- 1Section of Animal Ecology, Department of Ecological Science, Vrije Universiteit Amsterdam, Amsterdam, Netherlands
- 2Community and Conservation Ecology Group, Groningen Institute of Evolutionary Life Science, Rijksuniversiteit Groningen, Groningen, Netherlands
Extreme climatic events can have profound effects on ecosystems. Climate change is causing an increase in the frequency and intensity of extreme events, which raises the probability that natural ecosystems will be exposed to subsequent or simultaneous extreme events. Exposure to multiple extreme events may involve very different abiotic stressors, which makes it hard to compare their consequences for more than a single or a few species, limiting our knowledge of community level effects. Here, we propose a novel approach to assess how different abiotic stressors influenced soil arthropod communities in a coastal ecosystem, using a species abundance time series of more than 2 years. We first correlated shifts in community composition with the climatic conditions preceding the sampling dates. Temperature was found to be the most important factor influencing community composition in both locations. Based on local meteorological data, we then defined five types of extreme events which occurred during our monitoring period: heat, cold, drought, heavy precipitation, and high sea water levels. To compare the effect of different types of extreme climatic events on community composition, we calculated the multidimensional Hellinger distance between two subsequent sampling dates, which is a measure for the compositional dissimilarity between communities. Extreme events were expected to result in a larger change in community composition between sampling dates and thus a larger Hellinger distance. However, no significant difference in Hellinger distance was found for intervals with or without extreme events in three out of the four location-vegetation combinations. In the saline location with an open vegetation type we found that Hellinger's distance was reduced when extreme events had occurred, which is discussed in the light of the buffering potential of different vegetation types. Our study illustrates the novel use of an established method from the community ecological toolbox to facilitate direct comparison of different types of extreme climatic events on community composition. We highly encourage other ecologists with long-term monitoring datasets to perform similar analyses and test the general applicability of this method.
Introduction
With ongoing climate change, the intensity and frequency of various extreme climatic events will increase (Rahmstorf and Coumou, 2011; Hansen et al., 2012). Extreme events can be defined as climatic conditions exceeding variation in expected abiotic conditions based on historic conditions. Global heat maxima have steadily risen over the last decades, while at the same time, events of heavy rainfall and prolonged periods of drought have been increasing in prevalence, and this trend is predicted to continue for the years to come (Easterling, 2000). When surpassing tolerance levels, extreme events will have a severe impact on ecological communities with negative consequences for species, including altered growth rate and increased mortality (Grant et al., 2017; Ummenhofer and Meehl, 2017). However, species may differ in their climatic tolerance (Chown and Terblanche, 2006; Berg et al., 2010; Araújo et al., 2013; Franken et al., 2018), and some species will be able to cope with, or even benefit from the extreme conditions. Large interspecific differences in tolerance to climatic extremes will affect species composition of the entire community, either directly or indirectly via altered competition, predator-prey interactions, trophic cascades, and both bottom-up and top-down effects (Jentsch and Beierkuhnlein, 2008; Traill et al., 2010; van der Putten et al., 2010; Jiguet et al., 2011; Sentis et al., 2013; Cheng and Grosholz, 2016).
Ecosystems are usually not subjected to just one extreme event. They can be sequentially exposed to the same or to different types of climatic extremes, and in the latter case these exposures can even occur simultaneously, all of which may exert diverse effects on species and communities (Turnbull and Lindo, 2015; Bailey and van de Pol, 2016; Marrot et al., 2017). A clear example of multiple stressors occurring simultaneously is the combination of heat and drought, which has previously been found to have interactive effects on communities (Sheik et al., 2011; Dreesen et al., 2012). Gaining insight in the response of natural communities to different climatic stressors is essential to understand interactive effects of simultaneous or sequential exposure to different extreme events (Paine et al., 1998; Dreesen et al., 2014).
In order to be able to compare the effect of different extreme events across locations, study systems, and types of stressor involved, there is an urgent need for a common yardstick to measure community response. Such a yardstick may be provided by community ecological statistical methods, which are commonly employed to monitor entire communities over time. For instance, a statistical approach often applied to analyse data on species abundances over time is to calculate the (dis)similarity between ecological communities (Borcard et al., 2011; Tebby et al., 2017). When (dis)similarities are based only on community data, i.e., by using unconstrained ordination techniques, these distances are calculated independent of the abiotic conditions to which the community was exposed. We use a novel interpretation of the distance of the (dis)similarities between subsequent sample dates in multidimensional space (Ernest and Brown, 2001; Thibault and Brown, 2008) and propose that the relative effects of different extreme events on community composition can be directly compared using these distances.
In this study, we applied this novel interpretation of community dissimilarity to a species-abundance over time dataset of soil arthropod communities in a coastal ecosystem. This ecosystem is formed by a vegetated beach in front of the dunes. It is therefore exposed not only to stressors such as heat and drought, but also to inundation with salt water, which is a specific stressor for coastal systems and thus extends across ecosystem boundaries (Witteveen, 1988; Schlacher et al., 2008; Bolhuis et al., 2013; Schoeman et al., 2014). The arthropod communities were monitored for more than 2 years with frequent sampling intervals on two locations that differed in frequency of inundation with sea water. One of those locations was further subdivided in three vegetation types that differed in vegetation structure, plant height, and soil coverage, which may impact the effect of climate extremes on soil communities by providing micro-habitats (Scheffers et al., 2014; Woods et al., 2015; Keppel et al., 2017; Franken et al., 2018). Using species abundance of these natural communities over time, we could link the changes of community composition to events of abiotic stress in the field which allowed us to answer the following two main questions: (i) Which abiotic factors have the strongest influence on soil fauna community composition in vegetated beaches, and does this differ between the locations and vegetation types? (ii) Is the dissimilarity in species composition between communities at subsequent sampling dates larger if there was an extreme event in the interval between sampling dates?
