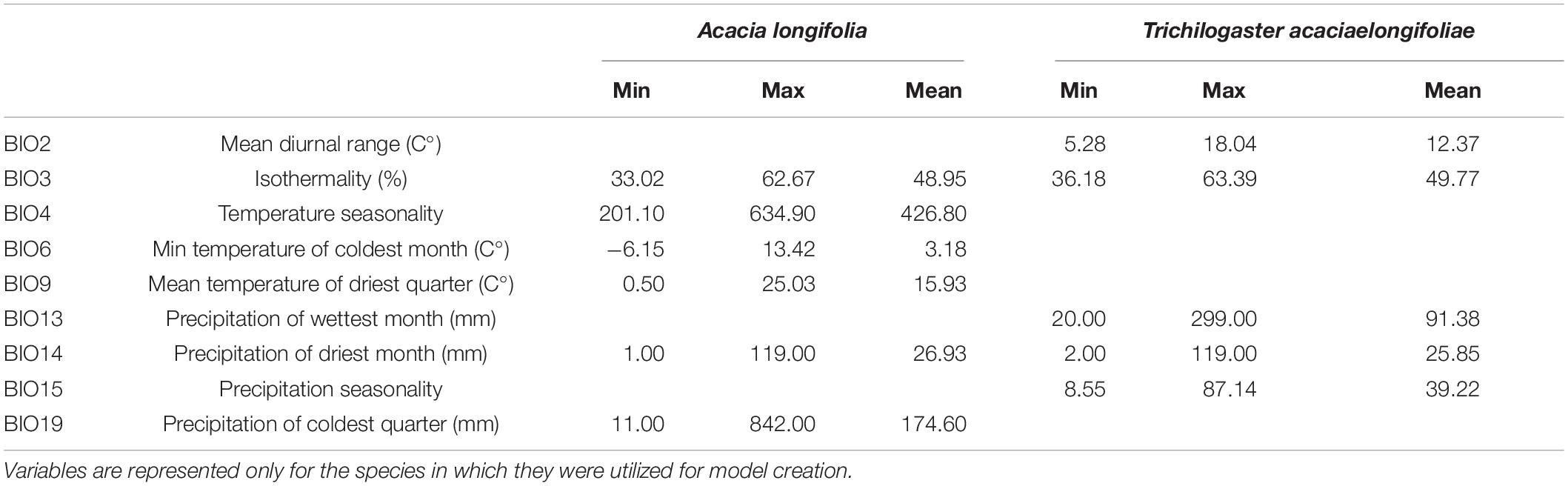
Table 1. Variable ID and minimum, maximum, and mean values across model training areas for Acacia longifolia and Trichilogaster acaciaelongifoliae of ecogeographical variables used in model creation.
Results
Niche Characterization
Niche overlap among A. longifolia was overall low between the three regions (less than 0.5, with 0 corresponding to no overlap and 1 to complete overlap), with the greatest overlap occurring between A. longifolia in South Africa and Portugal (0.416; Table 2). Niche equivalency, i.e., whether niche overlap remains constant when randomizing occurrences among groups, was found in all pairwise tests except for A. longifolia in Australia vs. Portugal and South Africa vs. Portugal. Niche similarity, i.e., whether groups are more similar than expected considering available background, was found in all pairwise tests. Similar niche dynamics were found for all A. longifolia comparisons, with predominant niche stability (>0.89), analogous niches across regions and very little expansion into novel areas of environmental space during introduction. Niche unfilling, i.e., areas of environmental space present in original range but not after introduction, ranged between 0.2 and 0.26 for all pairs except South Africa vs. Portugal, which had very little unfilling (<0.01). For T. acaciaelongifoliae in Australia vs. South Africa, niche unfilling was of similar magnitude to A. longifolia (0.25), but niche expansion was much more pronounced (0.56), with a corresponding decrease in niche stability (0.44; Table 2).
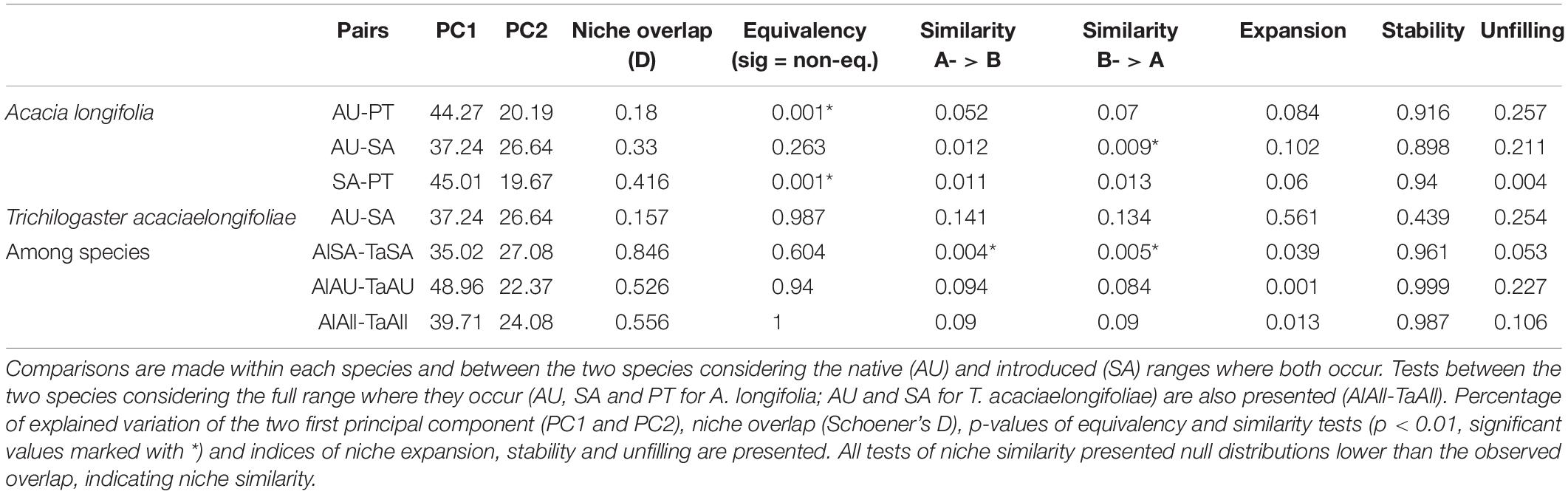
Table 2. Niche overlap and dynamics between all pairs of native (AU: Australia) and introduced (SA: South Africa; PT: Portugal) Acacia longifolia (Al) and Trichilogaster acaciaelongifoliae (Ta).
