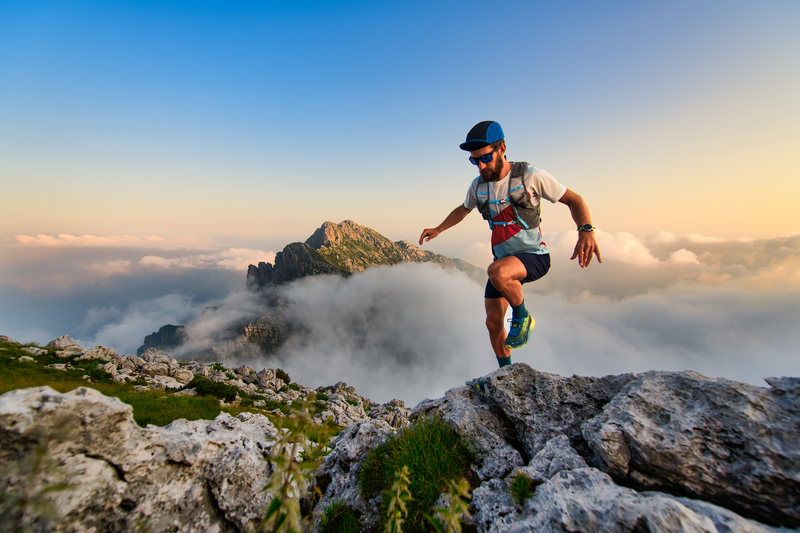
94% of researchers rate our articles as excellent or good
Learn more about the work of our research integrity team to safeguard the quality of each article we publish.
Find out more
PERSPECTIVE article
Front. Ecol. Evol. , 21 July 2020
Sec. Evolutionary and Population Genetics
Volume 8 - 2020 | https://doi.org/10.3389/fevo.2020.00242
This article is part of the Research Topic Coping with Climate Change: A Genomic Perspective on Thermal Adaptation View all 11 articles
The advent of new and affordable high-throughput sequencing techniques allows for the investigation of the genetic basis of environmental adaptation throughout the plant and animal kingdom. The framework of genotype-environment associations (GEA) provides a powerful link by correlating the geographic distribution of genotype patterns of individuals or populations with environmental factors on a spatial scale. We coarsely review the short history of GEA studies, summarizing available studies, organisms, data type, and data availability for these studies. GEA is a powerful tool in climate change research and we therefore focus on climate variables as environmental factors. While our initial aim was to compare results of existing studies to identify common patterns or differences in climate adaptation, we quickly realized that such a meta-analysis approach is currently unfeasible. Based on our literature review we discuss the current shortcomings and lack of data accessibility which impede meta-analyses. Such meta-analyses would allow to draw conclusions on traits and functions crucial to adapt to different environmental, e.g., climate conditions, across species. We thus make a strong call for standardized data and reposition structure for GEA studies. Moreover, the coordinated documentation of candidate genes associated to environmental factors could allow the establishment of a new and additional gene ontology domain “environmental association.” This would systematically link fitness relevant genes to the corresponding environmental factor.
Studying the genomic and molecular underpinnings of adaptation is a central aim in evolutionary biology. As abiotic and biotic conditions vary over space and time, organisms adapt to various local environmental conditions (Bradshaw and Holzapfel, 2001; Kawecki and Ebert, 2004). Alternatively, organisms may also be phenotypically plastic and able to thrive in variable environments. The degree of adaptability and/or plasticity is a crucial parameter in the face of global climate change, which is and will be affecting almost every organism and community across the globe. To make inferences on adaptability versus plasticity, scientists conduct life history experiments, imitating the environmental conditions of interest and determining the organisms’ responses in order to reveal the breadth of their genetic and phenotypic response spectrum. Over decades, laborious quantitative trait locus (QTL) approaches were the only means of obtaining information on the underlying genetic basis targeted by various scenarios of selection (Lynch and Walsh, 1998). However, such quantitative genetic studies are restricted to a limited number of model organisms with sufficient genetic resources (e.g., Mackay, 2014). With the advent of new sequencing technologies, it is now possible to investigate the genomic basis of adaptation to environmental drivers in model organisms, but even more importantly, also in a broad range of non-model organisms (Waldvogel et al., 2020). We can now determine which genes, which regulatory regions, which epigenetic mechanism, etc. play a role in adaptation to different environmental selection pressures. With the accumulation of such studies, we expect that general common patterns will emerge, yielding a deeper understanding of the genomic mechanisms of environmental adaptation and at the same time increasing the predictive power toward new selective challenges. This, however, requires the comparison of studies. In this paper, we will first rehearse the principles and approaches of genotype-environment association (GEA) studies, then review the existing literature, highlight the shortcomings and incompatibilities of current data report practises and finish with suggestions and recommendations that would allow meta-analyses in future.
Genotype-environment association studies, also called environmental association analyses (EAA), provide an approach to detect genetic signatures of selection that result from environmental factors by correlating geographic and genome-wide distributions of allele frequencies with environmental variation (Rellstab et al., 2015; Hoban et al., 2016; Forester et al., 2018). In contrast to classical FST-outlier approaches (see Whitlock and Lotterhos, 2015 for details), GEA allows for the detection of weakly selected loci which only show moderate or even subtle allele frequency shifts (Hancock et al., 2010; De La Torre et al., 2019), a pattern that is characteristic for polygenic adaptation (Pritchard and Di Rienzo, 2010; Berg and Coop, 2014). As in any association study, one needs to keep in mind that identified single nucleotide polymorphisms (SNPs) do not necessarily represent the functional site under selection (Wang et al., 2010). Candidate SNPs can simply be in close linkage to the actual functional site under selection, and the functional site may not be represented in the candidate list, e.g., due to coverage issues at the according site. It is thus important to combine several genomic levels of investigation, e.g., the candidate SNPs as such, candidate SNPs located within genes or in regions up- or downstream of genes with presumed regulatory function, as well as local covariation of candidate SNPs within the range of a defined genomic windows (e.g., resulting from variation in local recombination sites).