Materials and Methods
Field Locations
Soil arthropod communities were sampled in two locations across a vegetated zone on the sandy beach, so-called “green beach,” on the Dutch barrier island of Schiermonnikoog. This green beach has been formed after a large sand deposit in the 1990's (Bakker et al., 2005). We selected two locations along this green beach, which differed markedly in abiotic, geomorphological conditions, and vegetation. The first location is the north side of the island (N53.49788, E6.16238, 2.25 ± 0.05 m above sea level). The vegetation zone we sampled was situated on the beach close to the base of the dunes, from which fresh water is seeping into the vegetation zone on the green beach. New, primary dunes have formed in front of this vegetated zone, acting as a barrier against sea water inundation and together with rain water this makes the soil moisture conditions brackish to fresh with low salt concentrations (0.64 ± 0.04 mS cm−1, measured on 24 October 2012), therefore, we refer to this location throughout the text as the “brackish” location. Together, this situation gives rise to a relatively homogeneous vegetation structure and composition. The vegetation was in a succession state called a primary dune slack, harboring a community of relatively salt-sensitive species Agrostis sp., Samolus valerandi L., Salix repens L., Lythrum salicaria L., Hypochaeris radicata L., several moss species (Bryophyta sp.), and a low abundance of Glaux maritima L. In contrast, the sampling location on the southwestern side of the island was positioned in a vegetated zone further away from the base of the dunes (N53.47308, E6.13368, ~1.55 m above sea level) and because of its lower elevation and the absence of primary dunes this location was more frequently inundated with sea water. Due to the frequent inundations and absence of freshwater seepage from the dunes, the salinity of the groundwater was much higher than at the brackish location (9.12 ± 1.1 mS cm−1, measured on 24 October 2012) and we refer to this location as the “saline” location. The vegetation consisted of more salt-tolerant species, such as Carex extensa Gooden, Puccinellia maritima (Huds.) Parl., Plantago maritima L., Aster tripolium L., Spartina anglica x towsendii C.E.Hubb., Limonium vulgare Mill., and a high abundance of Glaux maritima L. Moreover, there was a strong heterogeneity in vegetation structure, especially plant height and soil coverage, resulting in three distinctive vegetation types. Patches of dense and tall tussocks of Carex extensa, fine grassy vegetation patches with Puccinella sp. and Festuca rubra L., and patches which were more open with microbial algal mats and Glaux maritima. Because of these differences in the “saline” location we subsampled these three vegetation types (N = 3 per vegetation type, per sampling date), whereas we randomly sampled in the “brackish” location (N = 5 per sampling date). In total, this resulted in 14 samples per sampling date. The locations, and the corresponding salinity measurements are visualized in Supporting Online Information 1.
Sampling, Animal Extraction, and Taxonomic Identification
Soil animals were collected using soil core sampling with approximately 6-week intervals at both locations from the 21st of February 2013 until the 23rd of April 2015, resulting in 17 sampling dates, eight in 2013, seven in 2014, and two in 2015. The samples were taken by gently twisting and pressing a metal soil corer of ø 10 cm at least 8 cm into the soil. Care was taken not to compress the soil cores, ensuring an optimal extraction of soil fauna later on. Of the soil core, the top 5 cm of soil and any vegetation growing on top of the core was transferred to a ø 10 cm plastic container with lid. The sealed containers were then transferred to the Vrije Universiteit Amsterdam for extraction of the soil fauna.
Soil cores were placed upside-down in Tullgren extraction funnels, which heat up the bottom of the soil cores (now the top side) and cool the vegetated (under) side of the core. The obtained gradient in temperature and drought forced soil animals to crawl down and fall down a funnel, into a vial with 96% ethanol in which they are preserved for further identification. Previous studies have shown that with this method >90% of the soil fauna is extracted in 3 weeks' time (van Straalen and Rijninks, 1982), therefore every core was extracted for a minimum of 25 days.
The soil fauna extracted from the soil cores was identified and counted using a Leica Wild M8 stereomicroscope with 6-50x magnification. Taxa were identified at the most detailed taxonomic level possible, and we selected epigeic taxa (i.e., surface-living species), for further analyses. For example, this included the Collembola and Acari, while excluding Diptera and Coleoptera larvae. The rationale for in- or excluding specific taxa, as well as the full dataset can be found in Supporting Online Information 2.
Abiotic Data
Abiotic data were retrieved from freely available online resources. Air temperature and precipitation data were taken from meteorological station “Lauwersoog” from The Royal Netherlands Meteorological Institute KNMI (2018a,b) which is ~8 km from the saline and ~10 km from the brackish location. Sea level data were taken from the Waterbase website (Waterbase, 2018), for measuring station “Wierumergronden,” which is ~13 km from both sampling locations. Data were used to compile a dataset with daily climatic conditions.
For 7 days preceding each sampling date, we calculated either the absolute maxima achieved (i.e., for the sea water levels and maximum temperature) or the average values (i.e., minimum and maximum temperature, precipitation, and evapotranspiration). In addition, sample year, month, and the day of the year (i.e., Julian day) were recorded. We checked for correlations between all these factors (see Supporting Online Information 3), and selected a subset of representative abiotic factors (i.e., one factor per highly correlated cluster) for further analysis. These were the explanatory continuous environmental variables: absolute maximum temperature, average precipitation and Julian day, and the factors: location, vegetation type, and sampling year. A summary of the sample dates and the corresponding abiotic variables is shown in Table 1. This data is used in the subsequent community analyses.
To determine the occurrence of an extreme event during the study period, we plotted the daily abiotic data for the total sampling period to define which abiotic conditions could be considered extreme. This resulted in the criteria of extreme events defined in Table 2. Per sampling interval, we checked if one or multiple of these criteria were met. If so, this was recorded as an occurrence of the corresponding type of extreme event. The specific sampling intervals during which extreme events took place can be found in Supporting Online Information 4.
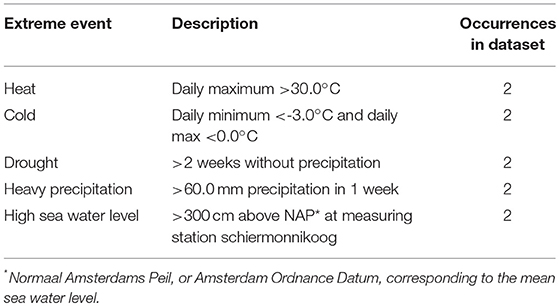
Table 2. Criteria used to identify extreme events of different abiotic variables, and the frequency of these events for the full sampling period.
Statistics
All data analysis was performed using R (R Core Team, 2016) in the RStudio environment (RStudio Team, 2015). The species abundance matrix was Hellinger-transformed to make it suitable for unconstrained ordination of species data (Legendre and Gallagher, 2001). This transformation was chosen to lessen the effect of several very abundant taxa, thereby making the score per sample more representative for the full community (Borcard et al., 2011). To analyse the transformed community data without constraining the data a priori to environmental variables, we first performed a Principal Component Analysis (PCA) on the samples from both locations combined. We then performed PCAs on subsets of the samples for the specific locations and vegetation types. To assess if the environmental variables of the 7 days prior to the sampling dates, and the important factors were correlated with the community samples, we fitted these using the EnvFit function from the vegan package (Oksanen et al., 2016). The environmental variables included were maximum temperature, average precipitation, maximum sea level, and Julian day. The included factors depended on the location-vegetation type subset of the data (e.g., for a PCA on a subset within a location, location itself would not be included as factor), but contained location (saline, brackish), vegetation type (open, grassy, or Carex vegetation) and sampling year (2013, 2014, or 2015).
