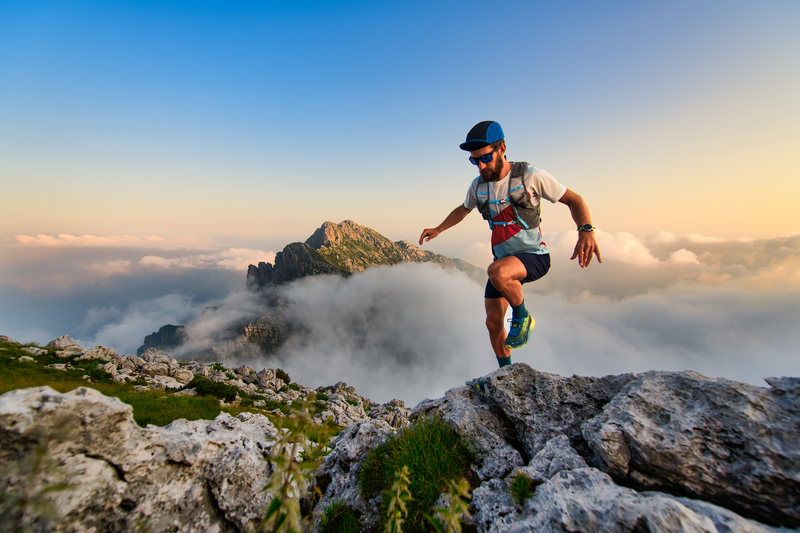
94% of researchers rate our articles as excellent or good
Learn more about the work of our research integrity team to safeguard the quality of each article we publish.
Find out more
REVIEW article
Front. Ecol. Evol. , 29 May 2019
Sec. Behavioral and Evolutionary Ecology
Volume 7 - 2019 | https://doi.org/10.3389/fevo.2019.00183
This article is part of the Research Topic Links Between Cognition and Fitness: Mechanisms and Constraints in the Wild View all 16 articles
Human-induced rapid environmental change (HIREC) poses threats to a variety of species, and if or how it changes phenotypes is a question of central importance bridging evolutionary ecology and conservation management. Social learning is one type of phenotypic plasticity that can shape organismal responses to HIREC; it allows organisms to acquire phenotypes on a timescale that closely tracks environmental change while minimizing the costs of individual learning. A common assumption in behavioral ecology, is that social learning is generally an adaptive way to cope with HIREC by facilitating the rapid spread of innovative responses to change. While this can be true, social learning can also be maladaptive. It may hinder the spread of adaptive behavior by causing a carryover of old, no longer adaptive behaviors that slow the response to HIREC or even promote the spread of maladaptive behaviors. Here, we present a conceptual framework outlining how an organism's evolutionary history can shape cognitive mechanisms, social behavior, and population composition, which in turn affect how an organism responds to HIREC. We review quantitative theory and empirical evidence spanning the cultural evolution and behavioral ecology literature discussing how social learning helps or hinders organismal or species' responses to HIREC. We highlight how mismatch of social learning mechanisms and time-lags in a post-HIREC environment can slow or limit the acquisition of adaptive behavior. We then discuss how different pathways of cultural transmission and social learning strategies can help or hinder responses to HIREC. We also review how HIREC may interfere with the transmission process by altering the public information sent from sender to receiver through the environment before receivers acquire any public information. Lastly, we discuss gaps and future directions including how animals integrate personal and social information, the interaction between personality and social learning, and social learning between heterospecifics.
All organisms must respond to the challenges created by human-induced rapid environmental change (HIREC) including novel enemies (Mack et al., 2000), novel resources (Marczak et al., 2007), habitat change, loss/fragmentation (Goudie, 2013), human harvesting (Mace and Reynolds, 2001), novel contaminants (Walker et al., 2014), and climate change (Walther et al., 2002). While organisms have always encountered environmental change, HIREC is of particular interest because it is often characterized by changes that occur quickly and are more extreme; they are of a greater magnitude, occur more frequently, and affect larger spatial scales. HIREC also brings about a high degree of novelty (Candolin and Wong, 2012). Essentially, HIREC is an extreme, rapid change from that which animals have experienced in their evolutionary history. How well and how rapidly organisms adjust to these changes is critically important for both individual fitness and species persistence (Candolin and Wong, 2012; Sih, 2013; Wong and Candolin, 2015). Consequently, there has been increasing interest in examples of evolutionary responses to HIREC (Singer et al., 1993; Skelly et al., 2007) which often involve adaptive behavioral responses (Sih et al., 2011; Sih, 2013; Wong and Candolin, 2015). Notably, an animal's ability to learn and adjust behaviors within its lifetime might serve as a crucial mechanism that allows it to rapidly adapt to HIREC situations. That is, even if animals exhibit maladaptive initial responses to HIREC, if they survive, they can potentially adjust behaviors via learning (Sih et al., 2011). These learned behaviors, in turn, may be important for affecting a species' post-HIREC evolutionary trajectories (Baldwin, 1896; Maynard Smith, 1987; Chevin et al., 2010).
Social learning can serve as a key process through which information and adaptive responses spread within populations (Whitehead, 2010). Following Heyes (1994), we define social learning as “learning that is influenced by observation of, or interaction with, another animal or its products.” This definition encompasses a variety of social learning processes where social factors influence the probability of acquiring public information (Danchin et al., 2004). Like individual learning, social learning is a type of phenotypic plasticity (West-Eberhard, 1989) that permits the acquisition of phenotypes on a timescale that more closely tracks environmental change, and with less of a time-lag, than genetic or epigenetic inheritance. Unlike individual learning, these phenotypes are inherited from others.
Quantitative models often assume that social learning has decreased costs and risks compared to individual learning; it outsources risk-taking and potentially costly mistakes to others (Boyd and Richerson, 1985; Aoki and Feldman, 2014). Cultural inheritance differs from genetic inheritance, as it occurs within an organism's lifetime and may utilize information from multiple demonstrators (or cultural parents), including genetically unrelated ones (Cavalli-Sforza and Feldman, 1981; Boyd and Richerson, 1985). Thus, the capacity for social learning to rapidly spread information and behaviors from a variety of demonstrators through a population makes it an important mechanism by which some species respond to rapid environmental change.
While there is compelling research on the ability of social learning to enhance responses to environmental change and its utility in conservation interventions (Whitehead et al., 2004; Whitehead, 2010; Greggor et al., 2016), our understanding of its potential to limit the spread of adaptive behavior in response to HIREC is lacking (Keith and Bull, 2017; Nieberding et al., 2018). The cultural evolution literature emphasizes the possibility that social learning leads to the spread of maladaptive behaviors or limits the spread of adaptive behaviors (Boyd and Richerson, 1985; Laland and Williams, 1998; Giraldeau et al., 2002). These limitations might be due to an over-reliance on social information after environmental change or the increased variance in fitness associated with social learning over individual learning. Social learning may also be maladaptive when an organism's social learning strategies, which evolved to allow individuals to cope with the volume and complexity of available social information in a population, lead to the acquisition of inaccurate information (Whitehead and Richerson, 2009). Additionally, social learning can result in maladaptive responses when offspring who rely on information from previous generations experience inertia, which hinders adaptive responses to change (Seppänen et al., 2007). This inertia, or time-lag, increases the likelihood that defunct information will be passed from demonstrators to observers, especially as the rate of environmental change increases (Rogers, 1988). Additionally, the interference of HIREC with the transmission of social information (i.e., the production, propagation, or detection of a signal) can result in a maladaptive response by an individual who is reliant on the disrupted signal (Patricelli and Blickley, 2006; Lürling and Scheffer, 2007; Ward et al., 2008). Broadly speaking, the overall benefit of social learning depends on the details of the social learning process, and how it is affected by HIREC.
Ultimately, whether a species' social learning response to HIREC is adaptive or maladaptive depends on its past environment (both within an individual's lifetime and on an evolutionary timescale), its current social learning pattern, and the type of HIREC it encounters. In this paper, we present a framework which connects these three factors to predict how social learning might facilitate or hinder organisms' adaptive responses to HIREC. Within this framework, we discuss the theory behind the evolution of social learning, the types of processes involved in social learning and the conditions under which particular types of social learning might make social learning maladaptive. These include the pathways of cultural transmission, or the generation from which an individual “inherits” the information, and social learning strategies, or the psychological mechanisms an individual uses to decide whom or what to copy. We also illustrate how HIREC might affect the propagation of social information through the environment from senders to receivers. Throughout the paper we present several HIREC scenarios and illustrate known examples, or potential examples, where social learning produces either adaptive or maladaptive responses to HIREC.
It may appear that the influence of social learning on individual responses to HIREC is well studied in behavioral ecology– particularly due to the pervasiveness of examples in popular culture like the spread of milk bottle-opening in blue tits (Hinde and Fisher, 1951). However, behavioral ecology lacks a cohesive framework for understanding when social learning facilitates adaptive responses to HIREC and when it results in maladaptive responses. Table 1 summarizes empirical examples of social learning under environmental change associated with HIREC; it is not meant to be comprehensive but is instead a collection of case studies that illustrate the breadth of social learning responses to HIREC.
Table 1. Empirical examples of how social learning interacts with HIREC for multiple taxa (mammals, birds, fish) and types of HIREC.
In addition to examples in which social learning is adaptive (Teitelbaum et al., 2016) and maladaptive (Szymanski and Afton, 2005) Table 1 includes instances in which HIREC disrupts social learning from occurring (Shannon et al., 2013). Numerous human-induced changes, including pervasive environmental conditions (e.g., ocean acidification Ferrari et al., 2012, eutrophication (Fischer and Frommen, 2013) and culling of individuals (Shannon et al., 2013) can lead to a reduction in the availability of useful or accurate social information. This occurs either because information is never transmitted or because individuals no longer receive information that is transmitted.
Table 1 includes both lab and field studies. It is important to note that studying social learning in the field is extremely challenging both logistically and analytically (McElreath et al., 2008; Kendal et al., 2009b; Reader and Biro, 2010). Thus, despite the importance of field studies in understanding how social learning interfaces with HIREC, many of the current examples come from lab-based studies. In some cases, captive research may more clearly illustrate the nuances of social learning dynamics in a changing world, where researchers are able to understand detailed mechanisms that underlie learning processes (e.g., Chivers et al., 2016). However, manipulations in captivity are not always specifically or feasibly matched to realistic HIREC scenarios. To better understand the complexities of social learning in a changing world, more field and lab-based research is needed to examine social learning in systems currently experiencing HIREC or under conditions which closely mimic HIREC.
We excluded studies that address social learning in response to novelty or other environmental conditions not specifically linked to HIREC from Table 1. However, these excluded studies can help us generate predictions for how social learning leads to adaptive and maladaptive responses to HIREC. Thus, we include many of these excluded studies throughout this paper. As HIREC is a pressing source of change that numerous species will have to contend with, more work looking at responses to HIREC are needed. For example, studying cultural transmission from an anthropological comparative approach has stimulated much research effort toward understanding culture in non-human primates relative to other mammals. However, many 20th century primate studies are criticized for lacking ecological validity (Custance et al., 2002). Additionally, while many insects (e.g., bees) do socially learn (reviewed in Grüter and Leadbeater, 2014), we found few empirical examples with direct relevance to HIREC.
