- Laboratoire d’Anthropologie et d’Imagerie de Synthèse, CNRS UMR 5288, Faculté de Médecine Purpan, Toulouse, France
The field of ancient genomics has undergone a true revolution during the last decade. Input material, time requirements and processing costs have first limited the number of specimens amenable to genome sequencing. However, the discovery that archeological material such as petrosal bones can show increased ancient DNA preservation rates, combined with advances in sequencing technologies, molecular methods for the recovery of degraded DNA fragments and bioinformatics, has vastly expanded the range of samples compatible with genome-wide investigation. Experimental procedures for DNA extraction, genomic library preparation and target enrichment have become more streamlined, and now also include automation. These procedures have considerably reduced the amount of work necessary for data generation, effectively adapting the processing capacity of individual laboratories to the increasing numbers of analyzable samples. Handling vast amounts of samples, however, comes with logistical challenges. Laboratory capacities, equipment, and people need to be efficiently coordinated, and the progress of each sample through the different experimental stages needs to be fully traceable, especially as archeological remains of animals or plants are often provided and/or handled by many different collaborators. Here we present CASCADE, a laboratory information management system (LIMS) dealing with the specificities of ancient DNA sample processing and tracking, applicable by large and small laboratories alike, and scalable to large projects involving the analysis of thousands of samples and more. By giving an account of the specimen’s progress at any given analytical step, CASCADE not only optimizes the collaborative experience, including real-time information sharing with third parties, but also improves the efficacy of data generation and traceability in-house.
Introduction
The first draft of the human genome was released in 2001, following 20 years of extensive collaborative efforts among large research centers across the world (Lander et al., 2001; Venter et al., 2001). The first prehistoric human genome was released almost a decade later (Rasmussen et al., 2010) and was immediately followed by the genome characterization of two extinct groups of hominins, Neanderthals (Green et al., 2010) and Denisovans (Reich et al., 2010). Now, another decade later, the number of human genomes characterized has become truly astronomical, and it is estimated that several millions of living individuals, and several thousand prehistoric ones, have had their genome sequenced (Marciniak and Perry, 2017; Brunson and Reich, 2019Regalado, 2019). Extensive time-series of genomes are also becoming available for organisms other than humans, including their pathogens (e.g., Yersinia pestis, the agent of the plague, see Spyrou et al., 2019 for a review), animal domesticates (e.g., horses, Fages et al., 2019), and plants (e.g., maize, Kistler et al., 2018). The number of published metagenomes sampled from the environment (e.g., ancient lake sediments, Pedersen et al., 2015) and mammal-associated microbial communities (e.g., in ancient dental calculus, Mann et al., 2018) is also on the rise.
The reasons for such a success are manifold. First, the DNA data production capacity and costs of next-generation sequencing instruments have constantly improved (Metzker, 2010; Goodwin et al., 2016). Second, specific types of osseous material, such as petrosal bones (Pinhasi et al., 2015) and tooth cementum (Damgaard et al., 2015), have been found to show better DNA preservation rates than previously explored sources, and to be generally less prone to contamination by environmental microbes. These developments have lowered the sequencing efforts needed to retrieve significant coverage of the focal genome and have consequently reduced the time required for and costs incurred by ancient genome characterization. Third, an entire array of innovative molecular solutions has been developed to facilitate the recovery and manipulation of ancient DNA (aDNA) molecules, including DNA extraction (Dabney et al., 2013; Boessenkool et al., 2017; Glocke and Meyer, 2017; Korlević and Meyer, 2019), incorporation into DNA libraries (Gansauge and Meyer, 2013; Gansauge et al., 2017; Carøe et al., 2018; Rohland et al., 2018), handling of DNA damage (Gansauge and Meyer, 2014; Gansauge et al., 2017), and target enrichment of hundreds of thousands to over a million pre-selected loci across the genome (Haak et al., 2015; Harney et al., 2018; Mathieson et al., 2018; Olalde et al., 2018). As a result, aDNA projects have become increasingly large-scale, and it is now common that several hundred of specimens are processed within a single study. For example, the survey of genome-wide variation among humans in Iberia carried out by Olalde and colleagues included 271 individual specimens spanning the last ∼5,000 years (Olalde et al., 2019), while the study from Damgaard et al. (2018) released no fewer than 137 genome sequences of ancient humans from across the Eurasian steppes and spanning the last 4,000 years.
This increase in scale is impressive, especially when contrasted with the challenges ancient genomics still faces, first of which is sample availability. While new methods have expanded the range of suitable samples, finding relevant samples in the first place can be very difficult. Once a promising excavation site is found, the number of suitable samples at this site is a priori unknown and may only become apparent after months if not years. However, once unearthed, samples should be processed rapidly to prevent further degradation of endogenous DNA. Unfortunately, sample processing is often destructive and hence reduces the availability of samples for other fields like e.g., Archeology. It is therefore important to assess as swiftly as possible whether samples found at a given site are suitable for ancient genomics to limit material destruction as much as possible, either because initial screening is negative or enough material has been obtained. This also helps prioritizing areas and/or time periods that require further excavation and sampling. Timely processing however, can be challenging due to available laboratory capacity. Because of the risk of further degradation and contamination, the handling of aDNA requires dedicated, well-isolated clean rooms which have to undergo regular cleaning and sterilization cycles between uses in order to prevent additional contamination. As a result of these specific requirements suitable laboratory space is often limited. Strict experimental procedures, laboratory facility maintenance, and resource management are thus indispensable for efficient sample processing especially when trying to scale up analysis. It is furthermore imperative that laboratory personnel have access to the full status of any given sample at all times, especially as experiments may require the processing of specific samples and/or the replication of particular steps. In addition to informing decisions and providing context for future specimens, being able to track sample information throughout the whole aDNA data production process is paramount to a number of quality control meta-analyses, such as detecting batch effects, including contaminated experimental sessions. These processes are also crucial for assessing performance of steps and/or protocols, project reporting, or for obtaining preliminary background information for grant applications. Such achievements require tools that are up to the task of staying on top of constantly growing and changing data as well as all underlying procedural steps.
In this study, we present CASCADE, a Laboratory Information Management System (LIMS), tailor-made for the genetic processing of paleontological remains. The Custom-made Archiving System for the Conservation of Ancient DNA Experimental data implemented within CASCADE provides a user-friendly, web-based environment to track all experimental phases involved in the preparation, extraction, library construction, amplification and sequencing of aDNA. It delivers a full environment capable of both storing and querying the information from a web browser. It also supports barcode assisted identification of tube content at all documented experimental steps. It is available for free, together with a companion documentation that provides full installation instructions and user guidelines. We anticipate that CASCADE will facilitate the traceability, sharing and long-term conservation of the experimental metadata associated with aDNA analyses.
Materials and Methods
CASCADE Setup
In order to make CASCADE as portable as possible, we decided to embed it inside a virtual machine (VM) available for all major operating systems (OS, e.g., Windows, Linux, Mac OSX). This was achieved using Oracle VM VirtualBox v5.2.1 A VM is a fully self-contained simulated computer system that can be executed on any host system (i.e., a physical computer) that has the VM software installed and enough resources for the simulated system. The VM created for CASCADE uses only a single simulated CPU and 2GB of RAM, and hence should be able to run on most currently available computer systems. It was tested without returning problems on desktop and laptop computers with Intel CORE i5 and CORE i7 CPUs and 8GB of RAM using either Windows 10, MacOS El Capitan/Mojave, or Ubuntu 18.04 LTS as host systems. As guest OS (i.e., the operating system running inside the VM), we chose Ubuntu 18.04 LTS,2 which is available free online.