For comparisons between A. longifolia and T. acaciaelongifoliae, niche overlap ranged between 0.53 (in Australia) and 0.85 (in South Africa). The niches of the two species were found to be equivalent and similarity was not rejected in any pairwise test. Niche stability was higher than 0.96 in all comparisons, with corresponding low values of niche expansion from A. longifolia to T. acaciaelongifoliae (<0.04). Niche unfilling from A. longifolia to T. acaciaelongifoliae was lowest in South Africa (0.05) and highest in Australia (0.23; Table 2). All significant (p-value < 0.01) niche similarity tests corresponded to values of overlap greater than the distribution of pseudoreplicates, indicating that the groups are more similar than expected considering the available background.
Species Distribution Models
All 280 models per species had AUC values above 0.7 and were thus included in the ensembles. AUC values for A. longifolia models ranged between 0.79 and 0.94, with a weighted average of 0.90 ± 0.02 (±SD). AUC of models for T. acaciaelongifoliae ranged between 0.71 and 0.92, with a weighted average of 0.85 ± 0.04 (±SD; Table 3).

Table 3. Number of occurrence records (N), average (and SD) Area Under the Curve (AUC) and average (and SD) permutation importance of each ecogeographical variable across all 280 species distribution model replicates for Acacia longifolia and Trichilogaster acaciaelongifoliae. ∗Indicate highest contributing variables.
Temperature seasonality (BIO4), minimum temperature of the coldest month (BIO6), and precipitation of the coldest quarter (BIO19) were the most important variables for the A. longifolia models, with marginal contributions from the remaining variables. Variable importance for T. acaciaelongifoliae was less straightforward, with all variables contributing somewhat to the models, yet isothermality (BIO3) and mean diurnal range of temperature (BIO2) were the variables with the most explanatory power (Table 3).
Suitable areas for A. longifolia were predicted through the Atlantic coast of the Iberian Peninsula and Morocco, in some areas extending up to 280 km inland (Figure 3). Suitability for A. longifolia was also identified in northern Spain, southern France, some areas of the Italian and Balkan peninsulas (particularly along the Adriatic coast), most of the Mediterranean coast of North Africa and the Middle East and all Mediterranean archipelagos. T. acaciaelongifoliae is predicted to have suitable climatic conditions in most of the Iberian coastline (except for a gap of approximately 300 km in northern Portugal), the entirety of the coastline of Morocco, most of the Mediterranean areas of Libya, Egypt, Israel, and Palestine and areas in the Adriatic coast and in the islands of Cyprus, Sicily and the Aegean sea (Figure 3 and Supplementary Figure 1). In total, 19% of the total area of the Mediterranean basin was predicted to be suitable for A. longifolia, and 41% of the area identified as suitable for A. longifolia is also predicted as suitable for T. acaciaelongifoliae. Currently established population of T. acaciaelongifoliae were all correctly identified as suitable by the modeling approach used, with the exceptions of the population located in the city of Coimbra (Figure 3 and Supplementary Figure 1) and a few very recently observed populations in the northern coast (not shown).
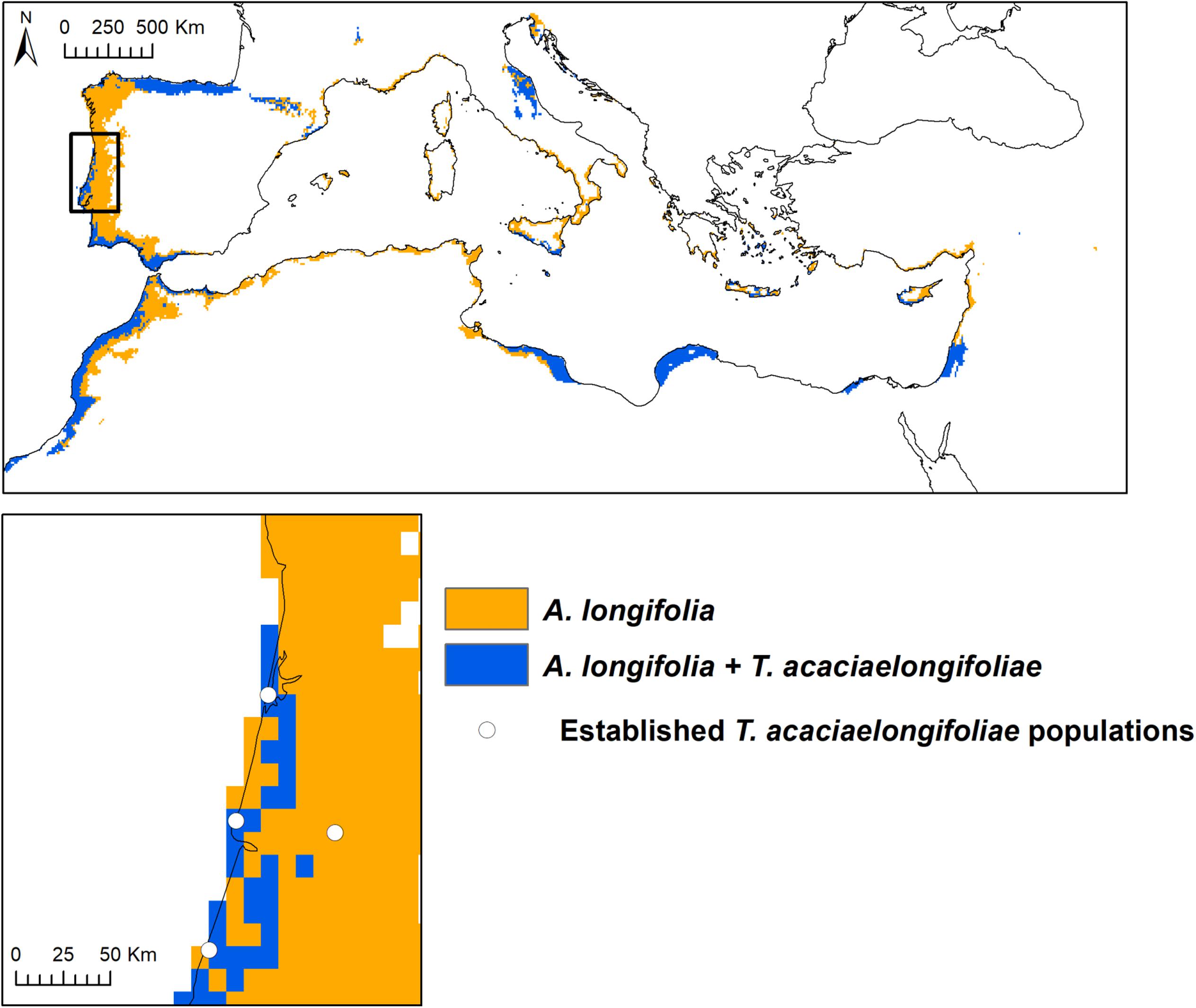
Figure 3. Spatial overlap between model predictions of suitability for Acacia longifolia and Trichilogaster acaciaelongifoliae in the Mediterranean region. Trichilogaster acaciaelongifoliae model was constrained to areas of predicted suitability for A. longifolia to prevent classification of suitability in areas where the species cannot persist due to lack of its host plant. Bottom: Populations of T. acaciaelongifoliae already established in Portugal as of 2018
Discussion
Niche Dynamics of A. longifolia and T. acaciaelongifoliae
While there are differences in niche dynamics between different ranges of A. longifolia and T. acaciaelongifoliae, the results are overall consistent with niche conservatism during the invasion/introduction process, respectively. Evidence for niche similarity on all cases suggests that observed differences in niches are related to differences in habitat availability and/or introduction pathways between Australia, South Africa and Portugal. Comparisons between Australia and South Africa revealed contrasting patterns for the two species, with A. longifolia invasion being associated with niche stability, and T. acaciaelongifoliae introduction being associated with strong niche expansion. It’s unlikely that this reflects actual expansion of T. acaciaelongifoliae into new environments in South Africa, as its dependency on A. longifolia would require the host plant to do the same, which is rejected by the evidence. Rather, non-climatic factors such as biotic interactions are likely to constrain the distribution of T. acaciaelongifoliae in Australia, where the species is native and as such suffers higher levels of parasitism and competes with other bud-feeding insects (Neser, 1984). Despite present, the lower level of such interactions in South Africa (Manongi and Hoffmann, 1995; Seymour and Veldtman, 2010) may have allowed the species to expand into previously unoccupied areas of niche space which are nonetheless contained within the realized niche of A. longifolia. The evidence for