- Department of Wildland Resources, The Ecology Center, Utah State University, Logan, UT, United States
Plant-soil feedbacks (PSFs) have gained attention as a potential mechanism of plant growth and coexistence, however, because they are typically measured using plant monocultures in greenhouse conditions, the link between PSFs and plant growth in field communities remains poorly tested. Here, PSFs for six native and four non-native species were measured in a 7-year, common-garden experiment. A plant community growth model was then parameterized either with PSF data (PSF model) or without PSF data (Null model). PSF and Null model predictions were compared to plant ground cover in experimental and natural communities. For eight of 10 species, plant cover at the end of the experiment differed among soils cultivated by different species. For native plants, the Null model incorrectly predicted rank-order abundance for three of four experimental communities and Null model predictions were not correlated with observed plant growth. In contrast, when PSF data were added to the same model, the model correctly predicted rank-order abundance for all four experimental communities and PSF model predictions were well-correlated with plant cover in experimental communities and on the landscape (R2 = 0.62). For non-native species, predictions from both models were correlated with observed species cover (R2 = 0.37 and 0.35, respectively), but there was no difference between PSF and Null model predictions. Previous studies at the study site have shown that PSF maintains alternate-state native and non-native plant communities. Here, it was shown that PSF is also critical for determining species composition within native plant communities, but that other mechanisms appear to be necessary to simulate the rapidly-fluctuating abundances of the short-lived, non-native species in this system. Using a relatively long-term field experiment, this study provided unusually direct evidence for the role of PSF in determining plant abundance in plant communities in field conditions, at least for long-lived native plants.
Introduction
Plant-soil feedbacks (PSFs) have gained attention for their potential to determine plant growth, succession, coexistence, and invasion (van der Putten et al., 2013; Bailey and Schweitzer, 2016; Zhang et al., 2019). PSFs are typically measured by comparing the growth of a plant on soils cultivated by conspecifics (“self” or “home” soils) to soils not cultivated by conspecifics (“other” or “away” soils; Bever, 1994; Bever et al., 1997). While there are many variations, PSF experiments are often performed by growing plant monocultures in a greenhouse for a roughly 3-month training phase and a 3-month test phase (Kulmatiski and Kardol, 2008; Brinkman et al., 2010). Plants that grow better on “self” than “other” soils have positive PSF, while plants that grow better on “other” than “self” soils have a negative PSF (Brinkman et al., 2010; Reinhart and Rinella, 2016; Teste et al., 2019). Mathematical models suggest that positive PSF encourages competitive exclusion while negative PSF encourages species coexistence through replacement (Bever, 2003; Eppinga et al., 2018; Mack et al., 2019).
There are, however, many reasons why PSFs measured in monocultures in greenhouse experiments may not be important to plant communities in the field (Poorter et al., 2016; Schittko et al., 2016). Greenhouse conditions may create PSFs that are not relevant to field communities. The sterilized soils that are often used in greenhouse PSF experiments are likely to encourage the growth of microbes that may not be common in field conditions (Hawkes et al., 2013; Kardol et al., 2013; Bergmann et al., 2016). Larger soil organisms may be almost completely excluded from greenhouse experiments (Cesarz et al., 2018). Moderate climate conditions in the greenhouse are also likely to change plant growth, soil organism growth, and their interactions relative to field soils (Heinze et al., 2016; Schittko et al., 2016; van der Putten et al., 2016; Fry et al., 2018). In addition to the effects of greenhouse conditions, plant monocultures may create conditions that are not common in the field. For example, diverse plant communities may create soil microbial communities that have greater disease suppressiveness than soil communities associated with plant monocultures (Compant et al., 2005; Latz et al., 2012). As a result, PSFs measured in plant monocultures may or may not be relevant to PSFs realized in plant communities (Poorter et al., 2016; Smith-Ramesh and Reynolds, 2017; Wubs and Bezemer, 2018).
The mathematical models used to infer PSF effects in communities may also be misleading. PSF models often make the unrealistic assumption that plant species are competitively equivalent (Crawford and Knight, 2017; Vincenot et al., 2017; Bezemer et al., 2018). However, to have large effects on plant abundance in communities, PSFs should be of a similar magnitude as differences in intrinsic growth rates among species (Revilla et al., 2013; Kulmatiski, 2016; Kulmatiski et al., 2016; Lekberg et al., 2018). Published data suggest that PSFs are sometimes large enough to overcome competitive inequality among species, suggesting that PSF, at least in some cases, will be an important driver of plant coexistence, though it is not known how commonly this occurs (Crawford and Knight, 2017; Lekberg et al., 2018; Mack et al., 2019).
Testing whether or not PSF determines plant growth in communities remains a central goal in PSF research (van der Putten et al., 2016; Smith-Ramesh and Reynolds, 2017; Lekberg et al., 2018). One way to test whether or not PSF effects are large enough to overcome the effects of other plant growth factors is to use PSF effects in plant growth models and compare model predictions to plant growth observed in plant communities (Mangan et al., 2010; Kulmatiski, 2018). This approach, however, has not been widely used (Bennett and Cahill, 2016; Chung and Rudgers, 2016; Schittko et al., 2016; Bennett et al., 2017; Teste et al., 2017).
The goal of this research was to test whether or not PSF data could be used to improve predictions of plant growth in communities. To meet this objective, PSFs were measured for six native and four non-native species in a 7-year, common-garden experiment. To test whether or not these measured PSFs were important to plant growth in communities, a plant growth model was parameterized either with PSF effects (PSF model) or without PSF effects (Null model) and model predictions were compared to plant growth in experimental plant communities and to plant growth on the landscape [as reported in Kulmatiski and Beard (2019)].
Materials and Methods
Research was conducted on the Newbon soil series (coarse-loamy, mixed mesic Typic Haploxerolls; Lenfesty, 1980), Winthrop, Washington (48.481 N, −120.117 W; elevation 780 m). Annual precipitation (380 mm) falls mostly as snow in the winter (November through March). There are two common plant community types on the landscape. Fields that have never been tilled represent most of the land in the hilly landscape and are dominated by native plants (62.4 ± 2%) with non-native plants less common (6.6 ± 0.8%; Kulmatiski and Beard, 2019). Fields that have been tilled and used for agriculture, primarily valley bottoms, and benches, are dominated by non-native plants (39.6 ± 2.1% absolute cover [mean ± std. dev.]) with native plants less common (19.2 ± 1.8%; Kulmatiski and Beard, 2019). Because these two plant community types (native and non-native dominated) are largely separated on the landscape, PSF effects on plant communities were determined for native and non-native plant communities separately.
