- 1Globe Institute, University of Copenhagen, Copenhagen, Denmark
- 2Institute of Oceanography, Vietnam Academy of Science and Technology, Nha Trang, Vietnam
- 3AquaBiota Solutions, Stockholm, Sweden
Coral reefs worldwide are rapidly declining due to increasing anthropogenic stressors and environmental changes, with large-scale mortalities of coral reefs observed in many locations across the globe. It has become clear that the microbiome of corals is important in understanding the causes of coral infections, although its exact role is yet to be fully understood. Here, we characterize the bacteria and fungi associated with the non-lesional and lesional (identified by discoloration and tissue loss) tissues of coral species from Vietnam. Metabarcoding of the bacterial 16S rRNA gene and the fungal ITS rRNA gene region were performed. We sampled across two Porites species with potentially multiple causes of stresses, yet the bacterial compositions of lesional regions were consistently different from non-lesional areas of the same coral. These differences were driven by a considerable and significant increase in OTU richness within the lesional region compared to the non-lesional region. While no single OTU was consistently associated with lesional tissue, indicator analysis revealed that nine OTUs were significantly more persistent in the lesional regions that could represent useful bioindicators of stress. Meanwhile, there were no indicator OTUs in the non-lesional region. Further investigations are needed to determine whether changing bacterial communities play a mechanistic role in inducing lesioning, or are opportunistically colonizing stressed corals.
Introduction
Around the world, corals are declining due to many different forms of stress. Reefs in Southeast Asia are no different. They are experiencing rapid deterioration, and it is apparent that the Indo-Pacific reefs are heading toward the same decline as that of the Caribbean reefs (Weil et al., 2006). Vietnam is regarded as one of the 27 most vulnerable places to reef loss in the world (Burke et al., 2011). With the increase of tourism and industrialization along the Vietnam coast, the coral cover is expected to decline as disease prevalence increases at faster rates than previously recorded (Lamb et al., 2014). These reefs are affected by anthropogenic stressors, such as pollution, runoff, and shoreline development (Latypov, 2006; Ponti et al., 2016). In addition, changes in environmental stressors, such as increased UV and increased temperatures, induced by climate change, have made corals more susceptible to stress (Carey, 2000; Webster et al., 2016). These stressors combined are causing significant coral reef degradation around the world (Mera and Bourne, 2018), and reports of coral disease have been increasing since their first observation in the 1970s (Antonius, 1973; Garrett and Ducklow, 1975). Furthermore, other biological stressors, such as predation and algae are also affecting coral reefs (Shaver et al., 2017; Brown et al., 2019). There are many types of stressors and corals are often forced to resist multiple stressors concurrently (Brown et al., 2019). Therefore, it is often difficult to determine whether a single factor or a combination of factors is causing the immune response in the coral.
Some stressors have the potential to affect the composition of the coral’s microbiome. A coral colony lives in symbiosis with dinoflagellates, bacteria, archaea, and fungi, known as the coral’s microbiome (Rädecker et al., 2015). A healthy microbiome can ward off diseases and help increase stress tolerance, as well as prevent drastic responses such as bleaching of the coral host (Rosado et al., 2019). A healthy microbial community is likely needed for the coral’s survival, but the importance of a healthy microbiome is often overlooked when discussing ecological resilience (McDevitt-Irwin et al., 2017). An altered microbiome composition has been observed on corals stressed by disease (Mera and Bourne, 2018) and when algae encroach onto corals (Brown et al., 2019). The extent of this perturbation can also vary depending on the species of coral, where the microbiomes of some coral species seem to exhibit limited changes, others can change profoundly in response to stress (Ziegler et al., 2019). Studies suggest that certain bacterial communities are associated with the health and resilience of corals, while other bacteria could serve as indicators of stress (Bourne et al., 2016; Glasl et al., 2017). Interactions between corals and algae can often result in a coloration change (Palmer et al., 2008; Hoeksema et al., 2019); this reaction, especially if color is pink, is considered a sign of injury or immune response in the coral.
Here, we present a study investigating the alteration of the microbiome in response to stressors in corals off the coast of Vietnam. We sampled Porites spp. corals both in regions showing signs of stress responses, such as algae interaction and possible disease infection, as well as reportedly less-affected regions. We characterized the bacterial and fungal composition using DNA metabarcoding. This study aims to quantify the relative effects of site variation, island effects, and lesion status in regulating the bacterial and fungal communities of Vietnamese Porites spp.
Materials and Methods
Ethics Statement
Corals for this study were collected following Vietnamese laws and following the guidelines and permits for sampling and export issued by the Institute of Oceanography (IO) of Nha Trang, Vietnam and the Marine Park Authorities (permit no. 205 2014). Samples were exported to the Natural History Museum of Denmark (University of Copenhagen) for processing.
Study Species: Porites spp.
This study focuses on genus Porites (specifically P. lobata and P. lutea) of Vietnam due to their important ecological role (Barshis et al., 2010). Porites are hard corals (Scleractinia) commonly found in a wide range of habitats within the distribution range of coral reefs. Some Porites spp. may be sensitive to environmental changes but massive species, such as P. lutea are tolerant of bleaching, disease outbreaks, and erosion (Obura, 2001; Barshis et al., 2010; Huang, 2012).
Study Sites
Samples were collected in May and June 2014 from a total of six sites from two regions: one near Nha Trang (central Vietnam) and the other Cu Lao Cau (south-central Vietnam) in Vietnam (Figure 1). In 2002, a Marine Protected Area (MPA) was established in the Nha Trang Bay region to help protect the marine habitats, such as mangroves and coral reefs (Dung, 2009; Nguyen, 2010; Ngoc, 2019). The MPA covers nearly 13,000 hectares and the coral reefs therein are thought to contain the highest biodiversity in Vietnam (Nguyen, 2010). Four sampling sites were located around Mun Island within the core zone of the MPA (Rainbow Reef, Madonna Beach, Seahorse Wave, and Debbie’s Beach) (Figure 1), where some recreational diving is allowed but monitored. All sites on Mun Island are considered to have high coral coverage and little to no algae, and the coral reefs in the area were observed to be less stressed than in nearby non-protected areas (Dung, 2009).
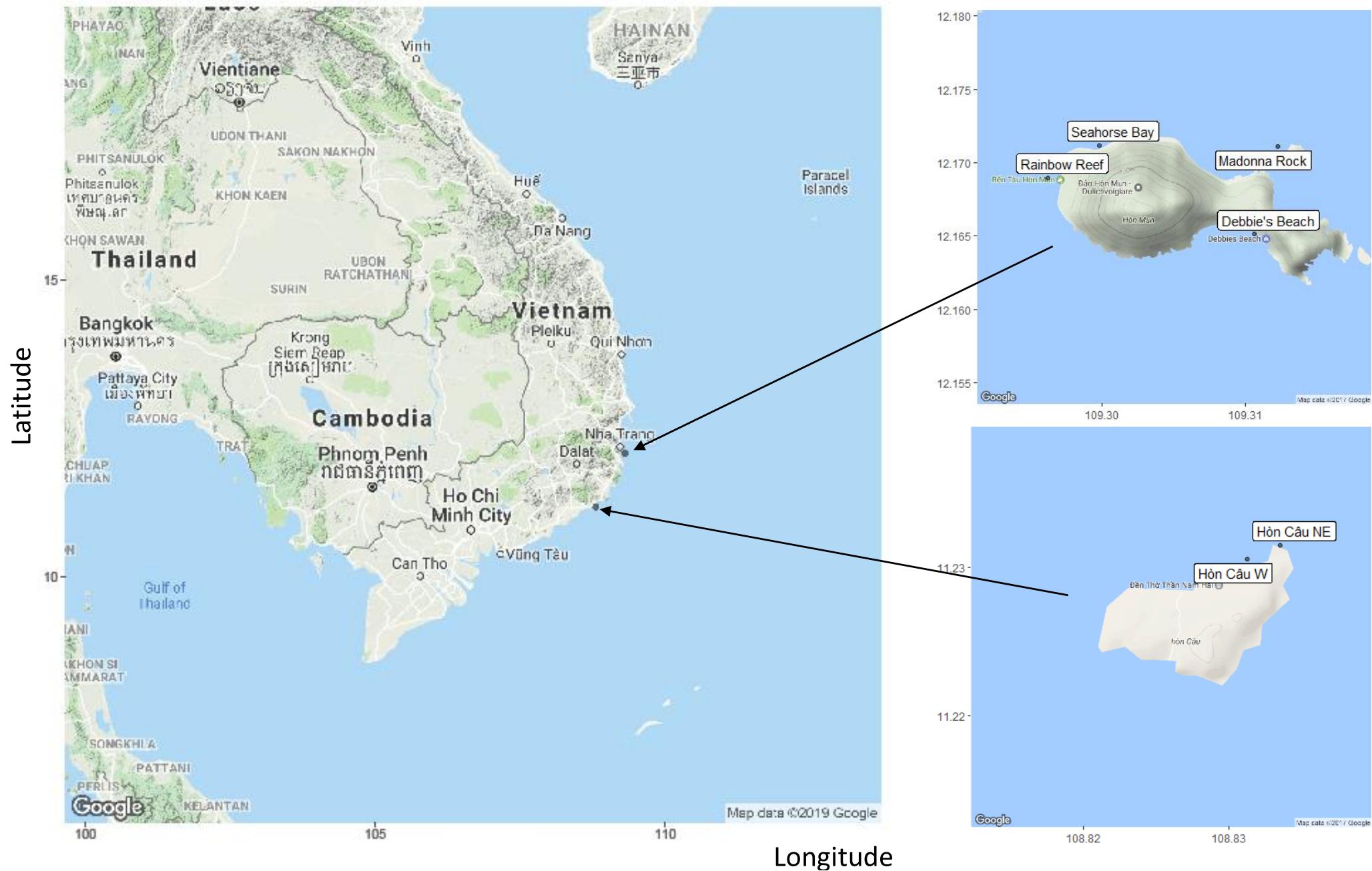
Figure 1. Map of the six sites in Vietnam with axis describing the longitude and latitude of sites. Mun Island (upper right corner) contains four sites: Seahorse Bay, Madonna Rock, Rainbow Reef, and Debbie’s Beach. Cau Island (lower right corner) is located in south-central Vietnam and has two sites—Cu Lao Cau northeast (NE) and Cu Lao Cau west (W). (2017) (Google Maps, 2017).
