- 1Department of Biology, Providence College, Providence, RI, United States
- 2Department of Biology, University of Richmond, Richmond, VA, United States
- 3Department of Ecology & Evolutionary Biology, Yale University, New Haven, CT, United States
- 4Instituto Gonçalo Moniz, Fundação Oswaldo Cruz, Ministério da Saúde, Bahia, Brazil
- 5Instituto de Saúde Coletiva, Universidade Federal da Bahia, UFBA, Bahia, Brazil
- 6Department of Epidemiology of Microbial Disease, Yale School of Public Health, Yale University, New Haven, CT, United States
Rats thrive in human-dominated landscapes and have expanded to a near global distribution. Norway rats (Rattus norvegicus) contaminate food, damage infrastructure, and are reservoirs for zoonotic pathogens that cause human diseases. To limit these negative impacts, entities around the world implement intervention and control strategies designed to quickly and drastically reduce the number of rats in a population. While the primary goal of these interventions is to reduce rat numbers and their detrimental activities, there are important, yet unexplored, population genetic implications for these rapid population declines. Here, we compare the population genetics of R. norvegicus before, immediately after, and several months following a rodenticide-based eradication campaign targeting rats in an urban slum of Salvador, Brazil. This slum has been the focus of long-term research designed to understand and reduce the risk of leptospirosis for people in this area. We also look for a clear source of rats contributing to population recovery, by either rebounding through breeding of local survivors, or by immigration/reinvasion of the site. We found evidence of severe genetic bottlenecks, with the effective population size dropping 85–91% after eradication, consistent with declines in population sizes. These rapid declines also led to a strong shift in the genetic structure of rats before and after the eradication campaign. Relatedness increased in two of the three study areas after eradication, suggesting reduced population sizes and an uneven impact of the campaign across colonies within the population. Lastly, dozens of low-frequency alleles (mean frequency of 0.037) observed before the campaign were undetected after the campaign, potentially lost from the population via drift or selection. We discuss the public health and ecological implications of these rapid genetic impacts of urban control efforts. Our data suggests that targeting the genetic viability of rat populations may be another important component for integrated pest management (IPM) strategies, designed to reduce urban rats.
Introduction
The Norway rat, or brown rat (Rattus norvegicus), is a pest species that is invasive in much of its near-global range. It is responsible for billions of dollars in damage annually to properties, city infrastructure, and food stocks (Stenseth et al., 2003; Pimentel et al., 2005). R. norvegicus is also a vector and reservoir of many zoonotic pathogens, and is therefore an important species for public health monitoring and control (Easterbrook et al., 2007; Himsworth et al., 2013b; Firth et al., 2014). As a result of their impact on agricultural losses, human health, infrastructure, and native ecosystems, brown rats are one of the most important nuisance species globally (Capizzi et al., 2014; Parsons et al., 2017).
R. norvegicus has been the target of many intervention campaigns to reduce their numbers and the risk they pose to people and ecosystems. These campaigns are designed to reduce rat numbers, control their geographic spread, or completely remove all rats from an area. Efforts to completely eradicate rats have been limited mostly to islands separated geographically from other populations that may serve as sources of recolonizing rats (Howald et al., 2007; Russell et al., 2010; Savidge et al., 2012). However, cities across the world invest heavily to control or reduce rat populations in close proximity to dense human settlements, even if complete eradication is unlikely (Bonnefoy et al., 2008; de Masi et al., 2009; Parsons et al., 2017). Intense control efforts, through chemical rodenticide intervention and lethal trapping, are commonly implemented in cities around the world.
Despite the money and time spent reducing their numbers, rat population sizes usually rebound rapidly in urban areas after a control campaign, a pattern known as the “boomerang effect” (Smith, 1963; de Masi et al., 2009). The rats that repopulate a treated area may come from residual individuals that were not removed during the initial intervention. Hastening this internal rebound of rats is the fact that the initial campaign reduces competition for resources among the remaining rats, increasing reproductive rates and juvenile recruitment (Smith, 1963; Stokes et al., 2009; Vadell et al., 2010). Alternatively, rats may re-colonize the targeted area from nearby, untargeted areas. During equilibrium periods, rats from other colonies and areas may have trouble penetrating into or establishing in the range of other populations due to the tight social structure of rat colonies. The competitive advantage for rats in established populations/colonies are known as priority effects, making the invasion of new rats difficult (Fraser et al., 2015). However, a reduction in rat numbers during intervention campaigns can disrupt these priority effects, making re-colonization by rats from other areas more likely (Pichlmueller and Russell, 2018). Currently, it is not understood what role local residual vs. outside colonizing rats contribute to the inevitable rebound of rat population sizes in an urban context.
While the number of rats may consistently rebound after a control campaign, very little is known about the genetic impacts of a population decline and subsequent recovery in urban rats. Genetic variation and effective population size (Ne) are expected to decline along with census population size. Also, genetic variation generally takes much longer to replace in a population than the rate at which it is lost (Nei et al., 1975). Researchers have applied tests of heterozygosity excess to look for historic genetic bottleneck events in urban rats (Gardner-Santana et al., 2009; Desvars-Larrive et al., 2018). However, these tests for bottlenecks have not been in the context of a rodent-control campaign. Further, data comparing the genetics of pre-control samples to rats present after the control campaign are only available for island systems (Abdelkrim et al., 2007; Russell et al., 2010; Pichlmueller and Russell, 2018). For most eradication campaigns, the decline in rats happens within weeks, and the recovery within months. This makes the commonly used heterozygosity tests less useful since they operate on a time scale of 2–4 times the Ne of a population (Piry et al., 1999). Given that lag time, Ne can be compared directly between pre- and post-control samples to detect any recent loss of genetic variation. Ne represents the size of an “ideal” population that can be expected to lose genetic diversity (through genetic drift) at the same rate as the focal population, which often has a very different census population size (Frankham, 1995; Kalinowski and Waples, 2002; Husemann et al., 2016). Ne is also considered a much better indicator of the genetic status and evolutionary fitness of a population (Reed, 2005). In addition to genetic bottlenecks, rapid population declines can also result in shifts in the genetic structure of a population. Rats are a colonial species with close kin relationships with other rats in their colonies. Eradication campaigns likely disrupt these social structures (Clapperton, 2006), which have the potential to alter the relatedness levels of individuals repopulating an area post-intervention. Lethal control of rats has been linked to novel social interactions and transmission of pathogens (Himsworth et al., 2013a; Lee et al., 2018).
In this study, we took advantage of an intense eradication campaign that occurred in 2015 in Salvador, Brazil, a city of 2,900,000 people that has experienced a 500% increase in human population size in the last 60 years. Much of this increase has happened in favela (slum) settlements, with little sanitation infrastructure, high populations of rats, and elevated risk of zoonotic diseases for residents (Reis et al., 2008; Felzemburgh et al., 2014; Hagan et al., 2016). Here, we focus on Pau da Lima, a favela community targeted for rat eradication because it has experienced high levels of human leptospirosis infection (Ko et al., 1999; Reis et al., 2008). We sampled a total of 241 rats before (n = 132) and after (n = 109) the 2015 eradication campaign, genotyped them at 16 microsatellite loci, and conducted analyses to look for the degree of genetic bottlenecking that occurred. In addition, we analyzed if and how the population genetic structure shifted after the campaign, and if there was any signature of a disruption of colony systems through relatedness metrics. To our knowledge, this study represents the first investigation of genetic impacts of rodent control efforts in cities.
Methods
Study Area and Sampling
We designed our sampling protocol around a rat-control intervention occurring within the Pau da Lima area of Salvador, Brazil in 2015. Pau da Lima is a favela with a high human population density and low socioeconomic status of residents, with 88% being considered squatters without a legal right to residence (Reis et al., 2008). This neighborhood has been the focus of long-term epidemiological research trying to lower the risk of leptospirosis for human inhabitants (Costa et al., 2014; Felzemburgh et al., 2014). It is comprised of four connected valleys, and the samples in this study were collected from three of these valleys (Valley 1, 2, and 4; Figure 1); Valley 3 has not been part of the larger long-term eco-epidemiological study (Panti-May et al., 2016). Previous research has found genetic divisions between the valleys (Kajdacsi et al., 2013; Richardson et al., 2017), as well as multiple paternity and high relatedness among mating pairs (Costa et al., 2016).