Plant-Soil Feedback Experiment
A roughly 1-ha area in an abandoned agricultural field, previously used to grow alfalfa (Medicago sativa), was used to establish a two-phase, “self” vs. “other” PSF experiment (Bever, 1994; Brinkman et al., 2010). In this experimental approach, target species were maintained for a 4-year Phase I to create different soil treatments. Plants were then removed with herbicide and re-planted with either the same species (i.e., self plots) or different species (i.e., other plots). A full factorial design was used for three native species and for three non-native species. In this factorial design each plant was grown on soils cultivated by each of the other plants in the community. Because of limited space, PSFs for the remaining four species were assessed using a “self vs. control” PSF approach which requires fewer replicates (Kulmatiski, 2016). This “self vs. control” portion of the experiment and resultant PSF values were reported previously (Kulmatiski et al., 2017), but the factorial portion of this experiment, use of all PSF values in a plant growth model, and comparison of model predictions to plant growth in experimental plant communities are new to this manuscript.
Dominant species on the landscape were selected for this experiment with some exceptions. Native species included an annual forb [Collomia grandiflora (COGR)], two perennial forbs [Lomatium dissectum (LODI) and Lupinus sericeus (LUSE)] and three perennial grasses [Festuca idahoensis (FEID), Koeleria cristata (KOCR), and Pseudoroegneria spicata Pursh A. Love (PSSP)]. Non-native species included an annual grass [Bromus tectorum (BRTE)], an annual forb [Lactuca serriola (LASE)], and two annual/perennial forbs [Centaurea diffusa Lam. (CEDI) and Sissymbrium loeselii (SILO)]. Because the experiment was conducted in 1.5 m2 experimental plots, the dominant, but large native shrubs, Purshia tridentata and Artemisia tridentata and the rhizomatous non-native forb, Cardaria draba were not used. The native annual forb COGR is a widespread but not dominant species but it was used to provide inference to the PSF of a common native annual. Poa bulbosa is a dominant non-native, but we were unable to establish it. Unless otherwise noted, species naming follows that of Hitchcock and Cronquest (1973).
Prior to Phase I of the experiment, the top 10 cm of soil, and presumably much of the weed seed bank was removed by bulldozer, 7.6 m3 of A-horizon soil from a native plant dominated field were mixed with sand from a nearby landslide, and all soils were disc-plowed to create a mixed agricultural-native-sand growth medium. A grid of 1.2 m-wide geotextile cloth was secured to the ground creating 750 1.5 m2 plots. Of these, 250 plots were used to create self-cultivated soil treatments (25 plots for each species). These plots were replanted with the same species in Phase II of the experiment providing a measurement of plant growth on “self” soils. An additional 250 plots were maintained free of vegetation to be used as “control” plots. In Phase II, each species was planted in 25 replicate “control” plots to provide a measure of plant growth on “non-self” or “other” soils. The remaining 250 plots were used for the factorial PSF experiment. For the three native species in N3 (Table 2) and the three non-native species in X3 (Table 2), each plant was grown on “self” soils as well as on soils cultivated by each of the other plant species in the community. Because these factorial designs require many more plots than the “self” vs. “control” approach, the factorial design was used only for three native plant species and three non-native plant species. All treatments were replicated 25 times, though in a few cases, more than 25 replicate “self” plots were used because additional plots had been created for a related experiment in the same field.
Each fall from 2006 to 2009, 12 g of seed from the target species was added to each plot. Each spring and summer from 2007 to 2010, non-target species were removed from each plot by hand weeding. In May 2010, all plots were surveyed. Plots where the target species did not represent 65% or more of standing vegetation were removed from the experiment. Beginning June 2010, all remaining quadrats were treated with a broad-spectrum herbicide application (30 ml of Roundup® herbicide, 0.2 kg active ingredient ha−1). Two weeks later, standing vegetation was clipped by hand and left in the plot. Plots were revisited over the next several months and additional herbicide spot-treatments and hand-pulling were used in quadrats where regrowth was observed. This created replicate plots with soils cultivated by target plant species.
Phase II began October 2010. Each species was replanted by seed in “self,” “other,” or “control” plots as appropriate. Non-target species were removed from all plots during the 2011, 2012, and 2013 growing seasons. In June 2013, percent cover of each plant was measured in each plot using visual estimation.
Plant-Community Experiment
Data from the PSF experiment were used in a plant growth model to predict the percent ground cover of plant species in communities. To test model predictions, three-species plant communities were grown in the field (Table 1). Three communities composed of native species and three communities composed of non-native species were grown for 4 years (2007–2010). Communities for which factorial PSF were available were grown again from 2010 to 2013 (i.e., N3 and X3 in Table 1). More specifically, in October 2006, six different three-species communities were established by seed (Table 1). Plots were the same size and randomly located among the plots used for the PSF experiment. The communities for which full factorial PSF data were available (i.e., N3 and X3) were replicated 120 times. The remaining four communities were replicated 50 times. As in the PSF experiment, between 2007 and 2010 all plots were seeded and weeded by hand. Plots in which target species did not represent 65% of total plant cover prior to the final weeding were removed from the experiment. Percent cover of each target species was determined June 2010. Also in June 2010, 60 plots that had grown the dominant native plant, P. spicata, were treated with herbicide and planted with either N3 or X3. This was done on P. spicata plots because this provided more inference into how these communities, for which factorial PSF data were available, grow on a common soil treatment and during a different time period and not just on control soils between 2007 and 2010.
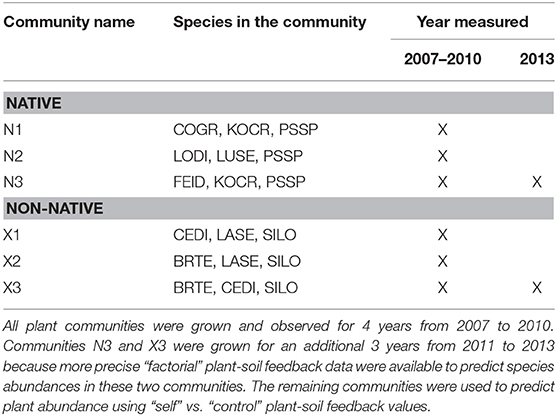
Table 1. Species compositions and the year of measurement for the six plant communities used in the “plant-community experiment.”