The power of GEA increases with (a) the completeness of genotypic information, and (b) the number of populations that cover and possibly replicate the environmental gradient on a broad geographical scale. Genotypic information is derived from genome-wide sequence data of multiple individuals allowing the estimation of allele frequencies per populations. Three major sequencing strategies can be distinguished, resulting in different types of genome-wide sequence information (hereafter referred to as genomic data): (1) reduced-representation sequencing (RRS) data of single individuals, (2) pooled sequencing (Pool-Seq) data of multiple individuals per population, (3) whole genome sequencing (WGS) data of single individuals (more details on pros and cons of the three data types in Waldvogel et al., 2020). Most GEA studies, and especially the pioneer studies of this approach, are based on RRS using SNP-arrays or restriction site associated DNA markers sequencing (RAD-Seq) data (Figure 1). These are highly cost effective and provide a snapshot in targeted marker regions or around restriction enzyme sites (Catchen et al., 2017), at the cost of mainly identifying more or less closely linked variation instead of the functional region itself. Another means to reduce sequencing effort but at the same time cover the complete genome are Pool-Seq approaches, in which multiple individuals (N ∼ 100) per population are pooled for sequencing (Schlötterer et al., 2014). This approach is a cost-effective way of obtaining genome wide information with the only downside of not being able to infer linkage (but see Feder et al., 2012). The third option, WGS, allows to obtain the most detailed information. However, it is characterized by large amounts of sequence data and also the most cost intense option. Here, whole genomes of single individuals are usually sequenced at low coverage. A crucial prerequisite for the latter two approaches is the existence of a reference genome of at least intermediate quality in terms of contiguity and annotation completeness.
Figure 1. Barplot depicting the increasing number of GEA studies per year, and the changes in the underlying sequence data types. SNP array data contain all studies making use of any type of SNP array, -panel and -chips. RAD-Seq summarizes studies using various types of GBS protocols. *Note: assessment until September 2019.
The constantly increasing number of GEA studies (Figure 1) share the common goal of identifying the genomic basis of environmental adaptation. Currently, however, each study focuses on its specific research questions, working on a single or a limited number of species and thus a small fraction of existing biodiversity. To obtain the overall picture on the evolutionary responses of biodiversity to environmental change, we need to compare studies and conciliate results within animal, plant and fungi species or even across the tree of life. A promising way to identify such general patterns are comprehensive meta-analyses (Nakagawa and Poulin, 2012). Indeed, our aim was to conduct such a meta-analysis to identify common patterns of climate adaptation, but we quickly realized that such an approach is currently unfeasible. Even though several recommendation papers covering GEA study designs already exist (especially Rellstab et al., 2015), compatibility among studies was not given. A multitude of data formats and incomplete data sets seem to be a common problem in ecology and evolution research (Whitlock, 2011; Parker et al., 2016; Culina et al., 2018; Poisot et al., 2019). We therefore compared the structure and accessibility of data from a representative fraction of GEA studies published between 2014 and mid-2019 (see assessment criteria below), which revealed the incompatibility or mainly inaccessibility of data, a prerequisite for any meta-analysis.
We focused on studies using mixed effect models as statistical framework implemented in the four currently most widely used tools: Bayenv (Coop et al., 2010), Bayenv2 (Günther and Coop, 2013), Baypass (Gautier, 2015), and LFMM (Latent factor mixed models; Frichot et al., 2013; details in Box 1). In short, Bayenv, a Bayesian approach, tests whether the null model including the environmental factor better fits the data when compared to a model that is purely based on neutral genetic structure. Bayenv2 uses the same approach but is optimized for Pool-Seq data (Günther and Coop, 2013). Baypass (Gautier, 2015) is another Bayesian framework to identify differentiated markers, but correcting for demographic effects. LFMM (Latent factor mixed models; Frichot et al., 2013) introduces neutral genetic structure as a random factor, with the advantage of simultaneously estimating the effects of environmental factors and neutral genetic structure.
BOX 1 | Statistical approaches to genotype-environment associations.
Statistical approaches for the inference of genotype-environment associations in genomic data sets are manifold. Aiming at the detection of multilocus selection patterns in response to environmental predictors, multivariate approaches that analyze many loci simultaneously can be considered most promising. We here outline a non-exhaustive selection of some commonly applied methods (and corresponding tools):
Differentiation-based methods
Allele frequencies of multiple populations are correlated with environmental variables. Statistical methods are based on mixed effect model, fitted to Bayesian or latent frameworks, to test correlations among multilocus allele frequencies of individuals or populations (response variable) and environmental factors (fixed factors), while accounting for population structure and relatedness between populations (random factor).