In this section and in Figure 1 we present a framework for understanding the role of social learning in shaping individual, population or species level responses to HIREC. The specifics of an organism's social learning response begin with its evolutionary history (Figure 1A) where environmental traits, including the rate of environmental change, ease of innovation, benefits and costs of adaptive behavior, and social structure, shape three components of social learning: (1) the proportion of individual to social learners (Figure 1B), (2) social learning pathways (Figure 1C), and (3) social learning strategies (Figure 1D). HIREC may interact with these components, and make social learning a less adaptive strategy, by altering the environmental traits important to the efficacy of social learning mechanisms creating mismatches of previously adaptive learning mechanisms to a post-HIREC world or an increased time-lag to acquiring adaptive behavior (discussed in section 3.1.2). The effects of these mismatches and time-lags affect the frequency of individuals with adaptive behavior post-HIREC, q, (Figure 1F), which affects the rate of increase of adaptive behavior, dq/dt (Figure 1G). Prior to an animal acting upon social information and HIREC interacting with evolved social learning mechanisms, HIREC may also directly affect the transmission process (Figure 1E). It can interfere with the ability of the demonstrator to transmit social information, the propagation of social information through the environment, or the ability of a receiver to acquire and process transmitted information.
Figure 1. Role of social learning in a species' response to HIREC. A species' evolutionary history, and the environmental traits associated with it, (A) shapes a number of factors important to social learning to produce an adaptive or maladaptive response. These factors include the percent of individual and social learners in a population (B), the social learning pathways (C) and strategies (D) they exhibit, and the transmission of information between individuals (E). HIREC can influence percent of individuals exhibiting the correct behavior shortly after HIREC (F) by changing environmental traits important for social learning mechanisms affecting the efficacy of these mechanisms and ultimately altering the rate of increase in correct behavior (G). It can also impact the rate of increase by altering environmental traits which subsequently disrupts the information transmission process. HIREC disrupts these social learning traits by either creating a fitness mismatch (yellow, dotted boxes) between behaviors in the pre- and post-HIREC environments, by increasing the time-lag (blue, long dashed box), or a combination of the two (green, short dashed box).
To better understand how HIREC interacts with social learning, it is important to understand the conditions that favor the evolution of social learning and how learning relates to environmental change more generally. Learning, whether it is individual or social, is adaptive when environments change. A common intuition, particularly in behavioral ecology, is that social learning is adaptive simply because it saves organisms the costs or risks of individual learning. However, there are additional nuances to the evolution of social learning.
Individual learning is a form of phenotypic plasticity- it is often favored over fixed, innate behaviors when the environment changes (Stephens, 1991; Dukas, 2008). However, individual learning (also called “sampling" in the behavioral ecology literature) is assumed to come with some cost. These costs include time-costs and lost foraging opportunities (Stephens and Krebs, 1986) or increased predation risk (Sih, 1992; Griffin, 2004). Social learning and the utilization of public information is often assumed to be adaptive because it decreases the costs of individual learning by utilizing the experiences of others as proving grounds for whether a new behavior is adaptive. Thus, if the costs of individual learning relative to the benefits of adaptive behavior are sufficiently high, we might predict that social learning is likely to occur.
In addition to the cost of individual learning, innovating a novel solution can be very challenging. Innovations in wild populations are rather rare and many of them do not spread socially (Perry et al., 2017). There are also many “failed” innovations that must be tried before an adaptive one spreads (Miu et al., 2018) and social incentives (i.e., increased status in the eyes of others) might be important for their transmission (Arbilly and Laland, 2017). If adaptive innovation is rare, the evolution of social learning might be favored to propagate novel solutions.
A common intuition, particularly in behavioral ecology, is that social learning is adaptive simply because it saves organisms the above mentioned costs or risks of individual learning. However, there are additional nuances to the evolution of social learning. We explore these nuances with the aid of a development of one of the simplest and best-studied gene-culture coevolution models (Rogers, 1988). In contrast to Rogers' original model, this version assumes infinite environmental states and innovation error.
We use this model to discuss three factors that are widely thought to have an important role in affecting when or if social learning is advantageous relative to individual learning: (1) the cost, c, of individual learning relative to the benefit, b, of adaptive behavior, (2) the ease with which an adaptive behavior may be innovated, s, and (3) the rate of environmental change, u, in an organism's evolutionary history. These three factors affect how organisms respond to environmental change, and insights from this model can be used to explore the direct and indirect impact of these factors on responses to HIREC.
For simplicity we model two fixed phenotypes, social learners that exist at a frequency of p and individual learners that exist at a frequency of 1 − p. Generations barely overlap- adults live just long enough to transmit behavior to juveniles before dying. The environment can change states once in each generation with a probability of u. This change, if it happens, occurs after individuals have an opportunity to socially learn, but before they use their strategy to cope with the environment. Individual learners, conversely, do not inherit adaptive behaviors from the previous generation. Instead, upon encountering a stimulus they try to innovate a solution and generate an appropriate novel phenotype. What was adaptive in previous environmental states or whether the environment recently changed does not affect their behavior. Adaptive behavior adds to baseline fitness a benefit of b. Non-adaptive behavior has zero benefit, leaving individuals with their baseline fitness, w0.
Individual learning always has a cost, c, regardless of whether individuals innovate successful behavior. In addition to this cost, only s proportion of innovations, regardless of environmental state, are adaptive (i.e., increase fitness by b).
Assuming b > c the fitness of individual learners is:
Note that the fitness of individual learners is not affected by environmental change, nor does it change over time.
We assume social learners acquire their phenotype by randomly copying a member of the previous generation via oblique transmission. A proportion of behaviors Q copied from the previous generation are adaptive at time t. The fitness of social learners is a function of time and is determined by the frequency of adaptive behavior copied from the previous generation Qt multiplied by its fitness benefit, b:
When adaptive behavior is common (Qt is high), social learners can do well since most learn from other individuals that exhibit the adaptive behavior without paying the costs of innovation.
Qt, the frequency of adaptive behavior in the current timestep copied from the previous generation has its own recursive dynamics and it is embedded in the recursion above:
Each phenotype produces offspring proportional to the above fitness functions. If the environment does not change after social learning at time t (i.e., u = 0), social learners may acquire adaptive behavior from individuals in the previous generation that existed at a frequency of Qt−1. If the environment does change in the first time step (i.e., u = 1), then none of the behavior social learners acquired from the previous generation is adaptive. After an environmental change, only individual learners can innovate the new adaptive behavior. Thus immediately after environmental change, the frequency of adaptive behavior is proportional to (1 − p)s: the (proportion of individual learners in the population) × (the ease of adaptive behavioral innovation). However, by the next time step, juvenile social learners can learn the adaptive behavior from adult individual learners who successfully innovated immediately after change. If most individuals are social learners (i.e., individuals that do not innovate), then for some time after environmental change, most social learners continue to adopt the old, now maladaptive behavior, and thus do poorly compared to individual learners (Equation 2). This ‘inertia of tradition’ slows the population's response to HIREC (Figure 1G).
These dynamics are illustrated in more detail in Figure 2. In the first time step after each environmental change (before individual learners can innovate), the frequency of adaptive behavior drops to zero (Figures 2A,C in blue). Social learners thus learn the old, now maladaptive, behavior which decreases their fitness to baseline and decreases their frequency in the population relative to individual learners (Figures 2B,D in red). Each successive environmental change is marked by a number indicating the timestep and an arrow on the x-axis. Panels a and b show these dynamics in a relatively stable environment (u = 0.05), while c and d show dynamics in a more rapidly changing environment (u = 0.25). At the beginning of the simulation, the population is at , the steady state of the frequency of adaptive behaviors specified in Equation 4.
Figure 2. Dynamics of the frequency of social learners, p (a,c in red) and the frequency of adaptive behavior Q (b,d in blue) under a continuous states version of the Rogers' model with innovation error. (A,B) (white background) are in a relatively stable environment where u = 0.05; (C,D) (gray background) are in a less stable environment where u = 0.25. Arrows on x-axis indicate where the environment changes states, rendering socially learned behavior from the previous generation non-adaptive. In this simulation wo = 1, s = 0.8 , b = 3 and c = 1. Simulations were initialized with Qt = 1 = 0 and .
In the less stable environment, frequent environmental changes keep the frequency of social learners low (Figure 2C). However, because most of the population consists of individual learners who have a high chance (80% in this simulation) of innovating the new adaptive behavior, both the frequency of adaptive behavior and thus the fitness of social learners rebounds rapidly (Figure 2D). Because social learners avoid the cost of individual learning, they increase in prevalence. However, before the frequency of social learners can get high, the next environmental change occurs and knocks the proportion of social learners back down (Figure 2D).
In contrast, in relatively stable environments, if adaptive behavior has had time to become common (Figure 2B) social learners avoid the cost of individual learning while generally learning the adaptive behavior (from either individual learners or other social learners). As long as the environment does not change, social learners gradually increase in prevalence and after a long period of stability, may approach fixation (Figure 2A). However, after the next environmental change, if most individuals are social learners, only a few individual learners are present to innovate the new adaptive behavior. Social learners thus continue to adopt old, non-adaptive behaviors primarily from other social learners. The population then has a low dq/dt and exhibits low resilience following environmental change; in other words, it takes a long time for the frequency of adaptive behavior (Figure 2B, see, in particular, the lag in recovery after changes in time steps 25 and 80) and the fitness of social learners to rebound. During this recovery phase the frequency of social learners declines because the fitness of social learners is low. Still, as long as environmental change remains infrequent, social learners continue to stay common (Figure 2A) and this continues to reduce the population's resilience to environmental change.
Note that in a very stable environment, organisms can evolve to rely on adaptive, innate tendencies instead of learning (Stephens, 1991; McNamara et al., 2016) (e.g., on islands that have always lacked major predators, prey are often bold and do not readily learn about novel dangers). In contrast, low to intermediate rates of change tend to favor social learning while frequent, rapid change favors individual learning that allows for the rapid adoption of novel adaptive behaviors. This trend is a specific case of the conventional wisdom that organisms that have evolved with environmental change are more ready for HIREC.
Although ongoing environmental change produces a cycle of decline and recovery of social learners after each change, it is useful to solve for the long-term steady-state frequency of social learners, and the invasion conditions for social and individual learners as that may provide insight.
To solve for the genetic steady state of social learners, we may solve the simultaneous system of equations:
for and there is a unique solution for the equilibrium values (denoted by hats) of the frequency of social learners and adaptive behavior:
Examining the invasion dynamics by setting p ≈ 0 we find that social learners may invade a population of individual learners when W(I) > Ŵ(S) or:
Setting p ≈ 1, individual learners may invade a population of social learners when W(I) < Ŵ(S) or:
It is easier for social learners to invade if they are sufficiently rare relative to individual learners (when p is small). This is a consequence of social learner fitness decreasing as p increases, yielding similar information-parasitism dynamics as the producer-scrounger game (Barnard and Sibly, 1981). A broad parameter space favors a mix of individual and social learners. Additionally, a substantial number of maladaptive behavior and social learners can be maintained at equilibrium– particularly under a range of parameter conditions when both innovation is hard (i.e., lower s) and the rate of environmental change is moderate to high. Due to the risk of environmental change, a rare social learner is always better off in a group of individual learners whose frequency of adaptive behavior is unaffected by environmental change. This reduces the ‘inertia of tradition’ that affects social learners. This inertia is minimized in conditions that favor a lower – populations with fewer social learners on average. We might predict that organisms who evolved in ancestral conditions that favor a low might fare better in HIREC scenarions as they may more quickly recover immediately after change.
Intriguingly, Rogers (1988) showed that at the mixed ESS conditions of and , the mean fitness of social or individual learners is never higher than either pure equilibrium. This is also true when social learners selectively copy individual learners (Boyd and Richerson, 1995). This suggests that something other than simply saving the costs of individual learning is important for social learning to evolve. This observation is commonly referred to as “Rogers' Paradox.”
Since the illustration of Rogers' Paradox, theoreticians have found multiple situations where social learning can raise population mean fitness such as spatial heterogeneity (Aoki and Nakahashi, 2008; Rendell et al., 2010), or when animals use “social learning contingencies” or combine individual with social learning (Boyd and Richerson, 1995; Enquist et al., 2007; McElreath et al., 2018). Under certain conditions, learning biases (e.g., a tendency to learn from successful individuals) often provide particular contexts where social learning is adaptive compared to random copying (reviewed in Aoki and Feldman, 2014). The broad point is that the specifics of social learning can have major effects on the adaptive benefits of social learning.