The virtual disk image (VDI), i.e., the file that contains the simulated hard disk of the VM, requires around 10GB of disk space which is mostly due to the size of the guest OS and other required software. Software includes the NGINX3 v.1.14.0 web-server executing the CASCADE source code, and the MySQL4 v14.14 database management system that handles all the data stored. Other prerequisites are the Laravel5 PHP framework v5.6, the Vue.js6 JavaScript framework v2.6.10, and the Bootstrap 47 JavaScript & CSS library. CASCADE’s back-end source code is fully written in PHP 7.2 using Laravel’s classes while the front-end is written in JavaScript with the support of Vue.js. Styling is based on Bootstrap 4 with the addition of vector graphics from the Font Awesome8 vector icon library. Vector graphics sourced from this library are under the Creative Commons Attribution 4.0 International license.9
CASCADE is accessed via web browser. It was developed specifically for Mozilla Firefox but has also been tested with recent versions of Google Chrome without encountering problems. Especially when the database reaches larger numbers of records (i.e., ten-thousands of entries), Chrome seems to perform better, although parts of the layout may be interpreted differently to Firefox. The source code as well as the fully installed and configured VM can be provided upon request (please direct requests to one of the following authors: LO, ludovic.orlando@univ-tlse3.fr; XM, xavier.mata@univ-tlse3.fr; CD, clio.dersarkissian@univ-tlse3.fr). We also provide an installation manual alongside the VM for those users willing to create and configure their own VM for running CASCADE. However, please note that the source code is available only upon request and requires an Atlassian Bitbucket10 account which can be created for free. Using Bitbucket’s collaborator feature allows us to safely exchange the code with trusted parties. In case such an account is not wanted, the source code can also be found in a folder inside the VM as indicated in the companion manual.
Our goal is to provide CASCADE free to all scientists interested. The procedures for obtaining CASCADE were, however, developed as precautionary measures to reduce the risk of hacking. To maximize safety, we also recommend installing CASCADE on computers connected to firewall-protected, laboratory-internal networks and allowing access from outside the network only through VPN.
It is the user’s responsibility to ensure the security of the computer installation, network configuration and password management for any computer running CASCADE in order to maintain the safety of the data stored in CASCADE. Hence, it should be installed and run only on computers for which access control can be guaranteed at all times, either directly or through the network. Moreover, as CASCADE can be used to store personal data (e.g., from collaborators and study participants), it is subjected to legislation and rules about the protection of personally identifiable information in force in the users’ country, state and/or institutions (e.g., GDPR in the European Union) and with which the user must comply (e.g., personal data anonymization, encryption, ethical clearance). Like all software under MIT license, CASCADE is provided “as is” without warranty of any kind. As a consequence, the authors of CASCADE cannot be held accountable for data/system loss as a result of security breaches.
Database Schema Design
Each relational database project begins with the schema design during which all attributes required to describe every aspect of the system are defined. These are then further processed to remove redundancies and establish relationships between the different parts of the data. This procedure is called database normalization, which aims at satisfying so-called “normal forms” (Codd, 1970). A data model that reaches at least the third normal form is usually described as “normalized” (Date, 2003). Once in this configuration, most if not all anomalies that might arise from adding, deleting, or modifying data are removed. This feature guarantees the present and future integrity of the data and represents one of the major strengths of relational databases. Another one is that the relations established between the data sets allow flexibly combining them in different ways so as to query exactly the information required for certain analyses or tasks.
Figures and Screenshots
Screenshots were taken using the screenshot tool of MacOS Mojave (v10.14.6) and subsequently processed in GIMP11 v.2.10. Where necessary, text was replaced with a “– REDACTED –” label to not expose account and personal information of our collaborators. All other Figures were created using Inkscape12 v0.92.4 and vector graphics from the Font Awesome vector icon library.
Results
General Overview
The core functionality of any LIMS revolves around the handling and tracking of laboratory specimens. In the case of most aDNA projects, these requirements include sample registration, pulverization, and DNA extraction from the obtained powder, followed by library preparation, amplification, quantification, pooling and sequencing. Additional steps which might be used to increase quality, specificity, or yield of endogenous sequences consist of DNA damage repair by enzymatic treatment, and/or DNA capture methods for target enrichment of specific DNA sub-fractions. A commonly used repair method is the incubation of aDNA extracts with an enzymatic mixture consisting of Uracil DNA glycosylase and Endonuclease VIII (USER treatment, New England Biolabs), which can eliminate most or even all those cytosines that have been deaminated post-mortem (Rohland et al., 2015), and which represent the most common aDNA damage (Briggs et al., 2007).
All the steps described above are implemented in the CASCADE processing pipeline (Figure 1). At each stage of the pipeline, primary and secondary data relating to the experimental step are recorded. Primary data include attributes directly related to a given specimen, e.g., the condition of the sample, the amount of powder obtained, or the volume of enzyme used for treatment. Secondary data on the other hand refer to attributes that are only indirectly related to the specimen e.g., the coordinates of the excavation site, the return address of the sample provider, or the details of the protocol used for processing. All these data are handled by CASCADE leveraging a relational database recording 1,155 attributes (fields or columns) grouped into 97 different record types (stored as record sets or tables) which are linked together through a network of 374 private key – foreign key relations (Tables 1–3).
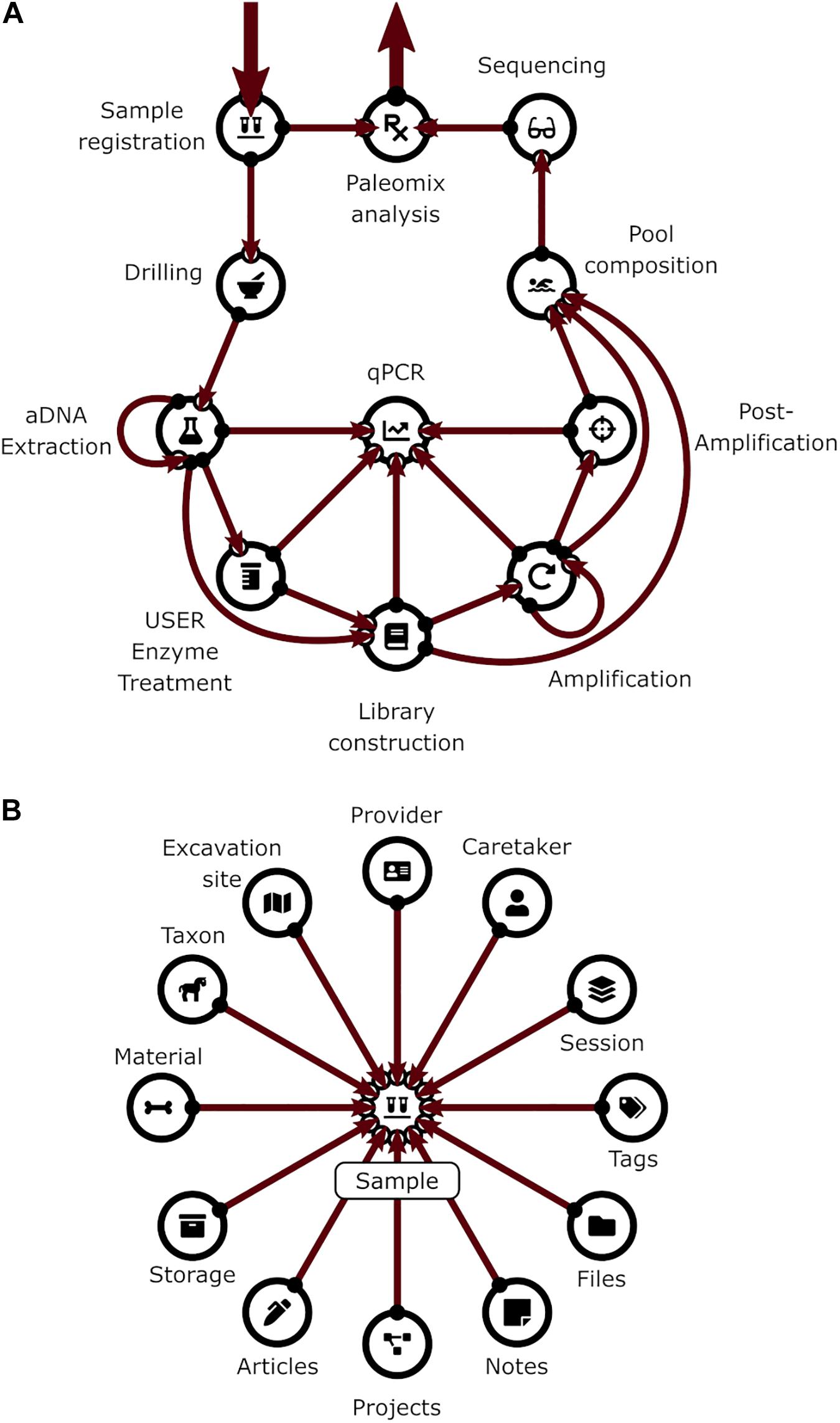
Figure 1. (A) Pipeline backbone graph showing the flow of experimental data through CASCADE. Samples and their related data are registered first. Subsequently, they proceed through the pulverization or “Drilling” stage to the extraction step. Once extracted, they continue to library generation, either directly or after undergoing USER treatment. Should analyses reveal that the initial extraction round did not succeed in releasing satisfactory endogenous material, new extractions can also be generated from the remaining extraction pellets (loop from and to extraction) before being further processed. Generated libraries can be pooled immediately or after additional amplification. Again, should initial amplification prove not to be sufficient, re-amplification (loop from and to amplification), target enrichment, or other post-amplification protocols can be executed prior to pooling. All intermediates from extraction to post-amplification can be included in analyses via the feature “qPCR.” Paths to the sequencing data generated for several samples of the same specimen (a “Sample group”) are combined and fed in the Paleomix computational pipeline (Schubert et al., 2014) for retrieving basic estimates, including endogenous content. The results obtained represent the final pipeline output. (B) Display of the most important non-experimental data types related to sample data. Samples have the largest number of relations to non-experimental data. Some of these are shared with other experimental data types (e.g., “Storage,” “Notes,” “Files”). Icons in both panels are the same as those used for CASCADE’s menus and taken from the Font Awesome vector icon library.
