- 1U.S. Department of Agriculture Forest Service, Rocky Mountain Research Station, Grasslands, Shrublands and Deserts Program, Reno, NV, United States
- 2U.S. Geological Survey, Nebraska Cooperative Fish and Wildlife Research Unit, School of Natural Resources, University of Nebraska, Lincoln, NE, United States
- 3U.S. Department of Agriculture, Forest Service, Rocky Mountain Research Station, Forest and Woodland Ecosystems Program, Flagstaff, AZ, United States
This review provides an overview and integration of the use of resilience concepts to guide natural resources management actions. We emphasize ecosystems and landscapes and provide examples of the use of these concepts from empirical research in applied ecology. We begin with a discussion of definitions and concepts of ecological resilience and related terms that are applicable to management. We suggest that a resilience-based framework for management facilitates regional planning by providing the ability to locate management actions where they will have the greatest benefits and determine effective management strategies. We review the six key components of a resilience-based framework, beginning with managing for adaptive capacity and selecting an appropriate spatial extent and grain. Critical elements include developing an understanding of the factors influencing the general and ecological resilience of ecosystems and landscapes, the landscape context and spatial resilience, pattern and process interactions and their variability, and relationships among ecological and spatial resilience and the capacity to support habitats and species. We suggest that a spatially explicit approach, which couples geospatial information on general and spatial resilience to disturbance with information on resources, habitats, or species, provides the foundation for resilience-based management. We provide a case study from the sagebrush biome that illustrates the use of geospatial information on ecological and spatial resilience for prioritizing management actions and determine effective strategies.
Introduction
Globally ecosystems are changing at an unprecedented rate largely due to human impacts, including land development and use, pollutants, invasive species, altered disturbance regimes, increasing CO2, and climate change. Changes in species distributions and the emergence of novel ecosystem states increasingly challenge our capacity to manage for biodiversity, ecosystem functioning, and human well-being (IPCC, 2014; Pecl et al., 2017). Effective management of ecosystems in this era of rapid change requires an understanding of an ecosystem's response not only to these stressors and disturbances but also to management actions. Clear formulation and application of ecological resilience concepts can provide the basis for managing ecosystems to enhance their capacity to cope with stressors and disturbances and help guide them through periods of reorganization. Periods of reorganization provide both crisis and opportunity, and management during these periods is critical. Ecological resilience concepts (see Table 1 for definitions) can provide the basis for increasing the capacity of systems to absorb, persist, and adapt to inevitable and unpredictable change (Curtin and Parker, 2014), and for taking advantage of management opportunities to transform systems to more desirable states.
Operationalizing ecological resilience concepts for management has been difficult because a framework for evaluating how ecosystem responses to disturbances and stressors vary over large heterogeneous landscapes and how this variation is related to ecological resilience has not been well developed or translated for the management community. Applying these concepts at scales relevant to management is becoming increasingly important as the scale and magnitude of ecosystem change increase. To date, much of the literature on ecological resilience has focused on theory, definitions, and broad conceptualizations regarding the application of resilience concepts (e.g., Gunderson, 2000; Folke et al., 2004, 2010; Walker et al., 2004; Folke, 2006; Gunderson et al., 2010). Much of the research has focused on the importance of species diversity and species functional attributes in affecting responses to stress and disturbance at fairly small (local) scales (e.g., Angeler and Allen, 2016; Baho et al., 2017; cf. Pope et al., 2014; Roberts et al., 2018).
Recently, two applications of ecological resilience have come to the forefront and are being used at scales relevant to management. Assessments of general resilience, or the broad ability of systems to maintain fundamental structures, processes, and functioning following disturbances (after Folke et al., 2010), are being used to evaluate differences in the responses of the ecosystems that comprise landscapes and identify which ecosystems are likely to exhibit critical transitions to alternative states (e.g., Hirota et al., 2011; Brooks et al., 2016; Levine et al., 2016). These assessments are based on an understanding of the relationships among an ecosystem's environmental characteristics, attributes and processes, and responses to stressors and disturbances (Chambers et al., 2014a,c, 2017a,b). Assessments of spatial resilience, or how spatial attributes, processes, and feedbacks vary over space and time in response to disturbances and affect the resilience of ecosystems (after Allen et al., 2016), are being used to evaluate the capacity of landscapes to support ecosystems and biodiversity over time. These assessments are based on an understanding of the changes in landscape composition and configuration in response to disturbances and the effects on ecosystems and species (Frair et al., 2008; Keane et al., 2009; Olds et al., 2012; Hessburg et al., 2013; McIntyre et al., 2014; Tambosi et al., 2014; Rappaport et al., 2015). The concept of spatial regimes (Sundstrom et al., 2017; Allen et al., 2018; Roberts et al., 2018) represents a novel integration of space into traditional regime shift and early warning research. Developing an understanding of both general and spatial resilience has become more tractable over time because of the rapid development of the field of landscape ecology and the number of tools and models now available (Turner, 1989; Wu and Loucks, 1995; McKenzie et al., 2011).
Managing for ecological resilience necessarily requires a multiscale approach because of the nested, hierarchical nature of complex systems (panarchy; Holling, 1973; Wu and Loucks, 1995; Allen et al., 2016). Incorporating larger scales provides the basis for directing limited management resources to those areas on the landscape where they are likely to have the greatest benefit (Holl and Aide, 2011; Allen et al., 2016; Chambers et al., 2017c). Restoration efforts or conservation measures for individual species or small areas within large landscapes are often applied with the best of intentions but are unlikely to succeed in the long-term if they do not consider the larger environmental context, pattern and process interactions, and essential ecosystem elements, such as biodiversity, habitat connectivity, and capacity to supply ecosystem services over time.
Here, we focus on the use of resilience concepts to guide natural resources management actions. We emphasize ecosystems and landscapes as focal levels of assessment and provide examples of the use of these concepts from empirical research in natural resources management. We begin by discussing definitions and concepts of ecological resilience and related terms that are applicable to management. We suggest that a resilience-based approach to management facilitates regional planning by providing the ability to locate management actions where they will have the greatest benefits. We review the six key components of a resilience-based approach, beginning with managing for adaptive capacity and selecting an appropriate spatial extent and grain. Critical elements include developing an understanding of the factors influencing the general and ecological resilience of ecosystems and landscapes, the landscape context and spatial resilience, pattern and process interactions and their variability, and relationships among ecological and spatial resilience and the capacity to support habitats and species. We suggest that a spatially explicit approach, which couples geospatial information on general and spatial resilience to disturbance with information on resources, habitats, or species, provides the foundation for prioritizing areas for management actions. We provide a simple decision support tool that illustrates the use of geospatial information on general and spatial resilience for prioritizing management actions and determining effective strategies.
Definitions
Definitions and concepts related to ecological resilience (Table 1) have been widely adapted in applied ecology, including conservation biology (Curtin and Parker, 2014), restoration ecology (Bradshaw and Chadwick, 1980; Aronson et al., 1993; Suding et al., 2004), range science (Westoby et al., 1989; Laycock, 1991; Briske et al., 2005, 2008), wildfire science (Moritz et al., 2011), fisheries ecology (Pope et al., 2014), and geomorphology (Brunsden and Thornes, 1979; Downs and Gregory, 1993; Phillips, 2009). In applied ecology, ecological resilience is often interpreted as a measure of the potential of a system to recover to a desired state, i.e., the capacity of an ecosystem to regain characteristic processes, structures, functions, and feedbacks following disturbance or management actions (e.g., Chambers et al., 2014a; Pope et al., 2014; Trombore et al., 2015; Seidl et al., 2016). However, it is important to recognize that ecological resilience also applies to undesirable states (Zelmer and Gunderson, 2009), which may be highly resilient to management actions designed to return them to an original state, or transform them into more desirable states. In this context, resilience management entails (1) actively maintaining or enhancing ecological processes, structural and functional characteristics, and feedbacks of intact or desirable states, (2) eroding the resilience of undesirable states and fostering transitions to more desirable alternative states, and (3) increasing the capacity of systems to cope with new or altered disturbance regimes and climate change (e.g., Pope et al., 2014).
Although many of the resilience definitions and concepts used in applied ecology were derived from Holling's (1973) original papers and resilience science, others have evolved independently. For example, in geomorphology, landform sensitivity is defined similarly to ecological resilience. It is the (1) the propensity of a system to change as governed by a set of driving and resisting forces, and (2) the capacity of the system to absorb or resist the effects of the disturbance (Downs and Gregory, 1993). Other definitions have been derived as new ecosystem threats and disciplines have emerged. For example, in invasive species ecology, resistance to invasion is defined as the abiotic and biotic attributes and ecological processes of an ecosystem that limit the population growth of an invading species (D'Antonio and Thomsen, 2004).
Confusion regarding use of the term resilience in applied ecology can arise because in disciplines such as disaster management, the term resilience is often used as a process, as in enhancing resilience. This use of the term is normative and should be avoided. In various other disciplines, like engineering and medicine, resilience is defined as a rate of recovery. Measuring rates of recovery is straight forward, and often desirable, but fails when a system is non-stationary and where thresholds and alternative states occur (Angeler and Allen, 2016). Striving for consistent use of the terms can help promote a common understanding of ecological resilience and facilitate its application to management. Recognizing the similarities and resolving the differences among the use of the definitions and concepts can help foster the necessary interdisciplinary collaboration for effective management.
Resilience Concepts
States, Transitions, and Thresholds
Following disturbances or management actions, ecosystems often fail to return to the pre-disturbance condition. One of the most important concepts related to ecological resilience is the idea that complex systems can exhibit non-equilibrium conditions and exist in various alternative states that differ in processes, structures, functions, and feedbacks (Lewontin, 1969; Holling, 1973). The existence of non-equilibrium dynamics and alternative states has been demonstrated for numerous systems. The causes of shifts in states can arise from human perturbations such as nutrient enrichment, nitrogen deposition, acid rain from NOx and SOx, over-harvesting of fisheries and wildlife stocks, and inappropriate livestock grazing (Scheffer et al., 2001; Folke et al., 2004; Sasakia et al., 2015). They can also emerge over time from a “command and control” approach in which management actions emphasize maximum output of one or few variables and ultimately reduce the range of natural variation in the system and result in a loss of system resilience, for example, by stabilizing river flows with dams, suppressing fires in fire-prone ecosystems, maximizing timber yield, or maintaining constant, high, deer populations (Holling and Meffe, 1996). Also, climate change may be further de-stabilizing processes, such as fire regimes (Westerling et al., 2006; Westerling, 2016; Littell et al., 2018) and affecting species distributions (Pecl et al., 2017; Shirk et al., 2018).
The actual shift in states may be triggered by stochastic events such as climatic extremes, disturbances like floods or wildfires, increased contagion of forest area, fuels, and forest density or insect outbreaks (Scheffer et al., 2001; Folke et al., 2004). They may also occur more gradually, for example, with changes in soil properties due to warming, nutrient enrichment, or acid rain that result in gradual species replacements, changes in functional group composition, and changes in trophic structures. Some of the best-studied examples include eutrophication of lakes and coastal oceans, shifts among grassy and woody cover types in rangelands, degradation of coral reefs, and regional climate change (Scheffer et al., 2001; Folke et al., 2004; Sasakia et al., 2015).
Systems can respond to disturbances or management actions in several different ways; developing an understanding of the tendency of a system to change states, and the factors influencing a change in state, is a key component of resilience-based management. The tendency of a system to shift states has often been illustrated using a ball and trough analogy. The size of the valley around a state (trough) is described as the basin of attraction and corresponds to the range of disturbance that a system (ball) can absorb without causing a shift to an alternative stable state, while the depth of the cup describes the intensity of disturbance that can be tolerated (Holling, 1973). Transitions among states are a function of the abiotic or biotic variables or events, acting independently or in combination, that contribute directly to loss of state resilience and result in shifts between states (Caudle et al., 2013). Thresholds represent the point at which there is an abrupt change in states, or where small changes in one or more external conditions produce large and persistent responses in an ecosystem (Angeler and Allen, 2016).
Some of the factors influencing a change in state for systems with high vs. low resilience are in Table 2. A system with a strong basin of attraction can absorb change and remain within the same state over a range of disturbances and management actions. These types of systems have been described as having relatively high ecological resilience (Scheffer et al., 2012). Conditions that contribute to a strong basin of attraction include favorable environmental conditions, strong feedbacks at multiple scales, and high levels of functional diversity and redundancy, which can stabilize the system and disturbances within the range of historic variability. A system with a weak basin of attraction may respond strongly to disturbance and move to an alternative state (Table 2). These types of systems have been described as having relatively low ecological resilience (Scheffer et al., 2012). Conditions that contribute to a weak basin of attraction include less favorable environmental conditions, inadequate species or functional groups to stabilize the systems, and disturbances that are outside of the range of historic variability. These systems typically represent the greatest challenge for managers as active management is often required and return to the initial state may not be possible if new conditions (e.g., increased CO2, climate warming, changes in soil chemistry or structure, invasive species) are driving the state change. A system with more than one basin of attraction may respond to disturbance by changing states and moving to a new basin of attraction, but reorganize and return to the original state once conditions improve (Table 2). These types of systems have high adaptive capacity to changes in environmental conditions (e.g., drought, flooding) or management (e.g., grazing, harvest rates).
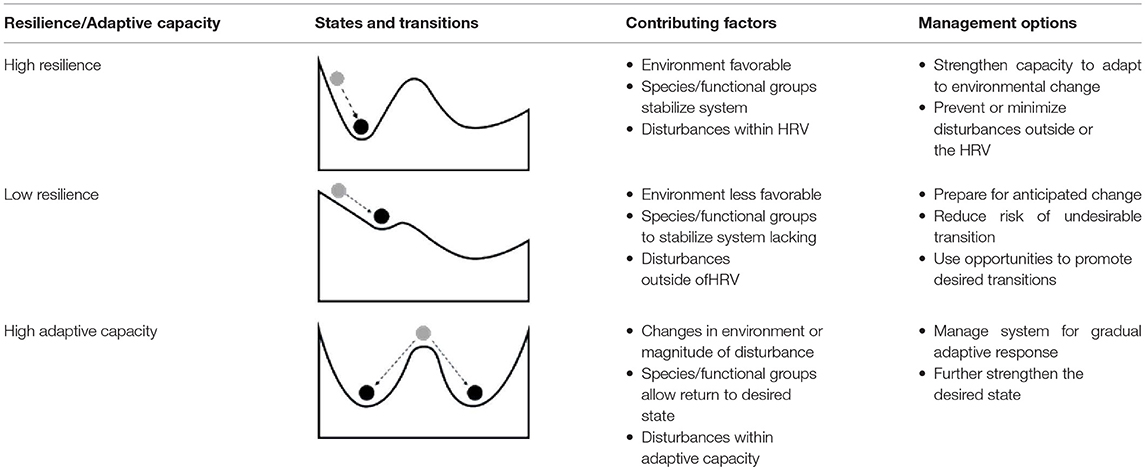
Table 2. Ball and trough diagrams illustrating differences in the response of ecosystems to stressors and disturbances, the factors that contribute to ecological resilience and adaptive capacity, and the management implications [adapted from Scheffer et al. (2012)].
Simple ball and trough diagrams help to conceptualize the changes possible in systems and the driving forces behind the changes, but in reality systems are highly complex and can exhibit multiple different trajectories and alternative states over time depending on the environmental factors, species and functional groups, and the type and characteristics of the disturbance. Also, system trajectories may be non-stationary due to a variety of internal and external drivers (Sundstrom et al., 2017). For example, with continued warming the relationship between climate and ecosystem responses to disturbances and management actions is likely to shift and managing for historical conditions may not maintain ecosystem goods, services, values, and biological diversity into the future (Millar et al., 2007; Hobbs et al., 2009). Recent analyses suggest that rather strong self-organization (positive feedbacks) keeps systems together, and that they may move in response to changing conditions unless or until a hard (e.g., mountain range) or incompatible boundary (strong soil difference) is reached (Allen et al., 2018; Roberts et al., 2018).
State-and-transition models (STMs) have long been used to describe the alternative states within ecosystems, factors causing the transitions, rates of transition, and potential restoration pathways. Range scientists and managers were among the first to adopt these concepts to describe changes in vegetation community composition due to factors such as drought, livestock grazing, and management actions (Archer, 1989; Westoby et al., 1989; Friedel, 1991; Laycock, 1991). Well codified STMs applicable to rangelands across the western U.S. have been developed by the USDA National Resources Conservation Service and their partners (Stringham et al., 2003; Briske et al., 2005; Bestelmeyer et al., 2009; Caudle et al., 2013). Most STMs developed for rangelands represent conceptual models based on expert opinion. However, empirically derived STMs have been used to relate plant community composition to factors such as climate, hydrologic regimes, soil processes, and management actions (Zweig and Kitchens, 2009; Karchergis et al., 2011; Bino et al., 2015), and to land-use change and changing disturbance regimes (Provencher et al., 2007; Daniel et al., 2016). Also, longer-term vegetation data have been used to evaluate transitions among communities, transition frequency, magnitude of accompanying compositional change, presence of unidirectional trajectories, and lack of reversibility within various timescales (Bagchi et al., 2012; Jamiyansharav et al., 2018).
Caution is needed when applying STMs to management problems. Most STM models require initial parameterization that involves assumptions about the numbers and types of states, their transition times, biotic and abiotic disturbances and stresses that create transitions or advance succession, and the frequency, patch sizes, and intensities of disturbances that might be expected. In essence, STMs do what the user tells them to do and consequently there is little opportunity for surprise. Thus, it is important to have relevant, independent datasets from the systems under observation, in order to validate and calibrate STM models before the results are accepted as representative of the modeled system (Keane, 2012).
