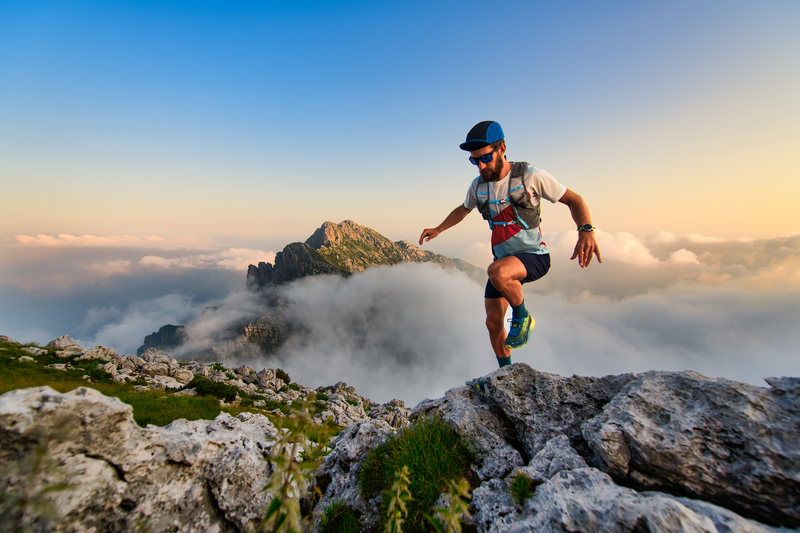
94% of researchers rate our articles as excellent or good
Learn more about the work of our research integrity team to safeguard the quality of each article we publish.
Find out more
ORIGINAL RESEARCH article
Front. Ecol. Evol. , 24 July 2018
Sec. Evolutionary and Population Genetics
Volume 6 - 2018 | https://doi.org/10.3389/fevo.2018.00107
This article is part of the Research Topic Polyploid Population Genetics and Evolution - From Theory to Practice View all 13 articles
Polyploidization is an ancient and recurrent process in plant evolution, impacting the diversification of natural populations and plant breeding strategies. Polyploidization occurs in many important crops; however, its effects on inheritance of many agronomic traits are still poorly understood compared with diploid species. Higher levels of allelic dosage or more complex interactions between alleles could affect the phenotype expression. Hence, the present study aimed to dissect the genetic basis of fruit-related traits in autotetraploid blueberries and identify candidate genes affecting phenotypic variation. We performed a genome-wide association study (GWAS) assuming diploid and tetraploid inheritance, encompassing distinct models of gene action (additive, general, different orders of allelic interaction, and the corresponding diploidized models). A total of 1,575 southern highbush blueberry individuals from a breeding population of 117 full-sib families were genotyped using sequence capture and next-generation sequencing, and evaluated for eight fruit-related traits. For the diploid allele calling, 77,496 SNPs were detected; while 80,591 SNPs were obtained in tetraploid, with a high degree of overlap (95%) between them. A linear mixed model that accounted for population and family structure was used for the GWAS analyses. By modeling tetraploid genotypes, we detected 15 SNPs significantly associated with five fruit-related traits. Alternatively, seven significant SNPs were detected for only two traits using diploid genotypes, with two SNPs overlapping with the tetraploid scenario. Our results showed that the importance of tetraploid models varied by trait and that the use of diploid models has hindered the detection of SNP-trait associations and, consequently, the genetic architecture of some commercially important traits in autotetraploid species. Furthermore, 14 SNPs co-localized with candidate genes, five of which lead to non-synonymous amino acid changes. The potential functional significance of these SNPs is discussed.
