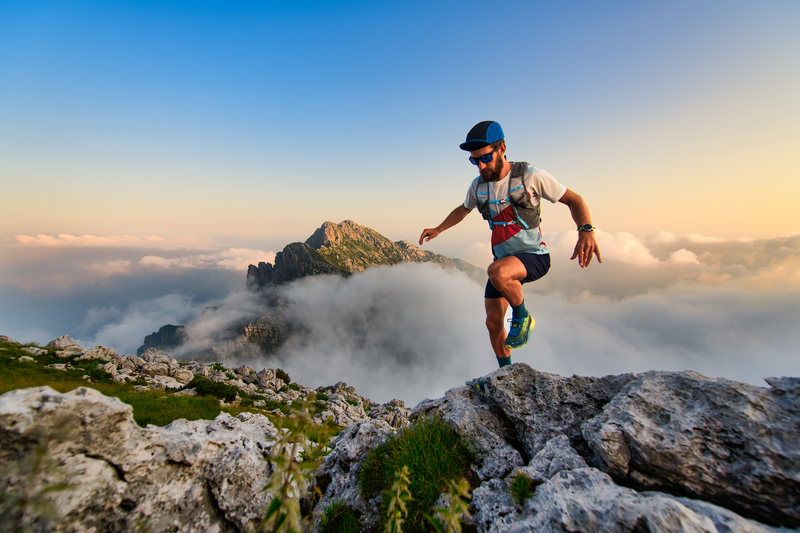
94% of researchers rate our articles as excellent or good
Learn more about the work of our research integrity team to safeguard the quality of each article we publish.
Find out more
HYPOTHESIS AND THEORY article
Front. Ecol. Evol. , 22 October 2019
Sec. Environmental Informatics and Remote Sensing
Volume 7 - 2019 | https://doi.org/10.3389/fevo.2019.00399
This article is part of the Research Topic Evidential Statistics, Model Identification, and Science View all 15 articles
For at least the past 25 years or so, there has been a twofold sense of “crisis” in ecology. One indication of this is the spate of articles and books calling for a reformation of the discipline and bearing such titles as “The New Ecology.” On the part of practitioners, the unease concerns its theories, concepts, and methods. On the part of the general public, the unease concerns the perceived “bias” of its results. This paper is an attempt by two philosophers of science to clarify one critical methodological issue—hypothesis/model testing—and in the process to identify ways to gird the objectivity of ecological claims. What is significant about our approach is a distinction between the tasks appropriate to Bayesian Inference and Evidential Statistics—confirming hypotheses on the one hand and measuring evidence for models on the other. These two inferential paradigms are contrasted with the testing methods long-dominant in the discipline—Fisher-Neyman-Pearson Significance Testing and Popper Falsificationism—and a case made for a much greater use of Bayesian and Evidentialist Methods. In particular, it is argued that Evidential Statistics, here in the form of the likelihood ratios of competing predictive and explanatory multiple models avoids the main forms of otherwise unsettling cognitive bias. It also provides a Darwinian alternative to the “convergence” accounts of objectivity associated with the development of physics which is more appropriate to ecology.
Twenty-five years ago, Shrader-Frechette and McCoy (1993) wrote that
On the whole, general ecological theory has, so far, been able to provide neither the largely descriptive, scientific conclusions often necessary for conservation decisions, nor the normative basis for policy.
Judging by the titles of more recent textbooks, and despite an immense amount of very interesting ecological research and theorizing carried out in the meantime, the situation appears basically unchanged. These books bear such titles as Scientific Method for Ecological Research (Ford, 2000), Ecological Understanding: The Nature of Theory and the Theory of Nature (Pickett et al., 2007) and The New Ecology: Re-Thinking A Science for the Anthropocene (Schmitz, 2017). All are premised on the complex claim that there is as yet little consensus on either the correct theoretical structures or the proper experimental/inferential methods of the subject; the result is that ecological science has not yet had the desired and necessary influence on policy formation and implementation.
Ford, for example, begins the final chapter of his book with a list of criticisms that he takes seriously. After all, they provide the motives for developing what he takes to be a new and improved approach.
i) There has been a lack of progress in ecology.
ii) No general theory has emerged.
iii) Ecological concepts are inadequate.
iv) Ecologists fail to test their theories.
Picket, Kolesa, and Jones echo the discontent. In their view, at least part of the problem stems from the fact that the great growth of ecological information has occurred in ever-more Balkanized sub-disciplines, each with its own assumptions, concepts, methods, and hypotheses. Hence, the progress made has been (in their word) “narrow,” focusing on specific scales and levels of organization, and making communication between sub-disciplinarians, not to mention with the general educated public, increasingly difficult. There is no larger and consistent picture on which to get a grip, no uniform set of methods to employ, and (although they do not put it this way) no firm basis on which to formulate, much less implement, coherent public policies–in particular regarding the multi-scale impacts of human actions on specific plant and animal populations. As with these other authors, Schmitz tries to provide a new and more implementable picture.
One source of the discontent both with and within ecology is the relative absence of understanding the role and scope of the methods used to test ecological hypotheses and models. The authors of this paper have been invited to expand this understanding by placing it in a larger philosophical perspective.
On October 3, 2018, the environment, development, and agricultural heads of the United Nations issued a joint statement declaring that
Forests are a major, requisite front of action in the global fight against climate change – thanks to their unparalleled capacity to absorb and store carbon. Stopping deforestation and restoring damaged forests could provide up to 30% of the climate solution (Da Silva et al., 2018).
All well and good. On the assumption (on which more later) that climate change is (to a significant degree) human-induced, and given that we have every reason to resist it, we need to stop deforestation and restore damaged forests. The rational place to begin is with a factual assessment of the situation. The immediate problem is that “there are two main data sources for tree loss, and they are increasingly contradictory” (Pearce, 2008). One source is the Global Forest Watch (GFW). Its data are compiled from satellite images by the World Resources Institute. These data indicate a decline in tree cover in 2017 of 72.6 million acres, almost 50% more than in 2015. The other source of deforestation data is the Global Forces Resource Assessment (FRA), which is based on government inventories compiled by the UN Food and Agricultural Organization. It estimates the annual loss at just 8.2 million acres, and adds that deforestation rates have declined by more than 50% since 2008. In individual countries the data-inconsistency is even more dramatic, the FRA showing forest gains in the US, for instance, while the GFW indicates big losses.
In this case, the data-inconsistency can be explained in terms of the types of data gathered—Landsat tree-cover images as against government-designated land uses—employed by the two organizations. More inclusive and sophisticated models are being developed1. But it is not at all clear whether they will reinforce the on-going attempt to protect intact forests or put the emphasis on re-growing temporarily degraded areas. The correct policy perspective depends, at least to an important extent, on the time-scale chosen. Once-deforested areas in New England are now overgrown with trees.
Even when there is a clear consensus among scientists about both fact and policy, the general public is often slow to follow. Yale Environment 3602 ran the headline, “Americans Who Accept Climate Change Outnumber Those Who Don't 5-1” on April 4, 2018, but a closer look at the survey numbers indicates that no more than 58% believe that global warming is mostly caused by human factors, and no more than 49% (2% less than in 2008) are “extremely” or “very” sure that it is really happening. Again according to the Yale survey, only 6% of the population believes that anything much can be done to slow or reverse it.
There are, of course, many reasons for the discrepancy between expert and popular opinion. Some of them are familiar—politics, economics, spatial and temporal scales. But what runs through all of them is distrust, sometimes of “science” generally, on religious or other cultural grounds, more often of ecology or similarly policy-connected disciplines. The main brief against them is that their research is often “biased,” aligned in one way or another with “liberal” or “environmentalist” agendas. In one word, ecology and its brethren are not “objective,” and for that reason not to be taken seriously. This is disturbing not only from a policy perspective, but also because a good percentage of ecological research is government-funded and depends on broad political support.
It is to be expected, then, that ecology textbooks would concentrate as they tend to do on questions concerning objectivity—how it is to be understood and obtained. Since the hallmark of and the means by which it is ensured, at least in our culture for the past several hundred years, has been the “scientific method,” much of the discussion in these books quickly focuses on it. The discussion of method, in turn, is deeply informed by the philosophy of science3.
But can philosophical reflection aid ecologists in either their methodology or their communication with the public? Our aim is to answer the question affirmatively by focusing on the objectivity of the claims that ecologists make. Objectivity in turn has to do with the methods by which these claims are tested. This is the nub of the controversies surrounding ecology as regards both its scientific status and reliable source of informed public policy. It is also the way in which the indispensability of philosophical reflection can best be demonstrated. A brief review of the testing methods already in widespread practice should provide context, and a distinction between the concepts of confirmation and evidence add clarity.
At present, inferential methods are routinely characterized within one or another statistical framework. It was not always thus. Discussions of theory-testing, at least among philosophers interested in the subject, were dominated in the middle years of the twentieth century by the so-called Hypothetico-Deductive or H-D model. On this model, to test a hypothesis is, schematically, to derive a statement, via initial and boundary conditions4, describing an observation. If the derivation is carried out before the observation is made, it is predicted; if detected or measured, the hypothesis is confirmed. If the derivation is carried out after the observation has been made, the hypothesis retrodicts and explains it. The underlying point remains the same: to test a theory is to derive statements describing observations or, ideally, experimental results. If verified, belief that the theory is true has to some indeterminate degree been justified. There were several variations on the H-D model, for example Hempel's view (1965) that not the observational consequences but the instantiations of empirical hypotheses justified or confirmed belief in them, but the leading theme remained untouched, that the credibility of scientific claims rested on successful prediction/explanation and that prediction/explanation in turn could be characterized by a simple deductive relationship between hypotheses and observations or (to use a more bracing and embracing term) data5.