niche expansion may also reflect the low number of occurrence records for T. acaciaelongifoliae in Australia, which thus fail to fully represent the realized niche. Gall-forming insects, particularly in native regions where they coexist with their hosts often in equilibrium, are frequently overlooked and understudied organisms, despite the importance of several species as biocontrol agents (Dennill, 1988; Harris and Shorthouse, 1996) or pests (Gil-Tapetado et al., 2018; Ferracini et al., 2019). This resulted in a relatively reduced dataset in Australia, vulnerable to spatial biases, which are known to affect niche estimates (Graham et al., 2008; Sánchez-Fernández et al., 2011). Regardless, the evidence for niche conservatism allowed us to combine the T. acaciaelongifoliae occurrence records for Australia and South Africa for the development of niche models, minimizing the effect of spatial biases.
The very high niche overlap between A. longifolia and the gall-former T. acaciaelongifoliae is consistent with the high specificity of T. acaciaelongifoliae with its host plant (Dennill et al., 1993; Marchante et al., 2011a). The realized niche of T. acaciaelongifoliae is found to be almost fully contained within the niche of A. longifolia on all regions where the two species co-occur (reflected in high niche stability and near zero niche expansion). In South Africa, niches of the two species were found to be more similar than expected given the available environmental background and niche unfilling was found to be the lowest among all interspecies comparisons. This suggests a near total colonization by T. acaciaelongifoliae of all environments where A. longifolia occurs, which has been reported by some authors (J. Hoffmann, personal communication). It also indicates that the aforementioned expansion of T. acaciaelongifoliae in South Africa in the absence of substantial negative biotic interactions took place exclusively within the range of A. longifolia, highlighting the high specificity of T. acaciaelongifoliae which is a key factor in its reliability as a biocontrol agent. Australia had the lowest niche overlap and highest niche unfilling among all interspecies comparisons, highlighting that T. acaciaelongifoliae is not known to occur (or reported) in many of the environments where A. longifolia is present in its native range. Again, this likely reflects non-climatic influences hindering the ability of T. acaciaelongifoliae to successfully colonize all A. longifolia habitats.
Implications for the Management of A. longifolia Including Biocontrol
The predicted suitable area for A. longifolia in the Mediterranean basin far exceeds the current distribution. Besides environmental conditions, introduction histories are known to greatly influence species distributions (Donaldson et al., 2014). One of the main pathways for A. longifolia introduction in Portugal was for sand binding of coastal dunes (Marchante et al., 2003). This coastal introduction, associated with habitat connectivity and dispersal constraints, may account for the current species distribution which is not known to extend as far inland as predicted by our model (with a few exceptions in some particular locations). This hypothesis seems likely when considering that A. longifolia (sensu lato) is known to occur much further inland in its native distribution than in Portugal (Court et al., 2020). Likewise, in South Africa, where A. longifolia was introduced also to stabilize inland slopes (Dennill and Donnelly, 1991), the species is present as far inland as 500 km, demonstrating that it can become established far from coastal climate given the adequate means of dispersal. Regardless, we cannot dismiss the possibility that factors not included in the models, such as biotic interactions or non-climatic abiotic variables, may constrain the distribution of A. longifolia beyond the model’s prediction. The current model predicts risk of A. longifolia invasion in most of the Mediterranean and, especially, Atlantic coastline of the Mediterranean Basin region. Areas of current A. longifolia invasion in the region besides Portugal include north-western Spain (Community of Galicia), which is correctly predicted as climatically suitable. A. longifolia is currently present, even if not yet considered as invasive, in several countries which are predicted as having suitable areas for the establishment of the species, namely France, Italy, Greece and Turkey (Akanil and Middleton, 2010; EFSA Panel on Plant Health, 2015). Our results suggest that particular care should be taken to prevent A. longifolia invasion in these regions where the species is already present within an area of suitable environment. The model for A. longifolia identified a small area of suitability in the southern Pyrenees Mountains, a region characterized by montane conditions that are uncharacteristic for the species. Species distribution models have a limited ability to extrapolate beyond the environmental conditions available in the training area and may produce unexpected results when environmental homology between training and projection areas is not assured (Elith and Leathwick, 2009). Despite our efforts to maximize homology by restricting the model projections to the Mediterranean Basin, mountain ranges in the region are likely to include extreme conditions which lead to extrapolation, resulting in less reliable predictions in these areas.