Ecological, General, and Spatial Resilience
Integration of resilience concepts with landscape concepts provides the basis for understanding how ecosystem attributes and processes interact with landscape structure to influence the responses of ecosystems to disturbances and stressors and their capacity to support resources, habitats, and species over time. In this context, the concepts of ecological, general, and spatial resilience are interrelated (Figure 1, Table 3). The ecological resilience of ecosystems and general resilience of large landscapes is a function of environmental characteristics, disturbance regimes, ecosystem attributes and processes, and ecological memory (e.g., Chambers et al., 2014a, 2016b; Germino et al., 2016). Environmental factors, including climate, topography, and soils, determine the abiotic and biotic attributes of ecosystems. The disturbances with which ecosystems evolved, such as drought, extreme wet periods, fire, wind throw, and flooding, influence both abiotic and biotic ecosystem attributes and processes (Pickett and White, 1985; Pickett et al., 1989) and thus ecological memory (Peterson et al., 1998; Johnstone et al., 2016). Anthropogenic disturbances, management actions, and climate change act not only on the abiotic and biotic attributes and processes of ecosystems, but also on ecosystem disturbance regimes to affect ecological and general resilience over time.
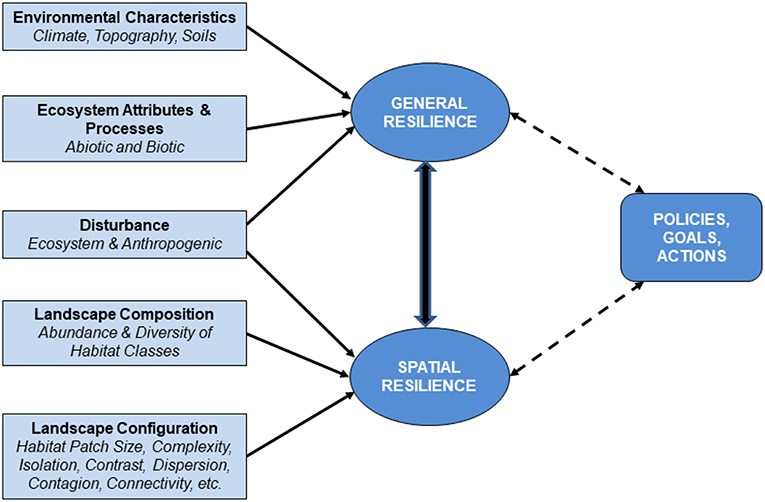
Figure 1. Illustration of the primary factors that influence ecological, general, and spatial resilience. Ecological resilience of ecosystems and general resilience of large landscapes to ecosystem and anthropogenic disturbances is a function of environmental characteristics, ecosystem attributes and processes, and ecosystem and anthropogenic disturbances. In the context of landscapes, spatial resilience to disturbance is determined largely by the composition, configuration, and functions of patches within landscapes. An understanding of ecological, general, and spatial resilience provides the ability to develop resilience-based frameworks and decision support tools to inform management policies, goals, and actions.
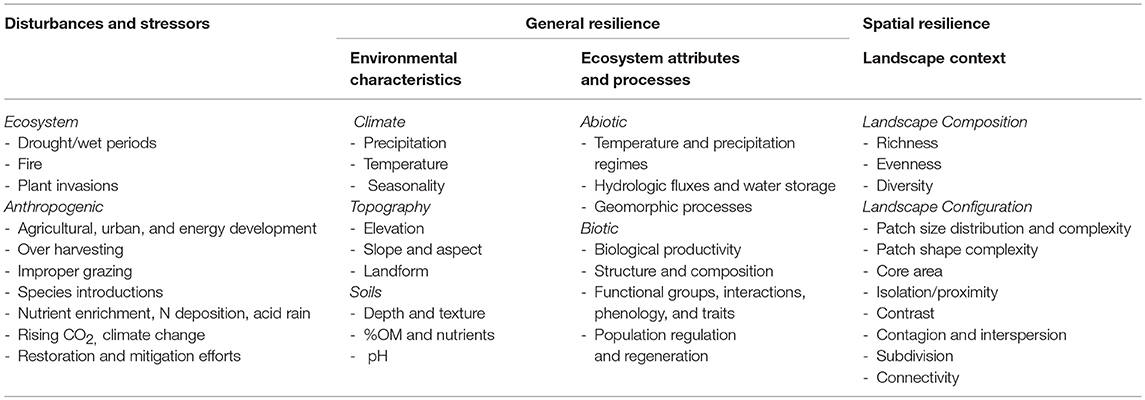
Table 3. Common disturbances and stressors, the factors that contribute to ecological, general, and spatial resilience to disturbances and stressors, and select indicator variables for each factor.
In a landscape context, spatial resilience to disturbance is largely determined by the composition, configuration, and functions of patches within landscapes (Figure 1, Table 3). Spatial resilience is an emergent property of the spatial arrangement, differences, and interactions among internal elements (i.e., those within the focal system), external elements (i.e., those outside the focal system), and other spatially relevant aspects of resilience (Cumming, 2011a,b). Ecosystem disturbances and stressors influence spatial patterns and constrain processes, and processes, in turn, feedback to drive the dynamics of pattern in landscapes. Anthropogenic disturbances, management actions, and climate change affect patterns and processes and thus spatial resilience over time.
Understanding the multi-scale patterns and process within landscapes that determine ecological and spatial resilience provides the underpinning for resilience-based management. Landscapes are hierarchical in nature and the levels are cross-connected (panarchy; Angeler and Allen, 2016). Differences in interactions among climate, vegetation, and disturbance exist at patch, meso, and broad landscape scales, influence different aspects of ecological and spatial resilience, and inform different aspects of the planning process. Assessments of ecological and spatial resilience conducted at meso to broad scales can be used to inform budget prioritization for management actions, such as pre-positioning of firefighting resources and post-fire rehabilitation, across ecoregions or even biomes (Chambers et al., 2017a,c). An understanding of the factors that influence ecological and spatial resilience at patch to meso scales, coupled with local data and expertise, can be used to select project areas and determine appropriate management strategies and treatments within areas prioritized for management (Chambers et al., 2017a,c).
Understanding ecological, general, and spatial resilience provides the capacity to develop resilience-based frameworks and decision support tools to inform management policies, goals, and actions (Figure 1). Geospatial information and knowledge of how the general resilience of ecosystems differs across large landscapes provides the basis for assessing relative ecosystem recovery potentials and risks of crossing critical thresholds (Chambers et al., 2017a,c; Ricca et al., 2018). Geospatial information and knowledge of how spatial resilience differs across the same landscapes provides the basis for evaluating spatial constraints on ecosystem recovery potential, availability of resources and habitats to support biodiversity, and connectivity among resources and habitats (Holl and Aide, 2011; Rudnick et al., 2012; McIntyre et al., 2014; Rappaport et al., 2015; Thatte et al., 2018; Kaszta et al., 2019). Combining information on ecological and spatial resilience with an understanding of the predominant ecosystem and anthropogenic disturbances and capacity to support habitats and species provides the basis for prioritizing management actions and determining effect strategies.
The Components of Resilience-Based Management
A resilience-based approach for developing conservation and restoration priorities and determining effective strategies has several components (Table 4). Each of these components should be considered when developing resilience-based management plans for large landscapes.
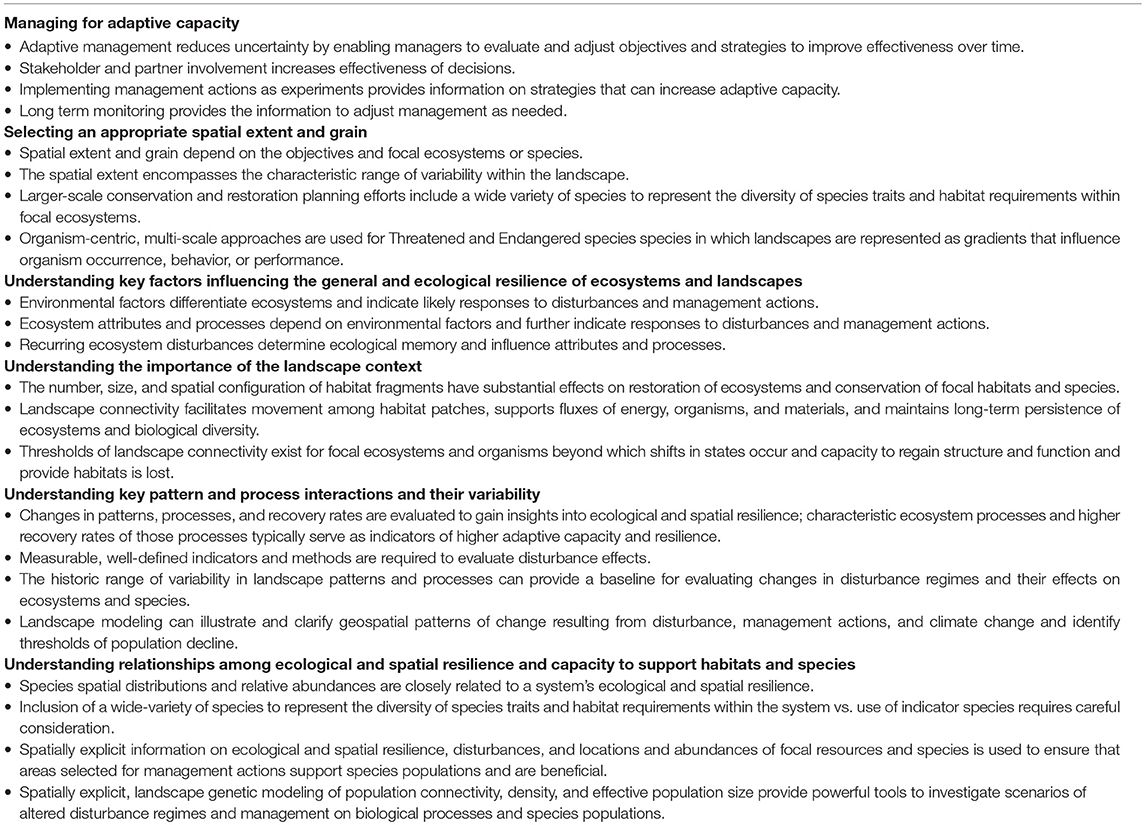
Table 4. Key components of a framework for resilience-based management that informs conservation and restoration priorities and strategies.
Managing for Adaptive Capacity
Resilience-based management will be most effective when developed in the context of long-term adaptive management programs. Adaptive management reduces uncertainty in the effectiveness of, and responses to, management actions by evaluating and adjusting management objectives and strategies to improve the effectiveness of management actions over time. Integrated adaptive management programs are a form of structured decision making (sensu Gregory et al., 2012) that facilitate “learning by doing” and aid land managers and stakeholders in examining the context, options, and probable outcomes of decisions through an explicit and repeatable process (Allen et al., 2011; Williams, 2011; Marcot et al., 2012). A framework that includes evaluating the effects of environmental drivers and management interventions on ecological resilience can provide the basis for developing an increased understanding of resilience over time and incorporating that learning into management (Johnson et al., 2013; Brown and Williams, 2015). The first step of the process, assessment, involves defining the problem, identifying objectives, and determining evaluation criteria. Key components are assessing the available information and data and eliciting both input from experts (Runge et al., 2011) and feedback from stakeholders and partners (Gregory et al., 2012). Benchmarks and references for evaluating success are developed that account for the historic range of variability (Keane et al., 2009; Hessburg et al., 2013; Seidl et al., 2016) and ecological memory (Peterson et al., 1998; Johnstone et al., 2016), but factor in ongoing changes (Millar, 2014). In the next step, design, the alternatives are defined, consequences and key uncertainties identified, and tradeoffs evaluated. The preferred alternative is then determined, and the decision is made to implement the preferred alternative and management action(s). Long-term monitoring is the last step and is key to assessing effects of management actions on resilience and learning over time (Angeler and Allen, 2016). Monitoring is used to evaluate ecological status and trends and whether or not management objectives for increasing ecological resilience are met, and then to adjust management objectives and actions as needed.
Dealing with uncertainty is one of the greatest challenges in decision making. Changes in administrative priorities, policies, and economic resources can all cause uncertainty in the types of decisions that should be made as well as the outcomes of those decisions. Several well-recognized sources of uncertainty exist that are specific to making natural resource decisions (USDI, 2009; Conroy et al., 2011; Williams, 2011). First, environmental uncertainty, or uncertainty in ecosystem and species responses to factors such as disturbances, weather events, climate change, and management actions, is a well-known source of uncertainty that characterizes all natural systems and requires little explanation. Second, partial observability, or the need to estimate and model the relevant “quantities” that characterize natural systems because of our inability to directly observe nature, often limits our ability to accurately determine the resource “quantities” that are the targets of management. For example, the hectares of habitat to support a particular species are often estimated from limited research on habitat requirements, often in a different location. Third, partial controllability, is the frequent inability to apply management actions directly and with high precision. This can lead to misinterpretation of the effectiveness of management actions. Fourth, structural uncertainty, is the uncertainty in the models that predict system responses to specific management actions. Structural uncertainty is often represented by alternative models of system dynamics, each with associated measures of relative credibility. Reducing this type of uncertainty is a key objective of adaptive management (Runge et al., 2011).
Dealing with uncertainty in decision making requires recognizing its existence, establishing rules whereby an optimal decision can be made in the face of uncertainty, and reducing uncertainty where possible (Conroy et al., 2011; Williams, 2011). There is increasing recognition that effectively addressing uncertainty and facilitating decision-making in the context of adaptive management may require new laws, policies, guidelines, or funding structures (Garmestani and Benson, 2013).
Selecting an Appropriate Spatial Extent and Grain
For planning purposes, the landscape must reflect a meaningful spatial extent and grain for the focal ecosystems and species (Cushman et al., 2013), and be representative of the characteristic range of variability within the landscape (Keane et al., 2009; Wiens et al., 2012). Assessing a larger range of conditions than occurs within the focal landscape provides the necessary information on the typical or characteristic variability of a landscape with high ecological and spatial resilience relative to the landscape of interest (Keane et al., 2009; Keane, 2012). For example, to understand how a particular forest or shrubland type interacts with its fire regime and develop meaningful benchmarks for fuels treatments and other management actions, it is necessary to understand the range of characteristics and spatial extents of the areas that burned historically within the type.
In larger-scale conservation and restoration planning efforts, the spatial extent of the landscape should include a wide variety of species to represent the diversity of species traits and habitat requirements within the focal ecosystems (e.g., Fajardo et al., 2014). Resilience is posited to derive, in part, from the distribution of species diversity within and across scales (and in particular, the diversity of functional traits; Peterson et al., 1998). Ecological systems can often compensate for the loss or population reduction of single species, though resilience may be diminished (Sundstrom and Allen, 2014; Sundstrom et al., 2018).
For planning efforts involving threatened or endangered (T&E) species, an organism-centric, multi-scale approach has been advocated (e.g., McGarigal and Cushman, 2005; Cushman et al., 2010) in which landscapes are represented as a series of gradients that influence organism occurrence, behavior, or performance. These landscapes often occupy spatial scales intermediate between an organism's normal home range and its regional distribution, but may encompass the entire range of a species or subspecies confined to a particular biome or set of ecoregions. Thus, it is most pragmatic to consider landscapes as having a large extent (>1,000's−10,000's of hectares) composed of an interacting mosaic of ecosystems and encompassing populations of many species.
In many cases, landscape boundaries are linked to management jurisdictions, such as parks or reserves (e.g., Schweiger et al., 2016). Landscapes defined by humans may or may not correspond with natural boundaries or spatial regimes (Sundstrom et al., 2017; Roberts et al., 2018). Identifying scales in ecosystems has been a major effort of resilience research in recent years; techniques have been described in Angeler and Allen (2016) and Allen et al. (2016), and include discontinuity analysis (Allen and Holling, 2008), Fisher Information (Spanbauer et al., 2014) and Multivariate Time Series Modeling (Angeler et al., 2016). The idea of spatial regimes has been used to identify self-similar, self-organizing, but non-stationary geographic regions. Combining spatial regime approaches with techniques that can identify natural scaling within a given regime holds promise for increasing understanding of spatial resilience in terrestrial ecosystems.
Understanding Key Factors Influencing the General and Ecological Resilience of Ecosystems and Landscapes
An understanding of the ecological and general resilience of ecosystems and landscapes provides the necessary information to (1) evaluate the differences in ecosystem responses to disturbance and their recovery potentials across landscapes, and (2) identify locations where ecosystems may exhibit critical transitions to novel alternative states in response to altered local or global drivers. Resilient ecosystems and landscapes have the ability to return to the prior or desired state.
Environmental characteristics are typically strong indicators of general and ecological resilience and are important factors in resilience-based assessments (Figure 1; Table 3). The early resilience literature identified the importance of a system's underlying environmental characteristics in determining the response of its component ecosystems to disturbance (Pickett and White, 1985; Pickett et al., 1989) at biome to patch scales (MacMahon, 1981; Turner, 1989; Wu and Loucks, 1995). Temperature coupled with amount and seasonality of precipitation largely determines the dominant life forms, ecological types, and productivity of ecosystems. An ecosystem's general resilience typically decreases as climatic conditions become more extreme (e.g., cold temperatures, hot temperatures coupled with low precipitation, low and variable precipitation; MacMahon, 1981). For example, amount of precipitation has been shown to strongly influence general resilience to changes in annual precipitation and other drivers at continental and regional scales. On the continents of Africa, Australia, and South America, spatial analyses of tree cover and annual precipitation indicate that changes in the general resilience of tropical forest, savanna, and treeless states varies in a universal way with precipitation and show where forest or savanna may most easily shift into an alternative state (Hirota et al., 2011). Relationships among seasonality of precipitation and growing season temperature also affect general resilience at regional scales (e.g., Paruelo and Lauenroth, 1996; Sala et al., 1997; Levine et al., 2016).
Ecosystem attributes and processes are important factors in analyses of general resilience and can include land cover of vegetation types, productivity indices, species functional traits, and modeled ecosystem processes, such as soil temperature and moisture regimes (Bradford et al., in press), and ecophysiological processes (Levine et al., 2016; Table 3). Longer term data on effects of disturbances and management actions and climate change projections make it possible to assess ecosystem state changes over time and to evaluate the potential for climate-induced thresholds (Hirota et al., 2011; Levine et al., 2016). For example, in Amazonia, remote-sensing and ground-based observations combined with size- and age-structured terrestrial ecosystem models demonstrate that water stress operating at the scale of individual plants, along with the spatial variation in soil texture, explains observed patterns of variation in ecosystem biomass, composition, and dynamics across the region, and strongly influences the response of the different ecosystems to changes in dry season length (Levine et al., 2016).