Bayesian models (implemented in BAYENV, BAYENV2 and BAYPASS; respectively Coop et al., 2010; Günther and Coop, 2013; Gautier, 2015) test for a correlation between allele frequencies and an environmental variable, while accounting for differences in sample size and population structure. The univariate approach to calculated Bayes factors per locus (Bayenv) was further extended by a differentiation-based approach in Bayenv2 (XTX statistics) and robustness of models was further refined in Baypass.
Latent factor mixed models (implemented in LFMM, Frichot et al., 2013) detect correlations between genetic and environmental variation while simultaneously inferring background levels of population structure using unobserved variables (latent factors).
Methods based on constrained ordinations
Multivariate statistical approaches like principal component analyses (PCA) have a long tradition in genetic data analysis (Cavalli-Sforza, 1966). While classical PCA do not use predictors (indirect ordination), methods based on constrained ordinations can find covariation of multiple loci with multivariate environmental patterns. Redundancy analysis (RDA) makes use of constrained ordinations by multivariate linear regression of genetic and environmental data (Forester et al., 2016).
To accumulate a list of studies (Supplementary Table 1) for our meta-statistics, we used the original articles in which the four tools have been published (see above for references). From these we followed the “cited by” option on Google Scholar as link to all citing articles (as of September 2019). These articles were manually curated to only retain GEA studies. We decided to include these four tools only, since they appeared to sufficiently reflect the broad patterns. Other GEA-tools that follow the approach of a redundancy analysis (RDA) are widely used in a broad ecological context, resulting in hundreds of citations of which only a small fraction was relevant for our purpose. Our assessment resulted in 159 empirical GEA studies (Figure 1; Supplementary Table 1), covering multiple data types, and various organisms (Figure 2). Data type, i.e., the type of genomic data used for the GEA, obviously reflected the progress of DNA sequencing technology (Figure 1): starting off with amplified fragment length polymorphism (AFLP) and SNP array data as RRS strategies, progressing toward WGS data of single individuals or pooled populations. Within our assessment, the majority of studies applied RRS strategies to generate the genomic input data and especially RAD-Seq was most commonly used in recent studies. Whilst animal and plant species are almost equally addressed in our assessment, fungal species are heavily underrepresented while other domains of the tree of life are missing (Figure 2A). The application of Bayesian modeling and latent factor mixed modeling is more or less balanced among the assessed GEA studies, nevertheless, LFMM is the most commonly applied tool (Figure 2B). Most studies based their GEA on a combination of multiple tools; according to our search criteria at least one belonged to the group of mixed effect models, the other(s) may have included additional statistical methods (Supplementary Table 1).
Figure 2. The fraction of studies based on animals and plants is almost equal (A). Frequency of tools used in GEA studies (B). Tools which were used in less than four studies are summarized under “others”: RDA, AutoLM, GEMMA, GLMM, Lositan, MLM, partial Mantel test, Moran spectral randomization, Samβada, Selestim, and Tassel.
Molecular ecology studies mainly applied GEAs to investigate the genomic basis underlying local adaptation, leading to a steady increase of GEA data (Figure 1). Numerous statistical frameworks and tools to perform GEAs are available including categorical tests, logistic regressions, matrix correlations, general linear models, and mixed effects models, nowadays accounting for confounding factors such as population structure (reviewed e.g., in Jones et al., 2013; Rellstab et al., 2015; Forester et al., 2018, and see Box 1). Keeping track of state-of-the-art methods, empirical studies therefore generally differ in their choice of the applied approaches. As a consequence, and due to variation in required input data types (especially the genomic data, see below), compatibility of results among GEA studies is currently not given. Due to lacking standards for data deposition of GEA results, both in content and format, a meaningful intersection of results in a meta-analysis framework is not possible. Among the 159 publications that we collected for this study, 19 studies qualified our pre-selection criteria: WGS data (Pool-Seq or WGS of single individuals) and investigation of genome-wide SNPs (in contrast to pre-specified candidate loci or candidate regions). Of these 19 studies only six (32%) contained the minimum information necessary to perform a meta-analysis, namely the gene IDs of candidate genes that were found to be significantly associated to an environmental factor, and the precise environmental factor itself. All other studies only report summary statistics of putative candidates, but do not give candidate specific information. Of these six studies, three studies investigated at least one temperature-related parameter [Fahrenkrog et al., 2017 (Populus deltoides); Henriques et al., 2018 (Apis mellifera); and Tabas-Madrid et al., 2018 (Arabidopsis thaliana)]. For proof of principle, we conducted a mini-meta analysis on these three studies (Supplementary Table 1). The number of temperature-associated candidate genes ranged between 108 and 466. These candidate genes did not overlap among the three species (based on gene annotation), however, we did find an overlap in functions between the candidate genes. There were 26 functions shared between all three species, as for example which DNA binding and oxidoreductase activity (Supplementary Table 1 for complete information on shared functions in three- and two-species comparisons). Interestingly, “cell wall modification” was amongst the functions shared between the two plant species as common temperature adaptation element. Our mini-analysis indicates that different genes are selected in response to different temperature regimes, i.e., no congruence on gene level between species, these genes however share similar functions. Please note that this mini-analysis by all means does not give any conclusive information, but it does show that even between plants and insects common temperature adaptation patterns on a functional level might be expected.
Building on existing guidelines (especially Rellstab et al., 2015) and considering the stepwise pipeline of GEA studies including different options for downstream analyses, we suggest the following standards for the deposition of GEA results to allow for a better compatibility across studies (see Table 1). Hereafter, we summarize the different data types needed for GEA studies, how they are currently obtained and give recommendations on how to deposit the data in a standardized fashion. Standardized data reposition is key to extrapolate results of single GEA studies to more general patterns and conclusions.