Trichilogaster acaciaelongifoliae is predicted to find suitable climate in a significant part of the potential A. longifolia distribution area. Current areas of A. longifolia invasion where T. acaciaelongifoliae is not predicted to find suitable conditions are located in the coastline of northern Portugal and Galicia, as well in the most inland areas of Portugal. Nevertheless, the existence of a well-established population of T. acaciaelongifoliae in the Portuguese city of Coimbra and very recent (2019; López-Núñez et al., in prep.) establishment in northern areas of Portugal (classified as unsuitable by the model) indicates that the approach used in this work may not be sufficient to fully characterize the niche of this species. Partial inconsistencies amongst modeling outputs and field observations occur in other studies (e.g., Gallien et al., 2012; Fischbein et al., 2019) for several reasons. In our study, agreement between model predictions and known distributions in the Mediterranean Basin was higher for A. longifolia than for T. acaciaelongifoliae, likely reflecting the difference in number and quality of occurrence records between the two species. Moreover, this may be explained by the fact that the biocontrol agent was only recently (2015) introduced in Portugal (Marchante et al., 2017), still not having enough time to spread and establish across the suitable area. Furthermore, the life history of T. acaciaelongifoliae is characterized by a near-total existence as an endoparasite, and as such the microenvironmental conditions and interactions with the host plant are likely to be better predictors of the species’ ability to form viable populations than macroclimatic factors. Despite the identification of ecogeographical variables that have a limiting effect on T. acaciaelongifoliae, the environmental triggers required for completion of this species’ life cycle are still poorly understood. T. acaciaelongifoliae has shown to be sensitive to such triggers, as the change in hemisphere (and consequent temporal mismatch between the species’ life cycle and seasonal queues) upon introduction to Portugal resulted in low establishment success in the first years (Marchante et al., 2017, López-Núñez et al., in prep.). Identifying these triggers is fundamental to properly assess the long-term robustness of effective biocontrol of A. longifolia mediated by T. acaciaelongifoliae. Ultimately, mechanistic approaches to modeling which integrate microhabitat and biotic interactions between host and parasite as well as an explicit understanding of how these factors contribute to the life cycle of T. acaciaelongifoliae (Palhas et al., in prep.) are expected to better approximate the fundamental niche and, when integrated with correlative approaches such as the one presented in this work, allow for more accurate predictions.
Despite the limitations described above, the high niche overlap between the two species shows promise regarding the effectiveness of T. acaciaelongifoliae for biocontrol of A. longifolia in other invaded areas worldwide. Particularly in New Zealand, where such a program is being considered (Northland Conservancy Department of Conservation, 2005; Northland Regional Council, 2019), distribution modeling approaches such as the one presented here may offer insight on whether and where biocontrol has a chance to be effective.
Conclusion
Predictive modeling is widely used for the prevention and management of biological invasions. The ability to predict invasion risk beforehand enables the application of preventive measures which are generally more cost-effective than attempting to eradicate already established invasive populations (Rejmánek et al., 2013). We have developed a predictive mapping of suitable areas for A. longifolia establishment in the Mediterranean basin, from which several areas potentially under invasion risk were identified in southern Europe. These areas represent priority targets for preventive measures in order to prevent future invasions. However, areas predicted as suitable are unlikely to share a similar risk of invasion. We uncovered a likely important role of introduction pathways in shaping the current distribution of A. longifolia across its invasive range. Understanding landscape permeability to dispersal and identifying dispersal corridors, which can be accomplished by connectivity analysis (e.g., Gonçalves et al., 2016) can potentially improve risk mapping.
Distribution models and assessments of ecological overlap are not frequently used to inform the implementation of biocontrol programs. The potential of these approaches for quantifying the adequacy of a biocontrol agent in terms of ecological overlap with the target species and ability to establish in target areas should not, however, be underestimated. Despite the limited data available for T. acaciaelongifoliae, we were able to identify substantial overlap between the biocontrol agent and the target invasive plant. We also uncovered an important role of biotic interactions constraining the realized distribution of T. acaciaelongifoliae in its native range. In the absence of such interactions in the introduced range, the species may be able to explore previously unavailable parts of its fundamental niche, potentially being able to colonize even more of the distribution of A. longifolia than predicted by the current model. These are promising predictions for the future of A. longifolia biocontrol in Portugal and in the Mediterranean basin, but also in other regions dealing with A. longifolia invasions.
Data Availability Statement
All datasets presented in this study are included in the article/Supplementary Material.
Author Contributions
All authors contributed to the study design. MD and NC collected the data. MD performed the analyses with important contributions from JRV, NC, and FL-N. MD wrote the manuscript with important contributions from all authors.
Funding
This work was supported by European funds FEDER and Portugal 2020/POCI and national funds by Portuguese Foundation for Science and Technology through project INVADER-IV (PTDC/AAG-REC/4896/2014) and CFE-Centre for Functional Ecology-Science for People & the Planet’s Strategic Plan (UIDB/04004/2020). FL-N is supported by FCT Ph.D. grant SFRH/BD/130942/2017. JRV was supported as a post-doc researcher at ICETA CIBIO/InBIO by national funds through FCT – Foundation for Science and Technology, DL57/2016/ICETA/EEC2018/13.
Conflict of Interest
The authors declare that the research was conducted in the absence of any commercial or financial relationships that could be construed as a potential conflict of interest.
Acknowledgments
The authors would like to thank Alan Wood, Kristine French, Tony Rebelo, Pride Mudavanhu, and Andy Sheppard for providing occurrence records.
