- Grupo Ecoloxía Animal (Lab 97), Torre CACTI (Centro de Apoyo Científico y Tecnológico a la Investigación), Centro de Investigación Mariña, Campus de Vigo, Universidade de Vigo, Vigo, Spain
Early environment often has profound effects on phenotypic development that last throughout the lifetime. It has been suggested that unpredictable environments may favor cognitive abilities. However, cognitive challenges during development may result in life-history trade-offs, because complex neural reorganization required for coping with these challenges entails stress and energy costs. In this study, we experimentally tested whether early exposure to different cognitive challenges influences cognitive ability, brain size and life-history traits in the three-spined stickleback (Gasterosteus aculeatus). For this, juvenile sticklebacks were challenged over a 2-month period to resolve four different learning tasks. Contrary to our predictions, individuals trained in learning tasks later showed a reduced ability to solve a new maze task, suggesting that these learning tasks during early life may impair the ability to cope with new cognitive challenges. Females from the learning task group showed smaller optic tectum volume than the control females. Fish from the learning task treatment reduced their growth during the experiment, then accelerated growth to catch up until the breeding season. Sticklebacks reared in an environment with learning tasks also suffered a greater mortality than the controls both during and after the experiment. For the first time to our knowledge, we demonstrated direct costs of living in environments with cognitive challenges by tracking long-term performances of sticklebacks exposed to dynamic challenges during early life. Our results show that environmental challenges during ontogeny trigger plastic responses, but contrary to the common belief, do not improve the ability to cope with new cognitive challenges.
Introduction
Environmental conditions during early development play a crucial role in shaping an organism’s phenotype with substantial and long-lasting consequences (West-Eberhard, 2003). Living in complex environments in which individuals repeatedly encounter cognitive challenges to gain resources during their early life may favor increased cognitive abilities (e.g., Mery and Kawecki, 2002; Fawcett et al., 2014; Dridi and Lehmann, 2015). Indeed, several studies have shown that complex environments produce plastic responses in brain functions (reviewed in Ebbesson and Braithwaite, 2012). Behavioral adjustments through learning should allow individuals to cope with environmental challenges, such as avoiding predators and finding food (Dukas, 2004; Mery, 2013). These challenges during ontogeny may trigger developmental pathways in cognitive functions for remembering, problem solving and decision-making (Kotrschal and Taborsky, 2010; Sauce et al., 2018), which can be costly processes (Niven and Laughlin, 2008; Chittka et al., 2009; Burns et al., 2010). Thus, the energetic costs of collecting, processing and storing information may mediate trade-offs between cognitive abilities and life-history traits (Burger et al., 2008). However, the effects of environment-driven cognitive challenges during early development on cognitive abilities and life-history traits have not hitherto been investigated.
Individuals should frequently make behavioral decisions to solve problems related to foraging and habitat selection. An individual’s behavior patterns expressed in specific conditions are the product of learning mechanisms, such as how it perceives its environments, values resources and remembers its past experiences (Fawcett et al., 2012). Environmental conditions and experiences, especially during early life, can modulate cognitive traits by changing neural development, structure and function (Fox et al., 2010; Buchanan et al., 2013). Cognitive challenges may promote neural plasticity and synaptic reorganization (e.g., Nilsson et al., 1999; Xu et al., 2009; Matzel and Kolata, 2010; Salvanes et al., 2013) or induce morphological changes in different brain regions (e.g., Lema et al., 2005; Scholz et al., 2015). However, the development and maintenance of neural structures in the brain, an organ with high energy demands, may trade off against other costly organs and biological functions. For instance, previous experimental studies showed that animals selected for learning or large brain size had a shorter lifespan despite the adaptive advantages of learning (e.g., Burger et al., 2008; Kotrschal et al., 2019) and this genetic trade-off is also expected at the individual level. Thus, the evolution of learning ability and brain functions may be constrained by longevity, possibly due to the cost of cognitive functions. However, it is unclear whether cognitive challenges during development later increases overall cognitive functions (Light et al., 2010) or, on the contrary, causes an overall cognitive impairment due to the costs associated with an investment in learning (e.g., Jaumann et al., 2013).
The cost of living in environments with cognitive challenges may arise from the energy requirement for neural reorganization occurring during the learning process (Dunlap and Stephens, 2016). Thus, learning is likely to divert energy away from other energetically costly functions, such as somatic growth (Fisher et al., 2006), immune system (Anton et al., 2007), and reproduction (Mery and Kawecki, 2003). In addition, cognitive challenges may have negative impact on life-history traits such as growth and reproduction through stress (Romero, 2004). Environmental challenges may elevate the production of stress hormones, with contrasting effects on learning depending on the stress level. A mild elevation of stress hormones is required for memory acquisition, but high levels of stress impair memory function (Sandi, 2013; Schwabe and Wolf, 2013). Prolonged secretion of glucocorticoids may also result in elevated oxidative stress, with negative consequences for fitness (Costantini, 2008; Haussmann and Marchetto, 2010), including cognitive deficits (Sorce and Krause, 2009). To explicitly test short- and long-term costs of growing in an environment with cognitive challenges, it is necessary to follow individual performances during the lifetime in animals that adjust their behavior (Fawcett et al., 2014) and modulate stress responses (Buchanan et al., 2013) during early development.
In this study, we experimentally tested whether early exposure to learning tasks influences cognitive ability and life-history traits, especially growth, maturation, and survival in the three-spined stickleback (Gasterosteus aculeatus). In natural populations, sticklebacks experience seasonal changes in foraging conditions and are able to learn new foraging tasks (Girvan and Braithwaite, 1998; Brydges et al., 2008; Feng et al., 2015). Compared to other vertebrates, neurogenesis persists longer and brain continuously grows during the lifetime in fishes (Zupanc and Horschke, 1995; Zupanc, 2001). Thus, changes in the early environment may influence brain development and cognitive abilities in juvenile sticklebacks (Kotrschal and Taborsky, 2010; Salvanes et al., 2013), with possibly long-lasting consequences. In this experiment, we repeatedly exposed F1 juvenile sticklebacks, originated from a wild population, to different learning tasks over a 2-month period.
We studied whether the exposure to different learning tasks influenced brain size, cognitive ability, growth in body mass and size, reproductive performances and survival of the fish. We predicted that the exposure to learning tasks during early development would increase the ability to solve new environmental challenges. We expected that sticklebacks would develop larger brains or brain regions when exposed to the learning tasks to meet increased demands for adjusting their behavioral phenotype. Alternatively, problem-solving ability and brain size would be reduced if learning tasks during development impose costs in neural or cognitive functions. Furthermore, we explored sex differences in changes of brain size and cognitive performance because brain size differs between the two sexes in stickleback species (Kotrschal et al., 2012; Herczeg et al., 2015; Toli et al., 2017) probably due to sex-specific reproductive roles (Bell and Foster, 1994; Herczeg et al., 2014). If repeated learning of different tasks entails costs, we expect its negative effects on life-history traits, such as growth and survival.
Materials and Methods
Experimental Fish
For this study, we used F1 individuals obtained from three-spined sticklebacks captured in Rio Ulla (Spain). In this population, sticklebacks reproduce by spawning repeatedly throughout a single relatively long breeding season, after which they die (Kim and Velando, 2016). Adult wild fish captured in February 2017 were individually housed in 8-L tanks. A total of 30 full-sibling families were produced between April and June 2017 by crossing 21 sires and 20 dams. Each adult bred up to twice with different mates by pairing with one mate at a time. Each fertilized clutch was collected from the nest within 3 h and individually incubated until hatching. F1 fish were fed daily (twice up to age 2 months, then once a day) to satiation (i.e., until all fish stopped eating and were not interested in food) on a progressive diet of newly hatched Artemia and a commercial pelleted diet (~0.1–0.3 g wet mass per tank; Gemma Micro, Skretting, Norway). Pellet food contained 59% protein, 14% fat, 14% ash, and 2% others compounds. Fish were fed (1) solely with Artemia from hatching to age 15 days, (2) with Artemia and food pellets from age of 15 days to 40 days and (3) solely with food pellets from age 40 days onward. Food pellets were provided >5 h before the administration of Artemia during the mixed diet regime. At age 40 days, a sample of 10 fish from each full-sib family was randomly selected and allocated into two 8-L “growth tanks,” each housing 5 individuals (n = 60 tanks and 300 individuals). The growth tanks were connected to closed water systems, in which water was continuously filtered for nitrification, aerated and temperature-controlled by the combined flow-through function. The programmed photoperiod and temperature in the tanks reflected the natural seasonal pattern in the region. Before the onset of experimental treatment, all fish were habituated to feed on moistened food pellets, which immediately sink on the bottom of the tank. In early September (at age 103.8 ± 11.5 days), all individuals were permanently marked with color elastomer tags (Northwest Marine Technologies, Shaw Island, WA, United States) under a low dose of benzocaine anesthetic to allow identification.