Upon registration of a given sample, the system generates a unique identifier and then incorporates additional information as the sample progresses through the pipeline. This feature enables unambiguous identification at every step until the sample reaches the final stage of data analysis. The partitioning of data and their interconnection by a web of relations allows users to flexibly combine individual data sets to query exactly the information required for a certain analysis or task. This flexibility is made available to users through a query system allowing them not only to create queries, but also to store and re-run them at a later stage (e.g., after new content has been added to the database). In effect, it grants users the power to add to the functionality of CASCADE as they see fit. In order to provide an example of what is possible, we have used this query system to generate a series of pre-built queries which we find useful in our own laboratory practice. These include, for example, summaries of samples available and/or processed per individual archeological site, region and/or time period, and overviews of the status of any given sample in the experimental production chain.
The Database User Interface
The database interface consists of two main parts, corresponding to the “Presets” and the “Experiments” sections. The “Presets” section describes all data that can exist independently of experiments but which form the majority of dependencies for experimental entries. These include, among other things, collaborator and user contact information, excavation sites, taxa, materials, protocols, and oligo-nucleotides used for preparing DNA library adapters, library indexing and amplification. The “Experiments” section mainly handles the actual experimental data, but also includes data types that are closely associated, such as imaging records (if available), sample groups, and analytical results of different types, depending on the experimental step considered.
In a typical use case scenario, any newly arrived sample is first checked for its “Presets” requirements (e.g., new collaborator, excavation site etc.) before the sample itself is added to CASCADE. Upon registration, the sample is automatically added to a “Waiting list” (Figure 2) which makes it immediately visible to all laboratory staff and enables them to book it for processing directly through this interface. Once processing starts, the sample status is continuously updated, which is communicated through the waiting list and also displayed on the sample’s detail page. For each pipeline step a new entry is automatically created ensuring that the list is always up to date and informing everyone interested about the current status of the sample and the progress it has made. To illustrate this feature, we have pre-filled CASCADE with a toy example, which shows specimens at different stages of the processing pipeline. More details about the database interface can be found in the companion manual.
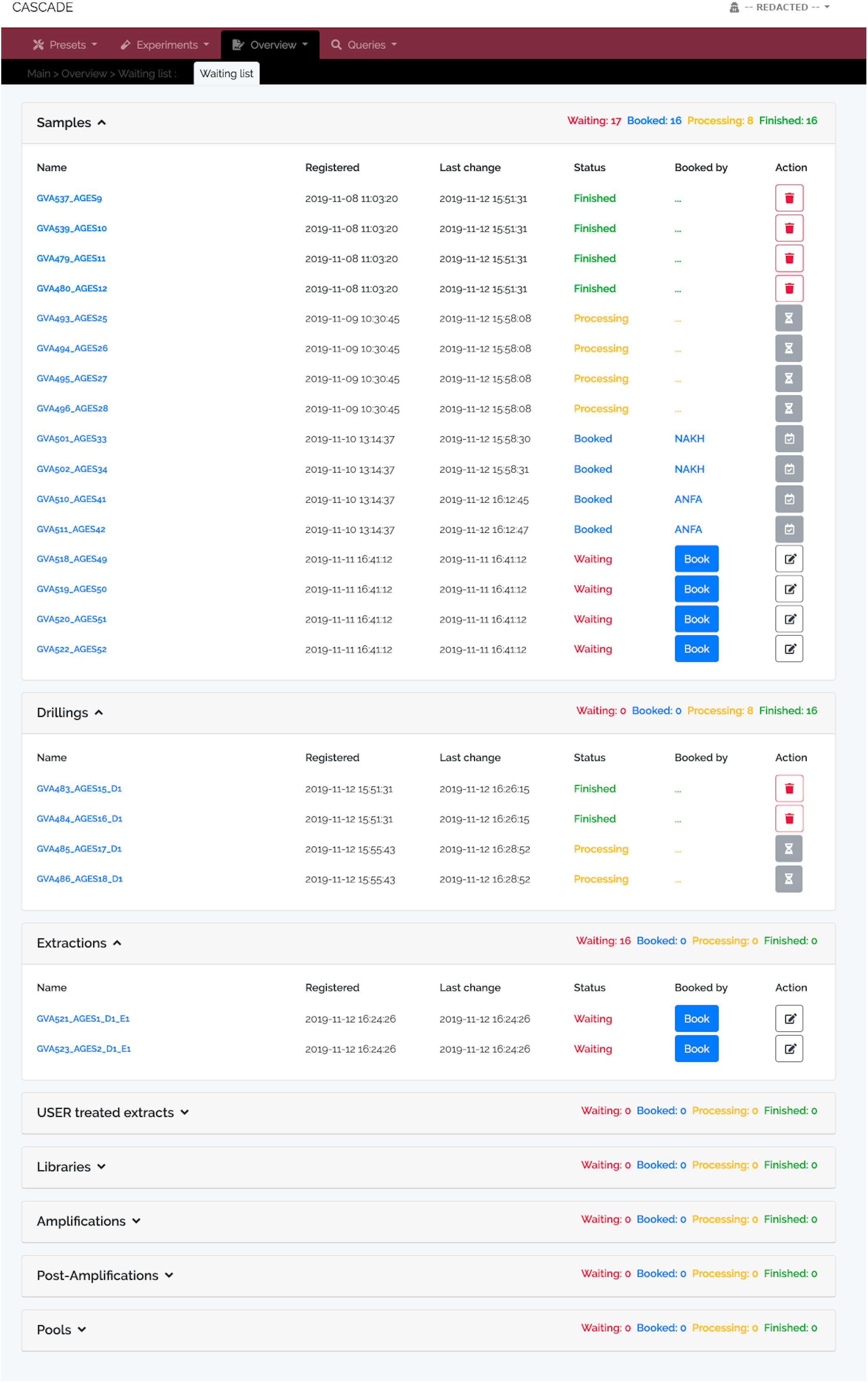
Figure 2. Waiting list showing specimens at different processing stages. Registered samples (“Samples,” top), “Drillings” (middle), and “Extractions” (bottom). No records are available for the other experimental data types at this moment, and, hence, none are displayed. The “Samples” panel shows four samples each either “Waiting” to be processed, “Booked” for processing (by two different users “NAKH” and “ANFA”), currently undergoing “Processing,” or already “Finished.” “Finished” entries show the delete trash button on the right allowing the user to remove them from the list and avoid clutter. Deletion does not happen automatically; it thus provides users with feedback of what has been done. Waiting entries have an edit button instead, which leads to a menu allowing all users to attach notes to the entry, e.g., special experimental conditions must be applied, location of the material, etc.
