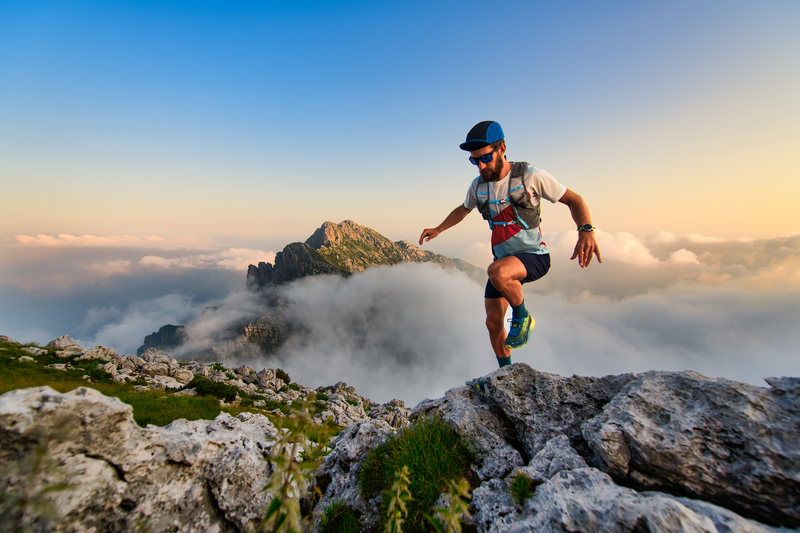
95% of researchers rate our articles as excellent or good
Learn more about the work of our research integrity team to safeguard the quality of each article we publish.
Find out more
ORIGINAL RESEARCH article
Front. Ecol. Evol. , 26 February 2020
Sec. Evolutionary Ecology of Social Behaviour
Volume 8 - 2020 | https://doi.org/10.3389/fevo.2020.00026
This article is part of the Research Topic Microbial Drivers of Sociality – from Multicellularity to Animal Societies View all 18 articles
Selection pressures from pathogens appear to play an important role in shaping social evolution. Social behavior, in particular brood care, is associated with pathogen pressure in wood-dwelling “lower” termites. Yet, generally pathogen pressure is predicted to be low in wood-dwelling termite species that never leave the nest except for the mating flight. In comparison, pathogen pressure is predicted to be higher in species that leave the nest to forage, and thus constantly encounter a diversity of microbes from their environment. We hypothesized that such differences in predicted pathogen pressure are also reflected by differences in the intensity of natural selection on immune genes. We tested this hypothesis in a phylogenetic framework, analyzing rates of non-synonymous and synonymous substitutions on single-copy immune genes. Therefore, we leveraged recent genomic and transcriptomic data from eight termite species, representing wood-dwelling and foraging species as well as 14 additional species spanning the winged insects (Pterygota). Our results provide no evidence for a role of pathogen pressure in selection intensity on single-copy immune genes. Instead, we found evidence for a genome-wide pattern of relaxed selection in termites.
Life Science Identifiers (as available Zoobank)
Ephemera danica:
urn:lsid:zoobank.org:act:06633F75-4809-4BB3-BDCB-6270795368D5
Coptotermes sp.:
urn:lsid:zoobank.org:pub:D6724B7F-F27A-47DC-A4FC-12859ECA0C71
Blattella germanica:
rn:lsid:zoobank.org:pub:1EA126BA-E9D2-4AA6-8202-26BA5B09B8AD
Locusta migratoria:
urn:lsid:zoobank.org:pub:D792A09E-844A-412A-BFCA-5293F8388F8C
Periplaneta americana (Blatta americana):
urn:lsid:zoobank.org:act:95113A55-4C6D-4DC7-A0E5-620BACADFFE5
Apis mellifera:
urn:lsid:zoobank.org:act:9082C709-6347-4768-A0DC-27DC44400CB2
Bombyx mori (Phalaena (Bombyx) mori):
urn:lsid:zoobank.org:act:215466E3-E77F-46E9-8097-372837D7A375
Drosophila melanogaster:
urn:lsid:zoobank.org:act:5B39F0AA-270D-4AA8-B9A3-C36A3A265910
Like in other organisms, pathogens seem to be important drivers of evolution in social insects. On the one hand, social insects are a “desirable” target for pathogens as an insect colony represents a large source of many potential hosts, all with a similar genetic background. Thus, if pathogens manage to enter a colony, they can exploit many individuals. However, social insects are also well protected as they evolved “social immunity” (Traniello et al., 2002; Cremer et al., 2007), a repertoire of defensive mechanisms that work at the colony level. For instance, behavioral task division limits contact to potentially infected individuals and can lead to their eviction. Also molecular mechanisms of social immunity exist, for example indirect immunization of colony members (e.g., Traniello et al., 2002; Cremer et al., 2007; Masri and Cremer, 2014) or impregnation of the nest walls with fungicidal compounds (Bulmer et al., 2009; Rosengaus et al., 2011). Thus, social immunity can be considered a selected emergent property of insect colonies where the whole is more than the sum of the individual parts (Rosengaus, personal communication). The evolution of social immunity aligns with the complexity of social organization, suggesting that selection pressure by pathogens could be a driver of complex social organization.
In termites, there is evidence for an association between selection pressure from pathogens, ecology, and social complexity. Although all termites are eusocial, the degree of worker altruism differs between termite lineages and aligns with ecology (Korb et al., 2012). Species of most early branching lineages of the termite phylogeny share a similar ecology called “one piece nesting” or “wood-dwelling” life type (Abe, 1987; Korb, 2007). Wood-dwelling termites nest in a single piece of wood that serves as food and shelter. “Workers” of wood-dwelling termites are developmentally totipotent immatures that become reproductives, and thus are sometimes considered “false” workers. In particular, species of the family Kalotermitidae display little brood care by false workers and form less complex societies, where the interactions are generally not altruistic but cooperative (Korb, 2007). Yet, there are differences in the degree of brood care between wood-dwelling species. Brood care appears to be malleable depending on nesting ecology (Korb et al., 2012). Brood care is negligible or absent in several dry wood termites such as Cryptotermes secundus (Kalotermitidae) that nest in sound dry wood. More intensive brood care (especially allogrooming) occurs in the dampwood termite (Archotermopsidae), Zootermopsis nevadensis, which nests in rotten, decaying wood (Korb et al., 2012). Brood care is also present in Zootermopsis angusticollis (Rosengaus and Traniello, 1993). Looking beyond termites having a wood-dwelling life type, brood care is more intensive in termite lineages that have altruistic workers with reduced developmental options (true workers) and complex social organization (Roisin and Korb, 2011) like Macrotermes. These species are central-place foragers (multiple-pieces nesting, see Abe, 1987; Korb, 2007) with nests separated from the foraging ground.