Cu Lao Cau is a small island located along the south-central Vietnam coast (Figure 1). The island has been under the protection of the Hon Cau MPA since 2011 (3 years prior to sampling) (Walton et al., 2015), although large fishing fleets are active near the island. The shallow fringing reefs (0–5 m) on the northeast side of the island has a high coverage of a diverse coral community and a wide variety of fish species. At 5 m depth, the corals were extensively overgrown by algae, and a large number of crown-of-thorns starfish (Achantaster plancii) were observed. Corals were sampled at two sites (Cu Lao Cau W, Cu Lao Cau NE) within this region. The sampling site on the west side of the island has high turbidity and corals were observed by local divers to have a higher presence of diseases than the northeast sampling site.
Sample Collection and Processing
Coral tissue samples were collected using SCUBA. Samples were collected from coral colonies that were identified as Porites in the field and were photographed using a Canon PowerShot D30 camera prior to sampling. The corals were initially identified based on the pictures following Veron (2000). Sampling efforts were set up in areas where corals were monitored for disease and presence of lesions. For this study, the lesional areas sampled were characterized by a pink coloration (sometimes also accompanied by a white coloration) with distinct, undulating edges, and the presence of either bare coral skeleton or algae (Coral Disease and Health Consortium, 2019) (Figure 2A). Non-lesional samples were collected within a phenotypically healthy region, at a minimum of 5 cm from the edge of lesioned regions (Figure 2B). A small tissue sample (∼2 mm3) was collected from lesional and non-lesional regions of each coral using razor blades. The sample was transferred to pre-numbered and re-sealable plastic bags. Samples were then centrifuged and the supernatant was then removed to prevent seawater contamination. Water samples were also taken at five sites. Details of sample collection and processing can be found in the Supplementary Material.
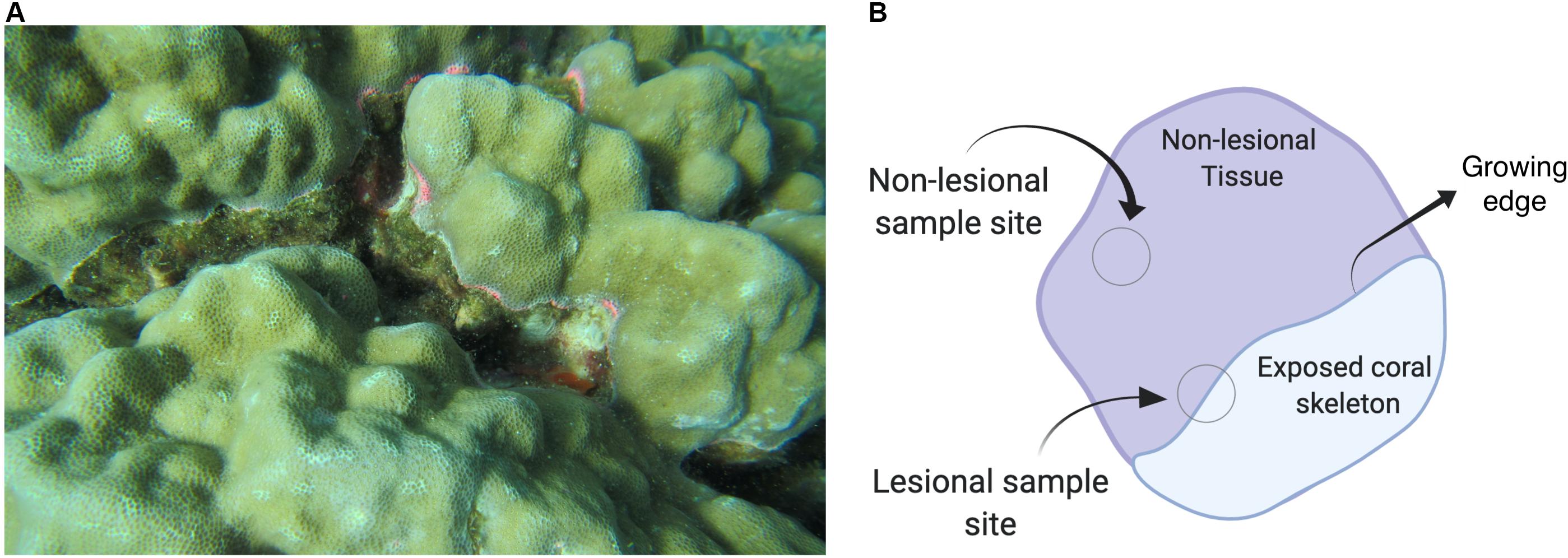
Figure 2. (A) Porites spp. This image shows a lesioned Porites coral. The diseased coral has a blotch of skeleton visible (covered by algae) where the growing edge is receding and shows signs of an immune response. Photos were taken by Kristín Rós Kjartansdóttir and Thomas Arn Hansen. (B) Image depicting the location of where samples were taken from. Image created with Biorender.com.
After each dive, samples were transferred to pre-labeled vials on board and were transported in either liquid nitrogen or on dry-ice to −80°C freezers in the laboratory at the Institute of Oceanography in Nha Trang, Vietnam. Samples were then transported to Denmark on dry-ice and stored at −80°C until further processing.
DNA Extraction
DNA extractions from coral tissue were performed using QIAGEN DNeasy Blood and Tissue kit (QIAGEN, Germany) following the manufacturer’s instructions, with a minor modification during the DNA elution stage, where samples were re-eluted in 200 μL 70°C 1X TE buffer (pH 8.0, Sigma). Five extraction negatives were included in the extraction process. All extractions were quantified using Qubit (Version 1.27) with High Sensitivity DNA assay (Invitrogen, Carlsbad, United States) and diluted to 1 ng μL–1. These dilutions, including the extraction negative, were used to identify fungi and bacteria in the coral, as well as for coral identification.
Coral Identification
All samples were morphologically identified during collection and checked with Sanger sequencing using the following genetic markers: mitochondrial putative control region (D-Loop) and cytochrome c oxidase I gene (COI). The D-Loop primers (Vollmer and Palumbi, 2002) were used to identify if samples were Porites spp. (forward (CR_F): 5′-GCTTAGACAGGTTGGTTGATTGCCC-3′ and reverse (CO3_R): 5′-CTCCCAAATACATAATTTGAACTA-3′). Samples were amplified with D-Loop primers using a PCR master mix as follows: 2.5 μl GeneAmp® 10X PCR Buffer I (Thermo Fisher Scientific) with 15 nM MgCl2, 0.2 μL dNTPs (25 mM, Invitrogen), 0.2 μL AmpliTaq DNA polymerase (5U μL–1), 1.5 μL forward primer (10 μM), 1.5 μL reverse primer (10 μM), 1 μL Bovine Serum Albumin (BSA) (20 mg mL–1) (New England Biolabs Inc.) (Pace et al., 1995), and 17.1 μL AccuGENE molecular grade water, then 1ng of Coral Tissue DNA. The DNA was amplified under conditions: 95°C – 5 min; (95°C – 30 s; 52°C – 30 s; 72°C – 1 min) × 35 cycles; 72°C – 7 min; hold at 4°C. All PCR products (5 μL) were visualized on 1–2% agarose gels stained with GelRed Nucleic Acid Gel Stain (Biotium) and separated by electrophoresis to confirm amplification success.
The samples that failed to amplify with the D-loop primers, were amplified with a general COI marker suitable for genus-level identification of corals (forward (ZCOI_F): 5′-TCAACTAATCATAAAGATATTGGTACG-3′ and reverse (ZCOI_R): 5′-TAAACCTCTGGATGCCCAAA-3′) (Forsman et al., 2009). AmpliTaq master mix (Thermo Fisher Scientific) was also used for this amplification, with the following thermocycler conditions: 95°C 5 min; (95°C – 30 s; 51°C – 45 s; 72°C – 90 s) × 35 cycles; 72°C – 5 min; hold at 4°C). Amplified PCR products (CO1: ∼ 638 bp, D-loop: ∼ 933bp) were purified and Sanger sequenced at Macrogen Inc. (Germany).
Sequences were analyzed using MEGA 7.0 (Kumar et al., 2016) and underwent BLAST analysis against the NCBI BLASTN database, and matches of > 98% were used as genus-level identifications (Ko et al., 2013).