Whilst in situ measurements of abiotic data are not available in most cases, public databases provide access to topo-climatic factors globally interpolated over large areas (e.g., WorldClim2, Fick and Hijmans, 2017; CHELSA, Karger et al., 2017), to global hydro-environmental data for watersheds and rivers at high spatial resolution (HydroATLAS, Linke et al., 2019), or to high resolution data on regional scale. Due to high levels of covariance among abiotic, and particularly climate factors, it is common to use the linearly uncorrelated principal components of the complete set of environmental variables. The handling and preparation of environmental input data, also for multivariate approaches, for GEA are detailed in Rellstab et al. (2015).
Irrespective of the source and choice of environmental factors used in a GEA, it is of major importance to deposit the matrix of sample ID, sample location and environmental variables (also including eigenvalues of principal components if applicable; for more details on ecological metadata handling see also Fegraus et al., 2005; Madin et al., 2007; Whitlock, 2011; Michener, 2015). We recommend including a comprehensive variable table in a processable format (not pdf) in the supplement, or as upload to public data archives, such as Dryad1 or gfbio2 (Table 1).
The choice of the genomic data type largely depends on a cost-benefit ratio among sample and genome size. To reveal genomic signatures of selection in association to environmental variability, it is highly important that sampled populations sufficiently cover the geographic area of interest. If, for example, the aim is to investigate genetic variability along a continental climatic gradient, not only the two extremes, but multiple populations along the gradient should be sampled, optimally even in replicates. Moreover, the number of individuals sampled per population needs to be sufficiently high to obtain reliable allele frequency estimates. Sequencing budgets have to be distributed in a way to satisfy both requirements: the number of populations across space and the number of individuals per population. Especially for organisms with large genome sizes, the sampling design can be a challenge and different sequencing strategies can be considered.
Since GEAs are of exploratory nature, we generally do not have pre-knowledge on the targets of selection in respect to the environmental variables of interest. We are thus interested in covering as much of an organism’s genome as possible. Whole genome individual resequencing is the recommended data type of choice, since it comprises individual information along the whole genome. Sequencing a pool of individuals is a cost-effective alternative for all organisms that have intermediate to large population sizes and small to intermediate genome sizes (Schlötterer et al., 2014) while still covering the whole genome (see details above). If, however, RRS is inevitable due to a limited number of individuals per population or very large genome sizes, we recommend targeted exome capture sequencing (e.g., Yeaman et al., 2016), or RNA-Seq (e.g., Roschanski et al., 2016; but see Knight, 2004) over RAD-Seq, SNP-arrays or the sequencing of previously known candidate genes. The rationale is that in GEA studies the targeted entities are candidate genes, which are the basis to infer the biological relevance and function of the selection targets. If gene sets are highly incomplete in the fragmented genomic data, as is the case with RAD-Seq, etc., there is a high chance that the actual target site of selection is not represented in the data. Incomplete results based on insufficient genomic resolution may thus produce misleading patterns (Lowry et al., 2017). However, even in WGS data, many candidate SNPs are just linked sites of variation without functional significance, and the “true” target of selection may also be missed (e.g., due to variance in the coverage distribution). Being able to investigate up- and downstream regions along the genome, and/or using a several kb spanning window-approach increases the reliability of identified putative candidates. Populations samples should cover the distribution range, sufficient number of individuals per population, and use WGS data whenever possible.
Rules and guidelines for data deposition of genomic sequences (including transcriptome sequences) are generally well coordinated for all relevant journals and publishers: genomic sequences as raw or trimmed read data and, if applicable, mapped data to genomic reference sequences have to be uploaded to either of the two major public platforms, NCBI (National Center for Biotechnology Information, United States), or the European Nucleotide Archive (ENA run by EMBL-EBI, United Kingdom). However, GEAs and downstream analyses depend on genomic variants between populations and criteria for variant calling are more or less arbitrary depending on custom settings. For a reliable replicability of results, we therefore recommend the deposition of primary variant tables (e.g., vcf format for WGS data, sync format for Pool-Seq data) as well as the final genomic input table (e.g., lfmm format) for the GEA implementation (Table 1). As for now, variant tables (vcf format) can be submitted to the NCBI SNP database or to EGA (European Genome-phenome Archive) by EMBL-EBI, and all other data types can be uploaded to Dryad or gfbio. Ideally, all data, from sequences to genomic and environmental metadata of a single study, would be deposited in a single database. For example, NCBI BioProjects, and/or ENA Projects could be developed to be more flexible, i.e., accepting various types of metadata.
Genotype-environment associations implementations will ultimately deliver information on loci significantly associated to variation in environmental factors across space (this holds for the actual target site of selection as well as closely linked sites). Structural annotation of individual loci delivers information about whether these loci are part of or contribute to the coding part of the genome, and this information is embedded in the annotation-file (gff) file. To obtain information on the function of genes, to investigate a higher level of organization, and to allow for a deeper biological interpretation of the GEA results, functional annotation is the next step. Gene ontology (GO) databases provide controlled vocabularies for the classification of gene products, and entries are manually curated (Gene Ontology Consortium, 2004). The database is structured in a “loosely hierarchical” manner, with three top (“parent”) domains: molecular function (MF), biological process (BP), and cellular component (CC). The obtained functional information can be used to perform a gene set enrichment analysis to obtain information on significantly overrepresented functions in the candidate gene list versus all genes in the genome. Similarly, information on covered pathways, the position of pathways, etc. can be obtained from the reactome database (GO), as well as reactome information3 can be obtained via a search of the protein sequences versus the interproscan database4.