Polyploidy is a widespread phenomenon among the flowering plants. Rounds of ancient and recent polyploidization events have been shaping the genomes and the evolutionary trajectories of plant lineages, driving phenotypic diversification (Adams and Wendel, 2005; Paterson, 2005; Jiao et al., 2011; Blischak et al., 2016). Expansion of phenotypic range and novel phenotypes often arise with polyploidization (Spoelhof et al., 2017). The genomic redundancy created by polyploidy allows relaxed selective constraints and functional divergence of gene copies, which can generate new phenotypes in the long-term evolutionary process (Adams and Wendel, 2005; Comai, 2005). Immediate phenotypic effects of polyploidy are also observed compared to their diploid progenitors, such as increased cell and organ size, changes in flowering time, and greater vigor and biomass (Osborn et al., 2003; Tamayo-Ordóñez et al., 2016). The molecular mechanisms contributing to phenotypic variation shortly after polyploidization are not well-understood, but probably involve more complex genetic and epigenetic effects of higher allelic dosage and heterosis (Osborn et al., 2003; Jackson and Chen, 2010; Renny-Byfield and Wendel, 2014; Fort et al., 2016). For example, genome-wide gene expression studies in resynthesized polyploid plants and yeasts have shown ploidy-dependent gene expression alterations, which likely affect the phenotype (Guo et al., 1996; Galitski et al., 1999; Osborn et al., 2003; Pumphrey et al., 2009; Jackson and Chen, 2010).
Polyploids exhibiting new phenotypic traits can outperform their diploid counterparts, occupy new niches, and become ecologically and agriculturally important (Tamayo-Ordóñez et al., 2016; Spoelhof et al., 2017). Many important crops are polyploids with varied ploidy levels and mode of origin (i.e., auto- or allopolyploids). However, despite the economic importance of polyploids and the impact that ploidy can have in the phenotypic expression, the effects of allelic dosage on quantitative traits remain largely unexplored. Most genetic studies in polyploids have so far relied on diploid models to simplify the polyploid data. The complex nature of polyploid genetic data (e.g., multiple alleles and mixed inheritance patterns) has hindered the understanding of genetic architecture of important traits (Dufresne et al., 2014). Moreover, molecular techniques and statistical methodologies were also constraints for polyploids, such as the challenge to define the allelic dosage (Garcia et al., 2013; Lu et al., 2013; Dufresne et al., 2014; Li et al., 2014a; Annicchiarico et al., 2015; Uitdewilligen et al., 2015; Schulz et al., 2016).
Due to the advances in new genotyping technologies, it is now possible to generate high-density single nucleotide polymorphism (SNP) data and evaluate the relative abundance of each allele based on read sequencing depth to infer the allelic dosage. Genome-wide association studies (GWAS) that consider allelic dosage can help uncover the genetic basis of complex traits by considering more realistic genetic models, and hence reducing the signal-to-noise ratio (Garcia et al., 2013; Grandke et al., 2016). Moreover, the effect of the genotype classes on the phenotypic variation can be tested under different gene action models to gain additional insights into additive and non-additive effects (Rosyara et al., 2016). The present study aimed to understand how modeling the allelic dosage influences the identification of SNPs significantly associated with blueberry fruit-related traits through GWAS analyses.
Blueberry has been recognized worldwide for its health benefits, becoming one of the crops with the highest consumer demand and productive trends (USDA, 2016). During blueberry improvement in the United States, interspecific hybridizations have been used for the development of “southern” highbush cultivars adapted to warmer climates. Crosses primarily involved the autotetraploid “northern” highbush blueberry (Vaccinium corymbosum L.) and the diploid evergreen blueberry (V. darrowii Camp) (Sharpe and Darrow, 1959). Tetraploid hybrids were achieved by the occurrence of unreduced gametes during pollen formation in the diploid species (Ortiz et al., 1992). Despite interspecific hybridizations, blueberry cultivars are considered autotetraploids with non-preferential bivalent chromosome pairing during meiosis and the absence of chromosome structural differentiation (Qu and Hancock, 1995; Qu et al., 1998; Lyrene et al., 2003). The conventional breeding program employs phenotypic recurrent selection, and the release of a new cultivar can take up to 15 years (Hancock et al., 2008). In a perennial polyploid species, such as blueberry, marker-assisted selection has the potential for accelerate the cultivar development process. In this sense, the GWAS analyses can also assist in the identification of causal polymorphisms or molecular markers associated with fruit-related traits relevant for blueberry breeding. The objective of this study is two-fold: (i) to compare the effects of diploid and tetraploid marker calling in population genetics and GWAS analysis; and (ii) to perform the first GWAS analysis for fruit-related traits in southern highbush blueberry.