Supplementary Material
The Supplementary Material for this article can be found online at: https://www.frontiersin.org/articles/10.3389/fevo.2020.576667/full#supplementary-material
Footnotes
- ^ https://www.gbif.org/en/
- ^ https://www.ala.org.au/
- ^ http://www.samuseum.sa.gov.au/
- ^ https://www.csiro.au/en/Research/Collections/ANIC
- ^ http://www.invasoras.pt
- ^ http://www.worldclim.org
References
Akanil, N., and Middleton, B. (2010). Leaf litter decomposition along the Porsuk River. Eskisehir, Turkey. Can. J. Bot. 75, 1394–1397. doi: 10.1139/b97-853
Anderson, R. P., and Raza, A. (2010). The effect of the extent of the study region on GIS models of species geographic distributions and estimates of niche evolution: preliminary tests with montane rodents (genus Nephelomys) in Venezuela. J. Biogeogr. 37, 1378–1393. doi: 10.1111/j.1365-2699.2010.02290.x
Barve, N., Barve, V., Jiménez-Valverde, A., Lira-Noriega, A., Maher, S. P., Peterson, A. T., et al. (2011). The crucial role of the accessible area in ecological niche modeling and species distribution modeling. Ecol. Modell. 222, 1810–1819. doi: 10.1016/j.ecolmodel.2011.02.011
Broennimann, O., Fitzpatrick, M. C., Pearman, P. B., Petitpierre, B., Pellissier, L., Yoccoz, N. G., et al. (2012). Measuring ecological niche overlap from occurrence and spatial environmental data. Glob. Ecol. Biogeogr. 21, 481–497. doi: 10.1111/j.1466-8238.2011.00698.x
Broennimann, O., Treier, U. A., Müller-Schärer, H., Thuiller, W., Peterson, A. T., and Guisan, A. (2007). Evidence of climatic niche shift during biological invasion. Ecol. Lett. 10, 701–709. doi: 10.1111/j.1461-0248.2007.01060.x
Cantor, S. B., Sun, C. C., Tortolero-Luna, G., Richards-Kortum, R., and Follen, M. (1999). A Comparison of C/B ratios from studies using receiver operating characteristic curve analysis. J. Clin. Epidemiol. 52, 885–892. doi: 10.1016/S0895-4356(99)00075-X
Clewley, G. D., Eschen, R., Shaw, R. H., and Wright, D. J. (2012). The effectiveness of classical biological control of invasive plants. J. Appl. Ecol. 49, 1287–1295. doi: 10.1111/j.1365-2664.2012.02209.x
Costello, D. A., Lunt, I. D., and Williams, J. E. (2000). Effects of invasion by the indigenous shrub Acacia sophorae on plant composition of coastal grasslands in south-eastern Australia. Biol. Conserv. 96, 113–121. doi: 10.1016/S0006-3207(00)00058-6
Court, A. B., Maslin, B. R., and Kodela, P. G. (2020). “Acacia longifolia,” in Flora of Australia, ed. P. G. Kodela (Canberra: Australian Biological Resources Study).
Dennill, G. B. (1985). The effect of the gall wasp Trichilogaster acaciaelongifoliae (Hymenoptera:Pteromalidae) on reproductive potential and vegetative growth of the weed Acacia longifolia. Agric. Ecosyst. Environ 14, 53–61. doi: 10.1016/0167-8809(85)90084-2
Dennill, G. B. (1987). Establishment of the Gall Wasp Trichilogaster acaciaelongifoliae (Pteromalidae) for the biological control of Acacia longifolia in South Africa. Agric. Ecosyst. Environ. 19, 155–168. doi: 10.1016/0167-8809(87)90015-6
Dennill, G. B. (1988). Why a gall former can be a good biocontrol agent: the gall wasp Trichilogaster acaciaelongifoliae and the weed Acacia longifolia. Ecol. Entomol. 13, 1–9. doi: 10.1111/j.1365-2311.1988.tb00327.x
Dennill, G. B. (1990). The contribution of a successful biocontrol project to the theory of agent selection in weed biocontrol—the gall wasp Trichilogaster acaciaelongifoliae and the weed Acacia longifolia. Agric. Ecosyst. Environ. 31, 147–154. doi: 10.1016/0167-8809(90)90216-Z
Dennill, G. B., and Donnelly, D. (1991). Biological control of Acacia longifolia and related weed species (Fabaceae) in South Africa. Agric. Ecosyst. Environ. 37, 115–135. doi: 10.1016/0167-8809(91)90142-K
Dennill, G. B., Donnelly, D., and Chown, S. L. (1993). Expansion of host-plant range of a biocontrol agent Trichilogaster acaciaelongifoliae (Pteromalidae) released against the weed Acacia longifolia in South Africa. Agric. Ecosyst. Environ. 43, 1–10. doi: 10.1007/s10971-012-2863-y
Dennill, G. B., and Gordon, A. J. (1990). Climate-related differences in the efficacy of the Australian gall wasp Trichilogaster acaciaelongifolia (Hymenoptera: Pteromalidae) released for the control of Acacia longifolia in South Africa. Environ. Entomol. 19, 130–136. doi: 10.1093/ee/19.1.130
Di Cola, V., Broennimann, O., Petitpierre, B., Breiner, F. T., D’Amen, M., Randin, C., et al. (2017). ecospat: an R package to support spatial analyses and modeling of species niches and distributions. Ecography 40, 1–14. doi: 10.1111/ecog.02671
Dinis, M., Merabet, K., Martínez-Freiría, F., Steinfartz, S., Vences, M., Burgon, J. D., et al. (2019). Allopatric diversification and evolutionary melting pot in a North African Palearctic relict: the biogeographic history of Salamandra algira. Mol. Phylogenet. Evol. 130, 81–91. doi: 10.1016/j.ympev.2018.10.018
Donaldson, J. E., Hui, C., Richardson, D. M., Robertson, M. P., Webber, B. L., and Wilson, J. R. U. (2014). Invasion trajectory of alien trees: the role of introduction pathway and planting history. Glob. Chang. Biol. 20, 1527–1537. doi: 10.1111/gcb.12486
EFSA Panel on Plant Health (2015). Risk to plant health in the EU territory of the intentional release of the bud-galling wasp Trichilogaster acaciaelongifoliae for the control of the invasive alien plant Acacia longifolia. EFSA J. 13:4079. doi: 10.2903/j.efsa.2015.4079
Elith, J., and Leathwick, J. R. (2009). Species distribution models: ecological explanation and prediction across space and time. Annu. Rev. Ecol. Evol. Syst. 40, 677–697. doi: 10.1146/annurev.ecolsys.110308.120159
Environmental Systems Research Institute (2012). ArcGIS 10.1. Redlands, CA: Environmental Systems Research Institute.