Coral Associated Bacteria Metabarcoding
PCR amplification of bacterial 16S ribosomal RNA gene region (16S rRNA gene) was performed using primers (forward (341F): 5′-CCTAYGGGRBGCASCAG-3′ and reverse (806R): 5′-GGACTACNNGGGTATCTAAT-3′). These primers flank the V3–V4 region of the bacterial 16S rRNA gene region and resulted in a PCR product of approximately 400 base pairs (bp). Additionally, internal tags ranging between six and eight nucleotides were added to the 5′ terminal of primers allowing for the multiplexing of samples (Hansen et al., 2012). In total, 80 different forward and reverse tags were used in accordance with Schnell et al. (2015), with no replication of tag sequences in libraries, and unique forward-reverse tag combinations used across entire MiSeq run. All samples were run with PCR master mix consisting of 2.5 μL 10X PCR GOLD buffer (Thermo Fisher Scientific), 2.5 μL GOLD MgCl2 (25 mM, Thermo Fisher Scientific), 0.2 μL dNTPs (25 mM, Invitrogen), 0.2 μL AmpliTaq GOLD polymerase (5U μL–1, Thermo Fisher Scientific), 0.2 μL BSA (20 mg mL–1) (New England Biolabs Inc.), and 12.6 μL AccuGENE molecular grade water. Tagged bacterial primers were then individually added (1.5 μL of forward and reverse primers (10 μM) per sample) to each reaction along with 3ng DNA. Also, a PCR negative was systematically added approximately every 16 samples (13 PCR negatives in total). The total reaction volume was 25 μL and all samples were run in Applied Biosystems 2720 Thermal Cycler (Applied Biosystems) with a setting of 95°C – 5 min; (95°C – 15 s; 55°C – 30 s; 72°C – 40 s) × 36 cycles; 72C – 4 min; hold at 4°C. Samples that amplified poorly were run in replicates with their pair (samples from a colony with both non-lesional and lesional samples). All PCR products were visualized on agarose gels and pooled. A total of six pools were used to construct six libraries using Illumina TruSeq DNA PCR-Free Library Preparation (Illumina, CA, United States). After library construction, a bead purification step was performed to remove primer dimers, which consisted of one volume of library product to 1.5 volume of Agencourt AMPure XP beads (Beckman Coulter, Germany). The libraries were run on an Agilent Technologies 2100 Bioanalyzer (Agilent, CA, United States) using the Agilent High Sensitivity DNA kit to determine the length of products and concentration for the pooling of libraries for sequencing. Libraries (including extraction, PCR, and library negatives) were subsequently sequenced on an Illumina MiSeq platform with 250 bp paired-end at the Danish National High-Throughput Sequencing Centre, GLOBE Institute, Copenhagen, Denmark.
Bioinformatics
A total of 26,529,179 single reads were produced across two MiSeq runs (for bacterial libraries of the corals, water, and negatives). All reads for bacterial data can be found under accession no: SRP149401 in Sequence Read Archive (SRA), where samples are already demultiplexed into samples. Initially, 16S rRNA gene region libraries were merged using VSEARCH (v 2.1.2) when there was a 100% match only (Rognes et al., 2016). After merging forward and reverse reads, the total amount of merged reads was 12,759,157 with a quality score (Q-score) of 39. Using a custom script, libraries were subsequently demultiplexed into individual samples using the unique tag combination attached to the forward and reverse primers. Only reads with exact matches of both tag sequences were kept, resulting in 6,028,673 merged reads. VSEARCH (v2.1.2) was used to denoise merged reads (a maximum error rate of 0.2%, approximately equivalent to <1 bp error per read) and to filter reads below 400 bp, while also calling Cutadapt (v 1.11) (Martin, 2011) to remove primers and internal tag sequences. After quality filtering, 5,812,821 reads remained. Reads were subsequently dereplicated and singletons removed, with 66,863 unique sequences identified in the dataset. Using the UPARSE-OTU algorithm in USEARCH (Edgar, 2013), OTUs were clustered at 97% similarity. Chimeras, accounting for 6% of reads, were removed during the OTU clustering step. Clustering resulted in a total of 3,786 operational taxonomic units (OTUs) and 4,103 chimeras. An OTU table was subsequently created from unique dereplicated reads and OTUs within USEARCH (v9.0.2132), before being converted to a BIOM format table. Taxonomy was assigned to each OTU using LCAClassifier v.2.0.4. within MEGAN v6.18 (Huson et al., 2016) using the Silva reference database v.128 (Quast et al., 2012). OTUs assigned to Eukaryotes were removed from the dataset, resulting in an OTU table containing 658,617 reads spread over 3573 OTUs. A substantial loss of reads occurred as a consequence of OTU1 (5,142,405 reads) being identified as the mitochondrial 12S rRNA gene from the Porites spp. which was removed from downstream analyses. The average amplicon length of OTUs was 412.23 bp ± 10.61. A total of 182 coral samples (including replicates), 18 water samples, 18 negatives from protocols ran with coral samples (five extraction negatives and 13 PCR negatives), and three negatives from protocol ran with water samples (two extraction negatives and one PCR negative) remained. Given the relatively low number of reads per sample, theoretical maximal diversity was also calculated for each sample (Chao1) using QIIME (VEGAN v2.5-6), while data were then analyzed in two separate ways (described below) to limit these effects. The results of water samples and their negatives were reported in Supplementary Material and excluded in the following datasets:
(1) Initially reads from each coral replicate were pooled into a single sample (referred to as the summed dataset). three OTUs were present in half of the negative controls and were therefore likely contaminants that were removed. Consequently, 593,527 reads were spanning 3391 OTUs in the 74 corals samples (35 pairs and four unpaired samples). The results of this approach are presented in the Supplementary Material.
(2) Alternatively, a more stringent approach was applied where replicates with the highest number of reads were selected as a representative for each sample, and all OTUs present in any negative were removed. Samples were rarified to an even 500 reads per sample. Consequently, there were 37,000 reads spread over the 74 coral samples (35 pairs and 4 unpaired samples), spanning 1,802 OTUs. This dataset will be referred to as the stringent dataset and will be the dataset that is presented in the results section of the main text.
Fungal metabarcoding and bioinformatics were also performed (Supplementary Material) with fungal reads undergoing BLAST against the UNITE database (v7.2) (Kõljalg et al., 2005). However, due to reads mostly being derived from the host, the results are not included in the main analyses, and only briefly discussed in this paper. Fungal reads were also deposited in the SRA and can be found the same accession as the bacteria (no: SRP149401).
Statistical Analyses
All statistical analyses were performed using the computing environment R (v 3.6.2), which were repeated for both the summed dataset and the stringent dataset (as outlined in the bioinformatic analyses). Initially, the OTU data underwent a Hellinger transformation before statistical analyses to downweigh the many zero values. A Bray-Curtis similarity matrix was constructed from the community data and non-metric multidimensional scaling (nMDS) was performed, which was visualized using ggplots (v 2.2.1) (Wickham, 2016). The effects of lesion status, site, and island effect on coral microbial community composition were initially tested using the envfit function. Additionally, a PERMANOVA was performed to correlate lesion status, site, and island effect against community variation simultaneously, while pair ID served as the strata. Betadisper was run on the similarity matrix and tested for variance in sites, island effect, and lesion status. The Bray-Curtis similarity matrix construction, nMDS, envfit tests, betadisper, and PERMANOVA were all performed using the Vegan package (v 2.4-6) (Oksanen et al., 2013).
Given the substantial differentiation between the communities of the lesional and non-lesional regions, samples were partitioned by their lesional status for independent community analyses. Multivariate generalized linear modeling (MGLM) analyzed lesional and non-lesional community variation separately against site and island effects using the negative binomial distribution, utilizing the MVABUND package (v 3.13.1) (Wang et al., 2012).
For assessing differences in OTU richness (as opposed to variation in the community composition), lesion status, and sites nested within island effect served as fixed effects in a linear mixed-effects model, with OTU richness (log-transformed) serving as the response variable and pair ID serving as the random effect (lme4 package, v1.1-15) (Bates et al., 2014). The significance of various factors on OTU richness was assessed by performing likelihood ratio tests on linear mixed effects models, using the drop1 function (from the stats package, v3.4.3). Meanwhile, differences in top phyla’s OTU richness and relative abundance were assessed using paired t-tests (stats package). Finally, indicator species analysis was performed to detect OTUs associated with the lesional and non-lesional regions, using the signassoc function of the Indicspecies package (v1.7.6) (De Caceres et al., 2016). P-values underwent Sidak’s correction for multiple comparisons to avoid false positives (Šidák, 1967).
Results
Coral Identification
All samples that underwent Sanger sequencing were assigned to P. lobata and P. lutea, however, it was not possible to distinguish between the two species. Due to this and their morphological similarities, we refer to samples as the genus (Porites spp.) throughout the rest of the manuscript.
Coral Associated Bacteria Composition
Non-lesional samples appeared phenotypically healthy with no sign of stress, whereas lesional samples were collected on the basis of showing an immune response, characterized by pink coloration and either exposed skeleton or algae on one side. After Hellinger transformation of the stringent dataset, and the calculation of a Bray-Curtis dissimilarity matrix, the community composition of the coral-associated bacteria was visualized with non-metric multidimensional scaling (nMDS) (Figure 3A, similar results showed in summed dataset Supplementary Figure S6A). The ordination demonstrates the clustering of the bacterial communities from lesional and non-lesional coral regions separately. The lesional and non-lesional communities showed no significant difference in community similarity, with the lesional samples sharing an average of 0.15 (15%) similarity and the non-lesional just 0.12 (12%) (t = 218476, p-value = 0.6381) (Figure 3B).