A key element in predicting a species' or population's response to HIREC is understanding the organisms' evolutionary history and how it shapes their ability to innovate adaptive behavioral responses to the novel conditions post-HIREC (Figure 1A).
If only individual learners can innovate, populations that have evolved to rely heavily on social learning will generally have a poorer response immediately after HIREC (see Figure 2). Of course, even populations with numerous individual learners will only respond well to HIREC if individual learners have a high probability of successful innovation, s. With regard to s, some species are simply better at domain general problem solving (Deaner et al., 2006; Reader et al., 2011) and likely have a higher probability of innovating adaptive behavioral responses to novel situations. In addition, all else the same, species that have evolved in highly variable environments (in space or time) will have experienced a history of selection favoring the ability to innovate that should result in higher s. This is the case unless they have typically experienced situations that are so novel that the required new behaviors have been exceptionally difficult to innovate (sometimes referred to as innovative “leaps” Miu et al., 2018). Notably, species that have experienced highly variable conditions should also have evolved a high proportion of individual learners (Figure 2C, Equation 4). The proportion of individual to social learners also depends on the cost of individual learning. Foragers that evolved in areas with high predation regimes or herbivores who evolved in areas with many toxic plants might have high costs of individual learning or sampling and thus be more likely to use social learning.
Evolved life history, social systems, and demographics are also important for understanding how social learning may affect species' responses to HIREC. At a basic level, generational overlap is necessary to provide access to older individuals who are repositories of knowledge to cope with ecologically rare events. However, older individuals may also have outdated information if environments change within their lifetime. More detailed effects of social systems can come via effects on patterns of interaction (social networks) that influence social learning pathways (e.g., horizontal vs. vertical or oblique transmission) and social learning strategies (section 3.3). That is, the evolved social system and life histories affect how many demonstrators are available to copy and what cues are available to utilize in a social learning strategy (e.g., a tendency to learn from others based on their age, sex, relatedness, or rank).
Immediately after HIREC, individuals and populations can suffer substantial reductions in fitness if their formerly adaptive behaviors are now mismatched and yield substantially lower fitness benefits than the new, adaptive behavior. While it is possible that previously adaptive behaviors are exaptations that happen to match adaptive behaviors post-HIREC (Gould, 1991), we focus here on mismatches. If the mismatch is severe enough it can cause population declines and even extirpation (Whitehead and Richerson, 2009).
As noted above, social learning can underlie maladaptive population responses to HIREC. For instance, social information about high quality resource patches or social facilitation (e.g., conspecific attraction) often permits animals to efficiently locate habitats with abundant resources (Valone and Templeton, 2002). HIREC, however, may render the use of social information less beneficial or even riskier (see Table 1 for examples). This mismatch is often exploited by humans and is sometimes referred to as a “social trap." For example, hunters use spinning wing decoys which mimic the appearance and movement of their target bird, mallard ducks, to lure them to hunting sites (Szymanski and Afton, 2005).
At a population level, the fitness cost of mismatched behavior after HIREC is even larger if social learning contributes to a time-lag in the spread of adaptive behavior. The time-lag for adaptive behavior to spread depends not only on the frequency of social learners (as displayed in Equation 3 and Figure 2) but also on the population density, the species' life history (e.g., whether they have overlapping generations) and social system (e.g., group size and social networks), and what social learning strategy they employ (see section 3.2 for an example in rats). In order to display an adaptive social learning response and minimize time lag, animals must interact sufficiently frequently, exhibit key behaviors at the appropriate time, and have the capacity to adopt novel behavior at the time of their exposure (Beck and Galef, 1989; Pike and Laland, 2010; Slagsvold and Wiebe, 2011; Thornton and Clutton-Brock, 2011). Animals living in small social groups or with limited social interactions may be unlikely to observe adaptive behavior or be more prone to suffer from time-lag post-HIREC and fare poorly. Animals that learn from a broader pool of demonstrators might better respond to HIREC than those which might only learn from a subset of potential demonstrators in a population.
Social learning permits inheritance of behavioral phenotypes within and across generations from many cultural parents via different social learning pathways (Figure 1C) (Cavalli-Sforza and Feldman, 1981). Individuals may learn behavior from their biological parents, a process referred to as vertical transmission. They may also use oblique transmission, and copy behavioral phenotypes from all possible adults in the previous generation. Individuals may also acquire their behavior from cohort mates within their generation, a process known as horizontal transmission.
HIREC may favor oblique or horizontal transmission over vertical transmission. Vertical transmission is more prone to time-lag than oblique transmission, and therefore only evolves at low levels of environmental change. Oblique and horizontal transmission provide the advantage of better accessing individual learners, and they can more quickly respond to environmental change unless natural selection is strong. The ability of sampling a broader pool of individuals simply increases the odds of acquiring adaptive behavior over relying on gaining it from one or two parents. However when selection maximizes fertility, copying one's parents is more likely to be adaptive– a child's existence is an honest indicator that its parents were doing something right. Thus, the conditions where we would predict vertical transmission to evolve is in behavioral domains that affect fertility rather than survival, when the behavior's effective environment is stable, and when natural selection is strong (McElreath and Strimling, 2008). In the context of HIREC, species that have limited access to oblique transmission (i.e., those that only interact within family-unit social systems) might respond poorly to environmental change given our predictions.
The hypothesis that oblique transmission copes with novelty better than vertical transmission is supported by empirical work in rats. Juvenile rats socially learn about the location of food and what to eat by following adults to food sources. Different species, however, rely on different social learning pathways and strategies. Norway rats have a tendency to incorporate foods into their diet that they smell on other adults via oblique transmission; they are very hesitant to consume foods they do not smell on other rats. In contrast black rats have a very strong preference for vertical transmission (mother to offspring) during a critical period. While both species are globally widespread, Norway rats have replaced black rats in recent years in many anthropogenically altered landscapes through much of the world. This may be in part because the rapid change at which novel food sources have been introduced in recent years may favor a flexible learning system such as oblique transmission over vertical transmission (Chou et al., 2000; Richerson, 2019).
Horizontal transmission, or copying age-mates, might also be less prone to time-lag issues than oblique and vertical transmission. This is especially true if organisms have a sensitive window (Fawcett and Frankenhuis, 2015) for social information, and older generations are less likely to sample or update information about the environment, as has been empirically supported in several species (Aplin et al., 2017; Barrett et al., 2017).
While oblique and horizontal transmission are typically prone to less time-lag, vertical transmission may be adaptive when historical knowledge about habitat quality and movement are important. The use of older individuals as repositories of knowledge has evolved in many social species and if the benefit of the information held by those individuals has not changed in a post HIREC world, their removal can be detrimental to a population's response to HIREC. The danger of removing the older, keystone individuals (Modlmeier et al., 2014) is particularly prevalent in transmitting knowledge about migratory pathways. Experimental translocations and relocations of bighorn sheep show that populations that maintained older, more knowledgeable individuals migrated more consistently and were able to find better forage (Jesmer et al., 2018). Herring develop migratory patterns early in life based on the migratory behavior of older conspecifics. However, fishing typically targets older, larger individuals. After some herring fisheries were reduced to two percent of their original size, the number of spawning sites reduced dramatically making populations of herring increasingly vulnerable to possible disturbances at the remaining spawning sites (Corten, 2002). Thus, the reliance on older individuals through oblique transmission may force individuals into maladaptive spawning decisions as a consequence of targeted over-fishing.
Ultimately, there exists an inherent tension in the costs and benefits of horizontal, oblique and vertical transmission. Individuals who have survived their juvenile stage almost certainly have some adaptive behaviors, making them a repository of information for rare events that have occurred within their lifetime. However, when the environment is likely to change, horizontal transmission may be more adaptive than oblique or vertical transmission, especially if age-mates are likely to innovate or if adaptive behaviors differ across age classes.
Perhaps one way to successfully cope with HIREC is to facultatively switch between transmission pathways. Experimental studies suggest that captive Zebra finches who were treated with cortisol, a stress hormone, relied primarily on oblique transmission when acquiring social information about a foraging task. Untreated control juveniles instead relied primarily on vertical transmission (Farine et al., 2015b). Vertical transmission often outperforms oblique transmission in very stable environments, whereas oblique transmission is better when the environment is changing. Thus switching from vertical transmission to oblique transmission might be adaptive if stress is a reliable cue that the environment has changed (i.e., juveniles are stressed because their parents fed them poorly in a post-HIREC environment).
Which social learning pathway to rely on becomes increasingly complicated if individuals change their propensity to socially learn within their lifetime. Critical periods are limited time windows in an organism's life where they are capable of learning, while sensitive windows are periods where individuals are more likely to update information and show plasticity within their lifetime (Fawcett and Frankenhuis, 2015). The ability to learn may decrease over development due to neurological changes or priorities shifting away from information acquisition toward other activities such as reproduction. The most well studied example of critical periods and social learning is bird song. Passerines, parrots, and hummingbirds learn songs via oblique or vertical transmission as juveniles. They then practice and refine this song. Later in development, testosterone causes neurological changes where the song types crystallize (Marler et al., 1988) and many, but not all (Nottebohm et al., 1987), birds lose the cognitive machinery to socially learn songs. In several species diet preferences (Terkel, 1996; Chou et al., 2000) and extractive foraging behaviors (Tebbich et al., 2001) may only be socially learned during critical periods.
Species that have a critical period or sensitive window, might fare poorly compared to open-ended, completely plastic learners post-HIREC. Juveniles that learn socially primarily when young (with little later updating) are more likely to copy older adults with outdated behavior. These same adults are also incapable of switching to a new behavioral optimum post-HIREC. If the environment changes, and cues that were learned to be useful during the juvenile critical period become less adaptive or dangerous, species with sensitive windows may do poorly. Thus, animal groups that are mostly comprised of post-critical period individuals, as a result of high survivorship from juvenile stage to adulthood or longevity, may do poorly post-HIREC. (the proportion of correct individuals shortly after HIREC) will be low and dq/dt (the rate of increase of correct behavior) will be slow.
Unbiased social learning might be an unrealistic expectation for many organisms. Some individuals may not have access to all available information in a population for reasons unrelated to cognition (Coussi-Korbel and Fragaszy, 1995; Barrett, 2018). More importantly, many organisms have transmission biases (Boyd and Richerson, 1985) or social learning strategies (Laland, 2004) that can help them hone in on adaptive behavior.
Social learning strategies refer to the psychological heuristics (or cognitive shortcuts) individuals use to choose whom or what to copy from a sea of multiple potential demonstrators and stimuli (Boyd and Richerson, 1985; Henrich and McElreath, 2003; Laland, 2004). These heuristics often have a speed/accuracy trade-off. Certain biases might help individuals hone in on an optimal (or good enough) behavior or demonstrator(s) to copy immediately post-HIREC. However, they also may have other risks associated with them as previously adaptive cues used in heuristic decision-making may not be adaptive post-HIREC (Figure 1D).
Various factors affect the costs and benefits of different social learning strategies. Individuals often must choose whom to learn from among multiple demonstrators who may differ in utility across social and ecological contexts. Acquiring and processing all available social information, when possible, may also be costly or impossible. Information acquired from particular demonstrators likely varies in utility among individuals (i.e., juveniles may not benefit from learning behaviors adaptive to adults). However, heuristics are not always optimal, and particular HIREC scenarios may render previously adaptive heuristics maladaptive. Various taxonomies have been made of the variety of social learning strategies (Henrich and McElreath, 2003; Rendell et al., 2011; Kendal et al., 2018). Here, we discuss in detail the ones most applicable to HIREC.