Furthermore, we wanted to compare the extent of dissimilarity in community composition between communities of subsequent sampling dates with and without the occurrence of extreme events in that time interval. Therefore, we constructed Hellinger distance matrices (Euclidean distance of Hellinger-transformed species data) for the four location-vegetation type combinations. For each of these, the centroid of the replicates per sampling date was calculated. We then calculated the Hellinger distance between these centroids or each set of subsequent sampling dates (sensu Ernest and Brown, 2001; Thibault and Brown, 2008). This resulted in a dataset with one Hellinger distance value for each sampling interval (e.g., distance between T0 to T1, T1 to T2, etc.). These distances were linked to the presence or absence of extreme events in the same time interval. We tested for differences in Hellinger distances between consecutive sampling dates with and without an extreme event with a student t-test. All assumptions of statistical independence and normality of residuals were met.
Results
Species Data
Of the 77,460 individual animals sampled over the course of our monitoring period, 69,460 met the criteria and thresholds for inclusion in the final analyses, which can be found in Supporting Online Information 2. Of these, 32,593 individuals were sampled from the brackish location, and 33,102 from the saline location, which was further subdivided in the vegetation types: open (5,220 ind.), grassy (11,452 ind.), and Carex vegetation (15,921 ind.), see Supporting Online Information 5. In the analysis, 51 taxa were considered, but the dominant taxa with more than 1% abundance were limited per location-vegetation type combination, ranging from 8 to 13 (see Figure S5). The most striking difference between the saline and brackish location is the shift in the dominant Collembola species: Isotoma sp. (>98% Isotoma riparia) in the saline location and Sminthurides malmgreni in the brackish location.
Overall Community Composition
We first assessed if there were clear patterns in the species composition that could be explained by the factors included in the analysis. The Principle Component Analysis (PCA) was carried out on the full species abundance matrix, including both locations. The PCA showed that 20.8% of the observed variation between samples could be explained by the first PC axis, 15.1% by the second PC axis, and 11.8% by the third axis, together explaining 48% of the variance in the species data. Three of the factors were significantly correlated to the patterns in community composition (Figure 1). In order of the highest goodness of fit, these were sampling location (R2 = 0.39, P < 0.001), maximum temperature (R2 = 0.15, P < 0.001), and sample year (R2 = 0.03, P < 0.005). This effect of sample year was driven by samples taken in 2015, which only consisted of two sampling dates at the beginning of the year, as it was the end of our sampling campaign. These two samples therefore correspond to overall lower temperatures, which is illustrated by the negative correlation of year 2015 with maximum temperature. When the samples of 2015 were removed from the analysis, there was only an effect of year in the Carex vegetation type of the saline location, but not in any of the other location-vegetation type combinations.
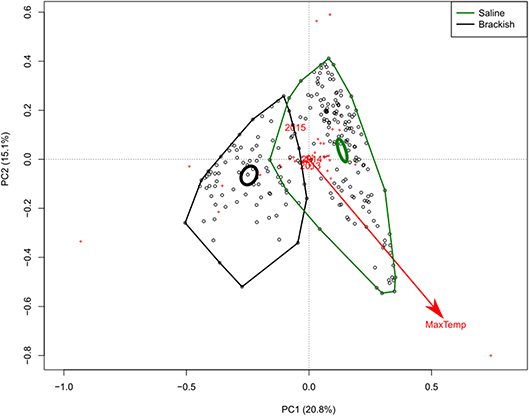
Figure 1. PCA axes 1 and 2 with the samples (black dots) of the full dataset, including both locations. Red plusses indicate the position of the different taxa. A polygon is drawn through all the outer sample points for each location (green for the saline location; black for the brackish location) and the inner thick circle indicates the 95% confidence interval of the centroid for the two locations. The environmental factors that significantly correlate with the samples are indicated by a red arrow for continuous variables or red names for factors with multiple levels.
Community Composition and Factors for Location-Vegetation Type Combinations
To test whether the same environmental factors are important in the different locations and vegetation types, we performed separate PCAs for each location and for each vegetation type within the saline location, and fitted the environmental variables on these subsets (Figure 2). For both locations we observed that the maximum temperature before the sampling date was the most important factor explaining the variance in species composition (brackish: R2 = 0.62, P < 0.001; saline: R2 = 0.21, P < 0.001), even giving a higher fit than vegetation type in the saline location (R2 = 0.14, P < 0.001). When observing the position of Isotoma sp. in the plots, it becomes apparent that they have a strong influence on community composition. This was linked to the maximum temperature before the sampling date for both locations (Figures 2A,B). The other significant factors for the brackish location included Julian day (R2 = 0.19, P < 0.001), precipitation (R2 = 0.10, P = 0.015) and sampling year (R2 = 0.07, P = 0.018). For the saline location, the only other significant factor explaining species data was the sampling year (R2 = 0.04, P = 0.023).
