- 1Department of Biological Sciences, Simon Fraser University, Burnaby, BC, Canada
- 2National Center for Ecological Analysis and Synthesis, Santa Barbara, CA, United States
- 3Bren School of Environmental Science and Management, University of California, Santa Barbara, Santa Barbara, CA, United States
- 4Imperial College London, Ascot, United Kingdom
- 5School of Aquatic and Fishery Sciences, University of Washington, Seattle, WA, United States
Many of the world's ecosystems are experiencing a suite of changes from anthropogenic activities; the multiple stressors from those activities result in cumulative impacts. Understanding how these activities translate into ecological consequences is exceedingly challenging because of the inherent complexity within natural systems and the variability in how stressors act and how species respond. While there have been substantial advancements within the field of cumulative effects assessment to address these issues and improve our understanding of the consequences of our actions, many challenges remain. Here, we detail advances and remaining challenges, and propose five priorities for addressing these challenges in the near future. In particular, we suggest prioritizing risk-based approaches that account for uncertainty in our understanding and establishing an underlying theory for when we expect particular impacts to occur. We also propose the need for a defined subdiscipline focused on cumulative effects, to help reduce the silos of research that are often disconnected, and to work toward a common set of definitions, methods and the consistent use of open data.
Introduction
The world's ecosystems are experiencing a suite of changes from exposure to multiple human disturbances. The consequences of these multiple stressors, termed cumulative effects, can result in substantial changes for individual species or entire ecosystems (Halpern et al., 2008; Vorosmarty et al., 2010). However, it is exceedingly challenging to understand the cumulative consequences of multiple stressors because the stressors themselves vary across space and time (Cocklin et al., 1992a), and species' responses are diverse and not clearly predictable (Crain et al., 2008; Przeslawski et al., 2014). Further, responses can be non-linear (Hunsicker et al., 2016) and can cause cascading effects within ecosystems (Kaplan et al., 2010; Griffith et al., 2012). Though these complicating factors present a profound challenge, there have been substantial developments within the field of cumulative effects assessment to address some of these complexities (Shopley and Fuggle, 1984; Cocklin et al., 1992b; Smit and Spaling, 1995; Duinker et al., 2012; Boyd et al., 2018; Hodgson and Halpern, 2018).
Multiple methods exist to assess cumulative effects, but each are limited in some way (Hodgson and Halpern, 2018). On the one hand, mapping-based approaches can identify areas or species that are most vulnerable to a high number of co-occurring (often 10 or more) stressors (Halpern et al., 2008; Maxwell et al., 2013). However, this method is generally based on assumptions of additive effects (Halpern and Fujita, 2013) and only considers direct impacts. Alternatively, experimental methods (and their synthesis in meta-analysis; Crain et al., 2008; Darling and Côté, 2008) can identify responses that are additive, synergistic, or antagonistic (Darling and Côté, 2008; Côté et al., 2016), and in some cases can identify indirect impacts; however, these approaches hit a practical limit beyond three stressors. That is, teasing out the unique and interactive contribution of individual and sets of stressors in a factorial experimental design is increasingly more complicated with four, five, 10, (or more) stressors (Boyd et al., 2018; Hodgson and Halpern, 2018).
Here, we review key advances within the field to better understand its current state and identify remaining challenges and priorities to overcome them. Our review was informed by input from experts in the field. We made an effort to conduct a survey of experts, to identify common and shared challenges and opportunities. While the response rate was not sufficiently high to permit a formal evaluation, the information we received proved to be useful in clarifying and informing our own opinions. Contributions from experts primarily contributed to identifying the advances and challenges described below. We list survey respondents (who chose to be acknowledged) in the Acknowledgments section.
Review of Advances to Date
We begin with a brief review of some major advances in understanding and managing cumulative effects. These advances have emerged from both cumulative effects research and the application of the ideas to broader societal and policy needs.
Advances in Conceptual Thinking
Scientists and managers have made important shifts toward recognizing that stressors do not occur in isolation and that many contributing factors govern the cumulative impact of multiple stressors. This is a notable shift that enables further development of cumulative effects assessment. While some cases clearly benefited from the former approach of focusing on a single agent of change (e.g., linking DDT pesticide to bird population collapses Porter and Wiemeyer, 1969), others have revealed its limits. For example, evidence suggests that the decline of bees in North America is a result of multiple stressors, and as a result conservation efforts would benefit from a diversity of mitigative measures (Goulson et al., 2015). This shift in thinking and approach is clear in both the exponential increase in scientific research on cumulative effects (Figure 1) and the institutionalization of cumulative effects thinking in management approaches such as Ecosystem-Based Management (EBM), Marine Spatial Planning and Systematic Conservation Planning.