Ferracini, C., Ferrari, E., Pontini, M., Saladini, M. A., and Alma, A. (2019). Effectiveness of Torymus sinensis: a successful long-term control of the Asian chestnut gall wasp in Italy. J. Pest Sci. 92, 353–359. doi: 10.1007/s10340-018-0989-6
Fischbein, D., Lantschner, M. V., and Corley, J. C. (2019). Modelling the distribution of forest pest natural enemies across invaded areas: towards understanding the influence of climate on parasitoid establishment success. Biol. Control 132, 177–188. doi: 10.1016/j.biocontrol.2019.02.016
Gallien, L., Douzet, R., Pratte, S., Zimmermann, N. E., and Thuiller, W. (2012). Invasive species distribution models - how violating the equilibrium assumption can create new insights. Glob. Ecol. Biogeogr. 21, 1126–1136. doi: 10.1111/j.1466-8238.2012.00768.x
Gil-Tapetado, D., Gómez, J. F., Cabrero-Sañudo, F. J., and Nieves-Aldrey, J. L. (2018). Distribution and dispersal of the invasive Asian chestnut gall wasp, Dryocosmus kuriphilus (Hymenoptera: Cynipidae), across the heterogeneous landscape of the Iberian Peninsula. Eur. J. Entomol. 115, 575–586. doi: 10.14411/eje.2018.055
Gonçalves, J., Honrado, J. P., Vicente, J. R., and Civantos, E. (2016). A model-based framework for assessing the vulnerability of low dispersal vertebrates to landscape fragmentation under environmental change. Ecol. Complex 28, 174–186. doi: 10.1016/j.ecocom.2016.05.003
Graham, C. H., Elith, J., Hijmans, R. J., Guisan, A., Townsend Peterson, A., Loiselle, B. A., et al. (2008). The influence of spatial errors in species occurrence data used in distribution models. J. Appl. Ecol. 45, 239–247. doi: 10.1111/j.1365-2664.2007.01408.x
Guisan, A., Petitpierre, B., Broennimann, O., Daehler, C., and Kueffer, C. (2014). Unifying niche shift studies: insights from biological invasions. Trends Ecol. Evol. 29, 260–269. doi: 10.1016/j.tree.2014.02.009
Gutiérrez-Rodríguez, J., Barbosa, A. M., and Martínez-Solano, Í (2017). Integrative inference of population history in the Ibero-Maghrebian endemic Pleurodeles waltl (Salamandridae). Mol. Phylogenet. Evol. 112, 122–137. doi: 10.1016/j.ympev.2017.04.022
Harris, P., and Shorthouse, J. D. (1996). Effectiveness of gall inducers in weed biological control. Can. Entomol. 128, 1021–1055. doi: 10.4039/Ent1281021-6
Henderson, L. (1999). The southern african plant invaders atlas (SAPIA) and its contribution to biological weed control. Afr. Entomol. Mem. 1, 159–163.
Henriksen, M. V., Chapple, D. G., Chown, S. L., and McGeoch, M. A. (2017). Gall wasp biocontrol of invasive Acacia longifolia: implications of strong bottom-up effects. Ecosphere 8:e02043. doi: 10.1002/ecs2.2043
Henriksen, M. V., Chapple, D. G., Chown, S. L., and McGeoch, M. A. (2019). The effect of network size and sampling completeness in depauperate networks. J. Anim. Ecol. 88, 211–222. doi: 10.1111/1365-2656.12912
Hijmans, R. J., Cameron, S. E., Parra, J. L., Jones, P. G., and Jarvis, A. (2005). Very high resolution interpolated climate surfaces for global land areas. Int. J. Climatol. 25, 1965–1978. doi: 10.1002/joc.1276
Impson, F. A. C., Kleinjan, C. A., Hoffmann, J. H., Post, J. A., and Wood, A. R. (2011). Biological control of australian Acacia species and Paraserianthes lophantha (Willd.) Nielsen (Mimosaceae) in South Africa. African Entomol. 19, 186–207. doi: 10.4001/003.019.0210
IPBES (2019). “Global assessment report on biodiversity and ecosystem services,” in Intergovernmental Science-Policy Platform on Biodiversity and Ecosystem Services, eds E. S. Brondizio, J. Settele, S. Díaz, and H. T. Ngo Bonn (Germany: IPBES Secretariat).
Jiménez-Valverde, A., and Lobo, J. M. (2007). Threshold criteria for conversion of probability of species presence to either–or presence–absence. Acta Oecol. 31, 361–369. doi: 10.1016/j.actao.2007.02.001
Köppen, W. (1900). Versuch einer Klassifikation der Klimate, vorzugsweise nach ihren Beziehungen zur Pflanzenwelt. Geogr. Z. 6, 593–611.
Lado, T. F. (2008). Molecular Ecology of Introduced Species in South Africa: The Bud Gall-Forming Wasp Trichilogaster acaciaelongifoliae and the Argentine ant Linepithema humile. PhD Thesis, Stellenbosch University, Stellenbosch.
Le Maitre, D. C., Gaertner, M., Marchante, E., Ens, E. J., Holmes, P. M., Pauchard, A., et al. (2011). Impacts of invasive Australian acacias: implications for management and restoration. Divers. Distrib. 17, 1015–1029. doi: 10.1111/j.1472-4642.2011.00816.x
Liu, C., Berry, P. M., Dawson, T. P., and Pearson, R. G. (2005). Selecting thresholds of occurrence in the prediction of species distributions. Ecography 28, 385–393. doi: 10.1111/j.0906-7590.2005.03957.x
López-Núñez, F. A., Heleno, R. H., Ribeiro, S., Marchante, H., and Marchante, E. (2017). Four-trophic level food webs reveal the cascading impacts of an invasive plant targeted for biocontrol. Ecology 98, 782–793. doi: 10.1002/ecy.1701
Lorenzo, P., González, L., and Reigosa, M. J. (2010). The genus Acacia as invader: the characteristic case of Acacia dealbata Link in Europe. Ann. For. Sci. 67:101. doi: 10.1051/forest/2009082
Manongi, F. S., and Hoffmann, J. H. (1995). The incidence of parasitism in Trichilogaster acaciaelongifoliae (Froggatt) (Hymenoptera: Pteromalidae), a gall-forming biological control agent of Acacia longifolia (Andr.) Willd. (Fabaceae) in South Africa. African Entomol. 3, 147–151.