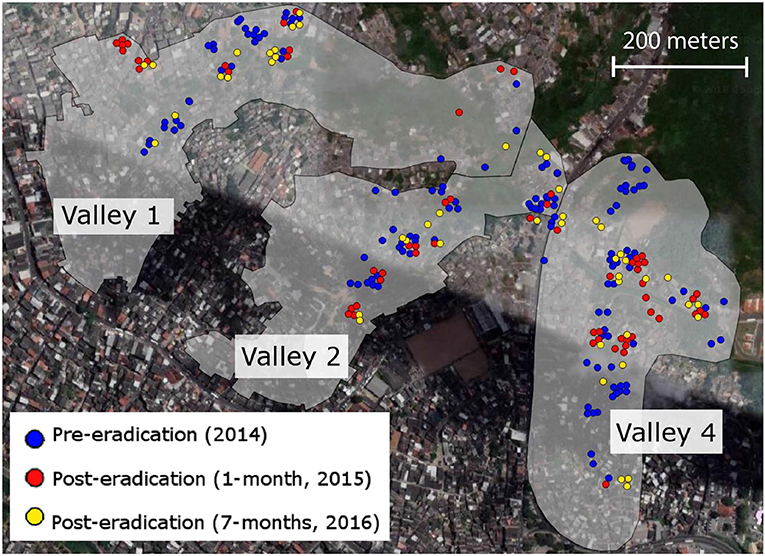
Figure 1. The Pau da Lima section of Salvador, Brazil is comprised of four valleys, three of which were the target of an eradication campaign in mid-2015. We collected tissue samples from 241 rats trapped immediately before the campaign (blue points), and then 1-month (red points) and 7-months (gold points) after the campaign. These three valleys have been the focus of long-term epidemiological work to reduce human incidence of leptospirosis carried by Rattus norvegicus rats.
We sampled 241 rats across the three Pau da Lima valleys, at three separate time points around a planned eradication campaign, using both live and snap traps (Figure 1). Between October and December 2014, we set traps at 108 sites across Pau da Lima and captured 34 rats from Valley 1, 47 rats from Valley 2, and 51 from Valley 4, representing 40 of the 108 trapping locations. The city of Salvador then conducted a lethal rat-control campaign between June and August of 2015. The intervention was performed by the Zoonotic Control Center (ZCC) of the Salvador city Secretary of Health. The ZCC's campaign included three pulses of rodenticide application as well as pre- and post-evaluation of rodent infestation, following visual-survey methodologies described previously in Brazil (de Masi et al., 2009) and other countries (Davis et al., 1977; Murphy and Oldbury, 2002). During a pre-campaign survey, the ZCC found that 39% of the households in the treatment area had signs of rodent infestation. This number dropped significantly to 21.1% (p < 0.05) after the campaign, indicating a 54% reduction in rat infestation in the evaluated households. We then trapped again 1 month after the eradication campaign ended, collecting 64 rats across the three valleys over a 3.5-week period between November and December of 2015 (Figure 1). Seven months after the campaign ended (March to April 2016), we collected tissue samples from another 45 rats trapped in the same areas of the three valleys (Figure 1). Trap sites at all time periods were geo-referenced and tail tissue was collected and stored in ethanol at −80°C until the DNA was extracted.
DNA Extraction and Genotyping
DNA was extracted from 2 to 5 mm tail tissue, using standard kit-based extraction protocols (Qiagen and ZyGEM). We then amplified 16 microsatellite loci previously identified as polymorphic in Rattus spp. using a touchdown PCR protocol (Kajdacsi et al., 2013). PCR amplicons were identified using capillary electrophoresis on an ABI 3730 DNA sequencer. GeneMarker software was used to score alleles and Microsatellite Toolkit v3.1 (Park, 2001) was used to check for scoring errors.
While loci were selected across chromosomes to prevent linkage disequilibrium, we evaluated whether loci conformed to Hardy-Weinberg equilibrium (HWE) for each locus in the R package “pegas” (Paradis, 2010), with significance estimated using 1,000 randomizations. Since the Pau da Lima valleys are unlikely to be a single gene pool, we separated each valley and year, and took the average HWE significance and tabulated the number of valley/year combinations. In doing so, only one locus (D5Cebr1) was identified as out of HWE and was therefore not used for subsequent analyses.
Genetic Bottleneck Analyses
We first looked for changes in the effective population size (Ne) in each valley before and after the eradication campaign. Even though rat populations rebound quickly after a control campaign, those census numbers do not reflect the genetic variation remaining in the population post-decline. The Ne is often much lower than the census population size for most species (Kalinowski and Waples, 2002; Husemann et al., 2016), averaging 10% of census size (Frankham, 1995). Ne is also a better indicator of the genetic viability and fitness of a population (Reed, 2005). In addition, our three sampling periods occurred over 16 months, or ~3–6 rat generations. We used the change in Ne as our metric of genetic bottleneck levels because methods such as heterozygosity excess in the program BOTTLENECK, and M-ratio measures of allele number and size range variance detect bottleneck events over longer time spans (2–4*Ne) than are relevant for our study (Piry et al., 1999; Garza and Williamson, 2001; Peery et al., 2012).
We estimated Ne using two approaches that utilize different properties of population genetics. We first estimated Ne using sibship assignment methods, based on the level of relatedness among individuals within a population (Wang, 2004, 2009). This analysis calculates sibship frequency (SF) levels, and was done in Colony2 using the recommended settings and a genotyping error rate of 0.01 (Jones and Wang, 2010). We also used NeEstimator (Do et al., 2014), which calculates Ne based on levels of linkage disequilibrium (LD). As Ne declines, genetic drift increases non-random associations among loci, thereby increasing signatures of LD (Hill, 1981; Waples, 2006). We used the random mating model and a threshold allele frequency of 0.02 (Waples and Do, 2008). Although both approaches require only a single sampling event, and have been shown to accurately estimate true Ne with sufficient sample sizes and polymorphic genetic loci (Wang, 2009; Waples and Do, 2010), the SF approach can be more accurate and robust to assumptions inherent to each estimate (Wang, 2016).
Discriminant Analysis of Genetic Structure
We used discriminant analysis of principal components (DAPC) to evaluate the overall genetic structure within the three Pau da Lima valleys, and if there were any changes in this structure after the eradication campaign. DAPC uses coefficients of locus allele loadings in linear combinations (PC axes) to maximize the variance between-groups while minimizing within-group variances in these loadings (Jombart et al., 2010). Empirical and simulated data have found that DAPC performs as well or better than other individual-based clustering methods [e.g., STRUCTURE; (Pritchard et al., 2000)], particularly when complex processes are operating (Jombart et al., 2010; Klaassen et al., 2012). In this analysis, we use DAPC to characterize basic genetic differences rather than to delineate new genetic groups. We used guidelines in the “adegenet” package v2.1.1 in R to conduct DAPC (Jombart et al., 2016). We retained the number of principal components necessary to explain ~90% of the cumulative variance in each DAPC run, which ranged from 35 to 40 PCs. We also retained a single discriminant function for each run.
Relatedness
Norway rats exhibit colonial social systems, where individuals within a colony are more related to each other than at random among the population (Calhoun, 1963; Costa et al., 2016). However, drastic reductions in population size may disrupt this social structure, leading to shifts in relatedness if some colonies are hit harder than others, or if the residual individuals remaining after the campaign come from a subset of colonies and inbreeding increases (Calhoun, 1963). We estimated the level of relatedness for each pair of rats (i.e., dyad) in our dataset, within each time period sampled (i.e., pre-eradication, as well as 1- and 7-months post-eradication). We used the R package “related” to calculate both the Queller and Goodnight (Queller and Goodnight, 1989) and the dyad maximum likelihood (dyadML; Milligan, 2003) metrics of relatedness. Each is commonly used in the literature, with attendant benefits and caveats (Milligan, 2003; Goudet et al., 2018). We then used the R package “dplyr” to calculate summary statistics for the distribution of relatedness values in each valley and sampling period, and the packages “ggplot2” and “ggpubr” to plot the density distributions and regressions of both the Queller and dyadML metrics. Lastly, we performed a Kruskal-Wallis rank sum test in the base “stats” package of R, followed by pairwise Wilcoxon tests to determine which of the three distributions in each valley (i.e., which sampling time period) were significantly different from each of the other two.