A relatively early and interesting case for the H-D model as a reliable way of testing wildlife, and by extension ecological, hypotheses generally, was made by Romesburg (1981), although in doing so he departed from the Positivist original in a significant way. On his account, wildlife science was dominated into the 1980's, although in his view wrongly, by the methods of “induction” and “retroduction.” On Romesburg's somewhat non-standard use of the terms, the former involves correlating variables, the amount of edge vegetation in fields, say, with an index of game abundance; the greater the degree of observed correlation, the more reliable the hypothesis linking the variables. The latter (retroductive) method involves providing an explanation of the observed linkages simply by providing a generalization from which all of them can be derived.
According to Romesburg, the major difficulty with both methods is that they are used to generate rather than to test hypotheses. In his view, a subsidiary difficulty with the inductive method is that it wrongly assimilates correlation to causation; that two variables are usually, if not also invariably, conjoined does not by itself demonstrate a direct (or directional) causal connection between them6. A reliable hypothesis must in one way or another explain the connection, it must provide a reason for and not simply “fit” it. A subsidiary difficulty with the retroductive method is that it is tied closely to the facts that it is invoked to explain; it doesn't provide a way of ruling out incompatible hypotheses that explain all the same facts. Although Romesburg doesn't put it this way, one might say that the inductive method leads to predictive but not explanatory hypotheses, the retroductive method to explanatory but not predictive hypotheses and that any adequate (“reliable”) hypothesis must be both explanatory and predictive. It is only if they satisfy both criteria that hypotheses are testable. In a Positivist vocabulary, induction and retroduction are methods of “discovery,” not “justification,” and discovery is methodologically moot; for the most part, adequate hypotheses are invented, products of insight and imagination. Justification alone has its own logic7.
The distinction between discovery and justification is classically Positivist, Romesburg's distinction between prediction and explanation8 is not. On the original H-D model, prediction and explanation are asymmetrical only with respect to the time, before or after the fact, when the derivation of an observational consequence is carried out9. Romesburg's case for restricting the model to explanatory, which is to say causal, hypotheses (although he does not frame it as a restriction) rests on the close connection he posited between wildlife science and public policy; it is only when “cause-effect relationships among variables are found [that] control [of outcomes] is possible” (p. 304).
The difficulties with the structural identity of prediction and explanation aside, a number of criticisms were later made of the H-D model (or better: account) of theory-testing. Three of these criticisms proved to be of special significance, not only because they undermined the H-D account, but more importantly because they led to alternative and very fruitful testing accounts. The first criticism was that the H-D account is no more than qualitative. It provides necessary and sufficient conditions for the truth of “D confirms H,” but without a rule for determining the degree to which it is able to do so. This is troubling. An adequate account of confirmation should capture the universally-held belief that some hypotheses are (perhaps much) better supported by the available data than others, and be able to measure the difference. The second criticism of the H-D account was that while it indicates a logical relationship, usually entailment, between hypothesis and data, it does not specify an inverse relationship, neither entailment nor any other, between data and hypothesis, no way, so to speak, to retrace the bottoms-up route. The third main criticism was that the H-D account is, without further modification and amendment, restricted to non-statistical hypotheses, typically illustrated by universal generalizations of the form “All A are B.” That is, from a simple statistical hypothesis of the form “Pr(B|A) = r” it does not follow logically that a description of A entails a description of B. In fact, it doesn't follow that the probability of an instance of B given an instance of A is equal to r. In such cases, the relationship between hypothesis and data must be inductive and characterized in probabilistic terms.
One way to lump all three of these criticisms together is to say that the hypothetico-deductive account had some serious gaps in it. The option was to fill the gaps and in the process reconfigure the structure of scientific testing. Several alternative and gap-filling accounts have been proposed. The first is deductive in character, the three others are statistical.
The first alternative was set out by Popper in his classic The Logic of Scientific Discovery (Popper, 1934/1959). His approach was striking both in its ease of application and intuitive appeal10: Re-construe the H-D account in such a way that there are no gaps to fill. In Popper's view, this is fortunate since there is no way in which they can be filled coherently in any case. His point of departure was the fact that while a hypothesis can never be “confirmed,” it can be falsified. The point is purely logical. No number of confirming instances, no matter how great, can ever guarantee that a universal generalization is true. Yet a single disconfirming consequence will show, other things being equal, that the generalization is false in a deductively straightforward way. It doesn't follow from the fact that any number of swans are white that all swans are, but it does follow from the fact that there is a black swan11 that the generalization concerning them is false. Moreover, in the case of falsification there are no gaps to fill, no new relationship between data and hypothesis to be discovered or invented, no need to add probabilistic operators and rules governing them to our traditional methods. The rule of modus ponens—if p then q and ~q, therefore ~p–by itself suffices as the “logic,” not so much of justification (for there is no such thing according to Popper) as of scientific discovery.
Popper reinforces his proposal by way of a reflection on actual scientific practice. Scientists do not keep repeating the same experiments in the attempt to pile up confirming consequences of a hypothesis (although they do attempt to diversify the conditions with respect to which these consequences are derived). Once an experiment has been performed, and replicated by others, they move on to other ways in which to test the hypothesis. But, Popper contends, to (really) test a hypothesis is to find new ways to falsify it, other kinds of data. Since no hypothesis can ever be established as true, the best one can say of a particular hypothesis is that it has survived a number of tests, the more varied and severe the better. A hypothesis which has so survived is said to be corroborated, i.e., has not been shown to be false. In science as in the biotic community, the fittest survive. The idea that biotic communities are self-regulating, that there is “a balance in nature,” is an old, indeed ancient, ecological truism. Yet it has been shown over the last 30 years or so that the assumptions on which such equilibrium rests do not hold generally12. This is, at least according to the conventional wisdom, what characterizes the scientific mind: never to accept some truth as given, but to question it constantly.
Popper is right to stress the “testing” intuition13. But whatever logical advantage a program of principled falsification enjoys is no more than apparent. The French physicist, philosopher, and historian of science, Duhem (1962) was perhaps the first to emphasize that hypotheses are never tested in isolation, but only in conjunction with other hypotheses and appropriate initial and boundary conditions14. A negative result does not by itself show which of these hypotheses or conditions is false. To put it another way, the logical asymmetry to which Popper draws attention is matched by another: a confirming prediction confirms all of the hypotheses and conditions from which it follows; a falsifying observation does not similarly falsify all of the hypotheses and conditions from which it follows15.
Finally, the Popperian methodology shares an important difficulty with H-D accounts generally. Both are premised on the assumption that hypotheses take the form of universal conditionals. But it is often the case, perhaps almost always in ecology, that hypotheses have a probabilistic or statistical form. We have already referred to the difficulty in deducing observational consequences from such hypotheses. The falsifiability criterion is similarly tailored to “All A are B” examples. It cannot deal effectively with the multi-factor multi-causal hypotheses typical of ecology. All of this said, it must be added at once that Popper's methodology has not itself been “falsified.” A great deal of valuable research has been carried out by ecologists in attempts to follow Popper's guidelines (albeit substituting “rejection” for falsification properly so-called, as is necessarily the case when hypotheses do not take the form of universal conditionals)16.
In brief, problems with Popper do not show that all of the research done in his name is either misguided or without value. They do prompt us to look for other accounts of hypothesis testing that avoid failures in Popper's own. It is in any case a mistake to fix on one method as uniquely satisfactory. Different testing methods are appropriate as different types of research questions are asked.
Significance or error-statistical testing in fact pre-dated the H-D model, both as regards its initial formalization and its widespread acceptance among ecologists. The latter undoubtedly had to do with the fact that ecological generalizations, even those taken as lawlike, are for the most part statistical in character. It involves a procedure not unlike Popper's. That is, it provides a way of rejecting (not falsifying) hypotheses and at least indirectly provides support for their alternatives. Variants on this testing theme are associated with Fisher, Neyman, and Pearson. Since it is so well-known among ecologists, to the extent that significance testing is virtually synonymous with “statistical testing,” and even “testing” tout court, there is no need for much detail. It suffices to point out in a very broad way why it is inadequate, and then to discuss briefly its recent redeployment by the philosopher Mayo (1996; 2018) and Mayo and Spanos (2010).
On its Fisher variant, the viability of a hypothesis is probed by comparing an observed result with the distribution of results predicted by the hypothesis. That is, any hypothesis (typically described as “null”) is rejected if an observed result (and results more deviant) would be predicted by the hypothesis with a low probability (P-value). Commonly, a result is judged “significant, if it is of such a magnitude that it would have been produced by chance not more frequently than once in twenty trials. This is an arbitrary, but convenient, level of significance for the practical investigator” (Fisher, 1929, p. 191), viz., no more than 5% of the time. On the other hand, if results as or more deviant than the observed results would be predicted more than 5% of the time, the proper “Fisherian” conclusion is not to accept the hypothesis, but to recognize a failure to reject the hypothesis. The obvious problem is that any number of otherwise incompatible hypotheses in the same area of research could predict the results and in this very general sense be confirmed17. The Fisher singular hypothesis account is too weak to discriminate them.
On the Neyman-Pearson variant18, “the only valid reason for rejecting a statistical hypothesis is that some alternative hypothesis explains the observed result with a greater degree of probability” (Pearson, 1938). One of the hypotheses compared is invariably in practice if not also in theory “null,” and the commonly accepted significance level continues to be conventionally set at 0.05, however arbitrary the number. In essence, an NP test is a Fisherian test of the null hypothesis using a test statistic designed to maximally differentiate between the two hypotheses. Usually, this statistic is the likelihood ratio for the two models or its logarithm.