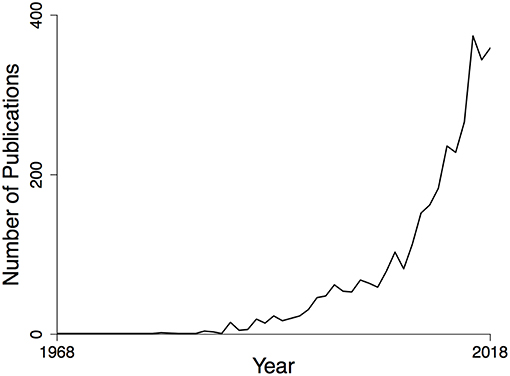
Figure 1. Trend in number of publications in Web of Science with the key words “cumulative effects”, “cumulative impacts” or “multiple stressors” from the fields of environmental sciences, ecology, marine freshwater biology, toxicology, environmental studies, biodiversity conservation and fisheries.
Improvements in Methodologies
An incredible diversity of methods has been developed and employed, providing academics and practitioners with a strong scientific toolbox. For example, there are methods to understand biological consequences at the physiological (Munday et al., 2009), population (Power, 1997; Hodgson et al., 2017), and ecosystem scales (Griffith et al., 2012; Griffith and Fulton, 2014). There is also an increasing focus on understanding non-linear responses (Hunsicker et al., 2016) and related tipping points (Selkoe et al., 2015). Indeed, the field has gained traction (Figure 1) with an increasing number of studies focused on understanding the consequences of multiple stressors. For more detail on the many methods that exist, we point readers to a host of synthesis papers offering additional background on specific approaches (Shopley and Fuggle, 1984; Cocklin et al., 1992b; Smit and Spaling, 1995; Duinker et al., 2012; Foley et al., 2017; Boyd et al., 2018; Hodgson and Halpern, 2018).
Increasing Access to Data
The current era of rapidly increasing data availability, i.e., the rise of “Big Data”, and the push toward open-data providing access to datasets of all sizes, is transforming the type, scope and speed of cumulative effects assessments. For example, we now have access to large data sets, such as satellite imagery, large data repositories of ecological and environmental data, and citizen science collection platforms. These allow us to better contextualize and identify multiple agents of ecological change and address types of questions that could not have been addressed before. For instance, Halpern et al. (2008) and Hampton et al. (2015) tapped information from a wide range of sources, including satellites, national reporting statistics, and expert judgement, to map the distribution of stressors affecting marine ecosystems globally. These data sources also allow us to expand the scope of our scientific investigations, by making it possible to address questions that require more data than a single lab can collect (Hampton et al., 2013, 2015; Soranno and Schimel, 2014). Questions such as how, where and how many ecosystems and species are affected by the impacts of climate change or invasive species could not be readily addressed through conventional single-investigator data collection programs. Moreover, the value that access to more data provides, goes beyond cumulative effects assessment, to ecology more generally.
Integration Into Legislation and Regulations
Natural resource policy and regulatory guidelines in many countries, for example Canada, New Zealand, the United Kingdom and the United States, explicitly require addressing cumulative effects in environmental impact assessments. While, challenges and limitations to cumulative effects assessment approaches used in specific countries remain (Cooper and Sheate, 2002; Canter and Ross, 2010; Greig and Duinker, 2011; Duinker et al., 2012; Foley et al., 2017), the codification in law of the need to account for cumulative effects creates a powerful mandate.
Where do we go From Here? Remaining Challenges and Opportunities for the Future
Despite rising awareness of the impact of cumulative effects on species and natural systems, and the many scientific and practical advances to date, the field faces a number of remaining challenges. In Table 1 we highlight key challenges and some potential solutions gleaned from our own experiences and expert input. We use these challenges to identify five priorities that we, as experts in this field, believe will contribute substantially to addressing remaining challenges in cumulative effects research. We note that this section identifies our perspectives on the field of cumulative effects, in an effort to be forward-looking.
Science to Aid Decision-Making
The complexity inherent in ecosystems and the processes that drive responses to multiple stressors means that most aspects of cumulative effects remain unstudied. The lack of data on species and ecosystem responses to anthropogenic stressors limits our ability to generate a deeper understanding of how, where, why, and when particular responses occur. Even a simple diagram of the pathways that lead to cumulative effects (Figure 2) illustrates the diversity of information needed to assess cumulative impacts. This problem is even more pronounced when one considers the spatial and temporal dynamics underlying these connections. For example, we often have information on the spatial distribution of human activities, but then have to assume or model the distribution of the various stressors associated with that human activity (Halpern and Fujita, 2013).