In a wide variety of systems, general and ecological resilience vary over environmental gradients at small landscape scales where aspect, slope, and topographic position affect solar radiation, erosion processes, effective precipitation, and soil development and, thus, the composition, structure, and productivity of communities. These environmental gradients influence land uses, such as livestock grazing (Bestelmeyer et al., 2011), disturbance patterns, such as the occurrence and severity of wildfires (Hessburg et al., 2016), ecosystem responses to those land uses and disturbances (e.g., Condon et al., 2011; Davies et al., 2012; Spasojevic et al., 2016), and restoration potential (Holl and Aide, 2011; de Souza Leite et al., 2013).
Integrated analyses of longer-term geospatial data, field data, and historical reconstructions provide the basis for understanding effects of disturbances on ecosystem attributes and processes over time and thus their ecological memory (Johnstone et al., 2016). The ecological memory of ecosystems is strongly associated with ecological and general resilience (Peterson et al., 1998; Peterson, 2002). Recurring ecosystem disturbances with characteristic frequency, severity, size, or other attributes influence geomorphic and hydrologic process and affect biogeochemical processes. These disturbances also exert strong selective pressure on species life-history strategies, which affect population survival and spread (Keeley et al., 2011). The processes, traits, individuals, and materials that persist after a disturbance, or the ecological memory of the system, shape responses to future disturbance (Johnstone et al., 2016). Ecological memory may be encoded across a range of spatial and temporal scales, from small, patch-scales to broad landscapes, and from decadal to evolutionary timescales (Johnstone et al., 2016).
In the context of landscapes, both the environmental characteristics and ecological memories of the focal systems influence general resilience. For example, in the four-corner region of the USA, remote sensing and species trait databases were used in combination with path analyses to evaluate if functional diversity across a range of woodland and forest ecosystems influences the recovery of productivity after wildfires (Spasojevic et al., 2016). Both topography (slope, elevation, and aspect) and functional diversity in regeneration traits (fire tolerance, fire resistance, ability to resprout) directly or indirectly influenced the recovery of productivity after wildfires.
Understanding the Importance of the Landscape Context and Spatial Resilience
An understanding of spatial resilience in the context of landscapes provides the necessary information for creating structurally and functionally connected networks that provide ecosystem services and conserve resources and species. Landscape patterns can either facilitate or impede the flow or movement of individuals, genes, and ecological processes. The landscape context is a critical element in both restoration and conservation ecology for (1) understanding the effects of disturbance on landscape patterns and processes, (2) evaluating the number, size, and spatial configuration of habitat fragments and degree of connectivity required to support restoration of ecosystems and conservation of focal habitats and species, and (3) determining thresholds of connectivity beyond which the capacity to regain structure and function is lost (Holl and Aide, 2011; Rudnick et al., 2012; McIntyre et al., 2014; Rappaport et al., 2015; Ricca et al., 2018).
Measuring metrics of the composition and configuration of landscapes (e.g., McGarigal et al., 2012) (Table 3) provides a quantitative framework to assess spatial structure and relate it to spatial resilience. Quantifying the range of states within a system in the context of landscape patterns under different disturbance and other process regimes is a core element of quantifying spatial resilience. A wide variety of tools and models exist for identifying landscape pattern metrics that provide interpretable (Neel et al., 2004) as well as consistent, universal, and strong measures of major attributes of landscape spatial structure (Cushman et al., 2008).
The landscape context can be as important as its general resilience and local site characteristics in influencing restoration effectiveness via effects related to the amount of habitat cover, connectivity among habitats, and relative isolation (de Souza Leite et al., 2013; Tambosi et al., 2014). Restoration effectiveness generally increases for restored areas in close proximity to neighboring patches and in landscapes with high habitat cover (see review in de Souza Leite et al., 2013). It also decreases with progressive changes in landscape development over time (Rappaport et al., 2015). Effects of landscape characteristics on restoration outcomes may vary with species characteristics and differ according to the population or community parameters (e.g., abundance, richness, composition) considered (de Souza Leite et al., 2013). Also, different landscape aspects mediate the effects of restoration actions on ecosystems, and the landscape metrics used for planning and monitoring need to be tailored to the system of interest.
The landscape context and spatial resilience are a central part of modern conservation ecology. The spatial composition and configuration of habitat plays a critical role in affecting species persistence (With and King, 1999); long-term persistence requires a sufficient number, size, and spatial configuration of habitat fragments (Hanski and Ovaskainen, 2002). The habitat requirements of species are individualistic, because each species has a unique ecological niche, which differs from that of all other species; multidimensional, because several to many important environmental variables typically define each species' habitat; and multiscale, because each of these environmental variables is likely to be related to space or other resource use at different spatial scales (e.g., Grand et al., 2004; Wasserman et al., 2012). For example, bald eagle habitat selection is driven by a number of environmental variables, but selection of each kind of habitat (such as for foraging or roosting locations) is driven by different variables at different scales (Thompson and McGarigal, 2002).
The “metapopulation capacity” is the likelihood of long-term population viability given a particular extent, configuration, and quality of habitat. Habitat loss and fragmentation reduce the metapopulation capacity of a landscape and make extinction more likely. Thus, in addition to knowing the extent and quality of the remaining habitat, identifying the habitat's spatial configuration and connectivity is essential to determining the effects on population size (Ovaskainen, 2002). The adverse effects of habitat loss and fragmentation on biodiversity can be divided into two dominant categories. First, as habitat is lost from the landscape, at some point there will be insufficient area of habitat to support a population, and the species will be extirpated from the landscape (Flather and Bevers, 2002). This is referred to as the area effect. Second, as habitat is lost and fragmented, individual habitat patches become more isolated from one another. As populations become subdivided, the movement of individuals among habitat patches (e.g., dispersal) may decrease or cease altogether, which may affect critical metapopulation processes such as gene flow, demographic rescue, and recolonization following local extinction (Fahrig and Merriam, 1994). This is referred to as the isolation effect.
Landscape connectivity is the ability of a landscape to facilitate or impede movement among habitat patches, support fluxes of energy, organisms and materials (e.g., seeds, biomass, pollen, nutrients, sediments) and maintain long-term persistence of both ecosystems and biological diversity (Saura and Pascual-Hortal, 2007; Foltête et al., 2012; Ng et al., 2013). It is a function of both the characteristics of the landscape (structural connectivity) and organism mobility (functional connectivity). A well-connected landscape enhances the spatial resilience of systems, allowing them to overcome sudden changes (e.g., climate changes, wildfires) by persistence, adaptation, and transformation processes. A reduction in landscape connectivity can be considered an early-warning indicator of shifts among stable or metastable states of systems (Zurlini et al., 2014). Landscape connectivity has been used as a surrogate for spatial resilience in ecoregional planning for wildlife (Cushman and Landguth, 2012; Cushman et al., 2016, 2018), forest (Theobald et al., 2011) and invasive species management (Alistair et al., 2013). It has also been used to evaluate the loss of individual wetlands in wetland complexes (Uden et al., 2014) and ecosystem provisioning for humans (Wu, 2013).
Landscape connectivity is an important measure of the spatial resilience of systems to climate change and other perturbations. For example, climate controls connectivity among prairie wetlands for migratory birds within and across the three main wetland complexes in the Great Plains of North America (McIntyre et al., 2014). Climate projections and bird species data suggest that changes in precipitation patterns due to climate change will likely reduce wetland network density and connectivity and result in reduced bird abundance where dispersal capacity will be as important as wet/dry conditions (McIntyre et al., 2014).
Thresholds of connectivity can be identified beyond which systems shift states and lose the capacity to provide resources and habitats (Frair et al., 2008; Thatte et al., 2018; Kaszta et al., 2019). For example, dense human settlements and roads with high traffic are detrimental to tigers (Panthera tigris) in Central India. Landscape genetics analyses and spatially-explicit simulations were used to examine current population connectivity of tigers across nine reserves (Thatte et al., 2018). Landscape genetic simulations modeled potential impacts of different scenarios of future land-use change and found that genetic variability (heterozygosity) will likely decrease in the future and small or isolated populations will have a high risk of local extinction. Scenarios where habitat connectivity was enhanced and maintained, stepping-stone populations were introduced/maintained, and tiger numbers were increased, led to lower overall extinction probabilities. As another example, to evaluate effects of alternative development and conservation scenarios on clouded leopards (Neofelis nebulosa) across Sabah, Borneo, coupled individual-based population, and genetic models were used (Kaszta et al., 2019). Landscape connectivity was highly correlated with predicted local population density and genetic diversity of clouded leopards, and there were substantial differences in how much each scenario impacted the distribution, abundance, and genetic diversity of the species.
Understanding Key Pattern and Process Interactions and Their Variability
Information on extents and patterns of disturbances and their interactions with ecosystem attributes and processes facilitates land use planning and enables selection of effective management strategies. Assessments can be designed to (1) evaluate the extents and magnitude of ecosystem and anthropogenic disturbances, (2) assess status and trends based on a recent history, and (3) identify thresholds of change in structure and function.
The impacts of disturbances on landscape pattern, structure, and function drive most ecosystem processes and ultimately set the bounds of management for most landscapes of the world (Keane et al., 2009). Ecosystem disturbance regimes describe the temporal and spatial characteristics of a disturbance agent; specifically, the cumulative effects of multiple disturbance events over space and time. Descriptions of disturbance regimes must encompass an area that is large enough so that the full range of disturbance sizes are represented, and long enough so that the full range of disturbance characteristics are captured (Keane et al., 2009). Anthropogenic disturbances, climate change, and management actions interact with ecosystem disturbance regimes and are essential considerations when quantifying and describing disturbance effects on general, ecological, and spatial resilience.
Changes in patterns, processes, and recovery rates under altered disturbance regimes can be evaluated to gain insights into ecological and spatial resilience, with characteristic ecosystem processes and higher recovery rates of those processes typically serving as indicators of higher adaptive capacity and resilience (Chambers et al., 2014a; Seidl et al., 2016). Measurable, well-defined indicators and methodologies are required to evaluate the effects of changes in ecosystem disturbance regimes and the interacting effects of anthropogenic disturbance (Angeler and Allen, 2016). Quantifying changes in disturbance and effects on pattern and processes requires a temporal dimension that can be obtained through long-term monitoring and datasets. In most cases, process-based approaches will be most useful for monitoring and assessing changes in ecological and spatial resilience over time (e.g., Lam et al., 2017).
The historical range of variation (HRV) has been used to assess ecological status and change by assuming recent historical variation represents the broad envelope of conditions (basin of attraction) that supports the self-organizing capacity of landscapes and thus resilience (Hessburg et al., 1999; Keane et al., 2009; Seidl et al., 2016). The historical range of variation (HRV) is based on the idea that the broad historical envelope of possible ecosystem conditions, such as disturbed area, vegetation cover type area, or patch size distribution, can provide a representative time series of reference conditions to guide land management (reviewed in Keane et al., 2009). The HRV is typically based on longer-term geospatial data from remote sensing, field data, and historical reconstructions. The available empirical data can be used to parameterize landscape simulation models, which then simulate ecological processes and extrapolate parameter values across entire regions (Keane, 2012).
The HRV has been used by managers to define ecological benchmarks for determining status, trend, and magnitude of change, and develop objectives and strategies for conservation and restoration management. Application of HRV concepts include prioritizing and selecting areas for possible restoration treatments (Reynolds and Hessburg, 2005; Hessburg et al., 2007, 2013) and identifying areas for conservation of biological diversity (Aplet and Keeton, 1999). Applying the HRV concept has been challenging because the scales of climate, vegetation, and disturbance interactions are inherently different across landscapes, field data in adequate abundance and appropriately scaled are seldom available to define HRV characteristics at many scales, and few statistical techniques exist to compare HRV time series data to current landscape composition and structure (Keane et al., 2009). Recent criticisms of HRV concepts are that historical conditions do not serve as a proxy for ecological resilience in this era of global change (Millar, 2014) and large-scale inferences for entire regions or ecosystems, such as for historical fire regimes, often entail substantial uncertainty and can yield equivocal results (Freeman et al., 2017).
Recently, spatially explicit models have been used to inform management planning processes and help define ecological benchmarks for determining status, trend, and magnitude of change, typically in a risk assessment framework. The interacting effects of ecosystem and anthropogenic disturbances on landscapes and species have been modeled for a wide-variety of landscapes and disturbance types to inform land use plans (e.g., Cushman et al., 2017; McGarigal et al., 2018; Ricca et al., 2018). Longer-term trends in climate have been used to forecast future variations of landscape patterns and processes using highly complex spatial empirical and mechanistic models to increase understanding of disturbance interactions (Loehman et al., 2017) and inform selection of indicators of ecological and spatial resilience (Bradford et al., in press). Like HRV simulation models, these models also entail uncertainties that must be recognized when developing management objectives and monitoring protocols and adapting management to changing conditions.
A primary role of landscape modeling is to clarify and illustrate patterns of risk over time. For example, Cushman et al. (2017) modeled the spatial pattern of risk of forest loss between 2010 and 2020 across Borneo as a function of topographical variables and landscape structure. They found that a random forest modeling framework, which uses landscape metrics as predictors at multiple scales, can be a powerful approach to landscape change modeling. Risk of forest loss differed among Borneo's three nations as a function of distance from the edge of the previous frontier of forest loss and the structure of the landscape, but in general very high rates of forest loss were predicted across the full extent of Borneo. Maps produced for the project showed clear spatial patterns of risk related to topography and landscape structure.
Landscape modeling and geospatial data can be used to evaluate the effects of landscape fragmentation and identify thresholds of change beyond which species population abundance declines. Resilience-based land use plans can be informed by data on hypothesized or observed thresholds, including in disturbance characteristics, population abundance, and landscape connectivity, and the likely impact of crossing those thresholds. Information on time lags and regional variation further informs these plans. For example, rapid expansion of energy development in some portions of the Intermountain West, USA, has prompted concern regarding impacts to declining Greater sage-grouse (Centrocercus urophasianus) populations. Potential thresholds in the relationships among lek attendance by male greater sage-grouse, the presence of oil or gas wells near leks (surface occupancy), and landscape-level density of well pads were developed using generalized linear models and generalized estimating equations (Harju et al., 2010). Surface occupancy of oil or gas wells adjacent to leks was negatively associated with male lek attendance, but time-lag effects suggested that there is a delay of 2–10 years between activity associated with energy development and its measurable effects on lek attendance.
Understanding Relationships Among General, Ecological, and Spatial Resilience and Capacity to Support Habitats and Species
Species spatial distributions and relative abundances are closely related to general, ecological, and spatial resilience. General and ecological resilience are related to climatic factors that determine species distributions, i.e., the bioclimatic range, and ecosystem attributes and processes that determine habitat suitability, such as availability of food, nutrients, and water. Spatial resilience is related to pattern and process interactions that affect gene flow, dispersal, and migration. Disturbance influences resilience through effects on the bioclimatic envelop and resource availability, such as extreme events like droughts or heat-waves and spatial resilience through factors that affect local movements, dispersal, and migration, such as development and transportation and energy corridors. Threshold crossings of both ecological and spatial resilience are indicated by decreases in species occurrence, abundance, and use or non-use of habitat.
The spatial scales and types of data used to evaluate the interrelationships of general and spatial resilience with capacity to support biological diversity depend on the management objectives and the focal landscape. Larger-scale conservation planning efforts ideally include a wide-variety of species to represent the diversity of species traits and habitat requirements within the focal landscape (e.g., Fajardo et al., 2014). In practice, indicator species or other surrogates are often used to monitor environmental changes, assess the efficacy of management, and provide warning signals for impending ecological shifts (see reviews in Jørgensen et al., 2013; Siddig et al., 2016). This approach is not without criticism and there should be strong justification for the species selected as indicators (Dale and Beyeler, 2001; Carignan and Villard, 2002; Cushman et al., 2010; Siddig et al., 2016). Considering the causes and effects of changes in populations beyond the predominant disturbances may improve change detection and thus management recommendations (Carignan and Villard, 2002). Including different taxa with varying affinities to the ecosystems within the system, spatial requirements, and sensitivities to the predominant disturbances may help identify the causes of change more precisely and limit errors of interpretation. The increasing availability of data, statistical tools, and comprehensive models relating species to resilience supports multi-species approaches (Sundstrom et al., 2018).
Ecological and spatial resilience have direct application to conservation management of threatened and endangered species. Spatially explicit information on a system's ecological and spatial resilience, predominant disturbances, and locations and abundances of focal resources and species provides information for evaluating the likely success of different types of management strategies. An understanding of the ecological resilience of the ecosystems that provide habitat for the focal species provides information on the management strategies most likely to succeed (e.g., Chambers et al., 2017a,c). Linking landscape metrics, such as patch size, shape, and connectivity, with landscape occupancy and use of focal species or species distribution models helps further ensure that areas selected for management support populations of the focal species, provide connectivity among populations, and are close enough to breeding centers for recolonization (Guisan and Thuiller, 2005; Doherty et al., 2016; Ricca et al., 2018). New approaches such as spatially explicit, individual-based population, and genetic models (e.g., Landguth and Cushman, 2010) and landscape genetic modeling of population connectivity, density and effective population size as functions of landscape structure (e.g., Balkenhol et al., 2016) provide powerful tools to directly investigate scenarios of altered disturbance regimes and landscape management on biological processes and species populations (e.g., Hearn et al., 2018; Macdonald et al., 2018; Thatte et al., 2018; Kaszta et al., 2019).
A Resilience-based Approach for Prioritizing Areas for Management and Selecting Appropriate Strategies
A strategic, multi-scale approach to management can be used to address the rapid changes occurring in global ecosystems. Knowledge of general and spatial resilience to disturbance coupled with information on key resources, habitats, or species and the predominant disturbances can be used to facilitate regional planning. Use of a spatially explicit approach can enable managers to quantify and visualize differences in resilience in relation to focal resources and disturbances, and then to both prioritize areas for management actions and determine the most effective strategies. Assessments conducted at meso to broad scales can be used to inform ecoregional to biome prioritization of management actions across large landscapes and to allocate budgets and manpower in a manner designed to maximize attainment of conservation and restoration objectives. Knowledge of ecological and spatial resilience at patch to meso scales based on literature review, and local data and expertise, can be used to select project areas and determine appropriate management strategies within areas prioritized for management (Chambers et al., 2017a,c; Crist et al., 2019). We illustrate this type of multi-scale, resilience-based framework for addressing ecosystem and anthropogenic disturbances with a case study from a highly imperiled area of the western U.S. —the sagebrush biome.