The NP approach differs from Fisher's view in a second respect as well. The Fisherian test has an inductive and rather open-ended character. The Fisherian P-value is just something for the scientist to think about when trying to come to grips with nature. The NP test on the other hand is set up to be a clear-cut decision procedure. A critical level (designated α) for the P-value of the null hypothesis is set a priori, with the result that you either accept the null hypothesis or accept the alternative. An artifact of the black or white nature of NP testing is that small differences in the data can make large differences in inference. While in a properly interpreted Fisherian test, the difference between a P-value of 0.051 and 0.049 makes very little difference, in a properly interpreted NP test, if the critical level has been set to 0.05, this small difference makes a great deal of inferential difference.
NP analysts realize that their procedure makes mistakes. Neymann and Pearson distinguished two types of errors: Type I (rejecting a true null hypothesis) and Type II (accepting a false null hypothesis). They console themselves with the belief that they can both measure and control the rate of errors. In fact the magnitude of those error rates is the sole measure of the validity of NP test inferences.
A cryptic consequence of NP test construction now emerges. The calculation of error rates is tightly bound to the assumption that one or the other alternative is true. If the data are generated by some process other than one of the two alternative hypotheses, the calculation of error rates may be deeply disrupted (see Dennis et al., 2019, for a detailed analysis of this problem).
Thus, while the inference from Fisherian tests may be too weak, the inference from NP tests may be too strong. As pointed out by Chatfield (1995), analyzing the wrong models is likely to be the greatest source of error in statistical analysis. Further errors are often made, in part because many ecological hypotheses lack measurable power and precision, in part because of the number and complexity of the variables to be taken into account in the case of field observations. The result, or so outsiders often agree, is a widespread lack of confidence in significance testing generally19. On both variants, it is too easy to attribute biological to mere statistical significance.
Mayo attempts to bolster confidence in error-statistical testing by imposing Popper-like severity constraints on it. However, there are at least two ways her account differs from Popper's. First, hers is probabilistic, his deductive. Second, she wants to “go smaller” and focus on testing individual statistical hypotheses; his focus is on testing “global theories” like Newton's and Einstein's. On Mayo's account, an adequately stringent test combines weak and strong severity principles. The weak principle has two key features. One is that a severe test is such that the probability is low that the test procedure would pass a hypothesis subjected to it if the hypothesis were false. The other feature is that the probability that the data agree with the alternative hypothesis is very low. On the strong severity principle, data provide good evidence for a hypothesis if it passes the severe test procedure, that is, is in agreement with the data. Like Popper, Mayo emphasizes that the more severe the test, the greater its probative value. She also shares with him the assumption that hypotheses may be tested individually, in a non-comparative context (or rather, that the test is always with respect to a hypothesis and its negation). But this assumption introduces the potential for bias, not simply by way of adding auxiliaries to it so as to square the hypothesis with the data once errors have been detected in it, but also by leaving out of account that other hypotheses might be better supported (more severely tested) by the same data. To alleviate this problem, Mayo and Spanos (2004) advocate “misspecification testing,” but this only helps for misspecifications that can be conceived of.
A third and increasingly influential option to the H-D model has been to fill the gaps in it by providing an inverse characterization of the way in which data directly confirm or otherwise support hypotheses. It does so by supplementing deductive logic with the full resources of probability theory and is known as Bayesian Inference.
The first gap in the H-D model is the absence of any way of determining both the means by which and the extent to which data confirm a hypothesis. The gap is filled by Bayes Theorem, so-named after its eighteenth century originator. The Theorem is easily derived from the axioms of probability theory together with a definition of conditional probability. The probabilities within it are interpreted as measures of belief. It states that if the probability of the data is not equal to zero, the posterior probability of the hypothesis is equal to its prior probability, i.e., the willingness of particular agents to bet that it is true, before new or additional data bearing on it have been gathered, multiplied by the probability of the data given the hypothesis divided by the “expectedness” of the data, i.e., the marginal probability of the data averaged over the hypothesis and its alternatives. More compactly,
• Pr(H|D) = [Pr(H) × Pr(D|H)]/Pr(D).
On the Bayesian account, to confirm (disconfirm) a hypothesis is just to raise (lower) its prior probability, viz.,
• D confirm H just in case Pr(H|D) > Pr(H)20.
This measure of confirmation is qualitative. There are alternative ways to measure the degree to which a hypothesis is confirmed, but a common metric is in terms of the difference between the prior and posterior probabilities, Pr(H|D) – Pr(H). Whether the degree is “high” or “low” depends on the particular confirmation measure chosen, the implicit standards of disciplinary scientific communities, and the research purposes of the investigator.
It follows as an immediate corollary of the Bayesian account of confirmation that it applies to probabilistic or statistical hypotheses as much as it does to universal conditionals, thus filling a second gap left open by the H-D and falsification accounts.
The third “gap,” if such it can be called, left open by the H-D and its falsification variant is that they provide no way on the basis of which to choose hypotheses to put to experimental test. For traditional H-D theorists, they have no particular advance rationale, for Popper they are merely “conjectures” on an individual scientist's part, the bolder the better. But as Aaron Ellison, one of a rather small number of ecologists in the 1980's to urge adoption of Bayesian methods, puts it (Ellison, 1986),
We rarely, if ever, test all possible hypotheses, and most of us use substantial prior knowledge about the behavior of a system in designing our experiments. Unlike classical frequentist statistical practice, Bayesian inference requires the investigator to state assumptions explicitly and use pre-existing information quantitatively to define the prior distribution or hypothesis (p. 1041).
In what are sometimes referred to as “empirical” or “standard” applications of Bayes Theorem, the prior probability distributions are estimated on the basis of observed relative frequencies in the data. In non-standard cases, the distributions are a function of the ecologist's previously acquired beliefs (including hunches and intuitions) about the object of investigation.
The fourth and final gap, very much underscored by Ellison, is that Bayesian inference lends itself in a uniquely transparent way to adaptive management and environmental decision-making. On the one hand, just as Bayesian agents begin, as most of us in fact (and rather unconsciously) do, with an initial probability distribution over plausible hypotheses and expected outcomes, up-dating and re-adjusting the distribution as data accumulate, continually learning from experience21, so too (ideally) adaptive land and wildlife managers treat decisions as hypotheses to be tested, choosing them where possible on the basis of past experience, and modifying them as necessary in the light of the observed outcomes to which they lead. To manage adaptively is to learn from experience, to acknowledge the inevitability of uncertainty is to be open to policy changes as additional data are brought to bear on policies already in place. That the degree of uncertainty with which initial decisions are made can be measured and then re-evaluated as time goes by, moreover, reassures the public that policy shifts are never arbitrary or capricious, and nearly always open to revision.
On the other hand, the usual decision-protocols routinely use Bayes Theorem to calculate optimal courses of action on the basis of the probability of outcomes and their respective utilities. A rational agent—manager, politician, or citizen—chooses the course of action that maximizes the product of the (posterior) probability of its outcome and its expected utility. This is as should be expected. We act rationally in such a way as to maximize our desires (utilities) given that we have particular beliefs (probabilities) concerning the future, at least insofar as our actions are intentional22.
Ellison admits that
Not all ecologists … appreciate the philosophical underpinnings of Bayesian inference. In particular, Bayesians and frequentists differ in their definition of probability and in their treatment of model parameters as random variables or estimates of true values. These assumptions must be addressed explicitly before deciding whether or not to analyze ecological data (Ellison, 2004, p. 509).
Agreed: the assumptions must be addressed. In brief, (a) the decision whether or not to use Bayesian methods depends on the type of research question being asked, (b) there are several clear differences between these types, and (c) an unaided used of Bayesian methods does not ensure the objectivity rightly held to follow from an appropriate use of “scientific method(s).”
There are various types of research question23. One is: given a datum, what should I believe, and to what degree? This question has to do with the confirmation of my beliefs. A second question is: what kind of evidence does the datum provide for one hypothesis as against another, and how strong is the evidence? Admittedly, “confirmation” and “evidence” are used interchangeably; D is often taken as evidence for H just in case D confirms H. But they should be distinguished rather sharply. Their conflation is the source of a great deal of error in philosophy, statistics, and perhaps also in the practice of science. Intuitively, confirmation is agent-dependent in the sense that a hypothesis is confirmed if and only if the agent's degree of belief in it is raised. Incorporating as it does an agent's belief, it in this same sense subjective. Evidence in the narrow technical sense used here, a relation between the likelihoods of data/models, however, is agent-independent; it has to do not with raising agents' prior degrees of belief in a hypothesis on the basis of the data subsequently collected, but in assessing the relative probability of the data under one hypothesis as opposed to under another. It is in this sense, objective, incorporating a logical and belief-free relation between data and hypothesis. It is also intuitively comparative. Evidence consists of data more probable on one hypothesis than on another. The greater the likelihood ratio, the stronger the evidence. In contrast, hypotheses are confirmed one at a time as the probability of (belief in) their truth is raised (strengthened).