Experimental Data Entry
In our own laboratory experience, new samples tend to arrive in batches rather than individually. Furthermore, we also almost exclusively process groups of samples in each pipeline step in order to maximize our clean lab capacity. We hence decided to organize experimental data entry for each step in “Sessions,” with each session corresponding to a group of samples processed at the same time. Not only does this allow us to keep track of which specimens were subjected to the same conditions, and hence to spot batch effects (e.g., failure to amplify caused by using a new tube of enzyme or switching reagent supplier), it also helps distinguish whether contamination present in one particular specimen could have been introduced in the laboratory or prior to arrival. It further makes data submission more convenient as entries that share many of their parameters can be submitted together rather than one by one, hence reducing the workload and the likelihood of introducing mistakes. On top of that, we decided to allow users to pause data submission and continue it at a later point in time. As a result, laboratory staff can make efficient use of the inevitable waiting time e.g., during pulverization, centrifugation, or incubation periods, which is a real asset, especially in a laboratory setting. While waiting for a specific step to be completed, the data accumulated until the ongoing experimental step can be submitted and the process suspended once the experimental step has finished. This feature thus enables to leverage any available experimental downtime thereby improving overall laboratory efficacy. In order to achieve this, however, we had to implement an intermediate set of tables to hold the temporary data. We therefore decided to provide this mechanism only for experimental data types.
A specimen’s progress through the different experimental steps (i.e., pulverization, DNA extraction, USER treatment, library construction, qPCR, amplification, capture/target enrichment, pooling, and sequencing) can be easily monitored via a “Status bar” feature available on the detail page of the corresponding sample (Figure 3A). A click on the icon of any type of experiment in this bar (Figure 3B) displays a list of all related entries of that type stored in the database (Figure 3C) and links directly to the corresponding detail pages should the processing of the focal entry be completed. While this feature allows tracking individual samples, tracking whole sets of samples is often equally desirable. For this reason, three higher-level detail menus, one each for collaborators, excavation sites, and taxa, were implemented (Figures 4–6). Each of these menus shows a list of all related samples (e.g., provided by a certain collaborator, excavated at a given site, or deriving from the same taxon) and hence helps sample management, provides archeological context, and supports decision making.
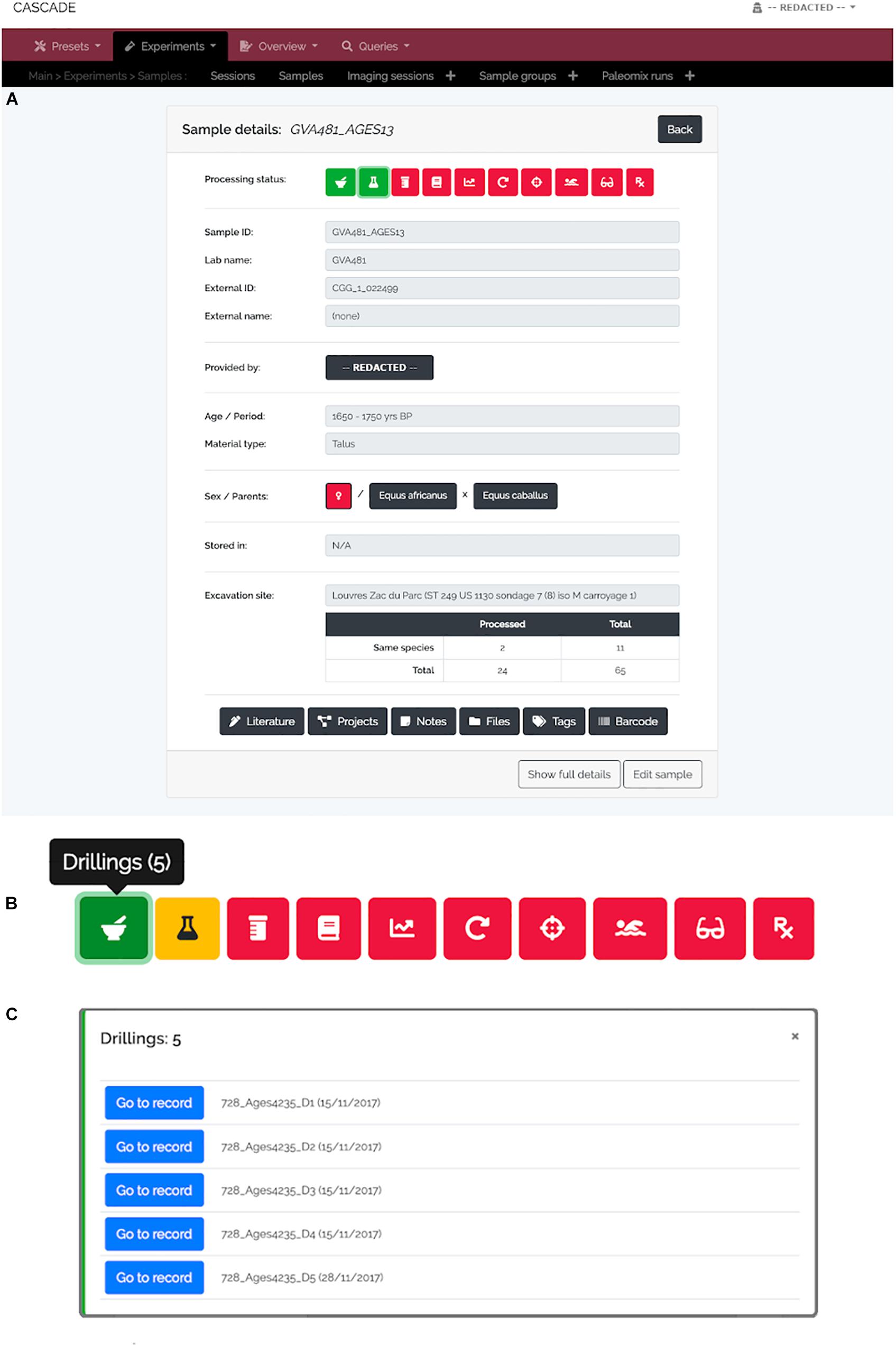
Figure 3. Sample detail view and status bar. (A) For each sample, a “Detail view” is available that summarizes the most important details about a given sample. The full details are available via the “Show full details” button at the bottom left. At the top of the menu, the “Status bar” shows the current processing status of a sample (red = “not done yet,” yellow = “currently processed,” green = “finished”). (B) The icons in the status bar represent the different experimental types (from left to right: “Drillings,” “Extractions,” “USER Treatments,” “Libraries,” “qPCRs,” “Amplifications,” “Post-Amplifications,” “Pools,” “Sequencing runs,” and “Paleomix runs”) and open a pop-up menu (C) when clicked in order to list all available specimens per type. The “Go to record” button then allows jumping directly to the detail view of the record of interest.
