- 1Nelson Institute for Environmental Studies, University of Wisconsin, Madison, WI, United States
- 2Biotechnical Faculty, University of Ljubljana, Ljubljana, Slovenia
- 3Panthera, New York, NY, United States
- 4Desert Ecology Research Group, The University of Sydney, Sydney, NSW, Australia
Rapid, global changes, such as extinction and climate change, put a premium on evidence-based, environmental policies and interventions, including predator control efforts. Lack of solid scientific evidence precludes strong inference about responses of predators, people, and prey of both, to various types of predator control. Here we formulate two opposing hypotheses with possible underlying mechanisms and propose experiments to test four pairs of opposed predictions about responses of predators, domestic animals, and people in a coupled, dynamic system. We outline the design of a platinum-standard experiment, namely randomized, controlled experiment with cross-over design and multiple steps to blind measurement, analysis, and peer review to avoid pervasive biases. The gold-standard has been proven feasible in field experiments with predators and livestock, so we call for replicating that across the world on different methods of predator control, in addition to striving for an even higher standard that can improve reproducibility and reliability of the science of predator control.
Introduction
Rapid planetary environmental changes challenge humanity's capacity for wise decisions about preventing wildlife extinctions and climate change (Blumm and Wood, 2017; Chapron et al., 2017; Ceballos and Ehrlich, 2018). Without certainty about the functional effectiveness of interventions to prevent future threats followed by reasoned discrimination between alternatives, most human decisions about how to intervene rely on assumptions and beliefs (i.e., perceived effectiveness) rather than evidence. This challenge is apparent in predator control in livestock systems, where recent reviews are unanimous about how little strong evidence exists for the effectiveness of interventions (van Eeden et al., 2018). The same concern applies to other wildlife-livestock interactions, such as badger control as an intervention against zoonotic disease (Jenkins et al., 2010; Donnelly and Woodroffe, 2012; Vial and Donnelly, 2012; Bielby et al., 2016) and livestock damage by wild pigs and elephants (Rodriguez and Sampson, 2019) and might similarly apply to crop damage and attacks on humans by wildlife.
For millennia, some people have killed large predators in direct competition for food and space. That practice continues today to protect domestic animals and crops from predators, although predators are now recognized for playing major roles in sustaining diversity and improving ecosystem resilience (Estes et al., 2011). Humans are the major cause of mortality of terrestrial carnivores globally, including extirpation, several cases of extinction of species, and protracted risks of extinction despite endangered species protections (Woodroffe and Ginsberg, 1998; Chapron et al., 2014; Treves et al., 2017a). Predator control plays a major role in human-induced mortality.
Here we define predator control as any human actions, either lethal or non-lethal, intended to prevent predatory animals from posing threats to domestic animals or other human interests. We apply the term ‘control' (or treatment) to connote the intended management intervention, regardless of whether it proves effective. In particular, removal (usually lethal) as a form of predator control offers an important link to the global problems summarized above because intentional, legitimate, or illegal predator control has been the major component of human-caused mortality (Conradie and Piesse, 2013; Treves et al., 2017a), despite scant evidence worldwide for effectiveness of lethal methods to protect human interests and little of the available evidence provides strong inference (Treves et al., 2016; van Eeden et al., 2018). Removal methods provide an important heuristic for experimental tests of hypotheses about predator control.
The traditional hypothesis is that removing predators would protect human interests. For example, while it might seem obvious that killing a lion whose jaws are about to close on a goat would protect the goat, the effectiveness of most lethal action against predators is not so obvious. Perhaps, killing a predator returning to a carcass soon after predation might protect other livestock (Woodroffe et al., 2005), but experiments with such methods also show surprisingly high error rates (Sacks et al., 1999). Indeed, recent, independent research in several regions found killing wild animals could exacerbate future threats to human interests, e.g., cougars (Cooley et al., 2009a; Peebles et al., 2013), birds (Bauer et al., 2018; Beggs et al., 2019), and wolves (Santiago-Avila et al., 2018a) – without requiring us to delve into the unresolved controversy and contested evidence about wolves in the Northern Rocky Mountains, USA or in Southern Europe (Wielgus and Peebles, 2014; Bradley et al., 2015; Fernández-Gil et al., 2015; Imbert et al., 2016; Poudyal et al., 2016; Kompaniyets and Evans, 2017). The uncertainties about predator removal reflect the indirect application unlike the lion and the goat hypothetical above.
Predator control is often applied far from a domestic animal loss and long afterwards, or applied pre-emptively to predators that cross paths with a human. The functional effectiveness of these indirect actions for preventing future threats is unclear and often not directly measured. Indirect predator controls are not obviously functionally effective, just as many biomedical interventions are administered far from unhealthy tissues or many hours after an acute symptom is detected. Indeed, the analogy is even closer as indirect biomedical interventions, such as in vitro tests, animal trials, and even initial clinical trials on human subjects are not considered sufficient evidence to market a proposed treatment as a therapeutic (functionally effective) medicine. Therefore, as with biomedical research, the field of predator control needs the “gold-standard” of randomized, controlled experiment without biases, and such trials should be designed to detect any direction of effect, whether human interests become less or more susceptible in the treated condition after a predator control intervention.
Here we (1) describe unresolved questions and uncertainties connected with predator control to protect domestic animals mainly, but also relevant to other human interests; we do not address predator control to influence wild prey abundances. (2) We articulate two opposing hypotheses, each with four predictions. (3) We identify five forms of biases pervasive in the field of predator control. Finally, (4) we propose a design for a “platinum-standard” experiment that can elevate the strength of inference beyond the important gold-standard by adding cross-over design and multiple steps to blind measurement, analysis, and peer review. Our review of evidence for effectiveness, gaps in knowledge, and recommended practice is timely and important. It is timely because scientific evidence for effectiveness of predator controls are hotly contested in several regions of the world (see for example, the citations to wolves above) and important because the ongoing biodiversity crises demands that the majority of our investments be targeted quickly at effective interventions that protect both species and human interests if we wish to slow human-caused extirpations worldwide.
Five Unresolved Questions About Predator Control
Most scientists would agree that predation vanishes when zero predators are present, but there is substantial disagreement about what happens with removal of part of the predator population. For predators and other wildlife posing problems for people, there remain substantial uncertainties about the consequences of removal for survivors and subsequent generations, effects on sympatric species, and additive or compensatory responses in other mortality and reproductive factors (Cote and Sutherland, 1997; Vucetich, 2012; Borg et al., 2015; Creel et al., 2015; Bauer et al., 2018; Beggs et al., 2019). Uncertainty about the result of predator removal might propagate into uncertainty about its functional effectiveness for protecting human interests as we explain below. Resolving these uncertainties might improve our understanding of functional effectiveness of predator control, but also bears on ancillary issues of preserving wildlife (predators or otherwise), and the ethics and economics of domestic animal husbandry and wildlife management. Therefore, the platinum-standard experiment we recommend in the following section has the potential to advance our understanding of many of the following issues.
Do Survivors Prey on Domestic Animals at Similar Rates After Removals?
Since at least 1983, scientists have questioned whether predators that survive control operations pose fewer, the same, or more threats after removal of their conspecifics (Tompa, 1983; Haber, 1996). Related to this, the literature is unclear whether and how the response of survivors might differ from response to other mortality causes. In some cases, newcomers might kill more domestic animals than previous residents had killed because social networks might be disrupted, as reported in cougars (Cooley et al., 2009a,b; Peebles et al., 2013); or survivors might turn to domestic animals when their conspecifics have been removed (Imbert et al., 2016; Santiago-Avila et al., 2018a), and other “spill-over” effects (Santiago-Avila et al., 2018a). A number of correlational studies have reported such effects (Peebles et al., 2013; Fernández-Gil et al., 2015), including four papers from one site that have all been disputed without consensus on their resolution (Wielgus and Peebles, 2014; Bradley et al., 2015; Poudyal et al., 2016; Kompaniyets and Evans, 2017).
Among the contested and uncertain effects of predator control is the behavioral reaction of predators that are deterred from one human property. Do they simply move from one human property to another? Such displacement of predators might arise from non-lethal methods (e.g., some believe a wolf with a hunger for domestic animals continues searching for such prey after being deterred from its first effort), or from lethal methods (e.g., do surviving wolves discontinue hunting domestic animals, even after a pack-mate was killed? or do they redouble their efforts because a hunting team-mate was lost?). The latter uncertainty might be magnified or reduced by the method of removal, because the capability of survivors to “learn” from the removal must depend on the stimuli associated and the conspicuousness of the cause-and-effect. Resolving such issues would require stronger inference about individual behavior of predators and the short- and long-term reactions to predator control.
Do Surviving Predators Compensate for Vacancies by Altered Reproductive Rates?
Research on coyotes (Canis latrans) and black-backed jackals (C. mesomelas) indicates that human-caused mortality can generate compensatory reproduction that might augment the number of breeding packs and elevate the predator density, both of which might raise the risk for domestic animals (Knowlton et al., 1999; Minnie et al., 2016).
How Much Predation on Domestic Animals Is Compensatory?
Given that the mortality rates of domesticates from non-predatory causes is usually higher than from predators and predators may be attracted to sites with weak, ill, or morbid domestic animals under minimal supervision (Allen and Sparkes, 2001; Odden et al., 2002, 2008), one should expect that predation on domestic animals would be partly compensatory (killing animals doomed to die of other causes), rather than additive as it is often assumed (Treves and Santiago-Ávila, in press).