The same idea, that “confirmation” and “evidence” vary conceptually, is perhaps best illustrated by “crucial experiments.” Such experiments discriminate one equally-well confirmed hypothesis from another and at the same time provide evidence for one as against the other. Although Darwin's explanation of evolution by way of natural selection had been generally accepted by that time, it remained an open question in the early 1940's whether mutations among bacteria occur as either an adaptive response to an environmental stimulus (an instance of the Lamarckian theory of the heritability of acquired characteristics) or randomly (in which case they are transmitted to the next generation as a function of reproductive fitness). Both theories had their defenders. In what is arguably the most famous single experiment in the history of ecology/evolutionary biology, Luria and Delbruck devised a way to test the two hypotheses (Luria and Delbruck, 1943). They exposed a number of parallel cultures to viruses known as phages, “subcellular parasites that infest, multiply within, and kill bacteria.” On the Lamarckian theory, bacteria adapt to their phage environment; hence, the number of mutations that occur should be both relatively small and constant across bacterial cultures. The Darwinian theory, that phage-resistant mutations occur randomly and prior to exposure entails that the number of phage-resistant mutations should vary dramatically from one culture to the next, and since available earlier in the process, the mutations accumulate much more rapidly where they occur in lines before phage exposure.
Slightly more precisely, Delbruck and Luria hypothesized that if phage-resistant mutations occurred after exposure, the number of survivors would approximate a Poisson distribution, on which the mean would equal the variance. What they found was that the variance was much greater than the mean. They then drew the Darwinian conclusion (as did the rest of the biological community) that bacterial mutations are indeed random, as are macro-organism mutations, rather than post-adaptive or “directed.”
This is what evidence does, allows us to discriminate in a straightforwardly objective way between hypotheses that may be otherwise equally well-confirmed. As we have just seen, there are cases in which there is strong evidence for one of a pair of equally well-confirmed hypotheses. There are also cases in which there is no such evidence25, cases in which the evidence is strong and the degree of confirmation low, and so on.
We can make this account of evidence more precise. It involves the comparison of the merits of two models, M1 and M2 (possibly, but not generally ~M1) relative to the data D and background information B.
• D is evidence for M1 as against M2 just in case Pr(D| M1) > Pr(D|M2)26.
This is often called the Likelihoodist (LR) account of evidence. It follows at once that “data” are to be distinguished from “evidence.” Data constitute evidence only with respect to models in a well-defined comparative context27. To put this more precisely, evidence is a data-based estimate of the relative discrepancy of two models to the generating process. In the case of simple models, the log-likelihood happens to be an estimate (up to a constant) of the Kullback-Leibler discrepancy between the generating process and a model. As with the original confirmation account, this formulation is qualitative. A commonly-used measure of the degree of evidence vis-à-vis a model comparison is the numerical ratio of the likelihoods28. Note in this connection that if 1 < LR ≤ 8, then D is often held to provide “weak” evidence for M1 as against M2, while when LR > 8, D provides “strong” evidence for M1 as against M2. Note also the shift from talking about “hypotheses” and “theories” to talking about “models.” Hypotheses are often formulated in verbal rather than mathematical terms and they rarely provide potential and predictive data-distributions. They can be either true or false. Models are mathematically-formulated and idealized data-generating mechanisms. They can generate data-distributions near or far from what might be termed the “naturally” generated distributions. Observed data or experimental results support model1 over model2 if the potential/predictive data generated by the first are by some agreed-upon measure closer to the observed data than the potential data generated by the second. Differences of information criteria are estimates of KL discrepancy differences that adjust for biases caused by estimation.
Although the terms have been used rather carelessly to this point, “hypothesis” is more helpfully associated with Bayesian inference, “model” with Evidential testing, leaving to the side any questions concerning how either relates to “theory” (which intuitively includes both hypotheses and models as components, links them by way of explanatory principles and basic concepts, and affords successful prediction of data in a wide variety of sub-disciplines; force is such a basic concept and the laws of motion the explanatory principles in classical physics, natural selection, and adaptation the basic concepts and explanatory principles in Darwinian biology)29.
Evidence and confirmation thus characterized differ in a number of important respects, some of which have already been mentioned. Data can provide a high or low degree of confirmation to a hypothesis while at the same time providing weak or strong evidence for it in a comparative context. This is to say that while D confirms H if and only if D constitutes evidence for M with respect to ~M30, there is no linear relationship between their respective degrees31. Moreover, given the way in which they are quantified, degrees of confirmation can vary between 0 and 1 exclusive, while numerical values of evidence on a likelihood ratio can range from 0 to ∞ inclusive (or -∞ to ∞ in the case of the log-likelihood ratio).
The distinction between confirmation and evidence is indispensable for both theory and practice, and not often made. But it also bears directly on the public understanding of the way in which science informs policy formation. Simply put, controversy with sweeping social, political, and economic consequences sometimes arises, to one extent or another, from failure to draw it.
A sample controversy has to do with the anthropogenic “global warming” hypothesis, that is, the hypothesis that present warming trends are human-induced. If it does not raise questions concerning foundational physical theories, it does with respect to their application32.
A wide spectrum of data raises the posterior probability of the hypothesis, in which case they confirm it. Indeed, in the view of most climatologists, this probability is very high. The Intergovernmental Panel on Climate Change contends that most of the observed temperature increase since the middle of the twentieth century has been caused by increasing concentrations of greenhouse gases resulting from human activity such as fossil fuel burning and deforestation. In part this is because the reasonable prior probability that global warming is human-induced is very high. It is assigned not on the basis of relative frequencies so much as on the explanatory power of the models linking human activity to the “greenhouse effect,” and thence to rising temperatures. In part, the posterior probability of the hypothesis is even higher because there are so many strong correlations in the data. Not only is there a strong hypothesized mechanism for relating greenhouse gases to global warming, this mechanism has been validated in detail by physical chemistry experiments on a micro scale, and as already indicated there is a manifold correlation history between estimated CO2 levels and estimated global temperatures. Of course, some climate skeptics emphasize how difficult it is to get standardized and reliable data for such a long period of time and from so many different places, others point out that it has not always been true that changes in CO2 levels precede changes in temperature. But the main skeptical lines of argument are that (a) the likelihood of the data on the alternative default (certainly simpler) hypothesis, that past and present warming is part of an otherwise “natural” and long-term trend, and therefore not “anthropogenic” is just as great, (b) that the data are at least as likely on other, very different hypotheses, among which solar radiation and volcanic eruption, (c) that not enough alternative hypotheses have been considered to account for the data. That is, among credible climate skeptics there is some willingness to concede that burning fossil fuels leads to CO2 accumulation in the atmosphere and that carbon dioxide is a greenhouse gas that traps heat before it can escape into the atmosphere, and that there are some data correlating a rise in surface temperatures with CO2 accumulation. But, the skeptics continue, these correlations do not “support,” let alone “prove,” the anthropogenic hypothesis because they can be equally well accounted for on the default, “natural variation” hypothesis or by some specific alternative. Since there is very little evidence for the hypothesis, it is not, the skeptics conclude, very well confirmed (and for this and other reasons massive efforts to reduce carbon emissions are a costly mistake). But this conclusion rests on a conflation of evidence with confirmation, and provides a striking reason why it is necessary to distinguish the two.
Data are evidentially relevant only if they discriminate hypotheses, and such data in the case of human-induced warming have been difficult to come by. That fact has premised at least part of the skeptics' argument that the rise in atmospheric CO2 comes from, e.g., the ocean, and is therefore “natural,” at the very least as likely a cause of the greenhouse gases responsible for temperature rise as the human-induced explanation. Such data have, however, been identified increasingly33. For example, most carbon atoms have an atomic mass of 12, but about 1% has an atomic mass of 13. Both kinds can form CO2 molecules, 12CO2 and 13CO2, distinguishable in the laboratory. To put a complex story very simply, it can be shown that if the CO2 atmosphere comes from the surface (and not the depths) of the ocean, then 13CO2 will increase over time. If the CO2 comes from fossil fuel burning, then the relative abundance of 13CO2 to 12CO2 will decrease. Experimental results show that the 13CO2/12CO2 ratio is decreasing, evidence for the hypothesis that fossil fuels rather than surface water is mainly responsible for rising levels of CO2 in the atmosphere, and hence (on the assumption that rising levels of CO2 are a cause of rising temperatures) for the anthropogenic hypothesis.
Two crucial differences between confirmation and evidence have been alluded to but must be underlined. First, confirmation is psychological in character, involving as it does changes in an agent's personal degree of belief that a hypothesis is true. Evidence is logical in character, an agent-independent relationship between models and data34. It follows not only that confirmation is “kinematic,” beliefs re-adjusted over time as data are accumulated, evidence “static,” an atemporal as well as impersonal relationship between models and data, but also that the probability operators in their respective accounts are not to be interpreted in the same way. Confirmation tracks changes in belief and thus degrees of uncertainty in an agent's mind. Evidence has to do, rather, and as already noted, with a logical relation. The former probabilities are psychological and in this sense “subjective,” the latter formal and for this reason “objective.”
It would seem to follow that since the credibility of their claims depends on the extent to which they are objective, the method of model statistics should be preferred by practicing ecologists. But this is not the end of the matter. On the one hand, traditional, i.e., self-described “subjective Bayesians” make a case for the objectivity of their method of testing hypotheses. On the other, so-called “objective Bayesians” both curb the source of subjectivity in applications of Bayes Theorem and play down if not also discount completely the subjective/objective distinction as an unwanted philosophical distraction. Since both approaches are increasingly popular, each must be examined.