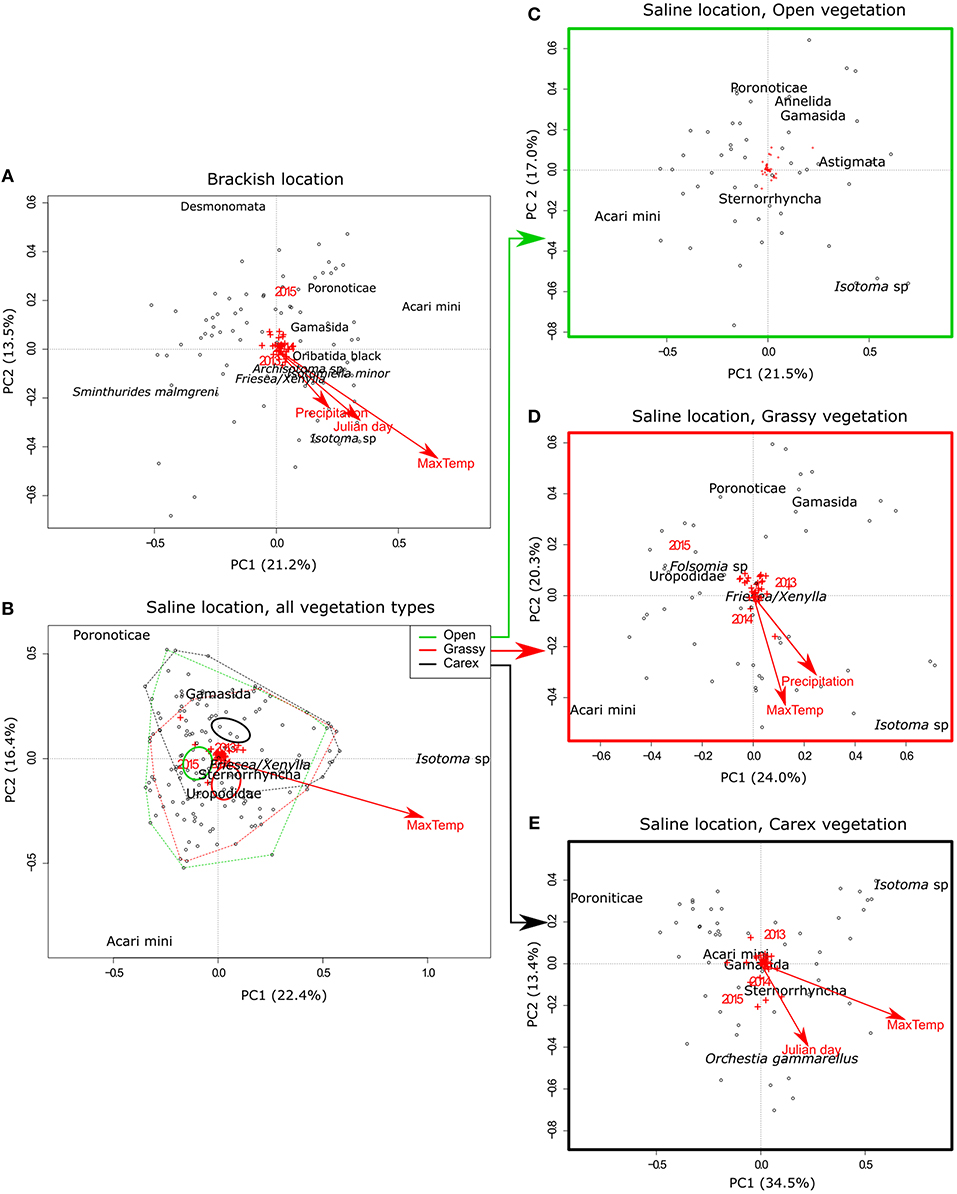
Figure 2. PCA axes 1 and 2 for the (A) brackish location, (B) saline location, with polygons drawn through all the outer sample points of the three vegetation types and the inner thick circle indicating the 95% confidence interval of the centroids for these locations, (C–E) subsets of the saline location, with (C) samples from open vegetation, (D) grassy vegetation, and (E) Carex vegetation. Black dots depict the samples in each plot. Taxa with an abundance of more than 2.5% per location or vegetation type are plotted textually (the exact location is the center of the text), the other taxa are visualized as red plusses. The environmental factors that significantly correlate with the samples are indicated by a red arrow for continuous variables or red names for factors with multiple levels.
The analysis of community composition at the saline location was further subdivided in the three vegetation types. Of these, the open vegetation did not show significant correlations with any of the environmental factors tested. The other two vegetation types showed a similar pattern with both maximum temperature (grassy vegetation: R2 = 0.16, P = 0.016; Carex vegetation: R2 = 0.54, P < 0.001) and sampling year (grassy vegetation: R2 = 0.15, P = 0.003; Carex vegetation: R2 = 0.11, P = 0.018) as significant factors. The grassy vegetation further had a significant correlation with precipitation (R2 = 0.12, P = 0.045) and the Carex vegetation had a significant correlation with Julian day (R2 = 0.20, P < 0.005). Again, of the dominant taxa, Isotoma sp. seemed to be highly related to the maximum temperatures, while Orchestia gammarellus was related to Julian day (and thus seasonality). Also, the Poronoticae mites were negatively correlated to the maximum temperatures, and thus seem to be relatively more abundant in the colder seasons.
Effect of Extreme Events
To test if extreme events, defined for each variable in Table 2, affected community composition in both locations, we tested whether the Hellinger distance (Euclidean distance on Hellinger-transformed species data) between subsequent sampling dates was larger when extreme events took place within the same time interval. We found that this was not the case in three out of the four location-vegetation type combinations (Figure 3). In the fourth comparison (i.e., saline location with open vegetation) the contrary was observed: Hellinger distances were on average smaller when extreme events took place [t(10.1) = 3.32, P = 0.008]. This effect seemed to be caused by all but one type of extreme event, as for heat, drought, heavy precipitation, and high sea water levels, the individual Hellinger's distances were lower than the first quartile of the Hellinger's distances in the time intervals were no extreme events occurred (Figure 3B).
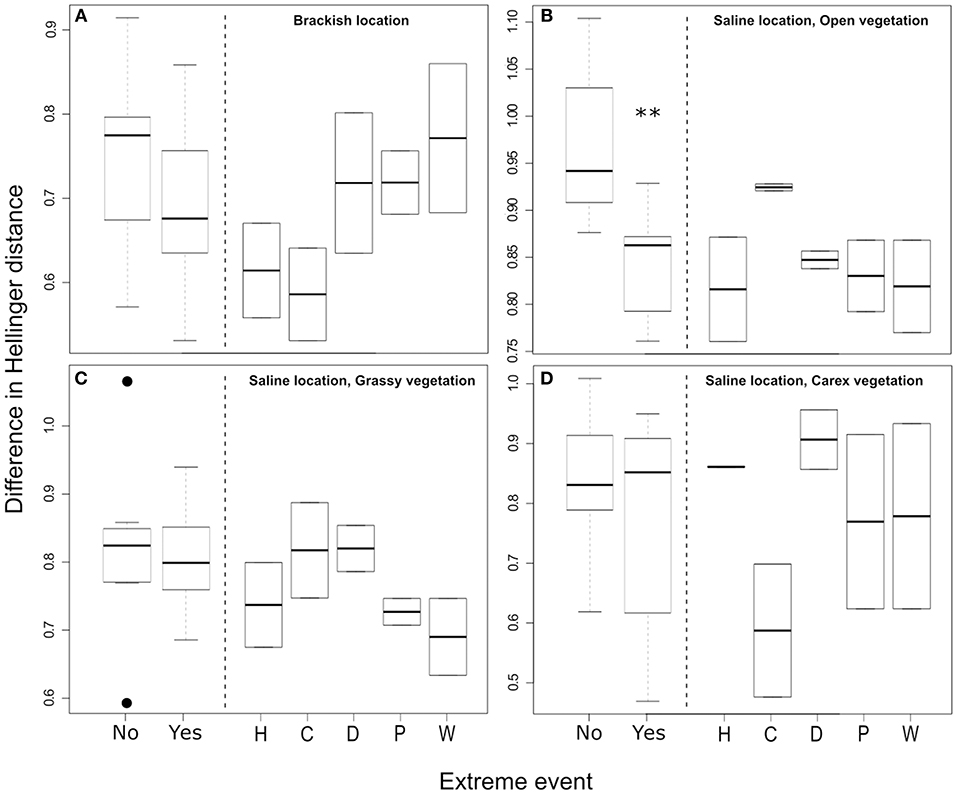
Figure 3. Box plots of the Hellinger distances (i.e., Euclidean distance on Hellinger transformed species data) between the centroids of two subsequent sample dates for the (A) brackish location, (B) saline location with open vegetation, (C) saline location with grassy vegetation, and (D) saline location with Carex vegetation. For each time interval, we defined if an extreme event of any type took place (e.g., No/Yes in the left hand side of the panels) by the criteria summarized in Table 2. No events took place in seven time intervals, and there were nine intervals where events did occur. On the left hand side, boxplots indicate the median with 1st and 3rd quartile ranges, whiskers indicate the minimum and maximum values within 1.5x the interquartile range. Dots indicate values falling outside of this range. On the right hand side, the upper and lower box limits indicate the absolute values, with the central line indicating their mean. **Indicate significance at the P < 0.01 level. The extreme events were further split in the right hand side of the panel, with letters indicating the different types of extreme events. H, Heat; C, Cold; D, Drought; P, Heavy precipitation; and W, High sea water level. The individual extreme events were not statistically tested as each type of event only occurred twice.