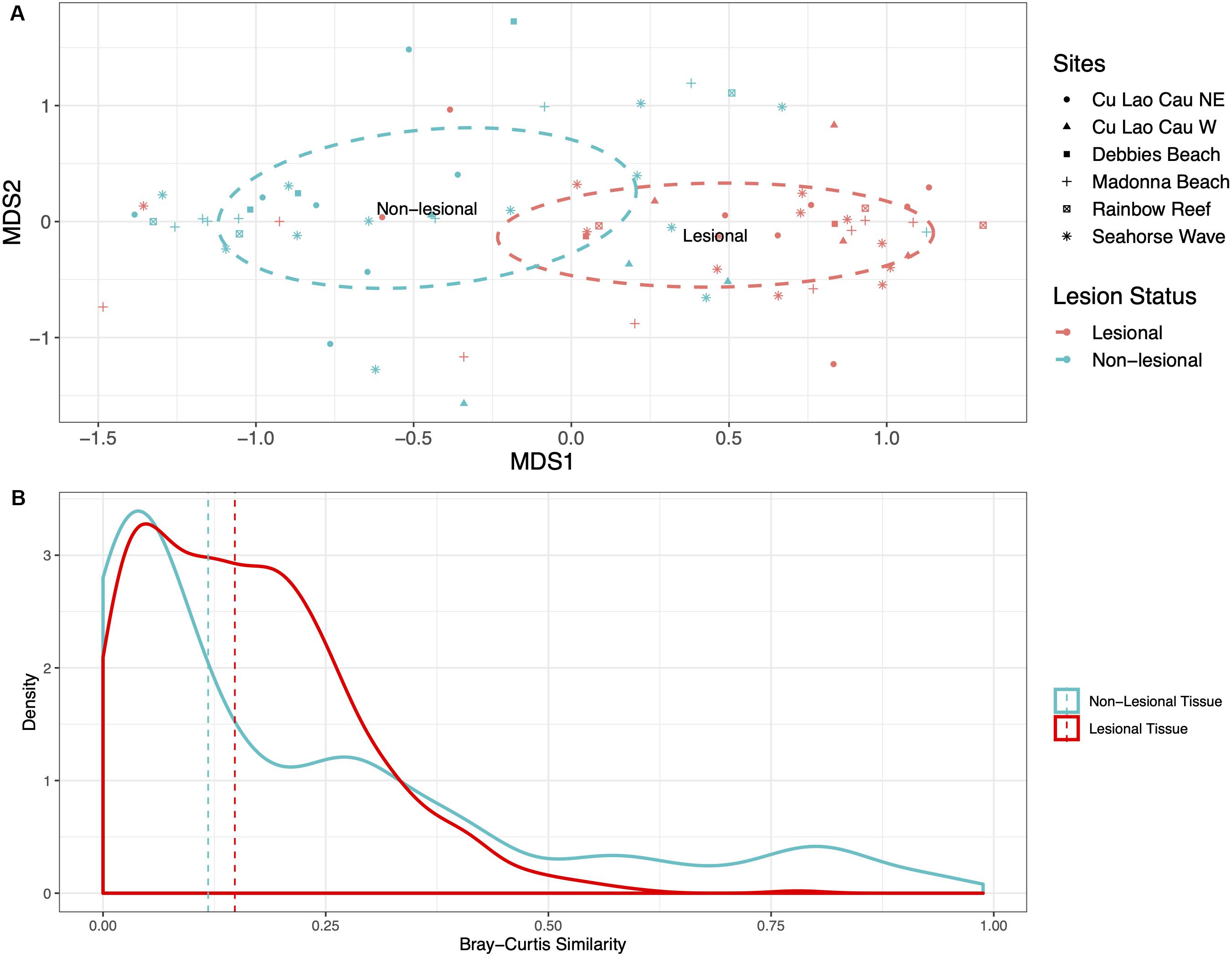
Figure 3. (A) nMDS plot shows data from rarefied Porites data clustering based on lesion status. The dashed circles are representative of the groups’ 95% confidence interval. (B) The Bray-Curtis similarity of the non-lesional and lesional tissue. Lesional tissue contained more similarity in composition with a mean of 0.15 similarity. Whereas, the non-lesional tissue was much more variable, with a mean of 0.12 similarity (the mean is represented by the dashed lines).
From the nMDS, envfit analyses were performed to test whether lesion status, sites, and island effects correlate with the bacterial composition. Sites were nested within the island, but each had no significant effect. Only lesion status was shown to be a significant driver of the bacterial composition (R2 = 0.080, p-value > 0.001, permutation 999). Differences in dispersion was tested with betadisper, finding no difference in dispersion associated with sites (F-value = 0. 818, p-value = 0.541), island effect (F-value = 0.337, p-value = 0.563), and lesion status (F-value = 0.085, p-value = 0.772). PERMANOVA analysis found that only lesion status correlated with the bacterial community variation (Table 1). MGLM was performed on the partitioned lesional and non-lesional bacterial communities separately (as the data was no longer paired) to determine whether there were any effects of site or island that were masked by the large variation associated with lesion status. Site and island effects did not significantly correlate with the bacterial communities of the non-lesional samples, whereas site significantly correlated with variation of the lesional communities (df = 5, deviance = 5086, p-value = 0.028, permutation = 500).

Table 1. PERMANOVA summary statistics for factors affecting the bacterial composition in non-lesional and lesional corals.
Bacterial OTU Richness of Lesional and Non-lesional Regions of Corals
Due to the rarefaction of samples to equal sampling depth, an alpha diversity index (Chao1) was calculated to estimate the theoretical maximum number of OTUs in samples. Theoretical maximums showed that lesional samples had considerably higher OTU richness than their non-lesional counterpart (Figure 4; t = 5.4455, p-value < 0.001). Within the stringent (rarefied) data, lesional regions had a mean of 86.5 OTUs while the non-lesional regions had a mean of 49.2 bacterial OTUs. Theoretical maximum richnesses (Chao1) found the mean of lesional samples to be nearly three-fold higher than that of the non-lesional samples (mean of lesional = 292.6 OTUs, mean of non-lesional = 108.1 OTUs). Log transformed bacterial OTU richness underwent linear mixed effect modeling, with lesion status, and sites nested within the island effects serving as the fixed effect within the model, and coral pair as the random effect, revealing only lesion status significantly correlated with OTU richness (LTR = 17.39, p-value < 0.001). Paired and unpaired samples were used, but uncertainty in random effect was estimated with paired samples. In addition, a Student’s t-test found that OTU richness was significantly different between lesion status (t = 4.124, df = 72, p-value < 0.001), and another Student’s t-test of Shannon diversity measure also found a significant difference between bacterial richness between lesional and non-lesional status (t = 4.037, df = 72, p-value < 0.001). The bacterial community of lesional regions was dominated by Proteobacteria, which represented 51.3% of the total bacterial composition, and accounted for 57.5% of reads in non-lesional regions. The Bacteroidetes and Cyanobacteria were the other two highly abundant bacterial phyla represented, accounting for 7.7% and 8% of reads in the lesional samples, and 29.2% and 24.9% in the non-lesional.
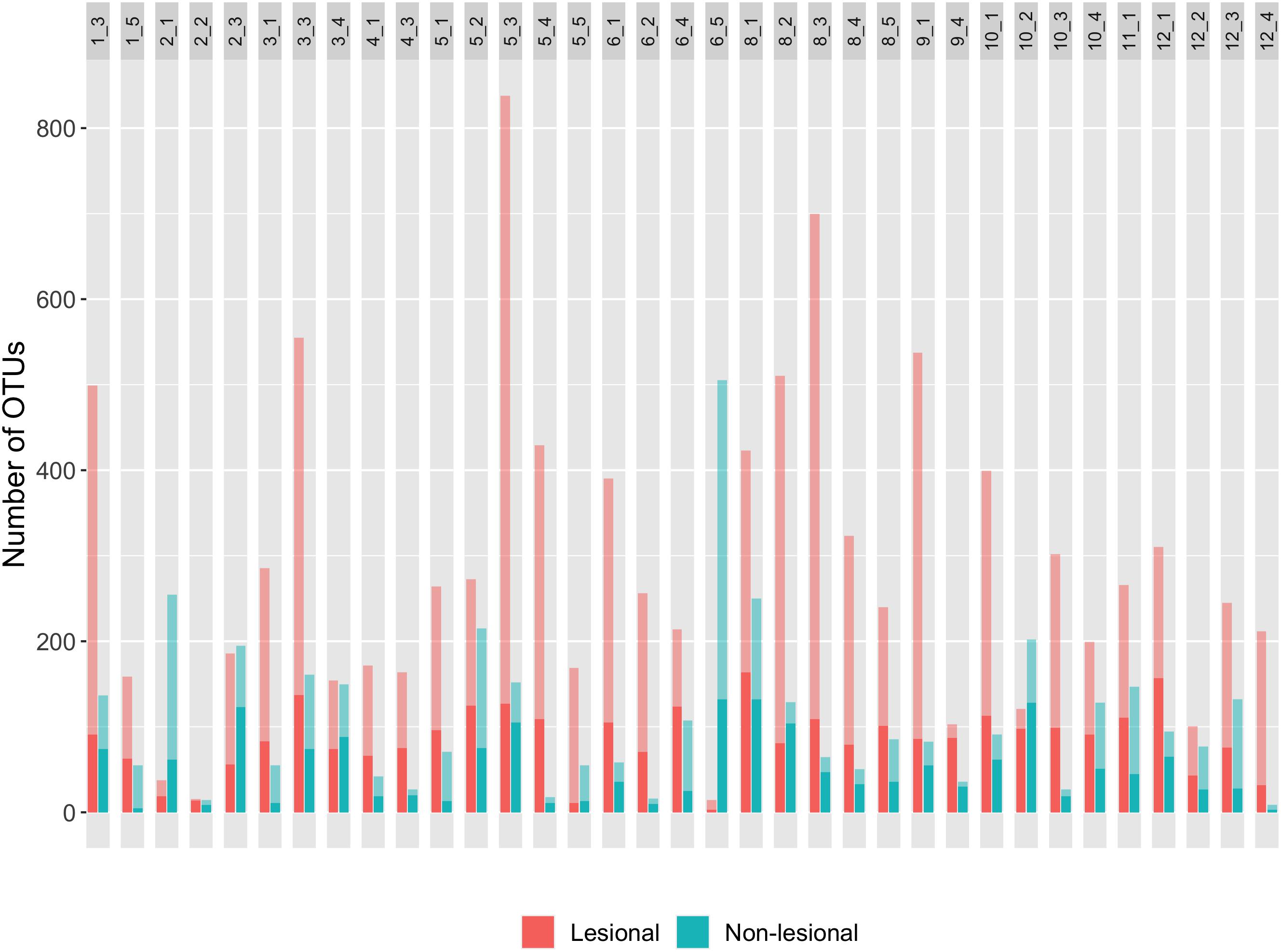
Figure 4. This plot contains the OTU richness of pairs (pair ID listed at the top of the respective bars) from rarefied data (Supplementary Figure S3 for non-rarefied OTU richness data). The number (X_X) in the gray box represents the sample pair, where the first X represents the transect number and the second X is the coral number. Thus, 1_3 is a coral pair containing a sample of the non-lesional and lesional tissues from the third coral on the first transect. The color of the bar represents the lesion status of the sample. The translucent coloration represents the theoretical maximum (Choa1 estimate) whereas, solid coloration is representative of OTU richness of rarefied data. Overall, lesional samples have more OTUs than their adjacent non-lesional samples.