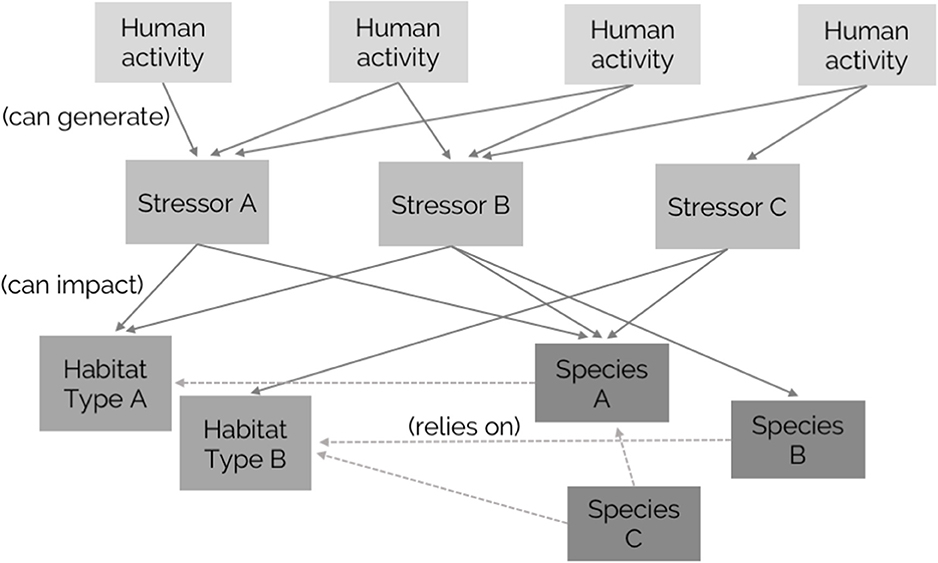
Figure 2. Hypothetical pathways of effects from human activities through to ecological components of the system.
Compounding the challenge of missing data is that relevant data for cumulative effects assessments are frequently hidden in resource management agency reports, environmental consultant files, or unpublished or unshared data from scientific publications. For example, the hundreds of environmental impact assessments that are done annually to evaluate the potential impact of human activities, represent a trove of difficult-to-access data relevant to cumulative effects assessments; these reports are often very substantial in length and the raw data is not open access. This problem is not unique to cumulative effects assessment but is especially salient in this context because of the added difficulty of the task, and the growing need for such assessments.
Additionally, decision-making frameworks in many jurisdictions impede the application of cumulative effects assessment. Legislation and associated policies and regulations often dictate the strength of environmental impacts management, and in many countries, these remain weak or non-existent. For example, concerns about the issue of professional reliance has arisen recently in Canada. Professional reliance is when we rely on professionals who are paid by industry to make decisions regarding the level of impact of a particular activity (Haddock, 2018). More broadly, there are issues in the focus of these assessments, such as a focus on project approval rather than sustainability (Noble, 2010), and there can be inconsistency in methods used between practitioners (Foley et al., 2017).
Perfectly understanding and predicting the ecosystem consequences from cumulative stressors, fully accounting for non-linearities and indirect effects within a system, is an unrealistic objective for scientific research, particularly in a decision making context (Schindler and Hilborn, 2015). Indeed, gaining unequivocal evidence linking ecological change to single stressors is challenging, as nature rarely provides perfect experiments. The question therefore becomes: what is a realistic and appropriate objective for cumulative effects assessments and what tools are available to purse that objective?
We see two important priorities that will aid the application of cumulative effects assessment in decision-making.
A more realistic objective is to have sufficient information to allow balanced decisions under unavoidable uncertainty. To achieve this objective, we need a decision framework that includes uncertainty and tools that can provide information in cases where information is limited. A risk-based framework provides such an approach, focusing attention and resources on issues and areas where the severity of consequences are likely greatest for things that people value most (Aven and Renn, 2009). This approach and method has recently been detailed for cumulative effects assessments (Stelzenmüller et al., 2018), where the authors identify how cumulative effects can be embedded in the risk management framework (see Figure 1 in Stelzenmüller et al., 2018).
In this case, the standard steps within risk management would be required, including risk identification, risk analysis, risk evaluation, and then risk management (ISO, 2009). The change to the standard cumulative effects assessment framework would primarily be in the analysis phase, where uncertainty would be explicitly considered as a factor in risk. Moreover, in the management and decision-making phase, this assessment process would require defining tolerance levels of “acceptable risk” and not just the more common approach of determination of significance, used in impact assessment processes. Risk assessments have been used more frequently, for example, in some fisheries impact assessments (Fletcher, 2005, 2014; Astles et al., 2006).
A key strategy within a risk assessment framework would be to develop heuristics, or rules of thumb, that help identify attributes of individuals, populations or ecosystems, or attributes of stressors, that increase the likelihood of particular effects. For example, we have found that the shape of a species' density dependence function can dictate whether population impacts are mitigated or magnified when exposed to two stressors at different points in a species life history (Hodgson et al., 2017). This is a heuristic that could be used to predict population impacts from multiple stressors, by identifying the form of the density dependence curve. In a decision-making context, knowing when certain effects are likely or unlikely to occur can help structure a decision analysis. Though many cumulative effects research test the outcomes from sets of stressors, there is a lack of theoretical underpinnings supporting predictive capacity of when particular impacts might be expected to occur. Identifying these characteristics for species, systems and stressors would be particularly useful for circumstances where we have limited data (Côté et al., 2016).