Application of the Resilience-Based Framework in the Sagebrush Biome
The sagebrush biome spans ~100 million hectares in western North America. Sagebrush ecosystems occur across broad environmental gradients and provide a large diversity of habitats that support more than 350 species of vertebrates (Suring et al., 2005). These ecosystems currently make up only about 59 percent of their historical area. The primary patterns, processes, and components of many sagebrush ecosystems have been significantly altered since Euro-American settlement in the mid-1800s (Knick et al., 2011; Miller et al., 2011). The predominant disturbances in sagebrush ecosystems are large-scale wildfire, invasion of exotic annual grasses, conifer expansion, energy development, conversion to cropland, and urban and exurban development (Davies et al., 2011; Knick et al., 2011; FWS, 2013; Coates et al., 2016). The continued loss and fragmentation of sagebrush habitats has placed many species at risk, including Greater sage-grouse (Centrocercus urophasianus; hereafter, GRSG), which was considered for listing under the U.S. Endangered Species Act in 2010 and 2015 (FWS, 2010; USDI FWS, 2015) and whose status will be reevaluated in 2020 (USDI FWS, 2015).
GRSG are a broadly distributed species that occupy a variety of environments containing sagebrush. They have been managed as umbrella species for the many other species of plants and animals that depend on sagebrush ecosystems (Suring et al., 2005; Knick et al., 2013). Listing of GRSG as an endangered species would place numerous restrictions on land uses (e.g., livestock grazing, energy exploration, and development) in those sagebrush ecosystems that provide habitat for the species and thus has widespread political and management ramifications. A high percentage of the land within the sagebrush biome is managed by state and federal agencies (ranging from 85% in the State of Nevada to 29% in the State of Montana) placing increased pressure on these agencies to develop effective conservation and restoration approaches.
A collaborative, interagency working group has developed a strategic, multi-scale framework based on resilience science to address the continued loss of sagebrush habitat and declines in GRSG populations (Chambers et al., 2014c, 2016a, 2017a,b; Crist et al., 2019). The resilience-based framework provides the geospatial data, analytical approaches, and decision support tools for prioritizing areas for management and determining effective strategies across the sagebrush biome (Figure 2). The framework is founded on understanding (1) general resilience, as indicated by environmental characteristics and ecosystem attributes and processes, (2) spatial resilience, based on landscape composition and configuration, and thus capacity to support high value resources, and (3) interactions of general and spatial resilience with the predominant disturbances. In-depth knowledge of the multi-scale patterns and process within sagebrush landscapes that determine resilience has been key to developing the framework (Box 1). Use of this multi-scale, resilience-based framework to address invasive grass-fire cycles in arid and semiarid shrublands and woodlands is illustrated in a companion paper in this journal (Chambers et al., 2019).
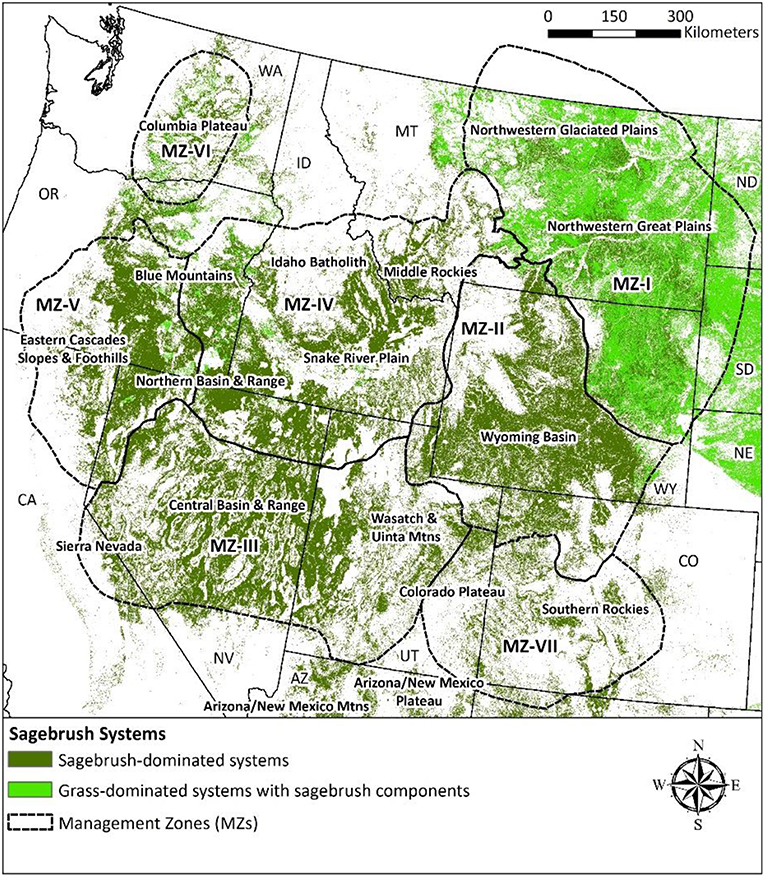
Figure 2. A map of the landscape cover of sagebrush-dominated ecological systems and grass-dominated ecological systems with sagebrush components in the sagebrush biome (Chambers et al., 2017a). The landscape cover of sagebrush (USGS, 2016) is overlaid on Level III Ecoregions (USEPA, 2017) and sage-grouse Management Zones (Stiver et al., 2006).
Box 1. The factors that influence the ecological (ER), general (GR), and spatial resilience (SR) of Cold Desert ecosystems and landscapes to wildfires and non-native grass invasions at patch and patch neighborhood, meso, and broad scales (based on Chambers et al., 2019). In these ecosystems ecological and general resilience to wildfires and resistance to non-native grass invasions varies over environmental gradients as a function of soil temperature and moisture regimes, productivity, historic fire regimes, and species adaptations to fire, as well as resistance to invasive annual grasses. Spatial resilience differs as a result of relative abundance of the dominant life forms (composition) and their spatial relationships (configuration). The patterns and processes that influence ecological and spatial resilience are linked, but are unique to each scale. The descriptions here represent endpoints of conditions across large landscapes.
Patch and patch neighborhood scale. Shrub size, composition, and abundance of perennial herbaceous species, and gap sizes among shrubs and herbaceous species influence resource availability, competitive interactions, and invasion of flammable invasive annual grasses, which in turn influence wildfire dynamics.
Low ER–Soils are warm and dry and productivity is relatively low. Low fuel biomass resulted in few historic fires, and plant species have few adaptations to fire. High climate suitability to invasive annual grasses (IAG) results in low resistance to their invasion. Recovery potentials depend on abundance of perennial native herbaceous species (PNH) that survive fires and resource availability for invaders.
➢ SR–In patches with high SR, PNH are relatively abundant. Small gap sizes among PNH result in strong competition and low abundance of IAG. Large gap sizes among shrubs decrease fire severity and abundant PNH result in site recovery after fire. Patches with low SR have the opposite conditions.
High ER–Soils are cool to cold and moist and productivity is high. High fuel biomass and historically short fire return intervals resulted in fire-adapted plant species. Low climate suitability to IAG results in high resistance to their invasion. Recovery potentials are typically high.
➢ SR–In patches with high SR, PNH are relatively abundant with small gap sizes among PNH. Large gap sizes among shrubs decrease fire severity and abundant, fire-tolerant shrubs, and PNH result in site recovery after fire. Low SR has the opposite conditions, but recovery potential is still moderately high.
Meso scale. Wildfire and invasion patterns, amount of fine fuel, fuel conditions, and fire weather influence fire event sizes and fire severity patch size distributions, which themselves create opportunities for restoration as well as invasion and affect future disturbances.
Low GR–Ecosystems are fuel-limited and had low fire return intervals historically. Invasion of IAG into shrublands increases fine fuels and flammability. Fine fuel availability and fire probability depends on antecedent precipitation. Improper livestock grazing increases woody fuels and fire severity. Large fire size is linked to extreme fire weather.
➢ SR–Areas with high SR have relatively high landscape cover of PNH, relatively high shrub cover, low cover of IAG, and low burned area. Areas with low SR have relatively low cover of PNH, high landscape cover of IAG, and high burned area.
High GR–Ecosystems are flammability or drought limited and had higher fire return intervals historically. Warmer and drier conditions decrease fuel moisture sufficiently for large wildfires to burn. Improper livestock grazing increases woody fuels and fire severity. Large fire size is linked to extreme fire weather.
➢ SR–Areas with high SR have relatively high landscape cover of PNH, relatively high shrub cover, a mosaic of small burned areas, and high connectivity. Areas with low SR have reduced cover of PNH and shrubs, extensive burned areas, and low connectivity. SR can be reduced by anthropogenic disturbances, such as oil and gas drilling, agriculture, and urban development, regardless of general resilience.
Broad ecoregional scale. Patterns of biophysical conditions influence broad scale invasion patterns and the fire delivery system, and determine fire size and severity and expansion of the invader.
GR–The climatic regime (relative aridity and seasonality of precipitation) influences the relative proportion of woody vs. perennial herbaceous species, fire seasonality and burned areas, and climactic suitability for IAG. Landscape heterogeneity and environmental conditions determine the proportions of ecosystems with low, moderate, and high ER. Landscapes with a higher proportion of low ER ecosystems, lower resistance to IAG, and higher fire risk have lower ER. High ER landscapes have the opposite conditions.
➢ SR–Landscapes with high SR are characterized by relatively low aridity and summer-dominated precipitation. Landscape cover of PNH and shrubs is relatively high, cover of IAG is low, and burned areas are within the historic range of variability. Landscapes with low SR are characterized by relatively high aridity and winter-dominated precipitation along with reduced landscape cover of PNH and shrubs, and higher landscape cover of IAG, and higher burned areas. SR can be reduced by anthropogenic disturbances, such as oil and gas drilling, agriculture, and urban development, regardless of general resilience.
The multi-scale, resilience-based framework described herein has been used by the U.S. Forest Service to develop fire risk assessments for all Forest Service lands that support GRSG and for the Intermountain Region. The concepts and approaches in the framework were incorporated into the “Department of the Interior's Integrated Rangeland Fire Management Strategy” (USDI, 2015) and have been used by the Bureau of Land Management to develop a multiyear program of work for Bureau of Land Management managed lands in the western part of the sagebrush biome.
Key Components
1. Management Objectives
Objectives for addressing loss of sagebrush habitat and declines in GRSG populations provide the basis for managing ecosystems to increase their capacity to reorganize and adjust to ongoing change while providing necessary ecosystem services. Overarching management objectives focus on both sagebrush ecosystems and GRSG populations and include:
• Increasing or maintaining resilience to disturbance and resistance to invasive annual grasses in sagebrush ecosystems across the biome.
• Ensuring the long-term conservation of GRSG by maintaining viable, well-distributed GRSG populations that are connected by healthy sagebrush ecosystems across their range.
A collaborative approach that includes federal and state agencies and other partners, such as non-governmental organizations, tribes, and private land owners, is used to develop management objectives for specific planning areas. Long-term monitoring is being implemented within the land management agencies to provide the capacity to adapt management over time and help ensure long-term success. For example, status and trend is monitored through the Bureau of Land Management's Assessment Inventory and Monitoring; Natural Resources Conservation Service's National Resources Inventory, both of which use common indicators and protocols.
2. Landscape Indicators of General Resilience and Resistance to Invasive Grasses
In sagebrush ecosystems, soil temperature and moisture regimes closely reflect climate and vegetation patterns and provide one of the most complete data sets for understanding and visualizing general resilience to disturbance and resistance to invasive annual grasses across the sagebrush biome (see reviews in Brooks et al., 2016; Chambers et al., 2016b, 2019; also Ricca et al., 2018; Bradford et al., in press). They have been mapped for most of the sagebrush biome and are available through the USDA Natural Resources Conservation Service, Web Soil Survey (https://websoilsurvey.nrcs.usda.gov). The dominant vegetation (ecological) types differ across the sagebrush biome and have been characterized according to soil temperature and moisture regimes, general resilience to disturbance, and resistance to invasive annual grasses (Chambers et al., 2017a) based on recent research (Chambers et al., 2007, 2014b, 2017b; Condon et al., 2011; Davies et al., 2012; Urza et al., 2017) and expert input. State-and-transition models, which provide information on the alternative states, ranges of variability within states, and processes that cause plant community shifts within states as well as transitions among states, have been developed for the dominant vegetation types (Chambers et al., 2017a). To facilitate landscape analyses and prioritization, soil temperature, and moisture regime subclasses have been used to categorize relative resilience to disturbance and resistance to invasive annual grasses as high, moderate, or low across the sagebrush biome (Figure 3; Maestas et al., 2016; Chambers et al., 2017a). Higher resolution categories can be developed for assessments conducted at ecoregional or sage-grouse Management Zone scales and detailed soils data are available for project area assessments.
3. Spatial Resilience and GRSG Populations
The breeding habitat model for GRSG (Doherty et al., 2016) provides one the best sources of information for understanding and visualizing spatial resilience in the context of GRSG populations. The breeding habitat model uses GRSG lek data (2010–2014) as a proxy for landscapes important to breeding birds. Leks are central to the breeding ecology of GRSG and the majority of nests occur relatively close to leks (within 6.3 km; Holloran and Anderson, 2005; Coates et al., 2013). The breeding habitat model evaluates the vegetation (i.e., landscape cover), climate, and landform characteristics as well as the type and amount disturbance around leks (within a radius of 6.4 km; Doherty et al., 2016), and provides an estimate of the probability of occurrence of breeding sage-grouse at a spatial resolution of 120 × 120 m. Model output is specific to the habitat characteristics of each sage-grouse Management Zone.
Breeding habitat probabilities for GRSG in Doherty et al. (2016) were used to develop three categories of breeding habitat probability for prioritizing management actions across large landscapes (Figure 4; Chambers et al., 2016a, 2017a). The categories were based on the probability of areas near leks (within a radius of 6.4 km) providing suitable breeding habitat and included: low (0.25 to <0.50); moderate (0.50 to <0.75); and high (0.75 to 1.00). Areas with probabilities of 0.01 to <0.25 were considered to be unsuitable for breeding habitat. Intersecting the resilience and resistance index with the breeding habitat probabilities for GRSG provides information on sage-grouse habitat availability and connectivity, potential for recovery following wildfire, and spatial constraints on recovery (Figure 5).
4. Interactions of General and Spatial Resilience With the Predominant Disturbances
The predominant disturbances differ across the sagebrush biome (Chambers et al., 2017a). Invasion of exotic annual grasses and development of grass-fire cycles is a large-scale disturbance in the western part of the biome that is an emerging threat in the eastern part of the biome (Chambers et al., 2019). A large-fire risk assessment for the United States has been developed from modeled burn probabilities and fire size distributions based on weather data, spatial data on fuel structure and topography, historical fire data, and fire suppression effects (Finney et al., 2011), which was recently updated (Short et al., 2016; Figure 6). Intersecting the resilience and resistance index, GRSG breeding habitat probabilities, and large fire risk provides spatially explicit information not only on the likelihood of large fires, but also on likely responses to those fires and effects on high value habitat (Figure 7). These maps can be scaled down to local field offices or project areas to facilitate planning designed to locate management strategies where they will be most effective.
5. Management Prioritization and Strategies
The resilience-based framework couples the geospatial data and maps with a sage-grouse habitat resilience and resistance matrix in order to facilitate prioritizing areas for management actions and selecting appropriate strategies (Table 5). The matrix is a decision-support tool that allows managers to consider how general resilience may be affecting recovery potential along with how the landscape context and spatial resilience may be influencing capacity to support GRSG populations. The different cells of the matrix are mapped in Figure 5. In the matrix and the map, as resilience to disturbance and resistance to invasive annual grasses go from low to high (indicated by the lower to upper rows), the recovery potential increases due to less change from the initial or desired state and a faster rate of recovery. As the probability of sage-grouse habitat goes from low to high within these same systems (indicated by the columns), the capacity to support high value habitat and resources increases as a function of the size and shape of habitat patches and their connectivity.
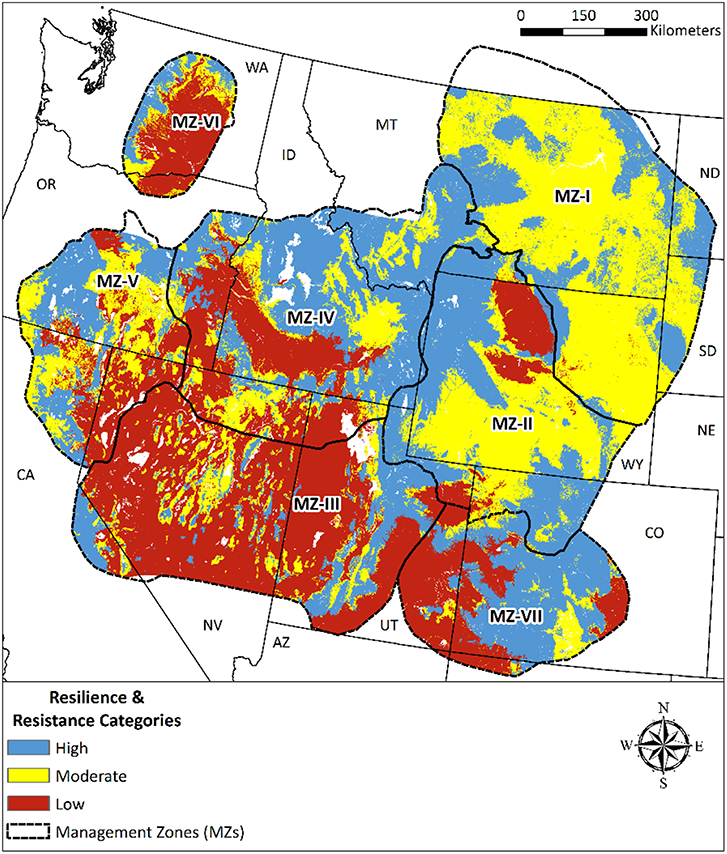
Figure 3. The soil temperature and moisture regime subclasses categorized according to high, moderate, and low resilience to disturbance and resistance to invasive annual grasses (Maestas et al., 2016; Chambers et al., 2017a) overlaid on the sage-grouse Management Zones (Stiver et al., 2006). The soil temperature and moisture regime data are from the USDA Natural Resources Conservation Service, Web Soil Survey (https://websoilsurvey.nrcs.usda.gov).