The average number of OTUs in each of the Proteobacteria, Cyanobacteria, and Bacteroidetes was significantly increased in the lesional communities compared to the non-lesional (Figure 5). Lesional samples contained on average 42.7 Proteobacteria OTUs, whereas non-lesional communities contained 19.8 Proteobacteria OTUs (t = 5.225, df = 72, p-value < 0.001). Similarly, the amount of OTUs corresponding to Cyanobacteria was an average of 15.3 OTUs in lesional samples, and 7.8 OTUs in non-lesional (t = 4.720, df = 72, p-value < 0.001). Bacteroidetes also increased in lesional samples with an average of 16.7 OTUs, whereas non-lesional samples had an average of 9.9 OTUs (t = 2.726, df = 72, p-value = 0.008).
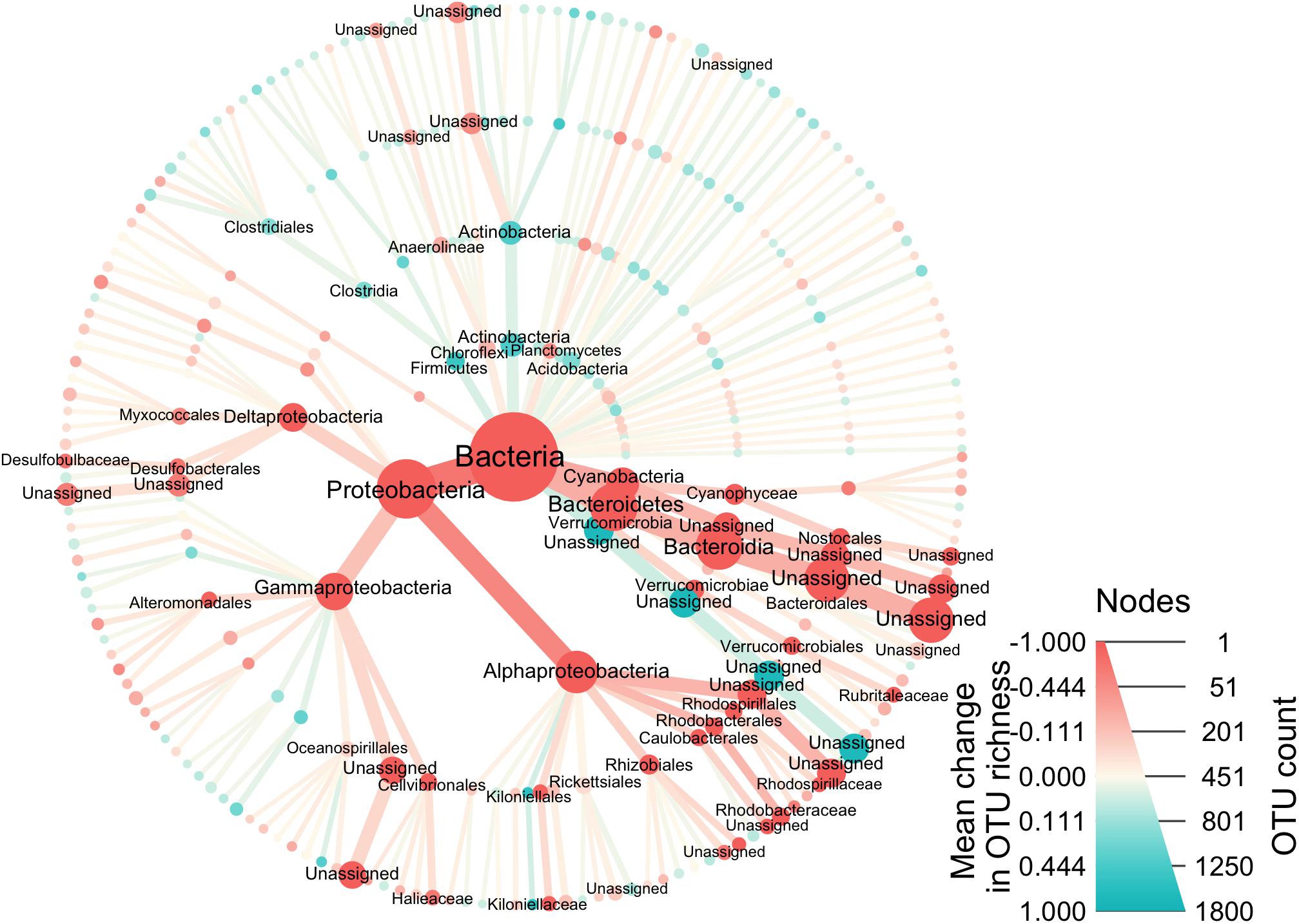
Figure 5. This phylogenetic heat tree shows the bacteria composition of the samples. The color of nodes and edges represents the mean change in OTU richness at each taxonomic group, with red indicating greater richness in lesional samples and blue in the non-lesional, while node size represents OTU richness of each taxonomic group across the dataset.
Indicator species analysis identified nine indicator OTUs for lesional samples, but none for the non-lesional samples (Figure 6). Of these lesional indicators, seven were assigned to the phylum Proteobacteria, six of which belong to the class Alphaproteobacteria. Two OTUs were not assigned to taxonomy beyond the class level, both of which were absent in the non-lesional, but found in 18.1% of lesional samples. One OTU was assigned to the order Caulobacterales, which was in 2.7% of non-lesional communities and 29.7% of the lesional. Two OTUs were classified to the family Rhodobacteraceae that had large differences in persistence associated with lesion status, but these were also present in non-lesional samples (Ruegeria spp. OTU: non-lesional – 48.6% and lesional – 83.8%; Rhodobacteraceae OTU: non-lesional – 16.2%, lesional – 54.1%). An Alphaproteobacteria OTU, Defluviicoccus spp., was absent in the non-lesional communities and in 18.9% of the lesional. There were two OTUs assigned to phylum Cyanobacteria. One of these OTUs was present in 2.7% of the non-lesional and 20.7% of the lesional. The other OTU was assigned to Limothrix spp. and it was present in nearly a quarter of lesional samples (24.3%) and very few of the non-lesional (2.7%). A Neptuniibacter spp. OTU was present in 5.4% of the non-lesional and 29.7% of the lesional.
