- 1UFZ – Helmholtz Centre for Environmental Research, Department of Soil System Science, Halle (Saale), Germany
- 2UFZ – Helmholtz Centre for Environmental Research, Department of Environmental Microbiology, Leipzig, Germany
- 3German Centre for Integrative Biodiversity Research (iDiv) Halle-Jena-Leipzig, Leipzig, Germany
Soil is populated by highly diverse microbial communities mediating important processes and functions. The distribution of microbes, however, is neither uniform nor random. Instead, it is dictated by physical, chemical, and biological processes and conditions and varying over small spatial and temporal scales. The feedbacks between these processes make the soil-microbe complex a self-organizing system capable of adapting to the continuously changing conditions mainly driven by the highly fluctuating water content. For making meaningful predictions on the spatiotemporal dynamics of soil microbes and their functions, we need to integrate knowledge from physics, chemistry, and biology in our modeling approaches. Here, we review modeling studies with a focus on spatiotemporal dynamics of bacteria and bacterial functions in soil microhabitats. We compare these studies along four dimensions: specific aim, model type (individual-based, population-based), scale, and considered physical, chemical, biological processes and aspects. A special emphasis is laid on modeling approaches considering processes and aspects influencing the spatial distribution of bacteria such as motility, vector-based dispersal and biofilm formation. This includes factors like soil structure, carbon and oxygen gradients, temporal variations in hydration conditions or anthropogenic disturbance events. By assessing the importance of different microscale bacterial processes, this review should contribute to the ongoing discussion on challenges related to the upscaling from the microscopic via the profile to the landscape scale. Recent technical advances to observe bacteria in soils or soil-like environments combined with multidisciplinary collaborations will help to shed light on currently understudied physical, chemical and biological interactions in the soil-microbe complex.
Introduction
Soils harbor myriads of microorganisms providing ecosystem services such as nutrient cycling, carbon storage, water filtering, structure stabilization, pest control, and root growth promotion (Nannipieri et al., 2003; Pulleman et al., 2012; Schloter et al., 2017). Microbial activity is controlled by the physical, chemical, and biological conditions prevailing on the microscale but also feeds back on the soil conditions making the soil-microbe complex a self-organizing system (Young and Crawford, 2004). These interactions are highly dynamic along with continuously changing environmental conditions. Water content, for example, is linked to precipitation patterns and affects the distribution of microbes and their supply with substrates and oxygen. To analyze and predict these interactions, we need to take into account the temporal and spatial dynamics at the microscale (μm–cm). Although emerging visualization techniques and microscale laboratory methods like microfluidics have substantially enhanced our possibilities to study microorganisms in complex spatial settings (Aleklett et al., 2018; Baveye et al., 2018; Juyal et al., 2019), simulation models are still the most efficient tool to systematically analyze their behavior over a broad range of conditions.
A widely used approach for simulating bacterial dynamics in a spatially explicit environment is individual-based modeling (IBM) (Kreft et al., 2013; Hellweger et al., 2016). In IBMs, bacterial cells are considered as single entities enabling the simulation of different and dynamic properties within each cell including their stochastic behavior and evolution (Grimm, 1999). They operate in a discrete space and often consider processes like bacterial motility thereby introducing spatial dynamics even in absence of explicit spatial heterogeneity. A well-known application is species coexistence with non-hierarchical relationships, known as the rock-paper-scissor model (Kerr et al., 2002; Menezes et al., 2019). With simple process-rules in a discrete homogeneous space, these models simulate spatial patterns in highly dynamic coexistence behavior. IBMs can run in complex 2- or 3-D spatial structures mimicking soil systems (Portell et al., 2018) or be combined with pore network models (Ebrahimi and Or, 2015). Whereas soil bacterial communities consist of vast numbers of cells, IBMs are often restricted by computational power, especially if they are combined with complex physical structure models (O’Donnell et al., 2007). To overcome this constraint, population-based modeling (PBM) can be applied. In PBM, all individuals of one population are assumed to be equal in process behavior and properties, and their dynamics are often described by differential equations. The deterministic nature of PBMs allows for simulating a broad range of environmental conditions and the influence of stochasticity herein (Esser et al., 2015). Although PBMs often exclude space, there is an increasing number of microbial PBMs operating on discrete or continuous spatial areas, for example mimicking bacterial activity in the rhizosphere (Zelenev et al., 2000; Dupuy and Silk, 2016).
Here, we aim to identify physical, chemical, and biological aspects influencing the dynamics of bacteria and their functions on the microscale and give an overview on existing modeling approaches integrating such aspects. We discuss neglected but important aspects as well as missing experimental data, and what challenges we need to tackle prospectively to upscale soil bacterial processes from the microscale to scales relevant for land management. We want to point out that there is also huge effort and progress in modeling the dynamics of fungi in soils. However, for fungi the choice of the appropriate scale is much more difficult than for bacteria as mycelia may extend over and exert an influence on several hectares of soil while consisting of hyphae with a thickness of only several micrometers. Therefore, models simulating fungal networks are distinguished in micro-, intermediate and macro-scale models. They focus on processes such as tip growth, substrate uptake and branching of single hyphae (Schnepf et al., 2011), dynamics of hyphae constituting the mycelium and the extension of sparse fungal colonies over millimeters to centimeters (Pajor et al., 2010; Boswell and Davidson, 2012) and fungal biomass distribution, substrate decomposition, and translocation (Falconer et al., 2005, 2012, 2015). Due to the huge differences in fungal and bacterial life-history traits, a comparison of modeling approaches considering the interactions with their physical, chemical, and biological environment is beyond the scope of this mini review.
Modeling Physical, Chemical and Biological Aspects of Soil Bacterial Ecosystems
Soil is fairly different from other habitats. Soils contain a vast amount of microbial diversity, which can exceed the diversity in aquatic ecosystems by several orders of magnitude (Or et al., 2007). Overall, the specificity of soil manifests itself in complex spatiotemporal dynamics of microbial communities arising from the interaction with their physical, chemical, and biological environment. This interplay drives soil ecosystem functions and services and has been considered in modeling approaches albeit to different extents (Figure 1 and Table 1).