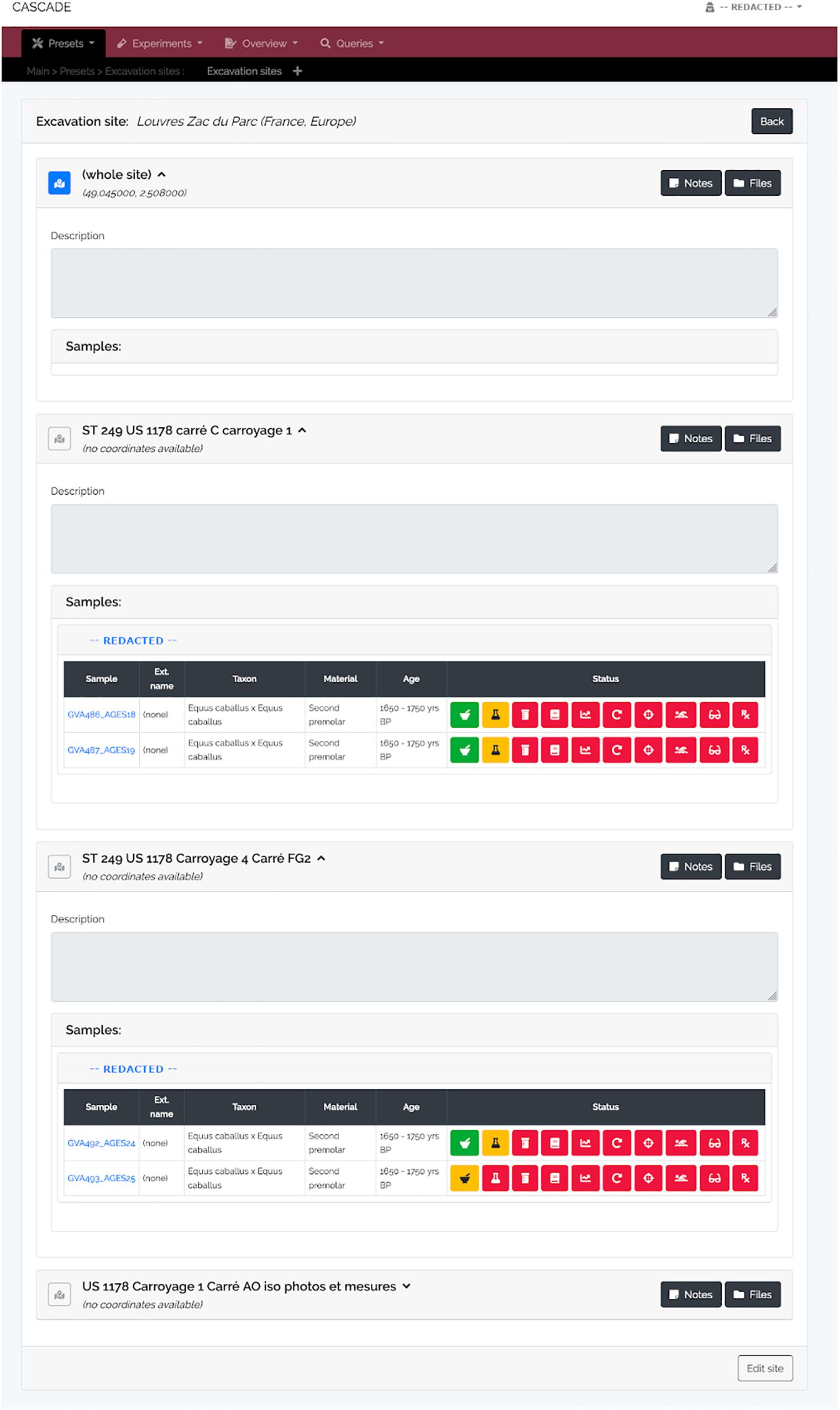
Figure 4. Excavation site detail view. For each site and its sub-sites (e.g., sectors in a larger area, different tombs or burial mounds, etc.) all available samples are listed, grouped by the sample provider. A click on the blue sample name leads to the sample’s detail view (see Figure 3A) while clicking on the provider name (here shown as “REDACTED” for privacy reasons) leads to that of the provider (see Figure 6). The displayed status bar works in the same way as described previously (see Figure 3B). As an additional feature, should GPS coordinates for a given site be available, clicking on the then blue map icon (gray and inactive if no coordinates are available) will automatically lead to a Google Maps view of that specific location.
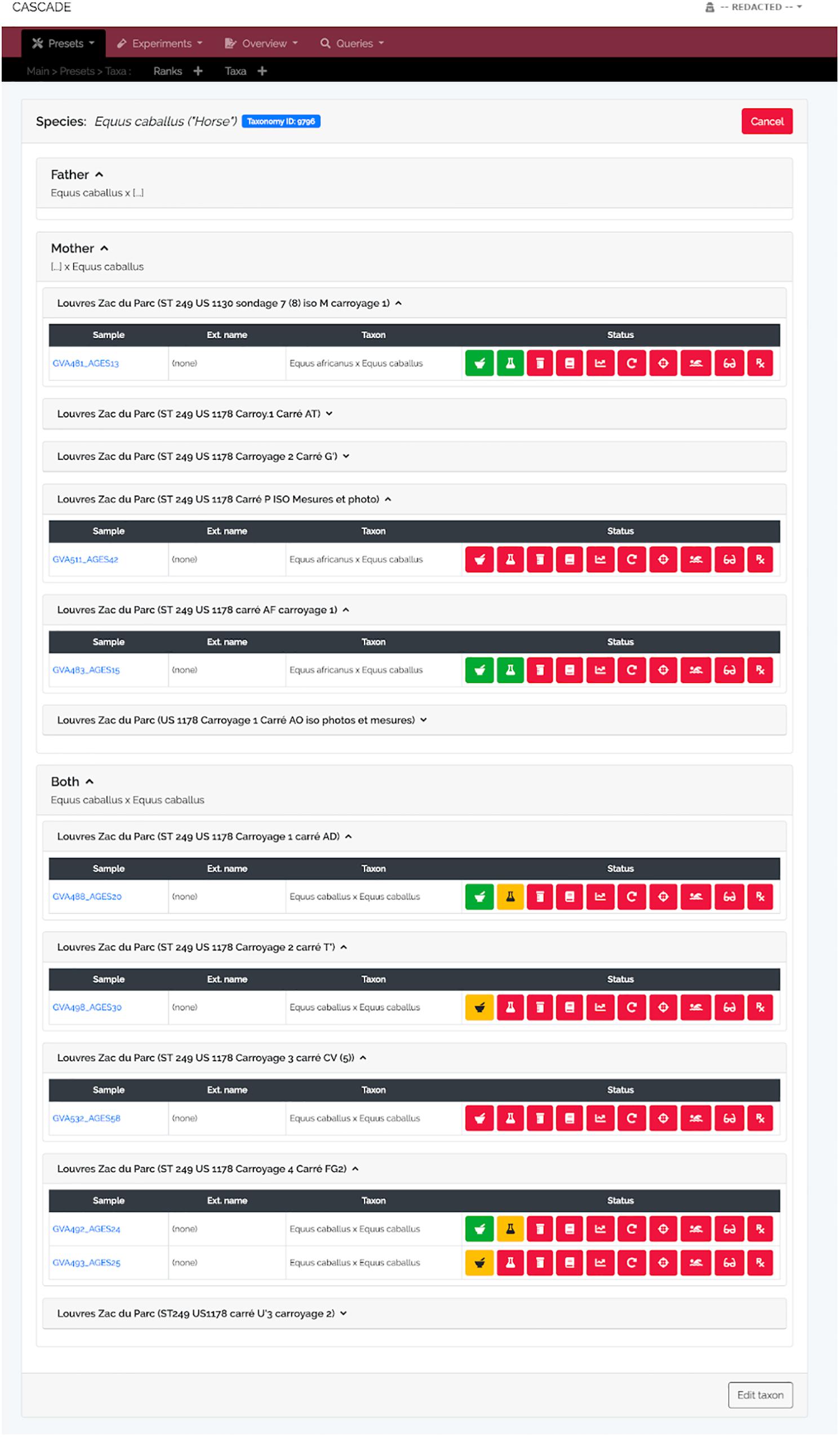
Figure 5. Taxon detail view. Similar to the detail view for excavation sites, the taxon detail view lists all samples stored in CASCADE that are associated with the selected taxon. However, only those samples are listed for which there is DNA-based taxon assignment. Samples for which the taxon is estimated on the basis of other criteria (e.g., morphology) will not be listed, as this would rapidly lead to excessively large, thus, impracticable numbers of samples to be displayed. Retrieved samples are grouped by the taxon of the father, mother, and both resulting in the first two groups listing exclusively hybrids (e.g., mules and hinnies). If an NCBI Taxonomy ID was submitted together with the taxon record, a blue button at the top links directly to the entry in the NCBI Taxonomy Browser.