How Do Sympatric Species of Predators Respond to Removal of Competitor Species?
As early as 1958, observers noticed the removal of larger-bodied predators led to an increase in smaller-bodied animals, whose damages to crops and domestic animals were perceived as worse than those of the former larger wildlife (Newby and Brown, 1958). Ecologists have long understood that release from competition leads to prey switches, range shifts, and other flexible, behavioral responses by surviving predators. For a particularly relevant example in our context, mesopredator release has been substantiated repeatedly after the removal of a larger, dominant competitor (Prugh et al., 2009; Allen et al., 2016; Minnie et al., 2016; Krofel et al., 2017; Newsome et al., 2017).
Does One Source of Predator Removal Affect Other Sources of Predator Removal?
Human-caused mortality is the major source of mortality for large carnivores worldwide. Therefore, interactions between human causes of death are important to our understanding of the intended and unintended effects of predator removal, as are the effects of interventions meant to curb human causes of mortality. For example, poaching (illegal killing by people) was found to be the major cause of mortality in four endangered wolf populations of the USA, and unregulated killing was the major cause in one Alaskan sub-population (Adams et al., 2008; Treves et al., 2017a). Those studies also revealed that poaching was systematically under-estimated by traditional measures of risk and hazard (Treves et al., 2017a) or that mortality of marked animals differed from that of unmarked animals under legal, lethal management regimes (Schmidt et al., 2015; Treves et al., 2017c; Santiago-Ávila, 2019; Treves, 2019a). For a pertinent example, after wolf-killing had been legalized or made easier (liberalized), wolf population growth in two U.S. states slowed over and above the number of wolves killed (Chapron and Treves, 2016a,b), notwithstanding a lively debate (Chapron and Treves, 2017a,b; Olson et al., 2017; Pepin et al., 2017; Stien, 2017). Four separate lead authors studying different datasets about the same Wisconsin wolf control system have now inferred that poaching rates or intentions rose with liberalized wolf-killing policies (Browne-Nuñez et al., 2015; Hogberg et al., 2015; Chapron and Treves, 2017a,b). Also, disappearances of radio-collared wolves rose substantially when liberalized killing policies were in place, in a competing risks framework (Treves, 2019a) citing (Santiago-Ávila, 2019). Therefore, a possible consequence of predator removal to protect human interests might be an increase in apparently unrelated mortality rates.
The Opposing Hypotheses and Four Predictions
The first hypothesis, “Turning down the heat” proposes that more predators would attack more domestic animals. When humans remove predators, threats to human interests will diminish because (A0) human removal of predators reduces predator abundance; or (B0) surviving predators will be deterred from threatening human interests by sensing the loss of conspecifics was caused by humans. On the human side, incentives to remove predators will stay the same or decline, because (C0) people will correctly perceive the effects of predator removal; and (D0) legal removal will reduce incentives for illegal removal (poaching).
By contrast, the “Turning up the heat” hypothesis proposes that after predator removal, surviving predators will threaten human interests more than they would otherwise. Therefore, when humans remove predators, threats will stay the same or rise because (A1) newcomers will fill vacancies quickly in higher numbers than the residents they replaced or (B1) survivors and newcomers will struggle to survive or reproduce without relying on human property (e.g., predators would find and capture domestic animals more predictably or more safely than wild foods). On the human side, incentives to remove predators will rise, because perceived effectiveness rarely matches functional effectiveness so (C1) people will call for more predator-killing despite ineffective or counter-productive outcomes; and (D1) legal removal will promote poaching. Figure 1 displays four pairs of opposed predictions and the feedback loops each can trigger with more detail presented in Table 1.
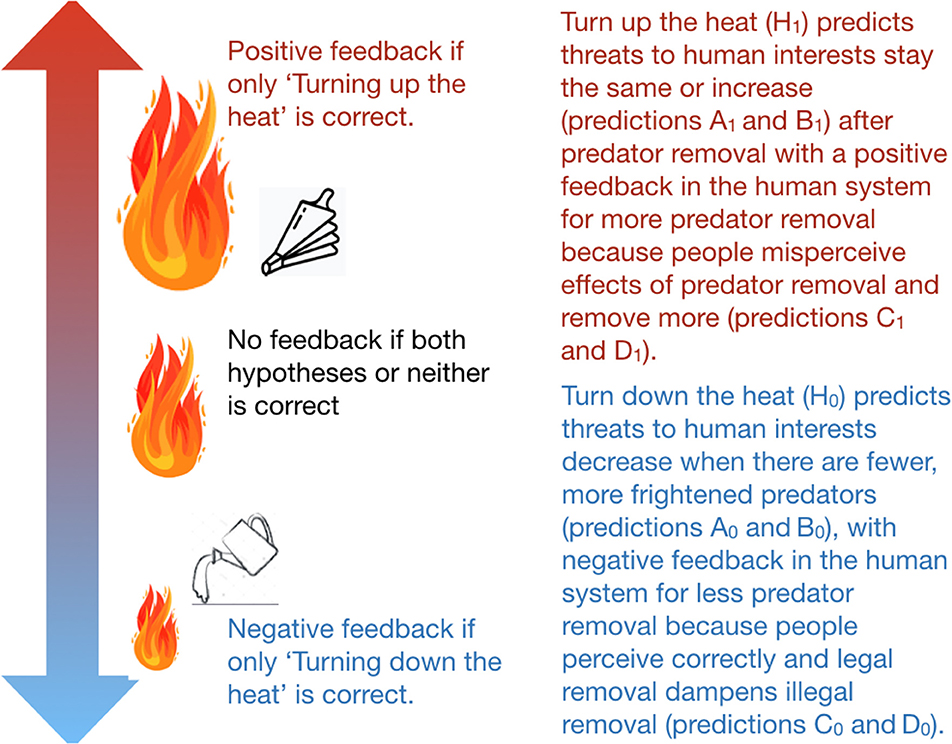
Figure 1. Predatory threats to human interests generate a socio-environmental system with potential coupling of the predator system to the human system. We present four pairs of opposed predictions in Table 1 and an explanation of how coupling to the human system occurs (text in red and blue fonts). Then we describe how positive negative or no feedback loops might arise (black font, water pail, and bellows).
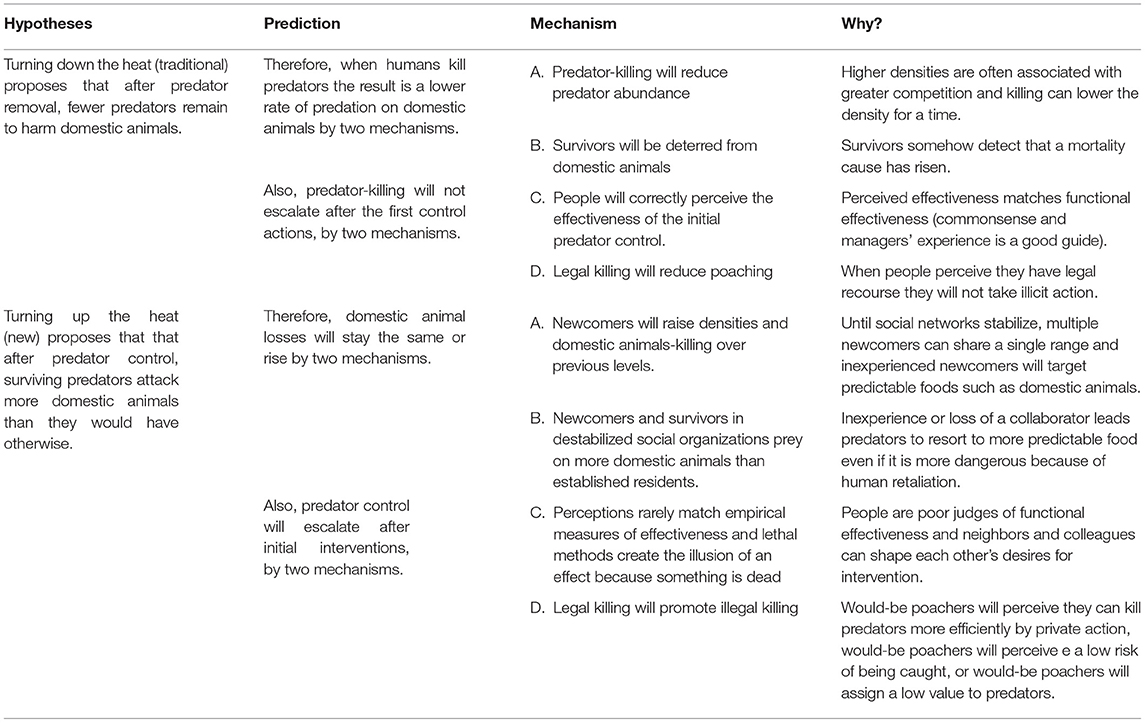
Table 1. Summary of opposed predictions from the traditional hypothesis of “Turning down the heat” (A–D, subscript zero) vs. the more recent “Turning up the heat” (A–D subscript 1).
