- 1Department of Ecology, Evolution, and Marine Biology, University of California, Santa Barbara, Santa Barbara, CA, United States
- 2Marine Science Institute, University of California, Santa Barbara, Santa Barbara, CA, United States
Rising rates of emerging infectious diseases (EIDs) demand creative, efficient, and integrative investigations to understand their transmission, ecological contingencies, and dynamics at wildlife-human interfaces. High-throughput sequencing (HTS) methodologies provide enormous potential to unravel these contingencies to improve our understanding, but their potential is only just starting to be realized. While recent work has largely focused on novel pathogen discovery at likely interfaces, high-throughput methods can also allow disease ecologists to better explore the critical effects of climate, seasonality, and land-use changes on EIDs. HTS can facilitate the creation of entire host-pathogen networks, integrate important microbiome and co-infection data, and even pinpoint important exposure routes at interfaces through environmental media. Here we highlight studies at the frontier of HTS and disease ecology research, identify current limitations, and outline promising future applications for EIDs.
Introduction
Increasing globalization, agricultural intensification, urbanization, and climatic changes have resulted in a marked recent increase in emerging infectious diseases (EIDs) (Jones et al., 2008, 2013; Hassell et al., 2016). While EIDs are often zoonotic, viral, and triggered by shifts in host behavior and ecology (Jones et al., 2008; Morse et al., 2012), they are also incredibly diverse and often display complex transmission dynamics, rendering prediction challenging (Holmes, 2013). Fortunately, despite this complexity, pressing questions in emerging disease ecology – including the effect of environmental conditions on infection risk, the role of host microbiome in altering disease dynamics, and multi-host multi-pathogen relationships (Lively et al., 2014) – may be effectively broached using molecular advances in the form of high-throughput sequencing (HTS). HTS already enables EID surveillance at interfaces where humans, wildlife, and domestic animals overlap, by screening for pathogens in likely reservoir hosts (Carroll et al., 2018). Yet, we have made relatively little use of the extraordinary potential of HTS approaches to understand how climate, season, behavior and other ecological factors affect pathogen diversity, in both the community of pathogens and the within-pathogen heterogeneity that allow for increases in virulence and infectivity.
While traditional PCR methods target a small slice of pathogen diversity in a sample, HTS allows researchers to identify a wide range of DNA sequences simultaneously using a specified genetic region (metabarcoding/amplicon-based methods) or all genetic material (metagenomics, Box 1). To date, health-oriented researchers have harnessed HTS extensively for novel pathogen discovery and surveillance (Didelot et al., 2012), and ecologists have used HTS to understand energy flows in food-web ecology (e.g., Pompanon et al., 2012), microbial functions in soil sciences (e.g., Fierer et al., 2012), and the movement of invasive, rare, or endangered species using environmental DNA (eDNA) (e.g., Rees et al., 2014). However, HTS has only recently been used to reveal the complex ecology of host-pathogen-environmental interactions, although interest in the topic is rapidly expanding (Figure 1). With dropping costs and development of field-stable preservation methods (e.g., Wegleitner et al., 2015), HTS is increasingly feasible worldwide. This will certainly allow for more novel pathogen discovery and expansion of effort in remote and under-served locations where EID risk is highest (Jones et al., 2008) and many pathogens are undescribed. It should also facilitate more robust investigations across broad geographic regions over time, which is ultimately needed to help identify important locations for continued surveillance.
Box 1. Key definitions.
Bioinformatics: a method of “conceptualizing biology in terms of molecules and applying information techniques (derived from disciplines such as applied math, computer science and statistics) to understand and organize the information associated with these molecules, on a large scale” (Luscombe et al., 2001).
Environmental DNA (eDNA): “genetic material obtained directly from environmental samples (soil, sediment, water, etc.) without any obvious signs of biological source material.” (Thomsen and Willerslev, 2015).
High Throughput Sequencing (HTS); also Next Generation Sequencing (NGS): “… [a] method used to determine a portion of the nucleotide sequence of an individual's genome. This technique utilizes DNA sequencing technologies that are capable of processing multiple DNA sequences in parallel.” (National Cancer Institute Dictionary of Genetic Terms)
Metabarcoding: “…[T]he automated identification of multiple species from a single bulk sample containing entire organisms or from a single environmental sample containing degraded DNA” (Taberlet et al., 2012).