For a reliable replicability of results and meta-analyses of GEA studies across organisms, the deposition of the gene ID list of selected candidate loci in table format and the full set of protein sequences in fasta format (both corresponding and referencing to the respective genome annotation version used), is crucial (Table 1). An additional column with according GO-IDs for each candidate is desirable, but can also be acquired based on the gene ID.
A first step to validate significant polymorphisms is the verification of allele frequencies by, e.g., Sanger resequencing of candidate SNPs in individuals of the target populations, or experimental populations. Such resequencing approaches can help to decrease the false positive rates, even if conducted for a subset of candidates only. Results of association studies are inherently correlative and consequently, validation of candidate genes requires experimentation (Pardo-Diaz et al., 2015).
Allele frequency information for target populations should be added as supplementary table to the study including individual specific genotypes.
Molecular validation approaches involve gene expression profiling and direct assays to test the molecular and/or ecological function (described in more detail in Pardo-Diaz et al., 2015). The detection of differential gene expression is especially important when significant loci are located up- or downstream of protein-coding regions, indicative of regulatory regions. Assays of MF mostly rely on transgenics, knockouts, knockdowns (e.g., with RNA interference, RNAi) and gene replacements. All of these assays are developed and optimized for model organisms and application to non-model species is more difficult. Nevertheless, constant development of functional tools can open doors for functional characterization also in non-model species, as e.g., with RNAi or CRISPR/Cas systems (Russell et al., 2017).
Optimally, this data would be of sufficient quality to be deposited on a public curated database such as Uniprot (uniprot.org), to make this information publicly available.
Finally, assays of ecological function aim at testing the fitness consequences of allelic substitutions at causal genes (Barrett and Hoekstra, 2011). Such selection experiments (e.g., experimental evolution or evolve and resequencing studies), however, suffer from being highly artificial due to laboratory conditions and being mostly restricted to few established model organisms (Pfenninger and Foucault, 2019). Performing analogous experiments in natural systems (e.g., transplant experiments, more details described in Pardo-Diaz et al., 2015) and adapting them to a broad range of taxa constitutes a major challenge for molecular ecology research. However, given the resources required in terms of work force, money and experimental facilities to causally link genotype with fitness at a single locus (e.g., Rosenblum et al., 2010), it appears unrealistic that more than a tiny fraction of the thousands of already and increasingly identified candidate loci will ever be validated as described above. Thus, meta-analyses of large numbers of taxonomically diverse organisms with similar selection regimes could be an effective means to cross-validate candidate loci playing a role in ecological adaptation.
This list of candidate genes should follow the above-mentioned criteria (also see Table 1), and include gene ID and associated environmental factor.
Experimental validation of candidate genes significantly associated to variation of environmental factors, or candidates obtained from meta-analyses will finally deliver the link from genotype to the environment. The continuous increase in genomic and transcriptomic resources will fuel the accumulation of GEA studies for more and more organisms. The development of novel methodologies for experimental validation of candidate genes will advance the accumulation of knowledge on genes contributing to adaptive responses to environmental variation. With this perspective as a guiding principle, we propose the initiation of a novel GO domain to be called “environmental association (EA)” for the standardized categorization of genes causally associated to environmental variation (Table 1). This GO domain could become the fourth parent domain alongside MF, BP, and CC, adding ecologically relevant information to gene products. Future GO enrichment analyses on the basis of this novel domain will generate a more structured insight in the molecular basis of environmental adaptation, potentially revealing so far hidden relations.
Global climate change and the associated environmental changes will heavily impact ecosystems in their current state. While knowing about the inescapability of these changes, we are largely lacking an understanding of the mechanisms of environmental adaptation and the adaptive potential of organisms (Fitzpatrick and Edelsparre, 2018). Changing climatic conditions impose new selective forces to many ecosystems, yet, with the exception of some few model species, the affected key traits of the majority of organisms remain unknown (Alberto et al., 2013; Gienapp et al., 2014). In order to understand how biodiversity will respond to climate change we will thus need methodological approaches that keep up with the pace of climate change (Waldvogel et al., 2020). To this end, GEA clearly bring the advantage that via the correlation of genomic variation and environmental variation, pre-knowledge of specific traits is not required, but rather target traits can be inferred from the resulting candidate loci (see above). The long list of GEA studies included in this review highlights the timeliness and broad applicability of GEA, especially in non-model species (Supplementary Table 1). So far, we learned from these studies that species indeed adapt to different climatic conditions and that species have multiple options to adapt to the same environmental variables (e.g., temperature). However, we are still lacking meta-analyses which would enable to extend the results of single-species studies to a more comprehensive picture allowing for global conclusions. It would also be highly desirable to include genetic variability and dispersal potential to the analytical framework to actually refine predictions by including species’ potential of rapid adaptation.