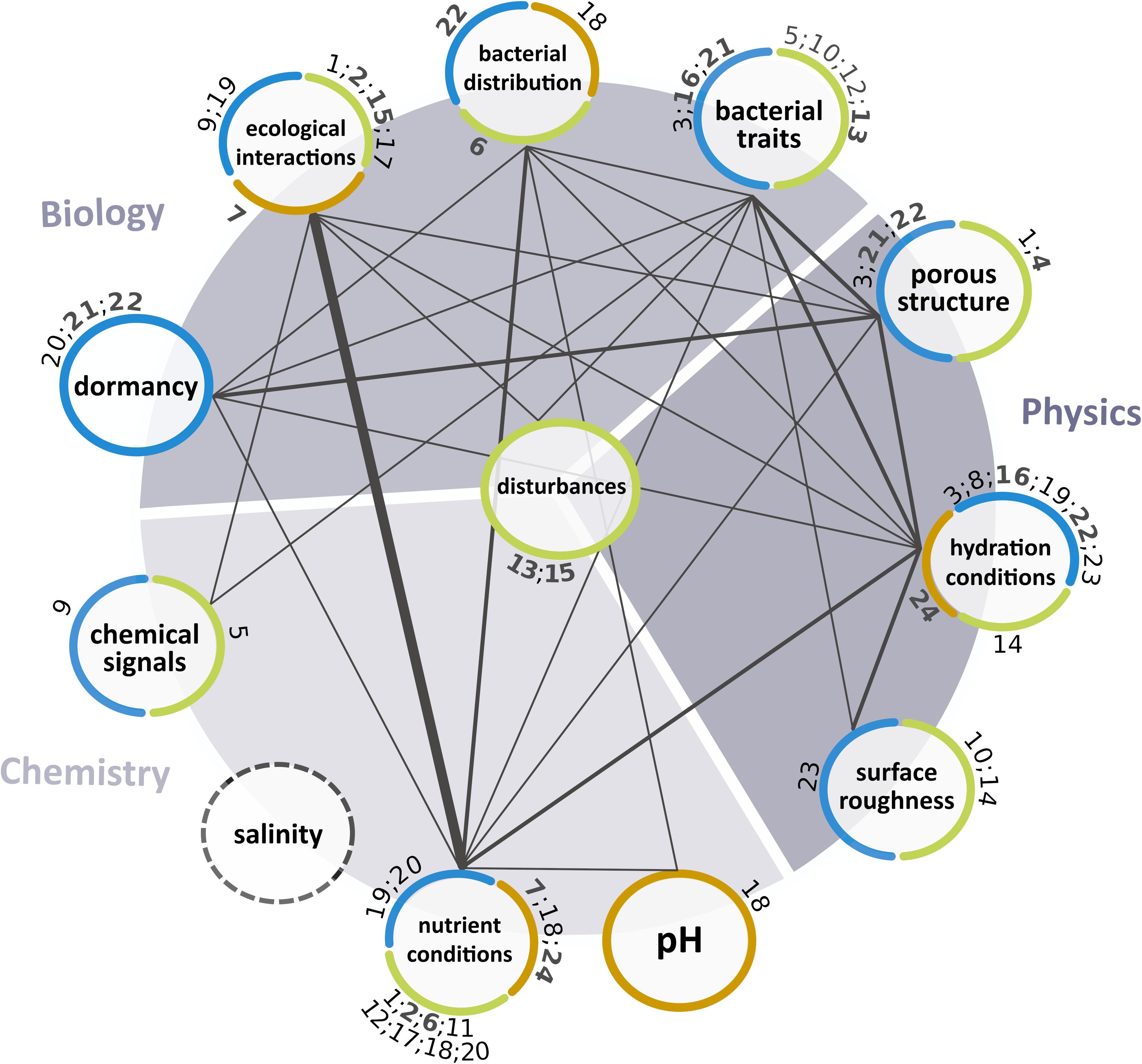
Figure 1. Physical, chemical, and biological aspects (circles) considered in the selected microscale models. The term “ecological interactions” summarizes interactions among bacteria and between bacteria and eukaryotes (e.g., fungi, plants). Color of the outline represents the simulation environment: explicit 2-D or 3-D pore networks (blue), grids (green), or root-shaped areas (orange). Connections indicate the joint consideration of two aspects in a model. Line thickness depicts the number of models considering both aspects ranging from one (1.5 pt), over two (3 pt) to five (7.5 pt). Numbers refer to the respective IBMs (black) or PBMs (gray, bold) in Table 1. Note that salinity was not considered in the selected models.
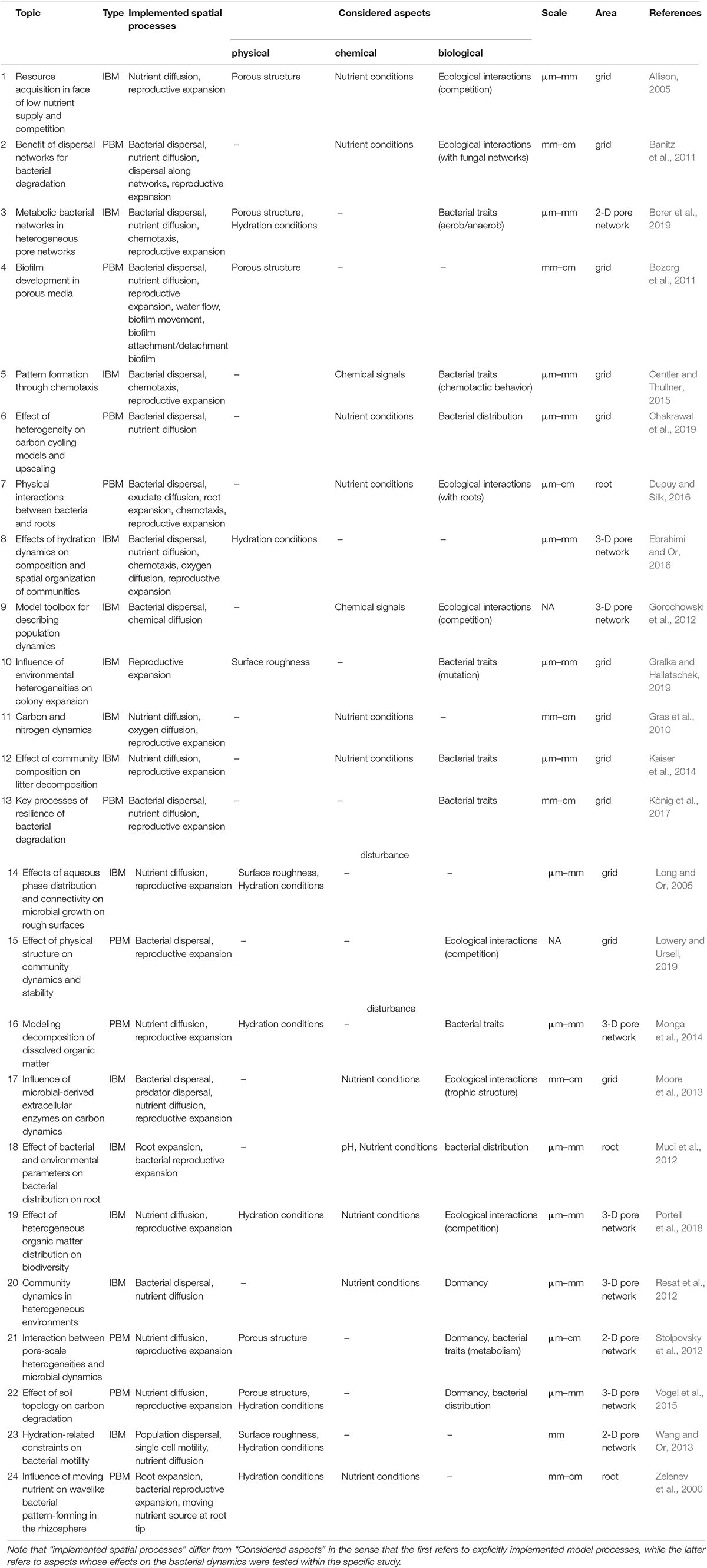
Table 1. Overview of different spatially explicit microbial models, thematic focus, implementation details and considered physical, chemical, and biological aspects (IBM, individual-based model; PBM, population-based model).