Recommending Unbiased Predator Control Experiments for Strong Inference
Platt (1964) hypothesized about scientific progress and his recommendations remain crucial to scientific progress today. Platt's hypothesis about the rate of progress in science was that certain fields advance slowly and others quickly because their practitioners varied in the efficiency with which they proposed and tested between alternative, opposed hypotheses. Platt endorsed Chamberlin's 130-year-old admonition to keep at least two authentic, opposed hypotheses in mind at all times, and disfavor the scientist's preferred hypothesis (Chamberlin, 1890). Platt (1964) observed that the slower fields of his time had become bogged down by the perceptions that their topic was too complex for simple experimental tests. Platt countered that their models were becoming too complex to be falsifiable. Falsifiability is a foundational principle of science. He also countered that models are hypotheses that should be tested regularly, not judged by how many explanatory variables they contained or by the endless collection of data. Subsequent writers have echoed his views in their own fields (biomedical research, paleo-sciences, and population biology, among others). Ioannidis spent decades documenting difficulties in replicating eye-catching findings, problems of positive publication bias wherein journals and scientists prefer to report significant findings even if effect sizes were small and statistical power was low (Ioannidis, 2005). Ioannidis also called attention to the waste associated with intentional or unintentional biases in biomedical clinical research (systematic errors in selection of replicates, treatment fidelity, measurement precision, or reporting). We follow Ioannidis, Platt and Chamberlin by categorizing five forms of bias pervasive in our subfield, and others we surmise:
• Selection bias (also known as sampling bias): arises when the choice of which study subjects receive the treatment and which subjects receive the placebo control is non-random (or when the sample is so small that even randomization cannot prevent treatment and control groups from differing significantly at the outset). Selection bias is common in predator control research (see examples in WebPanel 1 from Treves et al., 2016), because domestic animals are often selected by the owners or by experimenters to receive an intervention or not. Selection rather than randomization undermines strong inference about an intervention effect because subjects naturally vary in their response to an intervention and the circumstances surrounding them may influence the effects of a treatment. Therefore, selection bias might lead to subjects more likely to respond in the predicted way to the intervention being chosen.
Self-selection is a form of selection bias that has long been recognized as slanting results severely in fields as distinct as medicine and policy studies (Nie, 2004; Ioannidis, 2005). But experimenters have also been implicated in selection bias when they intentionally or unintentionally assign subjects non-randomly. Biomedical research still struggles with this bias when humans are responsible for assignment (Mukherjee, 2010).
• Treatment bias occurs where the intervention or placebo controls are administered without standardization or quality control. A common form of treatment bias in predator control is to tailor the intervention method, its intensity or timing, to the subjective impressions of the domestic animal owners or the agents implementing intervention (see examples in WebPanel 1 from Treves et al., 2016; Santiago-Avila et al., 2018a). For example, even the best experimental test of lethal methods for predator control failed to distinguish the techniques applied, e.g., pooling shooting, trapping, baiting with poison, poaching, or regulated hunting, into one category of intervention (Treves et al., 2016). If care in standardizing interventions is not taken, it is easy for implementers to put more effort into subjects that seem to need more intervention, or distribute the intervention by convenience, both of which can bias results.
• Measurement bias occurs when methods for measuring response variables or covariates are not uniform across intervention and placebo control groups (see examples in WebPanel 1 from Treves et al., 2016). Ideally, those collecting data on the intervention and the placebo control are unaware of which the subject received (blinding). Experiments with inconspicuous manifestations (e.g., some medicinal treatments) are easiest to blind, but experiments with long-lasting structural modifications might not adequately conceal conspicuous interventions. For example, lethal methods intended to protect domestic animals from predators are often inconspicuous (e.g., concealed traps) or brief in implementation (e.g., shooting), which would facilitate blinding, whereas many non-lethal methods are conspicuous (e.g., fencing, lights, guardian animals).
The amount of blinding (single-, double-, triple-, or quadruple-) refers to how many steps in the experiment are concealed from researchers or reviewers. The steps that might be blinded include: (i) those intervening randomly should be unaware of subject histories and attributes and should not communicate which subjects received the control or treatment intervention to others in the research team (this depends on having used an undetectable intervention above); (ii) those measuring the effects are unaware of which intervention the subject received (this too depends on having used an undetectable intervention); (iii) those interpreting results are unaware of which subjects received treatment or control; and (iv) those independently reviewing results are unaware of which subjects received treatment or control and unaware of the identity of the scientists who conducted the research.
• Reporting bias is introduced by scientists omitting data or methods, or reporting in a way that is not even-handed regarding treatment (see examples in WebPanel 1 from Treves et al., 2016). This bias arises when analysis of data, interpretation of results, or scientific communications misrepresent research methods or findings. The most severe form arises when the reporting favors the scientists' preferred outcomes and naturally this is the most common form. Blinding (see above), standardized analysis protocols, and registered reports (see below) might be reliable defenses against reporting bias.
• Publication bias occurs when reviewers' and editors' disfavor certain results or disfavor replication efforts, either because (a) reviewers or editors are unimpressed by confirmatory results and therefore unenthusiastic about publication, or (b) reviewers or editors are biased toward the prior conclusions when results are not confirmatory, and thereby recommend rejection of replication efforts that do not meet their expectations. Publication bias is being addressed by the spread of new editorial practices. For example, journals are now accepting registered reports (reviewers evaluate the methods before data are collected and then the journal commits to publishing accepted registered reports once the results are analyzed, provided that the methods did not change); implementing policies that favor replication efforts (e.g., concealing from peer reviewers if the results have been collected until the methods are accepted or rejected); or implementing double-blind independent peer review (when peers are blinded to author identity). Several journals in our field are now using these methods (Sanders et al., 2017).
The five types of bias described above weaken inference from otherwise strong experiments, but they do not illuminate the design features that produce strong inference. To illuminate these design features, we define inference first. Inference means “the drawing of a conclusion from known or assumed facts or statements; esp. in Logic, the forming of a conclusion from data or premises, either by inductive or deductive methods; reasoning from something known or assumed to something else which follows from it” (OED, 2018). A century of philosophy of science and evaluation of scientific research in many disciplines by numerous authors has revealed variation in the strength of inference (Chamberlin, 1890; Popper, 1959; Kuhn, 1962; Platt, 1964; Gould, 1980; Ioannidis, 2005; Mukherjee, 2010, 2016; Biondi, 2014; Gawande, 2016). We acknowledge the doubts these authors expressed about approximating the truth, yet like them, we reject the notion that scientific evidence cannot be verified, and the notion that all inferences are equally subjective—following Lynn (2006). Below, we define standards that increase our confidence in the accuracy of evidence. We propose a single continuum of strength of inference as in Figure 2.
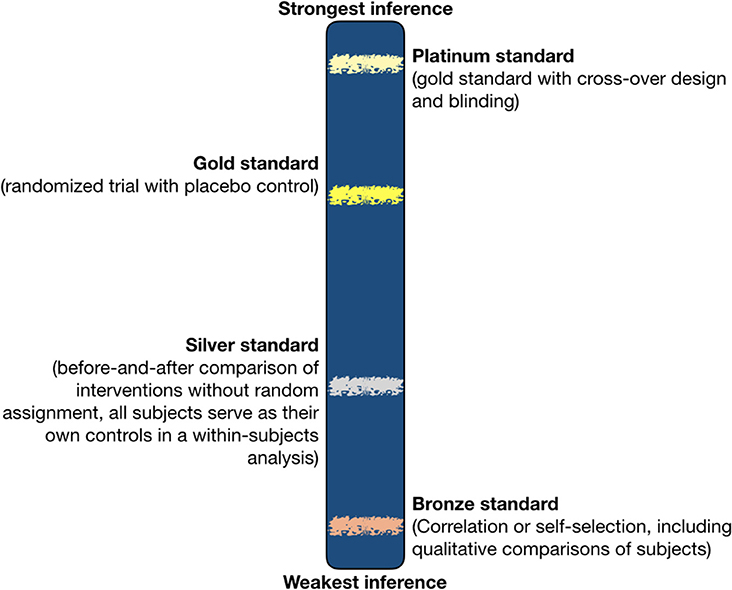
Figure 2. Strength of inference in relation to research design. The positions of standards along a continuum of strength of inference are approximated (fuzzy horizontal bars), because we cannot yet quantify strength of inference precisely. Also, evaluating the strength of inference from a particular study requires close reading of the methods and results to detect biases in design. However, the relative positions of the fuzzy lines for different standards are depicted to reflect the loss of confidence associated with the introduction of confounding variables or the lack of controls, e.g., silver standard tests lower the strength of inference by approximately half compared to gold standard, because all else being equal, they introduce one potentially confounding variable (the passage of time). All of the depicted standards presume no bias sufficient to undermine the reliability of a study.
Randomized controlled experiments with cross-over design, moderate or large sample sizes, and safeguards against bias, such as blinding, are the best available method to fairly evaluate interventions with strong inference about effectiveness. Even such experiments should be replicated by independent teams to be considered reliable (Ioannidis, 2005; Baker and Brandon, 2016; Goodman et al., 2016; Munafò et al., 2017; Alvino and PLoS One Editors, 2018). Figure 2 refers to confidence in inferences from a single research effort. A parallel but separate continuum might be developed for independent efforts at reproducibility, in short, a scientist places a given research effort along the continuum by virtue of the design of that effort.
Although we begin with the platinum standard as the strongest inference, we repeatedly refer to elements of the gold standard which are described below the platinum standard, because we anticipate that few if any studies in animal research will achieve the platinum standard. Therefore, we hold the platinum-standard out as an aspirational guideline super-imposed atop the gold, which we deem necessary to strong inference, in almost every case. We also recognize that silver and bronze standards for experimental design can yield useful information where gold and platinum standards seem infeasible, but we advocate prioritizing the latter. We also recommend that researchers explain why they were unable to randomize or measure suitable controls, as a standard practice, so readers can be alerted to weaker inference and perhaps to potential biases.