Learning Tasks
In October (at age 134.3 ± 11.7 days), the two tanks of each family were randomly assigned to either the “learning task” or the “control” group (n = 130 and 134 individuals, respectively, see Supplementary Figure 1). Due to relatively high natural mortality during early life stages of fish, the number of fish in the growth tanks ranged between 3 and 5 fish (mean ± S.E. number of fish: learning task group: 4.52 ± 0.728; control group: 4.63 ± 0.679) but did not differ between the experimental treatments (GLMM: LRT, = 0.155, p = 0.693).
During the experimental treatment, juveniles from the learning task and control groups were similarly exposed to four different apparatuses during 68 days. In the learning task group, the apparatuses were introduced into the growth tanks and fish had to adjust their behavior to learn how to access food rewards by discriminating between false and true entrances. The entrances were associated with two different colors; one indicates the entrance to reach the food and the other does not (see details in Supplementary Material, Apparatuses and Supplementary Figure 2). Sticklebacks have good color vision and rely heavily on this sense to recognize and locate prey (Rick et al., 2012; Wootton, 2012; Flamarique et al., 2013; Rennison et al., 2016). The control fish were exposed to similar apparatuses, but all entrances of different colors were open and food was provided inside and/or outside the apparatuses (see Supplementary Material for details of different control apparatuses). In order to reduce neophobic behaviors, fish in both groups were previously exposed to each apparatus for 24 h before the first trial, but without food rewards. The next day, the fish did not show neophobic behaviors and tried to access the apparatus immediately after the food was provided. During the treatment with each task, which lasted for 17 days, the fish were exposed to the apparatus every two days (n = 9 trials). The apparatuses were presented in the same order for all of the tanks and groups (learning task and control; see Supplementary Figure 3). The apparatus was introduced at a random position and orientation inside the tank and left for 20 min in each trial. The fish in the learning task group had to pass a correct entrance within 20 min to access the food (moistened pellets) inside the apparatus, while the control fish had easy access to the food during the same 20 min trial. In order to prevent differences in food acquisition in the experimental groups, we provided the similar amount of food for the control and learning tank groups during the trial days (0.11–0.18 g per tank, see Supplementary Material for details), well above (4–6×) the food needed to satiate all the fish in the tank (see above). Importantly, after each 20-min trial, the apparatus was removed from the tank and all the fish in the tank could access the remaining food and eat until satiation for many hours (>10 h). Thus, in both treatments, a large amount of food was easily accessible to individual fish, and access to food was constrained only during the 20 min trial in the learning task group. Fish were not fed between trials.
The control fish usually accessed the food immediately after the onset of each trial. In contrast, fish in the learning task group needed more time, but the time taken to access the food decreased over the course of the treatment (Supplementary Figure 4), indicating that fish successfully adjusted their behavior through experience. During the treatment with the apparatus 1, we recorded in the learning task group the maximum number of fish inside the food compartment at any given time during each trial. Indeed, this number significantly increased during the course of the treatment (GLMM: estimate = 0.047 ± 0.02, LRT, = 4.93, p = 0.026). Fish performances in the learning task group were video-recorded in the initial and final trials of each apparatus (n = 8 videos). We analyzed the videos to determine the number of attempts per individual to enter into the food compartment during the first 1 min. The number of attempts did not differ between the initial and final trials [F(1, 8) = 1.86, p = 0.22], suggesting that enhanced exploratory behavior was probably not responsible for the improvement of access to the food throughout the repeated trials. In these videos, the level of aggression during the first 10-min, measured as the number of attacks per individual, did not differ between the initial and final trials [F(1, 8) = 0.36, p = 0.57]. There was no difference in swimming activity of fish between the learning task and control trials (see Supplementary Material, Pilot study of physical activity).
Maze Test
We evaluated the cognition-related ability of each fish in a maze test twice, 1 week before (late September; when fish were on average 125.7 ± 11.9 days old) and 3 weeks after the experimental manipulation (early January; when fish were on average 233.3 ± 12.1 days old) to study individual changes in the ability to solve the task after the treatment. The maze consisted of a long glass tank divided into a home compartment and three additional consecutive compartments by three transparent partitions, each including a true entrance outlined in green color and a closed false entrance outlined in blue color (Supplementary Figure 5). In the last compartment of the maze, moistened food was provided. The food was visible from the home compartment. Before the test, fish were starved for 24 h. The focal fish was isolated by a transparent partition and acclimated for 30 s in the home compartment. Then, the partition was removed and we recorded fish performances for 300 s using a tripod-mounted digital video camera (Sony; Handycam DCR-SX44). To stablish the duration of the maze test, we conducted a pilot study using a sample of 20 non-experimental fish. Following the same protocol as explained above, we assessed the cognition-related ability of each fish in the maze test. In 18 out of 20, the fish completed the maze test within 300 s. Thus, we measured the time taken for each fish to reach the last compartment up to 300 s. In addition, we recorded in each test whether the fish made erroneous attempts to pass the partitions by touching the false entrances. The individuals that completed the maze test without any error were faster in reaching to the last compartment than those with errors [LMM; F(1, 294) = 7,011, p < 0.01], suggesting that the time taken to reach the last compartment indeed represent some cognitive abilities, such as the cognitive skills to find the true entrance.
If the fish did not reach the last compartment within 300 s, we repeated the test after 5 min of rest up to three times. In our pilot study, we observed that some fish remained in the home compartment for a longer period than 300 s, but responded to the test when they were retested. We assigned the maximum time (300 s) when the fish did not reach the last compartment in three consecutive maze tests (before the experimental treatment: in 37 out of 134 control and 49 out of 130 learning task fish; after the treatment: 31 out of 112 control and 30 out of 64 learning task fish). Prior to statistical analyses, the maze score (i.e., time) was transformed using GuanRank, a non-parametric ranking-based technique that converts right-censored data into a linear space of hazard ranks (Huang et al., 2017). A higher GuanRank-transformed value indicates a shorter time to reach the food rewards and thus a stronger ability to solve the maze test.
Sampling and Brain Measurements
In January, after the experimental manipulation and maze test (when the fish were on average 251.4 ± 11.3 days old), one fish from each growth tank (learning task group: n = 24; control group: n = 30) was euthanized with an overdose of benzocaine anesthetic. The whole brain of each individual was separated from the cranium under a stereomicroscope (Nikon SMZ1500). To prevent the brain from drying under the stereomicroscope light, we added a drop of phosphate-buffered saline solution to the brain, and it’s dorsal, ventral, and both lateral sides were photographed. All brain photographs were taken from a standard distance and angle. Width (W), height (H), and length (L) of four different brain regions (i.e., telencephalon, optic tectum, cerebellum, and hypothalamus) were determined from the digital photographs by using IMAGEJ software (see., Pollen et al., 2007; Gonda et al., 2011; Herczeg et al., 2015; Toli et al., 2017), and the volume of each region was estimated by using the ellipsoid model [V = (L × W × H)π/6]. Some brain regions, which were damaged during dissection, could not be measured. Telencephalon was determined in 15 fish from the learning task and 20 fish from the control group, optical tectum in 18 and 23, cerebellum in 19 and 25, and hypothalamus in 18 and 23. The whole brain volume was estimated as the sum of all different brain region volumes in the samples with all measurements (learning task group, n = 9; control group, n = 17). In 48 randomly selected brains, three independent measurements of volume were highly repeatable (R > 0.97, p < 0.001 in the four brain regions).
Growth, Reproduction, and Survival
All fish were weighed with a digital balance (to the nearest 0.001 g) and their standard length measured (to the nearest 1 mm) in three occasions; before and after the experimental manipulation (immediately after the maze tests; see above) and at the beginning of the breeding season (when fish were on average 281.6 ± 11.1 days old).