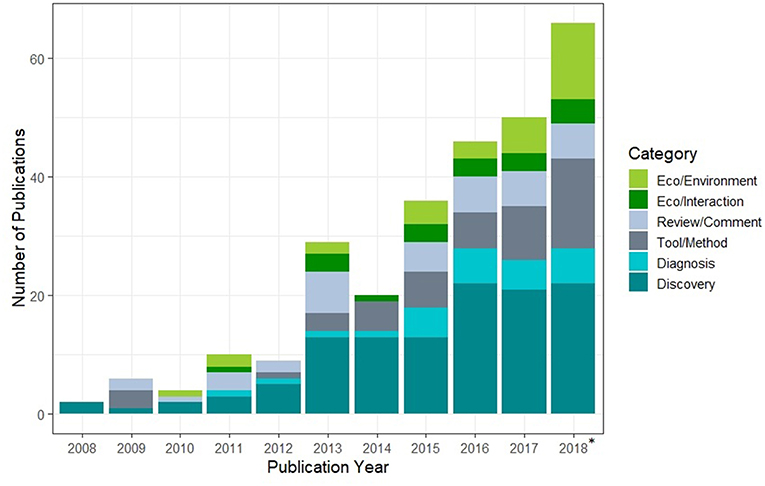
Figure 1. A Web of Science search for combinations of the words metabarcod* or metagenom* or eDNA and emerging pathogen, parasite, or emerging disease revealed 278 relevant publications, demonstrating the accelerating interest in using HTS approaches to understand the ecology of emerging diseases across a range of applications. Articles for 2018 do not include December publications. Categories were defined as: Eco/Environment—HTS used to detect changes across pathogen communities in different environments, Eco/Interaction—interactions of microbiota or other organisms with pathogen communities, Review/Comment—reviews or commentaries, Tool/Method—new protocols, tools, or comparisons of methods, Diagnosis—HTS used to diagnose disease or identify aetiological agents, Discovery—HTS used to discover or describe new pathogens.
Identifying Important Interfaces
Given cost and data handling constraints, it is critical to identify locations and contexts that are likely EID interfaces (Morse et al., 2012). In general, interfaces may be locations of increased human-wildlife contact, such as forest edges (Wilcox and Ellis, 2006), locations with substantial land-use change, such as increased agricultural intensification (Jones et al., 2013), or sites where humans have high contact rates with animals, such as rodents, that thrive in urban environments (Williams et al., 2018). EID transmission at dynamic interfaces of wildlife, livestock, and humans occurs via media, such as air, water, food, and bodily fluids (including vector bites), and many interactions are likely influenced by seasonal (Altizer et al., 2006), climatic (Harvell et al., 2002), or ecological context (Patz et al., 2004).
Host-Specific Surveillance for EIDs
Many HTS surveillance efforts directly screen wildlife hosts for pathogens that are either the causative agent or closely related to current EIDs, and research efforts have thus concentrated on viruses in domestic animals, bats, rodents, and non-human primates (e.g., Temmam et al., 2014; Carroll et al., 2018), which are primary reservoirs of EIDs. In bats alone, HTS studies have revealed viruses closely related to and known to cause Ebola, Nipah, Marburg, and other coronoviruses (Han et al., 2015). In particular, a prominent multinational research program, PREDICT, uses a combination of simple molecular and HTS techniques for viral surveillance in wildlife, livestock and humans at likely interfaces (Morse et al., 2012). These efforts have revealed more than 800 novel viruses and have contributed to an extensive database of zoonotic pathogens (Epstein and Anthony, 2017). Other studies have focused on diverse parasite types across hosts, including Chagas-like trypanosomes in bats (Dario et al., 2017), Babesia and Rickettsial pathogens in non-human primates (Nakayima et al., 2014), and pathogenic bacteria in urban mice (Williams et al., 2018).
While these studies collect valuable pathogen detection and identification data, most do not assess prevalence, ecological risk factors, or infection dynamics using multi-pathogen methods (Bodewes, 2018). Collecting concurrent data on host movements at interfaces across seasons could allow researchers to understand spatial, temporal, or host-specific attributes that contribute to pathogen diversity, especially in investigations of viral evolution and transmission pathways following outbreaks (e.g., Ramey et al., 2017). For example, one recent study used metabarcoding to determine feeding patterns and population structure of vampire bats that could be used to inform projections of rabies transmission across interfaces (Bohmann et al., 2018). Other studies have connected host microbial communities to parasite infection, revealing potential important evolutionary links (Zaiss and Harris, 2016; Rosa et al., 2018). Other studies that focus on ecological risk factors for pathogen infection (e.g., Oakgrove et al., 2014; Titcomb et al., 2017; Young et al., 2017) have been constrained to one or a few focal pathogens, but their scope can be expanded via HTS, promising to reveal insights for a wide range of diseases (Table 1).
Environmental Surveillance for EIDs
Despite swelling databases of host-parasite associations (e.g., Nunn and Altizer, 2005; Bensch et al., 2009; Urban et al., 2015), we still know relatively little of mechanistic sources of infection risk. Extending HTS use to explore hypotheses of transmission mechanisms and environmental sampling should enable researchers to pinpoint infection sources or dangerous interfaces. Although there is a clear need for elucidating mechanisms (Littlefair and Clare, 2016), progress had been slow for EIDs without insect vectors, given that indirect pathways are often complex and dynamic. For example, increasing evidence suggests that bat viruses are predominantly transmitted via secondary reservoirs that consume fruits contaminated with bat saliva (Han et al., 2015; Mann et al., 2015). Application of HTS to these suspected transmission sources could pinpoint problematic interfaces. Given that bats are frequently persecuted for their zoonotic connections (Schneeberger and Voigt, 2016), surveillance via intermediate hosts or likely transmission sources to humans (i.e., fruits) may even decrease persecution by identifying feasible interventions.