The Plant Community Growth Model
The best-performing of five plant growth models described by Kulmatiski et al. (2016) was used to simulate plant community composition (i.e., the Logistic Pot-level-K model). Briefly, in this continuous-time, logistic growth model, each plant's growth is a function of the proportional abundances of the soils cultivated by different plant species (Bever, 2003; Levine et al., 2006; Eppstein and Molofsky, 2007). The model assumes each plant's growth is limited by a community-level carrying capacity and total plant growth in the community. Carrying capacity was defined as the mean cover observed in native and non-native plant communities in the plant community experiment (i.e., 43 and 38%, respectively). Plants were assumed to start growth as seed (0.002 g) and time-step-specific growth rates were calculated for 40 time steps as (40√F/I) – 1, where F = final cover and I = initial cover. Final cover for each species on each soil treatment was determined from the cover observed at the end of the PSF experiment.
The model was parameterized with two datasets: Null and PSF. In the Null parameterization, only cover data from “control” soils was used. Control soils were soils that were maintained free of vegetation during Phase I. Plant growth on control soils was selected because this represents a standard method of measuring plant growth in a common-garden experiment (e.g., in a plant competition experiment). In the PSF parameterization, plant growth data from “self” and “other” soils was used. “Other” soils were species-specific when possible, or “control” soils when species-specific data were not available.
To better simulate multi-year plant growth, two changes to the model were made. First, to simulate annual senescence, after every 40 time steps, plant growth was decreased to equal 1% of the value in the previous timestep. Second, it was assumed that plants were affected more by “self” than “other” soils since plant roots grow within their own rhizosphere. To calculate this effect, “self” soils were calculated as the proportion of “self” plant abundance in the previous timestep plus an arbitrarily-selected 25% of the remainder of soil treatments. For example, if a plant represented 30% of a three-species plant community, that plant was estimated to grow on 30% + 0.25*(1–30%) = 47.5% “self” soils. The proportion of “other” soils was then down-weighted to account for the larger proportion of “self” soils. Null and PSF models were executed for 120 time steps to simulate growth of plant communities grown for 3 years (2011–2013), for 160 time steps for communities grown for 4 years (2007–2010) and for 400 time steps to simulate plant growth on the landscape.
Statistical Analyses
Plant growth data were primarily used to parameterize plant community growth models, but to determine if biomass differed by soil treatment, a one-factor GLMM was used with soil treatment as the fixed effect; analyses were performed by species because species by soil treatment comparisons were not of interest. Transformations to meet assumptions of homogeneity and normality were used as necessary. For all tests, a post-hoc Tukey–Kramer method was used to adjust for Type I error and determine pairwise differences among least square means. Means from raw data are reported.
To determine if PSF data improved Null model predictions across species, a Student's t-test on the absolute difference between observed and predicted values for the Null and PSF models was conducted. To determine the goodness-of-fit between observed and predicted values, a Pearson correlation coefficient was calculated and reported as an R2 value. Correlations were performed first for plant cover values at the end of the experiment, then again for annual and final cover values. Similarly, correlations were first performed for the experimental communities then also for a combined dataset that included species abundances from the experimental communities as well as species abundances from the landscape. Correlation P values are reported and considered significant when P < 0.05. Plant cover on the landscape was reported by Kulmatiski and Beard (2019).
Results
For all species except L. sericeus and L. serriola, ground cover differed among soil treatments (Tables 2, 3). For native plant cover in experimental communities, 10 of 12 PSF model predictions were closer to observed values than Null model predictions. More specifically, seven of nine predictions of species abundance at the end of the 4-year community experiment (Figure 1) and three of three predictions of species abundance at the end of the 3-year community experiment (Figure 2). A T-test of the absolute difference between predicted and observed species cover in experimental communities indicated that PSF predictions were closer to observed values than Null values (T(1, 11) = 5.95, P = 0.006). Similarly, PSF model predictions were correlated with native species cover in experimental communities (F(1, 10) = 32.6, P < 0.001, R2 = 0.77) but Null model predictions were not (F(1, 10) = 0.17, P = 0.686). Results were similar when annual predictions of species cover were correlated with annual observations. PSF model predictions were correlated with species cover (F(1, 34) = 90.3, P < 0.001, R2 = 0.73) but Null model predictions were not (F(1, 34) = 0.00, P = 0.992). PSF data also improved Null model predictions of species cover on the landscape (Figure 3). When data from the four experimental communities and plant cover on the landscape were combined, a T-test of the absolute difference between predicted and observed species cover indicated that PSF predictions were closer to observed values than Null values (T(1, 17) = 3.70, P = 0.010). Further, PSF model predictions of species cover in experimental communities and landscape communities were correlated with observed species cover (F(1, 16) = 26.6, P < 0.001, R2 = 0.62) but Null model predictions were not (F(1, 16) = 0.08, P = 0.78).
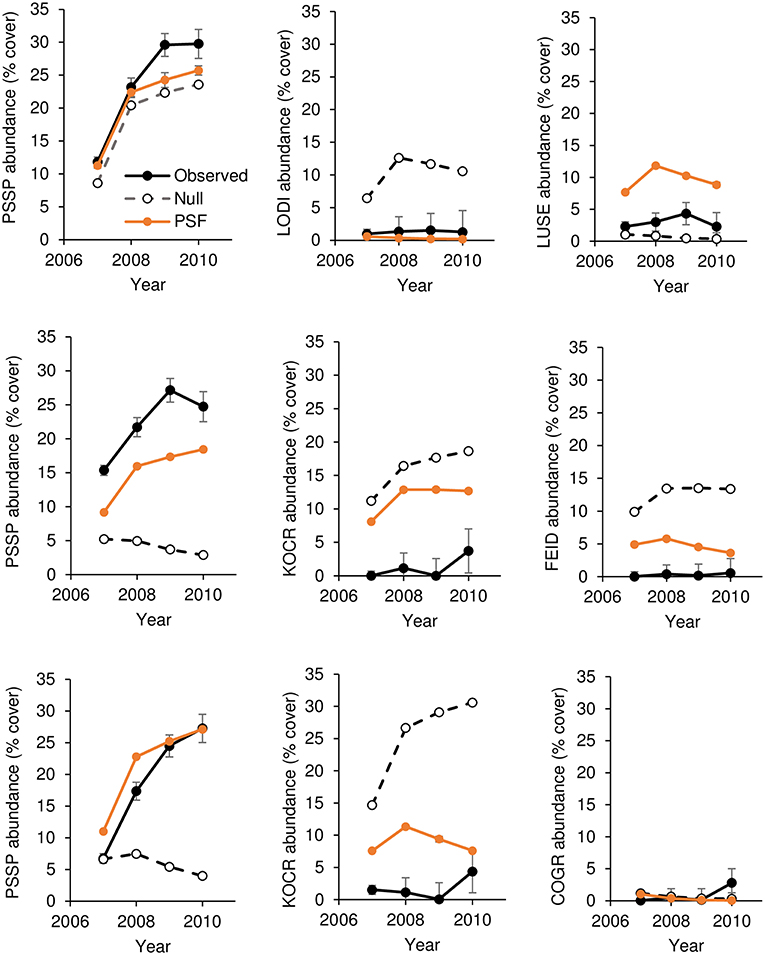
Figure 1. Observed and predicted abundance of native plant species in three-species experimental plant communities grown for 4 years (2007–2010). Species in a row are from the same three-species community. Model predictions were made either with plant-soil feedback effects (PSF) or without plant-soil feedback effects (Null). PSSP, Pseudoroegneria spicata; LODI, Lomatium dissectum; LUSE, Lupinus sericeus; COGR, Collomia grandifolia; FEID, Festuca idahoensis; KOCR, Koeleria cristata.