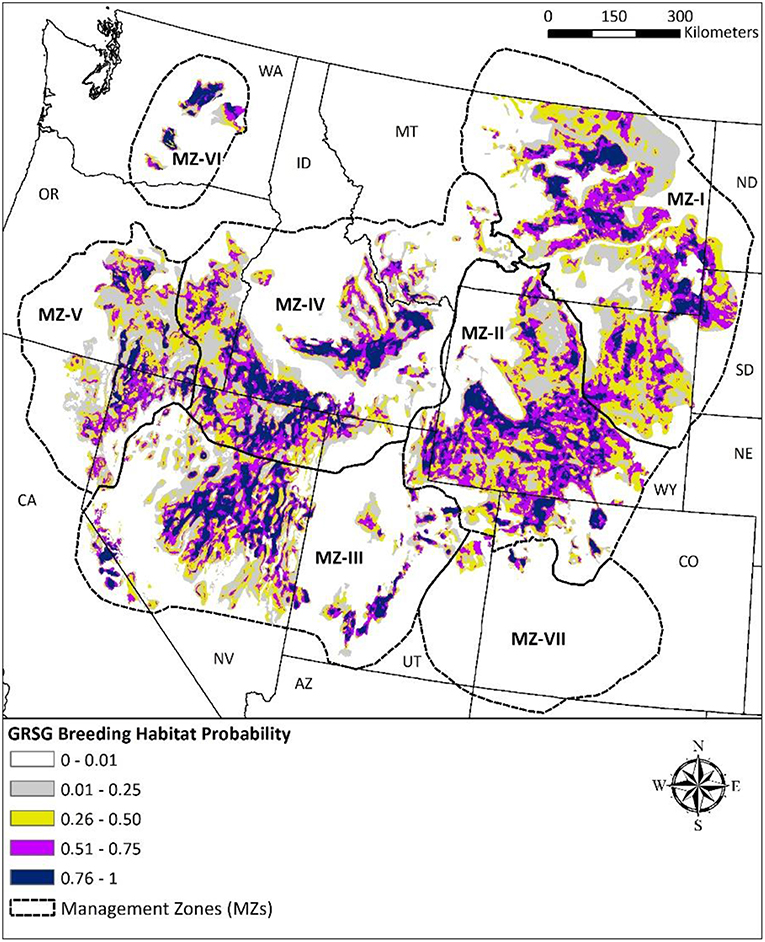
Figure 4. Modeled Greater sage-grouse breeding habitat probabilities based on landscape cover of vegetation, climate, and landform characteristics as well as the type and amount disturbance around breeding sites or leks (within a radius of 6.4 km; Doherty et al., 2016; Chambers et al., 2017a). Sage-grouse Management Zones (Stiver et al., 2006) and Priority Areas for Conservation (USDI FWS, 2015) developed by the States are overlaid.
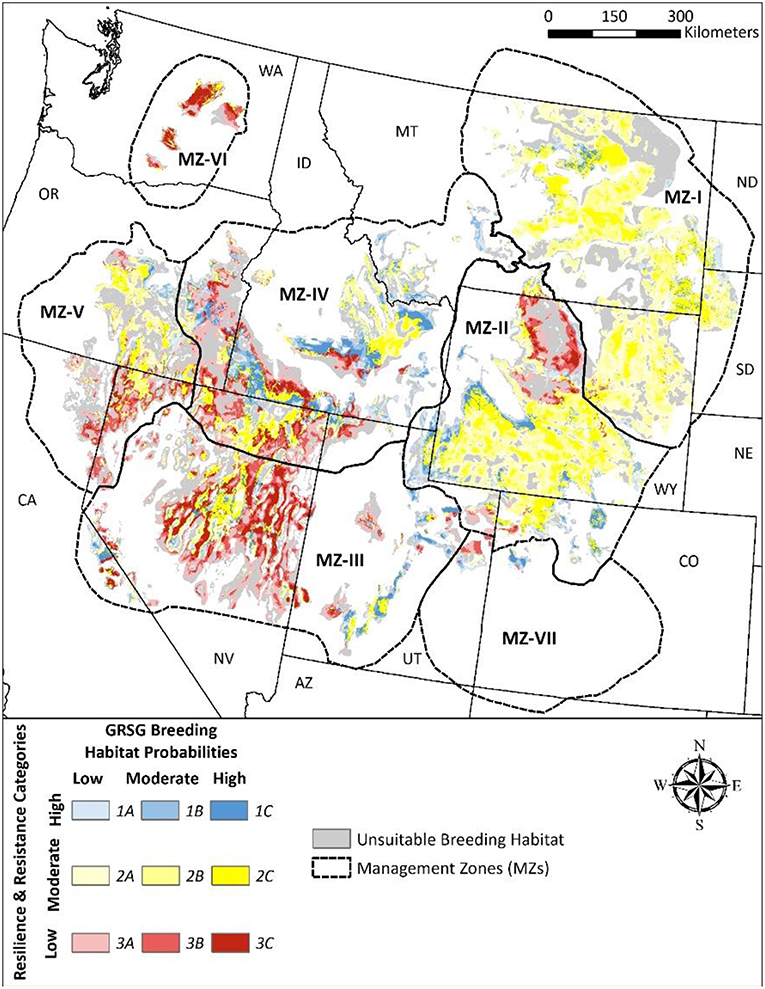
Figure 5. Greater sage-grouse breeding habitat probabilities (Doherty et al., 2016) intersected with resilience and resistance categories developed from soil temperature and moisture regime subclasses (Chambers et al., 2017a). Sage-grouse Management Zones (Stiver et al., 2006) and Priority Areas for Conservation (USDI FWS, 2015) developed by the States are overlaid. This map provides a spatial depiction of the sage-grouse habitat resilience and resistance matrix.
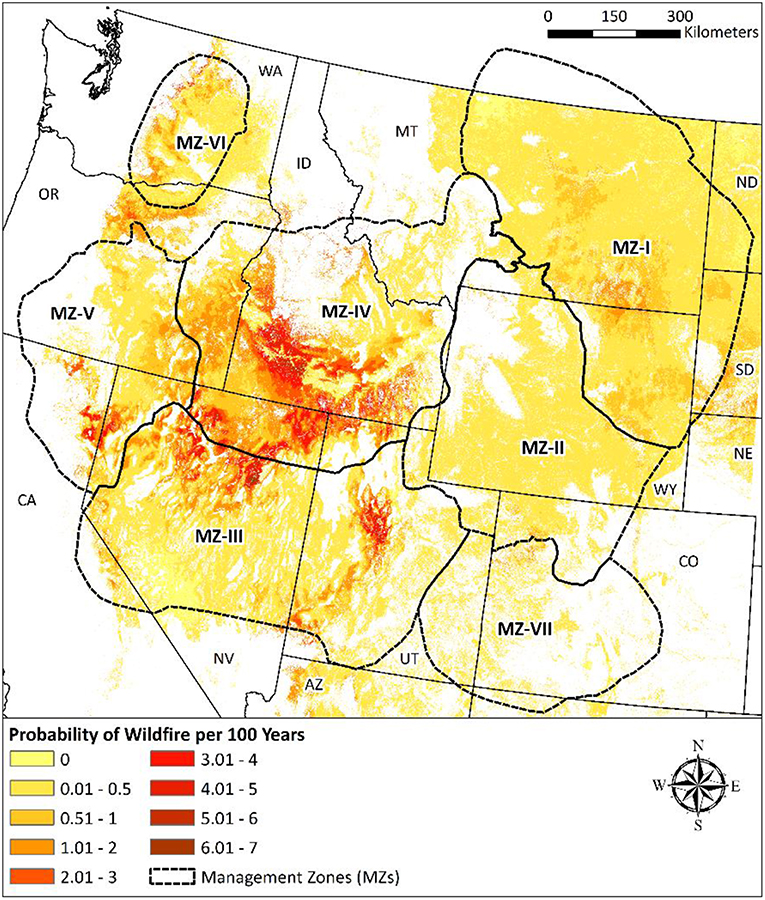
Figure 6. Large fire probability derived by simulating fire ignition and growth using the Fire Simulation (FSim) system (Finney et al., 2011; Short et al., 2016; Chambers et al., 2017a). Sage-grouse Management Zones (Stiver et al., 2006) are overlaid.
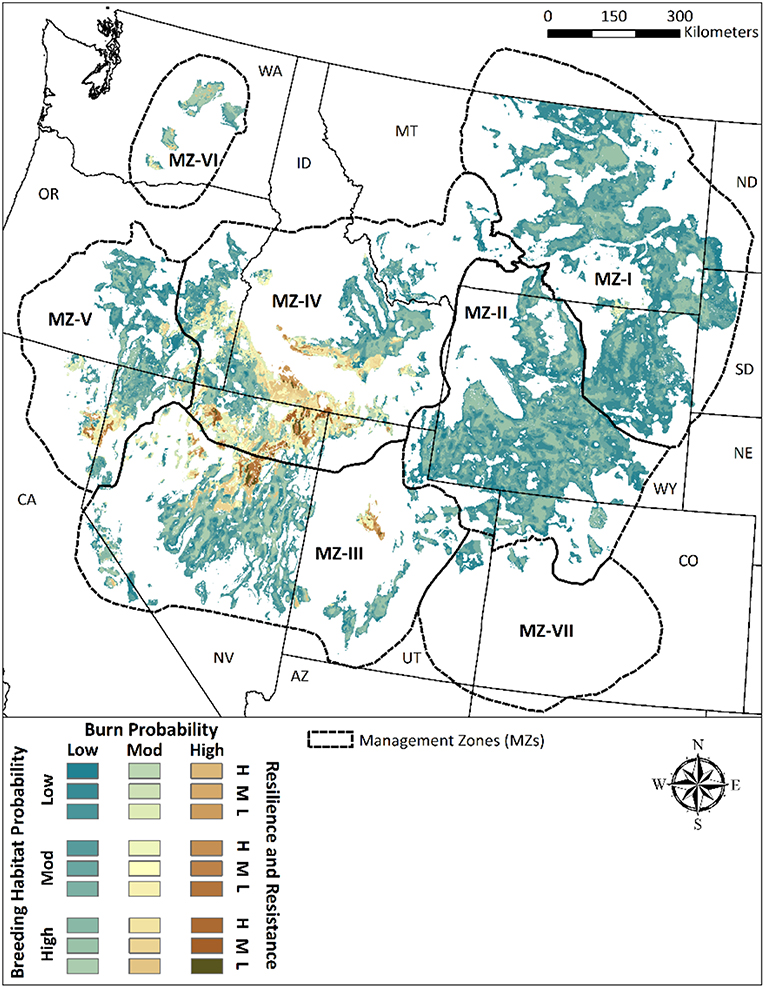
Figure 7. Fire risk map depicting different combinations of sage-grouse breeding habitat probability (Doherty et al., 2016), resilience to disturbance and resistance to invasive annual grasses (Chambers et al., 2017a), and large fire probability (Short et al., 2016). Sage-grouse Management Zones (Stiver et al., 2006) are overlaid. The map identifies sage-grouse habitats that are at highest risk from fire and the relative resilience and resistance of those areas (Chambers et al., 2017a).
The general resilience of an area strongly influences its response to both disturbances and management actions (Chambers et al., 2014a,b, 2017b). Areas with high general resilience often have the capacity to return to the prior or a desired state with minimal intervention (Table 5, 1A, 1B, 1C). Those with moderate general resilience depend on both the environment and ecosystem attributes and often require more detailed assessments to determine effective management strategies (Table 5, 2A, 2B, 2C). Areas with low general resilience are typically among the most difficult to improve and multiple management interventions coupled with preventative measures may be required to obtain a desired state after disturbance (Table 5, 3A, 3B, 3C).
The spatial resilience of an area is influenced by (1) resilience to disturbance and resistance to invasive grasses, which influence recovery potentials and the propensity to change states, and (2) anthropogenic developments, which fragment habitats, result in introductions of novel species, and can preclude return to prior states. An area with high sage-grouse breeding habitat probabilities with intact sagebrush ecosystems and high resilience to disturbance and resistance to invasive grasses (Table 5, 1C) may have relatively higher spatial resilience over time than one with low resilience and resistance. However, an area with low breeding habitat probabilities due to low landscape cover of sagebrush that has high resilience to disturbance and resistance to invasive grasses (Table 5, 1A) may have spatial resilience similar to an area with low resilience to disturbance and resistance to invasive grasses, if anthropogenic development, such as agricultural conversion or oil and gas development, is causing the loss of spatial resilience.
Areas with high sage-grouse breeding habitat probabilities are typically comprised of relatively intact habitat and resource patches, have high spatial resilience, and are high priorities for protective management (Table 5, 1C, 2C, 3C; Chambers et al., 2014a, 2017a,b). Regardless of the level of general resilience, protective management can be used in and adjacent to these areas to maintain habitat connectivity and ecological resilience. A diverse set of management strategies can be used including reducing or eliminating disturbances from land uses and development, establishing conservation easements, and utilizing early detection and rapid response approaches for invasive species (USDI, 2016). Areas with high sage-grouse breeding habitat but low general resilience are typically slower to recover following fire and surface disturbances and have lower resistance to invasive annual grasses. Consequently, these areas are at greater risk of habitat loss than areas with moderate to high resilience and resistance and are high priorities for protective management (Table 5, 3C, Figure 7; Chambers et al., 2014a; Chambers et al., 2017b).
Areas with moderate sage-grouse breeding habitat probabilities and thus spatial resilience often supported a higher proportion of leks in the past and have the capacity for improvement through restoration and other management strategies, particularly if anthropogenic developments are not causing the loss of resilience (Table 5, 1B, 2B, 3B; Knick et al., 2013; Chambers et al., 2014c, 2017b). Management strategies aim to improve resilience of known habitat or resource patches through activities like vegetation manipulation, invasive plant control, or habitat restoration. Habitat restoration can involve passive management, such as changes in levels of human uses like livestock grazing to improve ecological conditions. It can also involve active management such as controlling invading plant species to prevent development of invasive-grass fire cycles, and removing encroaching conifers or seeding or transplanting desirable plant species like sagebrush to increase connectivity. Management strategies may also aim to reduce the risk of altered disturbance regimes, such as wildfires outside of the historical range of variation (Figure 7).
Areas with low sage-grouse breeding habitat probabilities are characterized by habitat that may have supported active GRSG leks in the past, but that currently support few leks (Table 5, 1A, 1B, 1C). Spatial resilience and thus capacity to support desired resources and habitats has typically been reduced. If land use and development activities such as cropland conversion, energy and mineral development, and urban development are causing the decrease in spatial resilience, then improvement may not be feasible. However, if the area has the capacity to respond to management treatments and has the necessary connectivity to support species populations and allow recolonization, then improvement may still be possible (e.g., Doherty et al., 2016; Ricca et al., 2018). Although managers may decide to invest in improving these types of areas, the degree of difficulty and time frame required usually increases as general resilience decreases and these investments may not be ecologically or cost effective (Calmon et al., 2011); (Chambers et al., 2017a).
In those areas where climate change effects are projected to be severe, management actions may be needed that help ecosystems transition to new regimes (e.g., Millar et al., 2007; Halofsky et al., 2018a,b; Snyder et al., 2018). An understanding of the ecological memory of ecosystems and the role of species functional traits in conveying ecological resilience can be used in a management context to increase adaptive capacity. For example, selecting plant species for restoration with functional traits that allow them to persist in the face of novel disturbances and a warming environment may increase ecological and spatial resilience (Laughlin et al., 2017). In some areas, such as those converted to invasive annual grasses or at risk of conversion, it may be necessary to erode the resilience of a system and help it transition to a more desired alternative regime, or transition to an alternative state may be inevitable given other change (e.g., climate).
Caution is needed to avoid “coerced” resilience or the replacement of natural processes and feedbacks with external anthropogenic inputs. For example, much of the southern Great Plains in the United States has undergone a regime shift from grassland to juniper woodland; many protected grassland areas exist within this now woodland system that are still present due to intensive human intervention. Coerced resilience prevents an alternative state from emerging. If intensive management is stopped, the system may immediately flip to the state of the surrounding landscape (Twidwell et al., 2019), usually because the surrounding landscape has already entered an alternative state. This is a management and philosophical dilemma, as managers are left with three unsatisfactory choices: to maintain such protected areas through perpetual intensive intervention; to try and reverse the broader scale regime shift that occurred; or to let the protected area undergo the regime shift.
Careful assessment of the focal area will always be necessary to determine the relevance of a particular strategy or treatment because ecosystems occur over continuums of environmental conditions, such as effective precipitation, have differing land use histories and species compositions (Johnstone et al., 2016), and may be projected to experience different climate change effects. Using the best available information on the focal ecosystems and species and their responses to management actions can help ensure that treatments are located and strategies implemented in a manner that will meet conservation and restoration objectives. Using structured decision making in the context of adaptive management can help ensure that stakeholders are involved throughout the process.
Conclusion
Operationalizing the concepts of general, ecological, and spatial resilience provides the ability to address the effects of ecosystem and anthropogenic disturbances at scales relevant to managers. Evaluating the general and ecological resilience of the ecosystems that comprise landscapes requires developing an understanding of the relationships among the environmental characteristics, ecosystem attributes and processes, and responses to ecosystem and anthropogenic disturbances over time. Evaluating the spatial resilience of landscapes requires understanding the effects of changes in the composition and configuration of landscapes due to ecosystem and anthropogenic disturbances. Integration of resilience concepts in the context of landscapes provides the basis for knowing how ecosystem attributes and processes interact with landscape structure to influence the responses of ecosystems to disturbances and stressors and their capacity to support resources, habitats, and species over time.