Just as individual hosts may act as pathogen superspreaders, specific interfaces may act as hotspots of pathogen transmission (Paull et al., 2012). HTS now allows researchers to interrogate environmental media (e.g., water, soil, food) for a wide variety of pathogens simultaneously, a feat that was impossible until very recently. Application of HTS to eDNA has numerous potential benefits for advancing understanding of diseases at interfaces and in environmental media (soil, water, air) (Bass et al., 2015). Monitoring water sources using metagenomics has become increasingly popular (Nieuwenhuijse and Koopmans, 2017), with applications revealing pathogen community shifts across land-use types (Gu et al., 2018), pathogen movement via commercial ships (Lohan et al., 2016), and mapping of high-risk sites from wastewater sampling (Cantalupo et al., 2011). Further useful applications might enable researchers to explore the effect of environmental policy measures, land management changes (e.g., dam creation), or species (re)introductions on pathogen prevalence and diversity at important interfaces.
Vector Surveillance for EIDs
Screening vectors for pathogens is a clear application of HTS to understanding EID transmission at human-wildlife interfaces. This practice has expanded in the past decade, with particular focus on mosquitoes and ticks (Temmam et al., 2014). For example, recent work detected an emerging rickettsial pathogen in ticks in California (Bouquet et al., 2017), novel Bunyaviruses (a viral family that includes Crimean-Congo Hemorrhagic Fever and others) (Sakamoto et al., 2016; Bouquet et al., 2017), and numerous potentially-pathogenic bacterial genera in saliva across tick species (Qiu et al., 2014). While novel pathogen discovery is critical, understanding the ecology of vector-borne pathogens requires consideration of host communities, environmental and seasonal effects, and vector-pathogen interactions, among others (Table 1).
Recent molecular studies have considered how vector microbiomes may affect pathogens in different contexts. For example, one study used metabarcoding to determine that mosquito microbiota were more diverse in rural areas compared to urban areas, and varied substantially by species (Thongsripong et al., 2018). Other investigations have sought to identify microbial differences in infected mosquitoes (Villegas et al., 2018), ticks (Narasimhan et al., 2014), and triatomines (Gumiel et al., 2015). Furthermore, harnessing HTS to reveal feeding information with that of standard vector surveillance can allow the development of transmission networks, increasing our knowledge of disease dynamics, and ultimately informing public health approaches by identifying key species or pathogen superspreaders. One study detected decreasing diversity of hosts for sandflies and mosquitoes across a disturbance gradient (Kocher et al., 2017), demonstrating a potential system for investigation of pathogen prevalence and vector feeding networks in response to anthropogenic change. Another recent study simultaneously characterized diverse host species and prevalence of Trypanosoma cruzi, the causative agent of Chagas disease, by sequencing the guts of Triatomines to create a potential transmission network (Dumonteil et al., 2018). By comparing host feeding and transmission networks across ecological gradients or following changes to host communities (e.g., wildlife loss, increased livestock, invasive species) researchers could model likely future disease outcomes in changing ecosystems.
Holistic Approaches
Integrating data on pathogen sequences found in hosts, vectors and the environment will enable researchers to reveal seasonal, climatic, or ecological patterns affecting EID transmission. However, many HTS applications focus on pathogen detection in either hosts, vector, or the environment, and studies that do focus on these in tandem are usually constrained to single pathogens. For example, one study investigated prevalence of Cryptosporidium parvum in cattle hosts, humans, and water sources simultaneously to show potential pathways of environmental transmission (Peng et al., 2003). Another study allowed researchers to confirm that the majority of typhoid infections in Nepal were indirectly transmitted, and likely to be spread via public water spouts, where sequences of two different typhoid-causing pathogens were found (Baker et al., 2011). Future studies using HTS could allow researchers to draw similar conclusions for an entire suite of parasites. An integrated research approach that reveals pathogen diversity within hosts and across interfaces may allow researchers to better understand why certain pathogens are successfully transmitted while others are not. When armed with data on pathogen diversity in wild hosts, their behavior at interfaces, and pathogen prevalence in environmental media that humans and their livestock encounter, we may be able to develop successful barriers to transmission that promote wildlife conservation.
Limitations
There are several important limitations to HTS-based inferences about pathogens. For example, the metagenomic process tends to compound errors, particularly in environmental samples (Darling and Mahon, 2011), obscuring inferences about pathogen diversity. While noninvasive genetic approaches, such as eDNA with HTS, have a well-documented road map for methodological development to account for errors (Beja-Pereira et al., 2009), pathogens pose additional challenges beyond those encountered in the search for macrofaunal biodiversity from eDNA. In particular, pathogens and parasites are enormously diverse (Poulin and Morand, 2000), making development of primers to target a range of pathogens for metabarcoding challenging. Furthermore, database coverage of pathogens is substantially less-developed than in other organisms (Ryan et al., 2017), resulting in identification uncertainty and inaccurate estimates of pathogen richness. The solution is increased storage and management of pathogen genome and metagenome databases (McNeil et al., 2007; Carroll et al., 2018; Zhang et al., 2018) coupled with additional field and laboratory expertise to document the breadth of global pathogen diversity. Yet, costs are still high, especially in regions where pathogen diversity is highest and likely to have the strongest impacts on human and animal health (Jones et al., 2008; Ryan et al., 2017).