Frequency-dependent learning refers to learning strategies where the probability of acquiring behavior depends upon its frequency in a population. Aside from random copying or unbiased learning, the most commonly studied variety of frequency-dependent learning is positive-frequency dependence– varieties of which include conformist transmission, conformity-biased learning, and majority-biased learning. [Note: we are not using conformity as defined by Asch (1956) where majority influence causes individuals to abandon accurate personal information; for debates and the history of terminology related to positive frequency dependence see Aplin et al. (2015a) and Van Leeuwen et al. (2015)].
Positive frequency-dependence is disproportionately copying the most common trait in a population, or more generally the use of some type of “consensus” information to inform behavioral choice (McElreath et al., 2013). This may be a function of copying the number of individuals or behaviors (Aplin et al., 2015a). Negative frequency-dependence is also possible, but less theoretically and empirically well explored (Vilhunen et al., 2005). Evidence consistent with positive frequency-dependence has been found in many species (Pike and Laland, 2010; van de Waal et al., 2013; Aplin et al., 2015b). The evolutionary rationale behind positive frequency-dependence is drawing on the wisdom of the crowd– arguably the most common behaviors are those that are beneficial to most individuals in the population. Otherwise, they would have been selected against.
Much theory, and some empirical work, suggests that positive frequency-dependence is a rapid means to acquire adaptive behavior and outperforms other learning strategies when adaptive behavior is common (Baldini, 2012, 2013; McElreath et al., 2013) and in spatially heterogeneous environments (Boyd and Richerson, 1985; Henrich and Boyd, 1998). However, under certain conditions conformist transmission may slow (Laland and Williams, 1998; Henrich, 2001) or prevent (Henrich and Boyd, 1998; Kendal et al., 2010) the spread of adaptive behavior. If the environment changes such that historically adaptive, common behaviors become maladaptive, a rare novel adaptive behavioral phenotype will be unable to spread through a population that consists of pure positive frequency-dependent social learners. Strong conformity may prevent any behavioral change and, essentially, has an infinite time-lag. If individuals undergoing change combine positive frequency-dependence with some other type of learning (i.e., individual learning), positive frequency-dependence may retard, but not prevent, the spread of new, adaptive behaviors.
Whitehead and Richerson (2009) show that conformist transmission can cause population collapse in realistic, temporally variable environments. For computational ease, analytical models typically assume disturbances resemble “white noise.” Environmental disturbances are drawn from a normal distribution where extreme events at the tail are rare and occur with constant magnitude and variance over time. This often produces predictable phenomenon. However, “red noise” scenarios may more closely match realistic HIREC scenarios. Red noise produces environmental changes that occur with increased magnitude (more difference from previous environmental conditions), with increased frequency (extreme, novel events are more common), and less predictably (non-linear dynamics and sudden shifts to more extreme steady states are possible as mean and variance are not constant) than white-noise scenarios of environmental change (Richerson, 2019). Red noise distributions are commonly found when evaluating patterns of spatial heterogeneity or climate fluctuations– two scenarios that are extremely relevant to HIREC. Results from this model show that under certain red noise conditions, species cannot cope with environmental change if they are purely conformist (or potentially rank-biased). Populations that are smaller, or isolated from other groups due to social or geographic factors might be more likely to collapse particularly if they have evolved in a relatively stable environment and rely on conformity-bias. Limited migration from outgroups and drift in small populations may accelerate the near fixation of maladaptive behavior.
In short time scales conformity-biased learning has been shown to spread maladaptive behaviors. Lab studies with guppies (Poecilia reticulata) show that maladaptive information about foraging site location could spread in the population through conformity-biased learning, preventing these lab populations from exploiting the optimal path (Laland and Williams, 1998; Kendal et al., 2004). Post HIREC, positive-frequency dependent learning strategies tend to cause a time-lag or prevent individuals from copying rare adaptive behaviors making it a seemingly risky learning strategy to employ under these circumstances.
Payoff-biased learning includes learning strategies that utilize observable behavioral correlates of fitness (e.g., yield or efficiency) or outcomes of fitness (i.e., fecundity) to inform what or whom to copy. These behaviors are also sometimes contained under the umbrella of “success-biases," which also include using likely correlates of fitness associated with demonstrators such as rank or prestige (Baldini, 2012) to inform whom to copy (further discussed in the section 3.3.3 on model biases). Individuals may copy the most successful (on average) behavior, also known as “compare-means success-biased” (Baldini, 2013) or “pay-off-biased" learning (McElreath et al., 2008; Kendal et al., 2009a). Individuals may also copy the behaviors of the demonstrator with the best observable payoffs in a population (Baldini, 2013). Compare-means success-bias performs well when high-payoff behaviors are rare (Baldini, 2013), whereas “imitate the best" under performs when lower pay-off behaviors occasionally yield a high payoff due to stochasticity. Evidence consistent with success-biased learning has been found in one fish (Pike et al., 2010) and several primate species (Barrett et al., 2017; Vale et al., 2017; Bono et al., 2018).
If HIREC has directly observable fitness consequences like reducing foraging success or increasing opportunities for predation, evaluating the adaptive content of a behavior via pay-off biased learning is an excellent strategy for coping with HIREC. However, evaluating the content of seemingly successful behaviors or individuals might yield false positives because of stochasticity post-HIREC; we might predict this to be a problem when HIREC also decimates population size or the ability for individuals to acquire information from conspecifics. However, when successful behavior is particularly rare (Baldini, 2013), copying the single most successful individual might be the best strategy.
Payoff-biased strategies appears to be underutilized in nature (Mesoudi, 2011), likely because evaluating the content of a behavior may be costly or impossible. If the inherent meaning of behaviors is not understood (i.e., bird song or displays used to attract mates) or is computationally costly, it may be adaptive to bias attention toward particular demonstrators or “models,” who display cues (i.e., rank, health, fertility) that are likely to be correlated with adaptive behavior. Other times these cues may be indicative of phenotypic matching of socially learned behaviors (i.e., relatedness, age similarity, sex similarity). Additionally, when observable pay-offs are stochastic, then cues are sometimes a better proxy. Many of these cues, such as rank, abundance in a population, success of individuals, or mating success, are often coupled with fitness enhancing behaviors. Evolution might favor the use of particular social learning strategies that hone in on these cues if these cues are reliable over the course of evolutionary history.
HIREC may render model-biased learning maladaptive if it creates a mismatch by decoupling previously reliable cues from their fitness consequences, turning them from honest to dishonest signals. This can lead organisms into ecological traps (Schlaepfer et al., 2002), which will either cause some time-lag or entirely prevent an organism from switching to adaptive behavior. Additionally, behaviors that are socially learned, have high fitness consequences, and/or are only performed by individuals once (i.e., some mating or predation events) in their lifetime may be potentially prone to HIREC. For example, natural selection may favor the evolution of a learning strategy where an individual copies the oviposition or nestmaking site that was most common or chosen by the most fecund member of a population. If this decision is no longer adaptive post-HIREC, evolved social learning rules may become maladaptive unless organisms have some additional cognitive equipment or learned experience to bail them out.
Kin-biased learning (which also includes vertical transmission discussed in section 3.2) is one type of model bias. Kin-biased learning is common in many primates (Perry, 2009; Wrangham et al., 2016; Lamon et al., 2017) and carnivores (Mazur and Seher, 2008; Müller and Cant, 2010; Thornton and Clutton-Brock, 2011). In some cases, however, it is unclear whether this is a consequence of family-unit social systems or kin-biased social interactions rather than strategic social learning (Laland, 2004). In some species, kin-biases may drastically reduce the variety of social behaviors that an individual may acquire post-HIREC compared to social learning strategies that have them exploring outside of their family groups.
Furthermore, copying individuals that have indicators of success, such as rank, reproductive status, or physical condition may have longer time-lags than other social learning strategies if these cues are not equally relevant in the pre- and post-HIREC world. These indicators presumably take some time to be lost post-HIREC if they were reliable in the pre-HIREC world. Generally, we might predict that using social learning strategies which rely on a greater number of individuals may have a greater time-lag post-HIREC. However there exists an inherent tension; socially learning from multiple individuals reduces stochasticity due to sampling effects. Watching a seemingly successful behavior or individual might yield false results because of stochasticity, but can be adaptive when successful behavior is particularly rare (Baldini, 2013).
Content- or direct-biased learning, is when individuals have genetic predispositions to acquire or attend to social learning about particular stimuli (Boyd and Richerson, 1985). This genetic predisposition is also referred to as ‘evolutionary preparedness’ (Seligman, 1971; Davey, 1995; Lindström et al., 2016). This may occur because natural selection biases organisms to attend to social information or copy behavior about evolutionary stable or important cues like predators (Galef and Laland, 2005) or mate choice (Nöbel et al., 2018). Examples of direct-bias include food taboos and dietary preferences (Fessler and Navarrete, 2003; Henrich and Henrich, 2010) and preferential retention of information about dangerous animals (Broesch et al., 2014). Evolutionary simulations suggest that preparedness and social learning can coevolve in dangerous and stochastic environments. This coevolution may lead to suboptimal appearing behavior, although this is likely due to a tradeoff between flexibility and safety shaped by an organism's evolutionary history (Lindström et al., 2016). If HIREC reduces the reliability of ancestrally reliable cues upon which content-biases are based, this may strongly influence individuals to acquire outdated social information or choose a suboptimal behavior– a type of socially learned ecological trap (Schlaepfer et al., 2002).
For much of this paper we have discussed social learning as a stand-alone mechanism for gathering information about the environment. In reality animals likely integrate social and individual information, a family of learning strategies known as social learning contingencies. Surprisingly, the interplay between social and individual learning in wild populations is not thoroughly explored, despite models suggesting that the integration of personal and social learning is what renders social learning adaptive over any fixed strategy (Boyd and Richerson, 1995; Enquist et al., 2007; Aoki and Feldman, 2014). Adaptive plasticity in learning strategies, where individuals switch from social to individual learning if they suspect the environment is changing was also common among the best strategies in a recent computer simulation based social learning strategies tournament (Fogarty et al., 2012).
The interplay between social and individual learning is subtle and takes several forms. Oftentimes social information is used to explore behaviors, while individual information is used to settle on behavior regarding the incorporation of novel diet items (Galef and Whiskin, 2001) and changing foraging behaviors to maximize yields (McElreath et al., 2005; Barrett et al., 2017). Social learning can also reverse earlier individual preferences, even if that socially acquired information is contradictory to individually acquired information. Norway rats that were taught an aversion to a food item then increased their intake of that food after interacting with a demonstrator rat that had consumed the aversive item (Galef et al., 1997). Later work showed that rats who learned that a food item was toxic or unpalatable ignored their personal knowledge and consumed an unpalatable or potentially toxic food item after interacting with a rat that had eaten that lower quality food (Galef and Whiskin, 2008). Similar experiments have demonstrated a social component to reverse feeding aversions in captive spotted hyenas (Yoerg, 1991) for previously learned unpalatable novel foods. Thus social learning might be an important mechanism for changing initial impressions– a type of reversal learning. However we need future studies and theory to understand the adaptive significance and limitations of this in the face of HIREC, as sometimes an over-reliance on one type of information over the other could lead to maladaptive decision making (Avarguès-Weber et al., 2018).
In addition to altering the value of social information, HIREC can impair the transmission of information necessary for social learning to take place. For social learning to occur, a demonstrator must send information in the form of signals or cues through the environment to a receiver (Maynard Smith and Harper, 2003). This process has been shaped over evolutionary time, so when HIREC increases the mismatch between a population's current and ancestral environment, communication may be disrupted. Most research examining the maladaptive effects of social learning assume that the learner has successfully received a signal (Rieucau and Giraldeau, 2011). In reality, HIREC may disrupt the flow of information before it reaches the potential learner.