Bayesian inferential techniques inform decision-making processes. They do so by way of the fact that decisions are to be explained in part in terms of their beliefs and desires. This is to imply that whether the decisions themselves are good or bad is agent-dependent. It is but a short natural if not also logical step to conclude that they are all, even research decisions, “biased.” One much-discussed example is “confirmation bias,” focusing one's efforts on finding data that confirm one's beliefs and thus potentially misrepresenting what is in fact the case35. Meta-studies of reported ecological claims provide some support for this conclusion36, and of course it is a source of at least some of the public's resistance to take them seriously. In the case of Bayesian inference, the charge stems directly from the role that prior probabilities play. Such probabilities are “subjective” in the straightforward sense already indicated.
Traditional Bayesians contend that it is demonstrable (Walker, 1969) that the influence of priors “wash out” over time, in which case the inference is ultimately “objective.” So long as certain conditions (event-exchangeability and the like) hold and assumptions (concerning parameter identifiability and the omission of idiosyncratic priors) are made, the beliefs of different agents, no matter how unlike at the outset, will eventually converge to the maximum likelihood solution as data accumulate. What is not often appreciated is that the rate of convergence (or whether it occurs at all) depends both on the nature of the data and of the models. Unfortunately, the real world of science is not always asymptotic. Data cost money and take time to acquire. So while Bayesian inference and maximum likelihood may often agree, sometimes in real world analyses they do not – with practical consequences (Lele, under review; see also the further discussion of this point below).
Further, the idea that Bayesian convergence is tantamount to objectivity in an adequately strong sense of the word is misleading. On the one hand, “objectivity” is here equated with “inter- subjective agreement,” which is to say that for all of the consensus involved, the probability is not agent-independent37. Invariance is not to be confused with independence. However, much the prior probabilities might “wash out” numerically in the calculation of posterior probabilities via Bayes Theorem, they must still include reference to them in principle. The reference in principle is crucial not because it influences the calculation, but because it embeds stochasticity in the head as “uncertainty,” and not in the world. A fully objective scientific inference draws conclusions about the way the world is, and not about the way in which consensus, however general, has been reached.
On the other hand, the asymptotic intuition embedded in the notion of Bayesian convergence again leads naturally if not also logically to the conclusion that common agreement about the way things stand in the world is tantamount to truth. But this optimistic suggestion is hostage to the history of science. Commitment to the belief that in their inter-dependency, self-regulation, and complexity, undisturbed biotic communities evolve in the direction of greater complexity was “settled science” among ecologists for well-over a 100 years. Only relatively recently has it been more and more challenged. Convergence of belief doesn't entail its truth. Confidence may be raised, even to the point of near-certainty, when it is in hindsight unwarranted. This is why the rote response to those who question anthropogenic global warming–“it is the consensus of experts”—is far from conclusive and to much of the public unconvincing.
There is a 2-fold option for the increasing number of ecologists who find it more computationally convenient to use Bayesian up-dating techniques to analyze multi-layered/factor hierarchical or space-state model of complex data, but who are uneasy about the apparent subjectivity of prior probabilities in the inferences they make. This option is set out in a very lucid and thought-provoking way by Clark in his widely-cited paper, “Why environmental scientists are becoming Bayesians” (Clark, 2005). It consists of placing a constraint on allowable priors and easing the tension sometimes induced when metaphysics and method are mixed.
A variety of constraints on priors have been proposed. Most of them are epistemic in character. They range from total knowledge on the part of the up-dating agent to total ignorance, some version of applying the Principle of Indifference to the choice of priors. Although both have a long history, it is not entirely clear how each is to be made precise (see Bandyopadhyay and Brittan, 2010). Clark opts for the latter—a flat or non-informational constraint. It is mathematically-convenient to do so. Moreover, it ensures agent-independency in this sense, that the agent is assumed to know nothing about the hypothesis at hand at the outset of his or her inferential activities; no prior beliefs are presupposed (in which case, at least in principle, “the data are allowed to speak for themselves”). But it also harbors problems, several of which are set out by Lele (Frontiers of Ecology and Evolution, this issue), and illustrated by case studies of the survival of the kit fox and declines in amphibian populations.
Since it is immediately available to the reader, there is little point in rehashing the rather technical paper here. Suffice it to say that Lele draws several unintended but important consequences from the long-known fact (see Fisher, 1930) that flat parameters are not invariant under transformation. For our purposes, two are particularly important. The first is that in a sample viability analysis, the population prediction interval (PPI) obtained by maximum likelihood ratios (MLR) under two parameterizations of the data are similar, while those obtained by non-informative priors differ from each other and from the MLR PPI. Despite what Clark says (Clark, 2005, pp. 3 and 5), Bayesian inferences based on flat priors do not lead to the same (numerical) conclusions as likelihood-based inferences on the same data.
The second consequence (Clark, 2005, lines 258–259) is that “different versions of the non-informative priors on the natural parameters induce different priors (and hence biases) on the induced parameters of scientific interest.” Simply put, the fact that flat priors are not invariant under transformation can be used to demonstrate that while on occasion Bayesian inferences resemble likelihood-based inferences and appear bias-free, on closer examination and other occasions this is not the case.
Although Clark admits (Clark, 2005, p. 4) that “the importance of philosophy should not be understated,” the “focus” of his paper is that “the emergence of modern [viz., objective hierarchical] Bayes has little to do with philosophy, but rather comes from pragmatism.” But as Lele makes clear in some detail, Clark's failure to take philosophical questions concerning the concept of objectivity more seriously led him to ignore the problematic character of his answers to them, and opens up legal and legislative challenges to flat-prior ecological inferences which lack the requisite invariance under transformation and parameterization.
Modern Bayesianism is ostensibly (but problematically) superior to its unacceptably “subjective” original by way of restricting allowable priors. It is often held to be similarly superior to Likelihoodism in its apparently unique ability to compute the likelihood function in complex statistical inferences from and to hierarchical models. These models are very useful, indeed indispensable, in understanding the processes underlying complex ecological data. As Ponciano et al. (2009, p. 356) put it, “computing the likelihood function needed for such inferences requires an intractable, high-dimensional integral. [But] inferences using computer intensive Bayesian methods sidestep this difficulty by simulating observations from a prior distribution using one of the various Markov chain Monte Carlo algorithms.” This surmounting of very genuine computational problems is undoubtedly an important factor in the growing popularity of these Bayesian methods.
Lele et al. (2007, 2010) recognized that the Bayesian computational methods could be coopted to calculate fully frequentist maximum likelihood estimates and their standard errors using an approach called data cloning. Ponciano et al. (2009), developed an extension to data cloning (the data cloned likelihood ratio or DCLR) that in a similar way affords the calculation of likelihood ratios or the differences of information criterion values. These are the fundamental tools of evidence, and hence of evidence comparing hierarchical models.
Thus, the computational advantage enjoyed by Bayesian methods is no more than apparent. If one assumes that statistical paradigms should be (mainly) compared computationally and conceptually, and if (at least in the wake of Ponciano et al., 2009 and also Lele et al., 2007) there is nothing (basically) to choose between the Bayesian and Likelihood paradigms computationally, then the difference is conceptual, and in this sense “philosophical.” The announcement of philosophy's irrelevancy by Clark and others was premature.
It needs to be made clear that convergence per se is a demonstrable consequence of the Likelihood account of evidence. Indeed on any adequate statistical paradigm, inferences should improve as more model-relevant data are analyzed39. But there is an underlying problem confronting Bayesian convergence. It has been called “availability bias.” Bayesian model identification converges to truth only if the “true” model is in the set of hypotheses under consideration40. As the statistician Barnard (1949) once wrote:
To speak of the probability of a hypothesis implies the possibility of an exhaustive enumeration of all possible hypotheses, which implies a degree of rigidity foreign to the true scientific spirit. We should always admit the possibility that the experimental results may best be accounted for by a hypothesis which never entered our heads.
In fact, there are two problems here. The more general is that Bayesian convergence assumes that all investigators start out with the same model set, however at variance their initial degrees of uncertainty with respect to its members' truth. The more specifically Bayesian problem is that it makes little sense to assign prior probabilities to members of the model set unless that set is assumed closed. Both problems result from the “availability bias.”
But this bias, as also the “confirmation bias” mentioned earlier, is eliminated with the introduction of multiple models required by the Likelihood account of evidence. First, models on this account are pairwise compared, without assigning prior probabilities to any of them, i.e., without incorporating a subjective bias in the testing procedure. Second, data constitute evidence only in a contextual way, conditional on the two models compared. The most one can say is that one or the other is better supported, not that it is closer to the truth. The challenge is to find other models and new data against which models to compare it. Bayesian convergence does not allow for the heretofore unimagined, either with respect to the initial model set or heretofore unrealized conditions. As ecologists know perhaps too well, new models at different levels of organization are introduced all the time as explanatory insights emerge and ecosystems change41.
A striking example is provided by research on stress-induced mutation. As Foster (2007) puts it,
…after 20 years of research, evidence now suggests that various types of stresses induce responses that have mutagenic consequences, and that sometimes this essentially random process can appear to be directed…
Change, not stasis, is the rule. In this case what has emerged is a model on which mutations are generated even prior to the operation of Darwinian and Lamarckian selection pressures and appears consistent with both. This is to say that what we early took as an exemplary “crucial experiment,” viz., Delbruck and Luria's analysis of phage-resistant bacteria cultures, was not42. As Tukey said in a memorable paper (Tukey, 1960, p. 425), “Conclusions are established with careful regard to the evidence…[but] accepted subject to future rejection….[They are] taken to be of lasting value, but not everlasting value.”