The southern highbush blueberry population used in this study was generated as part of the breeding program at the University of Florida. For this study, 124 controlled crosses were made among 148 selected parents in February 2011. Seeds from each cross were cold-stratified for 5 months and planted in a greenhouse as a family in 2 L pots in November 2011. One hundred seedlings from each family were later transplanted to a high-density nursery (~20,000 plants per 0.2 ha) in a row-column design at the University of Florida Plant Science Research and Education Unit in Citra, Florida. In May 2013, a first round of selection was performed. Unselected plants were removed from the field and the remaining individuals constituted the 1,575 plants from 117 crosses used in this study.
The phenotypic evaluations were conducted during fruit ripening (6 weeks from the beginning of April to mid May 2014) and flowering (January 2015) periods when the plants were in their third growing season. Eight fruit-related traits were measured: weight, size, firmness, stem scar diameter, pH, soluble solids content, flower bud density, and yield. Yield was evaluated using a 1-to-5 rating scale, where 1 indicates none or very few berries on the plant and 5 is a yield comparable to standard commercial cultivars. The flower bud density refers to the number of flower buds on the top 20 cm of one representative upright shoot from the main stem, and was reported as number of buds per 20 cm of shoot. For the fruit traits, the average of five berries randomly selected from each genotype was calculated. Weight (g) was measured using an analytical scale (CP2202S, Sartorius Corp., Bohemia, NY). The same five berries were equatorially oriented to measure fruit size diameter (mm) and firmness (g*mm−1 compression force), with a minimum and maximum force threshold of 50 and 350 g, respectively, using the Firm-Tech II (BioWorks Inc., Wamego, KS). The picking stem scar was positioned upward on a tray in a light box with a digital SLR camera (Pentax K-x, Ricoh Imaging, Denver, CO) placed 50 cm above the berry. A ruler was also placed in each image as a size reference. The images were uploaded into FIJI (Schindelin et al., 2012), the scale was set using the ruler, and the scar diameter (mm) was measured for each berry. The blueberry juice was used to measure traits related to sensory quality. The soluble solids content (°Brix), an approximate surrogate measure of sugar content, was assessed using a digital pocket refractometer (Atago U.S.A, Inc., Bellevue, WA). The juice pH was measured using a glass pH electrode (Mettler-Toldeo, Inc., Schwerzenbach, Switzerland).
Total genomic DNA was extracted from leaf tissue of each plant using the E-Z 96 PlantDNAKit (Omega Bio-Tek, Norcross, GA). Genotyping was performed by RAPiD Genomics (Gainesville, FL, USA) using sequence capture. Briefly, 31,063 custom-designed biotinylated probes of 120-mer were developed based on the scaffolds of the blueberry draft genome sequence (2013 version) (Bian et al., 2014; Gupta et al., 2015). Sequencing was carried out in the Illumina HiSeq2000 platform using 100 cycle paired-end runs. Raw reads were first trimmed for minimum base quality of 20, demultiplexed, and barcodes were removed. Subsequently, reads were aligned to the blueberry genome (2013 version) using BWA v.0.7.12 (Li and Durbin, 2009).
Polymorphisms and genotypes were called using FreeBayes v.1.0.1, selecting the diploid (-p 2) and the tetraploid (-p 4) options (Garrison and Marth, 2012). Genotypes were represented by the count of alternative alleles. Therefore, for the diploid calling, genotypes were coded as 0 (AA), 1 (AB), or 2 (BB), where “A” and “B” refers to the reference and alternative alleles, respectively. The genotypes for the tetraploid calling were coded as 0 for nulliplex (AAAA), 1 for simplex (AAAB), 2 for duplex (AABB), 3 for triplex (ABBB), and 4 for quadruplex (BBBB). We performed a sample filtering by excluding individuals with more than 90% of missing data across SNPs (sample call rate = 0.9). SNPs were further filtered by: (i) minimum depth of coverage of 40; (ii) minimum genotype quality score of 10; (iii) only biallelic locus; (iv) maximum missing data of 0.7; (v) minor allele frequency of 0.05. The remaining missing genotypes were imputed with the mode of each locus as suggested by Rosyara et al. (2016).