The transmission of information can be broken down into three phases: 1) production of information by the sender, 2) transmission through the environment and 3) detection by the receiver. HIREC may interfere with any of these phases. The production of signals by demonstrators can be impacted by anthropogenic activities in two main ways. First, HIREC often results in declining population or group size. This is particularly true with anthropogenic disturbances that target specific keystone individuals (Modlmeier et al., 2014) in a population. For instance, human harvesting that targets the largest, oldest, and most knowledgeable animals in a population can lead to a decrease in high quality demonstrators (Shannon et al., 2013). When social information is beneficial, HIREC can cause an information-based Allee effect, where a decrease in population size reduces the availability of useful social information that further exacerbates population decline (Gil et al., 2018). Second, HIREC can reduce information production per demonstrator. For example, high temperatures and low food availability decrease the ability of damselfish to produce chemical alarm cues (Lienart et al., 2016). Because food availability and climate are tightly linked, this has the potential to impact species that rely heavily on conspecific chemical alarm cues to learn about predatory threats.
Environmental conditions resulting from HIREC can also interfere with the propagation of signals. This is especially common in aquatic environments where conditions like low pH and turbidity impair chemical and visual signals from traveling between individuals (Semel and Sherman, 2001; Brown et al., 2002; Leduc et al., 2004; Fischer and Frommen, 2013). Signal propagation is also negatively affected by HIREC for many plants, as volatile cues transmitted between individuals are degraded by pollution, thus reducing their reliability (Blande et al., 2014). As diesel pollution affects the ability of honey bees to use chemical cues to locate nectar resources (Lusebrink et al., 2015), it is possible that olfactory signals transmitted between organisms through the air are also affected by atmospheric pollutants introduced by HIREC.
Anthropogenic activities can also inhibit the detection of signals by observers. This phenomenon has been studied extensively near urban areas and roads where noise pollution masks auditory signals (reviewed in Patricelli and Blickley, 2006). For instance, Parris and Schneider (2009) compared the effects of traffic noise on two species of bird: the grey-shrike thrush and the grey fantail. The thrush calls with a lower sound frequency than the fantail. Thus, with increased low frequency traffic noise, the thrush had to shift to singing at a higher frequency but the fantail was not disturbed. This underscores another important point: the degree that HIREC interferes with transmission depends on the mismatch between the pre- and post-HIREC environment. Still, at sites with high traffic noise the probability of detecting either bird decreased. Thus the magnitude of change is also important. Even if the noise is loud animals may be able to shift the timing of their calling to compensate for the increased noise if it only occurs during a specific temporal window (Fuller et al., 2007).
Importantly, HIREC often includes simultaneous changes on multiple aspects of the environment, which may limit the ability of animals to transmit information successfully in all three phases of the transmission process. Ultimately, an effective transmission process is necessary for a social learning response. If animals cannot effectively communicate to one another, then whether social learning is an adaptive or maladaptive response may be a moot point as it may be significantly reduced in a post-HIREC world.
For social learning to be an avenue for organisms to escape HIREC, there must be observable variance in fitness of information post-HIREC. To illustrate, imagine a novel pollutant is introduced to a stream that reduces reaction time of an aquatic critter to a predator. This species may notice that conspecifics upstream (where concentrations of the novel pollutant are lower) appear to be in a better state; they may use that social information to decide to move upstream. Now, imagine that the same pollutant is introduced to a pond, such that it exists in equal concentrations around the whole pond and equally affects all individuals. Individuals may evaluate their state compared to conspecifics, note that it is similar, and thus not have access to any social information that would allow them to escape this dangerous HIREC scenario. Thus types of HIREC that harm all individuals in a population equally may be particularly harmful, as they limit the utility of social learning as an escape. In such contexts, memory of conditions pre-HIREC might be the only source of variance that could inform them to leave their local patch and seek out better conditions.
Social learning is a complex phenomenon whose evolution and efficacy are influenced by a multitude of factors. Social learning allows individuals to gather information about their environment while avoiding the costs of sampling it directly through individual learning. However the ease of innovation, rate of environmental change, and proportion of individual to social learners are also important in maintaining the fitness of social learners in a given population. While models examining the evolution and prevalence of social learning have focused on these social learning traits, it is important to note that they often exclude the ability of individuals to integrate individual and social information, a scenario which likely occurs frequently in nature.
We provide a framework for understanding the expression of adaptive and maladaptive responses to HIREC. It demonstrates that a species' evolutionary history shapes components of social learning important in producing a response to HIREC. These components include the proportion of individual to social learners in a population, social learning pathways, social learning strategies and the information transmission process. These social learning traits are important in determining the proportion of individuals exhibiting the correct behavior shortly after HIREC and the rate of increase of correct behavior in a population, both of which determine the long-term social learning response to HIREC. HIREC can disrupt social learning by changing environmental traits important for the components of social learning, creating a mismatch or a time-lag.
We close by noting several avenues for expanding the analysis of effects of social learning on responses to HIREC. First, while we focused on social vs. individual learning as an isolated trait, social learning tendencies and strategies might usually be correlated with other phenotypic traits (e.g., age, size, condition, rank, or social network position). How social learning affects individual and population responses to HIREC might then be mediated by the correlation with these other traits. In particular, a developing literature examines how learning tendencies (social and individual) are associated with personality traits such as boldness, exploratory tendency or neophobia [(Sih and Del Giudice, 2012; Aplin et al., 2014; Carter et al., 2014; Trompf and Brown, 2014; Griffin et al., 2015); but see Morand-Ferron et al. (2015)]. If, for example, bold individuals tend to be individual learners (willing to expose themselves to risks to learn about novel options), this could result in high mortality of individual learners in the face of novel predators, making it particularly difficult for an adaptive response to these novel predators to spread. In contrast, if individual learners are cautious, they might be more likely to survive encounters with novel predators and then potentially transmit the information to social learners.
Second, we focused on learning from conspecifics, but social learning from heterospecifics also occurs (Dawson and Chittka, 2012; Gil et al., 2018) as has been historically shown for public information like predator risk (Seyfarth et al., 1980; Ito and Mori, 2010) and food patch quality and location (Parejo et al., 2005; Farine et al., 2015a). If social learning is beneficial, then heterospecifics that share information can be information mutualists (Gil et al., 2018), whereas if it is costly, then heterospecifics can contribute to cross-species social traps. Many of the points that we discussed about conspecific social learning can also apply for cross-species social learning (e.g., a key component could be heterospecific learning strategies, or which species and which members of other species one learns from). An intriguing idea is the possibility of keystone information providers - species that numerous other species learn from (Farine et al., 2015a; Gil et al., 2018). In general, a future area of study is what can be termed the ‘community ecology’ of social learning.
In addition, we assumed that any innovative behavior that provided an increase in fitness for social learners would benefit a population that utilizes social learning. However, effective innovation and its cultural transmission could result in over exploitation of resources and might lead to eventual population decline, a potential consequence which requires further research. While this topic is discussed in research integrating cultural evolution and sustainability science in humans (Kline et al., 2018), it may be a risk to some animals using social learning to cope with HIREC. Long tailed macaques living in archipelagos off the coast of Thailand rely on stone tools to open bivalves and other resources on resource limited islands– behaviors that are likely culturally transmitted. There is evidence that tool-users at sufficient population densities drive down both population size and composition, removing larger shellfish from the populations. Researchers estimate that given sufficient time, tool-aided foraging might no longer benefit these populations and might in fact further threaten their persistence (Luncz et al., 2017).
Finally, we focused our analysis on the initial, relatively short term response to environmental change. On longer time scales, by affecting fitness, the behavioral response to HIREC affects population dynamics. Social learning that slows responses to HIREC or even result in social traps can contribute to population decline (Hale et al., 2015). In contrast, when social learning spreads adaptive behavior quickly, this can not only rescue populations, it can result in population ‘booms’ (e.g., urbanized or invasive pests) that can be followed by overexploitation and subsequent ‘busts’. And, in the even longer-term, post-HIREC, social learning tendencies and strategies might evolve and become a component of evolutionary rescue (Gonzalez et al., 2013). More empirical and modeling work on consequences of social learning for population (or even multi-species) dynamics, and for eco-evolutionary dynamics should be valuable and exciting.
All authors contributed to the formulation, writing, and revision of this manuscript and made direct intellectual contributions. They all approve of its publication.
BB was supported by the NSF (IOS 1456724 grant to AS) and via funding from the Max Planck Institute. EZ was supported by the NSF Bridge to the Doctorate Graduate Fellowship Program (1612490). This research was also written during the tenure of the NSF Graduate Research Fellowship awarded to LP (1612490).
The authors declare that the research was conducted in the absence of any commercial or financial relationships that could be construed as a potential conflict of interest.
This paper benefited from useful conversations and feedback from Pete Trimmer, Pete Richerson, Damien Farine, Lucy Aplin, and the Cognitive and Cultural Ecology Lab Group at the Max Planck Institute for Ornithology. Thank you to Simon Ducatez and Daizaburo Shizuka for their useful and thoughtful reviews.
Allen, J., Weinrich, M., Hoppitt, W., and Rendell, L. (2013). Network-based diffusion analysis reveals cultural transmission of lobtail feeding in humpback whales. Science 340, 485–488. doi: 10.1126/science.1231976
Aoki, K., and Feldman, M. W. (2014). Evolution of learning strategies in temporally and spatially variable environments: a review of theory. Theoret. Pop. Biol. 91, 3–19. doi: 10.1016/j.tpb.2013.10.004
Aoki, K., and Nakahashi, W. (2008). Evolution of learning in subdivided populations that occupy environmentally heterogeneous sites. Theor. Pop. Biol. 74, 356–368. doi: 10.1016/j.tpb.2008.09.006
Aplin, L. M., Farine, D. R., Mann, R. P., and Sheldon, B. C. (2014). Individual-level personality influences social foraging and collective behaviour in wild birds. Proc. R. Soc. Lond. B Biol. Sci. 281:20141016. doi: 10.1098/rspb.2014.1016
Aplin, L. M., Farine, D. R., Morand-Ferron, J., Cockburn, A., Thornton, A., and Sheldon, B. C. (2015a). Counting conformity: evaluating the units of information in frequency-dependent social learning. Anim. Behav. 110, e5–e8. doi: 10.1016/j.anbehav.2015.09.015
Aplin, L. M., Farine, D. R., Morand-Ferron, J., Cockburn, A., Thornton, A., and Sheldon, B. C. (2015b). Experimentally induced innovations lead to persistent culture via conformity in wild birds. Nature 518, 538–541. doi: 10.1038/nature13998
Aplin, L. M., Sheldon, B. C., and McElreath, R. (2017). Conformity does not perpetuate suboptimal traditions in a wild population of songbirds. Proc. Natl. Acad. Sci. U.S.A. 114, 7830–7837. doi: 10.1073/pnas.1621067114
Arbilly, M., and Laland, K. N. (2017). The magnitude of innovation and its evolution in social animals. Proc. R. Soc. Lond. B Biol. Sci. 284:1848. doi: 10.1098/rspb.2016.2385
Asch, S. E. (1956). Studies of independence and conformity: I. a minority of one against a unanimous majority. Psychol. Monogr. Gener. Appl. 70:1. doi: 10.1037/h0093718
Avarguès-Weber, A., Lachlan, R., and Chittka, L. (2018). Bumblebee social learning can lead to suboptimal foraging choices. Anim. Behav. 135, 209–214. doi: 10.1016/j.anbehav.2017.11.022
Baldini, R. (2012). Success-biased social learning: cultural and evolutionary dynamics. Theor. Pop. Biol. 82, 222–228. doi: 10.1016/j.tpb.2012.06.005
Baldini, R. (2013). Two success-biased social learning strategies. Theor. Pop. Biol. 86, 43–49. doi: 10.1016/j.tpb.2013.03.005
Barnard, C. J., and Sibly, R. M. (1981). Producers and scroungers: a general model and its application to captive flocks of house sparrows. Anim. Behav. 29, 543–550. doi: 10.1016/S0003-3472(81)80117-0
Barrett, B. J. (2018). Equifinality in empirical studies of cultural transmission. Behav. Process. 161, 129–138. doi: 10.1016/j.beproc.2018.01.011
Barrett, B. J., McElreath, R. L., and Perry, S. E. (2017). Pay-off-biased social learning underlies the diffusion of novel extractive foraging traditions in a wild primate. Proc. R. Soc. B 284:20170358. doi: 10.1098/rspb.2017.0358
Beck, M., and Galef, B. G. (1989). Social influences on the selection of a protein-sufficient diet by norway rats (rattus norvegicus). J. Compar. Psychol. 103:132. doi: 10.1037/0735-7036.103.2.132
Blande, J. D., Holopainen, J. K., and Niinemets, Ü. (2014). Plant volatiles in polluted atmospheres: stress responses and signal degradation. Plant Cell Environ. 37, 1892–1904. doi: 10.1111/pce.12352
Bono, A. E., Whiten, A., van Schaik, C., Kratzen, M., Eichenberger, F., Schnider, A., et al. (2018). Payoff- and sex-biased social learning interact in a wild primate population. Curr. Biol. 28, 2800–2805.e4. doi: 10.1016/j.cub.2018.06.015
Boyd, R., and Richerson, P. J. (1985). Culture and the Evolutionary Process. Chicago, IL: University of Chicago press.