Individuals that were not sacrificed for brain measurements and survived until the beginning of the breeding season were reallocated into individual tanks. Male sticklebacks express red nuptial coloration on their cheeks and throat during the breeding season, and females preferentially mate with redder males (Ostlund-Nilsson et al., 2006). Males that had started to express red coloration were provided with sand and polyester threads as nesting materials (n = 38). During the reproductive season (from April to August) each male was shown a gravid female from a stock (not used in this experiment) enclosed in a transparent glass for 5 min twice a week to prompt the expression of nuptial color and nest construction. Each male was photographed under standardized conditions in the middle of the breeding season (June) and the size and intensity of the red area measured using the digital image. The relative size of the red area was calculated as a percentage of the total lateral body area for statistical analyses. Females kept in their individual tanks (n = 63) were monitored daily to record maturation and the number of spawning events (evident from changes in abdomen size). The females started to spawn in March and stopped egg production by early August.
The survival of fish was monitored daily until the end of the breeding season (August 2018; when fish were on average 432.7 ± 11.1 days old). Dead fish were stored at −80°C for sex determination.
Sex Determination
We sexed fish by external morphology in the individuals survived until sexual maturation (n = 101) or by internal morphology (n = 60) or a molecular method (n = 98) in those dead or sampled before maturation. We used a part of the 3′UTR of the NADP-dependent isocitrate dehydrogenase locus for molecular sexing (Velando et al., 2017). In 5 fish, we failed to amplify the gene.
Statistical Analyses
We analyzed the effects of the experimental treatment in linear mixed-effect models (LMMs) and generalized linear mixed models (GLMMs) by using the lmer and glmer functions of the lme4 package in R v.3.5.1. In LMMs, all data conform normality (Shapiro–Wilk test) and equal variance between treatments (Levene’s Test) assumptions. In GLMMs, the significance of term was determined by Likelihood Ratio Test (LRT). In all models detailed below, we also explored two-way interactions between treatment and all other fixed effects, but the interactions were dropped in the final models when not statistically significant. Post-hoc comparisons were performed using Tukey’s post-hoc test.
We first tested whether there was any sampling bias in body size, body mass, and initial maze score between the learning task and control groups by using LMMs including treatment as a fixed effect and growth tank identity and family identity as nested random effects. The final maze score (GuanRank transformed) was analyzed in a LMM, including treatment, sex, and initial maze score (to account for any potential effect of prior cognitive ability on maze performance) as fixed terms and tank identity nested within family identity as random effects. We also tested if intra-tank coefficient of variation in maze score differed between the two treatments in a LMM including family identity as a random term. Sizes of the whole brain and brain regions were analyzed in LMMs, including treatment, sex and initial maze score as fixed effects and family identity as a random effect. Tank identity was not included as a random effect because only a fish per tank was used for brain measurements. Body size was included as an additional fixed effect in the analysis of the whole brain, and brain mass in the analyses of brain regions. Since some brain regions were damaged during the dissection, brain size cannot be estimated for all brains, but brain size and mass were highly correlated (r = 0.623, p < 0.001).
Fish growth was analyzed using repeated measures of body size and mass in LMMs with random slope and intercept accounting for any individual differences in growth rates between time points. In these analyses, treatment, time point of measurement (three-level factor), sex and initial maze score were included as fixed effects, and tank identity nested within family identity and fish identity as random effects. In these models, statistically significant treatment × time interaction indicates differential growth trajectories between the two treatment groups (Nicieza and Alvarez, 2009; Hector and Nakagawa, 2012). Additionally, we also analyzed the intra-tank coefficient of variation in body mass and size after the treatment in LMMs including the time of measurement and treatment as fixed effects and family identity as random effect.
Age at the expression of nuptial coloration, relative size of red area, intensity of the red nuptial coloration and date of nest construction of males and age at first spawning event of females were analyzed in LMMs including treatment and initial maze score as fixed effects and tank identity nested within family identity as random effects. The total number of spawning events of females was analyzed in a GLMM with a Poisson error structure and log function. Survival since the beginning of the experimental manipulation to the end of the breeding season was analyzed in a Cox proportional hazard model by using the “Coxme” package in R v.3.5.1 (Therneau and Therneau, 2015). We included treatment, sex and initial maze score as fixed effects and growth tank identity nested within family identity as random effects. Although initial number of fish per tank was similar between the two treatments (see above), it changed during the course of the experiment (Supplementary Figure 6) due to natural mortality, especially in the learning task group (see Results). In order to test if the observed effects are attributed to changes in the group size, we performed additional analyses by including the number of remaining fish in the growth tank at the onset of training with each apparatus (days 1, 17, 34, 51) in separate models. Except when indicated, similar results were achieved in these additional analyses.
Results
Initial Differences
There was no difference in initial body size [F(1, 235.4) = 1.40, p = 0.238], body mass [F(1, 235.1) = 0.12, p = 0.731] and maze score [F(1, 58.4) = 2.05, p = 0.157], which were measured prior to the experimental treatment, between the two experimental groups.
Effect of Treatment on Maze Test
The treatment had a significant effect on the maze score, which was determined 2 weeks after the experimental manipulation [F(1, 25.5) = 5.75, p = 0.024]. The experimental fish from the learning task group took 24% more time to reach the food rewards than the control fish (Figure 1). Sex and initial maze score and their interactions with treatment were not significant (all p > 0.089). Similar results were achieved when those fish that remained in the home compartment were removed from the analysis [treatment: F(1, 24.6) = 6.6, p = 0.017]. The intra-tank variation in maze score after the experimental manipulation was similar between the two experimental groups [F(1, 42) = 2.59, p = 0.115].
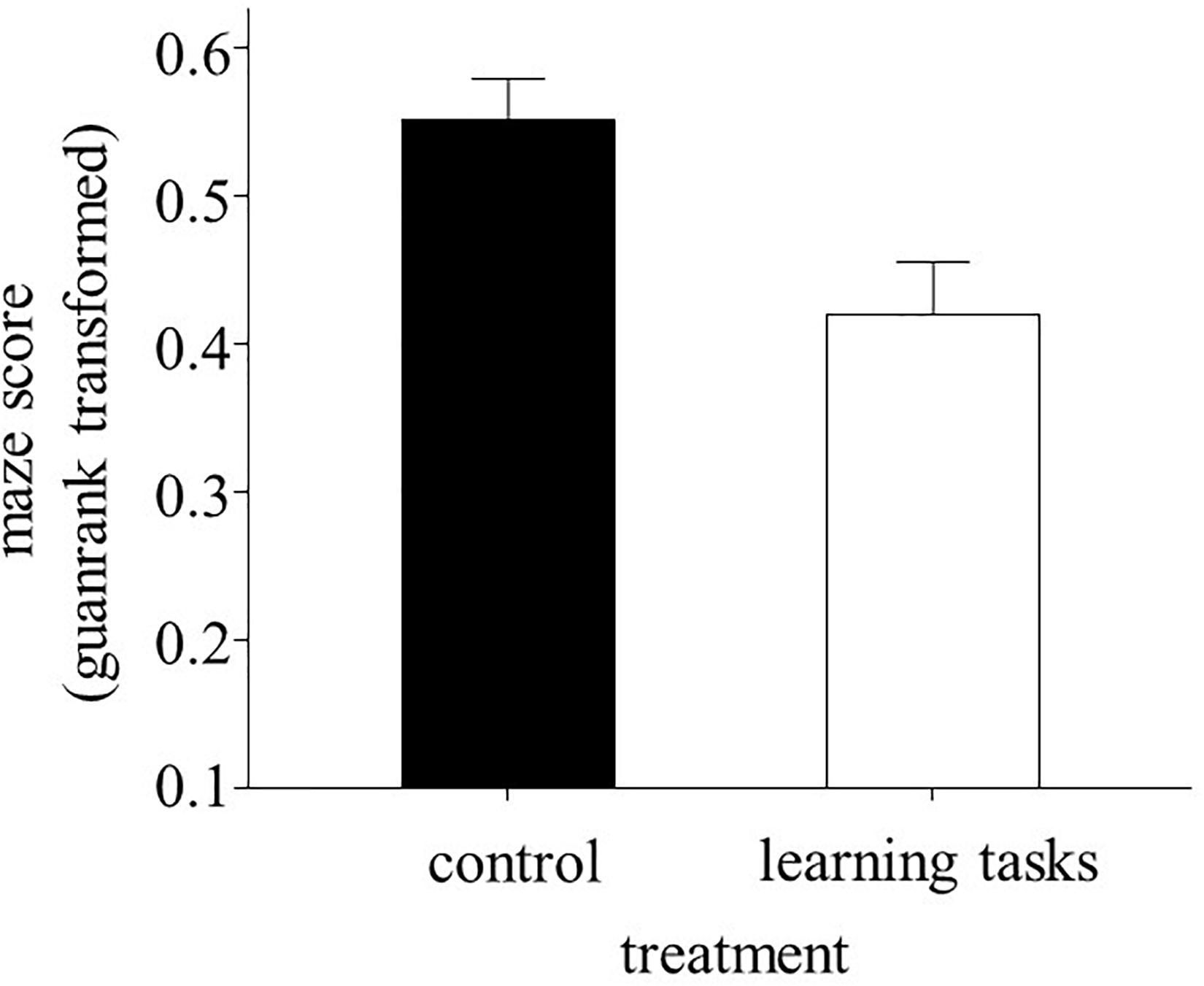
Figure 1. Mean ± S.E. maze scores (GuanRank-transformed time taken to cross the maze test) performed by juvenile sticklebacks after the 2-month experimental treatment (learning task group, n = 64; control group, n = 112). A higher maze score indicates a shorter time to reach the food rewards.