Physical Aspects of Soil Bacterial Ecosystems
Soils expose a high specific surface area (i.e., surface area per unit of mass) with less than 1% being covered by microbes (Chenu et al., 2002; Tecon and Or, 2017). This results in a patchy distribution of microbes and the emergence of spatially isolated bacterial microcolonies residing in poorly connected locations (Nunan et al., 2003; Young et al., 2008). The connectivity is controlled by a tortuous, dynamically changing pore network emerging from the complex arrangement of soil particles. The pore size and geometry determines in how far bacteria have access to their habitat. Narrow pores and pore neck diameters smaller than 0.2 μm prevent cell-to-cell contact and bacterial entry (Chenu et al., 2002). In addition, surface roughness of particles influences bacterial adhesion by shaping local diffusion fields (Mills, 2003; Long and Or, 2007; Wang and Or, 2010). When not fully saturated with water, soils exhibit three types of interfaces: solid-gas, solid-liquid, and liquid-gas. The liquid is retained in corners or adsorbed as thin films on surfaces (Tuller et al., 1999). Water flow and solute transport changes substantially with soil water content from slow seepage through smaller pores when dry to fast preferential flow through macropores at high water content (Or et al., 2007). Thereby, preferential flow paths transport higher loads of soluble carbon making them hotspots of biological activity (Bundt et al., 2001; Dechesne et al., 2014).
Using a 3-D IBM, Resat et al. (2012) modeled growth dynamics of a community consisting of species with different growth and survival strategies in response to heterogeneities in the structure and carbon distribution at the pore and aggregate scale. They showed that, although pore geometry had an influence, the trends of the growth dynamics at both scales were largely similar. This indicates that microscale simulations may be able to represent predicted dynamics occurring at larger scales. While this study assumed a fully saturated porous system, other modeling approaches revealed the importance of aqueous phase fragmentation on microbial processes and spatiotemporal dynamics. Using a roughness network, Long and Or (2007) simulated bacterial growth at different matric potentials and found that high matric potentials, which correspond to higher water contents and larger nutrient storage on the surface, led to higher abundances and faster expansion of the population. In subsequent studies, it was also shown that small reductions of the matric potential induced a sharp decrease in cell velocities to almost complete inhibition of colony expansion at −5 kPa (Wang and Or, 2010). The functional consequences of macropore topology, water saturation and bacterial distribution on biodegradation of a soluble substrate were assessed by coupling a lattice Boltzmann model and a compartmental biodegradation model in a 3-D pore scale model. CT images were used to reproduce environmental variations at the millimeter scale and the importance of physical and biological effects was tested in a full factorial design. A sensitivity analysis revealed the importance of physical factors for the biodegradation kinetics. Both, water saturation and pore topology influenced biodegradation by affecting diffusion and thus substrate availability for bacteria (Vogel et al., 2015).
Chemical Aspects of Soil Microbial Ecosystems
Under unsaturated conditions the matric potential is the major determinant of the soil water potential. Nevertheless, under specific conditions such as long drought periods, after fertilizer application, in certain locations near the soil surface or in the rhizosphere, the osmotic potential may become an important component of the water potential (Or et al., 2007). Moreover, irrigation increases soil salinity especially in arid regions where evaporation is high (Lambers, 2003). In saline soils, microbial biomass and activity tends to be reduced (Rietz and Haynes, 2003). In addition, processes such as bacterial chemotaxis and motility can be repressed (Steil et al., 2003). Conversely, rapid water infiltration can increase the water potential with effects on cell viability and integrity (Halverson et al., 2000). So far, modeling studies considering soil salinity effects on microbes are virtually absent. This may be due to the fact that the impact of the osmotic potential on convective and diffusive nutrient fluxes is negligible (Or et al., 2007; Tecon and Or, 2017) and empiric information on its effects on microbial processes are scarce. The situation is very similar for the soil redox potential, which is indicative of oxidation-reduction conditions. For a long time, the redox potential has received little attention in agricultural sciences. Soils were considered to be oxic and anoxia only expected in flooded and poorly drained soils. In addition, the measurement of the redox potential is associated with methodological difficulties including the high variability in space and time and the pH dependence (Husson, 2013). Oxygen concentrations vary with soil depth due to diffusion limitations and consumption by plant roots and microbes (Noll et al., 2005). Moreover, recent studies emphasized that anoxic microsites can comprise up to 85% of the pore volume also in unsaturated soils (Keiluweit et al., 2018). Anoxic regions arise in the interior of soil aggregates due to high microbial activity directly consuming the oxygen at the periphery and allowing aerobic and anaerobic microbial communities to coexist (Sexstone et al., 1985; Long and Or, 2005; Schlüter et al., 2018). In addition, oxygen availability determines the phylogenetic and physiological composition of soil bacterial communities as it selects for specialized metabolic types (Pett-Ridge and Firestone, 2005). Under anoxic conditions, the availability of alternative electron acceptors determines the type of anaerobic metabolism. Using an IBM with a 3-D angular pore network, Ebrahimi and Or (2016) exposed differently sized soil aggregates to variable carbon, water, and oxygen contents to estimate biogeochemical fluxes from soil profiles. They found that large aggregates support anaerobic activity under unsaturated conditions. Upscaling the model to the profile scale revealed a narrow region of denitrification activity in the soil profile, while deeper regions were devoid of aerobic and anaerobic activities due to low carbon concentrations (Ebrahimi and Or, 2016). Recently, Borer et al. (2018) investigated the impact of oxygen and carbon counter-gradients formed at the microscale inside soil aggregates and found that these gradients drive the segregation of aerobic and anaerobic bacterial populations which supported their coexistence (Borer et al., 2018).
In several studies, soil pH was proposed as the main factor determining the diversity of soil microbial communities (Fierer and Jackson, 2006; Lauber et al., 2009). In an arable soil, bacterial diversity was twice as high at pH 8 as at pH 4. In contrast, effects of pH on fungi were only minor (Rousk et al., 2010). Using molecular and geostatistical methods to analyze the distribution of microbial communities involved in N-cycling at the landscape scale, Bru et al. (2011) found that spatial variation in community abundances could largely be explained by environmental parameters with pH being dominant. However, similar to redox conditions, pH is often a bulk measure and conveys an imprecise picture of the conditions prevailing at the microscale. Despite its relevance for microorganisms, comparably few modeling studies integrate effects of pH. To study bacterial colonization of the root surface, Muci et al. (2012) used the cellular automata approach combined with an IBM and tested the influence and feedbacks of different environmental conditions including pH (Muci et al., 2012). Here, a lower pH decreases growth and activity of the bacteria, but was not systematically varied to test the direct effect of the pH on bacterial dynamics.