Resilience-based management uses a spatially explicit approach, which provides the ability to both quantify and visualize the differences in resilience in relation to focal resources and species and the predominant disturbances. It provides the capacity to determine locations on the landscape where conservation and restoration activities are most likely to be effective and to select the types of management actions that are most likely to succeed. Use of an adaptive management process that uses routine monitoring to adjust management actions in response to changing conditions is a requisite. Effective collaboration among managers, scientists, and stakeholders helps ensure that resilience-based approaches to management are developed that will be applied to conserve and restore ecosystems. Resilience-based management may require new laws, policies, guidelines, or funding structures in the Anthropocene. It will be most effective when scientific information is used to build consensus in collaborative venues.
Author Contributions
JC, CA, and SC conceived the idea and developed the manuscript outline. JC led the writing of the manuscript. CA and SC contributed critically to the draft. All authors gave final approval for publication.
Funding
This work was supported by USDA Forest Service, Rocky Mountain Research Station, and U.S. Geological Survey, Nebraska Cooperative Fish and Wildlife Research Unit.
Conflict of Interest Statement
The authors declare that the research was conducted in the absence of any commercial or financial relationships that could be construed as a potential conflict of interest.
The reviewer, MT, declared a shared affiliation, with no collaboration, with several of the authors, JC, SC, to the handling editor at time of review.
Acknowledgments
We thank Paul F. Hessburg, Peter J. Weisberg, Alexandra Urza, Brendan Alexander Harmon, and Matthew Thompson for insightful and helpful reviews of this manuscript. Jacob D. Hennig executed the figures for the case study.
References
Alistair, S. G., Pech, R. P., and Byrom, A. E. (2013). Connectivity and invasive species management: towards an integrated landscape approach. Biol. Invas. 15, 2127–2138. doi: 10.1007/s10530-013-0439-6
Allen, C. R., Angeler, D. G., Cumming, G. S., Folk, C., Twidwell, D., and Uden, D. R. (2016). Quantifying spatial resilience. J. Appl. Ecol. 53, 625–635. doi: 10.1111/1365-2664.12634
Allen, C. R., Birge, H. E., Angeler, D. G., Arnold, C. A. T., Chaffin, B. C., DeCaro, D. A., et al. (2018). Quantifying uncertainty and trade-offs in resilience assessments. Ecol Soc. 23:3. doi: 10.5751/ES-09920-230103
Allen, C. R., Fontaine, J. J., Pope, K. L., and Gamestani, A. J. (2011). Adaptive management for a turbulent future. J. Environ. Manag. 92, 1339–1345. doi: 10.1016/j.jenvman.2010.11.019
Allen, C. R., and Holling, C. S. (2008). Discontinuities in Ecosystems and Other Complex Systems. New York, NY: University of Columbia Press.
Angeler, D. G., and Allen, C. R. (2016). Quantifying resilience. J. Appl. Ecol. 53, 617–624. doi: 10.1111/1365-2664.12649
Angeler, D. G., Allen, C. R., Barichievy, C., Eason, T., Garmestani, A. S., Graham, N. A. J., et al. (2016). Management applications of discontinuity theory. J. Appl. Ecol. 53, 688–698. doi: 10.1111/1365-2664.12494
Aplet, G., and Keeton, W. S. (1999). “Application of historical range of variability concepts to biodiversity conservation,” in Practical Approaches to the Conservation of Biological Diversity, eds R. K. Baydack, H. Campa, and J. B. Haufler (New York, NY: Island Press, 71–86.
Archer, S. (1989). Have southern Texas savannas been converted to woodands in recent history? Amer. Nat. 134, 545–561. doi: 10.1086/284996
Aronson, J., Floret, C., LeFloch, E., Ovalle, C., and Pontanier, R. (1993). Restoration and rehabilitation of degraded ecosystems in arid and semi-arid lands. I. a view from the South. Restor Ecol. 1, 168–176. doi: 10.1111/j.1526-100X.1993.tb00023.x
Bagchi, S., Briske, D. D., Wu, X. B., McClaran, M. P., Besterlmeyer, B. T., and Fernández-Giménez, M. (2012). Empirical assessment of state-and-transition models with a long-term vegetation record from the Sonoran Desert. Ecol. Appl. 22, 400–411. doi: 10.1890/11-0704.1
Baho, D. L., Allen, C. R., Garmestani, A. S., Fried-Petersen, H. B., Renes, S. E., Gunderson, L., et al. (2017). A quantitative framework for assessing ecological resilience. Ecol. Soc. 22:17. doi: 10.5751/ES-09427-220317
Balkenhol, N., Cushman, S. A., Storfer, A. T., and Waits, L. P. (2016). Landscape Genetics: Concepts, Methods, Applications. Sussex: John Wiley and Sons.
Bestelmeyer, B. T., Goolsby, D. P., and Archer, S. R. (2011). Spatial perspectives in state-and-transition models: a missing link to land management? J. Appl. Ecol. 48, 746–757. doi: 10.1111/j.1365-2664.2011.01982.x
Bestelmeyer, B. T., Tugel, A. J., Peacock, G. L. J., Robinett, D. G., Shaver, P. L., Brown, J. R., et al. (2009). State-and transition models for heterogeneous landscapes: a strategy for development and application. Rangeland Ecol. Manag. 62, 1–15. doi: 10.2111/08-146
Bino, G., Sisson, S. A, Kingsford, R. T, Thomas, R. F., and Bowen, S. (2015). Developing state and transition models of floodplain vegetation dynamics as a tool for conservation decision-making: a case study of the macquarie marshes ramsar wetland. J. Appl. Ecol. 52, 654–664. doi: 10.1111/1365-2664.12410
Bradford, J. B., Schlaepfer, D. R., Lauenroth, W. K., Palmquist, K. A., Chambers, J. C., and Maestas, J. D. (in press). 21st century changes in soil temperature moisture regimes in North American drylands. Frontiers Ecol. Evol.
Bradshaw, A. D., and Chadwick, M. J. (1980). The Restoration of Land: The Ecology and Reclamation of Derelict and Degraded Land. Berkeley, CA: University of California Press.
Briske, D. D., Bestelmeyer, B. T., Stringham, T. K., and Shaver, P. L. (2008). Recommendations for development of resilience-based state-and-transition models. Rangeland Ecol. Manag. 61, 359–367. doi: 10.2111/07-051.1
Briske, D. D., Fuhlendorf, S. D., and Smeins, F. E. (2005). State-and-transition models, thresholds, rangeland health: a synthesis of ecological concepts and perspectives. Rangeland Ecol Manag. 58, 1–10. doi: 10.2111/1551-5028(2005)58<1:SMTARH>2.0.CO;2
Brooks, M. L., Brown, C. S., Chambers, J. C., D'Antonio, C. M., Keeley, J. E., and Belnap, J. (2016). “Exotic annual bromus invasions: comparisons among species and ecoregions in the Western United States,” in Exotic Brome-grasses in Arid and Semiarid Ecosystems of the Western US: Causes, Consequences, and Management Implications, eds M. J. Germino, J. C. Chambers, and C. S. Brown (New York, NY: Springer: Series on Environmental Management, 11–60.
Brown, E. D., and Williams, B. K. (2015). Resilience and resource management. Env. Manag. 56, 1416–1427. doi: 10.1007/s00267-015-0582-1
Brunsden, D., and Thornes, J. B. (1979). Landscape sensitivity and change. T. I. Brit. Geogr. 4, 463–484. doi: 10.2307/622210
Calmon, M., Brancalion, P. H. S., Paese, A., Aronson, J., Castro, P., Silva, S. C., et al. (2011). Emerging threats and opportunities for largescale ecological restoration in the atlantic forest of Brazil. Restor. Ecol. 19, 154–158. doi: 10.1111/j.1526-100X.2011.00772.x
Carignan, V., and Villard, M. (2002). Selecting indicator species to monitor ecological integrity: a review. Environ. Monit. Assess. 78, 45–61. doi: 10.1023/A:1016136723584
Caudle, D., DiBenedetto, J., Karl, M., Sanchez, H., and Talbot, C. (2013). Interagency Ecological Site Handbook for Rangelands. Available online at: http://jornada.nmsu.edu/sites/jornada.nmsu.edu/files/InteragencyEcolSiteHandbook.pdf
Chambers, J. C., Beck, J. L., Bradford, J. B., Bybee, J., Campbell, S., Carlson, J., et al. (2017a). Science Framework for Conservation and Restoration of the Sagebrush Biome: Linking the Department of the Integrated Rangeland Fire Management Strategy to Long-Term Strategic Conservation Actions. Part One. Science Basis and Applications. RMRS-GTR-360. Fort Collins, CO: U.S Department of Agriculture, Forest Service, Rocky Mountain Research Station.
Chambers, J. C., Beck, J. L., Campbell, S., Carlson, J., Christiansen, T. J., Clause, K. J., et al. (2016a). Using Resilience and Resistance Concepts to Manage Threats to Sagebrush Ecosystems, Gunnison Sage-Grouse, and Greater Sage-Grouse in Their Eastern Range: A Strategic Multi-Scale Approach. Gen. Tech. Rep. RMRS-GTR-356. Fort Collins, CO: U.S. Department of Agriculture, Forest Service, Rocky Mountain Research Station, 143. Available online at: https://www.treesearch.fs.fed.us/pubs/53201
Chambers, J. C., Board, D. I., Roundy, B. A., and Weisberg, P. J. (2017b). Removal of perennial herbaceous species affects response of cold desert shrublands to fire. J. Veg. Science. 28, 975–984. doi: 10.1111/jvs.12548
Chambers, J. C., Bradley, B. A., Brown, C. S., D'Antonio, C., Germino, M. J., Grace, J. B., et al. (2014a). Resilience to stress and disturbance, and resistance to Bromus tectorum L. invasion in the cold desert shrublands of western North America. Ecosystems 7, 360–375. doi: 10.1007/s10021-013-9725-5
Chambers, J. C., Brooks, M. L., Germino, M. J., Maestas, J. D., Board, D. I., Jones, M. W., et al. (2019). Operationalizing resilience and resistance concepts to address invasive grass-fire cycles. Front. Ecol. Evol. 7:185. doi: 10.3389/fevo.2019.00185
Chambers, J. C., Germino, M. J., Belnap, J., Brown, C. S., Schupp, E. W., and St. Clair, S. B. (2016b). “Plant community resistance to invasion by Bromus species—The role of community attributes,” in Exotic Brome-grasses in Arid and Semiarid Ecosystems of the Western US: Causes, Consequences, and Management Implications, eds M. J. Germino, J. C. Chambers, and C. S. Brown (New York, NY: Springer: Series on Environmental Management, 275–306.
Chambers, J. C., Maestas, J. D., Pyke, D. A., Boyd, C., Pellant, M., and Wuenschel, A. (2017c). Using resilience and resistance concepts to manage persistent threats to sagebrush ecosystems and Greater sage-grouse. Rangeland Ecol. Manage. 70, 149–164. doi: 10.1016/j.rama.2016.08.005
Chambers, J. C., Miller, R. F., Board, D. I., Pyke, D. A., Roundy, B. A., Grace, J. B., et al. (2014b). Resilience and resistance of sagebrush ecosystems: implications for state and transition models and management treatments. Rangeland Ecol. Manag. 67, 440–454. doi: 10.2111/REM-D-13-00074.1
Chambers, J. C., Pyke, D. A., Maestas, J., Pellent, M., Boyd, C. S., Campbell, S., et al. (2014c). Using Resistance and Resilience Concepts to Reduce Impacts of Annual Grasses and Altered Fire Regimes on the Sagebrush Ecosystem and Sage-Grouse– A Strategic Multi-Scale Approach. RMRS-GTR-326. (Fort Collins, CO: U.S. Department of Agriculture, Forest Service. Available online at: https://www.treesearch.fs.fed.us/pubs/46329
Chambers, J. C., Roundy, B. A., Blank, R. R., Meyer, S. E., and Whittaker, A. (2007). What makes great basin sagebrush ecosystems invasible by Bromus tectorum? Ecol. Monogr. 77, 117–145. doi: 10.1890/05-1991
Coates, P. S., Casazza, M. L., Blomberg, E. J., Gardner, S. C., Espinosa, S. P., Yee, J. L., et al. (2013). Evaluating greater sage-grouse seasonal space use relative to leks: Implications for surface use designations in sagebrush ecosystems. J. Wildlife Manag. 77, 1598–1609. doi: 10.1002/jwmg.618
Coates, P. S., Ricca, M. A., Prochazka, B. G., Brooks, M. L., Doherty, K. E., Kroger, T., et al. (2016). Wildfire, climate, and invasive grass interactions negatively impact an indicator species by reshaping sagebrush ecosystems. Proc. Natl. Acad. Sci U.S.A. 113, 12745–12750. doi: 10.1073/pnas.1606898113
Condon, L., Weisberg, P. L., and Chambers, J. C. (2011). Abiotic and biotic influences on Bromus tectorum invasion and Artemisia tridentata recovery after fire. Inter. J. Wildland Fire. 20, 1–8. doi: 10.1071/WF09082
Conroy, M. J., Runge, M. C., Nichols, J. D., Stodala, K. W., and Cooper, R. J. (2011). Conservation in the face of climate change: the role of alternative models, monitoring, and adaptation in confronting and reducing uncertainty. Biol. Conserv. 144, 1204–1213. doi: 10.1016/j.biocon.2010.10.019
Crist, M. R, Chambers, J. C., Phillips, S. L., Prentice, K. L., and Wiechman, L. A. eds. (2019). Science Framework for Conservation and Restoration of the Sagebrush Biome: Linking the Department of the Interior's Integrated Rangeland Fire Management Strategy to Long-Term Strategic Conservation Actions. Part 2. Management Applications. RMRS-GTR-389. Fort Collins, CO: U.S Department of Agriculture, Forest Service, Rocky Mountain Research Station.
Cumming, G. S. (2011a). Spatial resilience: integrating landscape ecology, resilience, and sustainability. Landscape Ecol. 26, 899–909. doi: 10.1007/s10980-011-9623-1
Curtin, C. G., and Parker, J. P. (2014). Foundations of resilience thinking. Conserv. Biol. 28, 912–923. doi: 10.1111/cobi.12321
Cushman, S. A., Elliot, N. B., Bauer, D., Kesch, K., Bahaa-el-din, L., Flyman, M., et al. (2018). Prioritizing core areas, corridors and conflict hotspots for lion conservation in southern Africa. PLoS ONE 13:e0196213. doi: 10.1371/journal.pone.0196213
Cushman, S. A., Elliot, N. B., Macdonald, D. W., and Loveridge, A. J. (2016). A multi-scale assessment of population connectivity in African lions in response to landscape change. Landscape Ecol. 31, 1337–1353. doi: 10.1007/s10980-015-0292-3
Cushman, S. A., Gutzwiller, K., Evans, J. S., and McGarigal, K. (2010). “The gradient paradigm: a conceptual and analytical framework for landscape ecology,” in Spatial Complexity, Informatics and Wildlife Conservation, eds S. A. Cushman and F. Huettmann (Tokyo: Springer, 83–108.
Cushman, S. A., and Landguth, E. L. (2012). Multi-taxa population connectivity in the northern Rocky Mountains. Ecol. Model. 231, 101–112. doi: 10.1016/j.ecolmodel.2012.02.011
Cushman, S. A., Lewis, J. S., and Landguth, E. L. (2013). Evaluating the intersection of a regional wildlife connectivity network with highways. Mov. Ecol. 1:12. doi: 10.1186/2051-3933-1-12
Cushman, S. A., Macdonald, E. A., Landguth, E. L, Malhi, Y., and Macdonald, D. W. (2017). Multiple-scale prediction of forest loss risk across Borneo. Landscape Ecol. 32, 1581–1598. doi: 10.1007/s10980-017-0520-0
Cushman, S. A., Mcgarigal, K., and Neel, M. C. (2008). Parsimony in landscape metrics: strength, universality and consistency. Ecol. Indicat. 8, 691–703. doi: 10.1016/j.ecolind.2007.12.002
Dale, V. H., and Beyeler, S. C. (2001). Challenges in the development and use of ecological indicators. Ecol Indic. 1, 3–10. doi: 10.1016/S1470-160X(01)00003-6
Daniel, C. J., Frid, L., Sleeter, B. M., and Fortin, M. J. (2016). State-and-transition simulation models: a framework for forecasting landscape change. Methods Ecol. Evol. 7, 1413–1423. doi: 10.1111/2041-210X.12597
D'Antonio, C. M., and Thomsen, M. (2004). Ecological resistance in theory and practice. Weed Technol. 18, 1572–1577. doi: 10.1614/0890-037X(2004)018[1572:ERITAP]2.0.CO;2
Davies, G. M., Bakker, J. D., Dettweiler-Robinson, E., Dunwiddie, P. W., Hall, S. A., and Downs, J. (2012). Trajectories of change in sagebrush-steppe vegetation communities in relation to multiple wildfires. Ecol. Appl. 22, 1562–1577. doi: 10.1890/1051-0761-22.5.1562
Davies, K. W., Boyd, C. S., Beck, J. L., Bates, J. D., Svejcar, T. J., and Gregg, M. A. (2011). Saving the sagebrush sea: an ecosystem conservation plan for big sagebrush plant communities. Biol. Conserv. 144, 2573–2584. doi: 10.1016/j.biocon.2011.07.016
de Souza Leite, M., Tambosi, L. R., Romitelli, I., and Metzger, J. P. (2013). Landscape ecology perspective in restoration projects for biodiversity conservation: a review. Natureza Conservação. 11, 108–118. doi: 10.4322/natcon.2013.019
Doherty, K. E., Evans, J. S., Coates, P. S., Juliusson, L. M., and Fedy, B. C. (2016). Importance of regional variation in conservation planning: a rangewide example of the greater sage-grouse. Ecosphere 7:e01462. doi: 10.1002/ecs2.1462
Downs, P. W., and Gregory, K. J. (1993). “The sensitivity of river channels in the landscape system,” in Landscape Sensitivity, eds D. S. G. Thomas and R. J. Allison (Chichester: Wiley), 15–30.