Marais, C., Van Wilgen, B. W., and Stevens, D. (2004). The clearing of invasive alien plants in South Africa: a preliminary assessment of costs and progress. S. Afr. J. Sci. 100, 97–103. doi: 10.1016/j.cep.2005.08.005
Marchante, E., Kjøller, A., Struwe, S., and Freitas, H. (2008a). Invasive Acacia longifolia induce changes in the microbial catabolic diversity of sand dunes. Soil Biol. Biochem. 40, 2563–2568. doi: 10.1016/j.soilbio.2008.06.017
Marchante, E., Kjøller, A., Struwe, S., and Freitas, H. (2008b). Short- and long-term impacts of Acacia longifolia invasion on the belowground processes of a Mediterranean coastal dune ecosystem. Appl. Soil Ecol. 40, 210–217. doi: 10.1016/j.apsoil.2008.04.004
Marchante, E., Kjøller, A., Struwe, S., and Freitas, H. (2009). Soil recovery after removal of the N2-fixing invasive Acacia longifolia: consequences for ecosystem restoration. Biol. Invasions 11, 813–823. doi: 10.1007/s10530-008-9295-1
Marchante, H., Freitas, H., and Hoffmann, J. H. (2011a). Assessing the suitability and safety of a well-known bud-galling wasp, Trichilogaster acaciaelongifoliae, for biological control of Acacia longifolia in Portugal. Biol. Control 56, 193–201. doi: 10.1016/j.biocontrol.2010.11.001
Marchante, H., Freitas, H., and Hoffmann, J. H. (2011b). Post-clearing recovery of coastal dunes invaded by Acacia longifolia: is duration of invasion relevant for management success? J. Appl. Ecol. 48, 1295–1304. doi: 10.1111/j.1365-2664.2011.02020.x
Marchante, H., López-Núñez, F. A., Freitas, H., Hoffmann, J. H., Impson, F., and Marchante, E. (2017). First report of the establishment of the biocontrol agent Trichilogaster acaciaelongifoliae for control of invasive Acacia longifolia in Portugal. EPPO Bull. 47, 274–278. doi: 10.1111/epp.12373
Marchante, H., Marchante, E., and Freitas, H. (2003). “Invasion of the Portuguese dune ecosystems by the exotic species Acacia longifolia (Andrews) Willd.: effects at the community level,” in Plant invasions: Ecological Threats and Management Solutions, eds L. E. Child, J. H. Brock, G. Brundu, K. Prach, P. Pysek, P. M. Wade, et al. (Netherlands: Backhuys Publishers), 75–85.
Marchante, H., Marchante, E., Freitas, H., and Hoffmann, J. H. (2015). Temporal changes in the impacts on plant communities of an invasive alien tree. Acacia longifolia. Plant Ecol. 216, 1481–1498. doi: 10.1007/s11258-015-0530-4
Naimi, B., Hamm, N. A. S., Groen, T. A., Skidmore, A. K., and Toxopeus, A. G. (2014). Where is positional uncertainty a problem for species distribution modelling? Ecography 37, 191–203. doi: 10.1111/j.1600-0587.2013.00205.x
Neser, S. (1984). “A most promising bud-galling wasp, Trichilogaster acacialongifoliae (Pteromalidae), established against Acacia longifolia,” in Proceedings of the VI International Symposium on Biological Control of Weeds, Vancouver: University of British Columbia, 797–803.
Northland Conservancy Department of Conservation (2005). Prospects for the Biological Control of Sydney Golden Wattle, Acacia Longifolia, Using Trichilogaster acaciaelongifoliae and Melanterius ventralis (No. LC0506/009). New Zealand: Lincoln.
Northland Regional Council (2019). Invertebrates Associated With Acacia Longifoliae Andr. (Willd.) (Leguminosaae) in Northland and Horowhenua. New Zealand: Lincoln.
Passos, I., Marchante, H., Pinho, R., and Marchante, E. (2017). What we don’t seed: the role of long-lived seed banks as hidden legacies of invasive plants. Plant Ecol. 218, 1313–1324. doi: 10.1007/s11258-017-0770-6
Peterson, A. T., Soberón, J., Pearson, R. G., Anderson, R. P., Martínez-Meyer, E., Nakamura, M., et al. (2011). Ecological Niches and Geographic Distributions. Princeton: Oxford, doi: 10.5860/CHOICE.49-6266
Petitpierre, B., Broennimann, O., Kueffer, C., Daehler, C., and Guisan, A. (2017). Selecting predictors to maximize the transferability of species distribution models: lessons from cross-continental plant invasions. Glob. Ecol. Biogeogr. 26, 275–287. doi: 10.1111/geb.12530
Petitpierre, B., Kueffer, C., Broennimann, O., Randin, C., Daehler, C., and Guisan, A. (2012). Climatic niche shifts are rare among terrestrial plant invaders. Science 335, 1344–1348. doi: 10.1126/science.1215933
Phillips, S. J., Anderson, R. P., and Schapire, R. E. (2006). Maximum entropy modeling of species geographic distributions. Ecol. Modell. 190, 231–259. doi: 10.1016/j.ecolmodel.2005.03.026
Prinsloo, G., and Neser, O. (2007). Revision of the pteromalid wasp genus Trichilogaster Mayr (Hymenoptera: Chalcidoidea): gall-inducers on Australian acacias. African Entomol. 15, 161–184. doi: 10.4001/1021-3589-15.1.161
Rejmánek, M., Richardson, D. M., and Pyšek, P. (2013). “Plant Invasions and Invasibility of Plant Communities,” in Vegetation Ecology, eds E. van der Maarel and J. Franklin (Oxford: John Wiley & Sons, Ltd), 387–424. doi: 10.1002/9781118452592.ch13
Richardson, D. M., and Kluge, R. L. (2008). Seed banks of invasive Australian Acacia species in South Africa: role in invasiveness and options for management. Perspect. Plant Ecol. Evol. Syst. 10, 161–177. doi: 10.1016/j.ppees.2008.03.001
Sánchez-Fernández, D., Lobo, J. M., and Hernández-Manrique, O. L. (2011). Species distribution models that do not incorporate global data misrepresent potential distributions: a case study using Iberian diving beetles. Divers. Distrib. 17, 163–171. doi: 10.1111/j.1472-4642.2010.00716.x