GEA are based on the idea of space-for-time and thus approximate the extent of climate change given the range of climate variation across the investigated geographic space (Rellstab et al., 2015). This indirect approximation can only inform about the extent of current climate variation observed in the investigated space and will be blind for new dimensions as are expected for many areas. The only solution to overcome this limitation is measuring genomic change over the actual course of climate change, i.e., tracking populations through time. Such time-for-time approaches are currently being implemented and already allowed the tracking of adaptive trajectories and quantification of the selection regime in natural populations (Pfenninger and Foucault, 2020). The GEA space for time approach can also be embedded in the time-for-time framework by building up time-series of repeated GEA. The repetition of GEA for a given system across a climate change-relevant time horizon can allow to relate changes across space (within single GEA) with changes across time (across repeated GEA) and thus identify the effects of changing climate conditions. Such GEA time-series that are ideally based on WGS of multiple populations across wide distribution ranges will deliver invaluable molecular ecological resources to build accurate prediction models of how species can respond to climate change (Waldvogel et al., 2020). Granted the here proposed standardization of data, GEA in combination with time-series data is a powerful and most promising tool to take on the challenge of understanding the effects of climate change bevor its consequences have brought too much damage.
Systematic deposition of GEA data in a standardized and structured format will set the ground for meta analyses to assess the associations of genotypes and the environment across species, phyla or even the tree of life. Our mini analysis already shows that interesting patterns are to be expected from this data. We here suggest standards for deposition of GEA results and call for a novel GO domain to be included in the gene ontology database. By the implementation of these standards, individual GEA studies will contribute to the growth of a powerful data resource which generates insight in the adaptability of species to environmental variables, especially climate variables. Building these data in a standardized way can furthermore help us to widen the perspective from single to multiple species or even phyla. This can be away forward in investigating biodiversity responses to changing climate conditions and can be the key to improved prediction models.
All datasets generated for this study are included in the article/Supplementary Material.
A-MW, BF, and MP conceived the article. A-MW drafted the manuscript. All authors contributed to data survey and writing of the manuscript.
The authors declare that the research was conducted in the absence of any commercial or financial relationships that could be construed as a potential conflict of interest.
We thank Nikolaus Wohlenberg, Quentin Foucault, and Andreas Wieser for their help in the literature summary. Three reviewers provided constructive recommendations for improving our manuscript.
The Supplementary Material for this article can be found online at: https://www.frontiersin.org/articles/10.3389/fevo.2020.00242/full#supplementary-material
Alberto, F. J., Aitken, S. N., Alía, R., González-Martínez, S. C., Hänninen, H., Kremer, A., et al. (2013). Potential for evolutionary responses to climate change - evidence from tree populations. Glob. Chang. Biol. 19, 1645–1661. doi: 10.1111/gcb.12181
Barrett, R. D. H., and Hoekstra, H. E. (2011). Molecular spandrels: tests of adaptation at the genetic level. Nat. Rev. Genet. 12, 767–780. doi: 10.1038/nrg3015
Berg, J. J., and Coop, G. (2014). A population genetic signal of polygenic adaptation. PLoS Genet. 10:e1004412. doi: 10.1371/journal.pgen.1004412
Bradshaw, W. E., and Holzapfel, C. M. (2001). Genetic shift in photoperiodic response correlated with global warming. Proc. Natl. Acad. Sci. U.S.A. 98, 14509–14511. doi: 10.1073/pnas.241391498
Catchen, J. M., Hohenlohe, P. A., Bernatchez, L., Funk, W. C., Andrews, K. R., and Allendorf, F. (2017). Unbroken: RADseq remains a powerful tool for understanding the genetics of adaptation in natural populations. Mol. Ecol. Resour. 17, 362–365. doi: 10.1111/1755-0998.12669
Cavalli-Sforza, L. L. (1966). Population structure and human variation. Proc. R. Soc. B Biol. Sci. 164, 362–379. doi: 10.2307/2801262
Coop, G., Witonsky, D., Di Rienzo, A., and Pritchard, J. K. (2010). Using environmental correlations to identify loci underlying local adaptation. Genetics 185, 1411–1423. doi: 10.1534/genetics.110.114819
Culina, A., Baglioni, M., Crowther, T. W., Visser, M. E., Woutersen-Windhouwer, S., and Manghi, P. (2018). Navigating the unfolding open data landscape in ecology and evolution. Nat. Ecol. Evol. 2, 420–426. doi: 10.1038/s41559-017-0458-2
De La Torre, A. R., Wilhite, B., and Neale, D. B. (2019). Environmental genome-wide association reveals climate adaptation is shaped by subtle to moderate allele frequency shifts in loblolly pine. Genome Biol. Evol. 11, 2976–2989. doi: 10.1093/gbe/evz220
Fahrenkrog, A. M., Neves, L. G., Resende, M. F. R., Dervinis, C., Davenport, R., Barbazuk, W. B., et al. (2017). Population genomics of the eastern cottonwood (Populus deltoides). Ecol. Evol. 7, 9426–9440. doi: 10.1002/ece3.3466
Feder, A. F., Petrov, D. A., and Bergland, A. O. (2012). LDx: estimation of linkage disequilibrium from high-throughput pooled resequencing data. PLoS One 7:e0048588. doi: 10.1371/journal.pone.0048588
Fegraus, E. H., Andelman, S., Jones, M. B., and Schildhauer, M. (2005). Emerging Technologies. Bull. Ecol. Soc. Am. 86, 158–168.