Building Inter- and Transdisciplinary Frameworks
Cumulative impacts are ultimately a trans-disciplinary issue, requiring different disciplines, perspectives, and approaches to fully understand how the complexity described above manifests as ecosystem consequences. Only relatively recently has interdisciplinary research [research that “analyzes, synthesizes and harmonizes links between disciplines into a coordinated and coherent whole” (Choi and Pak, 2006)] and trans-disciplinary research [research that “integrates the natural, social and health sciences in a humanities context, and transcends their traditional boundaries” (Choi and Pak, 2006)] been emphasized, yet it remains uncommon. This reality has perpetuated a number of key disconnects among people that hinder progress on cumulative effects research.
One of the primary disconnects is among disciplines within the biological sciences, an age-old challenge in academia. At the species level, multiple stressors affect species through many different pathways—direct mortality (e.g., harvest), physiology (e.g., increased temperature), indirect mortality (e.g., toxic pollution), morbidity (e.g., diseases), etc. – that require a range of disciplines (ecology, microbiology, physiology, etc.) to disentangle.
Equally important, the common disconnect between scientists and practitioners constrains academic research from being connected to real-world problems (Noble, 2010), limiting the likelihood that the science will be informed by, or have a chance of, changing management practice in meaningful ways. This disconnect is not simply a practical challenge; research is missing the opportunity to guide the questions addressed with a real-world context. Conversely, when project-specific assessments are conducted by practitioners, assessments can be restricted in scope, limiting the extent of the cumulative effects assessment overall (Noble, 2010; Singh et al., 2018) and not necessarily accounting for the broader context that an academic approach might include (Dubé, 2003). This disconnect between the fields in the approaches used and scales assessed (Dubé, 2003) limits the extent to which assessments are both thorough and useful to decision-making processes.
Additionally, the structure and mandate of resource management agencies arose and grew at a time when few people were thinking about how sectors interact with each other (i.e., the “silo” effect of resource management) or when multiple stressors were abundant enough to lead to common cumulative effects. The institutional structures of these agencies and the laws and regulations that guide them have, from our view, created inertia that challenges adequate or comprehensive treatment of cumulative effects. Efforts to shift focus to Ecosystem Management on land and later Ecosystem-Based Management in the ocean aimed to break through this inertia, with cumulative effects assessment a central pillar of contemporary EBM (Vitousek et al., 1997).
These disconnects cause inconsistencies in methods and terminology. In general, bringing a diversity of methods and approaches to a common research question is good, as it helps reveal different aspects of the same question while also bringing multiple lines of evidence to bear on the answer. Such diversity can also create challenges, though, when methods test different parts of the problem, leading to science proceeding like the elephant metaphor, where different people are touching different parts of an elephant and misidentify what they are touching. When different studies are testing different pairwise combinations of stressors (and rarely more than two) at different intensity ranges using different methods (lab, field, microcosm, etc.) at different spatial and temporal scales with different species at different abundances, it quickly becomes impossible to see the elephant for all of its parts. One way that scientists have tried to get around this disconnect is through the synthesis of multiple studies on similar stressors (Crain et al., 2008; Przeslawski et al., 2014), however, improved communication among cumulative effects researchers would help substantially.
We identify three priorities intended to help address these disconnects.
Because research works within academic disciplines (“silos”) and a disconnect between researchers and practitioners exists, greater opportunities for information and conceptual exchange will exist if cumulate effects becomes a subdiscipline. Much in the way that “climate science” and “sustainability” has enabled the development of shared language among physical, natural, and, increasingly, social scientists. Key to the development of these interdisciplinary fields was the growing awareness that no single discipline had sufficient tools to address the problem. Necessary steps were needed to recruit individuals from many disciplines into the field, and provide access to interdisciplinary professional meetings and societies for individuals to share information (Bettencourt and Kaur, 2011; Weart, 2013). In this way, shared frameworks and language could evolve.