Effect of Treatment on Brain Size
The treatment had sex-specific effect on the volume of optic tectum [treatment × sex: F(1, 23.4) = 8.02, p = 0.009; Supplementary Table 1]. Females from the learning task group had smaller optic tectum than the control females (Tukey post-hoc test; p = 0.014), whereas males did not show this difference (Tukey post-hoc test; p = 0.765; Supplementary Figure 7). When including the number of fish per tank at the onset of training with the last apparatus (day 51) as a covariate in the model of the volume of optic tectum, the main effect of the treatment was significant [F(1, 16.5) = 5.79, p = 0.028] but not the treatment × sex interaction [F(1, 28.7) = 2.74, p = 0.11]. In this additional analysis, the fish trained with the learning tasks showed a smaller optic tectum volume than the control fish (estimate: -0.86 ± 0.36). The volumes of the hypothalamus, telencephalon, cerebellum and the whole brain were not affected by the experimental treatment and its interaction with sex or initial maze score (p > 0.131 in all cases; Supplementary Table 1).
Effects of Treatment on Growth, Reproduction, and Survival
Growth trajectories differed between the learning task and control fish, both in body size [time × treatment: F(2, 169.2) = 24.75, p < 0.001] and mass [time × treatment: F(2, 243.2) = 11.42, p < 0.001]. Fish from the learning task group grew less than the control fish during the course of the treatment (Figure 2), showing reduced body size [F(1, 172.2) = 8.92, p = 0.003] and mass [F(1, 172.4) = 7.35, p = 0.007] after the experimental manipulation; then accelerated growth and attained similar body size [F(1, 112 = 0.22, p = 0.635] and mass [F(1, 112) = 0.33, p = 0.566] to the control fish at the breeding season. Similar results were obtained when daily growth rates were analyzed (see Supplementary Figure 8). This clearly indicates that catch-up growth occurred after the experimental manipulation in the learning task fish. Initial maze score, sex and their interactions with treatment did not affect growth trajectories in body mass and size (p > 0.14 in all cases). The intra-tank variations in body mass and size after the experimental manipulation were similar between the two experimental groups (p > 0.60 in both cases).
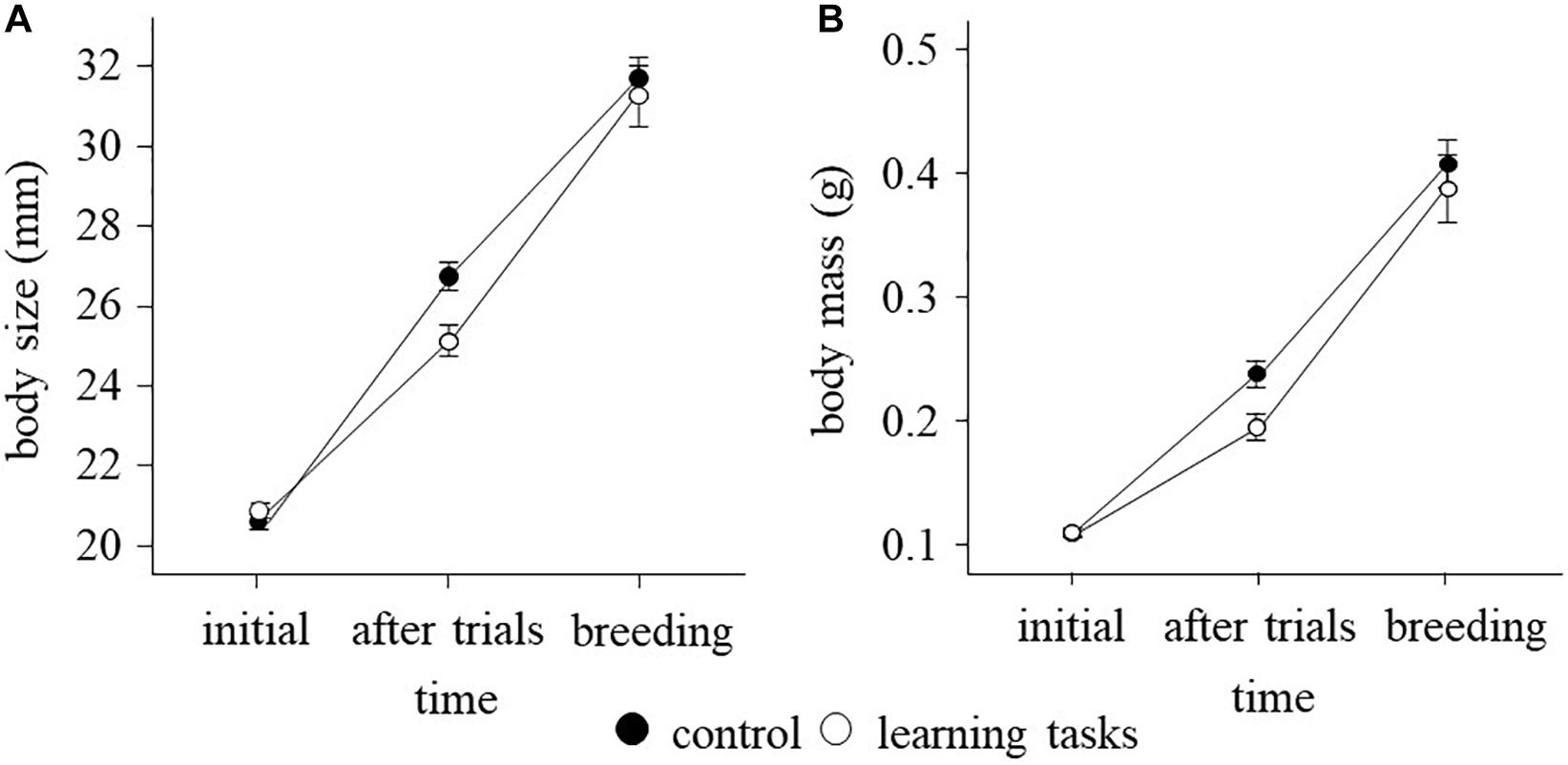
Figure 2. Mean ± S.E. (A) body size and (B) body mass of sticklebacks measured in three time points, before the experiment (September, n = 264), after the learning experiment (January, n = 176) and at the beginning of the breeding season (March, n = 114) in the learning task (white dots) and control (black dots) treatments.