Biological Aspects of Soil Microbial Ecosystems
Microorganisms live in multispecies communities and interact with their neighbors via diverse mechanisms. In addition to the structural soil properties discussed above, microbial interactions are an important driver of population structure and dynamics at the microscale. Population dynamics models predict that in populations with strong antagonistic interactions, coexistence is globally unstable and competitive exclusion eventually leads to dominance of one species (Fukami, 2015). In spatially structured systems, such interactions become manifest in the emergence of a spatial pattern allowing for coexistence. Using a modified Lotka–Volterra model in a 2-D environment, Lowery and Ursell (2019) demonstrated that structured environments fostered the coexistence of two microbial species that could not coexist under homogenous conditions (Lowery and Ursell, 2019). Portell et al. (2018) simulated organic matter decomposition of a community consisting of three strains of Arthrobacter sp. with different competitive abilities using the previously described pore network approach with soil CT images in combination with an IBM. Here, a spatially heterogeneous distribution of organic matter was important for tripartite coexistence. Effects of social interactions between microbes on organic matter turnover and nitrogen retention were also tested with an IBM developed by Kaiser et al. (2015). Microbes exploiting the catalytic activities of others increased nitrogen retention and organic matter accumulation. Also, Wang and Or (2013) used their previously introduced hybrid modeling framework to test the influence of hydration dynamics on the coexistence of bacterial species and found that fragmented and disconnected aquatic niches emerging under dry conditions provide shelter for less competitive species. With microcosm experiments on sand, Tecon et al. (2018) showed that the contact frequency of bacterial cells increases with decreasing water content supporting these observations with a previously developed IBM. Microbes can also be involved in mutualistic interactions. A recently developed IBM coupled to a metabolic network model for soil habitats can represent trophic interactions among bacterial species, predicting the spatial organization and segregation of bacterial populations in response to oxygen and carbon gradients (Borer et al., 2019). However, spatial structure in microbial communities can also arise through self-organization. Depending on the nature of the interaction, spatial segregation or local mixing may occur (Wang and Or, 2014; Cordero and Datta, 2016). In an IBM considering a cooperating consortium, self-organization was found to be important to enable particulate carbon turnover (Ebrahimi et al., 2019).
The spatial organization and distribution of microbes in soil can also be influenced by interactions with other organisms including plants, earthworms and fungi. The effects of the latter on bacterial dispersal and biodegradation in homogeneous and heterogeneous systems were investigated via 2-D reaction-diffusion modeling. The results indicated that fungi can help bacteria to overcome areas of restricted motility (e.g., under dry conditions) thereby improving biodegradation of pollutants (Banitz et al., 2011). The rhizosphere is a hotspot for microbial activity, and several modeling studies investigated the interaction between bacteria and plant roots (Zelenev et al., 2000; Muci et al., 2012; Dupuy and Silk, 2016). For example, Dupuy and Silk (2016) observed in a PBM the benefits for bacteria attaching to the growing root compared to free-living bacteria. For investigating environmental conditions leading to wavelike patterns of bacteria in the rhizosphere, Zelenev et al. (2000) also developed a PBM describing bacterial population dynamics on a growing root releasing nutrients.
Modeling Spatially Structured Disturbances
Anthropogenic disturbances (i.e., land management, climate change) can considerably affect the soil microbial community composition or activity directly (de Vries et al., 2012; Griffiths and Philippot, 2013) or indirectly due to effects on the physical, chemical, or biological microenvironment (Figure 1). However, investigating effects of spatial disturbance on soil microorganisms is quite challenging, especially on the microscale. Some experimental studies made use of microcosm approaches and observed interesting spatial effects on community structure and function (Altermatt et al., 2011; Veraart et al., 2011; Baho et al., 2012; Worrich et al., 2016), although not on the microscale. Here, microfluidics or micromodels are promising tools to mimic soil pore structure especially when combined with new imaging techniques allowing for small-scale observations (Aleklett et al., 2018; Baveye et al., 2018). Recently, Co et al. (2019) applied microfluidics and observed an increase in antibiotic tolerance in spatially structured compared to intermixed populations (Co et al., 2019). However, the usage of microfluidics is an emerging field and until fully established, the application of simulation models to investigate microscale spatial disturbance effects is a powerful tool. Yet, there are surprisingly few microbial models focusing on this. In previous work, we established a PBM for simulating the response of bacterial population dynamics and activity to spatially heterogeneous disturbances (Banitz et al., 2011; König et al., 2017). We used bacterial degradation as an example for an important function of the soil microbial ecosystem and systematically tested spatial effects of differently distributed, lethal disturbances on the recovery, the long-term resistance (König et al., 2018b), and collapse probability (König et al., 2018a). Although we used a simple spatial structure (i.e., applied no explicit pore structure) and applied an artificial disturbance mimicking, for instance, antibiotic pulses; we observed a high sensitivity of the functional stability to the spatial disturbance distribution in all studies. In general, a higher fragmentation of the disturbance increases functional stability and can even compensate a larger disturbance area (König et al., 2019). This relationship needs to be evaluated for natural systems with more complex spatial structures using experimental systems and more detailed pore-scale models.
The Problem of Upscaling
Many current research questions related to soil management or the response of terrestrial ecosystems to climate change need to be addressed on larger scales to derive management and mitigation options. For answering those large scale questions, we need to identify if and to what extent processes and interactions on the microscale have to be included at least effectively or conceptually into models operating on the profile or even on the landscape scale. Upscaling information from the highly dynamic soil-microbe complex is an ambitious task hampered by lack of data for parametrization and validation, computational constrains, spatial and temporal oscillation artifacts, or interactions of biological features with physical and chemical factors (e.g., microbial-mineral interactions) (Wang et al., 2014; Wieder et al., 2015). Moreover, already in microscale research different scales are analyzed probably hampering the comparability. Here, the reviewed model scales range from μm to cm scale, depending on the specific research question. For instance, models incorporating metabolic networks using methods like flux-balance analysis need to simulate the μm scale to depict single bacterial cells (Borer et al., 2019), while models focusing on the physical interaction between bacteria and roots need to simulate at larger scales to capture root size (Dupuy and Silk, 2016). We need to carefully consider for which research questions the effort of upscaling microbial processes is necessary to understand the whole systems dynamics. For instance, the integration of biological processes into soil models will likely increase our process understanding of biogeochemical cycles and carbon storage, especially under critical environmental conditions such as droughts (Fry et al., 2018). Spatial and temporal upscaling of models can be proceeded by applying different approaches like simplifying the model, averaging model outputs or model equations, or using effective parameters (Bierkens et al., 2000). Many soil models use simplified microbial dynamics by integrating turnover rates or microbial biomass as implicit bulk variable, for example, for simulation of carbon or nitrogen cycling on different scales (Manzoni and Porporato, 2009; Schimel and Schaeffer, 2012). Simplification of models allows for better understanding emergent model dynamics and feedbacks and reduces data requirements, which is often a problem for soil model parametrization, especially for biological parameters (Wieder et al., 2015; Baveye et al., 2018). A prerequisite for simplification should be that complex effects or processes are sufficiently understood. Then, one can successively reduce its complexity while continuously checking if the model outcome still fits our observations. Recently, it was shown that a soil organic carbon model with explicit microbial dynamics can reproduce long-term and global soil carbon data (Woolf and Lehmann, 2019). For integrating microscale effects into the prediction of greenhouse gas emission Ebrahimi and Or (2018) used a microbial numerical model to parameterize an analytical model with simplified aggregate structure allowing for upscaling to the landscape scale, though the prediction accuracy of average emission rates was only moderate in this case. The authors previously published several studies on microbial modeling on the aggregate or pore scale (e.g., Ebrahimi and Or, 2014, 2015), strengthening the implication that knowledge on the microscale is needed to reasonably scale-up processes of the soil-microbe complex. Extrapolation of microbial dynamics or mathematical formulations from the microscale to the macroscale requires a thorough understanding of the underlying mechanisms (Wang et al., 2014; Chakrawal et al., 2019).