Population genetics parameters were computed considering the polyploid and diploid scenarios. We estimated: (i) allele frequency; (ii) heterozygosity; (iii) linkage disequilibrium (LD) decay; and (iv) population structure. The allele frequency for each locus was obtained by counting the number of alternative alleles, divided by sample size, and ploidy level. The observed heterozygosity was calculated as a fraction of the number of heterozygote classes by the total number of loci. Pearson correlation tests (r2) were performed for pairwise LD estimation within scaffolds. All scaffolds were pooled to plot a genome-wide LD decay and boxplots of r2 values for categories of marker distances. The decay of LD over genetic distance was determined as the mean distance associated with an empirical LD threshold of r2 = 0.2. To assess the genetic structure of blueberry population, the Principal Components Analysis (PCA) was performed using the marker-based relationship matrix as input. Diploid and tetraploid genomic relationship matrices were computed with the AGHmatrix R-package (Amadeu et al., 2016). The Discriminant Analysis of Principal Components (DAPC) was conducted to cluster genetically similar individuals using the Bayesian Information Criterion (BIC) to select the best supported model, as implemented in the R package adegenet v. 1.3-1 (Jombart and Ahmed, 2011).
The SNP-trait association analyses were based on a linear mixed model, accounting for population structure (Q) and relative kinship (K) matrices as implemented in the GWASpoly R-package (Rosyara et al., 2016). The Q+K linear mixed model was:
where y is a vector of observed phenotypes; ε is a vector of random residual effects, with a multivariate normal distribution with a zero mean vector and an identity variance-covariance (VCOV) matrix; v is a vector of sub-populations effects, with incidence matrix Q; and u is a random polygenic effect, with a multivariate normal distribution with a zero mean vector and VCOV matrix proportional to a kinship matrix (K-matrix). The Z incidence matrix maps genotypes to observations, and the SNP effects are represented by the τ fixed vector. As pointed out by Rosyara et al. (2016), the matrix S depends on the genetic model assumed. In order to compare diploid and tetraploid pipelines, the Q+K model was implemented in both scenarios. For tetraploid, the K-matrix was constructed assuming tetrasomic inheritance (Slater et al., 2013), while for the diploid model it was built considering the algorithm proposed by VanRaden (2008). Both matrices were computed using the AGHmatrix R-package (Amadeu et al., 2016). To correct for population structure, PCA analysis was computed internally using the GWASpoly package and the four principal components were further used in GWAS analyses.
Eight gene action models were tested for the tetraploid genotype calling: general, additive, simplex dominant alternative (simplex-dom-alt), simplex dominant reference (simplex-dom-ref), duplex dominant alternative (duplex-dom-alt), duplex dominant reference (duplex-dom-ref), diplo-additive, and diplo-general. According to Rosyara et al. (2016), the general type of genetic model allows the SNP effect for each genotypic class to be arbitrary and statistically equivalent. In the additive model the SNP effect is proportional to the dosage of the minor allele. In the simplex dominant models, all the heterozygotes (AAAB, AABB, ABBB) are equivalent to one of the homozygotes (AAAA or BBBB). In the duplex dominant models, the duplex state (AABB) has the same effect as either the simplex (AAAB) and nulliplex (AAAA) or the triplex (ABBB) and quadriplex (BBBB) states. In the diploidized models (diplo), all heterozygous classes have the same effect, resembling a traditional diploid dosage model (AA, AB, BB), and have gene action models encompassing the general and additive effects. The diploid genotype calling was also used for GWAS analyses, using the following gene actions: diplo-general, diplo-additive, simplex-dom-alt, and simplex-dom-ref.