Reproductive traits of males (age at the expression of nuptial coloration, relative size of red area, intensity of the red nuptial coloration and nest building date) and females (age at first spawning and number of spawning events) were not affected by the experimental treatment (Supplementary Table 2). Analysis of survival revealed that fish from the learning task group had a significantly shorter lifespan than the control fish (Figure 3; Cox proportional hazard model, = 50.35, p < 0.001). The estimated life expectancy of fish was 387.9 ± 9.8 days in the control group and 298.5 ± 5.9 days in the learning task group. The treatment effect on survival was not influenced by sex and initial maze score and their interactions with treatment (p > 0.59 in all cases). The difference in survival between the two treatment groups was evident both during ( = 23.67, p < 0.001) and after ( = 43.51, p < 0.001) the period of experimental treatment (Figure 3).
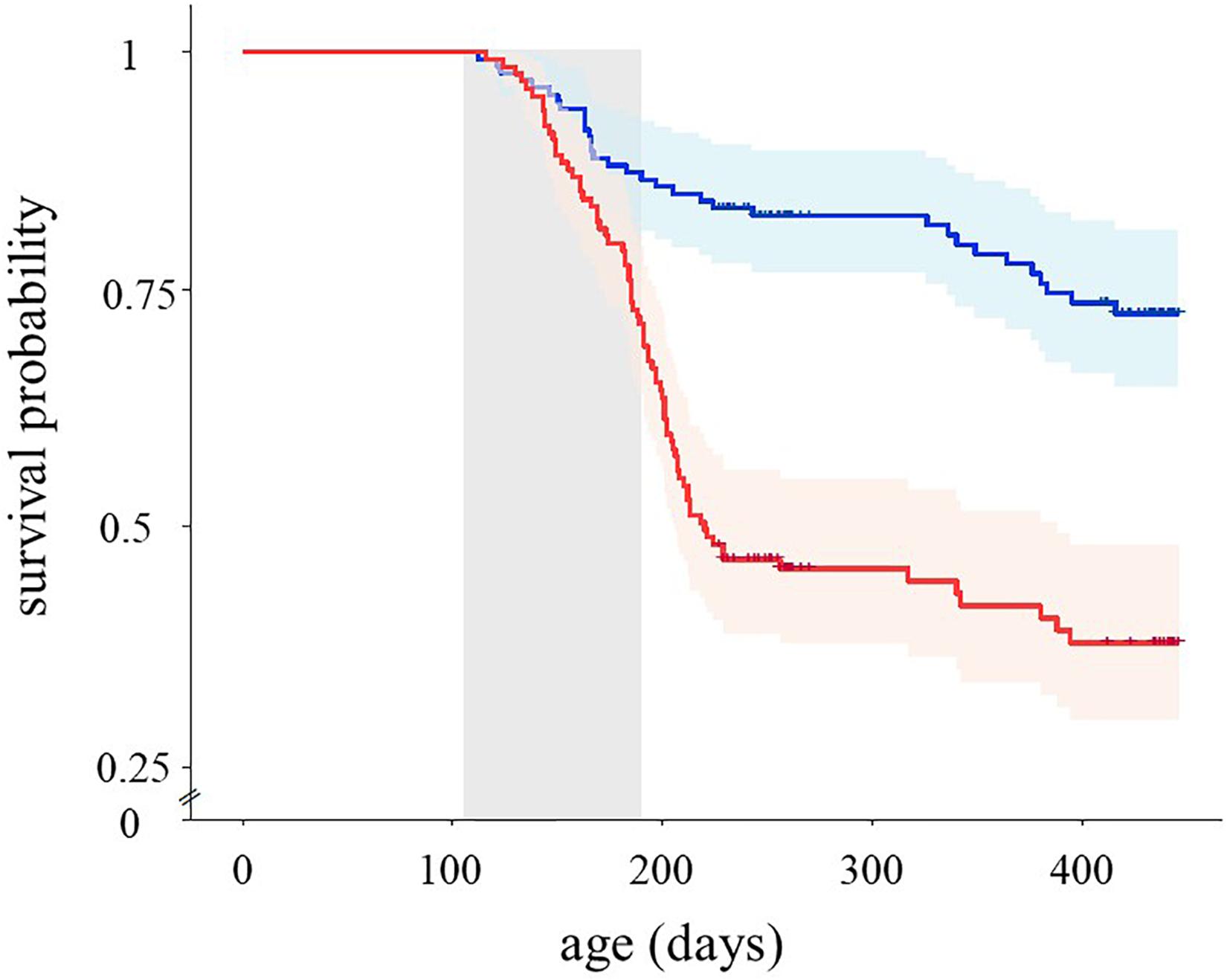
Figure 3. Estimated survival functions of sticklebacks reared in the learning task (red line, n = 130) and control treatments (blue line, n = 134) during the study. Gray shadow indicates the period of the experimental treatment. Crosses mark censorship events, i.e., individuals sampled for the brain analyses and those still alive at the end of the monitoring period.
Discussion
During the 2-month period of experimental treatment, juvenile fish challenged with different learning tasks successfully adjusted their behavior to the environment by shortening the time taken to access to food in the apparatus. Contrary to our predictions, however, fish exposed to learning tasks in their environments during the early life later showed a reduced ability to solve a maze test, suggesting that coping with the learning challenges may trade off against cognitive ability. Brain size did not increase in the learning task group. Females from the learning task group (but not males) had rather smaller optic tectum than the control females, suggesting a sex-specific negative effect of learning tasks on brain development. The treatment with the learning apparatuses also had negative effects on growth and survival. Juvenile fish exposed to learning tasks reduced their growth during the experimental period, then experienced a rapid catch-up growth until the breeding season. Moreover, sticklebacks reared under the learning task regime suffered greater mortality than the controls, both during and after the experimental period. Overall, our results reveal long-term costs of living in environments with cognitive challenges in terms of growth and problem-solving deficits in later life and their negative consequence in lifespan.
Sticklebacks from the learning task group showed a poorer maze score later in life, which reflects a weaker ability to obtain food in a new, complex environment. This effect was not due to differential mortality of individuals with different cognitive abilities because juvenile survival was not related to their initial maze score or its interaction with the treatment. Thus, our results suggest that repeated exposure to different learning challenges may produce an impairment of abilities, probably including cognitive abilities to solve a new environmental challenge. The exposure to different tasks probably stimulated several processes related to learning, remembering and decision-making (Kolata et al., 2007; Matzel and Kolata, 2010). In teleost fishes, complex associational neural mechanisms are acquired during associative training (Salas et al., 2006; Messias et al., 2016; Harmon et al., 2017). Thus, it is possible that these learning-induced neural changes in juvenile sticklebacks traded off against other cognitive functions during the development (Schwabe and Wolf, 2010). It is also possible that the contrasting information between the cues used in different trials and the final maze test might cause confusion for the individuals that were subjected to different challenges. Further studies using different cognitive assays are required to assess whether these negative effects of learning tasks on the ability to solve a maze test may be extended to general cognitive abilities (Rowe and Healy, 2014).
In our experiment, juvenile sticklebacks were exposed to four associative tasks in which they needed to find the entrance to food rewards. Since the control fish were exposed to similar apparatuses, the effects reported here most likely are due to a learning process in which fish had to discriminate between false and true entrances. The repeated stimulation through rewards, probably increased and reinforced the motivation to pay attention and learn (Warburton, 2003). It is possible that visual stimulation during the learning tasks lead to neural reorganization, which is a high energy demanding process (Niven and Laughlin, 2008). Another possible explanation for the negative effects on problem-solving ability may be attributed to differential physical activities or behaviors between the treatment groups. In our pilot study of physical activity, we did not find any effect of the experimental apparatuses on swimming activity, but we cannot discard the possibility that changes in activity or behavior during the treatment might produce some energetic costs.
In our experiment, juvenile sticklebacks were exposed to learning tasks in groups of several individuals, resembling the natural social environment of this species (Frommen et al., 2007). We did not detect any difference in intra-tank variations in maze scores and body size between the learning task and control groups, suggesting that the treatment did not induce within-group individual differences. During the training period, most experimental fish in each group reached the food reward, indicating that probably they socially learned how to access the food in the apparatus. Nevertheless, it is possible that the treatment with the apparatuses increased competition for food, and hence social stress (Creel et al., 2013). We did not measure cortisol levels in the experimental fish, but it is possible that these challenging environments elevated the glucocorticoid stress hormone, which facilitates memory acquisition and learning at low or mild levels of stress (Sandi, 2013; Schwabe and Wolf, 2013). However, higher levels of glucocorticoid hormones may have a negative impact on neurogenesis and neuron maintenance (Buchanan et al., 2013). Thus, in addition to energetic costs, the stress induced by different tasks may also be responsible for the negative effects of the learning task treatment on maze performance. Irrespective of the mechanism, our study suggests that the exposure to repeated learning tasks during early life that requires problem-solving may impair some cognitive abilities later in the life.