Given that in its present conceptual-methodological state ecology generally considered appears so unsettled43, it might be asked whether it really is a science, and not an area studies program, grouping together a number of rather different investigative activities under the general heading of “organisms and the environment.” The emphasis on “integration” or “synthesis” in some of the textbooks mentioned suggests an urgent need to find, or impose, a common core. The traditional way of understanding the question, “is it really a science and, if so, in what respects?” viz., “how closely does it resemble classical physics in its general aims and methods?” has rightly been rejected. No one any longer thinks that philosophers can determine in a more or less a priori fashion what the “right” concept of science is, or pretend that there is one (and only one) method of implementing it, or that all scientific laws must take the form of universal conditionals. But there is more to be said.
In a broad perspective, theoretical and conceptual clarification in ecology continue; their integration remains a somewhat distant goal. The possibility of methodological progress is nearer at hand. We have illustrated such progress in the case of hypothesis and model testing. There are at present two particularly plausible accounts, Bayesian confirmation and the Likelihoodist account of evidence. Their integration depends less on their unification than on assigning them their proper roles. Choosing ecological models to test and formulating/implementing environmental policies are (like betting) actions; they are to be explained or justified (as a normative Bayesian does) in terms of a (rational) agent's beliefs and desires. Beliefs and desires in turn are traditionally and, we think, most plausibly to be understood in personal probabilistic terms; some beliefs are more certain than others, some desires stronger. It follows that one should use Bayesian methods when the question is: what should I do? The resulting answer concerns the extent to which particular beliefs have been fortified in the process of up-dating (up-data-ing) them. Likelihood ratios, on the other hand, are agent independent. The probabilities embedded in them have nothing to do with either beliefs or desires, but with logical relations between sentences describing data and articulating models. Such ratios answer the question: which model (among those compared) is better supported by the data?
Insofar as they measure uncertainty, Bayesian inferences lead to irreducibly personal conclusions, however great the agreement respecting these conclusions proves to be over time among different people. The LR account of evidence compares models with their alternatives, and each accumulates support or not as the predictions to which they lead are verified. Since ecological models are for the most part stochastic, so too are the events and processes that, in a clear sense, they objectively represent. Greater methodological self-consciousness about the methods they use to test hypotheses/models should provide helpful guidance to ecological scientists and, in identifying (at least in general terms) the sources of their objectivity, make the policy recommendations of individual wildlife and wildland managers more credible with the general public44.
Our emphasis on the L-R account of evidence is not new, but it has yet to gain much ground in ecology. As a recent paper by Betini et al. (2017) discovered, “only 21 of 100 randomly selected studies from the ecological and evolutionary literature tested more than one hypothesis, only eight tested more than two hypotheses.” Yet as we have argued, it is only insofar as multiple models are pairwise compared vis-à-vis the data and in this way tested that the main forms of cognitive bias can be ruled out.
Two final notes. One is that both Bayesian confirmation and Likelihood evidence rely importantly, although not completely45, on the predictions to which hypotheses and models lead. Prediction in ecology is very difficult46. Humans are an integral part of many if not all of the ecological systems they study and their research and policy interventions alter them in the process of such study. As a result, a majority of ecologists still fall back on falsification and simplified versions of significance testing. It very much needs more methodological attention.
The other note is this. The Likelihood account leads to convergence, as do all good parameter estimators, in the sense that as favorable data continue to be accumulated, the evidence for particular models becomes stronger and stronger. However, there is not convergence toward a “final theory,” or even assumed that the “true model” is among those already under consideration. New explanations of events and instruments of their prediction may from 1 min to the next be discovered or invented. As in the case of Darwin's theory of natural selection, progress is measured in terms of the survival of mutations in the face of environmental pressures. From this point of view, testing methods do not result in approximations to some stipulated goal but are measures of survival value. What we term “Darwinian objectivity” presupposes competition between accounts, whether of ecological phenomena or appropriate methodologies.
In this deep way, the Likelihood account is well-suited to ecology. On it, models are never more or less true but epistemic stages in the course of evolution, contingent on conditions which are themselves subject to continuing change, and on the intervention in the biotic and abiotic environments of human beings whose behavior is itself conditioned on the success or failure of the models they test. Stochasticity and survival are fundamental dimensions of natural processes. So too are they features of any adaptive, objective and self-conscious account of model testing, and therefore of scientific method generally.
GB and PB originally conceived the main the theme of the paper. GB completed the first draft. Both authors contributed to the revisions of the manuscript and approved the final version for submission.
The authors declare that the research was conducted in the absence of any commercial or financial relationships that could be construed as a potential conflict of interest.
Two of the editors of this issue of Frontiers in Ecology and Evolution, Mark L. Taper and José M. Ponciano, have made some very useful suggestions regarding the paper. The three referees for this issue of Frontiers in Ecology and Evolution made a number of helpful comments on our original submission, all of which we have tried to address in our revisions.
1. ^It is worth noting that neither the GFW nor FRA models is to this point sensitive to changes in biodiversity or carbon uptake in the forests modeled, although both factors enter into cause-of-warming considerations.
2. ^Yale Environment 360 (2018, April 14). Americans Who Accept Climate Change Outnumber Those Who Don't 5 to 1.”
3. ^If we can take the textbooks by Pickett et al., and Ford as representative. Of course, a great many books on general ecology do not focus on methodological issues, although they do underline the necessity of re-conceptualizing the subject.
4. ^And if necessary, rules by which to translate theoretical terms in the hypotheses so that they had observational content and application, usually in the form of measurable quantities. These rules were often referred to as “operational definitions.” That said, there is no commonly-accepted way in which to characterize such “definitions.” Perhaps most often it is to provide quantitative indices for the application of theoretical terms, means by which they may be measured and thereby applied to observational or experimental data. It has proven to be particularly difficult to operationalize theoretical terms in ecology—think “ecosystem,” “niche,” and “diversity” (all of which have come to have normative dimensions). One virtue of testing mathematical models is that they postpone the problem; to test the model is simply to measure the quantities that it contains and verify the data-distributions in which it issues. It can later be decided how the model should, if desired, be integrated into a more explanatory and policy-guiding theory.
5. ^Elaboration of the H-D model included attempts to characterize “data” more precisely as well, including the methods of their measurement and the errors to which it would inevitably be subject, but nothing in what follows turns on these attempts.
6. ^They may be linked by a common cause or confounded with another variable, for example.
7. ^Saint-Mont (2018) has recently made an up-dated and well-informed case for the “inductive” (data-first) approach to testing. On the assumption that samples test generalizations about populations, the law of large numbers guarantees that the distance between them shrinks quickly as the sample increases in size, and “the true distribution comes into focus almost inevitably” (p. 686). Saint-Mont's perspective contrasts sharply with the hypothesis/model-first approach of the other accounts of testing we will consider (although he includes elements of these accounts in his own; the implication is correct, both models and data are involved inferentially, in this respect like the analysis of ecosystems, trophic cascade from the top down, nutrient supply from the bottom up). Although it is in certain respects problematic, he implicitly blurs Romesburg's line between causation/explanation and correlation, ignores problems associated with (random) sampling, and shares the questionable “true-model” aim of testing with other statistical paradigms. For all of its sophistication, Saint-Mont's view of testing represents a return to a form of Positivism on which the role of theoretical concepts in science/ecology is at best unclear and predictive success is the sole criterion of evaluation.
8. ^Or his corollary distinction between correlation and causation.
9. ^Romesburg's article, though written almost 40 years ago, still makes good reading, not only because of an extended (and mathematically-sophisticated) description of how Errington's constant threshold-of-security hypothesis (“For a given area and species, the number of animals surviving fall to spring can be no greater than a threshold value. This threshold accounts for all forms of natural mortality, barring catastrophic weather events, and is constant from year to year”) is to be reconstructed/tested on the H-D model, but also because of his careful attention to the details of evaluating the observational consequences (for the most part statistical) of the hypothesis, the vagaries of “general-purpose data” not collected under controlled conditions, and the necessity of cost/benefit analyses of experiments before they are actually initiated. For Errington's classic study (later modified to include a variable threshold), see Errington (1945).
10. ^Indeed, it is difficult to overstate the impact of Popper's account on the methodology of practicing scientists, among which ecologists. Thus, the bio-scientists Cassey and Blackburn (2006): “It is widely agreed that modern scientific inference relies on the vulnerability to refutation of its general theories, which have the characteristic quality of being both general and falsifiable.” Indeed, there are more references in the index to Ford's book to Popper than to anyone else, philosopher or scientist. Neither Ford nor Picket et al., discuss either Bayesianism or Evidentialism, although Pickett et al.'s, discussion of “pairwise alternative hypothesis testing” and the reference in it to Platt (1964) include elements of the latter.
11. ^The stunning Cygnus atratus discovered in 1790 by Latham.
12. ^See Botkin (1990). For Schmitz (2017), the “New Ecology” rests principally on a rejection of the twin classic theses that ecosystems are (relatively) self-regulating and isolated (from each other and, as objects of study, from human intervention).
13. ^Up to a point. There are notable examples of non-falsifiable zero-force principles that play an indispensable role, the First Law of Motion in Newtonian mechanics, the Hardy-Weinberg Law in ecology.
14. ^See Houston (2014) for a case study in ecology of the ways in which “the logic of every hypothesis is based on the underlying assumptions.”
15. ^Popper (1974, p. 1035) recognized the difficulty. Yet he does not resolve it beyond leaving it to “the scientific instinct of the investigator,” as did Duhem himself. See also The Logic of Scientific Discovery, p. 76n. It is also always possible in principle to re-interpret the allegedly falsifying data. See Kidwell and Holland (2002) for a taphonomic/stratigraphic re-interpretation of the fossil record on which it is consistent with classical evolutionary theory (and not, as Darwin himself was worried, a straightforward falsification of it).