Rare Allele Loss
During population bottlenecks and founder events, alleles are expected to be lost at a rate proportional to their frequency in the population (Maruyama and Fuerst, 1985). Through genetic drift, rare alleles are more likely to be lost than high frequency alleles, which concomitantly tend to increase in frequency during a bottleneck (Luikart et al., 1998). We calculated the frequency of every allele for each locus in each valley at each time period using the “readGenepop” function within the “diversity” package in R. We then identified the alleles that dropped out of the dataset between the pre- and post-eradication sampling periods and calculated the frequency of those alleles separately.
Results
Genetic Bottleneck Analyses
There was a sharp drop in the effective population size (Ne) between the pre and post-eradication samples for each valley, with the sibship frequency (SF) estimation (Figure 2A). The Ne in Valley 1 decreased by 74% between the pre-eradication and 1-month post-eradication sampling period. The Ne in Valley 2 decreased by 65% and in Valley 4 by 42% during that same period (Figure 2A, blue vs. red bars). The Ne remained low or decreased further in the second post-eradication population, sampled 7 months following the campaign (Figure 2A, gold bars). Ne estimates based on linkage disequilibrium (LD) levels showed similar patterns, with Valley 1 and Valley 2 experiencing a 91% and 85% drop, respectively, in Ne after the eradication campaign (Figure 2B). Ne remained low during the samples collected seven months after the campaign. Valley 4 did not show an immediate decline in LD-based Ne, as it increased by 16% between the first two sampling periods (Figure 2B, blue vs. red bars). However, consistent with all other valleys, there was an 85% decrease in Ne between the pre- and 7 months post-eradication sampling period (Figure 2B; gold bar).
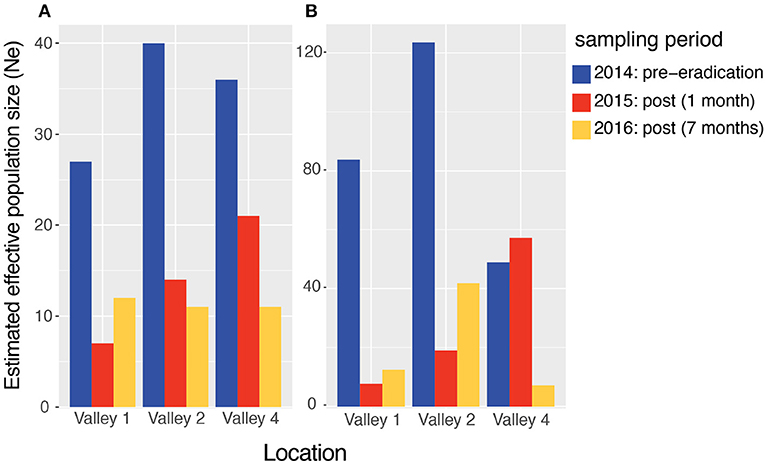
Figure 2. Point estimates of effective population size (Ne) in three valleys of the Pau da Lima area of Salvador, Brazil. 241 rats were sampled just before the city conducted an eradication campaign to reduce the rat population (late-2014, blue bars), 1 month following the campaign (2015, red bars), and then 7-months later (early-2016, gold bars). Ne was estimated using two different methods: (A) the sibship frequency and (B) linkage disequilibrium approaches. Full data can be found in Table S1.
To ensure that the Ne reductions were not an artifact of sample sizes that vary across the three sampling periods, we performed a rarefaction analysis and found that the results did not change qualitatively when we reduced the number of samples analyzed, to the minimum number collected at any time point. In fact, the reductions in Ne were larger in several cases. We also calculated Ne using combined genotype data from the two post-intervention sampling periods, but again, the results did not differ qualitatively. These data suggest that there are severe genetic consequences for urban rats that experience rapid population declines during lethal control initiatives.
Discriminant Analysis of Genetic Structure
There was a clear shift in the genetic structure of the rats in each valley before and after the eradication campaign (Figure 3). In each valley, the two post-eradication rat genotypes overlap significantly along the discriminant function axis. Post-eradication genotypes have minimal overlap with pre-eradication rat genotypes, suggesting that there was a rapid and intense change in the genetics of rat populations in each valley. The genotype discriminant distribution curve—a measure of genetic variation—showed less variation in Valley 1 than Valley 2 or Valley 4 (Figure 3). As with the Ne analysis above, we also ran a DAPC using combined genotype data from the two post-intervention sampling periods, in case the smaller sample sizes for each post-eradication period could shift results. But again, the results did not differ qualitatively from the default DAPCs done with each valley-time interval combination.
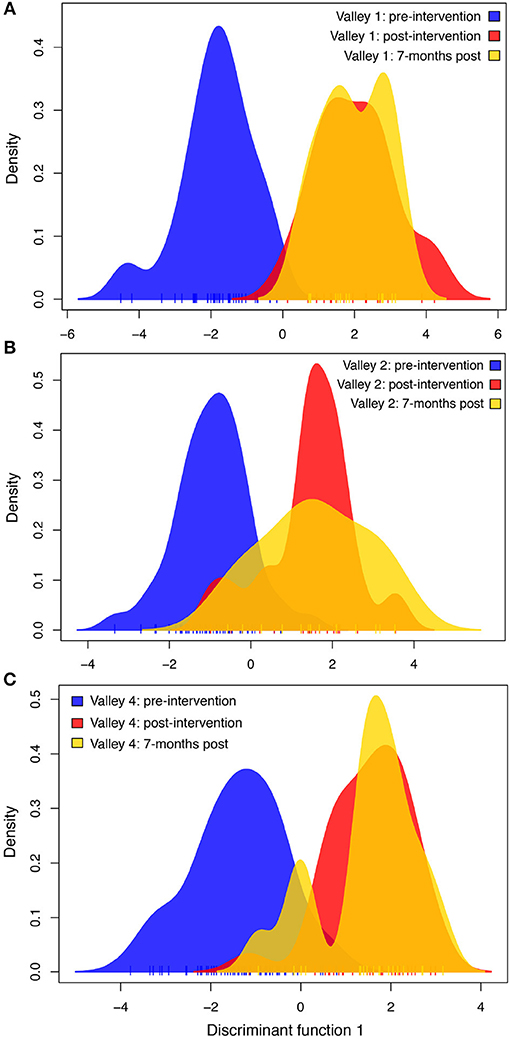
Figure 3. Discriminant analysis of principal components (DAPC) for (A) Valley 1, (B) Valley 2, and (C) Valley 4 at three time points—immediately before the chemical intervention campaign, 1 month following the campaign, and 7 months following the campaign. Each plot displays the first discriminant function on the x-axis, and the density of genotypes on the y-axis. Increasing distance on the x-axis between peak densities represents increasing genetic differences between samples at those time points. In all three valleys, the mean genetic signature of rats shifted after the chemical intervention (i.e., separation between blue curve and the red and yellow post-intervention curves). This difference persisted over the 7 months after the intervention campaign, despite demographic changes in the populations.
Relatedness
Valley 1 showed the highest levels of relatedness across all sampling periods, but the distributions of these values did not differ among the three time points sampled (Figure 4), suggesting no significant shift in the relatedness levels among rats in any sampling period. Rats from Valley 2 showed a significant increase in relatedness 1 month after the eradication campaign (Figure 5). This increase disappeared, though, 7 months after the campaign ended and the population rebounded (Figure 5D). Rats from Valley 4 also exhibited a significant increase in relatedness after the eradication campaign, which rose further 7 months after the campaign ceased (Figure 6). For all three valleys, the two measures of relatedness corresponded closely to each other (Figures 4B, 5B, 6B).