Boyd, R., and Richerson, P. J. (1995). Why does culture increase human adaptability? Ethol. Sociobiol. 16, 125–143. doi: 10.1016/0162-3095(94)00073-G
Broesch, J., Barrett, H. C., and Henrich, J. (2014). Adaptive content biases in learning about animals across the life course. Hum. Nat. 25, 181–199. doi: 10.1007/s12110-014-9196-1
Brown, G. E., Adrian, J. C.Jr, Lewis, M. G., and Tower, J. M. (2002). The effects of reduced ph on chemical alarm signalling in ostariophysan fishes. Can. J. Fish. Aquat. Sci. 59, 1331–1338. doi: 10.1139/f02-104
Candolin, U., and Wong, B. B. (2012). Behavioural Responses to a Changing World: Mechanisms and Consequences. Oxford: OUP Oxford.
Carter, A. J., Marshall, H. H., Heinsohn, R., and Cowlishaw, G. (2014). Personality predicts the propensity for social learning in a wild primate. PeerJ 2:e283. doi: 10.7717/peerj.283
Cavalli-Sforza, L. L., and Feldman, M. W. (1981). Cultural Transmission and Evolution: A Quantitative Approach. Princeton, NJ: Princeton University Press.
Chevin, L.-M., Lande, R., and Mace, G. M. (2010). Adaptation, plasticity, and extinction in a changing environment: towards a predictive theory. PLoS Biol. 8:e1000357. doi: 10.1371/journal.pbio.1000357
Chivers, D. P., McCormick, M. I., Allan, B. J., and Ferrari, M. C. (2016). Risk assessment and predator learning in a changing world: understanding the impacts of coral reef degradation. Sci. Rep. 6:32542. doi: 10.1038/srep32542
Chou, L.-S., Marsh, R. E., and Richerson, P. J. (2000). Constraints on social transmission of food selection by roof rats, rattus rattus. Acta Zool. Taiwan. 11, 95–109. doi: 10.6576/AZT.2000.11.(2).3
Corten, A. (2002). The role of“conservatism”in herring migrations. Rev. Fish Biol. Fish. 11, 339–361. doi: 10.1023/A:1021347630813
Coussi-Korbel, S., and Fragaszy, D. M. (1995). On the relation between social dynamics and social learning. Anim. Behav. 50, 1441–1453. doi: 10.1016/0003-3472(95)80001-8
Custance, D. M., Whiten, A., and Fredman, T. (2002). Social learning and primate reintroduction. Int. J. Primatol. 23, 479–499. doi: 10.1023/A:1014961415219
Danchin, É., Giraldeau, L.-A., Valone, T. J., and Wagner, R. H. (2004). Public information: From nosy neighbors to cultural evolution. Science 305, 487–491. doi: 10.1126/science.1098254
Davey, G. C. (1995). Preparedness and phobias: specific evolved associations or a generalized expectancy bias? Behav. Brain Sci. 18, 289–297. doi: 10.1017/S0140525X00038498
Dawson, E. H., and Chittka, L. (2012). Conspecific and heterospecific information use in bumblebees. PLoS ONE 7:e31444. doi: 10.1371/journal.pone.0031444
Deaner, R. O., van Schaik, C. P., and Johnson, V. (2006). Do some taxa have better domain-general cognition than others? a meta-analysis of nonhuman primate studies. Evol. Psychol. 4:147470490600400114. doi: 10.1177/147470490600400114
Ducatez, S., Audet, J., and Lefebvre, L. (2013). Independent appearance of an innovative feeding behaviour in antillean bullfinches. Anim. Cognit. 16, 525–529. doi: 10.1007/s10071-013-0612-4
Dukas, R. (2008). Evolutionary biology of insect learning. Ann. Rev. Entomol. 53, 145–160. doi: 10.1146/annurev.ento.53.103106.093343
Enquist, M., Eriksson, K., and Ghirlanda, S. (2007). Critical social learning: a solution to rogers's paradox of nonadaptive culture. Am. Anthropol. 109, 727–734. doi: 10.1525/aa.2007.109.4.727
Farine, D. R., Aplin, L. M., Sheldon, B. C., and Hoppitt, W. (2015a). Interspecific social networks promote information transmission in wild songbirds. Proc. R. Soc. Lond. B Biol. Sci. 282:20142804. doi: 10.1098/rspb.2014.2804
Farine, D. R., Spencer, K. A., and Boogert, N. J. (2015b). Early-life stress triggers juvenile zebra finches to switch social learning strategies. Curr. Biol. 25, 2184–2188. doi: 10.1016/j.cub.2015.06.071
Fawcett, T. W., and Frankenhuis, W. E. (2015). Adaptive explanations for sensitive windows in development. Front. Zool. 12:S3. doi: 10.1186/1742-9994-12-S1-S3
Ferrari, M. C., Manassa, R. P., Dixson, D. L., Munday, P. L., McCormick, M. I., Meekan, M. G., et al. (2012). Effects of ocean acidification on learning in coral reef fishes. PLoS ONE 7:e31478. doi: 10.1371/journal.pone.0031478
Fessler, D. M., and Navarrete, C. D. (2003). Meat is good to taboo: dietary proscriptions as a product of the interaction of psychological mechanisms and social processes. J. Cognit. Cult. 3, 1–40. doi: 10.1163/156853703321598563
Fischer, S., and Frommen, J. G. (2013). Eutrophication alters social preferences in three-spined sticklebacks (Gasterosteus aculeatus). Behav. Ecol. Sociobiol. 67, 293–299. doi: 10.1007/s00265-012-1449-6
Fogarty, L., Rendell, L., and Laland, K. (2012). Mental time travel, memory and the social learning strategies tournament. Learn. Motivat. 43, 241–246. doi: 10.1016/j.lmot.2012.05.009
Fouda, L., Wingfield, J. E., Fandel, A. D., Garrod, A., Hodge, K. B., Rice, A. N., et al. (2018). Dolphins simplify their vocal calls in response to increased ambient noise. Biol. Lett. 14:20180484. doi: 10.1098/rsbl.2018.0484
Fuller, R. A., Warren, P. H., and Gaston, K. J. (2007). Daytime noise predicts nocturnal singing in urban robins. Biol. Lett. 3, 368–370. doi: 10.1098/rsbl.2007.0134
Galef, B. G., and Laland, K. N. (2005). Social learning in animals: empirical studies and theoretical models. BioScience 55, 489–499. doi: 10.1641/0006-3568(2005)055[0489:SLIAES]2.0.CO;2
Galef, B. G., and Whiskin, E. E. (2001). Interaction of social and individual learning in food preferences of norway rats. Anim. Behav. 62, 41–46. doi: 10.1006/anbe.2000.1721
Galef, B. G., and Whiskin, E. E. (2008). ‘Conformity’ in norway rats? Anim. Behav. 75, 2035–2039. doi: 10.1016/j.anbehav.2007.11.012
Galef, B. G., Whiskin, E. E., and Bielavska, E. (1997). Interaction with demonstrator rats changes observer rats' affective responses to flavors. J. Compar. Psychol. 111:393.
Gil, M. A., Hein, A. M., Spiegel, O., Baskett, M. L., and Sih, A. (2018). Social information links individual behavior to population and community dynamics. Trends Ecol. Evol. 33, 535–548. doi: 10.1016/j.tree.2018.04.010
Giraldeau, L., Valone, T. J., and Templeton, J. J. (2002). Potential disadvantages of using socially acquired information. Philos. Trans. R. Soc. Lond. B Biol. Sci. 357, 1559–1566. doi: 10.1098/rstb.2002.1065
Gonzalez, A., Ronce, O., Ferriere, R., and Hochberg, M. E. (2013). Evolutionary rescue: an emerging focus at the intersection between ecology and evolution. Philos. Trans. R. Soc. Lond. B Biol. Sci. 368:20120404. doi: 10.1098/rstb.2012.0404
Goudie, A. S. (2013). The Human Impact on the Natural Environment: Past, Present, and Future. Oxford: John Wiley & Sons.