Future Directions
Several exciting modeling approaches considering different aspects affecting the spatiotemporal dynamics of soil microbial ecosystems arose in the last two decades. Our review reveals that we are on a good way to integrate physical, chemical and biological aspects for a systemic view on microscale effects in soil microbiology. However, further experimental work is needed to understand processes and effects which are not sufficiently understood such as salinity, pH effects or spatial disturbances. As already discussed, microfluidics are a promising approach for digging deeper into microbial interactions (Aleklett et al., 2018; Baveye et al., 2018) and the increasing resolution of imaging techniques enables us to observe spatial patterns of microbes in their soil microhabitats (Juyal et al., 2019). Also, more modeling work is needed integrating aspects of all disciplines to carefully investigate microbial dynamics and functions on the microscale to gain a systemic understanding of the soil microbial ecosystem. This understanding is necessary to enable soil modelers to include microbial processes in models operating on larger scales, without describing those processes in full detail. Another problem is a possible discrepancy between measured field values and actual local conditions on the microscale. The latter may have an effect on microbial activity which will be under- or overestimated when parametrizing our models with measured field data differing from local conditions. One of the key challenges for achieving the integration of microbial microscale dynamics to profile or landscape models is model validation. The observation of microbial data such as abundance, community composition, or respiration rate gets more and more into focus of long-term field experiments (Geisseler and Scow, 2014; Romero-Olivares et al., 2017). However, it will take decades to get reliable information on the long-term impact of climate change or agricultural practices on microbial dynamics and functions. Until then, a possibility is to validate our models by comparison of time series of specific microbial-related functions, as it was previously done, for instance, for soil carbon dynamics (Wieder et al., 2013; Woolf and Lehmann, 2019).
It remains a scientific challenge to model the soil-microbe complex and its related functions and we suggest collaborating closely with researchers from soil physics, chemistry, and biology to incorporate knowledge of different disciplines on all relevant scales.
Author Contributions
SK and AW designed the scope of the manuscript, performed the literature research, and prepared the manuscript. H-JV and HH contributed further ideas and substantially revised the manuscript.
Funding
This work was funded by the Helmholtz Association via the integrated project Controlling Chemicals Fate of the research topic Chemicals in the Environment within the research program Terrestrial Environment and by the German Federal Ministry of Education and Research in the project “BonaRes (Module B): BonaRes Centre for Soil Research” (grant 031B0511).
Conflict of Interest
The authors declare that the research was conducted in the absence of any commercial or financial relationships that could be construed as a potential conflict of interest.
References
Aleklett, K., Kiers, E. T., Ohlsson, P., Shimizu, T. S., Caldas, V. E., and Hammer, E. C. (2018). Build your own soil: exploring microfluidics to create microbial habitat structures. ISME J. 12, 312–319. doi: 10.1038/ismej.2017.184
Allison, S. D. (2005). Cheaters, diffusion and nutrients constrain decomposition by microbial enzymes in spatially structured environments. Ecol. Lett. 8, 626–635. doi: 10.1111/j.1461-0248.2005.00756.x
Altermatt, F., Bieger, A., Carrara, F., Rinaldo, A., and Holyoak, M. (2011). Effects of connectivity and recurrent local disturbances on community structure and population density in experimental metacommunities. PLoS One 6:e19525. doi: 10.1371/journal.pone.0019525
Baho, D. L., Peter, H., and Tranvik, L. J. (2012). Resistance and resilience of microbial communities–temporal and spatial insurance against perturbations. Environ. Microbiol. 14, 2283–2292. doi: 10.1111/j.1462-2920.2012.02754.x
Banitz, T., Fetzer, I., Johst, K., Wick, L. Y., Harms, H., and Frank, K. (2011). Assessing biodegradation benefits from dispersal networks. Ecol. Model. 222, 2552–2560. doi: 10.1016/j.ecolmodel.2010.07.005
Baveye, P. C., Otten, W., Kravchenko, A., Balseiro-Romero, M., Beckers, É, Chalhoub, M., et al. (2018). Emergent properties of microbial activity in heterogeneous soil microenvironments: different research approaches are slowly converging, yet major challenges remain. Front. Microbiol. 9:1929. doi: 10.3389/fmicb.2018.01929
Bierkens, M. F. P., Finke, P. A., and De Willigen, P. (2000). Upscaling and Downscaling Methods for Environmental Research. Dordrecht: Kluwer Academic Publishers.
Borer, B., Ataman, M., Hatzimanikatis, V., and Or, D. (2019). Modeling metabolic networks of individual bacterial agents in heterogeneous and dynamic soil habitats (IndiMeSH). PLoS Comput. Biol. 15:e1007127. doi: 10.1371/journal.pcbi.1007127
Borer, B., Tecon, R., and Or, D. (2018). Spatial organization of bacterial populations in response to oxygen and carbon counter-gradients in pore networks. Nat. Commun. 9:769. doi: 10.1038/s41467-018-03187-y
Boswell, G. P., and Davidson, F. A. (2012). Modelling hyphal networks. Fungal Biol. Rev. 26, 30–38. doi: 10.1016/j.fbr.2012.02.002
Bozorg, A., Sen, A., and Gates, I. D. (2011). A new approach to model the spatiotemporal development of biofilm phase in porous media. Environ. Microbiol. 13, 3010–3023. doi: 10.1111/j.1462-2920.2011.02578.x
Bru, D., Ramette, A., Saby, N. P., Dequiedt, S., Ranjard, L., Jolivet, C., et al. (2011). Determinants of the distribution of nitrogen-cycling microbial communities at the landscape scale. ISME J. 5, 532–542. doi: 10.1038/ismej.2010
Bundt, M., Widmer, F., Pesaro, M., Zeyer, J., and Blasera, P. (2001). Preferential flow paths: biological ‘hot spots’ in soils. Soil Biol. Biochem. 33, 729–738. doi: 10.1016/s0038-0717(00)00218-2
Centler, F., and Thullner, M. (2015). Chemotactic preferences govern competition and pattern formation in simulated two-strain microbial communities. Front. Microbiol. 6:40. doi: 10.3389/fmicb.2015.00040
Chakrawal, A., Herrmann, A. M., Koestel, J., Jarsjö, J., Nunan, N., Kätterer, T., et al. (2019). Dynamic upscaling of decomposition kinetics for carbon cycling models. Geosci. Model Dev. Discuss. doi: 10.5194/gmd-2019-133
Chenu, C., Stotzky, G., Huang, P., and Bollag, J. (2002). “Interactions between microorganisms and soil particles: an overview,”in Interactions Between soil Particles and Microorganisms eds P. M. Huang, J. -M. Bollag, and N. Senesi (Nwe York, NY: Wiley & Sons), 1–40.