The increase in social complexity and changes in ecology seem to align with the degree of immune challenge in two ways. First, wood-dwelling termites are confined within their nests, and hence it seems reasonable to assume that they are not exposed to microbial challenges as frequently as foraging termites. Second, within the group of wood-dwelling species, the nests of termites that live in sound drywood can be assumed to be microbe- and pathogen-poor when compared to nests of species that live in decaying dampwood. Rosengaus et al. (2003) provide evidence for the latter if we assume that pathogen pressure can be extrapolated from the cultivable fungal and bacterial loads that these authors measured. In fact, the dampwood termite, Z. angusticollis that was investigated in Rosengaus et al. (2003) seems to have huge constitutive investments in immune defense at different levels. This includes the individual as well as the colony level, potentially with socially acquired immunity (Rosengaus et al., 1999, 2011; Traniello et al., 2002; Cole and Rosengaus, 2019). The investment in immunity in dampwood termites of the genus Zootermopsis is also visible at the genome level. In the genome of Z. nevadensis, six copies of Gram-negative binding proteins (GNBPs) were found, more than in many other insect species (Terrapon et al., 2014). GNBPs can serve as microbial detectors and effectors alike. Four of these presumably termite-specific genes were also found in the genome of the fungus-growing termite Macrotermes natalensis (Termitidae, see Poulsen et al., 2014; Korb et al., 2015). M. natalensis is a foraging species with intensive brood care and a complex social organization. For other central-place foraging termites, such as several Australian Nasutitermes species (Termitidae), which also have a complex social organization, as well as for Reticulitermes, GNBPs had previously been shown to be under positive selection (Bulmer and Crozier, 2006). Additionally, selection on three immune genes (GNBP1, GNBP2, and Relish) differed between Australian Nasutitermitinae. The rate of adaptive evolution of GNBP2 and Relish were increased during the transition from feeding on dry grass stored in epigeal nests to feeding on decaying wood (Bulmer and Crozier, 2006; Rosengaus et al., 2011), providing additional evidence for immune challenges that are specific to species that live in decaying wood.
Based on these results, we hypothesized that selective pressure on immune defense genes (IGs) differs across termites depending on their life type (Korb et al., 2015). We tested the hypothesis that wood-dwelling termites, which do not leave the nest to forage outside show relaxed selection on IGs and fewer signs of positive selection compared to soil-foraging species. Additionally, we tested whether within the wood-dwelling life type, the dampwood termite Z. nevadensis had stronger signs of selection than other wood-dwellers that nest in sound wood.
In order to test for differences in selective forces acting on IGs between wood-dwelling and foraging species, we analyzed a set of 81 previously identified single-copy IGs (see section “Materials and Methods”) in eight termite species (published data see Misof et al., 2014; Poulsen et al., 2014; Terrapon et al., 2014; Harrison et al., 2018; Evangelista et al., 2019; data sources are provided in Supplementary Table S1). Four of the species are wood-dwelling species: C. secundus, Incisitermes marginipennis, Prorhinotermes simplex, and Z. nevadensis. The remaining four species are foraging species: Mastotermes darwiniensis, Reticulitermes santonensis (i.e., Reticulitermes flavipes)Coptotermes sp. and M. natalensis. These were analyzed in a phylogenetic framework of 22 species (one mayfly, 12 polyneopteran insects including above listed termites and their closest relatives Cryptocercidae, two paraneopteran, and six holometabolous insects) spanning winged insects to increase the statistical power of lineage specific tests for selection.
The species tree was inferred from a supermatrix including 1,178 single-copy orthologs (SCOs) and spanning an alignment length of 555,906 amino acid positions (partition coverage 100%, site-completeness score Ca = 74.61%, see Supplementary Material and Supplementary Figure S1). Termites were monophyletic with Cryptocercus as sister group, consistent with earlier work (e.g., Lo et al., 2000; Klass and Meier, 2006; Inward et al., 2007; Legendre et al., 2008). Phylogenetic relationships within termites are largely consistent with Evangelista et al. (2019) and are statistically maximally supported (Figure 1). Consistent with earlier work (e.g., Legendre et al., 2008), neither wood-dwellers nor foragers constitute monophyletic groups, confirming that several independent switches in life type were included in our analyses. More details on phylogenetic analyses are provided in the Supplementary Material.
Figure 1. Phylogenetic relationships. Best ML species tree (out of 50 trees) inferred from a super alignment of 1,178 single-copy orthologs (SCOs) with 555,906 amino acid positions (schematized for Holometabola and Paraneoptera, all ML trees had the same topology). Statistical bootstrap support was derived from 200 replicates. The tree was rooted with the mayfly Ephemera danica (the full tree is provided with the Supplementary Files on DRYAD). Color code: brown indicates wood-dwelling species (wd), green indicates foraging species (f). TR, derived from transcriptome assemblies (Misof et al., 2014; Evangelista et al., 2019); OGS, derived from official gene sets (Supplementary Table S1). The symbol “∗” indicates reference species whose OGS was used to create the ortholog set. Pictograms were kindly provided by H. Pohl, Jena.
Between 13 and 78 SCOs of IGs per species were included in the analyses (Table 1). We found no evidence for positive selection on the IGs (Table 2 and Supplementary Table S2).
Table 2. Summarized results of BUSTED analyses of single-copy IGs for each termite species tested against all other species in the alignment.
Next, we tested the hypothesis that selection on the IGs of wood-dwelling species is relaxed compared to foragers. We found 47 cases of significantly relaxed selection across all termite species analyzed (Table 1 and Supplementary Table S3, P < 0.05, FDR < 0.2). There was no evidence for a difference in the number of IGs under relaxed selection between the life types (generalized linear mixed effects model with binomial error distribution: df = 7, z = 0.096, P = 0.92). There was also no evidence for differences between species (generalized linear model assuming binomial error distribution: df = 7, z = −1.75–0, P = 0.08−1, ranges of z and P are for the different species). Because changes in the selection intensity on IGs could be obscured by genome-wide differences in selective constraint, it is important to test these hypotheses against the genomic background. To this end, we generated sets of background genes (BGs) that consisted of genes matching the GC-content and sequence length of each IG for each species (see section “Materials and Methods”). The number of IGs under relaxed selection did not differ significantly from that expected from the analysis of the BGs for any of the species [see 95% confidence intervals (CIs) for BG sets in Table 1]. In order to take genome-wide effects of selective constraint into account, when comparing selection intensity between wood-dwellers and foragers, (i) we calculated the ratio of genes under significantly relaxed selection between wood-dwellers and foragers for IGs and (ii) compared this ratio to that expected from BGs (see section “Materials and Methods”). If selection is relaxed specifically in the IGs of wood-dwellers relative to their genomic background, we expect the ratio of the number of significant genes under relaxed selection between wood-dwellers and foragers to be larger for the IGs than for the BGs. However, the ratio of the number of IGs under relaxed selection between wood-dwellers and foragers did not differ significantly from the expectation derived from BGs (Figure 2A), supporting the view that patterns of relaxed selection on IGs follow genome-wide trends in selective constraint.
Figure 2. Comparison of selection on immune genes to sets of genes that represent the genomic background. Relaxed selection on IGs does not differ from the genomic background. Red bar, immune genes (IGs); black dots, 100 sets of background genes (BGs). Curves represent smoothed point density. (A) The ratio of the number of genes under significantly relaxed selection between wood-dwellers and foragers (Rrelax). (B) The relative intensity of selection (Rk) as measured by the ratio of median k () between wood-dwellers and foragers.
Because we did not find any evidence for an increase in the number of IGs under significantly relaxed selection in wood-dwellers, we reasoned that a putative signal of relaxation of selective constraint might be more diffuse and only become visible as a general trend over all IGs investigated. In order to capture such more general trends, we assessed potential differences in k, a measure for the intensity of selection, for all IGs between life types. k did not differ significantly between species (Kruskal–Wallis test: df = 7, X 2 = 3.95, n = 322, P = 0.79, for n per species see Table 1) nor was it lower for wood-dwellers (Mann–Whitney U-test, one-sided: U = 11,690, n = 322, P = 0.41). This indicated similar selection intensity on IGs for wood-dwellers and foragers. For the comparison of k between life types it is, as above, important to take the selective constraint on the genomic background into account. k for the IGs did not differ significantly from k for sets of BGs for any of the species investigated (Table 1). Following the same rationale as above, we used the ratio of medians of k between wood-dwellers and foragers as a test statistic. This ratio can be interpreted as the relative intensity of selection between wood-dwellers and foragers. We found that the relative intensity of selection between wood-dwellers and foragers on IGs matched that of the BG sets (Figure 2B), again suggesting that the IGs follow genome-wide trends of selective constraint.