Fahrig, L., and Merriam, G. (1994). Conservation of fragmented populations. Conserv. Biol. 8, 50–59. doi: 10.1046/j.1523-1739.1994.08010050.x
Fajardo, J., Lessmann, J., Bonaccorso, E., Devenish, C., and Muñoz, J. (2014). Combined use of systematic conservation planning, species distribution modelling, and connectivity analysis reveals severe conservation gaps in a megadiverse country (Peru). PLoS ONE 9:e114367. doi: 10.1371/journal.pone.0114367
Finney, M. A., McHugh, C. W., Grenfell, I. C., Riley, K. L., and Short, K. C. (2011). A simulation of probabilistic wildfire risk components for the continental United States. Stoch. Environ. Res. Risk Assess. 25, 973–1000. doi: 10.1007/s00477-011-0462-z
Flather, C. H., and Bevers, M. (2002). Patchy reaction-diffusion and population abundance: the relative importance of habitat amount and arrangement. Am. Natural. 159, 40–56. doi: 10.1086/324120
Folke, C. (2006). Resilience: the emergence of a perspective for social-ecological systems analysis. Global Environ. Chang. 16, 253–267. doi: 10.1016/j.gloenvcha.2006.04.002
Folke, C., Carpenter, S. R., Walker, B., Scheffer, M., Chapin, T., and Rockström, J. (2010). Resilience thinking: integrating resilience, adaptability and transformability. Ecol. Soc. 15:20. doi: 10.5751/ES-03610-150420
Folke, C., Carpenter, S. R., Walker, B., Scheffer, M., Elmqvist, T., Gunderson, L., et al. (2004). Regime shifts, resilience, and biodiversity in ecosystem management. Annu. Rev. Ecol. Syst. 35, 557–581. doi: 10.1146/annurev.ecolsys.35.021103.105711
Foltête, J. C., Clauzel, C., and Vuidel, G. (2012). A software tool dedicated to the modelling of landscape networks. Environ. Model. Softw. 38, 316–327. doi: 10.1016/j.envsoft.2012.07.002
Frair, J. L., Merrill, E. H., Beyer, H. L., and Morales, J. M. (2008). Thresholds in landscape connectivity and mortality risks in response to growing road networks. J. Appl. Ecol. 45, 1504–1513. doi: 10.1111/j.1365-2664.2008.01526.x
Freeman, J., Kobziar, L., Rose, E. W., and Cropper, W. (2017). A critique of the historical-fire-regime concept in conservation. Cons. Biol. 31, 976–985. doi: 10.1111/cobi.12942
Friedel, M. H. (1991). Range condition assessment and the concept of thresh-olds. a viewpoint. J. Range Manag. 44, 422–426. doi: 10.2307/4002737
FWS (2010). Endangered and Threatened Wildlife and Plants; 12-Month Findings for Petitions to List the Greater Sage-Grouse (Centrocercus urophasianus) as Threatened or Endangered: Federal Register, 75, 13909–14014. Available online at: https://www.federalregister.gov/articles/2010/03/23/2010-5132/endangered-and-threatened-wildlife-and-plants-12-month-findings-for-petitions-to-list-the-greater (accessed May, 20, 2019).
FWS (2013). Greater Sage-Grouse (Centrocercus urophasianus) Conservation Objectives: Final Report. Denver, CO: U.S. Department of the Interior, Fish and Wildlife Service, 91. Available online at: https://www.fws.gov/greatersagegrouse/documents/COT-Report-with-Dear-Interested-Reader-Letter.pdf (accessed May 20, 2019).
Garmestani, A. S., and Benson, M. H. (2013). A framework for resilience-based governance of socialecological systems. Ecol. Soc. 18:9. doi: 10.5751/ES-05180-180109
Germino, M. J., Belnap, J., Stark, J. M., Allen, E. B., and Rau, B. M. (2016). “Ecosystem impacts of exotic annual invaders in the genus Bromus,” in Exotic Brome-grasses in Arid and Semiarid Ecosystems of the Western US: Causes, Consequences, and Management Implications. eds M. J. Germino, J. C. Chambers, and C.S. Brown (New York, NY: Springer: Series on Environmental Management), 61–98.
Grand, J., Buonaccorsi, J., Cushman, S. A., Griffin, C. R., and Neel, M. C. (2004). A multiscale landscape approach to predicting bird and moth rarity hotspots in a threatened pitch pine-scrub oak community. Conserv. Biol. 18, 1063–1077. doi: 10.1111/j.1523-1739.2004.00555.x
Gregory, R., Failing, L., Harstone, M., Long, G., McDaniels, T., and Ohlson, D. (2012). Structured Decision Making: a Practical Guide to Environmental Management Choices. West Sussex: John Wiley and Sons, Ltd.
Guisan, A., and Thuiller, W. (2005). Predicting species distribution: offering more than simple habitat models. Ecol. Letters. 8, 993–1009. doi: 10.1111/j.1461-0248.2005.00792.x
Gunderson, L. H. (2000). Ecological resilience – in theory and application. Ann. Rev. Ecol. Syst. 31, 425–439. doi: 10.1146/annurev.ecolsys.31.1.425
Gunderson, L. H., Allen, C. R., and Holling, C. S. (2010). Foundations of Ecological Resilience. New York, NY: Island Press.
Halofsky, J. E., Peterson, D. L., Dante-Wood, S. K, Hoang, L., Ho, J. J., and Joyce, L. A. eds. (2018a). Climate Change Vulnerability and Adaptation in the Northern Rocky Mountains, Parts 1 and 2. Gen. Tech. Rep. RMRS GTR-374. Fort Collins, CO: U.S. Department of Agriculture, Forest Service, Rocky Mountain Research Station.
Halofsky, J. E., Peterson, D. L., Ho, J. J., Little, N., and Joyce, L. A. eds. (2018b). Climate Change Vulnerability and Adaptation in the Intermountain Region, Parts 1 and 2. Gen. Tech. Rep. RMRS-GTR-375. Fort Collins, CO: U.S. Department of Agriculture, Forest Service, Rocky Mountain Research Station.
Hanski, I., and Ovaskainen, O. (2002). Extinction debt at extinction threshold. Conserv. Biol. 16, 666–673. doi: 10.1046/j.1523-1739.2002.00342.x
Harju, S. M., Dzialak, M. R., Taylor, R. C., Hayden-wing, L. D., and Winstead, J. B. (2010). Thresholds and time lags in effects of energy development on Greater sage-grouse populations. J. Wildlife Manage. 74, 437–448. doi: 10.2193/2008-289
Hearn, A. J., Cushman, S. A., Ross, J., Boossens, B., Hunter, L. T. B., and Macdonald, D. W. (2018). Spatio-temporal ecology of sympatric felids on borneo: evidence for resource partitioning? PlosONE 13:e0200828. doi: 10.1371/journal.pone.0200828
Hessburg, P. F., Reynolds, K. M., Keane, R. E., James, K. M., and Salter, R. B. (2007). Evaluating wildland fire danger and prioritizing vegetation and fuels treatments. Forest Ecol. Manag. 247, 1–17. doi: 10.1016/j.foreco.2007.03.068
Hessburg, P. F., Reynolds, K. M., Salter, R. B., Dickinson, J. D., Gaines, W. L., and Harrod, R. J. (2013). Landscape evaluation for restoration planning on the Okanogan-Wenatchee National Forest, USA. Sustainability 5, 805–840. doi: 10.3390/su5030805
Hessburg, P. F., Smith, B. G., and Salter, R. B. (1999). Detecting change in forest spatial patterns from reference conditions. Ecol. Appl. 9, 1232–1252. doi: 10.1890/1051-0761(1999)009[1232:DCIFSP]2.0.CO;2
Hessburg, P. F., Spies, T. A., Perry, D. A., Skinner, C. N., Taylor, A. H., Brown, P. M., et al. (2016). Tamm review: management of mixed-severity fire regime forests in Oregon, Washington, and Northern California. Forest Ecol. Manag. 366, 221–250. doi: 10.1016/j.foreco.2016.01.034
Hirota, M., Holmgren, M., Van Nes, E. H, and Scheffer, M. (2011). Global resilience of tropical forest and savanna to critical transitions. Science 334, 232–235. doi: 10.1126/science.1210657
Hobbs, R. J., Higgs, E., and Harris, J. A. (2009). Novel ecosystems: implications for conservation and restoration. Trends Ecol. Evol. 24, 599–605. doi: 10.1016/j.tree.2009.05.012
Holl, K. D., and Aide, T. M. (2011). When and where to actively restore ecosystems? Forest Ecol. Manag. 261, 1558–1563. doi: 10.1016/j.foreco.2010.07.004
Holling, C. S. (1973). Resilience and stability of ecological systems. Annu. Rev. Ecol. Syst. 4, 1–23. doi: 10.1146/annurev.es.04.110173.000245
Holling, C. S., and Meffe, G. K. (1996). Command and control and the pathology of natural resource management. Conserv. Biol. 10, 28–337. doi: 10.1046/j.1523-1739.1996.10020328.x
Holloran, M. J., and Anderson, S. H. (2005). Spatial distribution of greater sage-grouse nests in relatively contiguous sagebrush habitats. Condor 107, 742–752. doi: 10.1650/7749.1
IPCC (2014). Climate Change 2014: Synthesis Report. Contribution of Working Groups I, II and III to the Fifth Assessment Report of the Intergovernmental Panel on Climate Change. Available online at: www.ipcc.ch/report/ar5/syr/. doi: 10.1017/CBO9781107415416
Jamiyansharav, K., Fernandez-Gimenez, M. E., Angerer, J. P., Yadamsuren, B., and Dash, Z. (2018). Plant community change in three mongolian steppe ecosystems 1994-2013: applications to state-and-transition models. Ecosphere. 9:e02145. doi: 10.1002/ecs2.2145
Johnson, F. A., Williams, B. K., and Nichols, J. D. (2013). Resilience thinking and a decision-analytic approach to conservation: strange bedfellows or essential partners? Ecol. Soc. 18:27. doi: 10.5751/ES-05544-180227
Johnstone, J. F, Allen, C. D., Franklin, J. F., Frelich, L. E., Harvey, B. J., Higuera, P. E., et al. (2016). Changing disturbance regimes, ecological memory, and forest resilience. Front. Ecol. Environ. 14, 369–378. doi: 10.1002/fee.1311
Jørgensen, S. E., Burkhard, B., and Müller, F. (2013). Twenty volumes of ecological indicators – An accounting short review. Ecol. Indic. 28, 4–9. doi: 10.1016/j.ecolind.2012.12.018
Karchergis, E., Rocca, M. E., and Fernandex-Gimenez, M. E. (2011). Indicators of ecosystem function identify alternate states in the sagebrush steppe. Ecol. Appl. 21, 2781–2792. doi: 10.1890/10-2182.1
Kaszta, Z., Cushman, S. A., Hearn, A. J., Burnham, D., Macdonald, E. A., and Macdonald, D. W. (2019). Integrating Sunda clouded leopard conservation into development and restoration planning in Sabah. Biol. Conserv. 235, 63–76. doi: 10.1016/j.biocon.2019.04.001
Keane, R. E. (2012). “Creating historical range of variation (HRV) time series using landscape modeling: overview and issues, Chapter 9,” in Historical Environmental Variation in Conservation and Natural Resource Management, ed. J.A. Wiens, G. D. Hayward, D. Hugh, and C. Giffen (West Sussex: John Wiley and Sons, Ltd, 113–127.
Keane, R. E., Hessburg, P. F., Landres, P. B., and Swanson, F. J. (2009). The use of historical range and variability (HRV) in landscape management. Forest Ecol. Manag. 258, 1025–1037. doi: 10.1016/j.foreco.2009.05.035
Keeley, J. E., Pausas, J. G., Rundel, P. W., Bond, W. J., and Bradstock, R. A. (2011). Fire as an evolutionary pressure shaping plant traits. Trends Plant Sci. 16, 406–11. doi: 10.1016/j.tplants.2011.04.002
Knick, S. T., Hanser, S. E., Miller, R. F., Pyke, D. A., Wisdom, M. J., and Finn, S. P. (2011). “Ecological influence and pathways of land use in sagebrush,” in Greater Sage-Grouse—Ecology and Conservation of a Landscape Species and Its Habitats, Studies in Avian Biology 38, eds S. T. Knick and J.W. Connelly (Berkeley, CA: University of California Press, 203–251.
Knick, S. T., Hanser, S. E., and Preston, K. L. (2013). Modeling ecological minimum requirements for distribution of Greater sage-grouse leks: implications for population connectivity across their western range, U.S.A. Ecol. Evol. 3, 1539–1551. doi: 10.1002/ece3.557
Lam, V. Y. Y., Doropoulos, C., and Mumby, P. J. (2017). The influence of resilience-based management on coral reef monitoring: a systematic review. PloS ONE. 12:e0172064. doi: 10.1371/journal.pone.0172064
Landguth, E. L., and Cushman, S. A. (2010). CDPOP: a spatially explicit cost distance population genetics program. Mol. Ecol. Res. 10, 156–161. doi: 10.1111/j.1755-0998.2009.02719.x
Laughlin, D. C., Strahan, R. T., Huffman, D. W., and Sánchez Meador, A. J. (2017). Using trait-based ecology to restore resilient ecosystems: historical conditions and the future of montane forests in western North America. Restor. Ecol. 25, S135–S146. doi: 10.1111/rec.12342
Laycock, W. A. (1991). Stable states and thresholds of range condition on North American rangelands: a viewpoint. J. Range Manag. 44, 427–433. doi: 10.2307/4002738
Levine, N. M., Zhanga, K., Longo, M., Baccini, A., Phillips, O. L., Lewis, S. L., et al. (2016). Ecosystem heterogeneity determines the ecological resilience of the amazon to climate change. Proc Natl Acad Sci U.S.A. 113, 793–797. doi: 10.1073/pnas.1511344112
Littell, J. S., McKenzie, D., Wan, H. Y., and Cushman, S. A. (2018). Climate change and wildfire in the Western United States. Earth's Future 6, 1097–1111. doi: 10.1029/2018EF000878
Loehman, R. A., Keane, R. E., Holsinger, L. M, and Wu, Z. (2017). Interactions of landscape disturbances and climate change dictate ecological pattern and process: spatial modeling of wildfire, insect, and disease dynamics under future climates. Landscape Ecol. 32:1447. doi: 10.1007/s10980-016-0414-6
Macdonald, E. A., Cushman, S. A., Landguth, E. L., Hearn, A. J., Malhi, Y., and Macdonald, D. W. (2018). Simulating impacts of rapid forest loss on population size, connectivity and genetic diversity of Sunda clouded leopards in Borneo. PloS ONE 13:e0196974. doi: 10.1371/journal.pone.0196974
MacMahon, J. A. (1981). “Successional processes: comparisons among biomes with special reference to probable roles of and influences on animals,” in Forest Succession Concepts and Application, eds D.C. West, H. H. Shugart, and D.B. Botkin. (New York, NY: Springer-Verlag, 277–304.
Maestas, J. D., Campbell, S. B., Chambers, J. C., Pellant, M., and Miller, R. F. (2016). Tapping soil survey information for rapid assessment of sagebrush ecosystem resilience and resistance. Rangelands 38, 120–128. doi: 10.1016/j.rala.2016.02.002
Marcot, B. G., Thompson, M. P., Runge, M. C., Thompson, F. R., McNulty, S., Cleaves, D., et al. (2012). Recent advances in applying decision science to managing national forests. Forest Ecol. Manag. 285, 123–132. doi: 10.1016/j.foreco.2012.08.024
McGarigal, K., and Cushman, S. A. (2005). “The gradient concept of landscape structure,” in Issues and Perspectives in Landscape Ecology, eds J. A. Wiens and M. R. Moss (Cambridge, UK: Cambridge University Press), 112–119.
McGarigal, K., Cushman, SA., and Ene, E. (2012). FRAGSTATS v4: Spatial Pattern Analysis Program for Categorical and Continuous Maps. Computer software program produced by the authors at the University of Massachusetts, Amherst. Available online: http://www.umass.edu/landeco/research/fragstats/fragstats.html
McGarigal, K., Mallek, M., Estes, B., Tierney, M., Walsh, T., Thane, T., et al. (2018). Modeling Historical Range of Variability and Alternative Management Scenarios in the Upper Yuba River Watershed, Tahoe National Forest, California. Gen. Tech. Rep. RMRS-GTR-385. Fort Collins, CO: U.S. Department of Agriculture, Forest Service, Rocky Mountain Research Stations.
McIntyre, N. E, Wright, C. K., Swain, S., Hayhoe, K., Liu, G., Schwartz, F. W., et al. (2014). Climate forcing of wetland landscape connectivity in the great plains. Front. Ecol. Environ. 12, 59–64. doi: 10.1890/120369
McKenzie, D., Miller, C., and Falk, D. A. eds. (2011). The Landscape Ecology of Fire. New York, NY: Springer Science and Business Media.
Millar, C. I. (2014). Historic variability: Informing restoration strategies, not prescribing targets. J. Sustain. Forestry 33(suppl 1), S28–S42. doi: 10.1080/10549811.2014.887474
Millar, C. I., Stephenson, N. L., and Stephens, S. L. (2007). Climate change and forests of the future: managing in the face of uncertainty. Ecol. Appl. 17, 2145–2151. doi: 10.1890/06-1715.1
Miller, R. F., Knick, S. T., Pyke, D. A., Meinke, C. W., Hanser, S. E., Wisdom, M. J., et al. (2011). “Characteristics of sagebrush habitats and limitations to long-term conservation,” in Greater Sage-Grouse—Ecology and Conservation of a Landscape Species and Its Habitats, Studies in Avian Biology 38, eds S. T. Knick, and J. W. Connelly. (Berkeley, CA: University of California Press, 145–185.
Moritz, M. A., Hessburg, P. F., and Povak, N. A. (2011). “Native fire regimes and landscape resilience,” in The Landscape Ecology of Fire, eds D. McKenzie, C. Miller, and D. A. Falk (Dordrecht: Springer, 51–86.