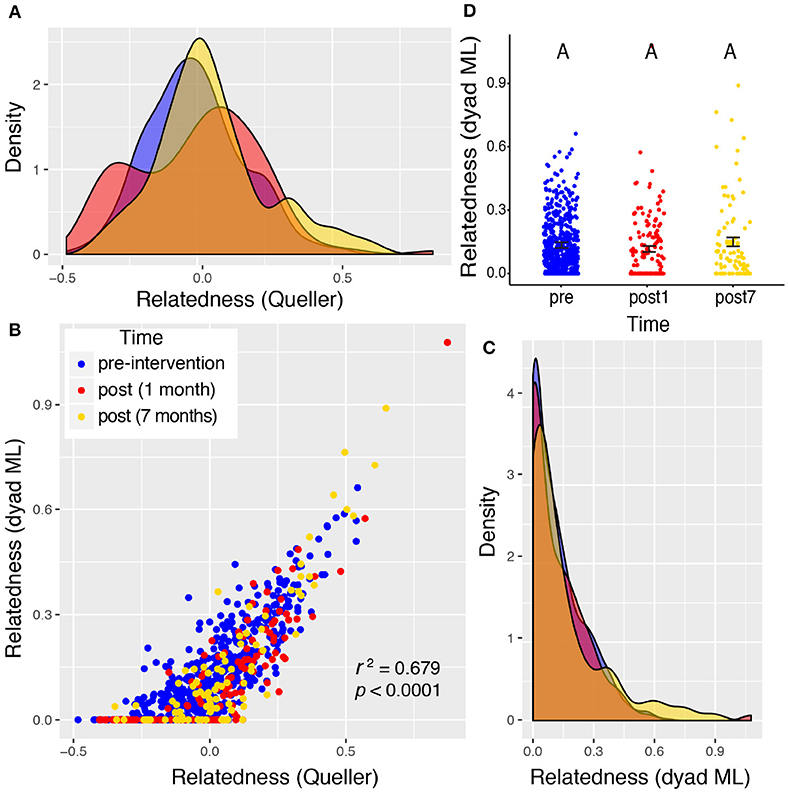
Figure 4. Valley 1: two very different measures of relatedness suggest that there was no significant change in the distribution of relatedness between individual rats sampled pre- and post-eradication in Valley 1, represented by density distributions below (A,C). The Queller-Goodnight relatedness coefficient can take negative values (A), while the Milligan dyad maximum likelihood (dyad ML) metric is bounded by zero and one (C). The two metrics are tightly correlated in this data set (B). A Kruskal–Wallis test was used to determine if the distribution of relatedness values differed among the three time points sampled around the 2015 eradication campaign (D). The upper-case letters above the points in panel (D) are all the same, indicating that there is no significant difference between the distributions of relatedness pre- and post-eradication. Black bars represent the mean ± 1SE.
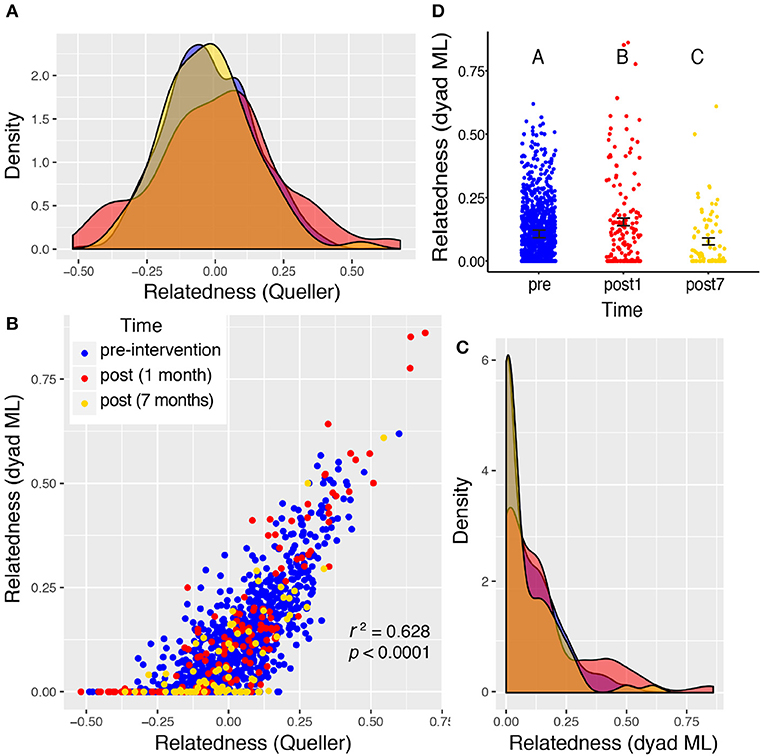
Figure 5. Valley 2: two very different measures of relatedness suggest that there is a significant change in the distribution of relatedness between individual rats sampled pre- and post-eradication in Valley 2, represented by density distributions below (A,C). The Queller-Goodnight relatedness coefficient can take negative values (A), while the Milligan dyad maximum likelihood (dyad ML) metric is bounded by zero and one (C). The two metrics are tightly correlated in this data set (B). A Kruskal–Wallis test was used to determine if the distribution of relatedness values differed among the three time points sampled around the 2015 eradication campaign (D). The upper-case letters above the points in panel (D) indicate that there is a significant difference between all three distributions of relatedness among the one pre- and two post-eradication periods. Black bars represent the mean ± 1SE.
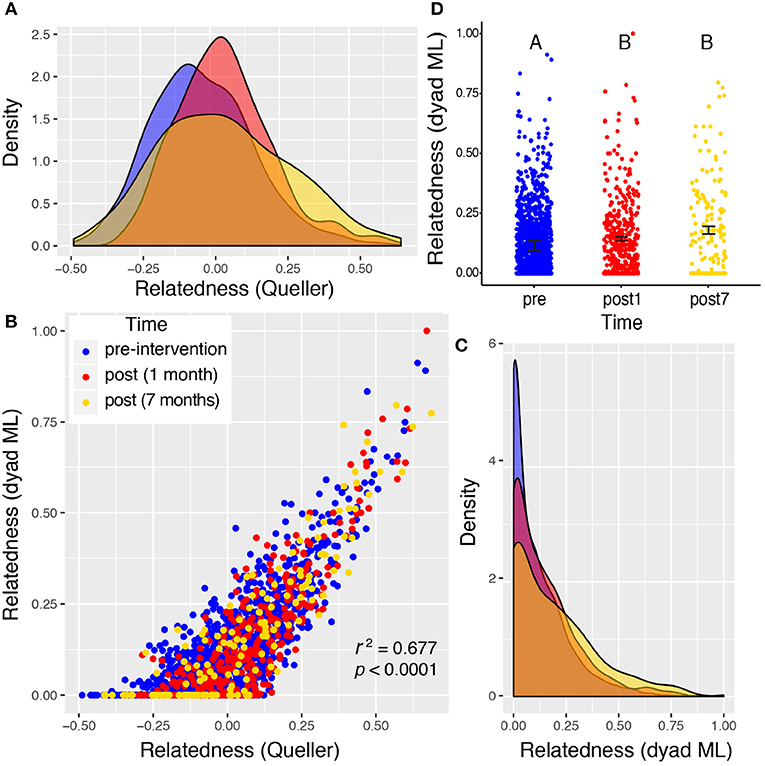
Figure 6. Valley 4: two very different measures of relatedness suggest that there is a significant change in the distribution of relatedness between individual rats sampled pre- and post-eradication in Valley 4, represented by density distributions below (A,C). The Queller-Goodnight relatedness coefficient can take negative values (a), while the Milligan dyad maximum likelihood (dyad ML) metric is bounded by zero and one (C). The two metrics are tightly correlated in this data set (B). A Kruskal–Wallis test was used to determine if the distribution of relatedness values differed among the three time points sampled around the 2015 eradication campaign (D). The upper-case letters above the points in panel (D) indicate that there is a significant difference between the distributions of relatedness from the pre- and post-eradication periods, but the two post-eradication periods are not significantly different from each other. Black bars represent the mean ± 1SE.
Rare Allele Loss
The average overall allele frequency across all alleles in the dataset before the eradication campaign was 0.164, meaning that each individual allele constituted, on average, 16.4% of the alleles observed across all genotypes at a locus. The pre-eradication frequency of just the alleles that dropped out after the eradication campaign was much lower at 0.037. This indicates that rare alleles were much more likely to be lost from the population, likely through genetic drift. Though it should be noted they may have been present in the population, but went undetected in our later sampling (i.e., if those alleles were not present in our sampled rats).
Discussion
Cities around the world have implemented intervention campaigns to reduce rat population sizes, usually in the form of lethal trapping or chemical eradication campaigns. But the genetic impacts of the control efforts on the remaining rat populations have not received much research attention. Here we find that an eradication campaign in Salvador, Brazil conducted in 2015 had rapid and severe genetic consequences for rats in three sections of the Pau da Lima favela. The effective population size declined between 85 and 91% after the eradication across the three sites (Figure 2), concurrent with a large shift in genetic structure (Figure 3) and the loss of dozens of alleles that had been at low frequency in the population. The levels of relatedness among the rats also increased significantly after eradication for two of the three locations. These data suggest that there are very likely to be long-term evolutionary implications to rat populations subject to control measures, even if their census numbers rebound quickly following the intervention campaigns.