Correction for multiple testing using a q-value threshold of 0.05 was applied to determine significant associations using the q-value R-package (Storey and Tibshirani, 2003). We also explored more and less conservative thresholds for declaring significance by using Bonferroni correction of 0.05 and q-value of 0.1, respectively. QQ-plots were used to evaluate the presence of confounding factors leading to an excess of significant associations.
The proportion of phenotypic variation explained by significant SNPs was approximated by the coefficient of determination (R2). The R2 was estimated considering a linear regression model that included the first four principal components from PCA analyses, the SNP marker parameterized in accordance with the gene action and a vector of random residual effects.
SNPs were characterized in silico for their genomic position and functional effect. SNPs were annotated using snpEff v.4.3 (Cingolani et al., 2012), using the blueberry draft genome (2013 version) and gene predictions. Predicted gene models were retrieved from the bitbucket repository https://bitbucket.org/lorainelab/blueberrygenome (Gupta et al., 2015). Candidate genes surrounding significantly associated SNPs were annotated using the Blast2GO tool with BLASTp search against the non-redundant protein database (Götz et al., 2008). We also searched for Arabidopsis thaliana v. TAIR10 orthologs using Phytozome v.12.1 BLASTp tool (https://phytozome.jgi.doe.gov).
A total of 1,575 blueberry plants from 117 crosses were phenotyped for eight fruit-related traits (yield, flower bud density, fruit weight, firmness, size, soluble solids content, pH, and scar diameter). Most traits followed a normal distribution, except yield which was evaluated on a 1-to-5 rating scale, and flower bud density which followed a Poisson distribution (Figure 1). High phenotypic correlation was only found between berry size and weight (r = 0.94) (Figure 1).
Figure 1. Phenotypic distribution and correlation of eight blueberry fruit-related traits for 1,575 individuals. Plots in the diagonal show the frequency distribution of each trait. Pearson's correlation coefficient between traits are indicated above the diagonal. Scatter plots below the diagonal illustrate the underlying relationship between traits.
After filtering the genotypic data, a total of 1,557 individuals and 77,496 SNPs were maintained for the diploid analyses; while 1,559 individuals and 80,591 SNPs were considered for tetraploid analyses. SNPs were sampled throughout the genome, although not evenly distributed, which was expected due to the target design strategy used in this study (Figure 2).
Figure 2. Distribution of filtered SNPs from the tetraploid pipeline in 100 kb windows across the 20 largest blueberry scaffolds (gray). The x-axis represents the distance in base pairs.
Tetraploid and diploid pipelines identified 74,941 common SNPs (around 95% of overlap). We assumed that the differences between pipelines were due to the algorithms implemented in the Freebayes software, which considers different criteria to define a SNP in each parameterization. As a consequence of the high degree of overlap, few differences were observed regarding the position and functional characterization of the SNPs in the blueberry genome (Figures 3A,B). Most SNPs were detected in non-coding regions; around 7% targeted exonic regions, mostly causing missense mutations. The distribution of the alternative allele frequency across loci was also similar for both approaches (Figure 3C), with the tetraploid model showing the mean allele frequency slightly lower (0.25 vs. 0.27). The main difference between the tetraploid and diploid scenarios was on the genotype calling (Figure 3D). For biallelic SNPs in autotetraploids, there are five possible genotypes, with three possible heterozygous states. For diploids, there are only three possible genotypes, with one heterozygous class. The probabilistic assignment of genotypes based on sequence read depth led to a higher heterozygosity for tetraploid compared to diploid genotype calling (0.42 vs. 0.34).
Figure 3. Characterization of SNPs identified in diploid and tetraploid pipelines. (A) Percentage of SNPs located in distinct genomic regions. Upstream and downstream regions refer to distances less than 5 kb from surrounding genes. (B) Functional effects of SNPs located in exonic regions. (C) Distribution of alternative (“B”) allele frequency. (D) Distribution of genotypic frequencies across loci.