Discussion
With extreme climatic events increasing in frequency, there is a need to pinpoint which extremes are most stressful to soil communities, and to facilitate a direct comparison of community responses to different climate extremes. In order to gain insight in the effects of the different types of extreme events, we tested which abiotic factors were most important in explaining the soil arthropod community composition of a vegetated coastal ecosystem. We found that maximum temperature during the week preceding the sampling dates had the strongest correlation with soil arthropod communities in both a saline and a brackish location. We then tested if we could directly compare the effects of different extreme events by looking at the Hellinger distance between the communities of subsequent sampling dates. We found no effect of extreme events on Hellinger distances in three location-vegetation type, and smaller Hellinger distances in saline location with an open vegetation type. This effect is discussed in relation to buffering potential of the different vegetation types.
Location Salinity and Temperature Were the Major Drivers of Community Composition
We observed that the soil arthropod community composition was largely driven by the differences between the two sampling locations, i.e., the brackish and saline sites (Figure 1). Site differences in soil salinity will have strong impacts on species (e.g., Witteveen and Joosse, 1987; Ellers et al., 2010), and thus on community composition of microbes (Dini-Andreote et al., 2014; Rath and Rousk, 2015), plants (van Tooren et al., 1983), and arthropods (Desender and Maelfait, 1999; Irmler et al., 2002; Pétillon et al., 2008). Species differ significantly in salt tolerance and many species are sensitive to salt water inundation. Hence, a shift in community composition from salt tolerant to salt sensitive species from the saline to the brackish site was expected. This was also reflected in the higher species richness in the brackish site. However, we cannot exclude that other factors, such as geomorphological dynamics, and vegetation structure could also have caused a difference in community composition between these sampling locations.
For both the locations, we found that the maximum temperature in the week preceding the sampling date had the highest correlation with community composition. The fact that maximum temperature in the saline site explained variance in species composition better than the difference between the three vegetation types was surprising, given the importance of vegetation structure, especially plant height and soil coverage, for arthropod community composition on salt marshes (Ford et al., 2017). However, the arthropod community composition of the three vegetation types did differ from each other and when analyzed separately, again highlighted the strong effect of the maximum temperature, especially for the grassy vegetation type and the Carex-dominated vegetation type (Figures 2D,E, respectively). Another important factor was Julian day, which was significant at both the brackish location and at the saline location with Carex-dominated vegetation. However, this was likely caused by the cyclic nature of temperature during the year. The effect of sampling year itself was also significant, albeit with low explanatory power, in almost all PCAs. This effect was driven mainly by the two sampling dates in early 2015 in the cold period of the year, and when these two sampling dates of 2015 were removed from the analysis, there was only an effect of year in the Carex vegetation type of the saline location. It is therefore likely that the effect of year is actually indirectly driven by temperature effects.
In both the brackish location and the saline location with grassy vegetation, the amount of precipitation was a significant factor influencing community composition. This is in line with another study that shows that an increase in intensity and frequency of precipitation decreases Collembola abundance and richness (Turnbull and Lindo, 2015), which is attributed to a decrease in the amount of habitable pore space. Although this may be a valid explanation in most terrestrial systems, this mechanism is unlikely to hold in our green beach ecosystem, as the sandy soil is densely packed, and the ground water level is usually very high, leaving the soil often water-locked (personal observations). On the other hand, a meta-analysis by Blankinship et al. (2011) show that precipitation increases soil biota abundances, but this pattern is largely driven by the inclusion of forest ecosystems in the data set. It is therefore likely that the effect precipitation is highly dependent on the type of ecosystem being investigated, as well as the local precipitation patterns.
Direct Comparison of Influence of Extreme Climatic Events on Community Composition
One of our aims in this study was to directly compare the effect of different naturally occurring extreme climatic events. Climatic extreme events can have major impacts on community composition (Bokhorst et al., 2012; Nielsen et al., 2012; Wernberg et al., 2013; Sergio et al., 2018). Therefore, we hypothesized that differences in community composition, as indicated by Hellinger's distance between subsequent sampling dates, would be enlarged if an extreme event had occurred between sampling dates. However, our results did not show the hypothesized increase of dissimilarity in species composition between subsequent sampling dates after an extreme climatic event took place. In three of the four location-vegetation type combinations we found no effects of extreme events on Hellinger distance. In the saline location with an open vegetation type we unexpectedly observed more similar communities, opposite to our expectation.
The absence of the expected pattern in the other location-vegetation type combinations raises two non-exclusive, alternative explanations. The first explanation would be that the communities were not affected by the extreme climatic events, e.g., the extreme climatic events as defined in this study—see Table 2—are not extreme enough for the animals in this highly variable environment to cause a detectable community shift. This raises an ongoing discussion on how to define extreme climatic events, whether this should be a strict climatological threshold, or whether it would be better to use threshold of biological responses (i.e., impact-related threshold) (Bailey and van de Pol, 2016; van de Pol et al., 2017). Here, we chose a static climatological threshold in order to be able to compare between species, as it is known that large interspecific differences in tolerance between species exist (e.g., Ellers et al., 2018; Franken et al., 2018). Using an impact-related threshold is not possible for communities consisting of multiple species with different biological responses, as long as there is no universal way to standardize the effects of extreme events on communities. Potentially the method presented in this paper can pave the way to such a standardization.
The second explanation would be that the Hellinger distance between pairs of subsequent sample dates is not sufficiently responsive to the relative change in community composition. However, the Euclidean distance (here applied on Hellinger-transformed data) is a commonly used method to compare communities, and has been shown to reflect changes in community composition (McCune et al., 2002; Borcard et al., 2011; Shirkhorshidi et al., 2015). Also the use of the distance between subsequent sampling dates has been applied successfully before (Ernest and Brown, 2001; Thibault and Brown, 2008). There are also some other methods available to assess community shifts over time, such as the use of Principle Response Curves (van den Brink et al., 2009; Florentine et al., 2015; Tebby et al., 2017), but this is less suitable to our situation, as we are using natural communities without a clear control community. Hence, we conclude that using Hellinger distances is an appropriate analysis tool to make community responses comparable across contexts.