An initial step is to better conceptualize the existing modes of cumulative effects assessment, so that those active in the discipline can better link their work to the broader whole. That is, a typology of existing research themes can identify pathways for inter-disciplinary work that is needed. Our typology is based on the areas of academic research in applied cumulative effects assessment focused at the species level: physiological, behavioral and demographic responses (Figure 3A). Similar silos typically exist at other ecological scales, such as with community or ecosystem-based research, however the species level provides a useful example here and the issues are broadly applicable. Additionally, silos exist between practitioners and researchers (Noble, 2010). Two areas we identify (Figure 3A) can broadly be described as research on ‘single end point' responses, whereby the impact of a stress is measured on a single biological process. Physiological responses are a common scale of analysis; they allow us to explore how exposure to one stressor affects the sensitivity two a second or third (Przeslawski et al., 2014) with respect to a single end-point (e.g., growth, survival). Alternatively, research might be concerned with behavioral responses, or other adaptation mechanisms, wherein attempts to avoid one stressor enhances exposure to a second stressor (Froehlich et al., 2014, 2017). Collectively, these two approaches illustrate the vulnerability of individuals or population demographic rates to multiple stressors. They cannot on their own address larger scale issues of population persistence and vulnerability. Meanwhile, other lines of research focus at the population scale, where the aim is to identify consequences of changing demographic rates on populations, and to identify when impacts of multiple stressors are magnified or mitigated (Power, 1997; Punt et al., 2015; Hodgson et al., 2017).
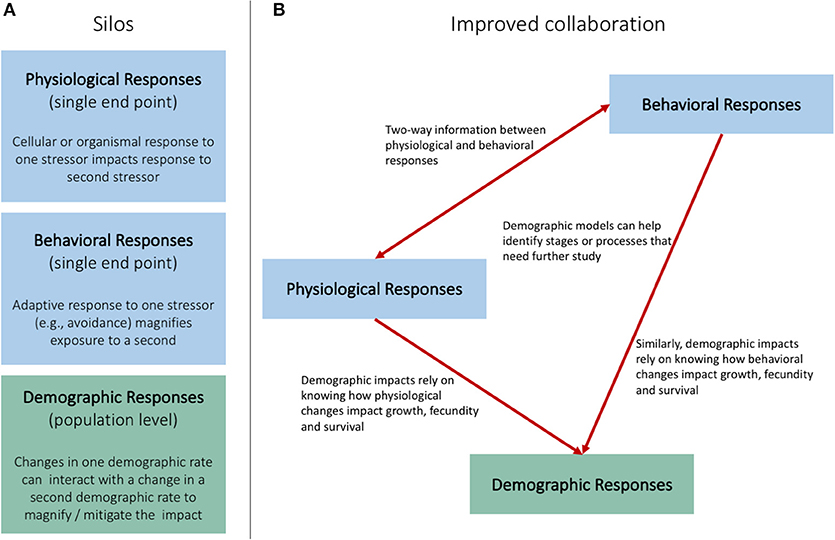
Figure 3. Conceptual diagram demonstrating an example of the “silos” of separate subdisciplines that study cumulative effects. (A) demonstrates each silo in isolation, where (B) addresses ways that each can be used to inform other areas of research.
Overall, research has been happening in each of these veins, but rarely across them. Moreover, use of the phrase “cumulative effects” is fundamentally distinct in each of these three branches. Physiological and behavioral methods approach cumulative effects with respect to organismal capacity to absorb impacts, while demographic methods deal with the population capacity to mitigate impacts from multiple stressors. We posit that a clearer terminology of what “cumulative effects” means is a clear initial step toward a holistic framework.
Further, the framework above also identifies clear ways for the silos to connect (Figure 3B). The physiological and behavioral approaches provide information on the extent of demographic response (e.g., survivorship, growth, fecundity), while population approaches can explore consequences of those changes for population persistence. Further, a population approach can identify critical demographic responses that govern population status, so that physiological and behavioral approaches can prioritize those responses. Similar inter-disciplinary approaches also apply to ecosystem scale impacts, where researchers focused on different parts of the same ecosystem could collaborate for an improved overall understanding.
This type of collaboration will be greatly enhanced if primary data and models are routinely made available as part of the publication process. Synthetic meta-analyses, which have proven pivotal in helping fields derive generalizations and heuristics, demand access to primary data or may be limited in the number of publications which can be included in the analysis. For example, Crain et al. (2008) performed a large statistical meta-analysis of interactive and cumulative impacts and had to dismiss roughly one quarter of the published findings because key data attributes were not reported. If they had access to raw data, they not only would have been able to include more data, but the range of suitable statistical analyses would have been much larger. Similarly, mathematical models that are made easy to explore by non-modelers will allow physiologists and other organismal biologists to better explore where experimental findings fit into a population-level understanding. This can in part be addressed through collaborations between experts across silos (e.g., Punt et al., 2015).
Conclusions
The challenges for humanity to sustainably live on the planet are becoming increasingly complex, creating a general need for more holistic approaches and systems-level thinking. Both the field of cumulative effects research and legislation surrounding impact assessment have come a long way. However, many challenges remain, as do opportunities for the field to better contribute to decision-making processes in the future. If we want to maintain the ecosystem services that humans rely on, scientists should continually ask themselves how they can contribute to solving these challenges. The priorities we identify are intended to improve the use and application of science in decision-making, while also fostering more rapid conceptual advances. We look forward to continued engagement with a diverse array of researchers active in this field, as only through working together will we be able to better tackle the challenges at hand.