Co, A. D., Vliet, S. V., and Ackermann, M. (2019). Emergent microscale gradients give rise to metabolic cross-feeding and antibiotic tolerance in clonal bacterial populations. Philos. Trans. R Soc. Lond. B Biol. Sci. 374:20190080. doi: 10.1098/rstb.2019.0080
Cordero, O. X., and Datta, M. S. (2016). Microbial interactions and community assembly at microscales. Curr. Opin. Microbiol. 31, 227–234. doi: 10.1016/j.mib.2016.03.015
de Vries, F. T., Liiri, M. E., Bjørnlund, L., Bowker, M. A., Christensen, S., Setälä, H. M., et al. (2012). Land use alters the resistance and resilience of soil food webs to drought. Nat. Clim. Change 2, 276–280. doi: 10.1038/nclimate1368
Dechesne, A., Badawi, N., Aamand, J., and Smets, B. F. (2014). Fine scale spatial variability of microbial pesticide degradation in soil: scales, controlling factors, and implications. Front. Microbiol. 5:667. doi: 10.3389/fmicb.2014.00667
Dupuy, L. X., and Silk, W. K. (2016). Mechanisms of Early Microbial Establishment on Growing Root Surfaces. Vadose Zone J. 15:vzj2015.06.0094. doi: 10.2136/vzj2015.06.0094
Ebrahimi, A., and Or, D. (2015). Hydration and diffusion processes shape microbial community organization and function in model soil aggregates. Water Resour. Res. 51, 9804–9827. doi: 10.1002/2015wr017565
Ebrahimi, A., and Or, D. (2016). Microbial community dynamics in soil aggregates shape biogeochemical gas fluxes from soil profiles - upscaling an aggregate biophysical model. Global Change Biol. 22, 3141–3156. doi: 10.1111/gcb.13345
Ebrahimi, A., and Or, D. (2018). On upscaling of soil microbial processes and biogeochemical fluxes from aggregates to landscapes. J. Geophys. Res. Biogeosci. 123, 1526–1547. doi: 10.1029/2017JG004347
Ebrahimi, A. N., and Or, D. (2014). Microbial dispersal in unsaturated porous media: characteristics of motile bacterial cell motions in unsaturated angular pore networks. Water Resour. Res. 50, 7406–7429. doi: 10.1002/2014wr015897
Ebrahimi, A., Schwartzman, J., and Cordero, O. X. (2019). Cooperation and spatial self-organization determine ecosystem function for polysaccharide-degrading bacteria. bioRxiv [Preprint].
Esser, D. S., Leveau, J. H., and Meyer, K. M. (2015). Modeling microbial growth and dynamics. Appl. Microbiol. Biotechnol. 99, 8831–8846.
Falconer, R., Bown, J. L., White, N. A., and Crawford, J. W. (2005). Biomass recycling and the origin of phenotype in fungal mycelia. Proc. R. Soc. Lond. 272, 1727–1734. doi: 10.1098/rspb.2005.3150
Falconer, R. E., Battaia, G., Schmidt, S., Baveye, P., Chenu, C., and Otten, W. (2015). Microscale heterogeneity explains experimental variability and non-linearity in soil organic matter mineralisation. PLoS One 10:e0123774. doi: 10.1371/journal.pone.0123774
Falconer, R. E., Houston, A. N., Otten, W., and Baveye, P. C. (2012). Emergent Behavior of Soil Fungal Dynamics. Soil Sci. 177, 111–119. doi: 10.1097/ss.0b013e318241133a
Fierer, N., and Jackson, B. (2006). The diversity and biogeography of soil bacterial communities. Proc. Natl. Acad. Sci. U.S.A. 103, 626–631. doi: 10.1073/pnas.0507535103
Fry, E. L., De Long, J. R., Álvarez Garrido, L., Alvarez, N., Carrillo, Y., Castañeda−Gómez, L., et al. (2018). Using plant, microbe, and soil fauna traits to improve the predictive power of biogeochemical models. Methods Ecol. Evol. 10, 146–157. doi: 10.1111/2041-210x.13092
Fukami, T. (2015). Historical contingency in community assembly: integrating niches. species pools, and priority effects. Annu. Rev. Ecol., Evol. Syst. 46, 1–23. doi: 10.1111/nph.13362
Geisseler, D., and Scow, K. M. (2014). Long-term effects of mineral fertilizers on soil microorganisms – A review. Soil Biol. Biochem. 75, 54–63. doi: 10.1016/j.soilbio.2014.03.023
Gorochowski, T. E., Matyjaszkiewicz, A., Todd, T., Oak, N., Kowalska, K., Reid, S., et al. (2012). BSim: an agent-based tool for modeling bacterial populations in systems and synthetic biology. PLoS One 7:e42790. doi: 10.1371/journal.pone.0042790
Gralka, M., and Hallatschek, O. (2019). Environmental heterogeneity can tip the population genetics of range expansions. eLife 8:e44359. doi: 10.7554/eLife.44359
Gras, A., Ginovart, M., Portell, X., and Baveye, P. C. (2010). Individual-based modeling of carbon and nitrogen dynamics in soils. Soil Sci. 175, 363–374. doi: 10.1111/gcb.13542
Griffiths, B. S., and Philippot, L. (2013). Insights into the resistance and resilience of the soil microbial community. FEMS Microbiol. Rev. 37, 112–129. doi: 10.1111/j.1574-6976.2012.00343.x
Grimm, V. (1999). Ten years of individual-based modelling in ecology: what have we learned and what could we learn in the future? Ecol. Model. 115, 129–148. doi: 10.1016/s0304-3800(98)00188-4
Halverson, L. J., Jones, T. M., and Firestone, M. K. (2000). Release of Intracellular solutes by four soil bacteria exposed to dilution stress. Soil Sci. Soc. Am. J. 64, 1630–1637. doi: 10.2136/sssaj2000.6451630x
Hellweger, F. L., Clegg, R. J., Clark, J. R., Plugge, C. M., and Kreft, J. U. (2016). Advancing microbial sciences by individual-based modelling. Nat. Rev. Microbiol. 14, 461–471. doi: 10.1038/nrmicro.2016.62
Husson, O. (2013). Redox potential (Eh) and pH as drivers of soil/plant/microorganism systems: a transdisciplinary overview pointing to integrative opportunities for agronomy. Plant Soil 362, 389–417. doi: 10.1007/s11104-012-1429-7
Juyal, A., Otten, W., Falconer, R., Hapca, S., Schmidt, H., Baveye, P. C., et al. (2019). Combination of techniques to quantify the distribution of bacteria in their soil microhabitats at different spatial scales. Geoderma 334, 165–174. doi: 10.1016/j.geoderma.2018.07.031
Kaiser, C., Franklin, O., Dieckmann, U., and Richter, A. (2014). Microbial community dynamics alleviate stoichiometric constraints during litter decay. Ecol. Lett. 17, 680–690. doi: 10.1111/ele.12269
Kaiser, C., Franklin, O., Richter, A., and Dieckmann, U. (2015). Social dynamics within decomposer communities lead to nitrogen retention and organic matter build-up in soils. Nat. Commun. 6:8960. doi: 10.1038/ncomms9960
Keiluweit, M., Gee, K., Denney, A., and Fendorf, S. (2018). Anoxic microsites in upland soils dominantly controlled by clay content. Soil Biol. Biochem. 118, 42–50. doi: 10.1016/j.soilbio.2017.12.002
Kerr, B., Riley, M. A., Feldman, M. W., and Bohannan, B. J. M. (2002). Local dispersal promotes biodiversity in a real-life game of rock–paper–scissors. Nature 418, 171–174. doi: 10.1038/nature00823
König, S., Köhnke, M. C., Firle, A.-L., Banitz, T., Centler, F., Frank, K., et al. (2019). Disturbance size can be compensated for by spatial fragmentation in soil microbial ecosystems. Front. Ecol. Evol. 7:290. doi: 10.3389/fevo.2019.00290
König, S., Worrich, A., Banitz, T., Centler, F., Harms, H., Kastner, M., et al. (2018a). Spatiotemporal disturbance characteristics determine functional stability and collapse risk of simulated microbial ecosystems. Sci. Rep. 8:9488. doi: 10.1038/s41598-018-27785-4
König, S., Worrich, A., Banitz, T., Harms, H., Kästner, M., Miltner, A., et al. (2018b). Functional resistance to recurrent spatially heterogeneous disturbances is facilitated by increased activity of surviving bacteria in a virtual ecosystem. Front. Microbiol. 9:734. doi: 10.3389/fmicb.2018.00734
König, S., Worrich, A., Centler, F., Wick, L. Y., Miltner, A., Kästner, M., et al. (2017). Modelling functional resilience of microbial ecosystems: analysis of governing processes. Environ. Model. Software 89, 31–39. doi: 10.1016/j.envsoft.2016.11.025
Kreft, J. U., Plugge, C. M., Grimm, V., Prats, C., Leveau, J. H., Banitz, T., et al. (2013). Mighty small: observing and modeling individual microbes becomes big science. Proc. Natl. Acad. Sci. U.S.A. 110, 18027–18028. doi: 10.1073/pnas.1317472110
Lambers, H. (2003). Introduction: dryland salinity: a key environmental issue in southern Australia. Plant Soil 257, 5–7.