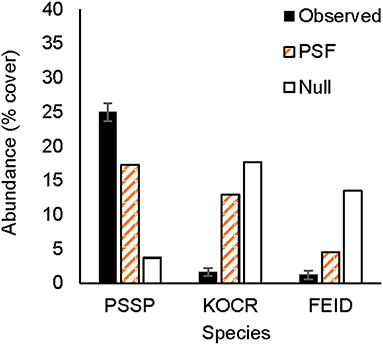
Figure 2. Observed and predicted abundance of native plant species in a three-species experimental plant community grown for 3 years (2011–2013). Model predictions were made either with plant-soil feedback effects (PSF) or without plant-soil feedback effects (Null). Species codes listed in the Figure 1.
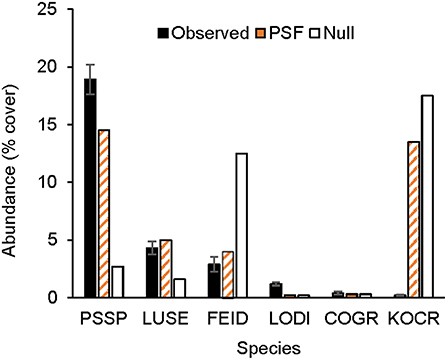
Figure 3. Observed and predicted native plant abundance. Observed plant abundance was recorded in 25 ex-arable fields over 13 years (Kulmatiski and Beard, 2019). Model simulations of plant community growth for these six common native species were performed for a 10-year period either with plant-soil feedback data (PSF; i.e., with information on plant growth rates on different soil treatments) or with a null model (Null) which was parameterized only with plant growth rates on control soils. PSSP, Pseudoroegneria spicata; LODI, Lomatium dissectum; LUSE, Lupinus sericeus; COGR, Collomia grandifolia; FEID, Festuca idahoensis; KOCR, Koeleria cristata.
For non-native plants, a T-test of the absolute difference between predicted and observed species cover in experimental communities indicated that PSF predictions were not different from Null values (T(1, 11) = 1.16, P = 0.81). Further, neither PSF model predictions (F(1, 10) = 4.71, P = 0.055) nor Null model predictions (F(1, 10) = 4.42, P = 0.061) were correlated with species cover in experimental communities (Figures 4, 5). Results were similar when annual predictions of species cover in experimental communities were compared to annual observations: neither PSF model predictions (F(1, 34) = 0.39, P = 0.536) nor Null model predictions (F(1, 34) = 0.06, P = 0.801) were correlated with observed annual species cover (Figure 4). Model predictions of non-native species cover on the landscape were better (Figure 6). When species cover data from experimental communities and the landscape were combined, both PSF model predictions (F(1, 14) = 8.17, P = 0.013, R2 = 0.37) and Null model predictions (F(1, 14) = 7.62, P = 0.015, R2 = 0.35) were correlated with non-native species cover in experimental and landscape communities. However, a T-test of the absolute difference between predicted and observed values indicated that PSF predictions of species cover were not closer to observed values than Null values (T(1, 15) = 1.15, P = 0.793).
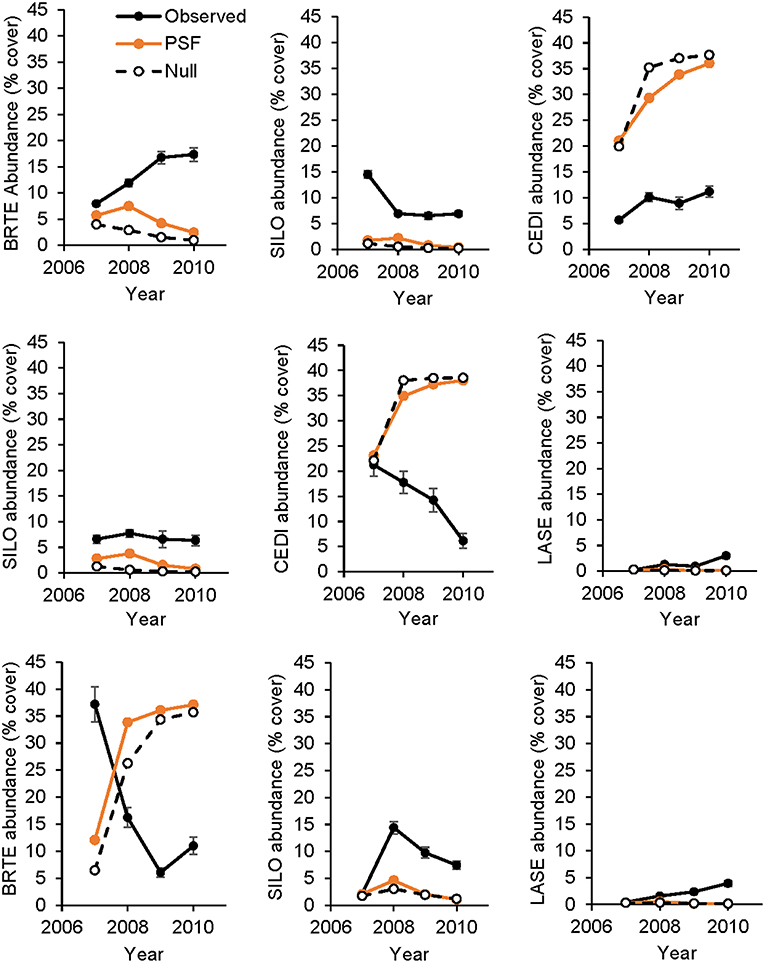
Figure 4. Observed and predicted abundance of non-native plant species in three-species experimental plant communities over time. Species in a row are from the same three-species community. Model predictions were made either with plant-soil feedback effects (PSF) or without plant-soil feedback effects (Null). BRTE, Bromus tectorum; CEDI, Centaurea diffusa; SILO, Syssymbrium loeselii; LASE, Lactuca serriola.