Finally, we hypothesized that our results might be affected by the particular selection pressures that act on Z. nevadensis, which is a wood-dwelling species, but lives in dampwood nests. Nests in dampwood have a high microbial loads, as has been shown for Z. angusticollis (Rosengaus et al., 2003). Hence, selection would be expected to be stronger on Z. nevadensis IGs than on IGs in the other wood-dwellers, resulting in a smaller fraction of genes under significantly relaxed selection in Z. nevadensis. We found no evidence for this hypothesis (generalized linear model assuming binomial error distribution: df = 3, z = 1.73, P = 0.084). The overall intensity of selection on IGs (k) also did not differ significantly between Z. nevadensis and the other wood-dwellers (Mann–Whitney U-test, U = 5,471, n = 215, P = 0.77). Similarly, it could be argued that the assumption of relaxed selection only holds for the dry wood-dwellers C. secundus and I. marginipennis, assuming that only dry wood is a truly pathogen poor substrate. We could not find a difference in the number of genes under relaxed selection between the dry wood-dwellers and the other species (generalized linear model with binomial error distribution: df = 7, z = 0.048, P = 0.96) nor for the intensity of selection over all IGs as measured by k (Mann–Whitney U-test: U = 10,538, n = 322, P = 0.37).
To our surprise, we observed that median k for IGs was smaller than one for seven of the eight investigated termite species (Table 1), indicating an overall relaxation of selection (Mann–Whitney U-test: U = 20,958, n = 322, P < 0.01). This signal was not IG specific: k for the sets of BGs was also significantly smaller than one for all species (P = 4 × 10e−18-4.8 × 10e−5), suggesting genome-wide relaxation of selection on the termite lineages compared to the background branches of the phylogeny.
In this study we combined recent genomic and transcriptomic resources to test whether termite ecology, in particular exposure to pathogens, might affect the evolution of immune genes. Surprisingly, and in contrast to studies from Drosophila (Clark et al., 2007; Sackton et al., 2007; Hill et al., 2019), we could not find evidence for positive selection on the IGs for eight termite species. We extended our analyses, employing recently developed tests to explicitly assess relaxed selection (Wertheim et al., 2015), revealing 47 cases of significantly relaxed selection in IGs.
We expected that the intensity of selection would differ between termites of the wood-dwelling and foraging life types, as foraging termites are assumed to experience higher selection pressure from pathogens due to higher exposure. Contrary to our expectation, we did not detect an effect of life type on signs of selection for immune genes. Neither did wood-dwelling species differ from the soil foraging species, nor did the dampwood termite Z. nevadensis differ from the other wood-dwelling termites. A possible explanation may be that IGs that occur in multiple copies in at least one of the species that we analyzed contribute to adaptation to selection pressure from pathogens. Such multi-copy genes were excluded from our analyses. Furthermore, recent selective sweeps, as described for termicin in Reticulitermes (Bulmer et al., 2010), are difficult to detect with our methodology. For the detection of recent selective events, comprehensive polymorphism data would be required. Also, social mechanisms such as the exclusion of infested individuals and the impregnation of the nest walls with fungicidal compounds may protect efficiently against pathogens entering a colony or infecting individuals, thus buffering selection pressure on IGs (Traniello et al., 2002; Cremer et al., 2007; Bulmer et al., 2009; Rosengaus et al., 2011; Masri and Cremer, 2014). Another possible explanation for similar selection pressure on IGs between wood-dwelling and foraging species is that both harbor complex gut microbial communities that are essential for termite survival (Waidele et al., 2017), and perform, in principle, similar functions in lignocellulose digestion and nitrogen acquisition across several of the species that we analyzed (Waidele et al., 2019). It seems reasonable to assume that the immune system is likely to play a role in modulating these communities in wood-dwellers and foragers alike resulting in constant selection pressure. Our results support the results of a study in ants (Roux et al., 2014) that spanned a similar evolutionary time frame and number of focal species. These authors also found no evidence for a relaxation of selective constraint specific to IGs that could be related to social immunity. However, the authors found several instances of positively selected genes showing that it is in principle possible to detect positive selection with dn/ds based methods in a similar setup. Harrison et al. (2018) were able to pinpoint several selected codons using genome sequences from Blattella germanica and C. secundus, again spanning similar divergence times. In general, BUSTED is more powerful than other methods to detect positive selection because it has decent power to detect not only pervasive, but also episodic selection, while being fairly independent from evolutionary divergence times (Murrell et al., 2015).
We applied state-of-the-art methods (Pond et al., 2005; Murrell et al., 2015; Wertheim et al., 2015) and carefully controlled our study by analyzing BGs that matched IGs in GC-content and sequence length (see section “Materials and Methods”). We also combined, to our knowledge, the largest data set of termite genomic and transcriptomic resources for our study that has been used in that context so far. Nonetheless, our study might lack statistical power: First, we have relatively few species for life type comparisons (4 vs. 4). Second, for five of these species (M. darwiniensis, I. marginipennis, R. santonensis, P. simulans, and Coptotermes sp.) only transcriptome data are available. Naturally, the number of genes under significantly relaxed selection is lower for the species for which only transcriptome data are available, as fewer genes could be annotated and investigated in the transcriptome data (Tables 1, 3 and Supplementary Table S4). However, potential biases in data availability were taken into account in the procedure to sample sets of matching BGs. Note that we did not apply the group-based approach of the BUSTED and RELAX tests that might have increased power to detect selection or its relaxation. This was due to fragmented data coverage of the species representing both life types. This fragmented coverage for many genes would have led to only a limited gain in power. Furthermore, the careful selection of BGs would have become infeasible because too few BGs matched the species composition in the group-based tests on IGs. We are hopeful that the group-based approach will become more powerful in the future, when more termite genome data become available. Nonetheless, we were able to detect 47 instances of relaxed selection, showing that the RELAX approach can be powerful in a setup like ours. Because RELAX specifically tests for relaxed selection, while other studies often infer potentially relaxed selection indirectly by faster evolution in the absence of positive selection (Roux et al., 2014; Partha et al., 2017), we think it should be the preferred method.
Table 3. Number of analyzed IGs with HyPhy-BUSTED and RELAX and respective BG analyses with HyPhy-RELAX for each of the included termite species.
When taking the genomic background and the sampling procedure into account (Figure 2), our data provide no evidence that selection intensity on SCO IGs differs between life types. Do our results imply that selection pressure on immune genes is the same between termite life types? No, we cannot conclude this as our study excluded IGs that are not SCOs. For example, several GNBPs were excluded from our study because they seemed to be present in multiple copies in at least one termite species that we analyzed (Znev_03257, Znev_03259). Different copies can be caste-specifically expressed in termites as shown for Z. nevadensis (Terrapon et al., 2014), and for Reticulitermes speratus (Mitaka et al., 2017), and they may be under positive selection as indicated by a study on GNBPs for several foraging Nasutitermes species (Bulmer and Crozier, 2006). Note that the original hypothesis for a difference in immune defense between wood-dwelling and foraging termites considered specifically GNBPs and AMPs (Korb et al., 2015). Other GNBPs were excluded because they were restricted to only some of the lineages that we based our ortholog set on (Znev_03260, Znev_02878, and Znev_00933). Thus, more studies are warranted that test selection on genes that are lineage-specific or might occur in multiple copies. However, orthology is difficult to infer for multi-copy genes making the restriction to SCOs a standard procedure (e.g., Dowling et al., 2016; Pauli et al., 2016; Mitterboeck et al., 2017; Ran et al., 2018; Brandt et al., 2019; Hill et al., 2019). Thus, we restricted our analysis to SCOs because orthology is a basic assumption of the current methods that identify selection in a powerful phylogenetic framework [e.g., codeML implemented in PAML, Yang, 2007; HyPhy applying BUSTED, see Murrell et al. (2015), and RELAX, see Wertheim et al. (2015)].