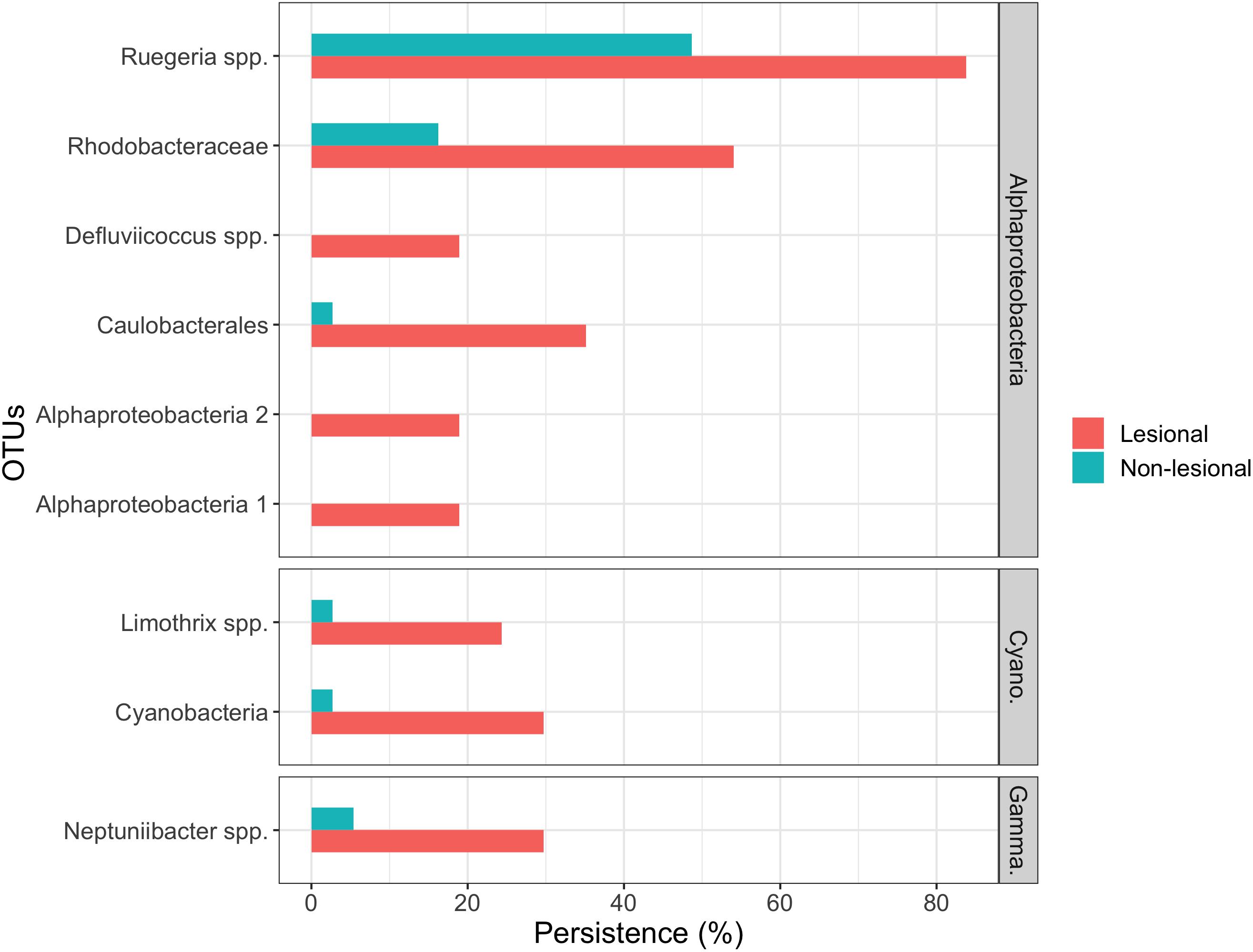
Figure 6. A summary of OTUs that are significant indicators for lesional corals. OTUs are grouped by Alphaproteobacteria, Cyanobacteria (Cyano.), and Gammaproteobacteria (Gamma.). OTUs were required to be in at least seven samples as well as have a Sidak corrected p-value of greater than 0.05 in the species indicator analysis.
Discussion
Here we show that the bacterial community composition of Porites spp. differs substantially when under stress (e.g., coral disease and algae interactions), driven by a near doubling of OTU richness within lesional regions of corals compared to the non-lesional areas (Figure 4; a pattern mimicked in the summed dataset, Supplementary Figure S3). The bacterial communities of the lesional tissues significantly differed from the non-lesional. However, despite the increased bacterial richness, variation in the bacterial community composition did not significantly differ (averaging 15% similarity in the lesional and just 12% in the non-lesional).
In this study, lesional samples were characterized as an area on the coral that had pink coloration, which has been previously been associated with an immune response by the coral (Palmer et al., 2008) and exposed skeleton or algae growth on the one side. Even though we were unable to determine the exact stressor or combination of stressors causing lesioning in this study, a change in the microbiome in the presence of lesions was evident across the corals we sampled. We found a shift from a less diverse bacterial community (non-lesional region) to a more diverse one (lesional region). Importantly, the lesional bacterial community was enriched by newly colonizing bacteria from a wide range of phylogenetic backgrounds (Figure 5). The summed dataset (consisting of the merged triplicate data) also supports the observed increase in OTU richness and the shift in the bacterial community. Lesions can be induced by a range of abiotic and biotic stressors (Peters, 2015) and these results found here and in other studies (Egan and Gardiner, 2016; Pratte et al., 2018) suggest that lesioned areas are associated with a loss of regulation of the microbiome. Algae interactions in Porites spp. often cause coloration at the interaction edge and tissue loss (Thinesh et al., 2019) as well as an increase in microbial diversity (Brown et al., 2019), which was also evident in this study. This reaction can be of concern since Porites spp. do not show any recovery of affected tissue (Thinesh et al., 2019). A shift in the microbiome has been suggested to be the result of compromised health, rather than driven by a single causative agent (Sweet and Bulling, 2017). Therefore, it remains unknown whether the colonization of many new bacterial species are simply opportunistically colonizing already compromised corals (passengers), or are inducing lesions (drivers).
The lack of regulation of the microbiome by a host is referred to as dysbiosis and has become an area of particularly intense research in recent years (Egan and Gardiner, 2016). Traditionally, researchers have analyzed for a predictable dysbiotic state (i.e., a perturbed microbiome) under stress, but more recently the Anna Karenina Principle (AKP) was suggested as an alternative model for predicting coral microbiome composition under stress (Zaneveld et al., 2017). The AKP suggests that many environmental stresses induce stochastic changes on the composition of the microbiome rather than deterministic, and therefore “each dysbiotic microbiome is dysbiotic in its own way” (Zaneveld et al., 2017). Furthermore, the coral communities affected by increased temperatures and algal growth seem to fit the AKP model (Casey et al., 2015). Here, differences are only seen in the composition of the bacterial communities, but not in β-diversity (geographical variation), which could suggest a deterministic change. The composition of lesional samples significantly varied between sites, which could suggest some sort of environmental variation between sites. However, no single bacterium was consistently associated with the lesioned state in our dataset. To make more robust hypotheses into the effects of changing coral microbiome associated with stress, future studies should also sample corals without visible signs of stress or disease, and compare these to the lesional and non-lesional regions of the corals.
The bacterial communities of Vietnamese corals demonstrate a community composition similar to those described in Huggett and Apprill (2019), who created a database of coral-associated bacteria from many studies. They found Proteobacteria, Cyanobacteria, Bacteriodetes, Firmicutes, and Actinobacteria are consistently the most abundant and species-rich phyla (Huggett and Apprill, 2019). Similarly, in this study, high levels of Proteobacteria, Cyanobacteria, Bacteroidetes, and Actinobacteria were associated with the Vietnamese Porites spp. (Supplementary Figure S7). Interestingly, OTUs dominating the water samples were not among the persistent OTUs seen in the coral samples, and are therefore unlikely to have driven the differences in bacterial communities observed between the lesional and non-lesional regions (Supplementary Table S5). Furthermore, Endozoicomonas are found in significantly higher abundance in the non-lesional samples (non-lesional – 39.9%; lesional – 10.8%), but were equally low in OTU richness despite the lesion status (non-lesional: average 1.5 OTUs; lesional – average 1.1 OTUs), suggesting that Endozoicomonas might be commensal bacteria that are lost/reduced in abundance when corals become lesioned. This drop in Endozoicomonas abundance has been seen in another study focusing on the bacterial communities of coral lesions associated with disease (Meyer et al., 2014). It has also reported in corals during other stress events, such as climate anomalies and algae interactions (McDevitt-Irwin et al., 2017; Brown et al., 2019), and is overall associated with corals’ resistance to pathogens (Bourne et al., 2013; Neave et al., 2016; Peixoto et al., 2017). Thus, stressors may cause a breakdown in the coral’s defense mechanism found in the microbiome, allowing for pathogens and opportunistic bacteria to invade.
While no single OTU could be associated with lesional status, nine potential bioindicators of lesional tissues were identified that were significantly more persistent in the lesional tissue. Meanwhile, seven of the nine indicator OTUs of lesional tissue were assigned to the phylum Proteobacteria (Figure 6). Two of the indicator OTUs belonged to the family Rhodobacteraceae. Rhodobacteraceae are thought to be opportunistic bacteria that bloom when corals are stressed (Welsh et al., 2015). Therefore, the presence of Rhodobacteraceae OTUs could be a potential indicator of coral health. The lack of any consistent OTU associated with lesional tissue could further suggest that there is no single stressor or no single pathogenic agent. However, without a defined cause of lesioning, it is not very likely to find a single microbe associated with lesions. It should also be noted that given the errors associated with assigning taxonomy to bacterial data produced via metabarcoding (Somervuo et al., 2017), caution is urged when using the assigned taxonomy from OTU data in understanding a taxonomic group’s role. However, as none of the indicator OTUs were assigned to the species level (with only two of nine assigned to the genus level), little can be inferred into the putative functioning of the identified indicator OTUs.
Both fungal and bacterial primers were dominated by reads derived from the corals. For the fungal data, this could be due to low numbers of coral-associated fungi, or similarity between the fungal and coral ITS regions (Supplementary Figure S1). The limited number of reads assigned to fungi do, however, suggest that Porites spp. maintain a similar fungal composition between the lesion and non-lesional regions, with potentially a modest increase in the number of OTUs in the lesional tissue. In addition, the primers showed Philaster apodigitiformis, a known secondary opportunistic ciliate, was found in nearly a third of the lesional samples and in low abundance within the non-lesional samples (Supplementary Table S2). This mimics previously observed trends, where P. apodigitiformis was found to increase in corals affected by white syndromes and brown-band diseases (Weil et al., 2006; Randall et al., 2015). Other species within the Philaster genus, have been reported as possibly playing a role in coral diseases (Sweet and Séré, 2016). However, sampling with more specific fungal primers or an untargeted metagenomic approach is required to better characterize the fungal communities associated with corals. Quantitative approaches such as quantitative PCR could also be applied to determine whether the fungi associated with corals are in biologically relevant abundances within the coral microbiome.