Randomization
Randomization is random assignment of subjects to intervention groups or to placebo control groups. Controls are considered essential to making reliable inferences about the effect of an intervention because variability and change are ubiquitous. A placebo control group contains subjects who have received everything but the hypothesized effective treatment and in exactly the same ways, times, and places, e.g., a sugar pill administered just like a medicinal pill, or blank ammunition (i.e., no projectile striking the predator) rather than lethal ammunition. Randomization is widely considered to be the most important step in eliminating bias in experiments because it can eliminate the most prevalent and pervasive selection bias by researchers unconsciously seeking desired effects of a treatment.
Cross-Over Design
Because of randomization, some subjects will begin as placebo controls and others in treatment conditions, but additionally in cross-over design, all subjects will reverse to the other condition at approximately the same time midway through the experiment. A third reversal further strengthens inference about the effect of treatment. Therefore, every subject experiences both the intervention and the placebo control. By so doing, excessive differences between subjects and local effects of time passing are rendered less confounding, by measuring the response of subjects to treatments minus the response of the same subjects to placebo control. Although this might appear to be silver-standard at first glance, it is combined with randomization, so some subjects begin as placebo control and end the study in the intervention group, therefore some subjects experienced change over time followed by intervention whereas others experienced the reverse. See for example, a predator control experiment with cross-over design (Ohrens et al., 2019a). When designing cross-over experiments, it might be important to allow time between the first and reversed treatment for effects to “wash out” and to account for the possible time lag or long-lasting effects of the treatment. Such “wash out” periods should be designed at a length appropriate to the effect under study and the memory capabilities of the animal species being affected or replacement time of the individual animals affected.
Why Before-and-After Comparisons Weaken Inference
Silver standard is defined as before-and-after comparisons of interventions. In silver standard studies, either every subject gets the intervention (no placebo control) or control subjects are not chosen randomly, and each subject is compared to itself before intervention. For example, the number of domestic animals lost prior to intervening is subtracted from the number of domestic animals lost after intervening. Before-and-after comparisons are also called case-control experiments or BACI (before and after comparison of impacts) and are often used when randomization is considered infeasible. If BACI includes randomization, we refer the reader to the gold-standard above. Much has been written on stronger and weaker inference in BACI designs (Murtaugh, 2002; Stewart-Oaten, 2003), with a good example in a related field to ours (Popescu et al., 2012). Statisticians seem to us to have reached consensus that non-random BACI designs should employ first-order (at least) serial autocorrelation statistics which treat within-subject measurements as time series and consider expert information on local events that might confound effects of treatment and the proportion of subjects so affected relative to total sample size.
Silver is a lower standard than gold because inference is weaker. At a minimum, silver-standard studies introduce a new variable, time, i.e., all subjects underwent the passage of time that affects individuals differently. Consider the analogy of a cold remedy. We know most people recover from colds over time. Therefore, any proposed treatment should work faster or better than the natural, healthy person's recovery from a cold. If the putative treatment for colds is tested by a silver-standard design, the inference that it was effective is difficult to distinguish from the inference that subject patients got better on their own as time passed. Non-randomized BACI might have difficulty distinguishing treatment effect from time effect if selection bias was introduced in who received the cold remedy (e.g., patients who volunteer for an experimental remedy are usually not a random sample of patients; Mukherjee, 2010). Predator control experiments are often good analogies to the hypothetical cold remedy. Domestic animals might be attacked by predators only once with no repeat, even in the absence of intervention (see previous section on uncertainties in predator control). Therefore, loss of a domestic animal might not be repeated simply because of the passage of time. The uncontrolled effect of time passing is why we rate silver-standard designs as producing inference that is half as strong as gold-standard designs. The presence of a control, comparison group chosen without selection bias is therefore essential to raising the strength of inference.
One can improve on silver-standard somewhat if one staggers treatment so that subjects do not all experience treatment at the same time. Such staggering might eliminate a simultaneous, brief confounding effect on all subjects (e.g., a weather event, a sudden phenological event in other species). Nevertheless, subjects still experience time passing even if not simultaneously. Researchers have addressed the confounding effect of time passing by removing treatment and monitoring their subjects again so there are three measurements at least: before-treatment baseline, after-treatment response, and after removal of treatment another response. While stronger than before-and-after comparisons, we still see two problems with recommending this design: First, the ability to remove treatment in the final phase implies the researcher has influence to manipulate the treatment, which begs the question why not treat randomly? Perhaps, the treatment is not under the influence of the researcher, but it ends for all subjects simultaneously or after a predetermined duration. If so, we place such studies higher than silver-standard but not gold-standard, as in Figure 2. Yet, this approach merits scrutiny for a second reason. The variable “time” still affected every subject in parallel with the treatment, so the n = 2 for the effect of time. If one wants strong inference about the effect of time independent of treatment one needs a higher n of re-treatments and removals. That would seem to drag out the trials and once again beg the question of why not work harder to randomize and cross-over? Therefore, we conclude that before-during-after designs do not improve much on silver standards, only approximating gold standard with many treatments and removals.
Correlations or descriptive observations, which we define as bronze standard of experimental designs, provide weaker inference than silver standard experiments because they do not clarify cause-and-effect directionality and the lack of intervention introduces numerous other potentially confounding effects on subjects. Of course, description and correlation may be important starting points when little is known about a system, but predator control has gone far beyond such a basic level of scientific observation, so we consider gold-standard experiments essential for strong inference about predator control.
Given the variety of situations in which animal research might be conducted, it is conceivable that a research team would find it impossible to design a platinum-standard experiment, a gold-standard experiment, or eliminate all potential biases. Accepting a lower standard than gold should be justified by arguments based on ethics, law, or impossibility, not convenience or vague references to socio-cultural acceptance. An immoral or illegal research method would make a gold-standard or better design infeasible. The common claim that experiments are infeasible due to cost should not be used as a blanket dismissal but instead quantified and examined rigorously as a design criterion. Recalling that expenditures are value judgments about one hypothesized social good compared to another, the value judgments should be kept separate from the issues of feasibility until the cost-efficient research design has been specified, not a priori or in the absence of data on current predator control expenditures. When governments sponsor predator control nationwide as the U.S. does through US Department of Agriculture Wildlife Services, millions of USD might be expended in unproven methods (Treves and Naughton-Treves, 2005; Bergstrom et al., 2014; Treves et al., 2016), so premature dismissal of methods for strong inference is on weaker grounds in such conditions and may even trigger conflict of interest concerns. Most arguments about feasibility should pass a test for authentic impossibility as follows.
To provide guidelines for situations in which gold- or platinum-standard experimental designs might be deferred until feasible, we have to differentiate feasibility from impossibility. Feasible (“Of a design, project, etc.: Capable of being done, accomplished or carried out;….” OED, 2018) should not be confused with impossible (“Not possible; that cannot be done or effected; that cannot exist or come into being; that cannot be, in existing or specified circumstances.” OED, 2018). We observe that the common usage of “impossible” often reflects a person's perception that they do not have the capability, time, or resources to accomplish something, in addition to authentic impossibility. We aim to distinguish those concepts to advance the field beyond unfalsifiable claims that gold-standard experiments were impossible, so the public should accept lower strength of inference. Authentic impossibility means one of two things: (1) that two actions or events are mutually exclusive although either is feasible (e.g., I cannot study the behavior of an animal and study its death at the same time); or (2) an action or event would violate physical laws (e.g., I cannot survive in outer space without a space suit). The latter example acknowledges that some technological innovations and scientific discoveries overcome former impossibilities, which underscores the distinction between “action x is impossible” and “action x is not currently feasible.” For practical purposes, most people's response to difficult situations can be rephrased as “I do not currently have the motivation, legal authority, time, skills, or resources to accomplish that action.” That is not the same as impossible because the obstacles might change over time. Although many actions are authentically impossible, most objections to improving the standards for inference about predator control are actually claims of feasibility. Few elements of the platinum standard or gold standard without bias are impossible. Rather they can be very difficult, and difficulty might make such designs infeasible. Therefore, claims of infeasibility demand scrutiny by independent reviewers and editors.
We call for higher scientific standards of predator control experiments and propose a design of the first-ever platinum-standard experiment providing strong inference derived from randomized, controlled experiments with cross-over design and without bias.
An Example of Platinum-Standard Experimental Design for Predator Control
In Figure 3, we provide a schematic design of a platinum-standard experiment. In line with our two opposing hypotheses (Figure 1; Table 1), the study designs should measure both threats to domestic animals and human attitudes toward predators and predator control. We suggest recruiting owners of domestic animals who are enthusiastic about controlled experiments, as participants and select replicates (e.g., herds of domestic animals) that are separated geographically by more than the maximum home range of the targeted predators, so we can be sure we are testing more than one individual predator. The most difficult element is the blinding in our opinion, but we recommend adoption of several of the blinding steps because these could eliminate biases in selection, treatment, measurement, reporting, and publication. Personnel should aim for multiple blinding when treatments and placebo controls are not conspicuously different (obvious from a distance), but lower rigor of blinding for inconspicuous interventions. In cases where a treatment is easily distinguished from a placebo control (conspicuous, long-lasting stimuli), we suggest protecting field measurements as follows.