Applying state-of-the-art methods to a comprehensive termite data set, for which genome or transcriptome data are currently available, we found no evidence for differences in selection on immune genes that correlate with termite life type. Our results suggest that the putative evolutionary response to differences in expected pathogen exposure can not be found in single-copy immune genes. Interestingly, we detected a signal of genome-wide relaxation of selective constraint in termites. We speculate that this could be related to their social organization that might lead to smaller effective population size (Romiguier et al., 2014; Rubenstein et al., 2019) because only the kings and queens reproduce, and hence contribute to the effective population size. In smaller populations, natural selection becomes less effective at purging deleterious mutations as well as at driving advantageous mutations to fixation (Ohta, 1973). This is equivalent to a relaxation of selection in smaller populations. Thus, small effective population sizes compared to the other insects in our study could have manifested as the genome-wide signal of relaxed selection that we observed in termites.
A comprehensive diagram summarized major analysis steps in Supplementary Figure S1.
As basis to identify SCOs, we designed an ortholog set from official gene sets (OGS) from available full (draft) genomes of four species: C. secundus, M. natalensis, Z. nevadensis, and B. germanica (Supplementary Table S1). The set of SCOs was inferred with the software OrthoFinder v.1.1.4 (Emms and Kelly, 2015) using default settings. As input, the OGS of respective species were downloaded from public databases as amino acid and nucleotide sequences. The OGS of C. secundus was kindly provided by the C. secundus consortium (via J. Korb) before it was published (Harrison et al., 2018). We only kept the longest isoform per orthologous group (OG). All OGs that included the amino acid Selenocysteine (U) were removed to avoid difficulties in downstream analyses as many software packages are not able to handle Selenocysteine. This was done using the package BioBundle [script isoformCleaner with boost 1.61.0 environment (Kemena, 2017, available from github1)]. SCOs inferred with OrthoFinder, were summarized with custom-made Python scripts (kindly provided by A. Faddeeva and L. Wissler, available upon request). This resulted in a set of 5,382 SCOs across the four selected species.
We included the four reference species that were used to create the ortholog set as well as genome and transcriptome data of 18 additional species in our analyses. Eight of the included species are termites: Coptotermes sp., I. marginipennis, M. darwiniensis, P. simplex, and R. santonensis (with published transcriptome data); C. secundus, Z. nevadensis, and M. natalensis (with published OGS), see Evangelista et al. (2019). Other included species were a representative of Cryptocercidae as it is supposed to be the sister group of termites (e.g., Lo et al., 2000; Inward et al., 2007), two other non-social cockroach species, and representatives from other polyneopteran, paraneopteran and holometabolous insects, and a mayfly as outgroup (Adams et al., 2000; Xia et al., 2004; Sinkins, 2007; Tribolium Genome Sequencing Consortium et al., 2008; Bonasio et al., 2010; International Aphid Genomics Consortium, 2010; Elsik et al., 2014; Misof et al., 2014; Poulsen et al., 2014; Terrapon et al., 2014; Wang et al., 2014; Mesquita et al., 2015; Pauli et al., 2016; Harrison et al., 2018; Evangelista et al., 2019; the full species list is provided in Supplementary Table S1). Access to transcriptome data (see Figure 1) was kindly granted by 1KITE before they were published, access to the OGS of the locust and the mayfly was granted by the i5K community.
The ortholog set was used as input for the assignment of putative SCOs (provided as Supplementary Files on DRYAD, https://doi.org/10.5061/dryad.j6q573n98. Inference and assignment of putative orthologs from genome and transcriptome data of the 18 species that were not included for generating the ortholog set was performed with OrthoGraph v.0.6.1 (Petersen et al., 2017). OrthoGraph is recommended to infer orthologs from transcriptome data for which no OGS are available (see Petersen et al., 2017). OrthoGraph analyses resulted in 5,366 SCOs that were identified in at least one species that was not used as reference species to create the ortholog set.
Individual SCOs were aligned at the amino acid level with MAFFT v7.310 using the L-INS-i algorithm (Katoh and Standley, 2013).
For inferring the species tree, we only kept those SCOs that were present in all 22 species. This resulted in 1,178 SCOs. Ambiguously aligned sections on the amino acid level were identified with Aliscore v2.2 (Misof and Misof, 2009; Kück et al., 2010) (settings: -r with all pairwise sequence comparisons, -e for gap-rich alignments, otherwise defaults) and masked with AliCUT v2.3 (Kück, 2011). Masked amino acid multiple sequence alignments (MSAs) were concatenated into a supermatrix (see also Supplementary Figure S2) with FASconCAT-G v.1.02 (Kück and Longo, 2014). We inferred phylogenetic relationships using a maximum-likelihood (ML) approach with IQTREE v1.5.4 (Nguyen et al., 2015; Chernomor et al., 2016). Statistical support was determined from 200 non-parametric, slow and thorough bootstrap replicates. We ensured bootstrap convergence with a posteriori bootstrap criteria (Pattengale et al., 2010) as implemented in RAxML (Stamatakis, 2014), v.8.2.11. The best ML tree, out of 50 inferred trees, which all showed an identical topology, was rooted with Ephemera danica using SeaView v.4.5.4 (Gouy et al., 2010; note that multiple tree viewers are not reliable, see Czech et al., 2017); trees were graphically edited with Inkscape (v.0.91)2. More details on the procedure of phylogenetic inference are provided in the Supplementary Material.
Methods to identify selection are sensitive to misalignments (Markova-Raina and Petrov, 2011; Privman et al., 2012). Therefore we performed extensive alignment clean-up. First, we identified and deleted badly aligned or gap-rich sequences on amino acid level with MaxAlign v1.1 (Gouveia-Oliveira et al., 2007). This procedure resulted in five SCOs with only one sequence which were excluded from further analyses. We subsequently compiled corresponding nucleotide (i.e., codon) MSAs with PAL2NAL (Suyama et al., 2006, v14.1, see Misof et al., 2014) using the 5,361 amino acid MSAs as blue-print. The nucleotide MSAs were then used for all following analyses. Second, we deleted all SCOs with less than four sequences (223 SCOs) leaving 5,138 SCOs. Third, we identified ambiguously aligned sections on amino acid level with Aliscore v2.2 (Misof and Misof, 2009; Kück et al., 2010) with the same settings as described for the species tree inference. Suggested sections were removed from the amino acid and correspondingly from the nucleotide MSAs with AliCUT v2.3 (Kück, 2011). Subsequent analyses were performed on the masked nucleotide MSAs. First, we classified 5,138 SCOs into 86 immune single-copy genes (IGs) and into the remaining 5,052 SCOs based on Supplementary Table S25 from Terrapon et al.2014, for Z. nevadensis) and Korb et al.2015, for Z. nevadensis and M. natalensis), see Supplementary Table S5. The 5,052 non-immune SCOs were used to generate gene sets from the genomic background, i.e., BGs that had similar GC-content and sequence length (see below) as the examined IGs. Note that from the 86 IGs (Supplementary Table S5) five IGs were excluded because there was no SCO fulfilling the criteria to serve as BG and these were not listed by Terrapon et al. (2014) or not reported by Korb et al. (2015). This left 81 IGs for analyses (detailed information are provided in the Supplementary Material).