In this study, we explored the composition of fungi and bacteria of Vietnamese Porites corals. This study is part of a growing number of studies reporting that stressors are causing a significant increase in the bacterial community within the coral microbiome. Furthermore, stressors such as algae interactions and coral diseases are likely associated with an imbalance in the microbiome. We show that the composition and richness of the bacterial community of corals are dependent on sampling lesioned or non-lesioned regions of the coral. We found that lesioning could be indicative of a form of dysbiosis and a loss of regulation of the coral’s associated bacterial community, but it remains unknown whether the perturbed bacterial community is driving the lesions or simply opportunistically colonizing corals afterward. Our study supports the statement of McDevitt-Irwin et al. (2017), that microbiomes experience an increase in diversity, regardless of the stressor, and that these changes in the microbiome may not be predictable but predominantly stochastic. A more thorough understanding of the role that the coral microbiome plays in healthy coral functioning is required, but also an improved understanding of the changes associated with specific abiotic and biotic stressors are required to aid efforts to conserve coral reefs that are increasingly under threat.
Data Availability Statement
The datasets generated for this study can be found in the NCBI, accession no: SRP149401, https://www.ncbi.nlm.nih.gov/sra/SRP149401.
Author Contributions
All authors contributed to substantial contributions to the conception or design of the work, or the acquisition, analysis or interpretation of data for the work, drafting the work or revising it critically for important intellectual content, provide approval for publication of the content, agreed to be accountable for all aspects of the work in ensuring that questions related to the accuracy or integrity of any part of the work are appropriately investigated and resolved.
Conflict of Interest
MH was employed by AquaBiota Solutions midway through the course of the study.
The remaining authors declare that the research was conducted in the absence of any commercial or financial relationships that could be construed as a potential conflict of interest.
Acknowledgments
We would like to thank the staff at the Institute of Oceanography in Vietnam for their support and cooperation in the field. Thanks to Jeremy Stein and crew at Rainbow Divers in Vietnam for supporting this study and for guiding us to the reefs around the Nha Trang area. Thanks to divers in Cu Lao Cham for assistance with dive logistics in Cu Lao Cau. LN-N and HD-N would like to send their acknowledgment to Projects of the Vietnam Academy of Science and Technology (VAST) for supporting to research activities of senior researchers in the period 2018-2020.
Supplementary Material
The Supplementary Material for this article can be found online at: https://www.frontiersin.org/articles/10.3389/fevo.2020.00151/full#supplementary-material
References
Antonius, A. (1973). “New observations on coral destruction in reefs,” in Proceedings of the Tenth Meeting of the Association of Island Marine Laboratories of the Caribbean, (Mayaguez: University of Puerto Rico).
Barshis, D. J., Stillman, J. H., Gates, R. D., Toonen, R. J., Smith, L. W., and Birkeland, C. (2010). Protein expression and genetic structure of the coral Porites lobata in an environmentally extreme Samoan back reef: does host genotype limit phenotypic plasticity. Mol. Ecol. 19, 1705–1720. doi: 10.1111/j.1365-294X.2010.04574.x
Bates, D., Maechler, M., Bolker, B., and Walker, S. (2014). lme4: Linear Mixed-Effects Models Using Eigen and S4. R package version 1, 1–23.
Bourne, D. G., Dennis, P. G., Uthicke, S., Soo, R. M., Tyson, G. W., and Webster, N. (2013). Coral reef invertebrate microbiomes correlate with the presence of photosymbionts. ISME J. 7, 1452–1458. doi: 10.1038/ismej.2012.172
Bourne, D. G., Morrow, K. M., and Webster, N. S. (2016). Insights into the coral microbiome: underpinning the health and resilience of reef ecosystems. Annu. Rev. Microbiol. 70, 317–340. doi: 10.1146/annurev-micro-102215-095440
Brown, A., Lipp, E. K., and Osenberg, C. W. (2019). Algae dictate multiple stressor effects on coral microbiomes. Coral Reefs 38, 229–240.
Burke, L., Reytar, K., Spalding, M., and Perry, A. (2011). Reefs at Risk Revisited. Available online at: http://www.wri.org/reefs (accessed November 20, 2016).
Carey, C. (2000). Infectious disease and worldwide declines of amphibian populations, with comments on emerging diseases in coral reef organisms and in humans. Environ. Health Perspect. 108, (Suppl. 1), 143–150. doi: 10.1289/ehp.00108s1143
Casey, J. M., Connolly, S. R., and Ainsworth, T. D. (2015). Coral transplantation triggers shift in microbiome and promotion of coral disease associated potential pathogens. Sci. Rep. 5:11903. doi: 10.1038/srep11903
Coral Disease and Health Consortium, (2019). Lesion Terminology and Visualization [Online]. Available at: https://cdhc.noaa.gov/diagnostics/lesion_terminology.aspx (accessed April 22, 2020).
De Caceres, M., Jansen, F., and De Caceres, M. M. (2016). Package ‘Indicspecies’. Available online at: https://vegmod.github.io/software/indicspecies (accessed May 3, 2017).
Dung, L. D. (2009). Nha Trang Bay marine protected area, Vietnam: initial trends in coral structure and some preliminary linkages between these trends and human activities (2002–2005). Aquat. Ecosyst. Health Manag. 12, 249–257. doi: 10.1080/14634980903146767
Edgar, R. C. (2013). UPARSE: highly accurate OTU sequences from microbial amplicon reads. Nat. Methods 10, 996–998. doi: 10.1038/nmeth.2604
Egan, S., and Gardiner, M. (2016). Microbial dysbiosis: rethinking disease in marine ecosystems. Front. Microbiol. 7:991. doi: 10.3389/fmicb.2016.00991
Forsman, Z. H., Barshis, D. J., Hunter, C. L., and Toonen, R. J. (2009). Shape-shifting corals: molecular markers show morphology is evolutionarily plastic in Porites. BMC Evol. Biol. 9:45. doi: 10.1186/1471-2148-9-45
Glasl, B., Webster, N. S., and Bourne, D. G. (2017). Microbial indicators as a diagnostic tool for assessing water quality and climate stress in coral reef ecosystems. Mar. Biol. 164:91.
Google Maps (2017). Google Maps [Online]. Available: https://www.google.com.au/maps/@-23.3394728,150.5201386,12z?hl=en (accessed October 18, 2017).
Hansen, C. H. F., Krych, L., Nielsen, D. S., Vogensen, F. K., Hansen, L. H., Sørensen, S. J., et al. (2012). Early life treatment with vancomycin propagates Akkermansia muciniphila and reduces diabetes incidence in the NOD mouse. Diabetologia 55, 2285–2294. doi: 10.1007/s00125-012-2564-7
Hoeksema, B. W., Van Der Schoot, R. J., Wels, D., Scott, C. M., and Ten Hove, H. A. (2019). Filamentous turf algae on tube worms intensify damage in massive Porites corals. Ecology 100:e02668. doi: 10.1002/ecy.2668
Huang, D. (2012). Threatened reef corals of the world. PLoS One. 7:e34459. doi: 10.1371/journal.pone.0034459
Huggett, M. J., and Apprill, A. (2019). Coral microbiome database: integration of sequences reveals high diversity and relatedness of coral-associated microbes. Environ. Microbiol. Rep. 11, 372–385. doi: 10.1111/1758-2229.12686
Huson, D. H., Beier, S., Flade, I., Górska, A., El-Hadidi, M., Mitra, S., et al. (2016). MEGAN community edition-interactive exploration and analysis of large-scale microbiome sequencing data. PLoS Comput. Biol. 12:e1004957. doi: 10.1371/journal.pcbi.1004957
Ko, H. L., Wang, Y. T., Chiu, T. S., Lee, M. A., Leu, M. Y., Chang, K. Z., et al. (2013). Evaluating the accuracy of morphological identification of larval fishes by applying DNA barcoding. PLoS One. 8:e53451. doi: 10.1371/journal.pone.0053451
Kõljalg, U., Larsson, K. H., Abarenkov, K., Nilsson, R. H., Alexander, I. J., Eberhardt, U., et al. (2005). UNITE: a database providing web-based methods for the molecular identification of ectomycorrhizal fungi. New Phytol. 166, 1063–1068. doi: 10.1111/j.1469-8137.2005.01376.x
Kumar, S., Stecher, G., and Tamura, K. (2016). MEGA7: molecular evolutionary genetics analysis version 7.0 for bigger datasets. Mol. Biol. Evol. 33, 1870–1874. doi: 10.1093/molbev/msw054
Lamb, J. B., True, J. D., Piromvaragorn, S., and Willis, B. L. (2014). Scuba diving damage and intensity of tourist activities increases coral disease prevalence. Biol. Conserv. 178, 88–96.
Latypov, Y. Y. (2006). Changes in the composition and structure of coral communities of Mju and Moon Islands, Nha Trang Bay, South China Sea. Russ. J. Mar. Biol. 32, 269–275.
Martin, M. (2011). Cutadapt removes adapter sequences from high-throughput sequencing reads. EMBnet J. 17, 10–12.