Gould, S. J. (1991). Exaptation: a crucial tool for an evolutionary psychology. J. Soc. Issues 47, 43–65. doi: 10.1111/j.1540-4560.1991.tb01822.x
Greggor, A. L., Thornton, A., and Clayton, N. S. (2016). Harnessing learning biases is essential for applying social learning in conservation. Behav. Ecol. Sociobiol. 71:16. doi: 10.1007/s00265-016-2238-4
Griffin, A. (2004). Social learning about predators: a review and prospectus. Anim. Learn. Behav. 32, 131–140. doi: 10.3758/BF03196014
Griffin, A. S., Guillette, L. M., and Healy, S. D. (2015). Cognition and personality: an analysis of an emerging field. Trends Ecol. Evol. 30, 207–214. doi: 10.1016/j.tree.2015.01.012
Grüter, C., and Leadbeater, E. (2014). Insights from insects about adaptive social information use. Trends Ecol. Evol. 29, 177–184. doi: 10.1016/j.tree.2014.01.004
Hale, R., Treml, E. A., and Swearer, S. E. (2015). Evaluating the metapopulation consequences of ecological traps. Proc. R. Soc. Lond. B Biol. Sci. 282:20142930. doi: 10.1098/rspb.2014.2930
Henrich, J. (2001). Cultural transmission and the diffusion of innovations: Adoption dynamics indicate that biased cultural transmission is the predominate force in behavioral change. Am. Anthropol. 103, 992–1013. doi: 10.1525/aa.2001.103.4.992
Henrich, J., and Boyd, R. (1998). The evolution of conformist transmission and the emergence of between-group differences. Evol. Hum. Behav. 19, 215–241. doi: 10.1016/S1090-5138(98)00018-X
Henrich, J., and Henrich, N. (2010). The evolution of cultural adaptations: fijian food taboos protect against dangerous marine toxins. Proc. R. Soc. Lond. B Biol. Sci. 277, 3715–3724. doi: 10.1098/rspb.2010.1191
Henrich, J., and McElreath, R. (2003). The evolution of cultural evolution. Evol. Anthropol. 12, 123–135. doi: 10.1002/evan.10110
Hinde, R. A., and Fisher, J. (1951). Further observations on the opening of milk bottles by birds. Br. Birds 44, 393–396. doi: 10.1111/j.1469-185X.1994.tb01506.x
Ito, R., and Mori, A. (2010). Vigilance against predators induced by eavesdropping on heterospecific alarm calls in a non-vocal lizard oplurus cuvieri cuvieri (reptilia: Iguania). Proc. R. Soc. Lond. B Biol. Sci. 277, 1275–1280. doi: 10.1098/rspb.2009.2047
Jesmer, B. R., Merkle, J. A., Goheen, J. R., Aikens, E. O., Beck, J. L., Courtemanch, A. B., et al. (2018). Is ungulate migration culturally transmitted? evidence of social learning from translocated animals. Science 361, 1023–1025. doi: 10.1126/science.aat0985
Keith, S. A., and Bull, J. W. (2017). Animal culture impacts species' capacity to realise climate-driven range shifts. Ecography 40, 296–304. doi: 10.1111/ecog.02481
Kendal, J., Giraldeau, L.-A., and Laland, K. (2009a). The evolution of social learning rules: payoff-biased and frequency-dependent biased transmission. J. Theor. Biol. 260, 210–219. doi: 10.1016/j.jtbi.2009.05.029
Kendal, R. L., Boogert, N. J., Rendell, L., Laland, K. N., Webster, M., and Jones, P. L. (2018). Social learning strategies: bridge-building between fields. Trends Cognit. Sci. 22, 651–665. doi: 10.1016/j.tics.2018.04.003
Kendal, R. L., Coolen, I., and Laland, K. N. (2004). The role of conformity in foraging when personal and social information conflict. Behav. Ecol. 15, 269–277. doi: 10.1093/beheco/arh008
Kendal, R. L., Galef, B. G., and van Schaik, C. P. (2010). Social learning research outside the laboratory: how and why? Learn. Behav. 38, 187–194. doi: 10.3758/LB.38.3.187
Kendal, R. L., Kendal, J. R., Hoppitt, W., and Laland, K. N. (2009b). Identifying social learning in animal populations: a new ‘option-bias’ method. PLoS ONE 4:e6541. doi: 10.1371/journal.pone.0006541
Kline, M. A., Waring, T. M., and Salerno, J. (2018). Designing cultural multilevel selection research for sustainability science. Sustainabil. Sci. 13, 9–19. doi: 10.1007/s11625-017-0509-2
Laland, K. N. (2004). Social learning strategies. Anim. Learn. Behav. 32, 4–14. doi: 10.3758/BF03196002
Laland, K. N., and Williams, K. (1998). Social transmission of maladaptive information in the guppy. Behav. Ecol. 9, 493–499. doi: 10.1093/beheco/9.5.493
Lamon, N., Neumann, C., Gruber, T., and Zuberbühler, K. (2017). Kin-based cultural transmission of tool use in wild chimpanzees. Sci. Adv. 3:e1602750. doi: 10.1126/sciadv.1602750
Leduc, A. O., Kelly, J. M., and Brown, G. E. (2004). Detection of conspecific alarm cues by juvenile salmonids under neutral and weakly acidic conditions: laboratory and field tests. Oecologia 139, 318–324. doi: 10.1007/s00442-004-1492-8
Lienart, G. D. H., Ferrari, M. C. O., and McCormick, M. I. (2016). Thermal environment and nutritional condition affect the efficacy of chemical alarm cues produced by prey fish. Environ. Biol. Fish. 99, 729–739. doi: 10.1007/s10641-016-0516-7
Lindeyer, C. M., and Reader, S. M. (2010). Social learning of escape routes in zebrafish and the stability of behavioural traditions. Anim. Behav. 79, 827–834. doi: 10.1016/j.anbehav.2009.12.024
Lindström, B., Selbing, I., and Olsson, A. (2016). Co-evolution of social learning and evolutionary preparedness in dangerous environments. PLoS ONE 11:e0160245. doi: 10.1371/journal.pone.0160245
Luncz, L. V., Tan, A., Haslam, M., Kulik, L., Proffitt, T., Malaivijitnond, S., et al. (2017). Resource depletion through primate stone technology. eLife 6:e23647. doi: 10.7554/eLife.23647
Lürling, M., and Scheffer, M. (2007). Info-disruption: pollution and the transfer of chemical information between organisms. Trends Ecol. Evol. 22, 374–379. doi: 10.1016/j.tree.2007.04.002
Lusebrink, I., Girling, R. D., Farthing, E., Newman, T. A., Jackson, C. W., and Poppy, G. M. (2015). The effects of diesel exhaust pollution on floral volatiles and the consequences for honey bee olfaction. J. Chem. Ecol. 41, 904–912. doi: 10.1007/s10886-015-0624-4
Mace, G. M., and Reynolds, J. D. (2001). “Exploitation as a conservation issue,” in Conservation of Exploited Species, eds J. D. Reynolds, G. M. Mace, K. H. Redford, and J. G. Robinson (Cambridge, UK: Cambridge University Press), 1–15.
Mack, R. N., Simberloff, D., Mark Lonsdale, W., Evans, H., Clout, M., and Bazzaz, F. A. (2000). Biotic invasions: causes, epidemiology, global consequences, and control. Ecol. Appl. 10, 689–710. doi: 10.1890/1051-0761(2000)010[0689:BICEGC]2.0.CO;2
Marczak, L. B., Thompson, R. M., and Richardson, J. S. (2007). Meta-analysis: trophic level, habitat, and productivity shape the food web effects of resource subsidies. Ecology 88, 140–148. doi: 10.1890/0012-9658(2007)88[140:MTLHAP]2.0.CO;2
Marler, P., Peters, S., Ball, G. F., Dufty Jr, A. M., and Wingfield, J. C. (1988). The role of sex steroids in the acquisition and production of birdsong. Nature 336:770. doi: 10.1038/336770a0
Mazur, R., and Seher, V. (2008). Socially learned foraging behaviour in wild black bears, Ursus americanus. Anim. Behav. 75, 1503–1508. doi: 10.1016/j.anbehav.2007.10.027
McElreath, M. B., Boesch, C., Kuehl, H., and McElreath, R. (2018). Complex dynamics from simple cognition: the primary ratchet effect in animal culture. Evolut. Behav. Sci. 12, 191–202. doi: 10.1037/ebs0000117
McElreath, R., Bell, A. V., Efferson, C., Lubell, M., Richerson, P. J., and Waring, T. (2008). Beyond existence and aiming outside the laboratory: estimating frequency-dependent and pay-off-biased social learning strategies. Philos. Trans. R. Soc. Lond. B. Biol. Sci. 363, 3515–3528. doi: 10.1098/rstb.2008.0131
McElreath, R., Lubell, M., Richerson, P. J., Waring, T., Baum, W., Edsten, E., et al. (2005). Applying evolutionary models to the laboratory study of social learning. Evol. Hum. Behav. 26, 483–508. doi: 10.1016/j.evolhumbehav.2005.04.003
McElreath, R., and Strimling, P. (2008). When natural selection favors imitation of parents. Curr. Anthropol. 49, 307–316. doi: 10.1086/524364
McElreath, R., Wallin, A., and Fasolo, B. (2013). “The evolutionary rationality of social learning,” in Simple Heuristics in a Social World, eds R. Hertwig, U. Hoffrage the ABC Research Group (Oxford: Oxford University Press), 381–408. doi: 10.1093/acprof:oso/9780195388435.003.0014
McNamara, J. M., Dall, S. R., Hammerstein, P., and Leimar, O. (2016). Detection vs. selection: integration of genetic, epigenetic and environmental cues in fluctuating environments. Ecol. Lett. 19, 1267–1276. doi: 10.1111/ele.12663
Mesoudi, A. (2011). An experimental comparison of human social learning strategies: payoff-biased social learning is adaptive but underused. Evol. Hum. Behav. 32, 334–342. doi: 10.1016/j.evolhumbehav.2010.12.001
Miu, E., Gulley, N., Laland, K. N., and Rendell, L. (2018). Innovation and cumulative culture through tweaks and leaps in online programming contests. Nat. Commun. 9:2321. doi: 10.1038/s41467-018-04494-0
Modlmeier, A. P., Keiser, C. N., Watters, J. V., Sih, A., and Pruitt, J. N. (2014). The keystone individual concept: an ecological and evolutionary overview. Anim. Behav. 89, 53–62. doi: 10.1016/j.anbehav.2013.12.020
Morand-Ferron, J., Hamblin, S., Cole, E. F., Aplin, L. M., and Quinn, J. L. (2015). Taking the operant paradigm into the field: associative learning in wild great tits. PLoS ONE 10:e0133821. doi: 10.1371/journal.pone.0133821
Müller, C. A., and Cant, M. A. (2010). Imitation and traditions in wild banded mongooses. Curr. Biol. 20, 1171–1175. doi: 10.1016/j.cub.2010.04.037
Nieberding, C. M., Dyck, H. V., and Chittka, L. (2018). Adaptive learning in non-social insects: from theory to field work, and back. Curr. Opin. Insect Sci. 27, 75–81. doi: 10.1016/j.cois.2018.03.008
Nöbel, S., Allain, M., Isabel, G., and Danchin, E. (2018). Mate copying in drosophila melanogaster males. Anim. Behav. 141, 9–15. doi: 10.1016/j.anbehav.2018.04.019
Nottebohm, F., Nottebohm, M. E., Crane, L. A., and Wingfield, J. C. (1987). Seasonal changes in gonadal hormone levels of adult male canaries and their relation to song. Behav. Neural Biol. 47, 197–211. doi: 10.1016/S0163-1047(87)90327-X
Ohashi, G., and Matsuzawa, T. (2011). Deactivation of snares by wild chimpanzees. Primates 52, 1–5. doi: 10.1007/s10329-010-0212-8
Parejo, D., Danchin, E., and Avilés, J. M. (2005). The heterospecific habitat copying hypothesis: can competitors indicate habitat quality? Behav. Ecol. 16, 96–105. doi: 10.1093/beheco/arh136
Parris, K. M., and Schneider, A. (2009). Impacts of traffic noise and traffic volume on birds of roadside habitats. Ecol. Soc. 14:29. doi: 10.5751/ES-02761-140129
Patricelli, G. L., and Blickley, J. L. (2006). Avian communication in urban noise: causes and consequences of vocal adjustment. Auk 123, 639–649. doi: 10.1642/0004-8038(2006)123[639:ACIUNC]2.0.CO;2
Perry, S. (2009). Conformism in the food processing techniques of white-faced capuchin monkeys (Cebus capucinus). Anim. Cognit. 12, 705–716. doi: 10.1007/s10071-009-0230-3
Perry, S. E., Barrett, B. J., and Godoy, I. (2017). Older, sociable capuchins (cebus capucinus) invent more social behaviors, but younger monkeys innovate more in other contexts. Proc. Natl. Acad. Sci. U.S.A. 114, 7806–7813. doi: 10.1073/pnas.1620739114