Rapid Genetic Bottlenecks During Lethal Control
Effective population size (Ne) is a comprehensive measure of genetic variation and the loss of such variation results from drift. Importantly it provides a much better measure of the long-term genetic viability of a population than census population size, accounting for the genetic variation available to serve as the grist of future evolution to changing conditions (Dlugosch and Parker, 2008). If genetic variation in the population is severely decreased during a rat-control campaign, the effective population size would also decrease relative to pre-eradication numbers (Vucetich et al., 1997). The magnitude of this decline indicates how severely the gene pool of the rat population was impacted by the control campaign.
In Salvador, the Ne dropped 42–74% between the pre- and post-eradication campaign sampling periods using the sibship frequency method. All three valleys show immediate drops in Ne 1 month after the eradication, and these declines persisted or further decreased 7 months post-eradication (Figure 2A). The reduction in Ne was much sharper using the linkage-disequilibrium approach, falling 85–91% between the pre- and post-eradication periods (Figure 2B). However, in Valley 4 the decrease was not seen until 7 months post-campaign.
Despite the “boomerang effect” commonly seen when rat population sizes rebound after the control campaign has ended (Smith, 1963; de Masi et al., 2009), genetic variation lost during these bottleneck events takes much longer to return, through a combination of immigration and new mutations arising (Nei et al., 1975). If an urban rat population is repeatedly subjected to bottlenecks in population size—and the attendant genetic bottlenecks—during frequent control campaigns, the levels of genetic variation lost should accumulate during each successive bottleneck. However, the drastic reduction in Ne we observed (42–91%, depending on measure and valley) in Salvador with just a single lethal-control campaign suggests that genetic variation is lost exceedingly fast. This would leave very little genetic variation in the populations for future evolution, and increases the risk of deleterious fitness consequences from genetic drift and inbreeding depression (Briskie and Mackintosh, 2004; Spielman et al., 2004).
Targeting the genetic variation of rat populations with the goal of increasing the risks associated with inbreeding, depression may be one avenue for urban pest control and integrated pest management (IPM) strategies (Hone, 1995; Kuriwada et al., 2010; Li et al., 2015). However, this is the same fundamental genetic process observed when a small number of founder individuals colonize a new area or island, with little evidence of deleterious effects on population growth from these founder effects, as seen with invasive rats and birds on islands (Abdelkrim et al., 2005; Low et al., 2018). Little research has been done on the relative fitness of urban rats, let alone how genetics factors into that fitness. It is possible that rat populations do suffer some fitness consequences after control campaigns reduce population sizes and allelic diversity. For example, both selection and drift can play a role in rare allele loss for important immune response loci in the major histocompatibility complex (MHC) (Sutton et al., 2011; Taylor et al., 2012). Alternatively, strong natural selection in cities may have quickly purged deleterious alleles as part of, or independent from, eradication campaigns.
Disruption of Population Genetic Structure
The eradication campaign in 2015 led to a large, abrupt shift in population genetic structure of the rats in all three valleys (Figure 3). This change in the genetic signature persisted or increased between the 1 and 7-months post-eradication sampling periods, as denoted along the discriminant function axis of the DAPC plot (Figure 3). This population genetic shift indicates that the genetic variation remaining after the eradication bottleneck is not a random subset of the gene pool present before the campaign. Population genetic structure around an eradication event has not received much research attention. However, Abdelkrim et al. (2007) used F-statistics and assignment probabilities (as measures of population structure) of individual rats remaining after an eradication of rats from two islands off the coast of Martinique, and found varying degrees of genetic structure and an increase in genetic diversity, suggesting a role for both internal rebound and recolonization on the two islands. Russell et al. (2010) compared the genetics of 51 samples from two species of rats on two islands near New Zealand, and found that the genetic structure before and after the control was consistent with those rats reinvading from the larger neighboring island, rather than internal rebound. Yet both internal rebound and new invasions from a mainland population played a role in increasing rat numbers on another small island after a 2012 control campaign (Pichlmueller and Russell, 2018). We extend this previous work on islands to an urban context, using DAPC and a large sample of 241 rats collected around an intense eradication campaign. We highlight the rapid shifts in population genetic structure possible with an intense population bottleneck. To explore the relative roles of internal rebound vs. outside dispersers in repopulating the area, we also conducted a supplemental DAPC analysis that included all rats from each valley at each time point. This analysis showed that valleys became more different genetically after the eradication campaign (Figure S1), indicating that rebounding rats are unlikely to be the result of large-scale immigration of dispersing rats from other, genetically dissimilar, areas.
Increasing Relatedness and Fitness Consequences
Norway rats exhibit a colonial social structure, where individuals in a colony are more closely related to each other than rats across the rest of the population (Costa et al., 2016). Given this social context, individuals may exhibit higher levels of relatedness after an eradication campaign if some colonies survive at higher rates and the residual breeding pairs are more related to each other than pre-eradication (i.e., a precursor to inbreeding). For example, if lethal baiting and trapping is deployed in a way that does not uniformly cover the focal area, this can lead to differential survival among colonies. Alternatively, if the eradication impacted all areas and colonies equally, then relatedness would not be expected to increase initially, though a gene pool with less overall genetic variation could still impact the fitness of the remaining population. The rodenticide application in 2015 in Pau da Lima was designed to be uniformly distributed across Valleys 1 and 4, with incidental application in Valley 2 based on leptospirosis risk. In practice, logistics associated with access to households and permission of residents may have led to deviations in the intended uniform distribution of the rodenticides applied. Logistical constraints are a reality in any urban control effort.
Relatedness levels increase in all three valleys after the eradication campaign. The distribution of relatedness values (across all pairs of rats within a sampling period) increased significantly in the post-eradication rat population for valleys 2 and 4 (Figures 5, 6). This same trend was seen in Valley 1, but not statistically significant (Figure 4). This pattern occurred using both the dyad maximum likelihood and the Queller & Goodnight metrics of relatedness, and those two metrics were tightly associated with each other (Figures 4B, 5B, 6B). The elevated relatedness among rats remaining after the control campaigns represents another risk for inbreeding in these post-eradication populations. Increasing relatedness can have fitness consequences, and has been found to reduced reproductive success, competitive ability and growth rates in invasive plant species (Burns, 2006; Elam et al., 2007). Yet there is evidence that some social animals prefer inbreeding despite documented deleterious impacts, such as elevated susceptibility to disease in American crows (Townsend et al., 2018). This raises the question of what tradeoffs exist between tight social relationships, inclusive fitness, and inbreeding depression. It is possible that urban rats benefit from sharing colonies with closely related individuals (e.g., territory defense, stable social structure), and may avoid some inbreeding tradeoffs by having relatively accelerated reproductive life cycles. One benefit may be less diverse parasite and pathogen communities harbored by isolated rats, and dispersal and culling can disrupt these relatively insular colonies (Angley et al., 2018; Lee et al., 2018; Minter et al., 2019).
Implications for Urban Rat Management
The current study is part of a multi-disciplinary effort to reduce the risk of leptospirosis for Pau da Lima residents, and a primary strategy is to reduce the population of Norway rats that serve as reservoirs of this bacterial pathogen (Ko et al., 1999; Costa et al., 2014; Richardson et al., 2017). Cities around the world are focused on reducing rat populations for reasons related to public health, quality of life, and protection of infrastructure (Bonnefoy et al., 2008; de Masi et al., 2009; Angley et al., 2018). The lethal control campaigns typically implemented are often successful at reducing the number of rats in the targeted areas yet are generally followed by a period of rapid population increase—the “boomerang effect” (Smith, 1963; de Masi et al., 2009). For that reason, a multifaceted IPM approach is needed to have longer-lasting impacts in reducing rat numbers. In addition to the lethal removal of rats, the amount of trash and other food sources need to be reduced. Habitat that can serve as harborage for rats also need to be managed, and infrastructure needs to be improved to prevent them from entering buildings.
The results of the current study also indicate that long-term genetic viability of urban rat populations may be another component relevant for any IPM strategy. If a single eradication campaign in Salvador can result in up to a 91% decline in effective population size, significant increases in relatedness, and the loss of dozens of rare alleles, then repeated campaigns may leave the remaining rat population genetically depauperate enough that severe inbreeding depression will eventually impart substantial fitness consequences. Over time, reduced fitness should erode a population's ability to reproduce at high rates and rebound after the bottleneck. These genetic impacts may be attenuated if immigration occurs from other populations with distinct genetic signatures, though previous research did not find evidence of this dispersal into Pau da Lima (Richardson et al., 2017).