To further reduce potential false positives that may originate from misalignments, we trimmed trailing ends of each MSA, i.e., each MSA started and ended with unambiguous nucleotides for all species. Because visual inspection of the trimmed MSAs still revealed putative misaligned nucleotides, we applied the GUIDe tree based AligNment ConfidencE approach (GUIDANCE) Guidance2 (Landan and Graur, 2008; Sela et al., 2015) version 2.02 using MAFFT as implemented alignment method on the trimmed MSAs (options: codon as sequence type, sequence cutoff = 0 and the default column cutoff = 0.93).
To test for evidence of positive selection we used BUSTED (Murrell et al., 2015) as implemented in the software package HyPhy (Pond et al., 2005). BUSTED uses a branch-site test for positive selection on entire genes in a foreground branch relative to the background branches in a phylogeny. A significant P-value means that at least one codon in the foreground branch has experienced at least an episode of positive selection. The high sensitivity of the method compared to tests from alternative packages (see e.g., Enard et al., 2016; Ebel et al., 2017; Venkat et al., 2018; Hill et al., 2019) and the option to define the foreground branches according to our research question made it perfectly suited for our study.
For inferring potential relaxation of selection, we used RELAX (Wertheim et al., 2015) as implemented in the software package HyPhy (Pond et al., 2005). RELAX has been designed to identify changes in the intensity of selection on a given protein-coding gene in a codon-based phylogenetic framework (see Wertheim et al., 2015). The basic expectation of RELAX is that under relaxed selection, the ω of sites under purifying and positive selection will move closer to neutrality. The change of ω for the selected sites relative to the background branches is quantified with the selection intensity parameter k, where
If parameter k is significantly larger than one, selection has been intensified along the test branches. If k is significantly smaller than one, selection has been relaxed.
We used BUSTED and RELAX as implemented in the software package HyPhy, version 2.4.0-alpha.2 (access: April, 2019). We performed BUSTED and RELAX for each gene in each termite species separately (focal species: foreground, remaining species in the alignment: background) and calculated false discovery rates (FDR) to correct for multiple testing. We then determined k for each of the termite species relative to all other species in the tree. We chose this species-wise analysis setup because we wanted to take species level differences in potential pathogen exposure into consideration. For example, Z. nevadensis that resides in dampwood might differ in microbial exposure from Cryptotermes and Incisitermes that reside in dry wood, which in turn might affect selection pressure on IGs. Furthermore, an effective selection of BGs was only feasible in the species-wise framework (see section “Discussion”). Results from the species-wise analyses were summarized by life type after the BUSTED and RELAX analyses.
To test whether or not signals of selection were specific to IGs or the consequence of genome-wide trends, we generated sets of BGs. To this end, we searched the 5,052 non-immune SCOs for genes that closely matched the GC-content (±5%) and the sequence length (±5%). Following this procedure, we generated lists of matching BGs for each IG and each species. From these lists, we randomly sampled 100 gene sets such that there was a matching BG for each IG that was analyzed in the respective species (e.g., for Z. nevadensis, 78 IGs were analyzed, thus each of the 100 sets of BGs contained therefore 78 BGs, see also Table 3. Lists of analyses BG that are similar in GC-content and sequence length of the IGs are provided for each species as Supplementary Files on DRYAD). We performed BUSTED and RELAX analyses for each termite species on all IGs and RELAX on the species’ respective BG set with default settings. The same cutoffs as for the IGs (P < 0.05, FDR < 0.2, k < 1) were applied to the BGs.
All analyses were performed on Linux Desktop PCs at the University of Freiburg, Germany and on the Linux HPC CSIRO Cluster Pearcey, Australia. Analyses results of all IGs are summarized in Supplementary Tables S2, S3; results of BGs are provided species-wise on DRYAD.
In order to assess potential differences in selection intensity on the IGs between species and life types, we summarized the RELAX results by counting the number of genes under significantly relaxed selection: genes with k < 1, P < 0.05, and FDR < 0.2 were considered. With our FDR cut-off, we followed the recommendation from Efron (2007) for genome-wide analyses. Potential differences were tested for statistical significance with generalized linear models with binomial error distribution using the functions glm and glmer from the lme4 R package [Bates et al., 2015, version 1.1-21 with R Core Team (2018), version 3.4.4]. The number of significant genes divided by the total number of genes analyzed was used as response variable. Species or life type were used as potential predictors. Varying sampling depths between species, as represented by the number of IGs analyzed per species, were taken into account as weights in the model. When comparing life types, species were treated as a random effect. See Supplementary File (RanalysisscriptfortermiteIGs.R on DRYAD, https://doi.org/10.5061/dryad.j6q573n98) for a detailed R analysis script with all models, commands and functions used.
We also analyzed parameter k to search for more diffuse trends in selection intensity that are distributed over the IGs so that individual IGs do not reach significance. According to its definition, k should map linearly on a logarithmic scale. However, we found six strong outliers on the logarithmic scale that were more than three standard deviations away from the mean [log (k) < −9, see Supplementary Table S3] that could make the analysis in a linear framework error prone. Visual inspection of the alignments underlying these extremely small values of k did not reveal any obvious misalignments that would justify their exclusion. Therefore, potential differences in k were assessed with non-parametric tests (Mann–Whitney U-test, Kruskal–Wallis test) that are robust to outliers.
Genome-wide trends in selection intensity can potentially obscure IG specific patterns or generate false positives. For example, changes in population size can affect the efficiency of both purifying and positive selection (Ohta, 1973) on a genome-wide scale. Population sizes might differ between species and life types in our study depending on reproductive rates and degrees of sociality. Therefore, it is essential to put the results for IGs into the context of the genomic background. To this end, we generated expected values for the number of significant genes and for k based on 100 sets of BGs (see above) per termite species, representing the genomic background. The median of k and 95% CIs from the BG-based distributions for each species were calculated with R (version 3.4.4), using the median and quantile functions with standard settings. In order to compare differences between life types while taking the genomic background into account, we calculated (i) the ratio of the number of genes that were significantly relaxed between wood-dwellers and foragers
and (ii) the ratio of median parameter k (, relative selection intensity):
These ratios were calculated for the IGs and the BGs. Then the ratio of the IGs was compared to their expectation from the BGs. Significant shifts in selection intensity that are specific to IGs should lead to shifts of Rrelax and Rki only for IGs. Thus, if there were IG specific patterns of relaxed selection, the ratios Rrelax and Rki for the IGs should represent extremes of the distribution of sets of BGs. Therefore, we only considered a signal as significantly specific for IGs if our test-statistics of the IGs were outside of the 95% CI calculated for the BGs. However, this was not the case in our study.
Supplementary Data (see Supplementary Material) used in this study can be found at the DRYAD digital repository, https://doi.org/10.5061/dryad.j6q573n98.
JK and FS conceived the study. KM, MS, and FS performed all analyses. FS, KM, and JK wrote the manuscript. All authors edited and approved the manuscript.
This project was supported by the German Science Foundation (DFG; KO1895/20-1, KO1895/20-2, STA 1154/2-1 Projektnummer 270882710). The article processing charge was funded by the German Research Foundation (DFG) and the University of Freiburg in the funding programme Open Access Publishing. Funders had no role in research design or decision to submit.