Ethics Statement
The survey which was conducted and used to inform this review was approved by the Simon Fraser University Office of Research Ethics, study number: 2018s0419.
Author Contributions
All authors listed contributed to all steps in the development of this publication, from conceptualization through to writing and editing. All authors approve it for publication.
Funding
EH is funded by the Liber Ero Postdoctoral Fellowship Program.
Conflict of Interest Statement
The authors declare that the research was conducted in the absence of any commercial or financial relationships that could be construed as a potential conflict of interest.
Acknowledgments
Thank you to the experts who contributed by identifying particular areas where cumulative effects research has made advancements, and where there are remaining challenges and priorities. In alphabetical order, thank you to Sebastian Birk, Thorston Bleckner, Emily Darling, Melissa Foley, Rebecca Martone, Jay Piggott, Jennifer Provencher, Gerald Singh, Andy Stock, and two experts who chose to remain anonymous. Thank you as well to our two reviewers and the handling editor Samuel Cushman.
References
Astles, K. L., Holloway, M. G., Steffe, A., Green, M., Ganassin, C., and Gibbs, P. J. (2006). An ecological method for qualitative risk assessment and its use in the management of fisheries in New South Wales, Australia. Fish. Res. 82, 290–303. doi: 10.1016/j.fishres.2006.05.013
Aven, T., and Renn, O. (2009). On risk defined as an event where the outcome is uncertain. J. Risk Res. 12, 1–11. doi: 10.1080/13669870802488883
Bettencourt, L. M., and Kaur, J. (2011). Evolution and structure of sustainability science. Proc. Natl. Acad. Sci. U.S.A. 108, 19540–19545. doi: 10.1073/pnas.1102712108
Boyd, P. W., Collins, S., Dupont, S., Fabricius, K., Gattuso, J.-P., Havenhand, J., et al. (2018). Experimental strategies to assess the biological ramifications of multiple drivers of global ocean change—a review. Global Change Biol. 24, 2239–2261. doi: 10.1111/gcb.14102
Côté, I. M., Darling, E. S., and Brown, C. J. (2016). Interactions among ecosystem stressors and their importance in conservation. Proc. R. Soc. B Biol. Sci. 283:2592. doi: 10.1098/rspb.2015.2592
Canter, L., and Ross, B. (2010). State of practice of cumulative effects assessment and management: the good, the bad and the ugly. Impact Assessment Project Appraisal 28, 261–268. doi: 10.3152/146155110X12838715793200
Choi, B. C. K., and Pak, A. W. P. (2006). Multidisciplinarity, interdisciplinarity and transdisciplinarity in health research, services, education and policy: 1. definitions, objectives, and evidence of effectiveness. Clin. Investig. Med. 29, 351–364.
Cocklin, C., Parker, S., and Hay, J. (1992a). Notes on cumulative environmental change. I: concepts and issues. J. Environ. Manag. 35, 31–49. doi: 10.1016/S0301-4797(05)80126-4
Cocklin, C., Parker, S., and Hay, J. (1992b). Notes on cumulative environmental change II: a contribution to methodology. J. Environ. Manag. 35, 51–67. doi: 10.1016/S0301-4797(05)80127-6
Cooper, L. M., and Sheate, W. R. (2002). Cumulative effects assessment: a review of UK environmental impact statements. Environ. Impact Assessment Rev. 22, 415–439. doi: 10.1016/S0195-9255(02)00010-0
Crain, C. M., Kroeker, K., and Halpern, B. S. (2008). Interactive and cumulative effects of multiple human stressors in marine systems. Ecol. Lett. 11, 1304–1315. doi: 10.1111/j.1461-0248.2008.01253.x
Darling, E. S., and Côté, I. M. (2008). Quantifying the evidence for ecological synergies. Ecol. Lett. 11, 1278–1286. doi: 10.1111/j.1461-0248.2008.01243.x
Dubé, M. G. (2003). Cumulative effect assessment in Canada: a regional framework for aquatic ecosystems. Environ. Impact Assessment Rev. 23, 723–745. doi: 10.1016/S0195-9255(03)00113-6
Duinker, P. N., Burbidge, E. L., Boardley, S. R., and Greig, L. A. (2012). Scientific dimensions of cumulative effects assessment: toward improvements in guidance for practice. Environ. Rev. 21, 40–52. doi: 10.1139/er-2012-0035
Essington, T. E., Levin, P. S., Marshall, K. N., Koehn, L., Anderson, L. G., Bundy, A., et al. (2016). Building Effective Fishery Ecosystem Plans: A Report from the Lenfest Fishery Ecosystem Task Force. Washington, DC: Lenfest Ocean Program.