Neel, M. C., McGarigal, K., and Cushman, S. A. (2004). Behavior of class-level landscape metrics across gradients of class aggregation and area. Landscape Ecol. 19:435. doi: 10.1023/B:LAND.0000030521.19856.cb
Ng, C. N., Xie, Y. J., and Yu, X. J. (2013). Integrating landscape connectivity into the evaluation of ecosystem services for biodiversity conservation and its implications for landscape planning. Appl. Geogr. 42, 1–12. doi: 10.1016/j.apgeog.2013.04.015
Olds, A. D., Pitt, K. A., Maxwell, P. S., and Connolly, R. M. (2012). Synergistic effects of reserves and connectivity on ecological resilience. J. Appl. Ecol. 49, 1195–1203. doi: 10.1111/jpe.12002
Ovaskainen, O. (2002). Long-term persistence of species and the SLOSS problem. J. Theor. Biol. 218, 419–433. doi: 10.1006/jtbi.2002.3089
Paruelo, J. M., and Lauenroth, W. (1996). Relative abundance of plant functional types in grasslands and shrublands of North America. Ecol. Appl. 6, 1212–1224. doi: 10.2307/2269602
Pecl, G. T., Araújo, M. B., Bell, J. D., Blanchard, J., Bonebrake, T. C., Chen, I. C., et al. (2017). Biodiversity redistribution under climate change: impacts on ecosystems and human well-being. Science 355, 1389–1400. doi: 10.1126/science.aai9214
Peterson, G. D. (2002). Contagious disturbance, ecological memory, and the emergence of landscape pattern. Ecosystems 5, 329–338. doi: 10.1007/s10021-001-0077-1
Peterson, G. D., Allen, C. R., and Holling, C. S. (1998). Ecological resilience, biodiversity, and scale. Ecosystems 1, 6–18. doi: 10.1007/s100219900002
Phillips, J. D. (2009). Changes, perturbations, and responses in geomorphic systems. Prog. Phys. Geography 33, 17–30. doi: 10.1177/0309133309103889
Pickett, S. T. A., Kolasa, J., Armesto, J. J., and Collins, S. L. (1989). The ecological concept of disturbance and its expression at various hierarchical levels. Oikos 54, 129–136. doi: 10.2307/3565258
Pickett, S. T. A., and White, P. S. (1985). The Ecology of Natural Disturbance and Patch Dynamics. Orlando, FL: Academic Press.
Pope, K. L., Allen, C. R., and Angeler, D. G. (2014). Fishing for resilience. T. N. Am. Fisheries Soc. 143, 467–478. doi: 10.1080/00028487.2014.880735
Provencher, L., Forbis, T. A., Frid, L., and Medlyn, G. (2007). Comparing alternative management strategies of fire, grazing, and weed control using spatial modeling. Ecol. Model. 209, 249–263. doi: 10.1016/j.ecolmodel.2007.06.030
Rappaport, D. I., Tambosi, L. R., and Metzger, J. P. (2015). A landscape triage approach: combining spatial and temporal dynamics to prioritize restoration and conservation. J. Appl. Ecol. 52, 590–601. doi: 10.1111/1365-2664.12405
Reynolds, K. M., and Hessburg, P. F. (2005). Decision support for integrated landscape evaluation and restoration planning. Forest Ecol. Manage. 207, 263–278. doi: 10.1016/j.foreco.2004.10.040
Ricca, M. A., Coates, P. S., Gustafson, K. B., Brussee, B. E., Chambers, J. C., Lisius, S., et al. (2018). Using indices of species distribution, resilience, and resistance as an ecological currency for conservation planning of Greater sage-grouse. Ecol. Appl. 28, 878–896. doi: 10.1002/eap.1690
Roberts, C. P., Burnett, J. L., Donovan, V. M., Wonkka, C., Bielski, C. H., Allen, C. R., et al. (2018). Early warnings for state transitions. Rangeland Ecol. Manage. 71, 659–670. doi: 10.1016/j.rama.2018.04.012
Rudnick, D. A., Ryan, S. J., Beier, P., Cushman, S. A., Dieffenback, F., Epps, C. W., et al. (2012). The Role of Landscape Connectivity in Planning and Implementing Conservation and Restoration Priorities. Issues in Ecology. Report No. 16. Washington, DC: Ecological Society of America.
Runge, M. C., Converse, S. J., and Lyons, J. E. (2011). Which uncertainty? using expert elicitation and expected value of information to design an adaptive program. Biol. Conserv. 144, 1214–1223. doi: 10.1016/j.biocon.2010.12.020
Sala, O., Lauenroth, W., and Golluscio, R. (1997). “Plant functional types in temperate semi-arid regions,” in Plant Functional Types: Their Relevance to Ecosystem Properties and Global Change, ed. T.M. Smith, H. H. Shugart and F. I. Woodward (Cambridge, MA: Cambridge University Press, 217–233.
Sasakia, T., Furukawa, T., Iwasakic, Y., Seto, M., and Mori, A. S. (2015). Perspectives for ecosystem management based on ecosystem resilience and ecological thresholds against multiple and stochastic disturbances. Ecol. Indic. 57, 395–408. doi: 10.1016/j.ecolind.2015.05.019
Saura, S., and Pascual-Hortal, L. (2007). A new habitat availability index to integrate connectivity in landscape conservation planning: comparison with existing indices and application to a case study. Landsc. Urban Plan. 83, 91–103. doi: 10.1016/j.landurbplan.2007.03.005
Scheffer, M., Carpenter, S. R., Foley, J. A., Folke, C., and Walker, B. (2001). Catastrophic shifts in ecosystems. Nature 413, 591–596. doi: 10.1038/35098000
Scheffer, M., Carpenter, S. R., Lenton, T. M., Bascompte, J., Brock, W., Dakos, V., et al. (2012). Anticipating critical transitions. Science 338, 334–338. doi: 10.1126/science.1225244
Schweiger, E. W., Grace, J. B., Cooper, B. D. B., and Britten, M. (2016). Using structural equation modeling to link human activities to wetland ecological integrity. Ecosphere 7:e01548. doi: 10.1002/ecs2.1548
Seidl, R., Spies, T. A., Peterson, D. L., Stephens, S. L., and Hick, J. A. (2016). Searching for resilience: addressing the impacts of changing disturbance regimes on forest ecosystem services. J. Appl. Ecol. 53, 120–129. doi: 10.1111/1365-2664.12511
Shirk, A. J., Cushman, S. A., Waring, K. M., Wehenkel, C. A., Leal-Sáenz, A., Toney, C., et al. (2018). Southwestern white pine (Pinus strobiformis) species distribution models project a large range shift and contraction due to regional climatic changes. For. Ecol. Manage. 411, 176–186. doi: 10.1016/j.foreco.2018.01.025
Short, K. C., Finney, M. A., Scott, J. H., Gilbertson-Day, J. W., and Grenfell, I. C. (2016). Spatial Dataset of Probabilistic Wildfire Risk Components for the Conterminous United States. Forest Service Research Data Archive. Fort Collins, CO: U.S. Department of Agriculture, Forest Service, Rocky Mountain Research Station.
Siddig, A. A. H., Ellison, A. M., Ochs, A., Villar-Leeman, C., and Lau, M. K. (2016). How do ecologists select and use indicator species to monitor ecological change? Insights from 14 years of publication in ecological indicators. Ecol. Indic. 60, 223–230. doi: 10.1016/j.ecolind.2015.06.036
Snyder, K. A., Evers, L., Chambers, J. C., Dunham, J., Bradford, J. G., and Loik, M. E. (2018). Effects of changing climate on the hydrological cycle in cold desert ecosystems of the great basin and columbia plateau. Rangeland Ecol. Manag. 72, 1–12. doi: 10.1016/j.rama.2018.07.007
Spanbauer, T. L., Allen, C. R., Angeler, D. G., Eason, T., Nash, K. L., Fritz, S. C., et al. (2014). Prolonged instability prior to a regime shift. PLoS ONE 9:e108936. doi: 10.1371/journal.pone.0108936
Spasojevic, M. J., Bahlai, C. S., Bradley, B. A., Butterfield, J., Tuanmu, M., STla, S. S., et al. (2016). Scaling up the diversity–resilience relationship with trait databases and remote sensing data: the recovery of productivity after wildfire. Glob. Chang. Biol. 22, 1421–1432. doi: 10.1111/gcb.13174
Stiver, S. J., Apa, A. D., Bohne, J. R., Bunnell, S. D., Diebert, P., Gardner, S., et al. (2006). Greater Sage-Grouse Comprehensive Conservation Strategy. Cheyenne, WY: Western Association of Fish and Wildlife Agencies, 442. Available online at: https://wdfw.wa.gov/publications/01317 (accessed May 20, 2019).
Stringham, T. K., Krueger, W. C., and Shaver, P. L. (2003). State and transition modeling: an ecological process approach. J. Range Manag. 56, 106–113. doi: 10.2307/4003893
Suding, K. N., Gross, K. L., and Houseman, G. R. (2004). Alternative states and positive feedbacks in restoration ecology. Trends Ecol. Evol. 19, 46–53. doi: 10.1016/j.tree.2003.10.005
Sundstrom, S., and Allen, C. R. (2014). Complexity versus certainty in understanding species' declines. Divers. Distribut. 20, 344–355. doi: 10.1111/ddi.12166
Sundstrom, S. M., Angeler, D. G., Barichievy, C., Eason, T., Garmestani, A. S., Gunderson, L. H., et al. (2018). The distribution and role of functional abundance in cross-scale resilience. Ecology 99, 2421–2432. doi: 10.1002/ecy.2508
Sundstrom, S. M., Eason, T., Nelson, R. J., Angeler, D. G., Barichievy, C., Garmestani, A. S., et al. (2017). Detecting spatial regimes in ecological systems. Ecol. Lett. 20, 19–32. doi: 10.1111/ele.12709
Suring, L. H., Rowland, M. M., and Wisdom, M. J. (2005). “Identifying species of conservation concern,” in, Habitat Threats in the Sagebrush Ecosystem: Methods of Regional Assessment and Applications in the Great Basin, ed M. J. Wisdom, M. M Rowland, and L.H. Suring (Lawrence, KS: Alliance Communications Group, 150–162.
Tambosi, L. R., Martensen, A. C., Ribeiro, M. C., and Metzger, J. P. (2014). Framework to optimize biodiversity restoration efforts based on habitat amount and landscape connectivity. Restor. Ecol. 22, 169–177. doi: 10.1111/rec.12049
Thatte, P., Joshi, A., Vaidyanathan, S., Landguth, E., and Ramakrishnan, U. (2018). Maintaining tiger connectivity and minimizing extinction into the next century: insights from landscape genetics and spatially-explicit simulations. Biol. Conserv. 218, 181–191. doi: 10.1016/j.biocon.2017.12.022
Theobald, D. M., Crooks, K. R., and Norman, J. B. (2011). Assessing effects of land use on landscape connectivity: loss and fragmentation of western U.S. forests. Ecol. Appl. 21, 2445–2458. doi: 10.1890/10-1701.1
Thompson, C. M., and McGarigal, K. (2002). The influence of research scale on bald eagle habitat selection along the lower Hudson River, New York (USA). Landscape Ecol. 17, 569–586. doi: 10.1023/A:1021501231182
Trombore, S., Brando, P., and Hartman, H. (2015). Forest health and global change. Science 349:814–818. doi: 10.1126/science.aac6759
Turner, M. G. (1989). Landscape ecology: the effect of pattern on process. Annu. Rev. Ecol. Syst. 20, 171–197. doi: 10.1146/annurev.es.20.110189.001131
Twidwell, D., Wonkka, C. L., Wang, H., Grant, W. E., Allen, C. R., Samuel D Fuhlendorf, C. R., et al. (2019). Coerced resilience in fire management. J. Environ. Manag. 240, 368–373. doi: 10.1016/j.jenvman.2019.02.073
Uden, D. R., Hellman, M. L., Angeler, D. G., and Allen, C. R. (2014). The role of reserves and anthropogenic habitat for functional connectivity and resilience of ephemeral wetlands. Ecol. Appl. 24, 1569–1582. doi: 10.1890/13-1755.1
Urza, A., Weisberg, P. J., Chambers, J. C., Board, D. I., Dhaemers, J., and Flake, S. (2017). Post-fire vegetation response at the woodland-shrubland interface is mediated by the pre-fire community. Ecosphere 8:e01851. doi: 10.1002/ecs2.1851
USDI (2009). Adaptive Management: The U.S. Department of the Interior Technical Guide. Adaptive Management Working Group. Washington, DC: U.S. Department of the Interior [USDI], 84. Available online at: https://pubs.er.usgs.gov/publication/70194537 (accessed May 18, 2019).
USDI (2015). An Integrated Rangeland Fire Management Strategy. Final Report to the Secretary of the Interior. Available online at: https://www.forestsandrangelands.gov/documents/rangeland/IntegratedRangelandFireManagementStrategy_FinalReportMay2015.pdf (accessed May 21, 2019).
USDI (2016). Safeguarding America's Lands and Waters From Invasive Species: A National Framework for Early Detection and Rapid Response. Washington DC: U.S. Department of the Interior, 55. Available online at: https://www.doi.gov/sites/doi.gov/files/National%20EDRR%20Framework.pdf (accessed May, 20, 2019).
USDI FWS (2015). Endangered and Threatened Wildlife and Plants; 12-Month Finding on a Petition to List the Greater Sage-Grouse (Centrocercus urophasianus) as an Endangered or Threatened Species. Proposed Rule. Fed. Register 80, 59858-59942. Available online at: http://www.gpo.gov/fdsys/pkg/FR-2015-10-02/pdf/2015-24292.pdf (accessed May 20, 2019).
USEPA (2017). Level II and Level III Ecoregions of the Continental United States: Corvallis, OR: U.S EPA-National Health and Environmental Research Laboratory, Map scale 1:750,000. Available online at: https://www.epa.gov/eco-research/ecoregions-north-america (accessed May, 20, 2019).
USGS (2016). LANDFIRE 2.0 Existing Vegetation Type Layer. Washington, DC: U.S. Department of the Interior, Geological Survey. Available online at: http://landfire.cr.usgs.gov/viewer/ (accessed May 20, 2019).
Walker, B., Holling, C. S., Carpenter, S. R., and Kinzig, A. (2004). Resilience, adaptability and transformability in social–ecological systems. Ecol. Soc. 9:5. doi: 10.5751/ES-00650-090205
Wasserman, T. N., Cushman, S. A., Shirk, A. S., Landguth, E. L., and Littell, J. S. (2012). Simulating the effects of climate change on population connectivity of the American marten (Martes Americana) in the northern Rocky Mountains, USA. Landscape Ecol. 27, 211–225. doi: 10.1007/s10980-011-9653-8
Westerling, A. L., Hidalgo, H. G., Cayan, D. R., and Swetnam, T. W. (2006). Warming and earlier spring increase western US forest wildfire activity. Science 313, 940–943. doi: 10.1126/science.1128834
Westerling, A. L. R. (2016). Increasing western US forest wildfire activity: sensitivity to changes in the timing of spring. Philos. T. Roy. Soc. B 371:20150178. doi: 10.1098/rstb.2015.0178
Westoby, M., Walker, B., and Noy-Meir, I. (1989). Opportunistic management for rangelands not at equilibrium. J. Range Manag. 42, 266–274. doi: 10.2307/3899492
Wiens, J. A., Hayward, G. D., Hugh, D., and Giffen, C. (2012). Historical Environmental Variation in Conservation and Natural Resource Management. West Sussex: John Wiley and Sons, Ltd.
Williams, B. K. (2011). Adaptive management of natural resources – framework and issues. J. Env. Manag. 92, 1346–1353. doi: 10.1016/j.jenvman.2010.10.041
With, K. A., and King, A. W. Extinction thresholds for species in fractal landscapes. (1999). Conserv. Biol. 13, 314–326. doi: 10.1046/j.1523-1739.1999.013002314.x
Wu, J. (2013). Landscape sustainability science: ecosystem services and human well-being in changing landscapes. Landsc. Ecol. 28, 999–1023. doi: 10.1007/s10980-013-9894-9
Wu, J., and Loucks, O. L. (1995). Balance of nature to hierarchical patch dynamics: a paradigm shift in ecology. Q. Rev. Biol. 70, 439–466. doi: 10.1086/419172
Zelmer, S. B., and Gunderson, L. H. (2009). Why resilience may not always be a good thing: lessons in ecosystem restoration from glen canyon and the Everglades. Nebraska Law Rev. 87, 893–949.
Zurlini, G., Jones, K. B., Riitters, K. H., Li, B., and Petrosillo, I. (2014). Early warning signals of regime shifts from cross-scale connectivity of land-cover patterns. Ecol. Indic. 45, 549–560. doi: 10.1016/j.ecolind.2014.05.018
Keywords: ecological resilience, spatial resilience, landscape context, ecosystems, natural resources management, restoration, conservation, management framework
Citation: Chambers JC, Allen CR and Cushman SA (2019) Operationalizing Ecological Resilience Concepts for Managing Species and Ecosystems at Risk. Front. Ecol. Evol. 7:241. doi: 10.3389/fevo.2019.00241
Received: 14 February 2019; Accepted: 11 June 2019;
Published: 12 July 2019.
Edited by:
Anouschka R. Hof, Wageningen University & Research, NetherlandsReviewed by:
Brendan Alexander Harmon, Louisiana State University, United StatesMatthew Thompson, Rocky Mountain Research Station, United States
At least a portion of this work is authored by Jeanne C. Chambers, Craig R. Allen and Samuel A. Cushman on behalf of the U.S. Government and, as regards Dr. Jeanne C. Chambers, Craig R. Allen, Samuel A. Cushman and the U.S. Government, is not subject to copyright protection in the United States. Foreign and other copyrights may apply. This is an open-access article distributed under the terms of the Creative Commons Attribution License (CC BY). The use, distribution or reproduction in other forums is permitted, provided the original author(s) and the copyright owner(s) are credited and that the original publication in this journal is cited, in accordance with accepted academic practice. No use, distribution or reproduction is permitted which does not comply with these terms.
*Correspondence: Jeanne C. Chambers, jeanne.chambers@usda.gov