In sticklebacks, some studies found that environmental complexity increases brain size (e.g., Herczeg et al., 2015) but others did not (e.g., Toli et al., 2017). In this study, brain size did not differ between the two treatments, but there was a sex-specific treatment effect on the relative size of one brain region. Females from the learning task group had smaller relative optic tectum volume than the control females, but this effect was not found in males. Cognitive processes may produce synaptic and neural reorganization within the optic tectum (Kinoshita et al., 2004). The neuromodulatory effects of female hormones or sex-specific energetic trade-off between brain function and gonadal development might underlie these sex-specific effects (Makantasi and Dermon, 2014; Pellegrini et al., 2016; Rystrom et al., 2019). A previous study on three-spined sticklebacks have shown that males have larger brains than females (Kotrschal et al., 2012). The treatment had similar effect on optic tectum volume in the two sexes when controlling for the changing group size effect, suggesting that the sex-specific effects might be due to conspecific interactions during the development. We did not find differences in the whole brain, but our results suggest that neuro-anatomical sexual dimorphism of certain brain region may be induced by early environmental conditions. However, these results should be taken with caution because, given the low sample size, our data have limited power to detect the subtle effects.
During the experiment, juveniles from the learning task group suffered reduced growth rates and increased mortality, suggesting that cognitive challenges have important fitness costs (Mery and Kawecki, 2003). In our experiment, the control fish grew and gained weight fast during the juvenile period, then slowed down somatic growth during the breeding season, as typically occur in this species (Kim et al., 2019). The learning task treatment changed the growth trajectory of the experimental fish that grew at a slower rate than the controls during the treatment, then accelerated growth to catch up and attained a similar body size and mass to the controls during the breeding season (Metcalfe and Monaghan, 2001). It is well-known that such catch-up growth has delayed deleterious effects, increasing oxidative damage in somatic and germline tissues in adults (Kim et al., 2019) and reducing reproductive rate and lifespan (Inness and Metcalfe, 2008; Lee et al., 2012; Ab Ghani and Merilä, 2014). The negative effect of the treatment on survival beyond the period of the experiment may be due to fast compensatory growth (Kim et al., 2019). It is also possible that the physiological costs (e.g., oxidative stress) of maintaining high levels of glucocorticoids negatively influence lifespan (Blas et al., 2007; Dowling and Simmons, 2009; Crespi et al., 2013). Although access to food was more difficult in the learning task group during trials, it is unlikely that the effect on survival was due to differences in the amount of food ingested or food monopolization since all the fish in the tanks could access to a large amount of food after the trials.
Conclusion
In conclusion, our study demonstrates that environmental challenges and learning during early life incur important costs that reduce cognitive ability to solve problems and generate sexual size dimorphism in a specific brain region, induce compensatory growth and finally shorten lifespan. Despite the common belief that learning during early life improves general cognitive function in animals including humans (Klingberg, 2010; Morrison and Chein, 2011), our results suggest that cognitive training can induce the opposite effect. Our results suggest that negative effects of early life stress on development and costly compensatory growth may mediate the trade-off between learning and lifespan (Burger et al., 2008).
Data Availability Statement
The datasets generated for this study can be found in the online repositories. The names of the repository/repositories and accession number(s) can be found below: Figshare digital repository (10.6084/m9.figshare.9989480).
Ethics Statement
The animal study was reviewed and approved by the Ethics Committee of the Universidade de Vigo and the Xunta de Galicia (ES360570181401/17/FUN01/BIOL AN.08/SYK01).
Author Contributions
NA-Q, AV, and S-YK planned the study. NA-Q performed the experiment and collected the data. NA-Q and AV developed the statistical analyses. All authors contributed to write the manuscript.
Funding
This work was supported by the Spanish Ministerio de Ciencia, Innovación y Universidades (CGL2015-69338-C2-1-P, PGC2018-095412-B-I00, and RYC-2015-18317) and the Consellería de Cultura, Educación e Ordenación Universitaria, Xunta de Galicia (ED431F 2017/07). NA-Q was supported by the FPI student grant from MICINN (BES-2016-078894).
Conflict of Interest
The authors declare that the research was conducted in the absence of any commercial or financial relationships that could be construed as a potential conflict of interest.
Acknowledgments
We would like to thank Jose C. Noguera, Belén Otero, Alberto da Silva, and Hugo Martínez for their invaluable help during the study.
Supplementary Material
The Supplementary Material for this article can be found online at: https://www.frontiersin.org/articles/10.3389/fevo.2020.562404/full#supplementary-material
References
Ab Ghani, N. I., and Merilä, J. (2014). Cross−generational costs of compensatory growth in nine−spined sticklebacks. Oikos 123, 1489–1498. doi: 10.1111/oik.01597
Anton, S., Dufour, M. C., and Gadenne, C. (2007). Plasticity of olfactory−guided behaviour and its neurobiological basis: lessons from moths and locusts. Entomol. Exp. Appl. 123, 1–11. doi: 10.1111/j.1570-7458.2007.00516.x
Bell, M. A., and Foster, S. A. (1994). The Evolutionary Biology of the Threespine Stickleback. Oxford: Oxford University Press.
Blas, J., Bortolotti, G. R., Tella, J. L., Baos, R., and Marchant, T. A. (2007). Stress response during development predicts fitness in a wild, long lived vertebrate. Proc. Natl. Acad. Sci. U.S.A. 104, 8880–8884. doi: 10.1073/pnas.0700232104
Brydges, N. M., Heathcote, R. J., and Braithwaite, V. A. (2008). Habitat stability and predation pressure influence learning and memory in populations of three-spined sticklebacks. Anim. Behav. 75, 935–942. doi: 10.1016/j.anbehav.2007.08.005
Buchanan, K. L., Grindstaff, J. L., and Pravosudov, V. V. (2013). Condition dependence, developmental plasticity, and cognition: implications for ecology and evolution. Trends Ecol. Evol. 28, 290–296. doi: 10.1016/j.tree.2013.02.004
Burger, J. M., Kolss, M., Pont, J., and Kawecki, T. J. (2008). Learning ability and longevity: a symmetrical evolutionary trade−off in Drosophila. Evol. Intern. J. Org. Evol. 62, 1294–1304. doi: 10.1111/j.1558-5646.2008.00376.x
Burns, J. G., Foucaud, J., and Mery, F. (2010). Costs of memory: lessons from ‘mini’brains. Proc. R. Soc. B Biol. Sci. 278, 923–929. doi: 10.1098/rspb.2010.2488
Chittka, L., Skorupski, P., and Raine, N. E. (2009). Speed–accuracy tradeoffs in animal decision making. Trends Ecol. Evol. 24, 400–407. doi: 10.1016/j.tree.2009.02.010
Costantini, D. (2008). Oxidative stress in ecology and evolution: lessons from avian studies. Ecol. Lett. 11, 1238–1251. doi: 10.1111/j.1461-0248.2008.01246.x
Creel, S., Dantzer, B., Goymann, W., and Rubenstein, D. R. (2013). The ecology of stress: effects of the social environment. Funct. Ecol. 27, 66–80. doi: 10.1111/j.1365-2435.2012.02029.x
Crespi, E. J., Williams, T. D., Jessop, T. S., and Delehanty, B. (2013). Life history and the ecology of stress: how do glucocorticoid hormones influence life−history variation in animals? Funct. Ecol. 27, 93–106. doi: 10.1111/1365-2435.12009
Dowling, D. K., and Simmons, L. W. (2009). Reactive oxygen species as universal constraints in life-history evolution. Proc. R. Soc. B Biol. Sci. 276, 1737–1745. doi: 10.1098/rspb.2008.1791
Dridi, S., and Lehmann, L. (2015). Environmental complexity favors the evolution of learning. Behav. Ecol. 27, 842–850. doi: 10.1093/beheco/arv184
Dukas, R. (2004). Evolutionary biology of animal cognition. Annu. Rev. Ecol. Evol. Syst. 35, 347–374.