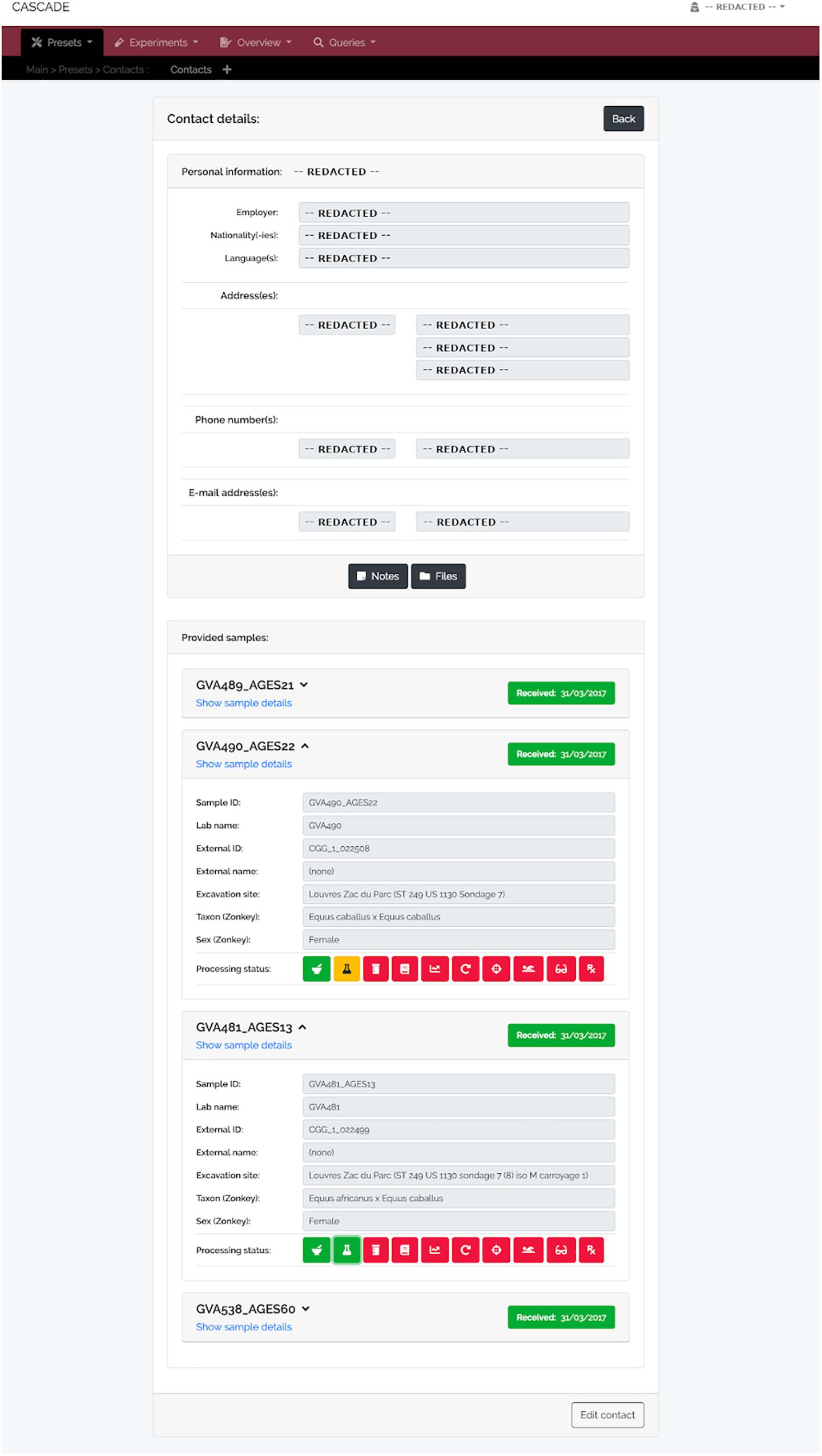
Figure 6. Contact detail view. This menu displays the contact information for each record stored in CASCADE (here shown as “REDACTED” for privacy reasons). It also provides direct access to all samples ever contributed by the example collaborator, as well as their details and status. This property allows evaluating how far samples have progressed through the pipeline which helps this collaborator to assess on demand whether enough data have already been collected to proceed with a project’s next step.
The Query System
As mentioned above, one of the great strengths of relational databases is to offer the possibility of freely combining data from different related records. This feature facilitates the detection of patterns or correlations between parts of the data that otherwise might not have been visible. This kind of data manipulation is usually done using Structured Query Language (SQL) queries. However, due to the vast possibilities of SQL queries and the power that they have over the data and structure of a database, making them available to users is very risky. This risk is both due to the damage that inexperienced users can inadvertently cause but also due to intentional damage by hackers. Another problem is that even SQL queries providing simple output can be complex to write, especially for beginners.
To address these problems, we implemented a Graphical User Interface (GUI) for the creation and execution of SQL “SELECT” statements which protects against accidental damage by users while facilitating the construction of complex queries. To further assist users in creating more complex data requests, simpler statements (termed “Moves”) can be created and stored and then later used in more complicated queries (“Strategies”) like normal tables. “Strategies” further allow all possible set operations (i.e., union, intersection, exclusion, and subtraction) on tables and subqueries, and hence provide all data operations that most users will ever need. We also provide a number of predefined queries that we have found useful in our workflow and which appear in the “Strategies” section. The first retrieves the number of samples each of our providers has entrusted to us. This feature helps keep track of all our collaborators, regardless of the number of samples provided. Of equal importance is another query that retrieves the list of collaborators who have contributed samples to a specific project. While CASCADE provides the possibility of assigning people directly to projects, some people might be overlooked, a possibility prevented by this query. Two other search options provide the list of different taxa or samples in hand for a given excavation site. A sixth query returns the number of samples available per excavation site, which helps assess whether we have enough specimens from a given region. Two other queries return all samples based on their country of origin and the different types of material (i.e., tissues) available per taxon, which has proven useful when assessing which material offers the best preservation conditions. Finally, the last query helps with reporting for grant-funding bodies by retrieving the number of articles published for the different projects. These queries are illustrated in Figure 7 (orange lines).
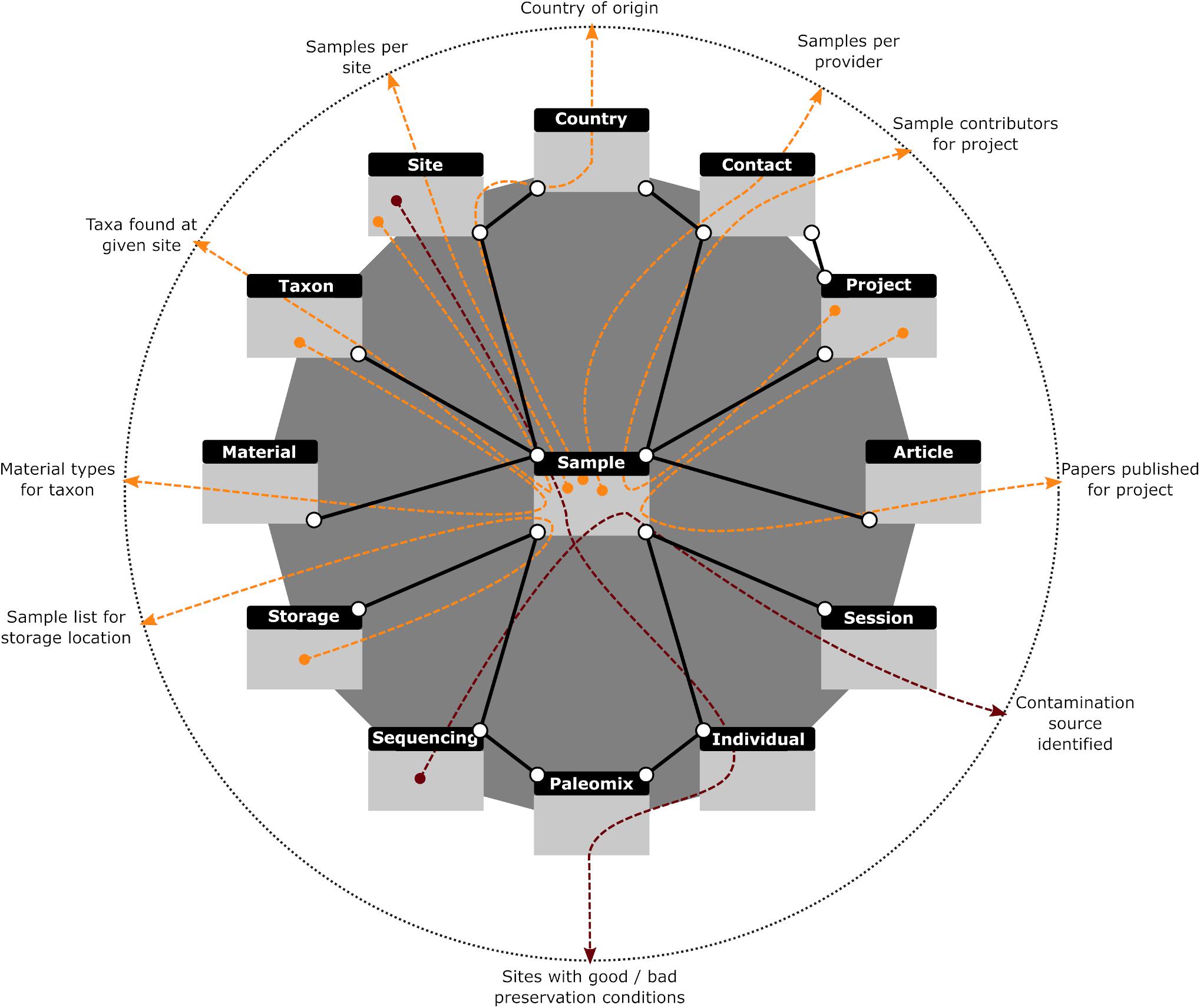
Figure 7. Graphical representation of database queries. Colored arrows (red and orange) demonstrate how different paths through the same connected data sets provide answers to various questions (described at dotted circle). All queries in orange are described in the main text and provided with CASCADE. The red queries illustrate other, less generic queries used to answer very specific questions and hence were not pre-built. Gray rectangles with black headers represent the tables dealing with the data described in each header. Solid black lines represent the relations existing between those data sets. A search query created with our interface first combines the requested data sources (e.g., tables or other previously created queries) based on given criteria, filters the combined data according to user specifications, then selects whatever data fields were requested by the user and returns the result in table format.