One important challenge of our methodology remains stress on stress effects by multiple subsequent events, which could have obscured the patterns we wanted to elucidate. In other words, if one severe event imposes a large community shift, the subsequent shift by the next event is by definition smaller if there was no time for the community to fully recover. Apart from increasing sampling frequency, and thereby reducing the chance of sampling subsequent extreme events, a possible solution would be to include the timing of the extreme event within the time interval (van de Pol et al., 2016). Alternative ways of analyzing a long-term community data set such as ours could also provide valuable insight into the drivers of community compositional changes and offer the possibility to test more general ecological theories. For example, by including differences between trophic guilds in island biogeography theory models, Alonso et al. (2015), were able to more accurately predict how reef fish communities would recover after an extreme event. Such analysis, however, falls beyond the scope of the generalizations intended in this study.
We observed smaller Hellinger distances between subsequent sampling dates in the open vegetation type after extreme events. This can potentially be attributed to stress on stress effects, which are more apparent in this open vegetation type than in the more buffered vegetations of the other three location-vegetation type combinations. Vegetation can provide strong buffering effects on abiotic factors, creating micro-habitats that effectively lessen the effects of extreme events (Scheffers et al., 2014; Woods et al., 2015; Keppel et al., 2017; Franken et al., 2018). As such buffering vegetation is absent in the open vegetation type, this is already a more challenging place to inhabit compared to the vegetated sites, considering the more severe environmental conditions in green beach ecosystems (Bakker et al., 2005). This could be the reason that in general, lower numbers of individuals are observed in this open vegetation type (Figure S5), e.g., communities are already challenged under regular conditions.
Conclusion
There is an increasing interest in the effects of different extreme climatic events on communities and ecosystems. However, making a direct comparison of shifts in species compositions of natural communities exposed to multiple natural extreme events is challenging, and has to our knowledge have never been performed. We propose that to compare different extreme events, our interpretation of the distance between subsequent community compositions in multidimensional space method can be a suitable tool. For more complex situations, such as sequential exposures, there are still some caveats in applying this methodology, but we hope this paper stimulates other researchers to think about and discuss the possibilities of using community data for direct comparisons of extreme events. We specifically encourage ecologists with suitable (long-term) community datasets to perform similar analyses to elucidate effects of different extreme events on their model ecosystem or community.
Data Availability Statement
All generated and analyzed datasets for this study are included in the manuscript and the Supplementary Files.
Author Contributions
OF, JE, and MB conceived the ideas and designed the sampling design. OF and SF performed the animal identification and counts. OF analyzed the data with valuable additions by WJ. OF provided a first draft of the manuscript which was improved by all authors. All authors gave final approval for publication.
Conflict of Interest Statement
The authors declare that the research was conducted in the absence of any commercial or financial relationships that could be construed as a potential conflict of interest.
Acknowledgments
The authors would like to thank everyone who participated in the fieldwork over the duration of the monitoring period: Héctor Alonso Fernández, Ben Dijk, Jasper Molleman, Kiki Kersten, Milou Huizinga, Katya Dimitrova, Rudo Verweij, Astra Ooms, and Bart Swaalf. This study was financed by NWO grant 821.01.015.
Supplementary Material
The Supplementary Material for this article can be found online at: https://www.frontiersin.org/articles/10.3389/fevo.2018.00195/full#supplementary-material
References
Alonso, D., Pinyol-Gallemí, A., Alcoverro, T., and Arthur, R. (2015). Fish community reassembly after a coral mass mortality: higher trophic groups are subject to increased rates of extinction. Ecol. Lett. 18, 451–461. doi: 10.1111/ele.12426
Araújo, M. B., Ferri-Yáñez, F., Bozinovic, F., Marquet, P. A., Valladares, F., and Chown, S. L. (2013). Heat freezes niche evolution. Ecol. Lett. 16, 1206–1219. doi: 10.1111/ele.12155
Bailey, L. D., and van de Pol, M. (2016). Tackling extremes: challenges for ecological and evolutionary research on extreme climatic events. J. Anim. Ecol. 85, 85–96. doi: 10.1111/1365-2656.12451
Bakker, J. P., Veeneklaas, R. M., Jansen, A., and Samwel, A. (2005). Een nieuw Groen Strand op Schiermonnikoog. Levende Nat. 106, 151–155.
Berg, M. P., Kiers, E. T., Driessen, G., van der Heijden, M., Kooi, B. W., Kuenen, F., et al. (2010). Adapt or disperse: understanding species persistence in a changing world. Glob. Change Biol. 16, 587–598. doi: 10.1111/j.1365-2486.2009.02014.x
Blankinship, J. C., Niklaus, P. A., and Hungate, B. A. (2011). A meta-analysis of responses of soil biota to global change. Oecologia 165, 553–565. doi: 10.1007/s00442-011-1909-0
Bokhorst, S., Phoenix, G. K., Bjerke, J. W., Callaghan, T. V., Huyer-Brugman, F., and Berg, M. P. (2012). Extreme winter warming events more negatively impact small rather than large soil fauna: shift in community composition explained by traits not taxa. Glob. Change Biol. 18, 1152–1162. doi: 10.1111/j.1365-2486.2011.02565.x