Technology is rapidly improving our ability to identify sequences to species or strain (Luo et al., 2012). Single marker studies with short base pair read lengths can provide uncertain results with sequences identified only to pathogen groups (i.e., Vibrio, Legionella, Mycobacterium) (Mahon et al., 2018). While multiple marker inferences (Olds et al., 2016) and growing bioinformatics programs will improve our ability to identify specific pathogens, technology, and primer development for longer and reliable sequence reads will be beneficial. Similarly, improved methods to detect rare and elusive species (Jerde et al., 2011), and to evaluate sampling adequacy (Grey et al., 2018) have been developed, but the broader experimental design framework to guide future work is still an area of active research. Increased application of HTS across a wide range of scientific fields has also increased demand for innovation in multivariate statistics to compare pathogen communities over space, time, and environmental gradients (Paliy and Shankar, 2016).
Finally, a critical challenge lies in determining which pathogens are, or will be, virulent in humans or their domestic animals and in what contexts (Temmam et al., 2014; Epstein and Anthony, 2017). While continued surveillance of both healthy and diseased animals will help to identify harmful pathogens (Levinson et al., 2013), HTS methods will often need to be used as a complement to traditional methods in diagnosing infectious diseases (Miller et al., 2013; Simner et al., 2018). While infectious disease outbreaks remain challenging to predict, HTS methods offer substantial advantages in improving much-needed surveillance and broader understanding of EIDs (Holmes et al., 2018).
Conclusions and Future Directions
In the past decade, the number of articles that combine HTS and disease ecology has increased more than 10-fold, revealing the potential for enormous gains in understanding the underlying ecology of EIDs. However, many studies have focused on novel pathogen discovery, and vital contextual information is missing. While substantial, current technological limitations are being addressed, expanding the breadth and reliability of HTS studies. By pairing ecological concepts with HTS and epidemiological expertise, researchers will better address outstanding knowledge gaps: the effect of anthropogenic changes on pathogen diversity, exposure, and infectivity; the primary and secondary routes of EID transmission through environmental media; multi-host-multi-pathogen dynamics; and development of complete host-parasite networks that further our understanding of these critically-important diseases.
Author Contributions
GT conceived the article and wrote the initial draft. CJ contributed sections and provided feedback. HY helped conceive the article and provided feedback.
Funding
GT was supported by NSF GRF (1650114) and NGS Early Career (EC-333R-18) grants. This work was also supported by the NSF (DEB - 1556786) awarded to HY. CJ was partially supported by USAID (AID-OAA-A-16-00057) and the Great Lakes Fishery Commission (2017 WEL 77011).
Conflict of Interest Statement
The authors declare that the research was conducted in the absence of any commercial or financial relationships that could be construed as a potential conflict of interest.
Acknowledgments
We thank two reviewers for their helpful comments in improving the original manuscript.
References
Aivelo, T., and Norberg, A. (2017). Parasite-microbiota interactions potentially affect intestinal communities in wild mammals. J. Anim. Ecol. 87, 438–447. doi: 10.1111/1365-2656.12708
Altizer, S., Dobson, A., Hosseini, P., Hudson, P., Pascual, M., and Rohani, P. (2006). Seasonality and the dynamics of infectious diseases. Ecol. Lett. 9, 467–484. doi: 10.1111/j.1461-0248.2005.00879.x
Avramenko, R. W., Redman, E. M., Lewis, R., Bichuette, M. A., Palmeira, B. M., Yazwinski, T. A., et al. (2017). The use of nemabiome metabarcoding to explore gastro-intestinal nematode species diversity and anthelmintic treatment effectiveness in beef calves. Int. J. Parasitol. 47, 893–902. doi: 10.1016/j.ijpara.2017.06.006