The authors declare that the research was conducted in the absence of any commercial or financial relationships that could be construed as a potential conflict of interest.
We acknowledge Bernhard Misof, Panagiotis Provataris, Coby Schal, Xavier Belles, Stephen Richards and the i5K community the usage of the official gene set of Ephemera danica, and Blattella germanica, and Xianhui Wang and Le Kang for access and usage of the official gene set of Locusta migratoria. We thank the 1KITE Dictyoptera group who granted us access to transcriptome assemblies before they were published. We thank David Enard (University of Arizona, United States), Dario Valenzano (MPI, Cologne, Germany), Ondrej Hlinka (CSIRO, Australia), Ryan Velazquez, and Sergej Pond for their useful input and help with analyses of the molecular sequence data using Guidance2 and HyPhy. KM thanks Thomas Pauli (University of Freiburg, Germany) for fruitful discussions and Hans Pohl (University of Jena, Germany), who kindly allowed the usage of several pictograms in Figure 1. We also thank the two reviewers for helpful comments on the manuscript.
The Supplementary Material for this article can be found online at: https://www.frontiersin.org/articles/10.3389/fevo.2020.00026/full#supplementary-material
Abe, T. (1987). “Evolution of life types in termites,” in Evolution and Coadaptation in Biotic Communities, eds S. Kawano, J. H. Connell, and T. Hidaka, (Tokyo: University of Tokyo press), 125–148.
Adams, M. D., Celniker, S. E., Holt, R. A., Evans, C. A., Gocayne, J. D., Amanatides, P. G., et al. (2000). The genome sequence of Drosophila melanogaster. Science 287, 2185–2195. doi: 10.1126/science.287.5461.2185
Bates, D., Maechler, M., Bolker, B., and Walker, S. (2015). Fitting linear mixed-effects models using lme4. J. Stat. Softw. 67, 1–48. doi: 10.18637/jss.v067.i01
Bonasio, R., Zhang, G., Ye, C., Mutti, N. S., Fang, X., Qin, N., et al. (2010). Genomic comparison of the ants Camponotus floridanus and Harpegnathos saltator. Science 329, 1068–1071. doi: 10.1126/science.1192428
Brandt, A., Bast, J., Scheu, S., Meusemann, K., Donath, A., Schütte, K., et al. (2019). No signal of deleterious mutation accumulation in conserved gene sequences of extant asexual hexapods. Sci. Rep. 9:5338. doi: 10.1038/s41598-019-41821-x
Bulmer, M. S., and Crozier, R. H. (2006). Variation in positive selection in termite GNBPs and Relish. Mol. Biol. Evol. 23, 317–326. doi: 10.1093/molbev/msj037
Bulmer, M. S., Bachelet, I., Raman, R., Rosengaus, R. B., and Sasisekharan, R. (2009). Targeting an antimicrobial effector function in insect immunity as a pest control strategy. Proc. Natl. Acad. Sci. U.S.A. 106, 12652–12657. doi: 10.1073/pnas.0904063106
Bulmer, M. S., Lay, F., and Hamilton, C. (2010). Adaptive evolution in subterranean termite antifungal peptides. Insect Mol. Biol. 19, 669–674. doi: 10.1111/j.1365-2583.2010.01023.x
Chernomor, O., von Haeseler, A., and Minh, B. Q. (2016). Terrace aware data structure for phylogenomic inference from supermatrices. Syst. Biol. 65, 997–1008. doi: 10.1093/sysbio/syw037
Clark, A. G., Eisen, M. B., Smith, D. R., Bergman, C. M., Oliver, B., Markow, T. A., et al. (2007). Evolution of genes and genomes on the Drosophila phylogeny. Nature 450, 203–218. doi: 10.1038/nature06341
Cole, E., and Rosengaus, R. (2019). Pathogenic dynamics during colony ontogeny reinforce potential drivers of termite eusociality: mate assistance and biparental care. Front. Ecol. Evol. 7:473. doi: 10.3389/fevo.2019.00473
Cremer, S., Armitage, S. A. O., and Schmid-Hempel, P. (2007). Social immunity. Curr. Biol. 17, R693–R702. doi: 10.1016/j.cub.2007.06.008
Czech, L., Huerta-Cepas, J., and Stamatakis, A. (2017). A critical review on the use of support values in tree viewers and bioinformatics toolkits. Mol. Biol. Evol. 34, 1535–1542. doi: 10.1093/molbev/msx055
Dowling, D., Pauli, T., Donath, A., Meusemann, K., Podsiadlowski, L., Petersen, M., et al. (2016). Phylogenetic origin and diversification of RNAi pathway genes in insects. Genome Biol. Evol. 8, 3784–3793. doi: 10.1093/gbe/evw281
Ebel, E. R., Telis, N., Venkataram, S., Petrov, D. A., and Enard, D. (2017). High rate of adaptation of mammalian proteins that interact with Plasmodium and related parasites. PLoS Genet. 13:e1007023. doi: 10.1371/journal.pgen.1007023
Efron, B. (2007). Size, power and false discovery rates. Ann. Statist. 35, 1351–1377. doi: 10.1214/009053606000001460
Elsik, G. C., Worley, K. C., Bennet, A. K., Beye, M., Camara, F., Childers, C. P., et al. (2014). Finding the missing honey bee genes: lessons learned from a genome upgrade. BMC Genomics 30:86. doi: 10.1186/1471-2164-15-86
Emms, D. M., and Kelly, S. (2015). OrthoFinder: solving fundamental biases in whole genome comparisons dramatically improves orthogroup inference accuracy. Genome Biol. 16:157. doi: 10.1186/s13059-015-0721-2
Enard, D., Cai, L., Gwennap, C., and Petrov, D. A. (2016). Viruses are a dominant driver of protein adaptation in mammals. eLife 5:e12469. doi: 10.7554/eLife.12469
Evangelista, D. A., Wipfler, B., Béthoux, O., Donath, A., Fujita, M., Kohli, M., et al. (2019). An integrative phylogenomic approach illuminates the evolutionary history of cockroaches and termites (Blattodea). Proc. R. Soc. B 286:20182076. doi: 10.1098/rspb.2018.2076
Gouveia-Oliveira, R., Sackett, P. W., and Pedersen, A. G. (2007). MaxAlign: maximizing usable data in an alignment. BMC Bioinformatics 8:312. doi: 10.1186/1471-2105-8-312
Gouy, M., Guindon, S., and Gascuel, O. (2010). SeaView version 4: a multiplatform graphical user interface for sequence alignment and phylogenetic tree building. Mol. Biol. Evol. 27, 221–224. doi: 10.1093/molbev/msp259
Harrison, M. C., Jongepier, E., Robertson, H. M., Arning, N., Bitard-Feildel, T., Chao, H., et al. (2018). Hemimetabolous genomes reveal molecular basis of termite eusociality. Nat. Ecol. Evol. 2, 557–566. doi: 10.1038/s41559-017-0459-1
Hill, T., Koseva, B. S., and Unckless, R. L. (2019). The genome of Drosophila innubila reveals lineage-specific patterns of selection in immune genes. Mol. Biol. Evol. 36, 1405–1417. doi: 10.1093/molbev/msz059
International Aphid Genomics Consortium (2010). Genome sequence of the pea aphid Acyrthosiphon pisum. PLoS Biol. 8:e1000313. doi: 10.1371/journal.pbio.1000313
Inward, D., Beccaloni, G., and Eggleton, P. (2007). Death of an order: a comprehensive molecular phylogenetic study confirms that termites are eusocial cockroaches. Biol. Lett. 3, 331–335. doi: 10.1098/rsbl.2007.0102
Katoh, K., and Standley, D. M. (2013). MAFFT multiple sequence alignment software version 7: improvements in performance and usability. Mol. Biol. Evol. 30, 772–780.