Lauber, C. L., Hamady, M., Knight, R., and Fierer, N. (2009). Pyrosequencing-based assessment of soil pH as a predictor of soil bacterial community structure at the continental scale. Appl. Environ. Microbiol. 75, 5111–5120. doi: 10.1128/AEM.00335-09
Long, T., and Or, D. (2005). Aquatic habitats and diffusion constraints affecting microbial coexistence in unsaturated porous media. Water Resour. Res. 41:W08408.
Long, T., and Or, D. (2007). Microbial growth on partially saturated rough surfaces: simulations in idealized roughness networks. Water Resour. Res. 43:W02409.
Lowery, N. V., and Ursell, T. (2019). Structured environments fundamentally alter dynamics and stability of ecological communities. Proc. Natl. Acad. Sci. U.S.A. 116, 379–388. doi: 10.1073/pnas.1811887116
Manzoni, S., and Porporato, A. (2009). Soil carbon and nitrogen mineralization: theory and models across scales. Soil Biol. Biochem. 41, 1355–1379. doi: 10.1016/j.soilbio.2009.02.031
Menezes, J., Moura, B., and Pereira, T. A. (2019). Uneven rock-paper-scissors models: patterns and coexistence. EPL Europhys. Lett. 126:18003. doi: 10.1209/0295-5075/126/18003
Mills, A. L. (2003). “Keeping in touch: microbial life on soil particle surfaces,” in Advances in Agronomy, Vol. 78, ed. D. L. Sparks (Amsterdam: Elsevier), 1–43.
Monga, O., Garnier, P., Pot, V., Coucheney, E., Nunan, N., Otten, W., et al. (2014). Simulating microbial degradation of organic matter in a simple porous system using the 3-D diffusion-based model MOSAIC. Biogeosciences 11, 2201–2209. doi: 10.5194/bg-11-2201-2014
Moore, J. C., Boone, R. B., Koyama, A., and Holfelder, K. (2013). Enzymatic and detrital influences on the structure, function, and dynamics of spatially-explicit model ecosystems. Biogeochemistry 117, 205–227. doi: 10.1007/s10533-013-9932-3
Muci, A. L., Jorquera, M. A., Ávila, ÁI., Rengel, Z., Crowley, D. E., and De La Luz Mora, M. (2012). A combination of cellular automata and agent-based models for simulating the root surface colonization by bacteria. Ecol. Model. 247, 1–10. doi: 10.1016/j.ecolmodel.2012.07.035
Nannipieri, P., Ascher, J., Ceccherini, M. T., Landi, L., Pietramellara, G., and Renella, G. (2003). Microbial diversity and soil functions. Eur. J. Soil Sci. 54, 655–670. doi: 10.1046/j.1351-0754.2003.0556.x
Noll, M., Matthies, D., Frenzel, P., Derakshani, M., and Liesack, W. (2005). Succession of bacterial community structure and diversity in a paddy soil oxygen gradient. Environ. Microbiol. 7, 382–395. doi: 10.1111/j.1462-2920.2005.00700.x
Nunan, N., Wu, K., Young, I. M., Crawford, J. W., and Ritz, K. (2003). Spatial distribution of bacterial communities and their relationships with the micro-architecture of soil. FEMS Microbiol. Ecol. 44, 203–215. doi: 10.1016/S0168-6496(03)00027-8
O’Donnell, A. G., Young, I. M., Rushton, S. P., Shirley, M. D., and Crawford, J. W. (2007). Visualization, modelling and prediction in soil microbiology. Nat. Rev. Microbiol. 5, 689–699. doi: 10.1038/nrmicro1714
Or, D., Smets, B. F., Wraith, J. M., Dechesne, A., and Friedman, S. P. (2007). Physical constraints affecting bacterial habitats and activity in unsaturated porous media – a review. Adv. Water Resour. 30, 1505–1527. doi: 10.1016/j.advwatres.2006.05.025
Pajor, R., Falconer, R., Hapca, S., and Otten, W. (2010). Modelling and quantifying the effect of heterogeneity in soil physical conditions on fungal growth. Biogeosciences 7, 3731–3740. doi: 10.5194/bg-7-3731-2010
Pett-Ridge, J., and Firestone, M. K. (2005). Redox fluctuation structures microbial communities in a wet tropical soil. Appl. Environ. Microbiol. 71, 6998–7007. doi: 10.1128/aem.71.11.6998-7007.2005
Portell, X., Pot, V., Garnier, P., Otten, W., and Baveye, P. C. (2018). microscale heterogeneity of the spatial distribution of organic matter can promote bacterial biodiversity in soils: insights from computer simulations. Front. Microbiol. 9:1583. doi: 10.3389/fmicb.2018.01583
Pulleman, M., Creamer, R., Hamer, U., Helder, J., Pelosi, C., Pérès, G., et al. (2012). Soil biodiversity, biological indicators and soil ecosystem services—an overview of European approaches. Curr. Opin. Env. Sust. 4, 529–538. doi: 10.1016/j.cosust.2012.10.009
Resat, H., Bailey, V., Mccue, L. A., and Konopka, A. (2012). Modeling microbial dynamics in heterogeneous environments: growth on soil carbon sources. Microb. Ecol. 63, 883–897. doi: 10.1007/s00248-011-9965-x
Rietz, D. N., and Haynes, R. J. (2003). Effects of irrigation-induced salinity and sodicity on soil microbial activity. Soil Biol. Biochem. 35, 845–854. doi: 10.1016/s0038-0717(03)00125-1
Romero-Olivares, A. L., Allison, S. D., and Treseder, K. K. (2017). Soil microbes and their response to experimental warming over time: a meta-analysis of field studies. Soil Biol. Biochem. 107, 32–40. doi: 10.1016/j.soilbio.2016.12.026
Rousk, J., Baath, E., Brookes, P. C., Lauber, C. L., Lozupone, C., Caporaso, J. G., et al. (2010). Soil bacterial and fungal communities across a pH gradient in an arable soil. ISME J. 4, 1340–1351. doi: 10.1038/ismej.2010.58
Schimel, J. P., and Schaeffer, S. M. (2012). Microbial control over carbon cycling in soil. Front. Microbiol. 3:348. doi: 10.3389/fmicb.2012.00348
Schloter, M., Nannipieri, P., Sørensen, S. J., and Van Elsas, J. D. (2017). Microbial indicators for soil quality. Biol. Fertility Soils 54, 1–10.