The LD and population structure were consistent between the ploidy models. The trend of LD decay in the blueberry breeding population can be observed by the r2 distribution across categories of base pair distances between SNPs in Figure 4. At the significance threshold (r2 = 0.2), the LD decay presented significant correlation between markers 73 Kb apart for the diploid model and 80 Kb apart for the tetraploid model (Supplementary Figure 1). In order to verify the possible influence of the population structure in the GWAS analysis, we performed PCA and DAPC cluster analyses. The results for both standardizations were very similar, with the tetraploid matrix explaining slightly more of the population genetic variation (28.19 vs. 24.78%) (Figure 5A). The comparison of the BIC values for the DAPC analysis suggested the presence of 50 groups in the population (Figure 5B), which showed similarities with the pedigree recorded in the population. Hence, in the GWAS analyses, we used the PCA scores to control for population stratification and the genomic relationship matrix to control for cryptic relatedness.
Figure 4. Boxplots showing the trend of LD decay as a relationship between r2 measures at different intervals of marker distances (Kb) for diploid and tetraploid standardizations.
Figure 5. Population structure of a blueberry breeding population of 117 full-sib families performed using diploid and tetraploid pipelines. (A) 2D-PCA plots performed using the diploid and tetraploid marker-based relationship matrix. Each individual is represented by a point in the hyperspace defined by the eigenvectors of the first and second principal components. (B) Bayesian Information Criterion (BIC) for number of clusters ranging from 0 to 156.
We performed GWAS analyses for eight fruit-related traits using the Q+K linear mixed model. A total of 77,496 and 80,591 SNPs were regressed individually in the diploid and tetraploid GWAS models, respectively. Manhattan plots displaying the significance threshold for each locus in their genomic location are shown in Supplementary Figures 2, 3. The inspection of QQ-plots did not show evidences of systematic bias in any trait or model evaluated (Supplementary Figures 4, 5).
Association analyses using the tetraploid genotypes and a q-value threshold of 0.05 allowed the identification of 23 significant SNPs associated with five traits and 11 were also significant after Bonferroni correction (Table 1). Six SNPs were identified by more than one gene action model. A total of 15 distinct SNPs were identified: seven for fruit size, two for scar diameter, three for soluble solids content, one for pH, and two for flower bud density (Figure 6A, Table 1). For fruit size, soluble solids content, and pH traits, dominance models were effective for detecting at least one association. However, the general model was the most effective at detecting associations. This class of model assumes that each genotype has its own effect and hence encompasses different gene actions. The inspection of the phenotypic variation across genotypes for significant SNPs identified by the general model suggested degrees of overdominance for some traits (e.g., see SNPs scaffold13749-868 and scaffold00818-130228 for flower bud density trait) (Supplementary Figure 6). Under a less conservative threshold, the number of distinct associations increased from 15 (q-value < 0.05) to 37 (q-value < 0.1) and new associations were detected for fruit weight and firmness traits (Figure 6A, Supplementary Table 1). It is also noteworthy that the same SNP located at scaffold00697, position 151000, was detected as significantly associated with fruit size and fruit weight, the two highly correlated traits (Figure 1).
Table 1. List of SNP-trait associations detected by diploid and tetraploid models under q-value of 0.05 and the candidate genes flaking significant SNPs.
Figure 6. SNP-trait associations detected by modeling tetraploid and diploid genotype callings. (A) Venn-diagrams comparing the number of distinct SNPs associated with fruit-related traits in the diploid and tetraploid scenarios under q-value thresholds of 0.05 (continuous lines) and 0.1 (dashed lines). (B) Circular Manhattan plot for fruit size. Outer and inner layers represent the diplo-general and general models fitted using diploid (2x) and tetraploid (4x) pipelines, respectively. (C) Circular Manhattan plot for scar diameter. Outer and inner layers represent the diplo-general and general models fitted using the diploid (2x) and tetraploid (4x) pipelines, respectively. SNPs were concatenated by their position in the genomic scaffolds and are displayed along the circular Manhattan plots according to their adjusted p-value. The significance threshold (q-value = 0.05) is represented by the gray circle in each layer. Vertical dashed gray lines highlight the significant SNPs. The names of significant SNPs are listed outside of the plot. SNPs identified for diploid and tetraploid pipelines are in orange and blue, respectively; while the common SNP identified in both pipelines is in black.