Dunlap, A. S., and Stephens, D. W. (2016). Reliability, uncertainty, and costs in the evolution of animal learning. Curr. Opin. Behav. Sci. 12, 73–79. doi: 10.1016/j.cobeha.2016.09.010
Ebbesson, L., and Braithwaite, V. (2012). Environmental effects on fish neural plasticity and cognition. J. Fish. Biol. 81, 2151–2174. doi: 10.1111/j.1095-8649.2012.03486.x
Fawcett, T. W., Fallenstein, B., Higginson, A. D., Houston, A. I., Mallpress, D. E., Trimmer, P. C., et al. (2014). The evolution of decision rules in complex environments. Trends Cogn. Sci. 18, 153–161.
Fawcett, T. W., Hamblin, S., and Giraldeau, L.-A. (2012). Exposing the behavioral gambit: the evolution of learning and decision rules. Behav. Ecol. 24, 2–11. doi: 10.1093/beheco/ars085
Feng, S., McGhee, K. E., and Bell, A. M. (2015). Effect of maternal predator exposure on the ability of stickleback offspring to generalize a learned colour–reward association. Anim. Behav. 107, 61–69. doi: 10.1016/j.anbehav.2015.05.024
Fisher, M. O., Nager, R. G., and Monaghan, P. (2006). Compensatory growth impairs adult cognitive performance. PLoS Biol. 4:e251. doi: 10.1371/journal.pbio.0040251
Flamarique, I. N., Cheng, C. L., Bergstrom, C., and Reimchen, T. E. (2013). Pronounced heritable variation and limited phenotypic plasticity in visual pigments and opsin expression of threespine stickleback photoreceptors. J. Exp. Biol. 216, 656–667. doi: 10.1242/jeb.078840
Fox, S. E., Levitt, P., and Nelson, C. A. (2010). How the timing and quality of early experiences influence the development of brain architecture. Child Develop. 81, 28–40.
Frommen, J. G., Mehlis, M., Brendler, C., and Bakker, T. C. (2007). Shoaling decisions in three-spined sticklebacks (Gasterosteus aculeatus)—familiarity, kinship and inbreeding. Behav. Ecol. Sociobiol. 61, 533–539. doi: 10.1007/s00265-006-0281-2
Girvan, J. R., and Braithwaite, V. A. (1998). Population differences in spatial learning in three–spined sticklebacks. Proc. R. Soc. B Biol. Sci. 265, 913–918. doi: 10.1098/rspb.1998.0378
Gonda, A., Välimäki, K., Herczeg, G., and Merilä, J. (2011). Brain development and predation: plastic responses depend on evolutionary history. Biol. Lett. 8, 249–252. doi: 10.1098/rsbl.2011.0837
Harmon, T. C., Magaram, U., McLean, D. L., and Raman, I. M. (2017). Distinct responses of Purkinje neurons and roles of simple spikes during associative motor learning in larval zebrafish. eLife 6:e22537.
Haussmann, M. F., and Marchetto, N. M. (2010). Telomeres: linking stress and survival, ecology and evolution. Curr. Zool. 56, 714–727. doi: 10.1093/czoolo/56.6.714
Hector, K. L., and Nakagawa, S. (2012). Quantitative analysis of compensatory and catch−up growth in diverse taxa. J. Anim. Ecol. 81, 583–593. doi: 10.1111/j.1365-2656.2011.01942.x
Herczeg, G., Gonda, A., Balázs, G., Noreikiene, K., and Merilä, J. (2015). Experimental evidence for sex-specific plasticity in adult brain. Front. Zool. 12:38. doi: 10.1186/s12983-015-0130-0
Herczeg, G., Välimäki, K., Gonda, A., and Merilä, J. (2014). Evidence for sex−specific selection in brain: a case study of the nine−spined stickleback. J. Evol. Biol. 27, 1604–1612. doi: 10.1111/jeb.12409
Huang, Z., Zhang, H., Boss, J., Goutman, S. A., Mukherjee, B., Dinov, I. D., et al. (2017). Complete hazard ranking to analyze right-censored data: an ALS survival study. PLoS Comp. Biol. 13:e1005887. doi: 10.1371/journal.pcbi.1005887
Inness, C. L., and Metcalfe, N. B. (2008). The impact of dietary restriction, intermittent feeding and compensatory growth on reproductive investment and lifespan in a short-lived fish. Proc. R. Soc. B Biol. Sci. 275, 1703–1708. doi: 10.1098/rspb.2008.0357
Jaumann, S., Scudelari, R., and Naug, D. (2013). Energetic cost of learning and memory can cause cognitive impairment in honeybees. Biol. Lett. 9:20130149. doi: 10.1098/rsbl.2013.0149
Kim, S. Y., Noguera, J. C., and Velando, A. (2019). Carry−over effects of early thermal conditions on somatic and germline oxidative damages are mediated by compensatory growth in sticklebacks. J. Anim. Ecol. 88, 473–483.
Kim, S.-Y., and Velando, A. (2016). Genetic conflict between sexual signalling and juvenile survival in the three-spined stickleback. BMC Evol. Biol. 16:52. doi: 10.1186/s12862-016-0613-4
Kinoshita, M., Hosokawa, T., Urano, A., and Ito, E. (2004). Long-term potentiation in the optic tectum of rainbow trout. Neurosci. Lett. 370, 146–150. doi: 10.1016/j.neulet.2004.08.017
Klingberg, T. (2010). Training and plasticity of working memory. Trends Cogn. Sci. 14, 317–324. doi: 10.1016/j.tics.2010.05.002
Kolata, S., Light, K., Grossman, H. C., Hale, G., and Matzel, L. D. (2007). Selective attention is a primary determinant of the relationship between working memory and general learning ability in outbred mice. Learn. Mem. 14, 22–28. doi: 10.1101/lm.408507
Kotrschal, A., Corral-Lopez, A., and Kolm, N. (2019). Large brains, short life: selection on brain size impacts intrinsic lifespan. Biol. Lett. 15:20190137. doi: 10.1098/rsbl.2019.0137
Kotrschal, A., Räsänen, K., Kristjansson, B. K., Senn, M., and Kolm, N. (2012). Extreme sexual brain size dimorphism in sticklebacks: a consequence of the cognitive challenges of sex and parenting? PLoS One 7:e30055. doi: 10.1371/journal.pone.0030055
Kotrschal, A., and Taborsky, B. (2010). Environmental change enhances cognitive abilities in fish. PLoS Biol. 8:e1000351. doi: 10.1371/journal.pbio.1000351
Lee, W.-S., Monaghan, P., and Metcalfe, N. B. (2012). The pattern of early growth trajectories affects adult breeding performance. Ecology 93, 902–912. doi: 10.1890/11-0890.1
Lema, S. C., Hodges, M. J., Marchetti, M. P., and Nevitt, G. A. (2005). Proliferation zones in the salmon telencephalon and evidence for environmental influence on proliferation rate. Comp. Biochem. Physiol. A Mol. Integr. Physiol. 141, 327–335. doi: 10.1016/j.cbpb.2005.06.003
Light, K. R., Kolata, S., Wass, C., Denman-Brice, A., Zagalsky, R., and Matzel, L. D. (2010). Working memory training promotes general cognitive abilities in genetically heterogeneous mice. Curr. Biol. 20, 777–782. doi: 10.1016/j.cub.2010.02.034
Makantasi, P., and Dermon, C. R. (2014). Estradiol treatment decreases cell proliferation in the neurogenic zones of adult female zebrafish (Danio rerio) brain. Neuroscience 277, 306–320. doi: 10.1016/j.neuroscience.2014.06.071
Matzel, L. D., and Kolata, S. (2010). Selective attention, working memory, and animal intelligence. Neurosc. Biobehav. Rev. 34, 23–30. doi: 10.1016/j.neubiorev.2009.07.002
Mery, F. (2013). Natural variation in learning and memory. Curr. Opin. Neurobiol. 23, 52–56. doi: 10.1016/j.conb.2012.09.001
Mery, F., and Kawecki, T. J. (2002). Experimental evolution of learning ability in fruit flies. Proc. Natl. Acad. Sci. U.S.A. 99, 14274–14279. doi: 10.1073/pnas.222371199
Mery, F., and Kawecki, T. J. (2003). A fitness cost of learning ability in Drosophila melanogaster. Proc. R. Soc. B Biol. Sci. 270, 2465–2469. doi: 10.1098/rspb.2003.2548
Messias, J. P., Santos, T. P., Pinto, M., and Soares, M. C. (2016). Stimulation of dopamine D1 receptor improves learning capacity in cooperating cleaner fish. Proc. R. Soc. B Biol. Sci. 283:20152272. doi: 10.1098/rspb.2015.2272
Metcalfe, N. B., and Monaghan, P. (2001). Compensation for a bad start: grow now, pay later? Trends Ecol. Evol. 16, 254–260. doi: 10.1016/s0169-5347(01)02124-3
Morrison, A. B., and Chein, J. M. (2011). Does working memory training work? The promise and challenges of enhancing cognition by training working memory. Psychon. Bull. Rev. 18, 46–60. doi: 10.3758/s13423-010-0034-0
Nicieza, A. G., and Alvarez, D. (2009). Statistical analysis of structural compensatory growth: how can we reduce the rate of false detection? Oecologia 159, 27–39. doi: 10.1007/s00442-008-1194-8
Nilsson, M., Perfilieva, E., Johansson, U., Orwar, O., and Eriksson, P. S. (1999). Enriched environment increases neurogenesis in the adult rat dentate gyrus and improves spatial memory. J. Neurobiol. 39, 569–578. doi: 10.1002/(sici)1097-4695(19990615)39:4<569::aid-neu10>3.0.co;2-f
Niven, J. E., and Laughlin, S. B. (2008). Energy limitation as a selective pressure on the evolution of sensory systems. J. Exp. Biol. 211, 1792–1804. doi: 10.1242/jeb.017574
Ostlund-Nilsson, S., Mayer, I., and Huntingford, F. A. (2006). Biology of the Three-Spined Stickleback. Boca Raton, FL: CRC press.