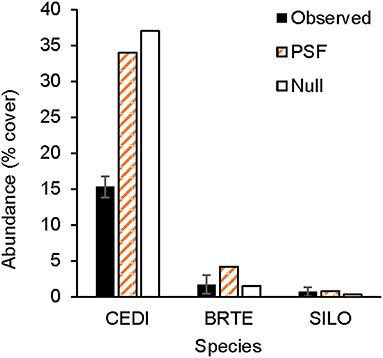
Figure 5. Observed and predicted abundance of non-native plant species in a three-species experimental plant community grown for 3 years (2011–2013). Model predictions were made either with plant-soil feedback effects (PSF) or without plant-soil feedback effects (Null). Species codes listed in the Figure 4.
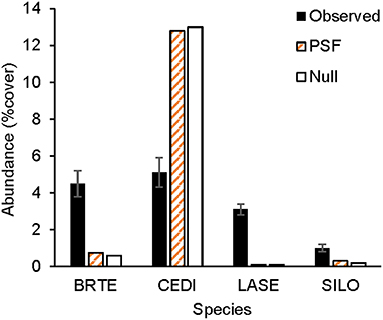
Figure 6. Observed and predicted non-native plant abundance. Observed plant abundance was recorded in 25 ex-arable fields over 13 years (Kulmatiski and Beard, 2019). Model simulations of plant community growth for these four common non-native species were performed for a 10-year period either with plant-soil feedback data (PSF; i.e., with information on plant growth rates on different soil treatments) or with a null model (Null) which was parameterized only with plant growth rates on control soils. BRTE, Bromus tectorum; CEDI, Centaurea diffusa; SILO, Syssymbrium loeselii; LASE, Lactuca serriola.
Discussion
Results from this 7-year field experiment provided uncommonly direct evidence for the role of PSFs in plant communities. As is commonly reported, plant growth differed among soil treatments for eight of 10 species (i.e., PSF; Tables 2, 3). More importantly, here it was shown that these PSFs were critical for predicting plant abundance, at least in native plant communities. Null model predictions, which only used plant growth data from common-garden or “control” soils, were not correlated with native plant cover in experimental plant communities, but when plant growth data from different soil treatments was included in this same model, predictions were well-correlated with plant cover in experimental plant communities (Figures 1, 2). Further, Null model predictions of rank-order abundance were incorrect for three out of four native experimental communities, but PSF model predictions were correct for all four communities. Further still, PSFs improved predictions of native plant growth on the landscape relative to Null model predictions (Figure 3). Across the experimental and landscape communities, PSF model predictions of native cover were well-correlated with observations (R2 = 0.62) while Null model predictions were not. In short, field-measured PSFs were critical for understanding native plant growth in plant communities in this system. This result is important because while PSFs are widely believed to be important in determining plant abundance, most evidence for the role of PSFs is derived from greenhouse experiments (Lekberg et al., 2018; Chung et al., 2019; Mack et al., 2019). Results from this study demonstrate that PSF measured in the field can help predict plant abundance in the field.
A logistic growth plant competition model in which competition coefficients are equal to a value of one was used in this study. In this type of model, the plant with the fastest growth rate will dominate the plant community. Among native species, the Null model incorrectly predicted K. cristata dominance because K. cristata attained the greatest cover on control soils. In contrast, the PSF model correctly predicted less K. cristata growth than the Null model because K. cristata grew poorly on “self” soils (i.e., negative PSF). The PSF model also correctly predicted P. spicata dominance because P. spicata grew well on “self” soils (i.e., a positive PSF). Thus, both positive and negative PSF were important for improving Null model predictions of plant growth in communities. This result is important because it provides an example of PSF effects in the context of intrinsic growth differences among species (Lekberg et al., 2018).
Because they are difficult to execute, factorial PSF experiments are uncommon (Reinhart and Rinella, 2016; Mack et al., 2019; Teste et al., 2019). As a result, little data is available to determine whether or not factorial experiments are needed to understand PSF effects in plant communities (Kulmatiski, 2016; Reinhart and Rinella, 2016; Teste et al., 2019). Results from the factorial portion of this study provided an example where factorial PSF data were needed to correctly predict plant community composition. K. cristata grew very poorly on P. spicata soils and well on F. idahoensis soils. As a result, K. cristata had a positive PSF with P. spicata soils and a negative PSF with F. idahoensis soils. These species-specific PSF values were critical for correct predictions of K. cristata and P. spicata cover. If K. cristata growth were predicted only from “self” and “control” soils, K. cristata would have been incorrectly predicted by a “self” vs. “other” PSF model to outcompete P. spicata.
While PSFs improved predictions of native plant abundance in communities, it is likely that other factors were also important to plant growth in this field experiment. It is possible, for example, that K. cristata may grow quickly in relatively high-resource monoculture plots, but that it is competitively suppressed in communities (Tsialtas et al., 2001; Fargione and Tilman, 2006; Lekberg et al., 2018). PSF effects and competitive suppression are not mutually exclusive, but it was not possible to determine the relative importance of these effects in this study. The fact that both the Null and PSF models overestimated K. cristata cover in experimental communities and in the field suggested that additional forms of negative density dependence may be needed to fully explain this species' abundance in native communities (Adler et al., 2007; Lekberg et al., 2018). Integration of PSF effects with other plant growth factors in conceptual and mathematical models has been widely recommended, but remains underdeveloped (van der Putten et al., 2013; Lekberg et al., 2018).
PSFs did not improve predictions for all species. Null and PSF model predictions of non-native cover did not differ and predictions from both models were poor relative to PSF model predictions of native cover. Null model predictions of non-native cover were poor because B. tectorum grew better in community plots than could be predicted from monoculture plots. Additionally, C. diffusa grew more poorly in community plots than could be predicted from monoculture plots. PSFs were either not large enough or not in the correct “direction” to improve Null model predictions. For example, because B. tectorum demonstrated a large positive PSF, the PSF model incorrectly predicted less B. tectorum cover in communities than the Null model.
Null and PSF model predictions of landscape-level non-native species cover were better than predictions of experimental species cover. Both models correctly predicted that C. diffusa would dominate and B. tectorum would be a subdominant with lower abundances of L. serriola and S. loeselii. A potential explanation for why landscape-level predictions of non-native cover where better than experimental-plot-level predictions is that landscape cover was determined from 25 fields that had been abandoned from agriculture between 1950 and 1999 (Kulmatiski and Beard, 2019). Averaging cover across these fields removes the large inter-annual variation seen in cover of the short-lived plants that dominate in these communities (Kulmatiski and Beard, 2019). For example, in experimental communities of native plants, rank-order abundance remained largely the same across 4 years of observations, while in non-native communities, rank-order abundance changed over time (Appendix Figure 1). It is likely that it is more difficult to predict the volatile dynamics of fast-growing, short-lived non-native species (Fukami and Nakajima, 2013; Suding et al., 2013).