In a larger evolutionary sense, repeated bottlenecks will leave less genetic variation available for the population to evolve and adapt in response to changing environments. This may be a particularly important handicap for urban-dwelling rodents, because cities impose strong and diverse natural selection pressures known to impact rodents (Donihue and Lambert, 2015; Harris and Munshi-South, 2017; Johnson and Munshi-South, 2017) and at the microgeographic spatial scales relevant for heterogeneous urban environments (Richardson et al., 2014). Genetic insights into rats provide another important dimension to our understanding of their use of and movement through the urban landscape, especially when compared across multiple cities (Richardson et al., 2016; Combs et al., 2018). For that reason, we recommend that genetic impacts on, and long-term viability of urban pest species, be considered as part of any comprehensive intervention campaign and IPM strategy.
Ethics Statement
The ethics committee for the use of animals from the Oswaldo Cruz Foundation, Salvador, Brazil approved the protocols used in this study (protocol number 003/2012), which adhered to the guidelines of the American Society of Mammalogists for the use of wild mammals in research and the guidelines of the American Veterinary Medical Association for the euthanasia of animals. These protocols were also approved by the Yale University Institutional Animal Care and Use Committee (IACUC), New Haven, Connecticut (protocol number 2012–11498).
Author Contributions
FC, AK, JC, AC, and JR designed the study. AP, CM, IS, AZ, TC, and JR conducted trapping, genetic assays, and data collection. JR and GS analyzed the data. JR wrote the article with input from FC, AC, AK, JC, and GS.
Conflict of Interest Statement
The authors declare that the research was conducted in the absence of any commercial or financial relationships that could be construed as a potential conflict of interest.
Acknowledgments
We thank the staff of Fiocruz and the Zoonosis Control Center in Salvador for their assistance in conducting the study. We thank the collaborative groups from the Urban Health Council of Pau da Lima, including residents and community leaders, as well as the Oswaldo Cruz Foundation. J. Shirvell, B. Kajdacsi, C. Hernandez, and C. Manole all contributed to the genotype data generation in this study. This work was funded by a U.S. National Science Foundation grant to JR (1738789), the Oswaldo Cruz Foundation and Secretariat of Health Surveillance, Brazilian Ministry of Health; the Wellcome Trust (102330/Z/13/Z); and a NSF-NIH grant from the Ecology and Evolution of Infection Diseases (EEID) program (1 R01 TW009504) and NIH grants (5 R01 AI052473, 5 U01 AI088752, 1 R25 TW009338, 1 R01 AI121207, F31 AI114245. Comments from Matt Combs, Jeff Markert, Lianne Bisch, and two reviewers greatly improved this manuscript.
Supplementary Material
The Supplementary Material for this article can be found online at: https://www.frontiersin.org/articles/10.3389/fevo.2019.00115/full#supplementary-material
References
Abdelkrim, J., Pascal, M., Calmet, C., and Samadi, S. (2005). Importance of assessing population genetic structure before eradication of invasive species: examples from Insular Norway rat populations. Conserv. Biol. 19, 1509–1518. doi: 10.1111/j.1523-1739.2005.00206.x
Abdelkrim, J., Pascal, M., and Samadi, S. (2007). Establishing causes of eradication failure based on genetics: case study of ship rat eradication in Ste. Anne archipelago. Conserv. Biol. 21, 719–730. doi: 10.1111/j.1523-1739.2007.00696.x
Angley, L. P., Combs, M., Firth, C., Frye, M. J., Lipkin, I., Richardson, J. L., et al. (2018). Spatial variation in the parasite communities and genomic structure of urban rats in New York City. Zoonoses Public Health 65, e113–e123. doi: 10.1111/zph.12418
Bonnefoy, X., Kampen, H., and Sweeney, K. (2008). Public Health Significance of Urban Pests. Copenhagen: World Health Organization (WHO).
Briskie, J. V., and Mackintosh, M. (2004). Hatching failure increases with severity of population bottlenecks in birds. Proc Natl Acad Sci U.S.A. 101, 558–561. doi: 10.1073/pnas.0305103101
Burns, J. H. (2006). Relatedness and environment affect traits associated with invasive and noninvasive introduced Commelinaceae. Ecol. Appl. 16, 1367–1376. doi: 10.1890/1051-0761(2006)016[1367:RAEATA]2.0.CO;2
Calhoun, J. B. (1963). The Ecology and Sociology of the Norway rat. Bethesda, MD: U.S. Dept. of Health, Education, and Welfare, Public Health Service. doi: 10.5962/bhl.title.112283
Capizzi, D., Bertolino, S., and Mortelliti, A. (2014). Rating the rat: global patterns and research priorities in impacts and management of rodent pests. Mamm. Rev. 44, 148–162. doi: 10.1111/mam.12019
Clapperton, B. K. (2006). A Review of the Current Knowledge of Rodent Behaviour in Relation to Control Devices. Wellington: New Zealand Department of Conservation.
Combs, M., Byers, K. A., Ghersi, B. M., Blum, M. J., Caccone, A., Costa, F., et al. (2018). Urban rat races: spatial population genomics of brown rats (Rattus norvegicus) compared across multiple cities. Proc. R. Soc. B Biol. Sci. 285:20180245. doi: 10.1098/rspb.2018.0245
Costa, F., Ribeiro, G. S., Felzemburgh, R. D., Santos, N., Reis, R. B., Santos, A. C., et al. (2014). Influence of household rat infestation on leptospira transmission in the urban slum environment. PLoS Negl. Trop. Dis. 8:e3338. doi: 10.1371/journal.pntd.0003338
Costa, F., Richardson, J. L., Dion, K., Mariani, C., Pertile, A. C., Burak, M. K., et al. (2016). Multiple paternity in the Norway Rat, Rattus norvegicus, from Urban Slums in Salvador, Brazil. J. Heredity 107, 181–186. doi: 10.1093/jhered/esv098
Davis, H., Casta, A., and Schatz, G. (1977). Urban Rat Surveys. Washington, DC: US Department of Health, Education, and Welfare.
de Masi, E., Vilaça, P. J., and Razzolini, M. T. P. (2009). Evalua on on the effectiveness of actions for controlling infestation by rodents in Campo Limpo region, Sao Paulo Municipality, Brazil. Int. J. Environ. Health Res. 19, 291–304. doi: 10.1080/09603120802592723
Desvars-Larrive, A., Hammed, A., Hodroge, A., Berny, P., Benoît, E., Lattard, V., et al. (2018). Population genetics and genotyping as tools for planning rat management programmes. J. Pest Sci. 92, 691–705. doi: 10.1007/s10340-018-1043-4
Dlugosch, K. M., and Parker, I. M. (2008). Founding events in species invasions: genetic variation, adaptive evolution, and the role of multiple introductions. Mol. Ecol. 17, 431–449. doi: 10.1111/j.1365-294X.2007.03538.x
Do, C., Waples, R. S., Peel, D., Macbeth, G. M., Tillett, B. J., and Ovenden, J. R. (2014). NeEstimator v2: re-implementation of software for the estimation of contemporary effective population size (Ne) from genetic data. Mol. Ecol. Resour. 14, 209–214. doi: 10.1111/1755-0998.12157
Donihue, C. M., and Lambert, M. R. (2015). Adaptive evolution in urban ecosystems. Ambio 44, 194–203. doi: 10.1007/s13280-014-0547-2
Easterbrook, J. D., Kaplan, J. B., Vanasco, N. B., Reeves, W. K., Purcell, R. H., Kosoy, M. Y., et al. (2007). A survey of zoonotic pathogens carried by Norway rats in Baltimore, Maryland, U. S. A. Epidemiol. Infect. 135, 1192–1199. doi: 10.1017/S0950268806007746