Baker, S., Holt, K. E., Clements, A. C., Karkey, A., Arjyal, A., Boni, M. F., et al. (2011). Combined high-resolution genotyping and geospatial analysis reveals modes of endemic urban typhoid fever transmission. Open Biol. 1:110008. doi: 10.1098/rsob.110008
Bass, D., Stentiford, G. D., Littlewood, D. T. J., and Hartikainen, H. (2015). Diverse applications of environmental DNA methods in parasitology. Trends Parasitol. 31, 499–513. doi: 10.1016/j.pt.2015.06.013
Beja-Pereira, A., Oliveira, R., Alves, P. C., Schwartz, M. K., and Luikart, G. (2009). Advancing ecological understandings through technological transformations in noninvasive genetics. Mol. Ecol. Resour. 9, 1279–1301. doi: 10.1111/j.1755-0998.2009.02699.x
Bensch, S., Hellgren, O., and Pérez-Tris, J. (2009). MalAvi: a public database of malaria parasites and related haemosporidians in avian hosts based on mitochondrial cytochrome b lineages. Mol. Ecol. Resour. 9, 1353–1358. doi: 10.1111/j.1755-0998.2009.02692.x
Bodewes, R. (2018). Novel viruses in birds: flying through the roof or is a cage needed? Vet. J. 233, 55–62. doi: 10.1016/j.tvjl.2017.12.023
Bohmann, K., Gopalakrishnan, S., Nielsen, M., Nielsen, L., dos, S. B., Jones, G., Streicker, D. G., et al. (2018). Using DNA metabarcoding for simultaneous inference of common vampire bat diet and population structure. Mol. Ecol. Resour. 18, 1050–1063. doi: 10.1111/1755-0998.12891
Bouquet, J., Melgar, M., Swei, A., Delwart, E., Lane, R. S., and Chiu, C. Y. (2017). Metagenomic-based Surveillance of pacific coast tick dermacentor occidentalis identifies two novel bunyaviruses and an emerging human ricksettsial pathogen. Sci. Rep. 7:12234. doi: 10.1038/s41598-017-12047-6
Cantalupo, P. G., Calgua, B., Zhao, G., Hundesa, A., Wier, A. D., Katz, J. P., et al. (2011). Raw sewage harbors diverse viral populations. MBio 2, e00180–11. doi: 10.1128/mBio.00180-11
Carroll, D., Daszak, P., Wolfe, N.D., Gao, G.F., Morel, C.M., Morzaria, S., et al. (2018). The global virome project. Science. 359, 872–874. doi: 10.1126/science.aap7463
Dario, M. A., Moratelli, R., Schwabl, P., Jansen, A. M., and Llewellyn, M. S. (2017). Small subunit ribosomal metabarcoding reveals extraordinary trypanosomatid diversity in Brazilian bats. PLoS Negl. Trop. Dis. 11:5790. doi: 10.1371/journal.pntd.0005790
Darling, J. A., and Mahon, A. R. (2011). From molecules to management: adopting DNA-based methods for monitoring biological invasions in aquatic environments. Environ. Res. 111, 978–988. doi: 10.1016/j.envres.2011.02.001
Didelot, X., Bowden, R., Wilson, D. J., Peto, T. E. A., and Crook, D. W. (2012). Transforming clinical microbiology with bacterial genome sequencing. Nat. Rev. Genet. 13, 601–612. doi: 10.1038/nrg3226
Dumonteil, E., Ramirez-Sierra, M.J., Pérez-Carrillo, S., Teh-Poot, C., Herrera, C., Gourbière, S., et al. (2018). Detailed ecological associations of triatomines revealed by metabarcoding and next-generation sequencing: implications for triatomine behavior and Trypanosoma cruzi transmission cycles. Sci. Rep. 8:4140. doi: 10.1038/s41598-018-22455-x
Epstein, J. H., and Anthony, S. J. (2017). Viral discovery as a tool for pandemic preparedness. Rev. Sci. Tech. 36, 499–512. doi: 10.20506/rst.36.2.2669
Fierer, N., Leff, J. W., Adams, B. J., Nielsen, U. N., Bates, S. T., Lauber, C. L., et al. (2012). Cross-biome metagenomic analyses of soil microbial communities and their functional attributes. Proc. Natl. Acad. Sci. U.S.A. 109, 21390–21395. doi: 10.1073/pnas.1215210110
Grey, E. K., Bernatchez, L., Cassey, P., Deiner, K., Deveney, M., Howland, K. L., et al. (2018). Effects of sampling effort on biodiversity patterns estimated from environmental DNA metabarcoding surveys. Sci. Rep. 8:8843. doi: 10.1038/s41598-018-27048-2
Gu, X., Tay, Q. X. M., Te, S. H., Saeidi, N., Goh, S. G., Kushmaro, A., et al. (2018). Geospatial distribution of viromes in tropical freshwater ecosystems. Water Res. 137, 220–232. doi: 10.1016/j.watres.2018.03.017
Gumiel, M., da Mota, F. F., Rizzo, V., de, S., Sarquis, O., Castro, D. P., Lima, M. M., et al. (2015). Characterization of the microbiota in the guts of Triatoma brasiliensis and Triatoma pseudomaculata infected by Trypanosoma cruzi in natural conditions using culture independent methods. Parasit. Vectors 8:245. doi: 10.1186/s13071-015-0836-z