Bolhuis, H., Fillinger, L., and Stal, L. J. (2013). Coastal microbial mat diversity along a natural salinity gradient. PLoS ONE 8:e63166. doi: 10.1371/journal.pone.0063166
Borcard, D., Gillet, F., and Legendre, Legendre, P. (2011). Numerical Ecology with R. New York, NY: Springer. doi: 10.1007/978-1-4419-7976-6
Cheng, B. S., and Grosholz, E. D. (2016). Environmental stress mediates trophic cascade strength and resistance to invasion. Ecosphere 7:1247. doi: 10.1002/ecs2.1247
Chown, S. L., and Terblanche, J. S. (2006). Physiological diversity in insects: ecological and evolutionary contexts. Adv. Insect Phys. 33, 50–152. doi: 10.1016/S0065-2806(06)33002-0
Desender, K., and Maelfait, J. P. (1999). Diversity and conservation of terrestrial arthropods in tidal marshes along the River Schelde: a gradient analysis. Biol. Conserv. 87, 221–229. doi: 10.1016/S0006-3207(98)00058-5
Dini-Andreote, F., De Cássia Pereira E Silva, M., Triadó-Margarit, X., Casamayor, E. O., Van Elsas, J. D., and Salles, J. F. (2014). Dynamics of bacterial community succession in a salt marsh chronosequence: evidences for temporal niche partitioning. ISME J. 8, 1989–2001. doi: 10.1038/ismej.2014.54
Dreesen, F. E., De Boeck, H. J., Janssens, I. A., and Nijs, I. (2012). Summer heat and drought extremes trigger unexpected changes in productivity of a temperate annual/biannual plant community. Environ. Exp. Bot. 79, 21–30. doi: 10.1016/j.envexpbot.2012.01.005
Dreesen, F. E., De Boeck, H. J., Janssens, I. A., and Nijs, I. (2014). Do successive climate extremes weaken the resistance of plant communities? an experimental study using plant assemblages. Biogeosciences 11, 109–121. doi: 10.5194/bg-11-109-2014
Easterling, D. R. (2000). Climate extremes: observations, modeling, and impacts. Science 289, 2068–2074. doi: 10.1126/science.289.5487.2068
Ellers, J., Berg, M. P., Dias, A. T. C., Fontana, S., Ooms, A., and Moretti, M. (2018). Diversity in form and function: vertical distribution of soil fauna mediates multidimensional trait variation. J. Anim. Ecol. 87, 933–944. doi: 10.1111/1365-2656.12838
Ellers, J., Dias, A. T. C., and Berg, M. P. (2010). Interaction milieu explains performance of species in simple food webs along an environmental gradient. Open Ecol. J. 3, 12–21. doi: 10.2174/1874213001003040012
Ernest, S. K. M., and Brown, J. H. (2001). Homeostasis and compensation: the role of species and resources in ecosystem stability. Ecology 82, 2118–2132. doi: 10.2307/2680220
Florentine, S., Milberg, P., Di Stefano, J., Westbrooke, M., and Graz, P. (2015). Decade-long response of arid-land mallee vegetation to fire, flooding and grazing in south-eastern Australia. J. Arid Environ. 121, 7–14. doi: 10.1016/j.jaridenv.2015.05.006
Ford, H., Evans, B., Van Klink, R., Skov, M. W., and Garbutt, A. (2017). The importance of canopy complexity in shaping seasonal spider and beetle assemblages in saltmarsh habitats. Ecol. Entomol. 42, 145–155. doi: 10.1111/een.12373
Franken, O., Huizinga, M., Ellers, J., and Berg, M. P. (2018). Heated communities: large inter- and intraspecific variation in heat tolerance across trophic levels of a soil arthropod community. Oecologia 186, 311–322. doi: 10.1007/s00442-017-4032-z
Grant, P. R., Grant, B. R., Huey, R. B., Johnson, M. T. J., Knoll, A. H., and Schmitt, J. (2017). Evolution caused by extreme events. Philos. Trans. R. Soc. B Biol. Sci. 372:20160146. doi: 10.1098/rstb.2016.0146
Hansen, J., Sato, M., and Ruedy, R. (2012). Perception of climate change. Proc. Natl. Acad. Sci. U.S.A. 109, E2415–E2423. doi: 10.1073/pnas.1205276109
Irmler, U., Heller, K., Meyer, H., and Reinke, H.-D. (2002). Zonation of ground beetles (Coleoptera: Carabidae) and spiders (Araneida) in salt marshes at the North and the Baltic Sea and the impact of the predicted sea level increase. Biodivers. Conserv. 11, 1129–1147. doi: 10.1023/A:1016018021533
Jentsch, A., and Beierkuhnlein, C. (2008). Research frontiers in climate change: effects of extreme meteorological events on ecosystems. Comptes Rendus Geosci. 340, 621–628. doi: 10.1016/j.crte.2008.07.002
Jiguet, F., Brotons, L., and Devictor, V. (2011). Community responses to extreme climatic conditions. Curr. Zool. 57, 406–413. doi: 10.1093/czoolo/57.3.406
Keppel, G., Anderson, S., Williams, C., Kleindorfer, S., and O'Connell, C. (2017). Microhabitats and canopy cover moderate high summer temperatures in a fragmented Mediterranean landscape. PLoS ONE 12:0183106. doi: 10.1371/journal.pone.0183106
KNMI (2018a). Temperature Data. Available online at: https://www.knmi.nl/nederland-nu/klimatologie/daggegevens
KNMI (2018b). Precipitation Data. Available online at: https://www.knmi.nl/nederland-nu/klimatologie/monv/reeksen
Legendre, P., and Gallagher, E. D. (2001). Ecologically meaningful transformations for ordination of species data. Oecologia 129, 271–280. doi: 10.1007/s004420100716
Marrot, P., Garant, D., and Charmantier, A. (2017). Multiple extreme climatic events strengthen selection for earlier breeding in a wild passerine. Philos. Trans. R. Soc. B Biol. Sci. 372:20160372. doi: 10.1098/rstb.2016.0372
McCune, B., Grace, J. B., and Urban, D. L. (2002). “Chapter 6: distance measures,” in Analysis of Ecological Communities, eds B. McCune, J. B. Grace and D. L. Urban (Gleneden Beach, OR: MjM Software Design), 45–57.
Nielsen, U. N., Wall, D. H., Adams, B. J., Virginia, R. A., Ball, B. A., Gooseff, M. N., et al. (2012). The ecology of pulse events: insights from an extreme climatic event in a polar desert ecosystem. Ecosphere 3, 1–15. doi: 10.1890/ES11-00325.1
Oksanen, J., Blanchet, F. G., Kindt, R., Legendre, P., Minchin, P. R., O'hara, R. B., et al. (2016). vegan: Community Ecology Package. Available online at: https://github.com/vegandevs/vegan/issues
Paine, R. T., Tegner, M. J., and Johnson, E. A. (1998). Compounded perturbations yield ecological surprises. Ecosystems 1, 535–545.