Schlüter, S., Henjes, S., Zawallich, J., Bergaust, L., Horn, M., Ippisch, O., et al. (2018). Denitrification in soil aggregate analogues-effect of aggregate size and oxygen diffusion. Front Environ. Sci. 6:17. doi: 10.3389/fenvs.2018.00017
Schnepf, A., Jones, D., and Roose, T. (2011). Modelling nutrient uptake by individual hyphae of arbuscular mycorrhizal fungi: temporal and spatial scales for an experimental design. Bull. Math. Biol. 73, 2175–2200. doi: 10.1007/s11538-010-9617-1
Sexstone, A. J., Revsbech, N. P., Parkin, T. B., and Tiedje, J. M. (1985). Direct measurement of oxygen profiles and denitrification rates in soil aggregates1. Soil Sci. Soc. Am. J. 49, 645–651. doi: 10.2136/sssaj1985.03615995004900030024x
Steil, L., Hoffmann, T., Budde, I., Völker, U., and Bremer, E. (2003). Genome-wide transcriptional profiling analysis of adaptation of Bacillus subtilis to high salinity. J. Bacteriol. 185, 6358–6370. doi: 10.1128/jb.185.21.6358-6370.2003
Stolpovsky, K., Gharasoo, M., and Thullner, M. (2012). The impact of pore-size heterogeneities on the spatiotemporal variation of microbial metabolic activity in porous media. Soil Sci. 177, 98–110. doi: 10.1097/ss.0b013e318241105d
Tecon, R., Ebrahimi, A., Kleyer, H., Erev Levi, S., and Or, D. (2018). Cell-to-cell bacterial interactions promoted by drier conditions on soil surfaces. Proc. Natl. Acad. Sci. U.S.A. 115, 9791–9796. doi: 10.1073/pnas.1808274115
Tecon, R., and Or, D. (2017). Biophysical processes supporting the diversity of microbial life in soil. FEMS Microbiol. Rev. 41, 599–623. doi: 10.1093/femsre/fux039
Tuller, M., Or, D., and Dudley, L. M. (1999). Adsorption and capillary condensation in porous media: liquid retention and interfacial configurations in angular pores. Water Resour. Res. 35, 1949–1964. doi: 10.1029/1999wr900098
Veraart, A. J., Faassen, E. J., Dakos, V., Van Nes, E. H., Lurling, M., and Scheffer, M. (2011). Recovery rates reflect distance to a tipping point in a living system. Nature 481, 357–359. doi: 10.1038/nature10723
Vogel, L. E., Makowski, D., Garnier, P., Vieublé-Gonod, L., Coquet, Y., Raynaud, X., et al. (2015). Modeling the effect of soil meso- and macropores topology on the biodegradation of a soluble carbon substrate. Adv. Water Resour. 83, 123–136. doi: 10.1016/j.advwatres.2015.05.020
Wang, G., and Or, D. (2010). Aqueous films limit bacterial cell motility and colony expansion on partially saturated rough surfaces. Environ. Microbiol. 12, 1363–1373. doi: 10.1111/j.1462-2920.2010.02180.x
Wang, G., and Or, D. (2013). Hydration dynamics promote bacterial coexistence on rough surfaces. ISME J. 7, 395–404. doi: 10.1038/ismej.2012.115
Wang, G., and Or, D. (2014). Trophic interactions induce spatial self-organization of microbial consortia on rough surfaces. Sci. Rep. 4:6757. doi: 10.1038/srep06757
Wang, Y. P., Chen, B. C., Wieder, W. R., Leite, M., Medlyn, B. E., Rasmussen, M., et al. (2014). Oscillatory behavior of two nonlinear microbial models of soil carbon decomposition. Biogeosciences 11, 1817–1831. doi: 10.5194/bg-11-1817-2014
Wieder, W. R., Allison, S. D., Davidson, E. A., Georgiou, K., Hararuk, O., He, Y., et al. (2015). Explicitly representing soil microbial processes in Earth system models. Global Biogeochem. Cy. 29, 1782–1800. doi: 10.1111/j.1461-0248.2012.01807.x
Wieder, W. R., Bonan, G. B., and Allison, S. D. (2013). Global soil carbon projections are improved by modelling microbial processes. Nat. Clim. Change 3, 909–912. doi: 10.1038/nclimate1951
Woolf, D., and Lehmann, J. (2019). Microbial models with minimal mineral protection can explain long-term soil organic carbon persistence. Sci. Rep. 9:6522. doi: 10.1038/s41598-019-43026-8
Worrich, A., Konig, S., Banitz, T., Centler, F., Frank, K., Thullner, M., et al. (2016). Bacterial Dispersal Promotes Biodegradation in Heterogeneous Systems Exposed to Osmotic Stress. Front. Microbiol. 7:1214. doi: 10.3389/fmicb.2016.01214
Young, I. M., and Crawford, J. W. (2004). Interactions and self-organization in the soil-microbe complex. Science 304, 1634–1637. doi: 10.1126/science.1097394
Young, I. M., Crawford, J. W., Nunan, N., Otten, W., and Spiers, A. (2008). “Microbial distribution in soils: physics and scaling,” in Advances in Agronomy, Vol. 100, ed. D. L. Sparks (Amsterdam: Elsevier), 81–121.
Keywords: soil processes, soil structure, bacterial models, simulation, upscaling
Citation: König S, Vogel H-J, Harms H and Worrich A (2020) Physical, Chemical and Biological Effects on Soil Bacterial Dynamics in Microscale Models. Front. Ecol. Evol. 8:53. doi: 10.3389/fevo.2020.00053
Received: 13 September 2019; Accepted: 19 February 2020;
Published: 20 March 2020.
Edited by:
Valerie Pot, INRA Centre Versailles-Grignon, FranceReviewed by:
Stilianos Fodelianakis, Swiss Federal Institute of Technology in Lausanne, SwitzerlandXavier Raynaud, Université Paris-Sorbonne, France
Copyright © 2020 König, Vogel, Harms and Worrich. This is an open-access article distributed under the terms of the Creative Commons Attribution License (CC BY). The use, distribution or reproduction in other forums is permitted, provided the original author(s) and the copyright owner(s) are credited and that the original publication in this journal is cited, in accordance with accepted academic practice. No use, distribution or reproduction is permitted which does not comply with these terms.
*Correspondence: Sara König, sara.koenig@ufz.de; Anja Worrich, anja.worrich@ufz.de