Pike, T. W., Kendal, J. R., Rendell, L. E., and Laland, K. N. (2010). Learning by proportional observation in a species of fish. Behav. Ecol. 21, 570–575. doi: 10.1093/beheco/arq025
Pike, T. W., and Laland, K. N. (2010). Conformist learning in nine-spined sticklebacks' foraging decisions. Biol. Lett. 6, 466–468. doi: 10.1098/rsbl.2009.1014
Reader, S. M., and Biro, D. (2010). Experimental identification of social learning in wild animals. Learn. Behav. 38, 265–283. doi: 10.3758/LB.38.3.265
Reader, S. M., Hager, Y., and Laland, K. N. (2011). The evolution of primate general and cultural intelligence. Philos. Trans. R. Soc. B Biol. Sci. 366, 1017–1027. doi: 10.1098/rstb.2010.0342
Rendell, L., Boyd, R., Cownden, D., Enquist, M., Eriksson, K., Feldman, M. W., et al. (2010). Why copy others? insights from the social learning strategies tournament. Science 328, 208–213. doi: 10.1126/science.1184719
Rendell, L., Fogarty, L., Hoppitt, W. J., Morgan, T. J., Webster, M. M., and Laland, K. N. (2011). Cognitive culture: theoretical and empirical insights into social learning strategies. Trends Cogn. Sci. 15, 68–76. doi: 10.1016/j.tics.2010.12.002
Richerson, P. J. (2019). An integrated bayesian theory of phenotypic flexibility. Behav. Process. 161, 54–64. doi: 10.1016/j.beproc.2018.02.002
Rieucau, G., and Giraldeau, L.-A. (2011). Exploring the costs and benefits of social information use: an appraisal of current experimental evidence. Philos. Trans. R. Soc. Lond. B Biol. Sci. 366, 949–957. doi: 10.1098/rstb.2010.0325
Rogers, A. R. (1988). Does biology constrain culture? Am. Anthropol. 90, 819–831. doi: 10.1525/aa.1988.90.4.02a00030
Schakner, Z. A., Buhnerkempe, M. G., Tennis, M. J., Stansell, R. J., van der Leeuw, B. K., Lloyd-Smith, J. O., et al. (2016). Epidemiological models to control the spread of information in marine mammals. Proc. R. Soc. Lond. B Biol. Sci. 283:2037. doi: 10.1098/rspb.2016.2037
Schakner, Z. A., Lunsford, C., Straley, J., Eguchi, T., and Mesnick, S. L. (2014). Using models of social transmission to examine the spread of longline depredation behavior among sperm whales in the gulf of alaska. PLoS ONE 9:e109079. doi: 10.1371/journal.pone.0109079
Schlaepfer, M. A., Runge, M. C., and Sherman, P. W. (2002). Ecological and evolutionary traps. Trends Ecol. Evol. 17, 474–480. doi: 10.1016/S0169-5347(02)02580-6
Seligman, M. E. (1971). Phobias and preparedness. Behav. Therapy 2, 307–320. doi: 10.1016/S0005-7894(71)80064-3
Semel, B., and Sherman, P. W. (2001). Intraspecific parasitism and nest-site competition in wood ducks. Anim. Behav. 61, 787–803. doi: 10.1006/anbe.2000.1657
Seppänen, J.-T., Forsman, J. T., Mönkkönen, M., and Thomson, R. L. (2007). Social information use is a process across time, space, and ecology, reaching heterospecifics. Ecology 88, 1622–1633. doi: 10.1890/06-1757.1
Seyfarth, R., Cheney, D., and Marler, P. (1980). Monkey responses to three different alarm calls: evidence of predator classification and semantic communication. Science 210, 801–803. doi: 10.1126/science.7433999
Shannon, G., Slotow, R., Durant, S. M., Sayialel, K. N., Poole, J., Moss, C., et al. (2013). Effects of social disruption in elephants persist decades after culling. Front. Zool. 10:62. doi: 10.1186/1742-9994-10-62
Sigaud, M., Merkle, J. A., Cherry, S. G., Fryxell, J. M., Berdahl, A., and Fortin, D. (2017). Collective decision-making promotes fitness loss in a fusion-fission society. Ecol. Lett. 20, 33–40. doi: 10.1111/ele.12698
Sih, A. (1992). Prey uncertainty and the balancing of antipredator and feeding needs. Am. Natur. 139, 1052–1069. doi: 10.1086/285372
Sih, A. (2013). Understanding variation in behavioural responses to human-induced rapid environmental change: a conceptual overview. Anim. Behav. 85, 1077–1088. doi: 10.1016/j.anbehav.2013.02.017
Sih, A., and Del Giudice, M. (2012). Linking behavioural syndromes and cognition: a behavioural ecology perspective. Philos. Trans. R. Soc. Lond. B. Biol. Sci. 367, 2762–2772. doi: 10.1098/rstb.2012.0216
Sih, A., Ferrari, M. C., and Harris, D. J. (2011). Evolution and behavioural responses to human-induced rapid environmental change. Evolut. Appl. 4, 367–387. doi: 10.1111/j.1752-4571.2010.00166.x
Singer, M. C., Thomas, C. D., and Parmesan, C. (1993). Rapid human-induced evolution of insect–host associations. Nature 366:681. doi: 10.1038/366681a0
Skelly, D. K., Joseph, L. N., Possingham, H. P., Freidenburg, L. K., Farrugia, T. J., Kinnison, M. T., et al. (2007). Evolutionary responses to climate change. Conser. Biol. 21, 1353–1355. doi: 10.1111/j.1523-1739.2007.00764.x
Slagsvold, T., and Wiebe, K. L. (2011). Social learning in birds and its role in shaping a foraging niche. Philos. Trans. R. Soc. Lond. B. Biol. Sci. 366, 969–977. doi: 10.1098/rstb.2010.0343
Stephens, D. (1991). Chnage, regularity, and value in the evolution of animal learning. Behav. Ecol. 2, 77–89. doi: 10.1093/beheco/2.1.77
Stephens, D. W., and Krebs, J. R. (1986). Foraging Theory. Princeton, NJ: Princeton University Press.
Szymanski, M. L., and Afton, A. D. (2005). Effects of spinning-wing decoys on flock behavior and hunting vulnerability of mallards in minnesota. Wildlife Soc. Bull. 33, 993–1001. doi: 10.2193/0091-7648(2005)33[993:EOSDOF]2.0.CO;2
Tebbich, S., Taborsky, M., Fessl, B., and Blomqvist, D. (2001). Do woodpecker finches acquire tool-use by social learning? Proc. R. Soc. Lond. B Biol. Sci. 268, 2189–2193. doi: 10.1098/rspb.2001.1738
Teitelbaum, C. S., Converse, S. J., Fagan, W. F., Böhning-Gaese, K., Ohara, R. B., Lacy, A. E., et al. (2016). Experience drives innovation of new migration patterns of whooping cranes in response to global change. Nat. Commun. 7:12793. doi: 10.1038/ncomms12793
Terkel, J. (1996). “Cultural transmission of feeding behavior in the black rat (rattus rattus),” in Social Learning in Animals: The Roots of Culture, eds C. Heyes, and B. G. Galef (Academic Press London), 17–47.
Thornton, A., and Clutton-Brock, T. (2011). Social learning and the development of individual and group behaviour in mammal societies. Philos. Trans. R. Soc. B Biol. Sci. 366, 978–987. doi: 10.1098/rstb.2010.0312
Trompf, L., and Brown, C. (2014). Personality affects learning and trade-offs between private and social information in guppies, Poecilia reticulata. Anim. Behav. 88, 99–106. doi: 10.1016/j.anbehav.2013.11.022
Vale, G. L., Davis, S. J., van de Waal, E., Schapiro, S. J., Lambeth, S. P., and Whiten, A. (2017). Lack of conformity to new local dietary preferences in migrating captive chimpanzees. Anim. Behav. 124, 135–144. doi: 10.1016/j.anbehav.2016.12.007
Valone, T. J., and Templeton, J. J. (2002). Public information for the assessment of quality: a widespread social phenomenon. Philos. Trans. R. Soc. B Biol. Sci. 357, 1549–1557. doi: 10.1098/rstb.2002.1064
van de Waal, E., Borgeaud, C., and Whiten, A. (2013). Potent social learning and conformity shape a wild primate's foraging decisions. Science 340, 483–485. doi: 10.1126/science.1232769
Van Leeuwen, E. J., Kendal, R. L., Tennie, C., and Haun, D. B. (2015). Conformity and its look-a-likes. Anim. Behav. 110, e1–e4. doi: 10.1016/j.anbehav.2015.07.030
Vilhunen, S., Hirvonen, H., and Laakkonen, M. V.-M. (2005). Less is more: social learning of predator recognition requires a low demonstrator to observer ratio in arctic charr (Salvelinus alpinus). Behav. Ecol. Sociobiol. 57, 275–282. doi: 10.1007/s00265-004-0846-x
Walker, C. H., Sibly, R., and Peakall, D. B. (2014). Principles of Ecotoxicology. Boca Raton, FL: CRC Press.
Walther, G.-R., Post, E., Convey, P., Menzel, A., Parmesan, C., Beebee, T. J., et al. (2002). Ecological responses to recent climate change. Nature 416:389. doi: 10.1038/416389a
Ward, A. J., Duff, A. J., Horsfall, J. S., and Currie, S. (2008). Scents and scents-ability: pollution disrupts chemical social recognition and shoaling in fish. Proc. R. Soc. Lond. B Biol. Sci. 275, 101–105. doi: 10.1098/rspb.2007.1283
Weinrich, M. T., Schilling, M. R., and Belt, C. R. (1992). Evidence for acquisition of a novel feeding behaviour: lobtail feeding in humpback whales, Megaptera novaeangliae. Anim. Behav. 44, 1059–1072. doi: 10.1016/S0003-3472(05)80318-5
West-Eberhard, M. J. (1989). Phenotypic plasticity and the origins of diversity. Ann. Rev. Ecol. Syst. 20, 249–278. doi: 10.1146/annurev.es.20.110189.001341
Whitehead, H. (2010). Conserving and managing animals that learn socially and share cultures. Learn. Behav. 38, 329–336. doi: 10.3758/LB.38.3.329
Whitehead, H., Rendell, L., Osborne, R. W., and Würsig, B. (2004). Culture and conservation of non-humans with reference to whales and dolphins: review and new directions. Biol. Conserv. 120, 427–437. doi: 10.1016/j.biocon.2004.03.017
Whitehead, H., and Richerson, P. J. (2009). The evolution of conformist social learning can cause population collapse in realistically variable environments. Evol. Hum. Behav. 30, 261–273. doi: 10.1016/j.evolhumbehav.2009.02.003
Wong, B., and Candolin, U. (2015). Behavioral responses to changing environments. Behav. Ecol. 26, 665–673. doi: 10.1093/beheco/aru183
Wrangham, R. W., Koops, K., Machanda, Z. P., Worthington, S., Bernard, A. B., Brazeau, N. F., et al. (2016). Distribution of a chimpanzee social custom is explained by matrilineal relationship rather than conformity. Curr. Biol. 26, 3033–3037. doi: 10.1016/j.cub.2016.09.005.
Keywords: social learning, rapid environment change, ecological trap, phenotypic plasticity, cultural evolution, communication, social learning strategies
Citation: Barrett B, Zepeda E, Pollack L, Munson A and Sih A (2019) Counter-Culture: Does Social Learning Help or Hinder Adaptive Response to Human-Induced Rapid Environmental Change? Front. Ecol. Evol. 7:183. doi: 10.3389/fevo.2019.00183
Received: 27 February 2019; Accepted: 07 May 2019;
Published: 29 May 2019.
Edited by:
Laure Cauchard, University of Aberdeen, United KingdomReviewed by:
Daizaburo Shizuka, University of Nebraska-Lincoln, United StatesCopyright © 2019 Barrett, Zepeda, Pollack, Munson and Sih. This is an open-access article distributed under the terms of the Creative Commons Attribution License (CC BY). The use, distribution or reproduction in other forums is permitted, provided the original author(s) and the copyright owner(s) are credited and that the original publication in this journal is cited, in accordance with accepted academic practice. No use, distribution or reproduction is permitted which does not comply with these terms.
*Correspondence: Brendan Barrett, YmJhcnJldHRAb3JuLm1wZy5kZQ==; Emily Zepeda, ZWF6ZXBlZGFAdWNkYXZpcy5lZHU=
†joint first authors
Disclaimer: All claims expressed in this article are solely those of the authors and do not necessarily represent those of their affiliated organizations, or those of the publisher, the editors and the reviewers. Any product that may be evaluated in this article or claim that may be made by its manufacturer is not guaranteed or endorsed by the publisher.
Research integrity at Frontiers
Learn more about the work of our research integrity team to safeguard the quality of each article we publish.