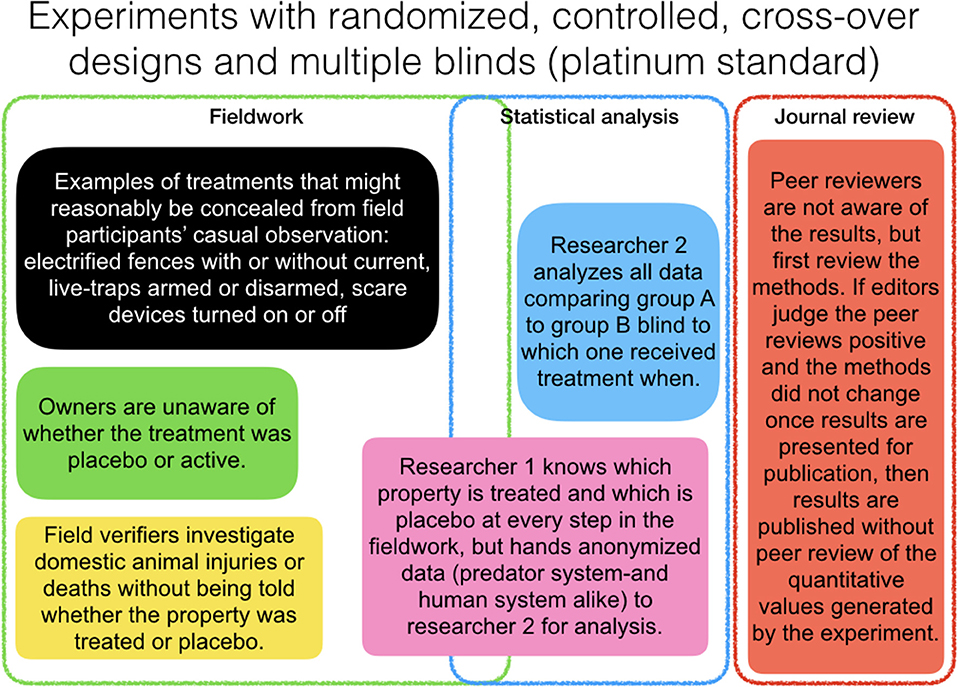
Figure 3. Template for a platinum-standard experiment (randomized, controlled, cross-over design with multiple blinding steps). The three stages shown are fieldwork, analysis, and publication. Rectangles indicate different individual people involved at one or more stages.
All field measurements should be divided in two or more tasks for different individuals. First, trail cameras and other covert data sets should be analyzed by members of a study team who are single-blinded to the treatment (e.g., in the lab later not in the field concurrently), whereas the field measurements of domestic animal loss or injury should be conducted by team members and a third party (e.g., government agents) who must agree among themselves on the interpretation. The latter team would not play a role in analyzing the effect of treatment or the former dataset (double-blind). When possible, triple-blinding would demand one part of team implement, one part work with domestic animal owners, and one part measure effects. The quadruple-blinding step would be reached if a registered report were accepted and independent reviewers were blind to results and author identities.
To test our hypotheses relating to the human system and the predator system, we recommend measures of predators, domestic animals, and of humans. We recommend social scientific surveys of human subjects to measure attitudes toward predators, toward the methods being employed, to government verifiers if appropriate; and measures of intentions to poach and to adopt predator control methods after the experiment ends, i.e., all variables of perceived effectiveness (Ohrens et al., 2019b). Ideally, outcomes would be measured for a year or more afterwards (Table 1). Intention to poach is not the same as actually poaching of course, but intention to poach might predict actual poaching and might be used to test the predictions in Figure 1 and Table 1, nonetheless (Treves et al., 2013, 2017b; Treves and Bruskotter, 2014). For domestic animals, we recommend careful verification, possibly including blind tests of interobserver reliability using carcasses of domestic animals that died of known causes (López-Bao et al., 2017). Also, measurement of threats to domestic animals should include close approach by predators in proximity to domestic animals even if no attack, injury, or loss occurs (Davidson-Nelson and Gehring, 2010). The use of camera traps and indirect sign surveys might prove useful for detecting approach and avoidance by predators, in addition to confirming that predators were present in the experimental site for both treatment and placebo control subjects and phases (Ohrens et al., 2019a). Only under special circumstances would live-capture and immobilization of predators be required, e.g., for control methods that are affixed to predators (Hawley et al., 2009).
In total, the length of time to complete such a platinum-standard experiment depends on certain factors we cannot prescribe precisely for an abstract trial. For one, the rate of threats to property interests and the difference in rates between placebo control and treatment would dictate the length of time needed to accumulate enough threats to detect a difference. For example, in one study (Ohrens et al., 2019a), 4 months was sufficient to reveal a statistical difference between placebo (no domestic animals attacked by pumas) and treatment (seven domestic animals attacked by pumas) but not to detect a difference for the Andean foxes nor to be confident of long-term effects (Khorozyan and Waltert, 2019). Nonetheless, we echo the sentiments of researchers calling for less adherence to traditional thresholds of significance (Amrhein et al., 2019), so even a reduction in risk equivalent to 1–2 standard deviations of the placebo control subjects might justify using or discarding a proposed treatment for predator control, regardless of the probability value generated by frequentist statistical tests (e.g., Chapron and Treves, 2017a).
Building an evidence-base on what works in predator control requires repeated studies in different contexts and long-term monitoring. As such, we suggest creating a consortium of international scientists dedicated to experiments on methods of predator control to oversee the entire procedure that can be replicated in different locations. For each study, the methods should be submitted before the actual field experiment begins, as registered reports, to reduce the risk that methods drift to accommodate obstacles in the field and to reduce publication bias.
Discussion
Despite over 20 years of searching for answers about predator control, the policy intervention of killing predators that threaten domestic animals has not been subjected to unbiased, randomized experimental tests of effectiveness (gold-standard) or higher (Treves et al., 2016). The closest that governments have come to this gold-standard are the United Kingdom's European badger experiments on the control of bovine tuberculosis (Jenkins et al., 2010; Donnelly and Woodroffe, 2012; Vial and Donnelly, 2012; Bielby et al., 2016). Other attempts have either been focused on small-bodied predators (often non-natives; Greentree et al., 2000), or experiments with coyote-sized (15 kg) or larger native predators in captivity or semi-free-ranging conditions (Knowlton et al., 1999) and a few of both types of studies that failed to achieve the gold standard because of one or more biases, such as researchers selecting the subjects to receive treatments and control subjects after the fact, irreproducible methods, omitting methods from peer-reviewed papers, or neglecting to measure or report accurately other predator control methods underway during the trial (lethal or non-lethal), or all these shortcomings combined (see examples in WebPanel 1 from Treves et al., 2016). Given the economic, ecological, conservation, and ethical interests scrutinizing this topic, the paucity of experiments that produce strong inference about the control of domestic animal predators has raised concerns about the validity of management practices and government policy in many regions (van Eeden et al., 2018).
We observe several common rebuttals that may explain the paucity of gold-standard evidence for lethal predator control. First, some fields have pleaded special conditions. For example, historical sciences like geology argue that random assignment to treatment and control is impossible when drawing inference about the past (Gould, 1980; Biondi, 2014). Predator control cannot claim such special constraints in our view.
Second, some argue that individual subject differences are so pervasive and influential that systematic studies cannot recommend what an individual does—only an expert assessment of local conditions can do so. See statisticians' debate this same issue in other areas of ecology (Murtaugh, 2002; Stewart-Oaten, 2003). Such calls to expert authority are anti-scientific because they maintain an “unmeasurable uniqueness” prevents generalization from any systematic study, no matter how strong the inference. This position is only tenable until experiments yielding strong inference are conducted.
Third, a related objection is that wild ecosystems have so many confounding variables that treatment effects will not be detectable. Essentially, the argument that inherent variability of subjects (or across testing sites) is too great, simply reflects an argument about the magnitude of treatment effects. A weak treatment effect might be undetectable against background variation. But we caution against making this claim unfalsifiable by failing to specify what varies too much (among the response variables or confounding variables), and against disingenuous assertions that experiments are impossible (see above).
We acknowledge that platinum is a very challenging standard for experiments. One might not install a costly intervention (e.g., kilometers of electric fence) only to take it down for the reversal of treatment to placebo control. Such constraints might lead one to use the lower gold standard, but we note that further arguments for weakening inference or introducing bias must be scrutinized carefully. The complaint of infeasibility cannot be allowed to become unfalsifiable. It demands scientific scrutiny by funders and by independent reviewers prior to accepting lower standards and weaker inference.
The research community has long understood that randomization, large sample sizes, and cross-over designs can overcome high between-subject variability. Indeed, the biomedical research community, for which randomized clinical trials of proposed medicines are often required by law, has faced serious questions about bias in clinical trials. However, these critiques rarely advocate “throwing the baby out with the bathwater” (Ioannidis, 2005), because no one has proposed a superior method to randomized, controlled trials for eliminating sampling errors and selection bias. For many fields, reasonable remedies for persistent biases have focused on the addition of safeguards against bias within randomized trials. For example, reverse-treatment or cross-over design that analyzes within-subject changes, is a useful way to reduce the confounding effects of high variability between subjects that might obscure a treatment effect when only group-level statistics are run.