A large body of research has suggested that PSF may help explain non-native and range-expanding plant success (Reinhart and Callaway, 2006; Eppstein and Molofsky, 2007; Suding et al., 2013). This study found that PSF improved predictions of native but not non-native plant community composition. These results are not mutually exclusive. Two previous studies in the study system explicitly examined the effects of native soils on non-native plants and non-native soils on native plants and found that PSFs can explain the presence of two alternate-state communities on the landscape (Kulmatiski et al., 2006; Kulmatiski, 2018). The current study focused on dynamics within native communities and within non-native plant communities. Here, it was found that PSFs were important in explaining growth within native plant communities but not within non-native plant communities.
Understanding the context under which PSF are important to plant community development remains a critical direction for future research (Bailey and Schweitzer, 2016; Smith-Ramesh and Reynolds, 2017; Fry et al., 2018). In addition to distinguishing the role of PSF in native vs. non-native plant communities, the context of study site and experimental design used in this study likely affected results. Relatively little is known about how PSF varies among ecosystems, but a literature review has suggested that they may be larger in the type of semi-arid system used in this study relative to more mesic (e.g., forested) systems (Kulmatiski et al., 2008). The duration of this study is unusual for PSF experiments and may also have been important (Kardol et al., 2013; Bezemer et al., 2018). It is possible that longer PSF experiments create PSF values that are more relevant to plant growth in communities due to co-selection or co-evolution in plant-microbial interactions that may take years to develop (Zuppinger-Dingley et al., 2016; van Moorsel et al., 2018). Determining how PSF develop over time is important for measuring and modeling plant communities but has rarely been addressed (Kardol et al., 2013; Bailey and Schweitzer, 2016; van Moorsel et al., 2018).
There is both strong evidence that plants change soils in ways that affect subsequent plant growth and also conceptual and mathematical evidence that these PSFs can maintain species diversity (Adler et al., 2007; Revilla et al., 2013; van der Putten et al., 2013). There are also a handful of studies that have found correlations between PSF and species abundance (Klironomos, 2002; Heinze et al., 2015), and a few studies have used observed PSF values in simulation models to explore potential PSF effects on plant coexistence and abundance (Chung and Rudgers, 2016; Bennett et al., 2017; Teste et al., 2017; Eppinga et al., 2018). Very few studies have attempted to explicitly predict plant growth using PSF data and simulation models (Mangan et al., 2010; Kulmatiski et al., 2016). This link is important because it is reasonable to expect that PSFs measured in monocultures may not reflect plant-plant or plant-soil-plant interactions in plant communities (Eisenhauer et al., 2012; Latz et al., 2012; Crawford and Knight, 2017; Lekberg et al., 2018). This study, therefore, provides an important link between classic PSF two-phase experimental data and plant growth in communities in the field (Poorter et al., 2016), and revealed that PSFs were critical for understanding native plant abundance in plant communities in the field.
Data Availability
The datasets generated for this study are available on request to the corresponding author.
Author Contributions
AK performed all aspects of this research.
Funding
This research was supported by the Utah Agricultural Experiment Station, Utah State University, and approved as journal paper #8983. Research was also supported by NSF award #1354129.
Conflict of Interest Statement
The author declares that the research was conducted in the absence of any commercial or financial relationships that could be construed as a potential conflict of interest.
The handling editor declared a past co-authorship with the author on two multi-authored papers.
Acknowledgments
Land use was approved by the Washington Department of Fish and Wildlife. Washington Department of Forest and Wildlife land managers, Jim Mountjoy, Kim Romain-Bondi, and Tom McCoy provided valuable advice and support for this research.
Supplementary Material
The Supplementary Material for this article can be found online at: https://www.frontiersin.org/articles/10.3389/fevo.2019.00326/full#supplementary-material
References
Adler, P. B., HilleRisLambers, J., and Levine, J. M. (2007). A niche for neutrality. Ecol. Lett. 10, 95–104. doi: 10.1111/j.1461-0248.2006.00996.x
Bailey, J. K., and Schweitzer, J. A. (2016). The rise of plant–soil feedback in ecology and evolution. Func. Ecol. 30, 1030–1031. doi: 10.1111/1365-2435.12691
Bennett, J. A., and Cahill, J. F. (2016). Fungal effects on plant-plant interactions contribute to grassland plant abundances: evidence from the field. J. Ecol. 104, 755–764. doi: 10.1111/1365-2745.12558
Bennett, J. A., Maherali, H., Reinhart, K. O., Lekberg, Y., Hart, M. M., and Klironomos, J. (2017). Plant-soil feedbacks and mycorrhizal type influence temperate forest population dynamics. Science. 355, 181–184. doi: 10.1126/science.aai8212
Bergmann, J., Verbruggen, E., Heinze, J., Xiang, D., Chen, B., Joshi, J., et al. (2016). The interplay between soil structure, roots, and microbiota as a determinant of plant–soil feedback. Ecol. Evol. 6, 7633–7644. doi: 10.1002/ece3.2456
Bever, J. D. (1994). Feedback between plants and their soil communities in an old field community. Ecology 75, 1965–1977. doi: 10.2307/1941601
Bever, J. D. (2003). Soil community feedback and the coexistence of competitors: conceptual frameworks and empirical tests. New Phytol. 157, 465–473. doi: 10.1046/j.1469-8137.2003.00714.x
Bever, J. D., Westover, K. M., and Antonovics, J. (1997). Incorporating the soil community into plant population dynamics: the utility of the feedback approach. J. Ecol. 85, 561–573. doi: 10.2307/2960528