Fletcher, W. J. (2005). The application of qualitative risk assessment methodology to prioritize issues for fisheries management. ICES J. Mar. Sci. J. du Cons. 62, 1576–1587. doi: 10.1016/j.icesjms.2005.06.005
Fletcher, W. J. (2014). Review and refinement of an existing qualitative risk assessment method for application within an ecosystem-based management framework. ICES J. Mar. Sci. J. du Cons. 72, 1043–1056. doi: 10.1093/icesjms/fsu142
Foley, M. M., Mease, L. A., Martone, R. G., Prahler, E. E., Morrison, T. H., Murray, C. C., et al. (2017). The challenges and opportunities in cumulative effects assessment. Environ. Impact Assessment Rev. 62, 122–134. doi: 10.1016/j.eiar.2016.06.008
Froehlich, H., Essington, T., Beaudreau, A., and Levin, P. (2014). Movement patterns and distributional shifts of Dungeness crab (Metacarcinus magister) and English sole (Parophrys vetulus) during seasonal hypoxia. Estuar. Coasts 37, 449–460. doi: 10.1007/s12237-013-9676-2
Froehlich, H. E., Essington, T. E., and McDonald, P. S. (2017). When does hypoxia affect management performance of a fishery? A management strategy evaluation of Dungeness crab (Metacarcinus magister) fisheries in Hood Canal, Washington, USA. Can. J. Fish. Aquat. Sci. 74, 922–932. doi: 10.1139/cjfas-2016-0269
Goulson, D., Nicholls, E., Botías, C., and Rotheray, E. L. (2015). Bee declines driven by combined stress from parasites, pesticides, and lack of flowers. Science 347:1255957. doi: 10.1126/science.1255957
Greig, L. A., and Duinker, P. N. (2011). A proposal for further strengthening science in environmental impact assessment in Canada. Impact Assessment Project Appraisal 29, 159–165. doi: 10.3152/146155111X12913679730557
Griffith, G. P., and Fulton, E. A. (2014). New approaches to simulating the complex interaction effects of multiple human impacts on the marine environment. ICES J. Marine Sci. 71, 764–774. doi: 10.1093/icesjms/fst196
Griffith, G. P., Fulton, E. A., Gorton, R., and Richardson, A. J. (2012). Predicting interactions among fishing, ocean warming, and ocean acidification in a marine system with whole-ecosystem models. Conserv. Biol. 26, 1145–1152. doi: 10.1111/j.1523-1739.2012.01937.x
Haddock, M. (2018). Professional Reliance Review: The Final Report of the Review of Professional Reliance in Natural Resource Decision-Making.
Halpern, B. S., and Fujita, R. (2013). Assumptions, challenges, and future directions in cumulative impact analysis. Ecosphere 4:art131. doi: 10.1890/ES13-00181.1
Halpern, B. S., Walbridge, S., Selkoe, K. A., Kappel, V. C., Micheli, F., D'Agrosa, C., et al. (2008). A global map of human impact on marine ecosystems. Science 319, 948–952. doi: 10.1126/science.1149345
Hampton, S. E., Anderson, S. S., Bagby, S. C., Gries, C., Han, X., Hart, E. M., et al. (2015). The Tao of open science for ecology. Ecosphere 6:art120. doi: 10.1890/ES14-00402.1
Hampton, S. E., Strasser, C. A., Tewksbury, J. J., Gram, W. K., Budden, A. E., Batcheller, A. L., et al. (2013). Big data and the future of ecology. Front. Ecol. Environ. 11, 156–162. doi: 10.1890/120103
Hodgson, E. E., Essington, T. E., and Halpern, B. S. (2017). Density dependence governs when population responses to multiple stressors are magnified or mitigated. Ecology 98, 2673–2683. doi: 10.1002/ecy.1961
Hodgson, E. E., and Halpern, B. S. (2018). Investigating cumulative effects across ecological scales. Conserv. Biol. 33, 22–32. doi: 10.1111/cobi.13125
Hunsicker, M. E., Kappel, V. C., Selkoe, K. A., Halpern, B. S., Scarborough, C., Mease, L., et al. (2016). Characterizing driver–response relationships in marine pelagic ecosystems for improved ocean management. Ecol. Appl. 26, 651–663. doi: 10.1890/14-2200
ISO (2009). ISO 31000 Risk management-Principles and Guidelines. Geneva: International Organisation of Standards.