Fick, S. E., and Hijmans, R. J. (2017). WorldClim 2: new 1-km spatial resolution climate surfaces for global land areas. Int. J. Climatol. 37, 4302–4315. doi: 10.1002/joc.5086
Fitzpatrick, M. J., and Edelsparre, A. H. (2018). The genomics of climate change. Science 359, 29–30. doi: 10.1126/science.aar3920
Forester, B. R., Jones, M. R., Joost, S., Landguth, E. L., and Lasky, J. R. (2016). Detecting spatial genetic signatures of local adaptation in heterogeneous landscapes. Mol. Ecol. 25, 104–120. doi: 10.1111/mec.13476
Forester, B. R., Lasky, J. R., Wagner, H. H., and Urban, D. L. (2018). Comparing methods for detecting multilocus adaptation with multivariate genotype–environment associations. Mol. Ecol. 27, 2215–2233. doi: 10.1111/mec.14584
Frichot, E., Schoville, S. D., Bouchard, G., and François, O. (2013). Testing for associations between loci and environmental gradients using latent factor mixed models. Mol. Biol. Evol. 30, 1687–1699. doi: 10.1093/molbev/mst063
Gautier, M. (2015). Genome-wide scan for adaptive divergence and association with population-specific covariates. Genetics 201, 1555–1579. doi: 10.1534/genetics.115.181453/-/DC1
Gene Ontology Consortium (2004). The gene ontology (GO) database and informatics resource. Nucleic Acids Res. 32, 258–261. doi: 10.1093/nar/gkh036
Gienapp, P., Reed, T. E., and Visser, M. E. (2014). Why climate change will invariably alter selection pressures on phenology. Proc. R. Soc. B Biol. Sci. 281:20141611. doi: 10.1098/rspb.2014.1611
Günther, T., and Coop, G. (2013). Robust identification of local adaptation from allele frequencies. Genetics 195, 205–220. doi: 10.1534/genetics.113.152462
Hancock, A. M., Alkorta-Aranburu, G., Witonsky, D. B., and Di Rienzo, A. (2010). Adaptations to new environments in humans: the role of subtle allele frequency shifts. Philos. Trans. R. Soc. B Biol. Sci. 365, 2459–2468. doi: 10.1098/rstb.2010.0032
Henriques, D., Wallberg, A., Chávez-Galarza, J., Johnston, J. S., Webster, M. T., and Pinto, M. A. (2018). Whole genome SNP-associated signatures of local adaptation in honeybees of the Iberian Peninsula. Sci. Rep. 8, 1–14. doi: 10.1038/s41598-018-29469-5
Hoban, S., Kelley, J. L., Lotterhos, K. E., Antolin, M. F., Bradburd, G., Lowry, D. B., et al. (2016). Finding the genomic basis of local adaptation: pitfalls. practical solutions, and future directions. Am. Nat. 188, 379–397. doi: 10.1086/688018
Jones, M. R., Forester, B. R., Teufel, A. I., Adams, R. V., Anstett, D. N., Goodrich, B. A., et al. (2013). Integrating landscape genomics and spatially explicit approaches to detect loci under selection in clinal populations. Evolution 67, 3455–3468. doi: 10.1111/evo.12237
Karger, D. N., Conrad, O., Böhner, J., Kawohl, T., Kreft, H., Soria-Auza, R. W., et al. (2017). Climatologies at high resolution for the earth’s land surface areas. Sci. Data 4, 1–20. doi: 10.1038/sdata.2017.122
Kawecki, T. J., and Ebert, D. (2004). Conceptual issues in local adaptation. Ecol. Lett. 7, 1225–1241. doi: 10.1111/j.1461-0248.2004.00684.x
Knight, J. C. (2004). Allele-specific gene expression uncovered. Trends Genet. 20, 113–116. doi: 10.1016/j.tig.2004.01.001
Linke, S., Lehner, B., Dallaire, C. O., Ariwi, J., Grill, G., Anand, M., et al. (2019). Global hydro-environmental sub-basin and river reach characteristics at high spatial resolution. Sci Data. 6:283. doi: 10.1038/s41597-019-0300-6
Lowry, D. B., Hoban, S., Kelley, J. L., Lotterhos, K. E., Reed, L. K., Antolin, M. F., et al. (2017). Breaking RAD: an evaluation of the utility of restriction site-associated DNA sequencing for genome scans of adaptation. Mol. Ecol. Resour. 17, 142–152. doi: 10.1111/1755-0998.12635
Lynch, M., and Walsh, B. (1998). Genetics and Analysis of Quantitative Traits. Sunderland, MA: Sinauer, 980.