Bezemer, T. M., Jing, J., Bakx-Schotman, J. T., and Bijleveld, E. J. (2018). Plant competition alters the temporal dynamics of plant-soil feedbacks. J. Ecol. 106, 2287–2300. doi: 10.1111/1365-2745.12999
Brinkman, E. P., Van der Putten, W. H., Bakker, E. J., and Verhoeven, K. J. F. (2010). Plant-soil feedback: experimental approaches, statistical analyses and ecological interpretations. J. Ecol. 98, 1063–1073. doi: 10.1111/j.1365-2745.2010.01695.x
Cesarz, S., Münzbergová, Z., and Eisenhauer, N. (2018). Soil microarthropods alter the outcome of plant-soil feedback experiments. Sci. Rep. 8:11898. doi: 10.1038/s41598-018-30340-w
Chung, Y. A., Collins, S. L., and Rudgers, J. A. (2019). Connecting plant–soil feedbacks to long-term stability in a desert grassland. Ecology 100:e02756. doi: 10.1002/ecy.2756
Chung, Y. A., and Rudgers, J. A. (2016). Plant–soil feedbacks promote negative frequency dependence in the coexistence of two aridland grasses. Proc. Biol. Sci. 283:20160608. doi: 10.1098/rspb.2016.0608
Compant, S., Duffy, B., Nowak, J., Clément, C., and Barka, E. A. (2005). Use of plant growth-promoting bacteria for biocontrol of plant diseases: principles, mechanisms of action, and future prospects. Appl. Environ. Microbiol. 71, 4951–4959. doi: 10.1128/AEM.71.9.4951-4959.2005
Crawford, K. M., and Knight, T. M. (2017). Competition overwhelms the positive plant–soil feedback generated by an invasive plant. Oecologia 183, 211–220 doi: 10.1007/s00442-016-3759-2
Eisenhauer, N., Reich, P. B., and Scheu, S. (2012). Increasing plant diversity effects on productivity with time due to delayed soil biota effects on plants. Basic Appl. Ecol. 13, 571–578. doi: 10.1016/j.baae.2012.09.002
Eppinga, M. B., Baudena, M., Johnson, D. J., Jiang, J., Mack, K. M. L., Strand, A. E., et al. (2018). Frequency-dependent feedback constrains plant community coexistence. Nat. Ecol. Evol. 2:1403. doi: 10.1038/s41559-018-0622-3
Eppstein, M. J., and Molofsky, J. (2007). Invasiveness in plant communities with feedbacks. Ecol. Lett. 10, 253–263. doi: 10.1111/j.1461-0248.2007.01017.x
Fargione, J., and Tilman, D. (2006). Plant species traits and capacity for resource reduction predict yield and abundance under competition in nitrogen-limited grassland. Funct. Ecol. 20, 533–540. doi: 10.1111/j.1365-2435.2006.01116.x
Fry, E. L., Johnson, G. N., Hall, A. L., Pritchard, W. J., Bullock, J. M., and Bardgett, R. D. (2018). Drought neutralises plant–soil feedback of two mesic grassland forbs. Oecologia 186, 1113–1125. doi: 10.1007/s00442-018-4082-x
Fukami, T., and Nakajima, M. (2013). Complex plant–soil interactions enhance plant species diversity by delaying community convergence. J. Ecol. 101, 316–324. doi: 10.1111/1365-2745.12048
Hawkes, C. V., Kivlin, S. N., Du, J., and Eviner, V. T. (2013). The temporal development and additivity of plant-soil feedback in perennial grasses. Plant Soil. 369, 141–150. doi: 10.1007/s11104-012-1557-0
Heinze, J., Sitte, M., Schindhelm, A., Wright, J., and Joshi, J. (2016). Plant-soil feedbacks: a comparative study on the relative importance of soil feedbacks in the greenhouse versus the field. Oecologia 181, 559–569. doi: 10.1007/s00442-016-3591-8
Heinze, J., Werner, T., Weber, E., Rillig, M. C., and Joshi, J. (2015). Soil biota effects on local abundances of three grass species along a land-use gradient. Oecologia 179, 249–259. doi: 10.1007/s00442-015-3336-0
Hitchcock, C. L., and Cronquest, A. (1973). Flora of the Pacific Northwest. University of Washington Press, Seattle, WA.
Kardol, P., De Deyn, G. B., Laliberté, E., Mariotte, P., and Hawkes, C. V. (2013). Biotic plant–soil feedbacks across temporal scales. J. Ecol. 101, 309–315. doi: 10.1111/1365-2745.12046
Klironomos, J. N. (2002). Feedback with soil biota contributes to plant rarity and invasiveness in communities. Nature 417, 67–70. doi: 10.1038/417067a
Kulmatiski, A. (2016). Factorial and ‘self vs. other’ plant soil feedback experiments produce similar predictions of plant growth in communities. Plant Soil. 408, 485–492. doi: 10.1007/s11104-016-2946-6
Kulmatiski, A. (2018). Community-level plant–soil feedbacks explain landscape distribution of native and non-native plants. Ecol. Evol. 8, 2041–2049. doi: 10.1002/ece3.3649
Kulmatiski, A., and Beard, K. H. (2019). Chronosequence and direct observation approaches reveal complementary community dynamics in a novel ecosystem. PLoS ONE 14:e0207047. doi: 10.1371/journal.pone.0207047
Kulmatiski, A., Beard, K. H., Grenzer, J., Forero, L., and Heavilin, J. (2016). Using plant-soil feedbacks to predict plant biomass in diverse communities. Ecology 97, 2064–2073. doi: 10.1890/15-2037.1
Kulmatiski, A., Beard, K. H., Norton, J. M., Heavilin, J. E., Forero, L. E., and Grenzer, J. (2017). Live long and prosper: plant–soil feedback, lifespan, and landscape abundance covary. Ecology 98, 3063–3073. doi: 10.1002/ecy.2011
Kulmatiski, A., Beard, K. H., and Stark, J. M. (2006). Soil history as a primary control on plant invasion in abandoned agricultural fields. J. Appl. Ecol. 43, 868–876. doi: 10.1111/j.1365-2664.2006.01192.x
Kulmatiski, A., Beard, K. H., Stevens, J. R., and Cobbold, S. M. (2008). Plant–soil feedbacks: a meta-analytical review. Ecol. Lett. 11, 980–992. doi: 10.1111/j.1461-0248.2008.01209.x
Kulmatiski, A., and Kardol, P. (2008). “Getting plant—soil feedbacks out of the greenhouse: experimental and conceptual approaches,” in Progress in Botany, Vol. 69, eds U. Lüttge, W. Beyschlag, and J. Murata (Berlin; Heidelberg: Springer), 449–472. doi: 10.1007/978-3-540-72954-9_18
Latz, E., Eisenhauer, N., Rall, B. C., Allan, E., Roscher, C., Scheu, S., et al. (2012). Plant diversity improves protection against soil-borne pathogens by fostering antagonistic bacterial communities. J. Ecol. 100, 597–604. doi: 10.1111/j.1365-2745.2011.01940.x
Lekberg, Y., Bever, J. D., Bunn, R. A., Callaway, R. M., Hart, M. M., Kivlin, S. N., et al. (2018). Relative importance of competition and plant–soil feedback, their synergy, context dependency and implications for coexistence. Ecol. Lett. 21, 1268–1281. doi: 10.1111/ele.13093
Lenfesty, C. D. (1980). Soil Survey of Okanogan County Area. Washington, DC: National Cooperative Soil Survey.