Kaplan, I. C., Levin, P. S., Burden, M., and Fulton, E. A. (2010). Fishing catch shares in the face of global change: a framework for integrating cumulative impacts and single species management. Can. J. Fish. Aquat. Sci. 67, 1968–1982. doi: 10.1139/F10-118
Maxwell, S. M., Hazen, E. L., Bograd, S. J., Halpern, B. S., Breed, G. A., Nickel, B., et al. (2013). Cumulative human impacts on marine predators. Nat. Commun. 4:2688. doi: 10.1038/ncomms3688
Munday, P., Crawley, N., and Nilsson, G. (2009). Interacting effects of elevated temperature and ocean acidification on the aerobic performance of coral reef fishes. Marine Ecol. Progr. Ser. 388, 235–242. doi: 10.3354/meps08137
Noble, B. F. (2010). Cumulative Environmental Effects and the Tyranny of Small Decisions: Towards Meaningful Cumulative Effects Assessment and Management. Page Natural Resources and Environmental Studies Institute Occasional Paper No. 8.
Porter, R. D., and Wiemeyer, S. N. (1969). Dieldrin and DDT: effects on sparrow hawk eggshells and reproduction. Science 165, 199–200. doi: 10.1126/science.165.3889.199
Power, M. (1997). Assessing the effects of environmental stressors on fish populations. Aquat. Toxicol. 39, 151–169. doi: 10.1016/S0166-445X(97)00020-9
Przeslawski, R., Byrne, M., and Mellin, C. (2014). A review and meta-analysis of the effects of multiple abiotic stressors on marine embryos and larvae. Global Change Biol. 21, 2122–2140. doi: 10.1111/gcb.12833
Punt, A. E., Foy, R. J., Dalton, M. G., Long, W. C., and Swiney, K. M. (2015). Effects of long-term exposure to ocean acidification conditions on future southern Tanner crab (Chionoecetes bairdi) fisheries management. ICES J. Marine Sci. 23:849–864. doi: 10.1093/icesjms/fsv205
Schindler, D. E., and Hilborn, R. (2015). Prediction, precaution, and policy under global change. Science 347, 953–954. doi: 10.1126/science.1261824
Selkoe, K. A., Blenckner, T., Caldwell, M. R., Crowder, L. B., Erickson, A. L., Essington, T. E., et al. (2015). Principles for managing marine ecosystems prone to tipping points. Ecosyst. Health Sustain. 1, 1–18. doi: 10.1890/EHS14-0024.1
Shopley, J. B., and Fuggle, R. F. (1984). Comprehensive review of current environmental impact assessment methods and techniques. J. Environ. Manag. 18:1.
Singh, G. G., Lerner, J., Mach, M., Clarke Murray, C., Ranieri, B., Peterson St-Laurent, G., et al. (2018). Scientific shortcomings in environmental impact statements internationally. PeerJ Prepr. 6:e27409v1. doi: 10.7287/peerj.preprints.27409v1
Smit, B., and Spaling, H. (1995). Methods for cumulative effects assessment. Environ. Impact Assessment Rev. 15, 81–106. doi: 10.1016/0195-9255(94)00027-X
Soranno, P. A., and Schimel, D. S. (2014). Macrosystems ecology: big data, big ecology. Front. Ecol. Environ. 12:3. doi: 10.1890/1540-9295-12.1.3
Stelzenmüller, V., Coll, M., Mazaris, A. D., Giakoumi, S., Katsanevakis, S., Portman, M. E., et al. (2018). A risk-based approach to cumulative effect assessments for marine management. Sci. Total Environ. 612, 1132–1140. doi: 10.1016/j.scitotenv.2017.08.289
Vitousek, P. M., Mooney, H. A., Lubchenco, J., and Melillo, J. M. (1997). Human domination of Earth's ecosystems. Science 277, 494–499. doi: 10.1126/science.277.5325.494
Vorosmarty, C. J., McIntyre, P. B., Gessner, M. O., Dudgeon, D., Prusevich, A., Green, P., et al. (2010). Global threats to human water security and river biodiversity. Nature 467, 555–561. doi: 10.1038/nature09440
Keywords: cumulative impacts, conservation, ecosystem-base management, resource management, prioritization
Citation: Hodgson EE, Halpern BS and Essington TE (2019) Moving Beyond Silos in Cumulative Effects Assessment. Front. Ecol. Evol. 7:211. doi: 10.3389/fevo.2019.00211
Received: 04 December 2018; Accepted: 21 May 2019;
Published: 11 June 2019.
Edited by:
Samuel A. Cushman, United States Forest Service (USDA), United StatesReviewed by:
Viorel Dan Popescu, Ohio University, United StatesAdrian Schiavini, National Council for Scientific and Technical Research (CONICET), Argentina
Copyright © 2019 Hodgson, Halpern and Essington. This is an open-access article distributed under the terms of the Creative Commons Attribution License (CC BY). The use, distribution or reproduction in other forums is permitted, provided the original author(s) and the copyright owner(s) are credited and that the original publication in this journal is cited, in accordance with accepted academic practice. No use, distribution or reproduction is permitted which does not comply with these terms.
*Correspondence: Emma E. Hodgson, emma.e.hodgson@gmail.com