Han, H. -J., Wen, H. -L., Zhou, C. -M., Chen, F. -F., Luo, L. -M., Liu, J., et al. (2015). Bats as reservoirs of severe emerging infectious diseases. Virus Res. 205, 1–6. doi: 10.1016/j.virusres.2015.05.006
Harvell, C. D., Mitchell, C. E., Ward, J. R., Altizer, S., Dobson, A. P., Ostfeld, R. S., et al. (2002). Climate warming and disease risks for terrestrial and marine biota. Science. 296, 2158–2162. doi: 10.1126/science.1063699
Hassell, J. M., Begon, M., Ward, M. J., and Fèvre, E. M. (2016). Urbanization and disease emergence: dynamics at the wildlife–Livestock–human interface. Trends Ecol. Evol. 32, 55–67. doi: 10.1016/j.tree.2016.09.012
Holmes, E. C. (2013). What can we predict about viral evolution and emergence? Curr. Opin. Virol. 3, 180–184. doi: 10.1016/j.coviro.2012.12.003
Holmes, E. C., Rambaut, A., and Andersen, K. G. (2018). Pandemics: spend on surveillance, not prediction. Nature 558, 180–182. doi: 10.1038/d41586-018-05373-w
Jerde, C. L., Mahon, A. R., Chadderton, W. L., and Lodge, D. M. (2011). “Sight-unseen” detection of rare aquatic species using environmental DNA. Conserv. Lett. 4, 150–157. doi: 10.1111/j.1755-263X.2010.00158.x
Jones, B. A., Grace, D., Kock, R., Alonso, S., Rushton, J., Said, M. Y., et al. (2013). Zoonosis emergence linked to agricultural intensification and environmental change. Proc. Natl. Acad. Sci.U.S.A. 110, 8399–8404. doi: 10.1073/pnas.1208059110
Jones, K. E., Patel, N. G., Levy, M. A., Storeygard, A., Balk, D., Gittleman, J. L., et al. (2008). Global trends in emerging infectious diseases. Nature 451, 990–993. doi: 10.1038/nature06536
Kocher, A., de Thoisy, B., Catzeflis, F., Valière, S., Bañuls, A. -L., and Murienne, J. (2017). iDNA screening: disease vectors as vertebrate samplers. Mol. Ecol. 26, 6478–6486. doi: 10.1111/mec.14362
Levinson, J., Bogich, T. L., Olival, K. J., Epstein, J. H., Johnson, C. K., Karesh, W., et al. (2013). Targeting surveillance for zoonotic virus discovery. Emerg. Infect. Dis. 19, 743–747. doi: 10.3201/eid1905.121042
Littlefair, J. E., and Clare, E. L. (2016). Barcoding the food chain: from Sanger to high-throughput sequencing. GENOME 59, 946–958. doi: 10.1139/gen-2016-0028
Lively, C. M., de Roode, J. C., Duffy, M. A., Graham, A. L., and Koskella, B. (2014). Interesting open questions in disease ecology and evolution. Am. Nat. 184(Suppl. 1), S1–8. doi: 10.1086/677032
Lohan, K. M. P., Fleischer, R. C., Carney, K. J., Holzer, K. K., and Ruiz, G. M. (2016). Amplicon-based pyrosequencing reveals high diversity of protistan parasites in ships' ballast water: implications for biogeography and infectious diseases. Microb. Ecol. 71, 530–542. doi: 10.1007/s00248-015-0684-6
Luo, C., Tsementzi, D., Kyrpides, N., Read, T., and Konstantinidis, K. T. (2012). Direct comparisons of illumina vs. Roche 454 sequencing technologies on the same microbial community DNA sample. PLoS ONE 7:e30087. doi: 10.1371/journal.pone.0030087
Luscombe, N. M., Greenbaum, D., and Gerstein, M. (2001). What is Bioinformatics? A proposed definition and overview of the Field. Method Inf. Med 4, 346–358. doi: 10.1055/s-0038-1634431
Mahon, A. R., Horton, D. J., Learman, D. R., Nathan, L. R., and Jerde, C. L. (2018). Investigating diversity of pathogenic microbes in commercial bait trade water. PeerJ 6:e5468. doi: 10.7717/peerj.5468
Mann, E., Streng, S., Bergeron, J., and Kircher, A. (2015). A review of the role of food and the food system in the transmission and spread of ebolavirus. PLoS Negl. Trop. Dis. 9:e0004160. doi: 10.1371/journal.pntd.0004160
McNeil, L. K., Reich, C., Aziz, R. K., Bartels, D., Cohoon, M., Disz, T., et al. (2007). The National Microbial Pathogen Database Resource (NMPDR): a genomics platform based on subsystem annotation. Nucleic Acids Res. 35, D347–D353. doi: 10.1093/nar/gkl947
Miller, R. R., Montoya, V., Gardy, J. L., Patrick, D. M., and Tang, P. (2013). Metagenomics for pathogen detection in public health. GENOME Med. 5:485. doi: 10.1186/gm485
Morse, S. S., Mazet, J. A., Woolhouse, M., Parrish, C. R., Carroll, D., Karesh, W. B., et al. (2012). Prediction and prevention of the next pandemic zoonosis. Lancet 380, 1956–1965. doi: 10.1016/S0140-6736(12)61684-5