16. ^An especially interesting ecological example is the study of individualist and community-unit concepts carried out by Shipley and Keddy (1987).
17. ^See Anderson et al. (2000). See also Läärä (2009).
18. ^Which might more accurately be called Neyman-Pearson or NP hypothesis testing.
19. ^A lack exacerbated by widespread inability to replicate results published in peer-reviewed articles. It is troubling without further explanation that (a) there is a growing number of P values per ecology article published (since “the more P values, the higher the odds that any given result will be significant even if it's just the result of chance”) and (b) “the reported value of the coefficient of determination, R2, has been falling steadily (suggesting a decrease in the marginal explanatory power of ecology).” See Low-Décarie et al. (2014), and for the first embedded quotation (Stokstad, 2014). Murtaugh (2014), among others, defends the traditional use of P values by ecologists, but on mathematical grounds.
20. ^Which might also be put in terms of the relevance of the data to the belief that H is true. I.e., If Pr(H|D) = Pr(H) then the data D are irrelevant re belief adjustment.
21. ^If learning from experience is to be possible, then it is reasonable to insist that learners should not have an a priori, and hence prior, beliefs that an empirical hypothesis is true to degree 1, i.e., could not possibly be false, or false to degree 0, i.e., could not possibly be true. Empirical hypotheses are never more than merely probable, which is to say that our beliefs concerning their truth are always uncertain to one degree or another. Nevertheless, some hypotheses are much better confirmed than others, and provide a more secure basis for action. It is the task of an adequate theory of confirmation, or so the Bayesian argues, to make clear the grounds of the difference. Although uncertainty can never be eliminated, it can be brought to heel.
22. ^Of course, this is no more than an idealization. In practice, policy decisions involve reconciling a number of different, often-conflicting, objectives, and there is no algorithm by means of which all can be optimized. The relatively recent discipline of multiple-criteria decision-making seeks to optimize, if not the criteria, then the trade-offs between them (see Deb, 2013).
23. ^Royall (1997) was perhaps the first statistician to distinguish sharply between confirmation and evidence questions in just the way that we do here. One of the anonymous referees of this paper has reminded us that the confirmation question is normative – what should an agent believe? – while the second, evidential, question asks the merely descriptive question – in what conditions do data provide evidence for a hypothesis. This distinction is important; what “should” be believed brings with it the presupposition that the agent is rational, and this presupposition, in turn, constrains the limits of belief, imposing a measure of “objectivity” on them. It is certainly more plausible to contend that D provide evidence for H just in case they bolster rational belief that it is true. The immediate difficulty with this sort of attempt to square confirmation with evidence is that it eventually requires imposing such strong constraints that all fully rational agents will assign the same prior probabilities at the outset of their inferences given that they share the same background information (see Williamson, 2005, pp. 11–12). There are several problems with the “unique probability constraint” (see Bandyopadhyay and Brittan, 2010), perhaps the most important of which is that “sharing the same background information” is vague if not also question-begging, an unhelpful proxy for objectivity. In part for this reason, traditional Bayesians make their case for objectivity not on the constraining of priors but the convergence of posterior probabilities. That convergence is not a sufficient condition of unbiased objectivity will be demonstrated later.
24. ^We use “the likelihood-ratio account of evidence, “evidential statistics,” and “Likelihoodism” somewhat interchangeably. It is important to note that the likelihood ratio is only an important special case of a more general class of measures that constitute the core of evidential statistics. See Lele (2004) on the “efficiency” of this particular evidential function.
25. ^See Rosenzweig's (1936).
26. ^Equivalently, the ratio of the two likelihoods is >1.
27. ^The quantity Pr(D|M) is usually referred to in the philosophical literature as a “likelihood.” But while numerically the likelihood of the model given the data is proportional to the probability of the data given the model, likelihood and probability differ conceptually; the likelihood is considered a function of the model, whereas the probability is considered a function of the data. The common philosophical notation of Pr(D|M) rather than the common statistical notation of L(M;D) is adopted here, but is not meant to imply that the model M needs to be considered a random variable.
28. ^Or the logarithm of the likelihood ratio. Nothing in the present discussion turns on the difference; the respective ordinal structures remain the same.
29. ^Some readers of this paper may be disappointed by the lack of precision in this definition of “theory.” They should look at Marquet et al. (2014): “In ecology, there is generally no consensus regarding the definition, role, and generality of theories….A summary of the ecological literature finds reference to 78 theories.”
30. ^I.e., it is provable that Pr(M|D) > Pr(M) just in case [Pr(D|M)/Pr(D| ~M)]> 1, i.e., when the two models are mutually exclusive and jointly exhaustive. Models, unlike hypotheses, don't often have negations, only alternatives. In this respect it differs from Bayesian-testing, which presupposes an implicit comparison between a hypothesis and its negation only. It is in this sense that evidence-testing allows for genuinely multiple models, Bayesian-testing does not.
31. ^One of the reviewers has corrected the original formulation of this claim, and has also urged us to make clear that the claim presupposes the difference measure of confirmation we have taken as our model. It should be added that while numerical similarities/dissimilarities between the proposed measures of confirmation and evidence vary with the way in which each is characterized, the ways in which the probability operators in each are interpreted—in terms of beliefs or bets in the case of confirmation, in terms of formal relations in the case of evidence—force a conceptual distinction between them, as does the ability to unravel such heretofore intractable problems in the foundations of statistics as the notorious “paradoxes of confirmation” (see Bandyopadhyay et al., 2016, Chapter 9) or to clarify one source of public policy controversies.
32. ^The following two paragraphs are drawn from Bandyopadhyay et al. (2016, pp. 40–44). References documenting the empirical claims made can be found there.
33. ^What follows draws on the very accessible overview by Farley (2008).
34. ^To avoid misunderstanding, the choice of models to test is not agent-independent, only the formal relationship between the models tested and the data-distributions in which they issue. Both Bayesianism and Evidential Statistics are “rationalist” or “top-down” in that they begin with hypotheses and models and then proceed to gather data, not the other way around. In this respect, both are to be sharply distinguished from frequentist approaches which begin with correlations in the data gathered. In that Mayo begins with simple statistical hypotheses, her approach (Mayo, 2018, p. 85) is in a related sense “bottoms-up.”
35. ^See Kahneman (2011, p. 81): “Contrary to the rules of philosophers [or at least of Karl Popper], who advise testing hypotheses by trying to refute them, people (and scientists quite often) seek data that are likely to be compatible with the beliefs they currently hold.”
36. ^For documentation of such bias see Fanelli (2010) and Holman et al. (2015).
37. ^Nor, for that matter, independent of the many pressures brought to bear on the up-dating of beliefs by disciplinary communities (in the person of editorial staffs and funding agencies).
38. ^Another referee helpfully asked for a brief comment on cloning.
39. ^Because model misspecification is allowed in an evidential framework, data-model consistency is not identical with classical consistency. Of course, if the generating process is actually a model in the model set, it should be asymptotically identified. Under model misspecification, however, misleading and weak evidence still both need to go to zero as sample size increases to infinity. Asymptotically the model selected should be the model in the model set closest to the generating process.
40. ^That the “true model” is assumed to be in the model set follows from the fact that the prior probabilities of the hypotheses considered must sum to 1. See Lindley (2001) for an attempt to avoid the problem by pointing out that Bayesian inference is always conditional on a set of models and “convergence” understood as relative to it. To relativize convergence in this way, however, is to relativize “true model,” and with it the “objectivity” that Bayesian convergence is intended to ensure.
41. ^Chamberlain (1897), Platt (1964), and Burnham and Anderson (2002) among others have understood the virtues of multiple models. On the LR account of testing, evidence has real bite only when it serves to distinguish between them. Human-caused and ocean-temperature caused global warming are not simply mutually-exclusive and (we assume for present purposes) jointly-exhaustive alternatives; stronger or weaker evidence for and against them can be gathered in a genuinely comparative context. Apparently such a context has not yet been developed for the deforestation hypotheses mentioned above.
42. ^See Cairns et al. (1988) for the initial clue re stress-induced mutation, and Houston (2014) for a multi-model approach to re-thinking the rejection of at least two classic population equilibrium hypotheses.
43. ^Some would say it is in a state of crisis, despite all of the enormously illuminating work done over the last several generations in its many sub-disciplines, island-biogeography among them.
44. ^See Maunder and Piner (2015): “Bayesian analysis accommodates the use of prior information in integrated assessments, allowing sharing of information from other species. It also allows for the representation of uncertainty in a probabilistic context, which is ideal for decision analysis.” In this way Maunder and Piner take it as supplementing Likelihood testing which is widely used in fisheries management.
45. ^The extent to which they explain and aid understanding of ecological events and processes also factors into their evaluation.
46. ^See Dietze (2017) and Maris (2018).
Anderson, D., Burnham, K., and Thompson, W. (2000). Null hypothesis testing: problems, prevalence, and an alternative. J. Wildl. Manag. 64, 912–923. doi: 10.2307/3803199
Bandyopadhyay, P., and Brittan, G. (2010). Two dogmas of strong objective Bayesianism. Int. Stud. Philos. Sci. 24, 45–65. doi: 10.1080/02698590903467119
Bandyopadhyay, P., Brittan, G., and Taper, M. (2016). Belief, Evidence, and Uncertainty. New York, NY: Springer.