Mackay, T. F. C. (2014). Epistasis and quantitative traits: using model organisms to study gene-gene interactions. Nat. Rev. Genet. 15, 22–33. doi: 10.1038/nrg3627
Madin, J., Bowers, S., Schildhauer, M., Krivov, S., Pennington, D., and Villa, F. (2007). An ontology for describing and synthesizing ecological observation data. Ecol. Inform. 2, 279–296. doi: 10.1016/j.ecoinf.2007.05.004
Michener, W. K. (2015). Ecological data sharing. Ecol. Inform. 29, 33–44. doi: 10.1016/j.ecoinf.2015.06.010
Nakagawa, S., and Poulin, R. (2012). Meta-analytic insights into evolutionary ecology: an introduction and synthesis. Evol. Ecol. 26, 1085–1099. doi: 10.1007/s10682-012-9593-z
Pardo-Diaz, C., Salazar, C., and Jiggins, C. D. (2015). Towards the identification of the loci of adaptive evolution. Methods Ecol. Evol. 6, 445–464. doi: 10.1111/2041-210X.12324
Parker, T. H., Forstmeier, W., Koricheva, J., Fidler, F., Hadfield, J. D., Chee, Y. E., et al. (2016). Transparency in ecology and evolution: real problems. Real Solutions. Trends Ecol. Evol. 31, 711–719. doi: 10.1016/j.tree.2016.07.002
Pfenninger, M., and Foucault, Q. (2019). Genomic processes underlying rapid adaptation of a natural Chironomus riparius population to unintendedly applied experimental selection pressures. Mol. Ecol. 29, 536–548. doi: 10.1111/mec.15347
Pfenninger, M., and Foucault, Q. (2020). Quantifying the selection regime in a natural Chironomus riparius population. bioRxiv [Preprint]. doi: 10.1101/2020.06.16.154054
Poisot, T., Bruneau, A., Gonzalez, A., Gravel, D., and Peres-Neto, P. (2019). Ecological data should not be so hard to find and reuse. Trends Ecol. Evol. 34, 494–496. doi: 10.1016/j.tree.2019.04.005
Pritchard, J. K., and Di Rienzo, A. (2010). Adaptation - not by sweeps alone. Nat. Rev. Genet. 11, 665–667. doi: 10.1038/nrg2880
Rellstab, C., Gugerli, F., Eckert, A. J., Hancock, A. M., and Holderegger, R. (2015). A practical guide to environmental association analysis in landscape genomics. Mol. Ecol. 24, 4348–4370. doi: 10.1111/mec.13322
Roschanski, A. M., Csilléry, K., Liepelt, S., Oddou-Muratorio, S., Ziegenhagen, B., Huard, F., et al. (2016). Evidence of divergent selection for drought and cold tolerance at landscape and local scales in Abies alba Mill. in the French Mediterranean Alps. Mol. Ecol. 25, 776–794. doi: 10.1111/mec.13516
Rosenblum, E. B., Römpler, H., Schöneberg, T., and Hoekstra, H. E. (2010). Molecular and functional basis of phenotypic convergence in white lizards at White Sands. Proc. Natl. Acad. Sci. U.S.A. 107, 2113–2117. doi: 10.1073/pnas.0911042107
Russell, J. J., Theriot, J. A., Sood, P., Marshall, W. F., Landweber, L. F., Fritz-Laylin, L., et al. (2017). Non-model model organisms. BMC Biol. 15:55. doi: 10.1186/s12915-017-0391-5
Schlötterer, C., Tobler, R., Kofler, R., and Nolte, V. (2014). Sequencing pools of individuals — mining genome-wide polymorphism data without big funding. Nat. Rev. Genet. 15, 749–763. doi: 10.1038/nrg3803
Tabas-Madrid, D., Méndez-Vigo, B., Arteaga, N., Marcer, A., Pascual-Montano, A., Weigel, D., et al. (2018). Genome-wide signatures of flowering adaptation to climate temperature: regional analyses in a highly diverse native range of Arabidopsis thaliana. Plant Cell Environ. 41, 1806–1820. doi: 10.1111/pce.13189
Waldvogel, A.-M., Feldmeyer, B., Rolshausen, G., Exposito-Alonso, M., Rellstab, C., Kofler, R., et al. (2020). Evolutionary genomics can improve prediction of species’ responses to climate change. Evol. Lett. 4, 4–18. doi: 10.1002/evl3.154
Wang, K., Dickson, S. P., Stolle, C. A., Krantz, I. D., Goldstein, D. B., and Hakonarson, H. (2010). Interpretation of association signals and identification of causal variants from genome-wide association studies. Am. J. Hum. Genet. 86, 730–742. doi: 10.1016/j.ajhg.2010.04.003
Whitlock, M. C. (2011). Data archiving in ecology and evolution: best practices. Trends Ecol. Evol. 26, 61–65. doi: 10.1016/j.tree.2010.11.006
Whitlock, M. C., and Lotterhos, K. E. (2015). Reliable detection of loci responsible for local adaptation: inference of a null model through trimming the distribution of FST. Am. Nat. 186, S24–S36. doi: 10.1086/682949
Keywords: meta-anaylses, literature survey, environmental association analysis, gene ontology category, candidate genes
Citation: Waldvogel A-M, Schreiber D, Pfenninger M and Feldmeyer B (2020) Climate Change Genomics Calls for Standardized Data Reporting. Front. Ecol. Evol. 8:242. doi: 10.3389/fevo.2020.00242
Received: 17 March 2020; Accepted: 06 July 2020;
Published: 21 July 2020.
Edited by:
Inês Fragata, University of Lisbon, PortugalReviewed by:
Helena Kristiina Wirta, University of Helsinki, FinlandCopyright © 2020 Waldvogel, Schreiber, Pfenninger and Feldmeyer. This is an open-access article distributed under the terms of the Creative Commons Attribution License (CC BY). The use, distribution or reproduction in other forums is permitted, provided the original author(s) and the copyright owner(s) are credited and that the original publication in this journal is cited, in accordance with accepted academic practice. No use, distribution or reproduction is permitted which does not comply with these terms.
*Correspondence: Markus Pfenninger, TWFya3VzLlBmZW5uaW5nZXJAc2VuY2tlbmJlcmcuZGU=
Disclaimer: All claims expressed in this article are solely those of the authors and do not necessarily represent those of their affiliated organizations, or those of the publisher, the editors and the reviewers. Any product that may be evaluated in this article or claim that may be made by its manufacturer is not guaranteed or endorsed by the publisher.
Research integrity at Frontiers
Learn more about the work of our research integrity team to safeguard the quality of each article we publish.