In addition to generating these types of targeted queries, the advanced search functionality built into CASCADE is equally useful for combining information from different tables into custom-built index views similar to those that already exist for the different menus. These “virtual tables” are first created as user-specific advanced searches and subsequently converted using the edit menu. Once converted, “virtual tables” cannot be used as source in advanced searches anymore, which makes their scope fixed, but are now available to everyone as queryable index views which, in contrast to their built-in counterparts, provide the same filtering possibilities available in advanced searches. We have provided three such queries in the “Tables” section of the basic search feature.
Another possible use of the query feature is the generation of informative labels for bags, bottles, and tubes used at any step of the experimental pipeline handled by CASCADE. To achieve this result, customized queries retrieve exactly the data required for any individual laboratory’s labeling needs and are exported as tab-delimited text files that can serve as input for most label-printing software and equipment available at the host laboratory. The data obtained can also be used for the production of one-dimensional and two-dimensional barcodes (e.g., QR codes). Due to their data density, the latter type is especially useful for labeling samples and tubes. This feature allows to store and retrieve all essential information about a specimen by simply scanning the attached barcode with barcode scanner or even a smartphone (in our laboratory we make use of “Barcode Scanner” for Android based on the ZXing open source barcode scanning library), without the need for a connection to CASCADE. Barcodes can also be used to directly access all sample information stored in the database. For this, users generate batches of IDs for their own personal use which can be assigned to any experimental data type and enable the recovery of the related data record. As these IDs can be created before any sample information is available, they can be printed in advance as barcodes and attached to individual tubes as experiments make progress. This is especially helpful in cases where no printers or computers are available wherever the different tubes are processed and/or stored (e.g., the ancient DNA clean rooms).
Discussion
The exponentially growing size of aDNA projects makes it increasingly difficult to keep all experimental metadata fully tractable for laboratory users and their collaborators. By integrating LIMS features tailored to the experimental procedures underlying aDNA analyses, CASCADE not only provides the first solution toward this objective, but also empowers experimental work and collaborative sharing through the possibility of automatic queries providing real-time information about ongoing progress and results. CASCADE can be accessed remotely from a web-browser by any user provided with a protected personal login account. It is made available for free, thereby helping to build the capacity of those smaller laboratories, which cannot afford the purchase of commercial LIMS solutions. It also contributes to the long-term preservation of important experimental information that may prove essential for the integration of available data to future projects, especially as the underlying methodology is constantly evolving.
In order to safeguard all experimental information handled by CASCADE, we have implemented two separate backup mechanisms. The first mechanism is configured during the installation and setup of the VM. It automatically generates full backups on a weekly basis in addition to daily incremental ones that allow a full reset of the database to its last functional state. The second mechanism can be manually triggered from inside CASCADE so as to force data download at critical stages. Like for the first mechanism, the output produced this way can be used to re-initialize the database. More importantly though, it can also be used to initialize a new copy of the database rather than just reset an existing one. In addition, it provides all data in a tab-delimited text format. This attribute guarantees that data stored in CASCADE will always be fully accessible in an easy-to-process format so that it can be transferred should newer and better lab management solutions become available.
Data Availability Statement
All datasets generated for this study are included in the article/supplementary material.
Author Contributions
LO conceived the project and coordinated work. DD and AFa designed the database schema and coordinated the data restructuring process. DD created the virtual servers, figures, and programmed the database. AFa developed the pool feature concept, managed the input of all laboratory members on the database design, and curated the data. AFr developed the tag and sample group feature concepts and performed break testing of the database. AS-O developed the waiting list feature concept. XM tested installation procedures. AFa, SS, LT-C, LC, SW, CD, AFr, and AS-O tested the database. DD, AFa, and LO wrote the manuscript, with input from all co-authors. All authors contributed to the article and approved the submitted version.
Funding
This work was supported by the Initiative d’Excellence Chaires d’attractivité, Université de Toulouse (OURASI) and the Villum Fonden miGENEPI research project. LO has received funding from the European Research Council (ERC) under the European Union’s Horizon 2020 research and innovation program (grant agreement No. 681605-PEGASUS). This project has received funding from the European Union’s Horizon 2020 research and innovation program under the Marie Skłodowska-Curie grant agreement No. 795916-NEO.
Conflict of Interest
The authors declare that the research was conducted in the absence of any commercial or financial relationships that could be construed as a potential conflict of interest.
Acknowledgments
We thank Dr. Naveed Khan and the staff of the AGES research laboratory (Archeology, Genomics, Evolution and Societies), especially Dr. Morgane Gibert, Dr. Catherine Thèves, and Dr. Tomasz Suchan, for their input on the database design, help with data restructuring, database testing and quality control. We also thank Corentin Deppe for his help in input data management.
Footnotes
- ^ https://www.virtualbox.org/wiki/VirtualBox
- ^ http://releases.ubuntu.com/18.04/
- ^ https://www.nginx.com/
- ^ https://www.mysql.com/
- ^ https://laravel.com/
- ^ https://vuejs.org/
- ^ https://getbootstrap.com/
- ^ https://fontawesome.com/
- ^ https://creativecommons.org/licenses/by/4.0/
- ^ https://bitbucket.org/product/
- ^ https://www.gimp.org/
- ^ https://www.inkscape.org
References
Boessenkool, S., Hanghøj, K., Nistelberger, H. M., Der Sarkissian, C., Gondek, A. T., Orlando, L., et al. (2017). Combining bleach and mild predigestion improves ancient DNA recovery from bones. Mol. Ecol. Res. 17, 742–751. doi: 10.1111/1755-0998.12623
Briggs, A. W., Stenzel, U., Johnson, P. L. F., Green, R. E., Kelso, J., Prüfer, K., et al. (2007). Patterns of damage in genomic DNA sequences from a Neandertal. PNAS 104, 14616–14621. doi: 10.1073/pnas.0704665104
Brunson, K., and Reich, D. (2019). The promise of paleogenomics beyond our own species. Trends Genet. 35, 319–329. doi: 10.1016/j.tig.2019.02.006
Carøe, C., Gopalakrishnan, S., Vinner, L., Mak, S. S. T., Sinding, M. H. S., and Samaniego, J. A. (2018). Single-tube library preparation for degraded DNA. Methods Ecol. Evol. 2, 410–419. doi: 10.1111/2041-210X.12871
Codd, E. F. (1970). A relational model of data for large shared data banks. Commun. ACM 13, 377–387. doi: 10.1145/362384.362685