Kemena, C. (2017). BioBundle. Available from https://github.com/CarstenK/BioBundle. doi: 10.1093/molbev/mst010 (accessed February 18, 2017).
Klass, K.-D., and Meier, R. (2006). A phylogenetic analysis of Dictyoptera (Insecta) based on morphological characters. Entomol. Abh. 63, 3–50.
Korb, J., Buschmann, M., Schafberg, S., Liebig, J., and Bagneres, A. G. (2012). Brood care and social evolution in termites. Proc. R. Soc. B 279, 2662–2671. doi: 10.1098/rspb.2011.2639
Korb, J., Poulsen, M., Hu, H., Li, C., Boomsma, J. J., Zhang, G., et al. (2015). A genomic comparison of two termites with different social complexity. Front. Genet. 6:e9. doi: 10.3389/fgene.2015.00009
Kück, P. (2011). AliCUT v. 2.3. Available from https://github.com/PatrickKueck/AliCUT (accessed October 2, 2016).
Kück, P., and Longo, G. C. (2014). FASconCAT-G: extensive functions for multiple sequence alignment preparations concerning phylogenetic studies. Front. Zool. 11:81. doi: 10.1186/s12983-014-0081-x
Kück, P., Meusemann, K., Dambach, J., Thormann, B., von Reumont, B. M., Wägele, J. W., et al. (2010). Parametric and non-parametric masking of randomness in sequence alignments can be improved and leads to better resolved trees. Front. Zool. 7:10. doi: 10.1186/1742-9994-7-10
Landan, G., and Graur, D. (2008). Local reliability measures from sets of co-optimal multiple sequence alignments. Pac. Symp. Biocomput. 13, 15–24.
Legendre, F., Whiting, M. F., Bordereau, C., Cancello, E. M., Evans, T. A., and Grandcolas, P. (2008). The phylogeny of termites (Dictyoptera: Isoptera) based on mitochondrial and nuclear markers: implications for the evolution of the worker and pseudergate castes, and foraging behaviors. Mol. Phylogenet. Evol. 48, 615–627. doi: 10.1016/j.ympev.2008.04.01
Lo, N., Tokuda, G., Watanabe, H., Rose, H., Slaytor, M., Maekawa, K., et al. (2000). Evidence from multiple gene sequences indicates that termites evolved from wood-feeding cockroaches. Curr. Biol. 10, 801–804. doi: 10.1016/s0960-9822(00)00561-3
Markova-Raina, P., and Petrov, D. (2011). High sensitivity to aligner and high rate of false positives in the estimates of positive selection in the 12 Drosophila genomes. Genome Res. 21, 863–874. doi: 10.1101/gr.115949.110
Masri, L., and Cremer, S. (2014). Individual and social immunisation insects. Trends Immunol. 35, 471–482. doi: 10.1016/j.it.2014.08.005
Mesquita, R. D., Vionette-Amaral, R. J., Lowenberger, C., Rivera-Pomar, R., Monteiro, F. A., Minx, P., et al. (2015). Genome of Rhodnius prolixus, an insect vector of Chagas disease, reveals unique adaptations to hematophagy and parasite infection. Proc. Natl. Acad. Sci. U.S.A. 112, 14936–14941. doi: 10.1073/pnas.1506226112
Misof, B., and Misof, K. (2009). A Monte Carlo approach successfully identifies randomness in multiple sequence alignments: a more objective means of data exclusion. Syst. Biol. 58, 21–34. doi: 10.1093/sysbio/syp006
Misof, B., Liu, S., Meusemann, K., Peters, R. S., Donath, A., Mayer, C., et al. (2014). Phylogenomics resolves the timing and pattern of insect evolution. Science 346, 763–767. doi: 10.1126/science.1257570
Mitaka, Y., Kobayashi, K., and Matsuura, K. (2017). Caste-, sex-, and age-dependent expression of immune-related genes in a Japanese subterranean termite, Reticulitermes speratus. PLoS One 12:e0175417. doi: 10.1371/journal.pone.0175417
Mitterboeck, T. F., Liu, S., Adamowicz, S. J., Fu, J., Zhang, R., Song, W., et al. (2017). Positive and relaxed selection associated with flight evolution and loss in insect transcriptomes. Gigascience 10, 1–14. doi: 10.1093/gigascience/gix073
Murrell, B., Weaver, S., Smith, M. D., Wertheim, J. O., Murrell, S., Aylward, A., et al. (2015). Gene-wide identification of episodic selection. Mol. Biol. Evol. 32, 1365–1371. doi: 10.1093/molbev/msv035
Nguyen, L. T., Schmidt, H. A., von Haeseler, A., and Minh, B. Q. (2015). IQ-TREE: a fast and effective stochastic algorithm for estimating maximum-likelihood phylogenies. Mol. Biol. Evol. 32, 268–274. doi: 10.1093/molbev/msu300
Ohta, T. (1973). Slightly deleterious mutant substitutions in evolution. Nature 246, 96–98. doi: 10.1038/246096a0
Partha, R., Chauhan, B. K., Ferreira, Z., Robinson, J. D., Lathrop, K., Nischal, K. K., et al. (2017). Subterranean mammals show convergent regression in ocular genes and enhancers, along with adaptation to tunneling. eLife 6:e25884. doi: 10.7554/eLife.25884
Pattengale, N. D., Alipour, M., Bininda-Emonds, O. R., Moret, B. M., and Stamatakis, A. (2010). How many bootstrap replicates are necessary? J. Comput. Biol. 17, 337–354. doi: 10.1089/cmb.2009.0179
Pauli, T., Vedder, L., Dowling, D., Petersen, M., Meusemann, K., Donath, A., et al. (2016). Transcriptomic data from panarthropods shed new light on the evolution of insulator binding proteins in insects. BMC Genomics 17:861. doi: 10.1186/s12864-016-3205-1
Petersen, M., Meusemann, K., Donath, A., Dowling, D., Liu, S., Peters, R. S., et al. (2017). Orthograph: a versatile tool for mapping coding nucleotide sequences to clusters of orthologous genes. BMC Bioinformatics 18:111. doi: 10.1186/s12859-017-1529-8
Pond, S. L. K., Frost, S. D. W., and Muse, S. V. (2005). HyPhy: hypothesis testing using phylogenies. Bioinformatics 21, 676–679. doi: 10.1093/bioinformatics/bti079
Poulsen, M., Hu, H., Li, C., Chen, Z., Xu, L., Otani, S., et al. (2014). Complementary symbiont contributions to plant decomposition in a fungus-farming termite. Proc. Natl. Acad. Sci. U.S.A. 111, 14500–14505. doi: 10.1073/pnas.1319718111
Privman, E., Penn, O., and Pupko, T. (2012). Improving the performance of positive selection inference by filtering unreliable alignment regions. Mol. Biol. Evol. 29, 1–5. doi: 10.1093/molbev/msr177
R. Core Team (2018). R: A Language and Environment for Statistical Computing. Vienna: R Foundation for Statistical Computing.