The fourth objection we have encountered is that it is unethical to the animals to experiment with lethal predator control. That judgment seems to depend on relative harms, such as whether domestic animals are dying because an ineffective method is in place, or whether wild or feral animals are dying but a non-lethal method that is equally or more effective is known to exist. To reduce the ethical concerns and legal restrictions on humane killing, lethal predator control can be replaced by simulation, such as moving the captured predators into captivity for one field season and releasing them after the experiment. In this case, captive conditions should be designed and managed in a way that achieves humane treatment, minimizes social disruptions, and avoids habituation of predators to human stimuli. For example, captive predators should be fed with wild prey carcasses from road-kill and exposure to people should be minimized while kept in captivity (We anticipate the concern that without a gunshot or explosive it is not a realistic simulation that “teaches” survivors something. However, the verisimilitude of non-lethal removal might be increased by firing a blank gunshot or firing an explosive at trap sites after the removal of predator to captivity). The above steps only reduce suffering by predators but do not eliminate them. Therefore, a clear, logical ethical argument that balances current, ongoing harms against future reductions in harm should be attempted and subjected to independent review, as recommended and practiced in other contexts and wildlife management situations (Lynn, 2018; Santiago-Avila et al., 2018b; Lynn et al., 2019).
Finally, some opposition to randomized, controlled experiments claim that property owners will reject being assigned the placebo. In small-scale experiments, both assumptions were called into question a decade ago in Michigan, USA (Davidson-Nelson and Gehring, 2010; Gehring et al., 2010). In 2019, an experiment in Tarapacá, Chile, with 11 herds of domestic camelids used cross-over design, recruited owners to serve as controls, and used a participatory intervention planning process to facilitate implementation of the experiment (Ohrens et al., 2019a). We recognize that socioeconomic and cultural dimensions of conflict with predators can be real barriers to implementing experiments (Naughton-Treves, 1997; Naughton-Treves and Treves, 2005; Florens and Baider, 2019). We predict that teams armed with tools and techniques from the communication sciences will succeed in addressing site-specific, sociopolitical barriers to evidence-based management (Treves et al., 2006; Treves, 2019b), except perhaps in the most adamantly anti-science interest groups. We also acknowledge that certain jurisdictions might sustain for long periods a mix of owners and government agents who refuse to consider experimental evaluation of their favored, predator control methods. All the authors have experienced this. We have either chosen to work elsewhere or persuaded the needed actors. Often a subset of owners will agree, and government staff are not always needed for such experiments. In other cases, changes of leadership have led to changes in acceptance of experiments. But this can cut both ways and we encourage researchers to adopt the tools of the communication sciences to recruit participants when anti-science views are an obstacle or when cultural ideological clashes will slow the acceptance of new ideas or evidence (Dunwoody, 2007).
We realize that implementing gold- and platinum-standard research in predator control will face substantial logistical, financial, and cultural barriers. We anticipate that these experiments will succeed where domestic animal owners themselves have recognized the need for a scientific solution, where the jurisdiction is permissive of the methods including both the predator control methods and the blinding procedures, where authentic placebo controls are possible, and where between-subject variability and within-subject differences over time do not confound treatment effects. However, the paucity of randomized, controlled experiments without bias, and disparate standards of evidence across the field of predator control have consequences for policy and management decisions and highlight the need to modernize the field and increase scientific standards of predator control research. We argue that the approach to predator control research that we have outlined here presents a critical opportunity to inject evidence into decision-making which will benefit both humans and non-humans while fulfilling a responsibility that scientists have to the broadest public including future generations.
Author Contributions
AT conceived of and led the writing of the manuscript. All other authors helped to write the manuscript.
Funding
AT and MK received support from the Brooks Institute for Animal Rights Law & Policy. G. Chapron, F. J. Santiago-Avila, W. S. Lynn, C. Fox, and K. Shapiro provided valuable advice. MK was supported by the Slovenian Research Agency (P4- 0059). LE was supported by an Australian-American Fulbright fellowship.
Conflict of Interest
The authors declare that the research was conducted in the absence of any commercial or financial relationships that could be construed as a potential conflict of interest.
References
Adams, L. G., Stephenson, R. O., Dale, B. W., Ahgook, R. T., and Demma, D. J. (2008). Population dynamics and harvest characteristics of wolves in the Central Brooks Range, Alaska. Wildlife Monographs 170, 1–25. doi: 10.2193/2008-012
Allen, B. L., Lundie-Jenkins, G., Burrows, N. D., Engeman, R. M., Fleming, P. J. S., and Leung, L. K. P. (2016). Does lethal control of top-predators release mesopredators? A re-evaluation of three Australian case studies. Ecol. Manage. Restor. 15, 193–195. doi: 10.1111/emr.12118
Allen, L. R., and Sparkes, E. C. (2001). The effect of dingo control on sheep and beef cattle in Queensland. J. Appl. Ecol. 38, 76–87. doi: 10.1046/j.1365-2664.2001.00569.x
Alvino, G., and PLoS One Editors. (2018). Building Research Evidence Towards Reproducibility of Animal Research. PLOS ONE blog News & Policy. Available online at: https://blogs.plos.org/blog/2018/08/06/building-research-evidence-towards-reproducibility-of-animal-research/%20accessed%2029%20November%202019
Amrhein, V., Greenland, S., and Mcshane, B. (2019). Scientists rise up against statistical significance. Nature 557, 305–307. doi: 10.1038/d41586-019-00857-9
Baker, M., and Brandon, K. (2016). 1,500 scientists lift the lid on reproducibility. Nature 533, 452–454. doi: 10.1038/533452a
Bauer, S., Lisovski, S., Eikelenboom-Kil, R. J. F. M., Shariati, M., and Nolet, B. A. (2018). Shooting may aggravate rather than alleviate conflicts between migratory geese and agriculture. J. Appl. Ecol. 55, 2653–2662. doi: 10.1111/1365-2664.13152
Beggs, R., Tulloch, A. I. T., Pierson, J., Blanchard, W., Crane, M., and Lindemayer, D. L. (2019). Patch-scale culls of an overabundant bird defeated by immediate recolonization. Ecol. Appl. 29:e01846. doi: 10.1002/eap.1846
Bergstrom, B. J., Arias, L. C., Davidson, A. D., Ferguson, A. W., Randa, L. A., and Sheffield, S. R. (2014). License to kill: reforming federal wildlife control to restore biodiversity and ecosystem function. Conserv. Lett. 7, 131–142. doi: 10.1111/conl.12045
Bielby, J., Vial, F., Woodroffe, R., and Donnelly, C. A. (2016). Localised badger culling increases risk of herd breakdown on Nearby, Not Focal, Land. PLOS ONE 10:e164618. doi: 10.1371/journal.pone.0164618
Biondi, F. (2014). Paleoecology grand challenge. Front. Ecol. Evol. 2:50. doi: 10.3389/fevo.2014.00050
Blumm, M. C., and Wood, M. C. (2017). “No Ordinary Lawsuit”: climate change, due process, and the public trust doctrine. Am. Univ. Law Rev. 67, 1–83. Available online at: https://papers.ssrn.com/sol3/papers.cfm?abstract_id=2954661
Borg, B. L., Brainerd, S. M., Meier, T. J., and Prugh, L. R. (2015). Impacts of breeder loss on social structure, reproduction and population growth in a social canid. J. Anim. Ecol. 84, 177–187. doi: 10.1111/1365-2656.12256
Bradley, E. H., Robinson, H. S., Bangs, E. E., Kunkel, K., Jimenez, M. D., Gude, J. A., et al. (2015). Effects of wolf removal on livestock depredation recurrence and wolf recovery in Montana, Idaho, and Wyoming. J. Wildl. Manage. 79, 1337–1346. doi: 10.1002/jwmg.948
Browne-Nuñez, C., Treves, A., Macfarland, D., Voyles, Z., and Turng, C. (2015). Tolerance of wolves in Wisconsin: a mixed-methods examination of policy effects on attitudes and behavioral inclinations. Biol. Conserv. 189, 59–71. doi: 10.1016/j.biocon.2014.12.016
Ceballos, G., and Ehrlich, P. R. (2018). The misunderstood sixth mass extinction. Science 60, 1080–1081. doi: 10.1126/science.aau0191
Chamberlin, T. C. (1890). The method of multiple working hypotheses. Science 15, 754–759. doi: 10.1126/science.148.3671.754
Chapron, G., Epstein, Y., Trouwborst, A., and López-Bao, J. V. (2017). Bolster legal boundaries to stay within planetary boundaries. Nat. Ecol. Evol. 1:86. doi: 10.1038/s41559-017-0086
Chapron, G., Kaczensky, P., Linnell, J. D. C., Von Arx, M., Huber, D., Andrén, H., et al. (2014). Recovery of large carnivores in Europe's modern human-dominated landscapes. Science 346, 1517–1519. doi: 10.1126/science.1257553
Chapron, G., and Treves, A. (2016a). Blood does not buy goodwill: allowing culling increases poaching of a large carnivore. Proc. Roy. Soc. B 283:20152939. doi: 10.1098/rspb.2015.2939
Chapron, G., and Treves, A. (2016b). Correction to ‘Blood does not buy goodwill: allowing culling increases poaching of a large carnivore'. Proc. Roy. Soc. 283:20162577. doi: 10.1098/rspb.2016.2577
Chapron, G., and Treves, A. (2017a). Reply to comment by Pepin et al. 2017. Proc. Roy. Soc. B 284, 20162571. doi: 10.1098/rspb.2016.2571
Chapron, G., and Treves, A. (2017b). Reply to comments by Olson et al. 2017 and Stien 2017. Proceedings of the Royal Society B 284, 20171743.
Conradie, C., and Piesse, J. (2013). “The Effect of Predator Culling on Livestock Losses: Ceres, South Africa, 1979 – 1987”. Cape Town: Centre for Social Science Research; University of Cape Town.