Nakayima, J., Hayashida, K., Nakao, R., Ishii, A., Ogawa, H., Nakamura, I., et al. (2014). Detection and characterization of zoonotic pathogens of free-ranging non-human primates from Zambia. Parasit. Vectors 7:490. doi: 10.1186/s13071-014-0490-x
Narasimhan, S., Rajeevan, N., Liu, L., Zhao, Y. O., Heisig, J., Pan, J., et al. (2014). Gut microbiota of the tick vector ixodes scapularis modulate colonization of the lyme disease spirochete. Cell Host Microbe 15, 58–71. doi: 10.1016/J.CHOM.2013.12.001
Nieuwenhuijse, D. F., and Koopmans, M. P. (2017). Metagenomic sequencing for surveillance of food- and waterborne viral diseases. Front. Microbiol. 8:230. doi: 10.3389/fmicb.2017.00230
Nunn, C. L., and Altizer, S. M. (2005). The global mammal parasite database: an online resource for infectious disease records in wild primates. Evol. Anthropol. Issues News Rev. 14, 1–2. doi: 10.1002/evan.20041
Oakgrove, K. S., Harrigan, R. J., Loiseau, C., Guers, S., Seppi, B., and Sehgal, R. N. M. (2014). Distribution, diversity and drivers of blood-borne parasite co-infections in Alaskan bird populations. 44, 717–727. doi: 10.1016/j.ijpara.2014.04.011
Olds, B. P., Jerde, C. L., Renshaw, M. A., Li, Y., Evans, N. T., Turner, C. R., et al. (2016). Estimating species richness using environmental DNA. Ecol. Evol. 6, 4214–4226. doi: 10.1002/ece3.2186
Paliy, O., and Shankar, V. (2016). Application of multivariate statistical techniques in microbial ecology. Mol. Ecol. 25, 1032–1057. doi: 10.1111/mec.13536
Patz, J. A., Daszak, P., Tabor, G. M., Aguirre, A. A., Pearl, M., Epstein, J., et al. (2004). Unhealthy lanscapes: policy recommendations on land use change and infectious disease emergence. Environ. Health Perspect. 112, 1092–1098. doi: 10.1289/ehp.6877
Paull, S. H., Song, S., McClure, K. M., Sackett, L. C., Kilpatrick, A. M., and Johnson, P. T. (2012). From superspreaders to disease hotspots: linking transmission across hosts and space. Front. Ecol. Environ. 10, 75–82. doi: 10.1890/110111
Peng, M. M., Wilson, M. L., Holland, R. E., Meshnick, S. R., Lal, A. A., and Xiao, L. (2003). Genetic diversity of Cryptosporidium spp. in cattle in Michigan: implications for understanding the transmission dynamics. Parasitol. Res. 90, 175–180. doi: 10.1007/s00436-003-0834-5
Pompanon, F., Deagle, B. E., Symondson, W. O., Brown, D. S., Jarman, S. N., and Taberlet, P. (2012). Who is eating what: diet assessment using next generation sequencing. Mol. Ecol. 21, 1931–1950. doi: 10.1111/j.1365-294X.2011.05403.x
Poulin, R., and Morand, S. (2000). The diversity of parasites. Q. Rev. Biol., 277–293. doi: 10.1086/393500
Qiu, Y., Nakao, R., Ohnuma, A., Kawamori, F., and Sugimoto, C. (2014). Microbial population analysis of the salivary glands of ticks; a possible strategy for the surveillance of bacterial pathogens. PLoS ONE 9:0103961. doi: 10.1371/journal.pone.0103961
Ramey, A. M., Hill, N. J., Cline, T., Plancarte, M., De La Cruz, S., Casazza, M. L., et al. (2017). Surveillance for highly pathogenic influenza A viruses in California during 2014?2015 provides insights into viral evolutionary pathways and the spatiotemporal extent of viruses in the Pacific Americas Flyway. Emerg. Microbes Infect. 6:e80. doi: 10.1038/emi.2017.66
Rees, H. C., Maddison, B. C., Middleditch, D. J., Patmore, J. R. M., and Gough, K. C. (2014). The detection of aquatic animal species using environmental DNA - a review of eDNA as a survey tool in ecology. J. Appl. Ecol. 51, 1450–1459. doi: 10.1111/1365-2664.12306
Rosa, B. A., Supali, T., Gankpala, L., Djuardi, Y., Sartono, E., Zhou, Y., et al. (2018). Differential human gut microbiome assemblages during soil-transmitted helminth infections in Indonesia and Liberia. Microbiome 6:33. doi: 10.1186/s40168-018-0416-5
Ryan, U., Paparini, A., and Oskam, C. (2017). New technologies for detection of enteric parasites. Trends Parasitol. 33, 532–546. doi: 10.1016/J.PT.2017.03.005
Sakamoto, J. M., Ng, T. F. F., Suzuki, Y., Tsujimoto, H., Deng, X., Delwart, E., et al. (2016). Bunyaviruses are common in male and female Ixodes scapularis ticks in central Pennsylvania. PeerJ 4:2324. doi: 10.7717/peerj.2324
Schneeberger, K., and Voigt, C. C. (2016). “Zoonotic viruses and conservation of bats,” in Bats in the Anthropocene: Conservation of Bats in a Changing World (Cham: Springer International Publishing), 263–292.