Elam, D. R., Ridley, C. E., Goodell, K., and Ellstrand, N. C. (2007). Population size and relatedness affect fitness of a self-incompatible invasive plant. Proc. Natl. Acad. Sci. U.S.A. 104, 549–552. doi: 10.1073/pnas.0607306104
Felzemburgh, R. D., Ribeiro, G. S., Costa, F., Reis, R. B., Hagan, J. E., Melendez, A. X., et al. (2014). Prospective study of leptospirosis transmission in an urban slum community: role of poor environment in repeated exposures to the Leptospira agent. PLoS Negl. Trop. Dis. 8:e2927. doi: 10.1371/journal.pntd.0002927
Firth, C., Bhat, M., Firth, M. A., Williams, S. H., Frye, M. J., Simmonds, P., et al. (2014). Detection of zoonotic pathogens and characterization of novel viruses carried by commensal rattus norvegicus in New York city. MBio 5. doi: 10.1128/mBio.01933-14
Frankham, R. (1995). Effective population size/adult population size ratios in wildlife: a review. Genet. Res. 66, 95–107. doi: 10.1017/S0016672300034455
Fraser, C. I., Banks, S. C., and Waters, J. M. (2015). Priority effects can lead to underestimation of dispersal and invasion potential. Biol. Invasions 17, 1–8. doi: 10.1007/s10530-014-0714-1
Gardner-Santana, L. C., Norris, D. E., Fornadel, C. M., Hinson, E. R., Klein, S. L., and Glass, G. E. (2009). Commensal ecology, urban landscapes, and their influence on the genetic characteristics of city-dwelling Norway rats (Rattus norvegicus). Mol. Ecol. 18, 2766–2778. doi: 10.1111/j.1365-294X.2009.04232.x
Garza, J. C., and Williamson, E. G. (2001). Detection of reduction in population size using data from microsatellite loci. Mol. Ecol. 10, 305–318. doi: 10.1046/j.1365-294x.2001.01190.x
Goudet, J., Kay, T., and Weir, B. S. (2018). How to estimate kinship. Mol. Ecol. 27, 4121–4135. doi: 10.1111/mec.14833
Hagan, J. E., Moraga, P., Costa, F., Capian, N., Ribeiro, G. S., Wunder, E. A., et al. (2016). Spatiotemporal determinants of Urban leptospirosis transmission: four-year prospective cohort study of slum residents in Brazil. PLoS Negl. Trop. Dis. 10:e0004275. doi: 10.1371/journal.pntd.0004275
Harris, S. E., and Munshi-South, J. (2017). Signatures of positive selection and local adaptation to urbanization in white-footed mice (Peromyscus leucopus). Mol. Ecol. 26, 6336–6350. doi: 10.1111/mec.14369
Hill, W. G. (1981). Estimation of effective population size from data on linkage disequilibrium. Genet. Res. 38, 209–216. doi: 10.1017/S0016672300020553
Himsworth, C. G., Bidulka, J., Parsons, K. L., Feng, A. Y., Tang, P., Jardine, C. M., et al. (2013a). Ecology of Leptospira interrogans in Norway rats (Rattus norvegicus) in an inner-City Neighborhood of Vancouver, Canada. PLoS Negl. Trop. Dis. 7:2270. doi: 10.1371/journal.pntd.0002270
Himsworth, C. G., Parsons, K. L., Jardine, C., and Patrick, D. M. (2013b). Rats, cities, people, and pathogens: a systematic review and narrative synthesis of literature regarding the ecology of rat-associated zoonoses in urban centers. Vector Borne Zoonotic Dis. 13, 349–359. doi: 10.1089/vbz.2012.1195
Hone, J. (1995). Spatial and temporal aspects of vertebrate pest damage with emphasis on feral pigs. J. Appl. Ecol. 32:311.
Howald, G., Donlan, C. J., Galván, J. P., Russell, J. C., Parkes, J., Samaniego, A., et al. (2007). Invasive rodent eradication on Islands. Conserv. Biol. 21, 1258–1268. doi: 10.1111/j.1523-1739.2007.00755.x
Husemann, M., Zachos, F. E., Paxton, R. J., and Habel, J. C. (2016). Effective population size in ecology and evolution. Heredity 117, 191–192. doi: 10.1038/hdy.2016.75
Johnson, M. T. J., and Munshi-South, J. (2017). Evolution of life in urban environments. Science 358:eaam8327. doi: 10.1126/science.aam8327
Jombart, T., Devillard, S., and Balloux, F. (2010). Discriminant analysis of principal components: a new method for the analysis of genetically structured populations. BMC Genet. 11:94. doi: 10.1186/1471-2156-11-94
Jombart, T., Kamvar, Z. N., Lustrik, R., et al. (2016). Adegenet: an R package for the Exploratory Analysis of Genetic and Genomic Data. R package version 2.0.1.
Jones, O. R., and Wang, J. (2010). COLONY: a program for parentage and sibship inference from multilocus genotype data. Mol. Ecol. Resour. 10, 551–555. doi: 10.1111/j.1755-0998.2009.02787.x
Kajdacsi, B., Costa, F., Hyseni, C., Porter, F., Brown, J., Rodrigues, G., et al. (2013). Urban population genetics of slum-dwelling rats (Rattus norvegicus) in Salvador, Brazil. Mol. Ecol. 22, 5056–5070. doi: 10.1111/mec.12455
Kalinowski, S. T., and Waples, R. S. (2002). Relationship of effective to census size in fluctuating populations. Conserv. Biol. 16, 129–136. doi: 10.1046/j.1523-1739.2002.00134.x
Klaassen, C. H., Gibbons, J. G., Fedorova, N. D., Meis, J. F., and Rokas, A. (2012). Evidence for genetic differentiation and variable recombination rates among Dutch populations of the opportunistic human pathogen Aspergillus fumigatus. Mol. Ecol. 21, 57–70. doi: 10.1111/j.1365-294X.2011.05364.x
Ko, A. I., Reis, M. G., Dourado, C. M. R., Johnson, W. D., and Riley, L. W. (1999). Urban epidemic of severe leptospirosis in Brazil. Lancet 354, 820–825. doi: 10.1016/S0140-6736(99)80012-9
Kuriwada, T., Kumano, N., Shiromoto, K., and Haraguchi, D. (2010). Effect of mass rearing on life history traits and inbreeding depression in the sweetpotato Weevil (Coleoptera: Brentidae). J. Econ. Entomol. 103, 1144–1148. doi: 10.1603/EC09361
Lee, M. J., Byers, K. A., Donovan, C. M., Bidulka, J. J., Stephen, C., Patrick, D. M., et al. (2018). Effects of culling on leptospira interrogans carriage by rats. Emerg. Infect. Dis. 24, 356–360. doi: 10.3201/eid2402.171371
Li, S. P., Cadotte, M. W., Meiners, S. J., Hua, Z. S., Shu, H. Y., Li, J. T., et al. (2015). The effects of phylogenetic relatedness on invasion success and impact: deconstructing Darwin's naturalisation conundrum. Ecol. Lett. 18, 1285–1292.
Low, G. W., Chattopadhyay, B., Garg, K. M., Irestedt, M., Ericson, P., Yap, G., et al. (2018). Urban landscape genomics identifies fine-scale gene flow patterns in an avian invasive. Heredity 120, 138–153. doi: 10.1038/s41437-017-0026-1
Luikart, G., Allendorf, F. W., Cornuet, J. M., and Sherwin, W. B. (1998). Distortion of allele frequency distributions provides a test for recent population bottlenecks. J. Heredity 89, 238–247. doi: 10.1093/jhered/89.3.238
Maruyama, T., and Fuerst, P. A. (1985). Population bottlenecks and nonequilibrium models in population genetics. II. Number of alleles in a small population that was formed by a recent bottleneck. Genetics 111, 675–689.
Minter, A., Himsworth, C. G., Byers, K. A., Childs J. E., Ko, A. I., Costa, A. et al. (2019). Tails of two cities: age and wounding are associated with carriage of leptospira interrogans by Norway Rats (Rattus norvegicus) in ecologically distinct Urban environments. Front. Ecol. Evol. 7:14. doi: 10.3389/fevo.2019.00014
Murphy, R. G., and Oldbury, D. J. (2002). “Rat control by local authorities within the UK,” in Proceedings of the 4th International Conference on Urban Pests (ICUP), eds S. C. Jones, J. Zhai, and W. H. Robinson (Charleston), 351–355.