Pétillon, J., Georges, A., Canard, A., Lefeuvre, J. C., Bakker, J. P., and Ysnel, F. (2008). Influence of abiotic factors on spider and ground beetle communities in different salt-marsh systems. Basic Appl. Ecol. 9, 743–751. doi: 10.1016/j.baae,0.2007.08.007
R Core Team (2016). R: A Language and Environment for Statistical Computing. R Foundation for Statistical Computing, Vienna. Available online at: https://www.r-project.org/. Available at: https://www.r-project.org/
Rahmstorf, S., and Coumou, D. (2011). Increase of extreme events in a warming world. Proc. Natl. Acad. Sci. U.S.A. 108, 17905–17909. doi: 10.1073/pnas.1101766108
Rath, K. M., and Rousk, J. (2015). Salt effects on the soil microbial decomposer community and their role in organic carbon cycling: a review. Soil Biol. Biochem. 81, 108–123. doi: 10.1016/j.soilbio.2014.11.001
RStudio Team (2015). RStudio: Integrated Development for R. RStudio, Inc. Boston, MA. Available online at: http://www.rstudio.com/. Available at: http://www.rstudio.com/
Scheffers, B. R., Edwards, D. P., Diesmos, A., Williams, S. E., and Evans, T. A. (2014). Microhabitats reduce animal's exposure to climate extremes. Glob. Change Biol. 20, 495–503. doi: 10.1111/gcb.12439
Schlacher, T. A., Schoeman, D. S., Dugan, J., Lastra, M., Jones, A., Scapini, F., et al. (2008). Sandy beach ecosystems: key features, sampling issues, management challenges and climate change impacts. Mar. Ecol. 29, 70–90. doi: 10.1111/j.1439-0485.2007.00204.x
Schoeman, D. S., Schlacher, T. A., and Defeo, O. (2014). Climate-change impacts on sandy-beach biota: crossing a line in the sand. Glob. Change Biol. 20, 2383–2392. doi: 10.1111/gcb.12505
Sentis, A., Hemptinne, J. L., and Brodeur, J. (2013). Effects of simulated heat waves on an experimental plant-herbivore-predator food chain. Glob. Change Biol. 19, 833–842. doi: 10.1111/gcb.12094
Sergio, F., Blas, J., and Hiraldo, F. (2018). Animal responses to natural disturbance and climate extremes: a review. Glob. Planet. Change 161, 28–40. doi: 10.1016/j.gloplacha.2017.10.009
Sheik, C. S., Beasley, W. H., Elshahed, M. S., Zhou, X., Luo, Y., and Krumholz, L. R. (2011). Effect of warming and drought on grassland microbial communities. ISME J. 5, 1692–1700. doi: 10.1038/ismej.2011.32
Shirkhorshidi, A. S., Aghabozorgi, S., and Ying Wah, T. (2015). A comparison study on similarity and dissimilarity measures in clustering continuous data. PLoS ONE 10:e0144059. doi: 10.1371/journal.pone.0144059
Tebby, C., Joachim, S., Van den Brink, P. J., Porcher, J. M., and Beaudouin, R. (2017). Analysis of community-level mesocosm data based on ecologically meaningful dissimilarity measures and data transformation. Environ. Toxicol. Chem. 36, 1667–1679. doi: 10.1002/etc.3701
Thibault, K. M., and Brown, J. H. (2008). Impact of an extreme climatic event on community assembly. Proc. Natl. Acad. Sci. U.S.A. 105, 3410–3415. doi: 10.1073/pnas.0712282105
Traill, L. W., Lim, M. L. M., Sodhi, N. S., and Bradshaw, C. J. (2010). Mechanisms driving change: altered species interactions and ecosystem function through global warming. J. Anim. Ecol. 79, 937–947. doi: 10.1111/j.1365-2656.2010.01695.x
Turnbull, M. S., and Lindo, Z. (2015). Combined effects of abiotic factors on Collembola communities reveal precipitation may act as a disturbance. Soil Biol. Biochem. 82, 36–43. doi: 10.1016/j.soilbio.2014.12.007
Ummenhofer, C. C., and Meehl, G. A. (2017). Extreme weather and climate events with ecological relevance: a review. Philos. Trans. R. Soc. B Biol. Sci. 372:20160135. doi: 10.1098/rstb.2016.0135
van de Pol, M., Bailey, L. D., McLean, N., Rijsdijk, L., Lawson, C. R., and Brouwer, L. (2016). Identifying the best climatic predictors in ecology and evolution. Methods Ecol. Evol. 7, 1246–1257. doi: 10.1111/2041-210X.12590
van de Pol, M., Jenouvrier, S., Cornelissen, J. H. C., and Visser, M. E. (2017). Behavioural, ecological and evolutionary responses to extreme climatic events: challenges and directions. Philos. Trans. R. Soc. B Biol. Sci. 372:20160134. doi: 10.1098/rstb.2016.0134
van den Brink, P. J., den Besten, P. J., bij de Vaate, A., and ter Braak, C. J. (2009). Principal response curves technique for the analysis of multivariate biomonitoring time series. Environ. Monit. Assess. 152, 271–281. doi: 10.1007/s10661-008-0314-6
van der Putten, W. H., Macel, M., and Visser, M. E. (2010). Predicting species distribution and abundance responses to climate change: why it is essential to include biotic interactions across trophic levels. Philos. Trans. R. Soc. B Biol. Sci. 365, 2025–2034. doi: 10.1098/rstb.2010.0037
van Straalen, N. M., and Rijninks, P. C. (1982). The efficiency of Tullgren apparatus with respect to interpreting seasonal changes in age structure of soil arthropod populations. Pedobiologia 24, 197–209.
van Tooren, B. F., Schat, H., and ter Borg, S. J. (1983). Succession and fluctuation in the vegetation of a Dutch beach plain. Vegetatio 53, 139–151. doi: 10.1007/BF00044897
Waterbase (2018). Sea Water Levels. Available online at: http://live.waterbase.nl/waterbase_wns.cfm?taal=en
Wernberg, T., Smale, D. A., Tuya, F., Thomsen, M. S., Langlois, T. J., De Bettignies, T., et al. (2013). An extreme climatic event alters marine ecosystem structure in a global biodiversity hotspot. Nat. Clim. Change 3, 78–82. doi: 10.1038/nclimate1627
Witteveen, J. (1988). The impact of the salinity of soil-water and food on the physiology, behaviour and ecology of salt-marsh Collembola. Funct. Ecol. 2, 49–55.
Witteveen, J., and Joosse, E. N. G. (1987). Growth, reproduction and mortality in marine littoral Collembola at different salinities. Ecol. Entomol. 12, 459–469.
Keywords: Collembola (arthropoda: hexapoda), insects and arachnids, heat, drought, Hellinger distance, spiders (Araneae), community composition, extreme climatic events
Citation: Franken O, Ferreira SSD, Jesse WAM, Berg MP and Ellers J (2018) A Common Yardstick to Measure the Effects of Different Extreme Climatic Events on Soil Arthropod Community Composition Using Time-Series Data. Front. Ecol. Evol. 6:195. doi: 10.3389/fevo.2018.00195
Received: 16 July 2018; Accepted: 06 November 2018;
Published: 23 November 2018.
Edited by:
Mirco Bundschuh, Swedish University of Agricultural Sciences, SwedenReviewed by:
David Alonso, Consejo Superior de Investigaciones Científicas (CSIC), SpainPeter Convey, British Antarctic Survey (BAS), United Kingdom
Copyright © 2018 Franken, Ferreira, Jesse, Berg and Ellers. This is an open-access article distributed under the terms of the Creative Commons Attribution License (CC BY). The use, distribution or reproduction in other forums is permitted, provided the original author(s) and the copyright owner(s) are credited and that the original publication in this journal is cited, in accordance with accepted academic practice. No use, distribution or reproduction is permitted which does not comply with these terms.
*Correspondence: Oscar Franken, b3NjYXJmcmFua2VuQGdtYWlsLmNvbQ==;by5mcmFua2VuQHZ1Lm5s
†These authors share senior authorship