Cooley, H. S., Wielgus, R. B., Koehler, G. M., and Maletzke, B. T. (2009a). Source populations in carnivore management: cougar demography and emigration in a lightly hunted population. Anim. Conserv. 12, 321–328. doi: 10.1111/j.1469-1795.2009.00256.x
Cooley, H. S., Wielgus, R. B., Robinson, H. S., Koehler, G. M., and Maletzke, B. T. (2009b). Does hunting regulate cougar populations? A test of the compensatory mortality hypothesis. Ecology 90, 2913–2921. doi: 10.1890/08-1805.1
Cote, I. M., and Sutherland, W. J. (1997). The effectiveness of removing predators to protect bird populations. Conserv. Biol. 11, 395–405. doi: 10.1046/j.1523-1739.1997.95410.x
Creel, S., Becker, M., Christianson, D., DröGe, E., Hammerschlag, N., Hayward, M. W., et al. (2015). Questionable policy for large carnivore hunting. Science 350, 1473–1475. doi: 10.1126/science.aac4768
Davidson-Nelson, S. J., and Gehring, T. M. (2010). Testing fladry as a nonlethal management tool for wolves and coyotes in Michigan. Hum. Wildlife Interact. 4, 87–94. doi: 10.26077/mdky-bs63
Donnelly, C., and Woodroffe, R. (2012). Reduce uncertainty in UK badger culling. Nature 485:582. doi: 10.1038/485582a
Dunwoody, S. (2007). “The challenge of trying to make a difference using media messages,” in Creating a Climate for Change, eds S. C. Moser and L. Dilling (Cambridge: Cambridge University Press), 89–104. doi: 10.1017/CBO9780511535871.008
Estes, J. A., Terborgh, J., Brashares, J. S., Power, M. E., Berger, J., Bond, W.J., et al. (2011). Trophic downgrading of planet earth. Science. 333, 301–306. doi: 10.1126/science.1205106
Fernández-Gil, A., Naves, J., Ordiz, A. S., Quevedo, M., Revilla, E., and Delibes, M. (2015). Conflict misleads large carnivore management and conservation: brown bears and wolves in Spain. PLos ONE 11:e015154. doi: 10.1371/journal.pone.0151541
Florens, F. B. V., and Baider, C. (2019). Mass-culling of a threatened island flying fox species failed to increase fruit T growers' profits and revealed gaps to be addressed for effective conservation. J. Nat. Conserv. 47, 58–64. doi: 10.1016/j.jnc.2018.11.008
Gehring, T. M., Vercauteren, K. C., Provost, M. L., and Cellar, A. C. (2010). Utility of livestock-protection dogs for deterring wildlife from cattle farms. Wildl. Res. 37, 715–721. doi: 10.1071/WR10023
Goodman, S., Fanelli, D., and Ioannidis, J. (2016). What does research reproducibility mean? Sci. Trans. Med. 8:341ps312. doi: 10.1126/scitranslmed.aaf5027
Gould, S. J. (1980). The promise of paleobiology as a nomothetic, evolutionary discipline. Paleobiology 6, 96–118. doi: 10.1017/S0094837300012537
Greentree, C., Saunders, G., Mcleod, L., and Hone, J. (2000). Lamb predation and fox control in south-eastern Australia. J. Appl. Ecol. 37, 935–943. doi: 10.1046/j.1365-2664.2000.00530.x
Haber, G. C. (1996). Biological, conservation, and ethical implications of exploiting and controlling wolves. Conserv. Biol. 10, 1068–1081. doi: 10.1046/j.1523-1739.1996.10041068.x
Hawley, J. E., Gehring, T. M., Schultz, R. N., Rossler, S. T., and Wydeven, A. P. (2009). Assessment of shock collars as nonlethal management for wolves in wisconsin. J. Wildl. Manage. 73, 518–525. doi: 10.2193/2007-066
Hogberg, J., Treves, A., Shaw, B., and Naughton-Treves, L. (2015). Changes in attitudes toward wolves before and after an inaugural public hunting and trapping season: early evidence from Wisconsin's wolf range. Environ. Conserv. 43, 45–55. doi: 10.1017/S037689291500017X
Imbert, C., Caniglia, R., Fabbri, E., Milanesi, P., Randi, E., Serafini, M., et al. (2016). Why do wolves eat livestock? Factors influencing wolf diet in northern Italy. Biol. Conserv. 195, 156–168. doi: 10.1016/j.biocon.2016.01.003
Ioannidis, J. P. (2005). Why most published research findings are false. PLOS Med. 2:e124. doi: 10.1371/journal.pmed.0020124
Jenkins, H., Woodroffe, R., and Donnelly, C. (2010). The duration of the effects of repeated widespread badger culling on cattle tuberculosis following the cessation of culling. PLoS ONE 5:e9090. doi: 10.1371/journal.pone.0009090
Khorozyan, I., and Waltert, M. (2019). How long do anti-predator interventions remain effective? Patterns, thresholds and uncertainty. Roy. Soc. Open Sci. 6:e190826. doi: 10.1098/rsos.190826
Knowlton, F. F., Gese, E. M., and Jaeger, M. M. (1999). Coyote depredation control: an interface between biology and management. J. Range Manage. 52, 398–412. doi: 10.2307/4003765
Kompaniyets, L., and Evans, M. (2017). Modeling the relationship between wolf control and cattle depredation. PLoS ONE 12:e0187264. doi: 10.1371/journal.pone.0187264
Krofel, M., Špacapan, M., and Jerina, K. (2017). Winter sleep with room service: denning behaviour of brown bears with access to anthropogenic food. J. Zool. 302. doi: 10.1111/jzo.12421
López-Bao, J. V., Frank, J., Svensson, L., ÅKesson, M., and Langefors, A. S. (2017). Building public trust in compensation programs through accuracy assessments of damage verification protocols. Biol. Conserv. 213, 36–41. doi: 10.1016/j.biocon.2017.06.033
Lynn, W. S. (2006). “Between science and ethics: what science and the scientific method can and cannot contribute to conservation and sustainability,” in Gaining Ground: In Pursuit of Ecological Sustainability, ed D. Lavigne (Limerick: University of Limerick, 191–205.
Lynn, W. S. (2018). Bringing ethics to wild lives: shaping public policy for barred and northern spotted owls. Soc. Anim. 26, 217–238. doi: 10.1163/15685306-12341505
Lynn, W. S., Santiago-Ávila, F. J., Lindenmeyer, J., Hadidian, J., Wallach, A., and King, B. J. (2019). A moral panic over cats. Conserv. Biol. 33, 769–776. doi: 10.1111/cobi.13346
Minnie, L., Gaylard, A., and Kerley, G. (2016). Compensatory life-history responses of a mesopredator may undermine carnivore management efforts. J. Appl. Ecol. 53, 379–387. doi: 10.1111/1365-2664.12581
Munafò, M. R., Nosek, B. A., Bishop, D. V. M., Button, K. S., Chambers, C. D., Percie Du Sert, N., et al. (2017). A manifesto for reproducible science. Nat. Hum. Behav. 1:21. doi: 10.1038/s41562-016-0021
Murtaugh, P. A. (2002). On rejection rates of paired intervention analysis. Ecology 83, 1752–1761. doi: 10.1890/0012-9658(2002)083[1752:ORROPI]2.0.CO;2
Naughton-Treves, L. (1997). Farming the forest edge: vulnerable places and people around Kibale National Park. Geograph. Rev. 87, 27–46. doi: 10.2307/215656
Naughton-Treves, L., and Treves, A. (2005). “Socioecological factors shaping local support for wildlife in Africa,” in People and Wildlife, Conflict or Coexistence? ed R. Woodroffe, S. Thirgood, and A. Rabinowitz (Cambridge: Cambridge University Press, 253–277.
Newby, F., and Brown, R. (1958). A new approach to predator management in Montana. Montana Wildlife 8, 22–27.
Newsome, T. M., Greenville, A. C., Cirović, D., Dickman, C. R., Johnson, C. N, Krofel, M., et al. (2017). Top predators constrain mesopredator distributions. Nat. Commun. 8:15469. doi: 10.1038/ncomms15469
Nie, M. (2004). State wildlife policy and management: the scope and bias of political conflict. Publ. Admin. Rev. 64, 221–233. doi: 10.1111/j.1540-6210.2004.00363.x
Odden, J., Herfindal, I., Linnell, J. D. C., and Andersen, R. (2008). Vulnerability of domestic sheep to lynx depredation in relation to roe deer density. J. Wildl. Manage. 72, 276–282. doi: 10.2193/2005-537
Odden, J., Linnell, J. D. C., Moa, P. F., Herfindal, I., Kvam, T., and Andersen, R. (2002). Lynx depredation on sheep in Norway. J. Wildl. Manage. 66, 98–105. doi: 10.2307/3802876
OED (2018). Oxford English Dictionary. Available online at: http://www.oed.com.ezproxy.library.wisc.edu/ (accessed May 13, 2017).