Levine, J. M., Pachepsky, E., Kendall, B. E., Yelenik, S. G., and Lambers, J. H. R. (2006). Plant–soil feedbacks and invasive spread. Ecol. Lett. 9, 1005–1014. doi: 10.1111/j.1461-0248.2006.00949.x
Mack, K. M. L., Eppinga, M. B., and Bever, J. D. (2019). Plant-soil feedbacks promote coexistence and resilience in multi-species communities. PLoS ONE 14:e0211572. doi: 10.1371/journal.pone.0211572
Mangan, S. A., Schnitzer, S. A., Herre, E. A., Mack, K. M. L., Valencia, M. C., Sanchez, E. I., et al. (2010). Negative plant-soil feedback predicts tree-species relative abundance in a tropical forest. Nature 466, 752–755. doi: 10.1038/nature09273
Poorter, H., Fiorani, F., Pieruschka, R., Wojciechowski, T., Putten, W. H., Kleyer, M., et al. (2016). Pampered inside, pestered outside? Differences and similarities between plants growing in controlled conditions and in the field. New Phytol. 212, 838–855. doi: 10.1111/nph.14243
Reinhart, K. O., and Callaway, R. M. (2006). Soil biota and invasive plants. New Phytol. 170, 445–457. doi: 10.1111/j.1469-8137.2006.01715.x
Reinhart, K. O., and Rinella, M. J. (2016). A common soil handling technique can generate incorrect estimates of soil biota effects on plants. New Phytol. 210, 786–789. doi: 10.1111/nph.13822
Revilla, T. A., Veen, G. C., Eppinga, M. B., and Weissing, F. J. (2013). Plant–soil feedbacks and the coexistence of competing plants. Theor. Ecol. 6, 99–113. doi: 10.1007/s12080-012-0163-3
Schittko, C., Runge, C., Strupp, M., Wolff, S., and Wurst, S. (2016). No evidence that plant–soil feedback effects of native and invasive plant species under glasshouse conditions are reflected in the field. J. Ecol. 104, 1243–1249. doi: 10.1111/1365-2745.12603
Smith-Ramesh, L. M., and Reynolds, H. L. (2017). The next frontier of plant–soil feedback research: unraveling context dependence across biotic and abiotic gradients. J. Veg. Sci. 28, 484–494. doi: 10.1111/jvs.12519
Suding, K. N., Harpole, S., Fukami, T., Kulmatiski, A., MacDougall, A. S., Stein, C., et al. (2013). Consequences of plant–soil feedbacks in invasion. J. Ecol. 101, 298–308. doi: 10.1111/1365-2745.12057
Teste, F. P., Kardol, P., Turner, B. L., Wardle, D. A., Zemunik, G., Renton, M., et al. (2017). Plant-soil feedback and the maintenance of diversity in Mediterranean-climate shrublands. Science 355, 173–176. doi: 10.1126/science.aai8291
Teste, F. P., Kardol, P., Turner, B. L., Wardle, D. A., Zemunik, G., Renton, M., et al. (2019). Toward more robust plant–soil feedback research: comment. Ecology e02590. doi: 10.1002/ecy.2590
Tsialtas, J. T., Handley, L. L., Kassioumi, M. T., Veresoglou, D. S., and Gagianas, A. A. (2001). Interspecific variation in potential water-use efficiency and its relation to plant species abundance in a water-limited grassland. Funct. Ecol. 15, 605–614. doi: 10.1046/j.0269-8463.2001.00555.x
van der Putten, W. H., Bardgett, R. D., Bever, J. D., Bezemer, T. M., Casper, B. B., Fukami, T., et al. (2013). Plant-soil feedback: the past, the present and future challenges. J. Ecol. 101, 265–276. doi: 10.1111/1365-2745.12054
van der Putten, W. H., Bradford, M. A., Pernilla Brinkman, E., van de Voorde, T. F. J., and Veen, G. F. (2016). Where, when and how plant–soil feedback matters in a changing world. Funct. Ecol. 30, 1109–1121. doi: 10.1111/1365-2435.12657
van Moorsel, S. J., Hahl, T., Wagg, C., De Deyn, G. B., Flynn, D. F., Zuppinger-Dingley, D., et al. (2018). Community evolution increases plant productivity at low diversity. Ecol. Lett. 21, 128–137. doi: 10.1111/ele.12879
Vincenot, C. E., Cartenì, F., Bonanomi, G., Mazzoleni, S., and Giannino, F. (2017). Plant–soil negative feedback explains vegetation dynamics and patterns at multiple scales. Oikos 126, 1319–1328. doi: 10.1111/oik.04149
Wubs, E. R., and Bezemer, T. M. (2018). Plant community evenness responds to spatial plant–soil feedback heterogeneity primarily through the diversity of soil conditioning. Funct. Ecol. 32, 509–521. doi: 10.1111/1365-2435.13017
Zhang, P., Li, B., Wu, J., and Hu, S. (2019). Invasive plants differentially affect soil biota through litter and rhizosphere pathways: a meta-analysis. Ecol. Lett. 22, 200–210. doi: 10.1111/ele.13181
Keywords: plant soil feedback, field experiment, invasive, native, plant community, model, factorial, prediction
Citation: Kulmatiski A (2019) Plant-Soil Feedbacks Predict Native but Not Non-native Plant Community Composition: A 7-Year Common-Garden Experiment. Front. Ecol. Evol. 7:326. doi: 10.3389/fevo.2019.00326
Received: 01 May 2019; Accepted: 13 August 2019;
Published: 28 August 2019.
Edited by:
Martijn Bezemer, Netherlands Institute of Ecology (NIOO-KNAW), NetherlandsReviewed by:
Debra Zuppinger-Dingley, University of Zurich, SwitzerlandKadri Koorem, Netherlands Institute of Ecology (NIOO-KNAW), Netherlands
Copyright © 2019 Kulmatiski. This is an open-access article distributed under the terms of the Creative Commons Attribution License (CC BY). The use, distribution or reproduction in other forums is permitted, provided the original author(s) and the copyright owner(s) are credited and that the original publication in this journal is cited, in accordance with accepted academic practice. No use, distribution or reproduction is permitted which does not comply with these terms.
*Correspondence: Andrew Kulmatiski, YW5kcmV3a3VsbWF0aXNraUBob3RtYWlsLmNvbQ==