McDevitt-Irwin, J. M., Baum, J. K., Garren, M., and Vega Thurber, R. L. (2017). Responses of coral-associated bacterial communities to local and global stressors. Front. Mar. Sci. 4:262. doi: 10.3389/fmars.2017.00262
Mera, H., and Bourne, D. G. (2018). Disentangling causation: complex roles of coral-associated microorganisms in disease. Environ. Microbiol. 20, 431–449. doi: 10.1111/1462-2920.13958
Meyer, J. L., Paul, V. J., and Teplitski, M. (2014). Community shifts in the surface microbiomes of the coral Porites astreoides with unusual lesions. PLoS One 9:e100316. doi: 10.1371/journal.pone.0100316
Neave, M. J., Apprill, A., Ferrier-Pagès, C., and Voolstra, C. R. (2016). Diversity and function of prevalent symbiotic marine bacteria in the genus Endozoicomonas. Appl. Microbiol. Biotechnol. 100, 8315–8324. doi: 10.1007/s00253-016-7777-0
Ngoc, Q. T. K. (2019). Assessing the value of coral reefs in the face of climate change: the evidence from Nha Trang Bay, Vietnam. Ecosyst. Serv. 35, 99–108.
Nguyen, T. M. H. (2010). Fisheries Benefits from Protecting Corals: the Case of the Nha Trang Bay Marine Protected area and Trao Reef Marine Reserve. Tromsø: Universitetet i Tromsø.
Obura, D. O. (2001). Can differential bleaching and mortality among coral species offer useful indicators for assessment and management of reefs under stress. Bull. Mar. Sci. 69, 421–442.
Oksanen, J., Blanchet, F. G., Kindt, R., Legendre, P., Minchin, P. R., O’hara, R., et al. (2013). Package ‘vegan’. Community Ecology package, version 2(9).
Pace, C. N., Vajdos, F., Fee, L., Grimsley, G., and Gray, T. (1995). How to measure and predict the molar absorption coefficient of a protein. Protein Sci. 4, 2411–2423. doi: 10.1002/pro.5560041120
Palmer, C. V., Mydlarz, L. D., and Willis, B. L. (2008). Evidence of an inflammatory-like response in non-normally pigmented tissues of two scleractinian corals. Proc. R. Soc. B Biol. Sci. 275, 2687–2693. doi: 10.1098/rspb.2008.0335
Peixoto, R. S., Rosado, P. M., Leite, D. C. D. A., Rosado, A. S., and Bourne, D. G. (2017). Beneficial microorganisms for corals (BMC): proposed mechanisms for coral health and resilience. Front. Microbiol. 8:341. doi: 10.3389/fmicb.2017.00341
Peters, E. C. (2015). “Diseases of coral reef organisms,” in Coral Reefs in the Anthropocene, ed. C. Birkeland, (Dordrecht: Springer), 147–178.
Ponti, M., Fratangeli, F., Dondi, N., Reinach, M. S., Serra, C., and Sweet, M. J. (2016). Baseline reef health surveys at Bangka Island (North Sulawesi, Indonesia) reveal new threats. PeerJ 4:e2614. doi: 10.7717/peerj.2614
Pratte, Z. A., Longo, G. O., Burns, A. S., Hay, M. E., and Stewart, F. J. (2018). Contact with turf algae alters the coral microbiome: contact versus systemic impacts. Coral Reefs 37, 1–13.
Quast, C., Pruesse, E., Yilmaz, P., Gerken, J., Schweer, T., Yarza, P., et al. (2012). The SILVA ribosomal RNA gene database project: improved data processing and web-based tools. Nucleic Acids Res. 41, D590–D596. doi: 10.1093/nar/gks1219
Rädecker, N., Pogoreutz, C., Voolstra, C. R., Wiedenmann, J., and Wild, C. (2015). Nitrogen cycling in corals: the key to understanding holobiont functioning? Trends Microbiol. 23, 490–497. doi: 10.1016/j.tim.2015.03.008
Randall, C. J., Jordán-Garza, A. G., and van Woesik, R. (2015). Ciliates associated with signs of disease on two Caribbean corals. Coral Reefs 34, 243–247.
Rognes, T., Flouri, T., Nichols, B., Quince, C., and Mahé, F. (2016). VSEARCH: a versatile open source tool for metagenomics. PeerJ 4:e2584. doi: 10.7717/peerj.2584
Rosado, P. M., Leite, D. C., Duarte, G. A., Chaloub, R. M., Jospin, G., da Rocha, U. N., et al. (2019). Marine probiotics: increasing coral resistance to bleaching through microbiome manipulation. ISME J. 13, 921–936. doi: 10.1038/s41396-018-0323-6
Schnell, I. B., Bohmann, K., and Gilbert, M. T. P. (2015). Tag jumps illuminated–reducing sequence-to-sample misidentifications in metabarcoding studies. Mol. Ecol. Resour. 15, 1289–1303. doi: 10.1111/1755-0998.12402
Shaver, E. C., Shantz, A. A., McMinds, R., Burkepile, D. E., Vega Thurber, R. L., and Silliman, B. R. (2017). Effects of predation and nutrient enrichment on the success and microbiome of a foundational coral. Ecology 98, 830–839. doi: 10.1002/ecy.1709
Šidák, Z. (1967). Rectangular confidence regions for the means of multivariate normal distributions. J. Am. Stat. Assoc. 62, 626–633.
Somervuo, P., Douglas, W. Y., Xu, C. C., Ji, Y., Hultman, J., Wirta, H., et al. (2017). Quantifying uncertainty of taxonomic placement in DNA barcoding and metabarcoding. Methods Ecol. Evol. 8, 398–407.
Sweet, M. J., and Bulling, M. T. (2017). On the importance of the microbiome and pathobiome in coral health and disease. Front. Mar. Sci. 4:9. doi: 10.3389/fmars.2017.00009
Sweet, M. J., and Séré, M. G. (2016). Ciliate communities consistently associated with coral diseases. J. Sea Res. 113, 119–131.
Thinesh, T., Jose, P. A., Ramasamy, P., Meenatchi, R., Selvan, K. M., and Selvin, J. (2019). Differential coral response to algae contact: Porites tissue loss, praise for Halimeda interaction at southeast coast of India. Environ. Sci. Pollut. Res. 26, 17845–17852. doi: 10.1007/s11356-019-05024-1
Vollmer, S. V., and Palumbi, S. R. (2002). Hybridization and the evolution of reef coral diversity. Science 296, 2023–2025. doi: 10.1126/science.1069524
Walton, A., Hang, T. M., Hien, B. T. T., Duong, K. T., Hien, N. B., Bac, P. V., et al. (2015). Vietnam Marine Protected Area Management Effectiveness Evaluation. Gland: IUCN.
Wang, Y., Naumann, U., Wright, S. T., and Warton, D. I. (2012). mvabund–an R package for model-based analysis of multivariate abundance data. Methods Ecol. Evol. 3, 471–474.
Webster, N. S., Negri, A. P., Botte, E. S., Laffy, P. W., Flores, F., Noonan, S., et al. (2016). Host-associated coral reef microbes respond to the cumulative pressures of ocean warming and ocean acidification. Sci. Rep. 6:19324. doi: 10.1038/srep19324
Weil, E., Smith, G., and Gil-Agudelo, D. L. (2006). Status and progress in coral reef disease research. Dis. Aquat. Organ. 69, 1–7. doi: 10.3354/dao069001
Welsh, R. M., Rosales, S. M., Zaneveld, J. R., Payet, J. P., McMinds, R., Hubbs, S. L., et al. (2015). Alien vs. Predator: pathogens open niche space for opportunists, unless controlled by predators. PeerJ 3:e1537v1. doi: 10.7717/peerj.3315
Zaneveld, J. R., McMinds, R., and Thurber, R. V. (2017). Stress and stability: applying the Anna Karenina principle to animal microbiomes. Nat. Microbiol. 2:17121. doi: 10.1038/nmicrobiol.2017.121
Keywords: microbiome, molecular ecology, metabarcoding, bacteria, fungi
Citation: Rasmussen L, Barnes C, Mak SST, Kjartansdóttir KR, Hansen TA, Doan-Nhu H, Nguyen-Ngoc L, Guldberg Frøslev T, Hellström M and Hansen AJ (2020) Increased Bacterial Richness Associated With Lesions Within the Porites spp. of Vietnam. Front. Ecol. Evol. 8:151. doi: 10.3389/fevo.2020.00151
Received: 25 November 2019; Accepted: 04 May 2020;
Published: 27 May 2020.
Edited by:
Ulisse Cardini, Stazione Zoologica Anton Dohrn, ItalyReviewed by:
Grazia Marina Quero, Italian National Research Council, ItalyLucia Pita, GEOMAR Helmholtz Center for Ocean Research Kiel, Germany
Copyright © 2020 Rasmussen, Barnes, Mak, Kjartansdóttir, Hansen, Doan-Nhu, Nguyen-Ngoc, Guldberg Frøslev, Hellström and Hansen. This is an open-access article distributed under the terms of the Creative Commons Attribution License (CC BY). The use, distribution or reproduction in other forums is permitted, provided the original author(s) and the copyright owner(s) are credited and that the original publication in this journal is cited, in accordance with accepted academic practice. No use, distribution or reproduction is permitted which does not comply with these terms.
*Correspondence: Linett Rasmussen, bGluZXR0LnJhc211c3NlbkBiaW8ua3UuZGs=
†ORCID: Linett Rasmussen, orcid.org/0000-0002-8050-5732
‡Present address: Kristín Rós Kjartansdóttir and Thomas Arn Hansen, Rigshospitalet, Copenhagen, Denmark