Barnard, G. (1949). Statistical inference. J. R. Stat. Soc. Ser. B 11, 115–149. doi: 10.1111/j.2517-6161.1949.tb00028.x
Betini, G., Avgar, T., and Fryxell, J. (2017). Why are we not evaluating multiple competing hypotheses in ecology and evolution? R. Soc. Open Sci. 4:160756. doi: 10.1098/rsos.160756
Botkin, D. (1990). Discordant Harmonies: A New Ecology for the Twenty-First Century. New York, NY: Oxford University Press.
Burnham, K., and Anderson, D. (2002). Model Selection and Multi-Model Information: A Practical Information-Theoretic Approach, 2nd Edn. New York, NY: Springer.
Cairns, J., Overbaugh, J., and Miller, S. (1988). The origin of mutants. Nature (London) 335, 142–145. doi: 10.1038/335142a0
Cassey, P., and Blackburn, T. (2006). Reproducibility and repeatability in ecology. Bioscience 56, 958–959. doi: 10.1641/0006-3568(2006)56[958:RARIE]2.0.CO;2
Chamberlain, T. (1897). The method of multiple working hypotheses. J. Geol. 5, 837–848. doi: 10.1086/607980
Chatfield, C. (1995). Model uncertainty, data mining and statistical inference. J. R. Stat. Soc. Ser A 158, 419–466. doi: 10.2307/2983440
Clark, J. (2005). Why environmental scientists are becoming Bayesians. Ecol. Lett. 8, 2–14. doi: 10.1111/j.1461-0248.2004.00702.x
Da Silva, J., Steiner, A., and Schreiner, E. (2018, October 3). Forests: a natural solution to climate change, crucial for a sustainable future. United Nations Development Programme.
Deb, K. (2013). An evolutionary based Bayesian design optimization approach under incomplete information. Eng. Optim. 45, 151–165. doi: 10.1080/0305215X.2012.661730
Dennis, B., Poinciano, J., Taper, M., and Lele, S (2019). Errors in statistical inference under model misspecification: evidence, hypothesis testing, and AIC. Front. Ecol. Evol. doi: 10.3389/fevo.2019.00372
Dietze, M. (2017). Prediction in ecology: a first principles framework. Ecol. Appl. 27, 2048–2070. doi: 10.1002/eap.1589
Ellison, A. (1986). An introduction to Bayesian inference for ecological research and decision-making. Ecol. Appl. 64, 1036–1046.
Ellison, A. (2004). Bayesian inference in ecology. Ecol. Lett. 7, 509–520. doi: 10.1111/j.1461-0248.2004.00603.x
Errington, P. (1945). Some contributions of a fifteen-year local study of the northern bob-white to a knowledge of population phenomena. Ecol. Monogr. 15, 1–34. doi: 10.2307/1943293
Fanelli, D. (2010). Do pressures to publish increase scientists' bias? An empirical support from US states data. PLoS ONE 5:e10271. doi: 10.1371/journal.pone.0010271
Farley, J. (2008). The scientific case for modern anthropogenic global warming. Monthly Rev. 60. doi: 10.14452/MR-060-03-2008-07_5
Fisher, R. (1929). The statistical method in psychical research. Proc. Soc. Psych. Res. 39, 189–192.
Foster, P. (2007). Stress-induced mutagenesis in bacteria. Crit. Rev. Mol. Biol. 42, 373–397. doi: 10.1080/10409230701648494
Hempel, C. (1965). Studies in the Logic of Confirmation. Aspects of Scientific Explanation. New York, NY: Free Press.
Holman, L., Head, M., Lanfear, R., and Jennions, M. (2015). Evidence of experimental bias in the life sciences: why we need blind data recording. PLoS Biol. 13:e1002190. doi: 10.1371/journal.pbio.1002190
Houston, M. (2014). Disturbance, productivity, and species diversity: empiricism vs. logic in ecology theory. Ecology 9, 2382–2396. doi: 10.1890/13-1397.1
Kidwell, S., and Holland, S. (2002). The quality of the fossil record: implications for evolutionary analyses. Annu. Rev. Ecol. Syst. 33, 561–588. doi: 10.1146/annurev.ecolsys.33.030602.152151
Läärä, E. (2009). Statistics: reasoning on uncertainty, and the insignificance of testing null. Ann. Zool. Fennici 46, 138–157. doi: 10.5735/086.046.0206
Lele, S. (2004). “Evidence function and the optimality of the law of likelihood,” in The Nature of Scientific Evidence, eds M. Taper and S. Lele. Chicago, IL: University of Chicago Press.
Lele, S., Dennis, B., and Lutscher, F. (2007). Data cloning: easy maximum likelihood estimation for complex ecological models using Bayesian Markov Chain Mlonte Carlo methods. Ecol. Lett. 10, 551–563. doi: 10.1111/j.1461-0248.2007.01047.x
Lele, S. R., Nadeem, K., and Schmuland, B. (2010). Estimability and likelihood inference for generalized linear mixed models using data cloning. J. Am. Stat. Assoc. 105, 1617–1625. doi: 10.1198/jasa.2010.tm09757
Lindley, D. (2001). The philosophy of statistics. J. R. Stat. Soc. 49, 293–337. doi: 10.1111/1467-9884.00238
Low-Décarie, E., Chivers, C., and Grenados, M. (2014). Rising complexity and falling explanatory power in ecology. Front. Ecol. Environ. 12, 412–418. doi: 10.1890/130230
Luria, S., and Delbruck, M. (1943). Mutations of bacteria from virus sensitivity to virus resistance. Genetics 28:491.
Maris, V. (2018). Prediction in ecology: promises, obstacles, and clarifications. Oikos 127, 171–183. doi: 10.1111/oik.04655
Marquet, P., Allen, A. P., Brown, J. M., Dunne, J. A., Enquist, B. J., Gillooly, J. M., et al. (2014). On theory in ecology. Bioscience 64, 701–710. doi: 10.1093/biosci/biu098
Maunder, M., and Piner, K. (2015). Contemporary fisheries stock assessment: many issues still remain. ICES J. Mar. Sci. 72, 7–18. doi: 10.1093/icesjms/fsu015
Mayo, D., and Spanos, A. (2004). Methodology in practice: statistical misspecification testing. Philos. Sci. 71, 1007–1025.
Pearce, F. (2008, October 9). Conflicting data: how fast is the world losing its forests? Yale Environment 360.
Pickett, S., Kolesa, J., and Jones, C. (2007). Ecological Understanding: The Nature of Theory and the Theory of Nature, 2nd Edn. Amsterdam: Elsevier.
Ponciano, J., Taper, M., Dennis, B., and Lele, S. (2009). Hierarchical models in ecology: confidence intervals, hypothesis testing, and model selection using data cloning. Ecology 90, 356–362. doi: 10.1890/08-0967.1
Popper, K. (1974). Intellectual Autobiography. The Philosophy of Karl Popper, ed P. Schilpp. LaSalle, IL: Open Court.
Romesburg, C. (1981). Wildlife science: gaining reliable knowledge. J. Wildl. Manag. 45, 293–313. doi: 10.2307/3807913
Rosenzweig, S. (1936). Some implicit factors in diverse methods of psychotherapy. Am. J. Orthopsychiatry 6, 412–415. doi: 10.1111/j.1939-0025.1936.tb05248.x
Saint-Mont, U. (2018). Where Fisher, Neyman and Pearson went astray: on the logic (plus some history and philosophy) of statistical tests. Adv. Soc. Sci. Res. 5, 672–691. doi: 10.14738/assrj.58.4867
Schmitz, O. (2017). The New Ecology: Rethinking a Science for the Anthropocene. Princeton, NJ: Princeton University Press.
Shipley, B., and Keddy, P. (1987). The individualistic and community-unit concepts as falsifiable hypotheses. Vegetatio 69, 47–55. doi: 10.1007/BF00038686
Shrader-Frechette, K., and McCoy, E. (1993). Method in Ecology: Strategies for Conservation. Cambridge: Cambridge University Press.
Tukey, J. (1960). Conclusions vs. decisions. Technometrics 2, 423–433. doi: 10.1080/00401706.1960.10489909
Walker, A. M. (1969). On the asumptotic behavior of posterior distributions. J. R. Stat. Soc. Ser. B 31, 423–433.
Keywords: bayesian inference, evidential statistics, significance testing, falsificationism, hypothetico-deductivism
Citation: Brittan G Jr and Bandyopadhyay PS (2019) Ecology, Evidence, and Objectivity: In Search of a Bias-Free Methodology. Front. Ecol. Evol. 7:399. doi: 10.3389/fevo.2019.00399
Received: 19 March 2019; Accepted: 08 October 2019;
Published: 22 October 2019.
Edited by:
Yukihiko Toquenaga, Graduate School of Life and Environmental Sciences, University of Tsukuba, JapanReviewed by:
Jonah N. Schupbach, The University of Utah, United StatesCopyright © 2019 Brittan and Bandyopadhyay. This is an open-access article distributed under the terms of the Creative Commons Attribution License (CC BY). The use, distribution or reproduction in other forums is permitted, provided the original author(s) and the copyright owner(s) are credited and that the original publication in this journal is cited, in accordance with accepted academic practice. No use, distribution or reproduction is permitted which does not comply with these terms.
*Correspondence: Prasanta Sankar Bandyopadhyay, cHNiQG1vbnRhbmEuZWR1
Disclaimer: All claims expressed in this article are solely those of the authors and do not necessarily represent those of their affiliated organizations, or those of the publisher, the editors and the reviewers. Any product that may be evaluated in this article or claim that may be made by its manufacturer is not guaranteed or endorsed by the publisher.
Research integrity at Frontiers
Learn more about the work of our research integrity team to safeguard the quality of each article we publish.