Dabney, J., Knapp, M., Glocke, I., Gansauge, M. T., Weihmann, A., Nickel, B., et al. (2013). Complete mitochondrial genome sequence of a Middle Pleistocene cave bear reconstructed from ultrashort DNA fragments. Proc. Natl. Acad. Sci. U.S.A. 110, 15758–15763. doi: 10.1073/pnas.1314445110
Damgaard, P. B., Marchi, N., Rasmussen, S., Peyrot, M., Renaud, G., Korneliussen, T., et al. (2018). 137 ancient human genomes from across the Eurasian steppes. Nature 557, 369–374. doi: 10.1038/s41586-018-0094-2
Damgaard, P. B., Margaryan, A., Schroeder, H., Orlando, L., Willerslev, E., and Allentoft, M. (2015). Improving access to endogenous DNA in ancient bones and teeth. Sci. Rep. 5:11184. doi: 10.1038/srep11184
Fages, A., Hanghøj, K., Khan, N., Gaunitz, C., Seguin-Orlando, A., Leonardi, M., et al. (2019). Tracking five millennia of horse management with extensive ancient genome time series. Cell 177, 1419–1435. doi: 10.1016/j.cell.2019.03.049
Gansauge, M. T., Gerber, T., Glocke, I., Korlevic, P., Lippik, L., Nagel, S., et al. (2017). Single-stranded DNA library preparation from highly degraded DNA using T4 DNA ligase. Nucleic Acids Res. 45:e79. doi: 10.1093/nar/gkx033
Gansauge, M. T., and Meyer, M. (2013). Single-stranded DNA library preparation for the sequencing of ancient or damaged DNA. Nat. Protoc. 8, 737–748. doi: 10.1038/nprot.2013.038
Gansauge, M. T., and Meyer, M. (2014). Selective enrichment of damaged DNA molecules for ancient genome sequencing. Genome Res. 24, 1543–1549. doi: 10.1101/gr.174201.114
Glocke, I., and Meyer, M. (2017). Extending the spectrum of DNA sequences retrieved from ancient bones and teeth. Genome Res. 27, 1230–1237. doi: 10.1101/gr.219675.116
Goodwin, S., McPherson, J., and McCombie, W. (2016). Coming of age: ten years of next-generation sequencing technologies. Nat. Rev. Genet. 17, 333–351. doi: 10.1038/nrg.2016.49
Green, R. E., Krause, J., Briggs, A. W., Maricic, T., Stenzel, U., Kircher, M., et al. (2010). A Draft Sequence of the Neandertal Genome. Science 5979, 710–722. doi: 10.1126/science.1188021
Haak, W., Lazaridis, I., Patterson, N., Rohland, N., Mallick, S., Llamas, B., et al. (2015). Massive migration from the steppe was a source for Indo-European languages in Europe. Nature 522, 207–211. doi: 10.1038/nature14317
Harney, É, May, H., Shalem, D., Mallick, S., Rohland, N., Lazaridis, I., et al. (2018). Ancient DNA from Chalcolithic Israel reveals the role of population mixture in cultural transformation. Nat. Commun. 9:3336. doi: 10.1038/s41467-018-05649-9
Kistler, L., Maezumi, S. Y., Gregorio de Souza, J., Przelomska, N. A. S., Malaquias Costa, F., Smith, O., et al. (2018). Multiproxy evidence highlights a complex evolutionary legacy of maize in South America. Science 362, 1309–1313. doi: 10.1126/science.aav0207
Korlević, P., and Meyer, M. (2019). Pretreatment: removing DNA contamination from ancient bones and teeth using sodium hypochlorite and phosphate. Methods Mol. Biol. 1963, 15–19. doi: 10.1007/978-1-4939-9176-1_2
Lander, E. S., Linton, L. M., Birren, B., Nusbaum, C., Zody, M. C., Baldwin, J., et al. (2001). Initial sequencing and analysis of the human genome. Nature 409, 860–921. doi: 10.1038/35057062
Mann, A. E., Sabin, S., Ziesemer, K., Vågene, ÅJ., Schroeder, H., Ozga, A. T., et al. (2018). Differential preservation of endogenous human and microbial DNA in dental calculus and dentin. Sci. Rep. 8:9822. doi: 10.1038/s41598-018-28091-9
Marciniak, S., and Perry, G. H. (2017). Harnessing ancient genomes to study the history of human adaptation. Nat. Rev. Genet. 18, 659–674. doi: 10.1038/nrg.2017.65
Mathieson, I., Alpaslan-Roodenberg, S., Posth, C., Szécsényi-Nagy, A., Rohland, N., Mallick, S., et al. (2018). The genomic history of southeastern Europe. Nature 555, 197–203. doi: 10.1038/nature25778
Metzker, M. (2010). Sequencing technologies – the next generation. Nat. Rev. Genet. 11, 31–46. doi: 10.1038/nrg2626
Olalde, I., Brace, S., Allentoft, M. E., Armit, I., Kristiansen, K., Booth, T., et al. (2018). The Beaker phenomenon and the genomic transformation of northwest Europe. Nature 555, 190–196. doi: 10.1038/nature25738
Olalde, I., Mallick, S., Patterson, N., Rohland, N., Villalba-Mouco, V., Silva, M., et al. (2019). The genomic history of the Iberian Peninsula over the past 8000 years. Science 363, 1230–1234. doi: 10.1126/science.aav4040
Pedersen, M. W., Overballe-Petersen, S., Ermini, L., Sarkissian, C. D., Haile, J., Hellstrom, M., et al. (2015). Ancient and modern environmental DNA. Philos. Trans. R. Soc. Lond. B Biol. Sci. 370:20130383. doi: 10.1098/rstb.2013.0383
Pinhasi, R., Fernandes, D., Sirak, K., Novak, M., Conell, S., Alpaslan-Roodenberg, S., et al. (2015). Optimal Ancient DNA yields from the inner ear part of the human petrous bone. PLoS One 10:e0129102. doi: 10.1371/journal.pone.0129102
Rasmussen, M., Li, Y., Lindgreen, S., Pedersen, J. S., Albrechtsen, A., Moltke, I., et al. (2010). Ancient human genome sequence of an extinct Palaeo-Eskimo. Nature 463, 757–762. doi: 10.1038/nature08835
Regalado, A. (2019). More Than 26 Million People Have Taken an at-Home Ancestry Test. MIT Technology Review. Available online at: https://www.technologyreview.com/2019/02/11/103446/ (accessed December 2, 2019).
Reich, D., Green, R. E., Kircher, M., Krause, J., Patterson, N., Durand, E. Y., et al. (2010). Genetic history of an archaic hominin group from Denisova Cave in Siberia. Nature 468, 1053–1060. doi: 10.1038/nature09710
Rohland, N., Glocke, I., Aximu-Petri, A., and Meyer, M. (2018). Extraction of highly degraded DNA from ancient bones, teeth and sediments for high-throughput sequencing. Nat. protoc. 13, 2447–2461. doi: 10.1038/s41596-018-0050-5
Rohland, N., Harney, E., Mallick, S., Nordenfelt, S., and Reich, D. (2015). Partial uracil-DNA-glycosylase treatment for screening of ancient DNA. Philos. Trans. R. Soc. Lond. B Biol. Sci. 370:20130624. doi: 10.1098/rstb.2013.0624
Schubert, M., Ermini, L., Der Sarkissian, C., Jónsson, H., Ginolhac, A., Schaefer, R., et al. (2014). Characterization of ancient and modern genomes by SNP detection and phylogenomic and metagenomic analysis using PALEOMIX. Nat. protoc. 9:1056. doi: 10.1038/nprot.2014.063
Spyrou, M. A., Bos, K. I., Herbig, A., and Krause, J. (2019). Ancient pathogen genomics as an emerging tool for infectious disease research. Nat. Rev. Genet. 20, 323–340. doi: 10.1038/s41576-019-0119-1
Keywords: ancient DNA, laboratory management, database, LIMS, traceability, conservation, collaborative sharing
Citation: Dolle D, Fages A, Mata X, Schiavinato S, Tonasso-Calvière L, Chauvey L, Wagner S, Der Sarkissian C, Fromentier A, Seguin-Orlando A and Orlando L (2020) CASCADE: A Custom-Made Archiving System for the Conservation of Ancient DNA Experimental Data. Front. Ecol. Evol. 8:185. doi: 10.3389/fevo.2020.00185
Received: 19 December 2019; Accepted: 25 May 2020;
Published: 23 June 2020.
Edited by:
Nic Rawlence, University of Otago, New ZealandReviewed by:
Axel Barlow, Nottingham Trent University, United KingdomAndré Elias Rodrigues Soares, Uppsala University, Sweden
Christian N. K. Anderson, Brigham Young University, United States
Copyright © 2020 Dolle, Fages, Mata, Schiavinato, Tonasso-Calvière, Chauvey, Wagner, Der Sarkissian, Fromentier, Seguin-Orlando and Orlando. This is an open-access article distributed under the terms of the Creative Commons Attribution License (CC BY). The use, distribution or reproduction in other forums is permitted, provided the original author(s) and the copyright owner(s) are credited and that the original publication in this journal is cited, in accordance with accepted academic practice. No use, distribution or reproduction is permitted which does not comply with these terms.
*Correspondence: Ludovic Orlando, ludovic.orlando@univ-tlse3.fr; orlando.ludovic@gmail.com
†These authors have contributed equally to this work