Simner, P. J., Miller, S., and Carroll, K. C. (2018). Understanding the promises and hurdles of metagenomic next-generation sequencing as a diagnostic tool for infectious diseases. Clin. Infect. Dis. 66, 778–788. doi: 10.1093/cid/cix881
Taberlet, P., Coissac, E., Hajibabaei, M., and Rieseberg, L. H. (2012). Towards next-generation biodiversity assessment using DNA metabarcoding. Mol. Ecol. 21, 1789–1793. doi: 10.1111/j.1365-294X.2012.05542.x
Temmam, S., Davoust, B., Berenger, J. M., Raoult, D., and Desnues, C. (2014). Viral metagenomics on animals as a tool for the detection of zoonoses prior to human infection? Int. J. Mol. Sci. 15, 10377–10397. doi: 10.3390/ijms150610377
Thomsen, P. F., and Willerslev, E. (2015). Environmental DNA – An emerging tool in conservation for monitoring past and present biodiversity. Biol. Conserv. 183, 4–18. doi: 10.1016/j.biocon.2014.11.019
Thongsripong, P., Chandler, J. A., Green, A. B., Kittayapong, P., Wilcox, B. A., Kapan, D. D., et al. (2018). Mosquito vector-associated microbiota: metabarcoding bacteria and eukaryotic symbionts across habitat types in Thailand endemic for dengue and other arthropod-borne diseases. Ecol. Evol. 8, 1352–1368. doi: 10.1002/ece3.3676
Titcomb, G., Allan, B. F., Ainsworth, T., Henson, L., Hedlund, T., Pringle, R. M., et al. (2017). Interacting effects of wildlife loss and climate on ticks and tick-borne disease. Proc. R. Soc. B Biol. Sci. 284:20170475. doi: 10.1098/rspb.2017.0475
Urban, M., Pant, R., Raghunath, A., Irvine, A. G., Pedro, H., and Hammond-Kosack, K. E. (2015). The Pathogen-Host Interactions database (PHI-base): additions and future developments. Nucleic Acids Res. 43:D645–D655. doi: 10.1093/nar/gku1165
Villegas, L. E. M., Campolina, T. B., Barnabe, N. R., Orfano, A. S., Chaves, B. A., Norris, D. E., et al. (2018). Zika virus infection modulates the bacterial diversity associated with &ITAedes aegypti&IT as revealed by metagenomic analysis. PLoS ONE 13:0190352. doi: 10.1371/journal.pone.0190352
Wegleitner, B. J., Jerde, C. L., Tucker, A., Chadderton, W. L., and Mahon, A. R. (2015). Long duration, room temperature preservation of filtered eDNA samples. Conserv. Genet. Resour. 7, 789–791. doi: 10.1007/s12686-015-0483-x
Wilcox, B., and Ellis, B. (2006). Forests and emerging infectious diseases of humans. Unasylva 57, 11–18.
Williams, S. H., Che, X., Paulick, A., Guo, C., Lee, B., Muller, D., et al. (2018). New York City House Mice (Mus musculus) as potential reservoirs for pathogenic bacteria and antimicrobial resistance determinants. MBio 9, e00624–e00618. doi: 10.1128/mBio.00624-18
Young, H. S., McCauley, D. J., Dirzo, R., Nunn, C. L., Campana, M. G., Agwanda, B., et al. (2017). Interacting effects of land use and climate on rodent-borne pathogens in central Kenya. Philos. Trans. R. Soc. London B Biol. Sci. 372:20160116. doi: 10.1098/rstb.2016.0116
Zaiss, M. M., and Harris, N. L. (2016). Interactions between the intestinal microbiome and helminth parasites. Parasite Immunol. 38, 5–11. doi: 10.1111/pim.12274
Keywords: high-throughput (HT) approaches, emerging infectious disease (EID), wildlife-human interaction, metabarcoding analyses, metagenomic analyses, eDNA, pathogen diversity
Citation: Titcomb GC, Jerde CL and Young HS (2019) High-Throughput Sequencing for Understanding the Ecology of Emerging Infectious Diseases at the Wildlife-Human Interface. Front. Ecol. Evol. 7:126. doi: 10.3389/fevo.2019.00126
Received: 01 September 2018; Accepted: 28 March 2019;
Published: 16 April 2019.
Edited by:
Nichola J. Hill, Tufts University, United StatesReviewed by:
Luke McNally, University of Edinburgh, United KingdomInger Suzanne Prange, Appalachian Wildlife Research Institute (United States), United States
Copyright © 2019 Titcomb, Jerde and Young. This is an open-access article distributed under the terms of the Creative Commons Attribution License (CC BY). The use, distribution or reproduction in other forums is permitted, provided the original author(s) and the copyright owner(s) are credited and that the original publication in this journal is cited, in accordance with accepted academic practice. No use, distribution or reproduction is permitted which does not comply with these terms.
*Correspondence: Georgia C. Titcomb, Z2VvcmdpYXRpdGNvbWJAZ21haWwuY29t