Ran, J.-H., Shen, T.-T., Wang, M.-M., and Wang, X.-Q. (2018). Phylogenomics resolves the deep phylogeny of seed plants and indicates partial convergent or homoplastic evolution between Gnetales and angiosperms. Biol. Sci. 285:20181012. doi: 10.1098/rspb.2018.1012
Roisin, Y., and Korb, J. (2011). “Social organization and status of workers in termites,” in Biology of Termites: a Modern Synthesis, eds D. E. Bignell, Y. Roisin, and N. Lo, (Dordrecht: Springer), 133–164. doi: 10.1007/978-90-481-3977-4_6
Romiguier, J., Lourenco, J., Gayral, P., Faivre, N., Weinert, L. A., Ravel, S., et al. (2014). Population genomics of eusocial insects: the costs of a vertebrate-like effective population size. J. Evol. Biol. 27, 593–603. doi: 10.1111/jeb.12331
Rosengaus, R. B., and Traniello, J. F. (1993). Temporal polyethism in incipient colonies of the primitive termite Zootermopsis angusticollis: a single multi-age caste. J. Insect Behav. 6, 237–252. doi: 10.1007/BF01051507
Rosengaus, R. B., Moustakas, J. E., Calleri, D. V., and Traniello, J. F. (2003). Nesting ecology and cuticular microbial loads in dampwood (Zootermopsis angusticollis) and drywood termites (Incisitermes minor, I. schwarzi, Cryptotermes cavifrons). J. Insect Sci. 3:31. doi: 10.1093/jis/3.1.31
Rosengaus, R. B., Traniello, J. F., and Bulmer, M. S. (2011). “Ecology, behavior and evolution of disease resistance in termites,” in Biology of Termites: A Modern Synthesis, eds D. E. Bignell, Y. Roisin, and N. Lo, (Dordrecht: Springer), 165–192.
Rosengaus, R. B., Traniello, J. F., Chen, T., and Brown, J. J. (1999). Immunity in a social insect. Naturwissenschaften 86, 588–591. doi: 10.1007/s001140050
Roux, J., Privman, E., Moretti, S., Daub, J. T., Robinson-Rechavi, M., and Keller, L. (2014). Patterns of positive selection in seven ant genomes. Mol. Biol. Evol. 31, 1661–1685. doi: 10.1093/molbev/msu141
Rubenstein, D. R., Ågren, J. A., Carbone, L., Elde, N. C., Hoekstra, H. E., Kapheim, K. M., et al. (2019). Coevolution of genome architecture and social behavior. Trends Ecol. Evol. 34, 844–855. doi: 10.1016/j.tree.2019.04.011
Sackton, T. B., Lazzaro, B. P., Schlenke, T. A., Evans, J. D., Hultmark, D., and Clark, A. G. (2007). Dynamic evolution in the innate immune system in Drosophila. Nat. Genet. 39, 1461–1468. doi: 10.1038/ng.2007.60
Sela, I., Ashkenazy, H., Katoh, K., and Pupko, T. (2015). GUIDANCE2: accurate detection of unreliable alignment regions accounting for the uncertainty of multiple parameters. Nucleic Acids Res. 43, W7–W14. doi: 10.1093/nar/gkq443
Sinkins, S. (2007). Genome sequence of Aedes aegypti, a major arbovirus vector. Science 316, 1718–1723. doi: 10.1126/science.1138878
Stamatakis, A. (2014). RAxML version 8: a tool for phylogenetic analysis and post-analysis of large phylogenies. Bioinformatics 30, 1312–1313. doi: 10.1093/bioinformatics/btu033
Suyama, M., Torrents, D., and Bork, P. (2006). PAL2NAL: robust conversion of protein sequence alignments into the corresponding codon alignments. Nucleic Acids Res. 34, W609–W612. doi: 10.1093/nar/gkl315
Terrapon, N., Li, C., Robertson, H. M., Ji, L., Meng, X., Booth, W., et al. (2014). Molecular traces of alternative social organization in a termite genome. Nat. Commun. 5:3636. doi: 10.1038/ncomms4636
Traniello, J. F. A., Rosengaus, R. B., and Savoie, K. (2002). The development of immunity in a social insect: evidence for the group facilitation of disease resistance. Proc. Natl. Acad. Sci. U.S.A. 99, 6838–6842. doi: 10.1073/pnas.102176599
Tribolium Genome Sequencing Consortium S., Gibbs, R. A., Weinstock, G. M., Brown, S. J., and Denell, R. (2008). The genome of the model beetle and pest Tribolium castaneum. Nature 452, 949–955. doi: 10.1038/nature06784
Venkat, A., Hahn, M. W., and Thornton, J. W. (2018). Multinucleotide mutations cause false inferences of lineage-specific positive selection. Nat. Ecol. Evol. 2, 1280–1288. doi: 10.1038/s41559-018-0584-5
Waidele, L., Korb, J., Voolstra, C. R., Dedeine, F., and Staubach, F. (2019). Ecological specificity of the metagenome in a set of lower termite species supports contribution of the microbiome to adaptation of the host. Anim. Microbiome 1:13. doi: 10.1186/s42523-019-0014-2
Waidele, L., Korb, J., Voolstra, C. R., Künzel, S., Dedeine, F., and Staubach, F. (2017). Differential ecological specificity of protist and bacterial microbiomes across a set of termite species. Front. Microbiol. 8:2518. doi: 10.3389/fmicb.2017.02518
Wang, X., Fang, X., Yang, P., Jiang, X., Jiang, F., Zhao, D., et al. (2014). The locust genome provides insight into swarm formation and long-distance flight. Nat. Commun. 5:2957. doi: 10.1038/ncomms3957
Wertheim, J. O., Murrell, B., Smith, M. D., Pond, S. L.-K., and Scheffler, K. (2015). RELAX: detecting relaxed selection in a phylogenetic framework. Mol. Biol. Evol. 32, 820–832. doi: 10.1093/molbev/msu400
Xia, Q., Zhou, Z., Lu, C., Cheng, D., Dai, F., Li, B., et al. (2004). A draft sequence for the genome of the domesticated silkworm (Bombyx mori). Science 306, 1937–1940. doi: 10.1126/science.1102210
Keywords: immunity, social insects, termites, selection, comparative genomics
Citation: Meusemann K, Korb J, Schughart M and Staubach F (2020) No Evidence for Single-Copy Immune-Gene Specific Signals of Selection in Termites. Front. Ecol. Evol. 8:26. doi: 10.3389/fevo.2020.00026
Received: 07 August 2019; Accepted: 29 January 2020;
Published: 26 February 2020.
Edited by:
Peter H. W. Biedermann, Julius Maximilian University of Würzburg, GermanyReviewed by:
Mark Bulmer, Towson University, United StatesCopyright © 2020 Meusemann, Korb, Schughart and Staubach. This is an open-access article distributed under the terms of the Creative Commons Attribution License (CC BY). The use, distribution or reproduction in other forums is permitted, provided the original author(s) and the copyright owner(s) are credited and that the original publication in this journal is cited, in accordance with accepted academic practice. No use, distribution or reproduction is permitted which does not comply with these terms.
*Correspondence: Fabian Staubach, ZmFiaWFuLnN0YXViYWNoQGJpb2xvZ2llLnVuaS1mcmVpYnVyZy5kZQ==
Disclaimer: All claims expressed in this article are solely those of the authors and do not necessarily represent those of their affiliated organizations, or those of the publisher, the editors and the reviewers. Any product that may be evaluated in this article or claim that may be made by its manufacturer is not guaranteed or endorsed by the publisher.
Research integrity at Frontiers
Learn more about the work of our research integrity team to safeguard the quality of each article we publish.