Nei, M., Maruyama, T., and Chakraborty, R. (1975). The bottleneck effect and genetic variability in populations. Evolution 29, 1–10. doi: 10.1111/j.1558-5646.1975.tb00807.x
Panti-May, J. A., Carvalho-Pereira, T. S. A., Serrano, S., Pedra, G. G., Taylor, J., Pertile, A. C., et al. (2016). A two-year ecological study of Norway rats (Rattus norvegicus) in a Brazilian Urban Slum (G Chapouthier, Ed,). PLoS ONE 11:e0152511. doi: 10.1371/journal.pone.0152511
Paradis, E. (2010). pegas: an R package for population genetics with an integrated?modular approach. Bioinformatics 26, 419–420. doi: 10.1093/bioinformatics/btp696
Parsons, M. H., Banks, P. B., Deutsch, M. A., Corrigan, R. F., and Munshi-South, J. (2017). Trends in urban rat ecology: a framework to define the prevailing knowledge gaps and incentives for academia, pest management professionals (PMPs) and public health agencies to participate. J. Urban Ecol. 3:jux005. doi: 10.1093/jue/jux005
Peery, M. Z., Kirby, R., Reid, B. N., Stoelting, R., Doucet-Bëer, E., Robinson, S., et al. (2012). Reliability of genetic bottleneck tests for detecting recent population declines. Mol. Ecol. 21, 3403–3418. doi: 10.1111/j.1365-294X.2012.05635.x
Pichlmueller, F., and Russell, J. C. (2018). Survivors or reinvaders? Intraspecific priority effect masks reinvasion potential. Biol. Conserv. 227, 213–218. doi: 10.1016/j.biocon.2018.09.020
Pimentel, D., Zuniga, R., and Morrison, D. (2005). Update on the environmental and economic costs associated with alien-invasive species in the United States. Ecol. Econ. 52, 273–288. doi: 10.1016/j.ecolecon.2004.10.002
Piry, S., Luikart, G., and Cornuet, J.-M. (1999). Computer note. BOTTLENECK: a computer program for detecting recent reductions in the effective size using allele frequency data. J. Heredity 90, 502–503. doi: 10.1093/jhered/90.4.502
Pritchard, J. K., Stephens, M., and Donnelly, P. (2000). Inference of population structure using multilocus genotype data. Genetics 155, 945–959.
Queller, D. C., and Goodnight, K. F. (1989). Estimating relatedness using genetic-markers. Evolution 43, 258–275. doi: 10.1111/j.1558-5646.1989.tb04226.x
Reed, D. H. (2005). Relationship between population size and fitness. Conserv. Biol. 19, 563–568. doi: 10.1111/j.1523-1739.2005.00444.x
Reis, R. B., Ribeiro, G. S., Felzemburgh, R. D., Santana, F. S., Mohr, S., Melendez, A. X., et al. (2008). Impact of environment and social gradient on Leptospira infection in urban slums. PLoS Negl. Trop. Dis. 2:e228. doi: 10.1371/journal.pntd.0000228
Richardson, J. L., Brady, S. P., Wang, I. J., and Spear, S. F. (2016). Navigating the pitfalls and promise of landscape genetics. Mol. Ecol. 25, 849–863. doi: 10.1111/mec.13527
Richardson, J. L., Burak, M. K., Hernandez, C., Shirvell, J. M., Mariani, C., Carvalho-Pereira, T. S., et al. (2017). Using fine scale spatial genetics of Norway rats to improve control efforts and reduce leptospirosis risk in urban slum environments. Evol. Appl. 10, 323–337. doi: 10.1111/eva.12449
Richardson, J. L., Urban, M. C., Bolnick, D. I., and Skelly, D. K. (2014). Microgeographic adaptation and the spatial scale of evolution. Trends Ecol. Evol. 29, 165–176. doi: 10.1016/j.tree.2014.01.002
Russell, J. C., Miller, S. D., Harper, G. A., MacInnes, H. E., Wylie, M. J., and Fewster, R. M. (2010). Survivors or reinvaders? Using genetic assignment to identify invasive pests following eradication. Biol. Invasions 12, 1747–1757. doi: 10.1007/s10530-009-9586-1
Savidge, J. A., Hopken, M. W., Witmer, G. W., Jojola, S. M., Pierce, J. J., and Burke, P. W. (2012). Genetic evaluation of an attempted Rattus eradication on Congo Cay, U.S. Virgin Islands, identifies importance of eradication units. Biol. Invasions 14, 2343–2354. doi: 10.1007/s10530-012-0233-x
Smith, L. (1963). An experimental rat eradication program in an urban area. Public Health Rep. 78, 807–811. doi: 10.2307/4591943
Spielman, D., Brook, B. W., Briscoe, D. A., and Frankham, R. (2004). Does inbreeding and loss of genetic diversity decrease disease resistance? Conserv. Genet. 5, 439–448. doi: 10.1023/B:COGE.0000041030.76598.cd
Stenseth, N. C., Leirs, H., Skonhoft, A., Davis, S. A., Pech, R. P., Andreassen, H. P., et al. (2003). Mice, rats, and people: the bio-economics of agricultural rodent pests. Front. Ecol. Environ. 1, 367–375. doi: 10.1890/1540-9295(2003)001[0367:MRAPTB]2.0.CO;2
Stokes, V. L., Banks, P. B., Pech, R. P., and Spratt, D. M. (2009). Competition in an invaded rodent community reveals black rats as a threat to native bush rats in littoral rainforest of south-eastern Australia. J. Appl. Ecol. 46, 1239–1247. doi: 10.1111/j.1365-2664.2009.01735.x
Sutton, J. T., Nakagawa, S., Robertson, B. C., and Jamieson, I. G. (2011). Disentangling the roles of natural selection and genetic drift in shaping variation at MHC immunity genes. Mol. Ecol. 20, 4408–4420. doi: 10.1111/j.1365-294X.2011.05292.x
Taylor, S. S., Jenkins, D. A., and Arcese, P. (2012). Loss of Mhc and neutral variation in peary caribou: genetic drift is not mitigated by balancing selection or exacerbated by mhc allele distributions (A Stow, Ed.). PLoS ONE 7:e36748. doi: 10.1371/journal.pone.0036748
Townsend, A. K., Taff, C. C., Jones, M. L., Getman, K. H., Wheeler, S. S., Hinton, M. G., et al. (2018). Apparent inbreeding preference despite inbreeding depression in the American crow. Mol. Ecol.
Vadell, M. V., Cavia, R., and Suárez, O. V. (2010). Abundance, age structure and reproductive patterns of Rattus norvegicus and Mus musculus in two areas of the city of Buenos Aires. Int. J. Pest Manag. 56, 327–336. doi: 10.1080/09670874.2010.499479
Vucetich, J. A., Waite, T. A., and Nunney, L. (1997). Fluctuating population size and the ratio of effective to census population size. Evolution 51, 2017–2021. doi: 10.1111/j.1558-5646.1997.tb05123.x
Wang, J. (2004). Sibship reconstruction from genetic data with typing errors. Genetics 166, 1963–1979. doi: 10.1534/genetics.166.4.1963
Wang, J. (2009). A new method for estimating effective population sizes from a single sample of multilocus genotypes. Mol. Ecol. 18, 2148–2164. doi: 10.1111/j.1365-294X.2009.04175.x
Wang, J. (2016). A comparison of single-sample estimators of effective population sizes from genetic marker data. Mol. Ecol. 25, 4692–4711. doi: 10.1111/mec.13725
Waples, R. S. (2006). A bias correction for estimates of effective population size based on linkage disequilibrium at unlinked gene loci*. Conserv. Genet. 7, 167–184. doi: 10.1007/s10592-005-9100-y
Waples, R. S., and Do, C. (2008). LDNE: a program for estimating effective population size from data on linkage disequilibrium. Mol. Ecol. Resour. 8, 753–756. doi: 10.1111/j.1755-0998.2007.02061.x
Keywords: genetic bottleneck, eradication, invasive species, public health, vector control, intervention, integrated pest management, Rattus
Citation: Richardson JL, Silveira G, Soto Medrano I, Arietta AZ, Mariani C, Pertile AC, Carvalho Pereira T, Childs JE, Ko AI, Costa F and Caccone A (2019) Significant Genetic Impacts Accompany an Urban Rat Control Campaign in Salvador, Brazil. Front. Ecol. Evol. 7:115. doi: 10.3389/fevo.2019.00115
Received: 29 November 2018; Accepted: 21 March 2019;
Published: 06 June 2019.
Edited by:
Michael H. Parsons, Fordham University, United StatesReviewed by:
Wayne C. Zipperer, United States Forest Service (USDA), United StatesEmily Puckett, University of Memphis, United States
Copyright © 2019 Richardson, Silveira, Soto Medrano, Arietta, Mariani, Pertile, Carvalho Pereira, Childs, Ko, Costa and Caccone. This is an open-access article distributed under the terms of the Creative Commons Attribution License (CC BY). The use, distribution or reproduction in other forums is permitted, provided the original author(s) and the copyright owner(s) are credited and that the original publication in this journal is cited, in accordance with accepted academic practice. No use, distribution or reproduction is permitted which does not comply with these terms.
*Correspondence: Jonathan L. Richardson, jrichardson4@gmail.com