Schoener, T. W. (1970). Nonsynchronous spatial overlap of lizards in patchy habitats. Ecology 51, 408–418. doi: 10.2307/1935376
Seymour, C. L., and Veldtman, R. (2010). Ecological role of control agent, and not just host-specificity, determine risks of biological control. Austral Ecol. 35, 704–711. doi: 10.1111/j.1442-9993.2009.02077.x
Shaw, R. H., Ellison, C. A., Marchante, H., Pratt, C. F., Schaffner, U., Sforza, R. F. H., et al. (2018). Weed biological control in the European Union: from serendipity to strategy. BioControl 63, 333–347. doi: 10.1007/s10526-017-9844-6
Simberloff, D., Martin, J.-L., Genovesi, P., Maris, V., Wardle, D. A., Aronson, J., et al. (2013). Impacts of biological invasions: what’s what and the way forward. Trends Ecol. Evol. 28, 58–66. doi: 10.1016/j.tree.2012.07.013
Sun, Y., Broennimann, O., Roderick, G. K., Poltavsky, A., Lommen, S. T. E., and Müller-Schärer, H. (2017). Climatic suitability ranking of biological control candidates: a biogeographic approach for ragweed management in Europe. Ecosphere 8:e01731. doi: 10.1002/ecs2.1731
Thuiller, W., Lafourcade, B., Engler, R., and Araújo, M. B. (2009). BIOMOD - a platform for ensemble forecasting of species distributions. Ecography 32, 369–373. doi: 10.1111/j.1600-0587.2008.05742.x
Vale, C. G., Campos, J. C., Silva, T. L., Gonçalves, D. V., Sow, A. S., Martínez-Freiría, F., et al. (2016). Biogeography and conservation of mammals from the west sahara-sahel: an application of ecological niche-based models and GIS. Hystrix, Ital. J. Mammol. 27, 1–10. doi: 10.4404/hystrix-27.1-11659
VanDerWal, J., Shoo, L. P., Graham, C., and Williams, S. E. (2009). Selecting pseudo-absence data for presence-only distribution modeling: how far should you stray from what you know? Ecol. Modell. 220, 589–594. doi: 10.1016/j.ecolmodel.2008.11.010
Vicente, J. R., Alagador, D., Guerra, C., Alonso, J. M., Kueffer, C., Vaz, A. S., et al. (2016). Cost-effective monitoring of biological invasions under global change: a model-based framework. J. Appl. Ecol. 53, 1317–1329. doi: 10.1111/1365-2664.12631
Vicente, J. R., Alves, P., Randin, C., Guisan, A., and Honrado, J. (2010). What drives invasibility? A multi-model inference test and spatial modelling of alien plant species richness patterns in northern Portugal. Ecography 33, 1081–1092. doi: 10.1111/j.1600-0587.2010.6380.x
Vicente, J. R., Fernandes, R. F., Randin, C. F., Broennimann, O., Gonçalves, J., Marcos, B., et al. (2013). Will climate change drive alien invasive plants into areas of high protection value? An improved model-based regional assessment to prioritise the management of invasions. J. Environ. Manag. 131, 185–195. doi: 10.1016/j.jenvman.2013.09.032
Vicente, J. R., Randin, C. F., Gonçalves, J., Metzger, M. J., Lomba, Â, Honrado, J., et al. (2011). Where will conflicts between alien and rare species occur after climate and land-use change? A test with a novel combined modelling approach. Biol. Invasions 13, 1209–1227. doi: 10.1007/s10530-011-9952-7
Vilà, M., Espinar, J. L., Hejda, M., Hulme, P. E., Jarošík, V., Maron, J. L., et al. (2011). Ecological impacts of invasive alien plants: a meta-analysis of their effects on species, communities and ecosystems. Ecol. Lett. 14, 702–708. doi: 10.1111/j.1461-0248.2011.01628.x
Warren, D. L., Glor, R. E., and Turelli, M. (2008). Environmental niche equivalency versus conservatism: quantitative approaches to niche evolution. Evolution 62, 2868–2883. doi: 10.1111/j.1558-5646.2008.00482.x
Werner, C., Zumkier, U., Beyschlag, W., and Máguas, C. (2010). High competitiveness of a resource demanding invasive acacia under low resource supply. Plant Ecol. 206, 83–96. doi: 10.1007/s11258-009-9625-0
Wilson, J. R. U., Gairifo, C., Gibson, M. R., Arianoutsou, M., Bakar, B. B., Baret, S., et al. (2011). Risk assessment, eradication, and biological control: global efforts to limit Australian acacia invasions. Divers. Distrib. 17, 1030–1046. doi: 10.1111/j.1472-4642.2011.00815.x
Keywords: biological invasions, biocontrol, distribution modeling, niche conservatism, Acacia longifolia, Trichilogaster acaciaelongifoliae
Citation: Dinis M, Vicente JR, César de Sá N, López-Núñez FA, Marchante E and Marchante H (2020) Can Niche Dynamics and Distribution Modeling Predict the Success of Invasive Species Management Using Biocontrol? Insights From Acacia longifolia in Portugal. Front. Ecol. Evol. 8:576667. doi: 10.3389/fevo.2020.576667
Received: 26 June 2020; Accepted: 25 September 2020;
Published: 19 October 2020.
Edited by:
Rubén G. Mateo, Autonomous University of Madrid, SpainReviewed by:
Aitor Gastón, Polytechnic University of Madrid, SpainTiago Vasconcelos, São Paulo State University, Brazil
Copyright © 2020 Dinis, Vicente, César de Sá, López-Núñez, Marchante and Marchante. This is an open-access article distributed under the terms of the Creative Commons Attribution License (CC BY). The use, distribution or reproduction in other forums is permitted, provided the original author(s) and the copyright owner(s) are credited and that the original publication in this journal is cited, in accordance with accepted academic practice. No use, distribution or reproduction is permitted which does not comply with these terms.
*Correspondence: Marco Dinis, ZGluaXNtYXJjbzZAZ21haWwuY29t; Joana R. Vicente, anN2aWNlbnRlQGZjLnVwLnB0; Elizabete Marchante, ZW1hcmNoYW50ZUB1Yy5wdA==; Hélia Marchante, aG1hcmNoYW50ZUBnbWFpbC5jb20=