Pellegrini, E., Diotel, N., Vaillant-Capitaine, C., Maria, R. P., Gueguen, M.-M., Nasri, A., et al. (2016). Steroid modulation of neurogenesis: focus on radial glial cells in zebrafish. J. Steroid Biochem. Mol. Biol. 160, 27–36. doi: 10.1016/j.jsbmb.2015.06.011
Pollen, A. A., Dobberfuhl, A. P., Scace, J., Igulu, M. M., Renn, S. C., Shumway, C. A., et al. (2007). Environmental complexity and social organization sculpt the brain in Lake Tanganyikan cichlid fish. Brain Behav. Evol. 70, 21–39. doi: 10.1159/000101067
Rennison, D. J., Owens, G. L., Heckman, N., Schluter, D., and Veen, T. (2016). Rapid adaptive evolution of colour vision in the threespine stickleback radiation. Proc. R. Soc. B Biol. Sci. 283:20160242. doi: 10.1098/rspb.2016.0242
Rick, I. P., Bloemker, D., and Bakker, T. C. (2012). Spectral composition and visual foraging in the three-spined stickleback (Gasterosteidae: Gasterosteus aculeatus L.): elucidating the role of ultraviolet wavelengths. Biol. J. Linn. Soc. 105, 359–368. doi: 10.1111/j.1095-8312.2011.01796.x
Romero, L. M. (2004). Physiological stress in ecology: lessons from biomedical research. Trends Ecol. Evol. 19, 249–255. doi: 10.1016/j.tree.2004.03.008
Rowe, C., and Healy, S. D. (2014). Measuring variation in cognition. Behav. Ecol. 25, 1287–1292. doi: 10.1093/beheco/aru090
Rystrom, T. L., Bakker, T., and Rick, I. P. (2019). Mate assessment behavior is correlated to learning ability in female threespine sticklebacks. Curr. Zool. 65, 295–304. doi: 10.1093/cz/zoz010
Salas, C., Broglio, C., Durán, E., Gómez, A., Ocaña, F. M., Jiménez-Moya, F., et al. (2006). Neuropsychology of learning and memory in teleost fish. Zebrafish 3, 157–171. doi: 10.1089/zeb.2006.3.157
Salvanes, A. G. V., Moberg, O., Ebbesson, L. O., Nilsen, T. O., Jensen, K. H., and Braithwaite, V. A. (2013). Environmental enrichment promotes neural plasticity and cognitive ability in fish. Proc. Biol. Sci. 280:20131331. doi: 10.1098/rspb.2013.1331
Sandi, C. (2013). Stress and cognition. Wiley Interdiscip. Rev. Cogn. Sci. 4, 245–261. doi: 10.1002/wcs.1222
Sauce, B., Bendrath, S., Herzfeld, M., Siegel, D., Style, C., Rab, S., et al. (2018). The impact of environmental interventions among mouse siblings on the heritability and malleability of general cognitive ability. Philos. Trans. R. Soc. Lond. B Biol. Sci. 373:20170289. doi: 10.1098/rstb.2017.0289
Scholz, J., Allemang-Grand, R., Dazai, J., and Lerch, J. P. (2015). Environmental enrichment is associated with rapid volumetric brain changes in adult mice. Neuroimage 109, 190–198. doi: 10.1016/j.neuroimage.2015.01.027
Schwabe, L., and Wolf, O. T. (2010). Learning under stress impairs memory formation. Neurobiol. Learn. Mem. 93, 183–188. doi: 10.1016/j.nlm.2009.09.009
Schwabe, L., and Wolf, O. T. (2013). Stress and multiple memory systems: from ‘thinking’to ‘doing’. Trends Cogn. Sci. 17, 60–68. doi: 10.1016/j.tics.2012.12.001
Sorce, S., and Krause, K.-H. (2009). NOX enzymes in the central nervous system: from signaling to disease. Antioxid. Redox Signal. 11, 2481–2504. doi: 10.1089/ars.2009.2578
Toli, E. A., Noreikiene, K., DeFaveri, J., and Merilä, J. (2017). Environmental enrichment, sexual dimorphism, and brain size in sticklebacks. Ecol. Evol. 7, 1691–1698. doi: 10.1002/ece3.2717
Velando, A., Costa, M. M., and Kim, S.-Y. (2017). Sex-specific phenotypes and metabolism-related gene expression in juvenile sticklebacks. Behav. Ecol. 28, 1553–1563. doi: 10.1093/beheco/arx129
Warburton, K. (2003). Learning of foraging skills by fish. Fish Fish. 4, 203–215. doi: 10.1046/j.1467-2979.2003.00125.x
West-Eberhard, M. J. (2003). Developmental Plasticity and Evolution. Oxford: Oxford University Press.
Wootton, R. J. (2012). A Functional Biology of Sticklebacks. Berlin: Springer Science & Business Media.
Xu, T., Yu, X., Perlik, A. J., Tobin, W. F., Zweig, J. A., Tennant, K., et al. (2009). Rapid formation and selective stabilization of synapses for enduring motor memories. Nature 462:915. doi: 10.1038/nature08389
Zupanc, G. K. (2001). Adult neurogenesis and neuronal regeneration in the central nervous system of teleost fish. Brain Behav. Evol. 58, 250–275. doi: 10.1159/000057569
Keywords: brain, cognition, costs of learning, Gasterosteus aculeatus, learning, life-history
Citation: Álvarez-Quintero N, Velando A and Kim S-Y (2020) Long-Lasting Negative Effects of Learning Tasks During Early Life in the Three-Spined Stickleback. Front. Ecol. Evol. 8:562404. doi: 10.3389/fevo.2020.562404
Received: 15 May 2020; Accepted: 28 August 2020;
Published: 18 September 2020.
Edited by:
Peter Schausberger, University of Vienna, AustriaReviewed by:
Barbara Taborsky, University of Bern, SwitzerlandIngolf Rick, University of Bonn, Germany
Copyright © 2020 Álvarez-Quintero, Velando and Kim. This is an open-access article distributed under the terms of the Creative Commons Attribution License (CC BY). The use, distribution or reproduction in other forums is permitted, provided the original author(s) and the copyright owner(s) are credited and that the original publication in this journal is cited, in accordance with accepted academic practice. No use, distribution or reproduction is permitted which does not comply with these terms.
*Correspondence: Náyade Álvarez-Quintero, nayade@uvigo.es