Ohrens, O., Bonacic, C., and Treves, A. (2019a). Non-lethal defense of livestock against predators: flashing lights deter puma attacks in Chile. Front. Ecol. Environ. 17, 32–38. doi: 10.1002/fee.1952
Ohrens, O., Santiago-Ávila, F. J., and Treves, A. (2019b). “The twin challenges of preventing real and perceived threats to human interests,” in Human-Wildlife Interactions: Turning Conflict into Coexistence, eds B. Frank, S. Marchini, and J. Glikman (Cambridge: Cambridge University Press), 242–264. doi: 10.1017/9781108235730.015
Olson, E. R., Crimmins, S., Beyer, D. E., Macnulty, D., Patterson, B., Rudolph, B., et al. (2017). Flawed analysis and unconvincing interpretation: a comment on Chapron and Treves 2016. Proc. Roy. Soc. Lond. B 284:20170273. doi: 10.1098/rspb.2017.0273
Peebles, K., Wielgus, R. B., Maletzke, B. T., and Swanson, M. E. (2013). Effects of remedial sport hunting on cougar complaints and livestock depredations. PLoS ONE 8:e79713. doi: 10.1371/journal.pone.0079713
Pepin, K., Kay, S., and Davis, A. (2017). Comment on: “Blood does not buy goodwill: allowing culling increases poaching of a large carnivore”. Proc. Roy. Soc. B 284:e20161459. doi: 10.1098/rspb.2016.1459
Popescu, V. D., De Valpine, P., Tempel, D., and Peery, M. Z. (2012). Estimating population impacts via dynamic occupancy analysis of Before-After Control-Impact studies. Ecol. Appl. 22, 1389–1404. doi: 10.1890/11-1669.1
Popper, K. (1959). The Logic of Scientific Discovery. Abingdon-on-Thames: Routledge. doi: 10.1063/1.3060577
Poudyal, N., and Baral, N. T A.S. (2016). Wolf lethal control and depredations: counter-evidence from respecified models. PLoS ONE 11:e0148743. doi: 10.1371/journal.pone.0148743
Prugh, L. R., Stoner, C. J., Epps, C. W., Bean, W. T., Ripple, W. J., Laliberte, A. S., et al. (2009). The rise of the mesopredator. Bioscience 59, 779–791. doi: 10.1525/bio.2009.59.9.9
Rodriguez, S. L., and Sampson, C. (2019). Expanding beyond carnivores to improve livestock protection and conservation. PLoS Biol. 17:e3000386. doi: 10.1371/journal.pbio.3000386
Sacks, B. N., Blejwas, K. M., and Jaeger, M. M. (1999). Relative vulnerability of coyotes to removal methods on a northern California ranch. J. Wildl. Manage. 63, 939–949. doi: 10.2307/3802808
Sanders, J., Blundy, J., Donaldson, A., Brown, S., Ivison, R., Padgett, M., et al. (2017). Transparency and openness in science. Roy. Soc. Open Sci. 4. Available online at: https://royalsocietypublishing.org/doi/10.1098/rsos.160979
Santiago-Ávila, F. J. (2019). Survival rates and disappearances of radio-collared wolves in Wisconsin. Ph.D dissertation, University of Wisconsin-Madison, Madison, WI, United States.
Santiago-Avila, F. J., Cornman, A. M., and Treves, A. (2018a). Killing wolves to prevent predation on livestock may protect one farm but harm neighbors. PLoS ONE. 13:e0209716. doi: 10.1371/journal.pone.0209716
Santiago-Avila, F. J., Lynn, W. S., and Treves, A. (2018b). “Inappropriate consideration of animal interests in predator management: towards a comprehensive moral code,” in Large Carnivore Conservation and Management: Human Dimensions and Governance, ed T. Hovardos (New York, NY: Taylor & Francis), 227–251. doi: 10.4324/9781315175454-12
Schmidt, J. H., Johnson, D. S., Lindberg, M. S., and Adams, L. G. (2015). Estimating demographic parameters using a combination of known-fate and open N-mixture models. Ecology 56, 2583–2589. doi: 10.1890/15-0385.1
Stewart-Oaten, A. (2003). On rejection rates of paired intervention analysis: comment. Ecology 84, 2795–2799. doi: 10.1890/02-3115
Stien, A. (2017). Blood may buy goodwill - no evidence for a positive relationship between legal culling and poaching in Wisconsin. Proc. Roy. Soc. B 284:20170267. doi: 10.1098/rspb.2017.0267
Tompa, F. S. (1983). “Problem wolf management in British Columbia: Conflict and program evaluation,” in Wolves in Canada and Alaska: Their Status, Biology and Management, ed. L. N. Carbyn (Edmonton, AB: Canadian Wildlife Service, 112–119.
Treves, A. (2019a). “Peer review of the proposed rule and draft biological report for nationwide wolf delisting,” Department of Interior (Washington, DC: Department of Interior, U.S. Fish and Wildlife Service). Available online at: https://www.fws.gov/endangered/esa-library/pdf/Final%20Gray%20Wolf%20Peer%20Review%20Summary%20Report_053119.pdf
Treves, A. (2019b). Standards of Evidence in Wild Animal Research. Madison, WI: The Brooks Institute for Animal Rights Policy and Law.
Treves, A., Artelle, K. A., Darimont, C. T., and Parsons, D. R. (2017a). Mismeasured mortality: correcting estimates of wolf poaching in the United States. J. Mammal. 98, 1256–1264. doi: 10.1093/jmammal/gyx052
Treves, A., Browne-Nunez, C., Hogberg, J., Karlsson Frank, J., Naughton-Treves, L., Rust, N., et al. (2017b). “Estimating poaching opportunity and potential,” in Conservation Criminology, ed. M. L. Gore (New York, NY: John Wiley and Sons), 197–212. doi: 10.1002/9781119376866.ch11
Treves, A., and Bruskotter, J. T. (2014). Tolerance for predatory wildlife. Science 344, 476–477. doi: 10.1126/science.1252690
Treves, A., Krofel, M., and Mcmanus, J. (2016). Predator control should not be a shot in the dark. Front. Ecol. Environ. 14, 380–388. doi: 10.1002/fee.1312
Treves, A., Langenberg, J. A., López-Bao, J. V., and Rabenhorst, M. F. (2017c). Gray wolf mortality patterns in Wisconsin from 1979 to 2012. J. Mammal. 98, 17–32. doi: 10.1093/jmammal/gyw145
Treves, A., and Naughton-Treves, L. (2005). “Evaluating lethal control in the management of human-wildlife conflict,” in People and Wildlife, Conflict or Coexistence? eds R. Woodroffe, S. Thirgood, and A. Rabinowitz (Cambridge: Cambridge University Press), 86–106. doi: 10.1017/CBO9780511614774.007
Treves, A., Naughton-Treves, L., and Shelley, V. S. (2013). Longitudinal analysis of attitudes toward wolves. Conserv. Biol. 27, 315–323. doi: 10.1111/cobi.12009
Treves, A., and Santiago-Ávila, F. J. (in press). Myths assumptions about human-wildlife conflict coexistence. Conserv. Biol.
Treves, A., Wallace, R. B., Naughton-Treves, L., and Morales, A. (2006). Co-managing human-wildlife conflicts: a review. Hum. Dimens. Wildlife 11, 1–14. doi: 10.1080/10871200600984265
van Eeden, L. M., Eklund, A., Miller, J. R B., López-Bao, J. V., Chapron, G., Cejtin, M. R., et al. (2018). Carnivore conservation needs evidence-based livestock protection. PLoS Biol. 16:e2005577. doi: 10.1371/journal.pbio.2005577
Vial, F., and Donnelly, C. (2012). Localized reactive badger culling increases risk of bovine tuberculosis in nearby cattle herds. Biol. Lett. 8, 50–53. doi: 10.1098/rsbl.2011.0554
Vucetich, J. A. (2012). Appendix: the influence of anthropogenic mortality on wolf population dynamics with special reference to Creel and Rotella (2010) and Gude et al. (2011) in the Final peer review of four documents amending and clarifying the Wyoming gray wolf management plan. Congress. Fed. Reg. 50, 78–95. Available online at: https://www.federalregister.gov/documents/2012/05/01/2012-10407/endangered-and-threatened-wildlife-and-plants-removal-of-the-gray-wolf-in-wyoming-from-the-federal
Wielgus, R. B., and Peebles, K. (2014). Effects of wolf mortality on livestock depredations. PLoS ONE 9:e113505. doi: 10.1371/journal.pone.0113505
Woodroffe, R., and Ginsberg, J. R. (1998). Edge effects and the extinction of populations inside protected areas. Science 280, 2126–2128. doi: 10.1126/science.280.5372.2126
Keywords: effective, intervention, randomized controlled trials, experiments, predator control, standards of evidence, strong inference, wildlife damage
Citation: Treves A, Krofel M, Ohrens O and van Eeden LM (2019) Predator Control Needs a Standard of Unbiased Randomized Experiments With Cross-Over Design. Front. Ecol. Evol. 7:462. doi: 10.3389/fevo.2019.00462
Received: 08 July 2019; Accepted: 18 November 2019;
Published: 12 December 2019.
Edited by:
Vincenzo Penteriani, Spanish National Research Council (CSIC), SpainReviewed by:
Viorel Dan Popescu, Ohio University, United StatesEmiliano Mori, University of Siena, Italy
Copyright © 2019 Treves, Krofel, Ohrens and van Eeden. This is an open-access article distributed under the terms of the Creative Commons Attribution License (CC BY). The use, distribution or reproduction in other forums is permitted, provided the original author(s) and the copyright owner(s) are credited and that the original publication in this journal is cited, in accordance with accepted academic practice. No use, distribution or reproduction is permitted which does not comply with these terms.
*Correspondence: Adrian Treves, atreves@wisc.edu