Considering the diploid genotype calling and a q-value threshold of 0.05, we detected seven significant SNPs associated with two fruit-related traits (Table 1). Out of these, one association was significant after Bonferroni correction. We found three distinct SNPs associated with scar diameter and four with flower bud density (Figure 6A, Table 1). The general model was the most effective for all traits. Under a less conservative threshold, the number of distinct associations increased from 7 (q-value < 0.05) to 14 (q-value < 0.1) and new associations were detected for berry size, firmness, pH, soluble solids content traits (Figure 6A, Supplementary Table 1).
Overall, more SNP-trait associations were identified by modeling the genotypes as tetraploid than as diploid (Figures 6A,B). Associations for fruit size, soluble solids content, and pH were only detected using tetraploid models, considering a q-value threshold of 0.05. However, there were four SNPs for flower bud density and one for scar diameter that were only detected by modeling diploid genotypes. Moreover, both models were able to detect the same two SNPs for scar diameter (Figure 6C, Table 1). No significant association was found for firmness, fruit weight, and yield traits with any ploidy and model tested under this moderate threshold.
We identified candidate genes flanking SNPs significantly associated with traits based on the annotation of the blueberry genome (see Table 1 for q-value < 0.05 and Supplementary Table 1 for q-value < 0.1). Among the protein-coding genes surrounding the seven distinct SNPs associated with fruit size trait, we found a putative lipase (CUFF.5533.1), a RING-type E3 ubiquitin ligase (CUFF.6059.2), a xyloglucan endotransglucosylase (CUFF.38641.1), a hypersensitive-induced response protein 1 (gene.g14573.t1), and a chloroplast rhomboid-like protease (CUFF.39364.1). Two SNPs in high LD and few base pairs apart were located at the gene encoding the chloroplast RHOMBOID-like protease, one of them leading to a missense mutation (Figure 7).
Figure 7. SNP effect on fruit size. (A) Candidate gene encoding a chloroplast rhomboid-like protease (CUFF.39364.1) where a missense variant was detected in the second exon. (B) Significance of SNPs detected by additive (gray dots) and general (blue dots) gene action models along the scaffold01404. The green dashed line indicates the genic region affected by the two significant SNPs. Double bars indicate out of scale. (C) Scaffold positions of the SNPs, highlighting the SNPs associated with the trait in green. (D) Pairwise linkage disequilibrium (correlation coefficient r2) between markers along the scaffold.
For scar diameter, three distinct SNP-trait associations were detected. Annotation was found only for one of the surrounding genes, which encoded a pentatricopeptide repeat-containing protein (CUFF.20851.1).
Three significant SNPs were found for solid soluble content. Two SNPs occurred at genes potentially encoding proteins with a role in the ubiquitin-mediated protein degradation pathway: a ubiquitin-activating enzyme E1 (CUFF.53548.1) and an E3 ubiquitin ligase (CUFF.16799.1).
For the flower bud density trait, six significant SNPs were found, with four potentially causing missense mutations. Out of those, two SNPs in high LD were located at a gene encoding a zf-RVT domain-containing protein (CUFF.60704.1), one at a gene encoding heat shock protein hsp83-90 (CUFF.13871.1), and another at a gene encoding a kinase U-box domain-containing protein (CUFF.57663.1).
For pH trait, no functional annotation was found for the flanking gene.
GWAS analyses in autopolyploids impose additional steps not required in diploids, including the estimation of allele copy number and usage of genetic models that account for dosage effects (Garcia et al., 2013; Dufresne et al., 2014; Rosyara et al., 2016). To circumvent this problem, an alternative has been to use knowledge and methods applied to diploid species in polyploid analyses (Mollinari and Serang, 2015). In this work, we have demonstrated that assuming a diploid parameterization onto a tetraploid species affects the results of a GWAS study. Furthermore, this study is the first to utilize association genetics to understand the genetic architecture and molecular basis of fruit-related traits in blueberry.
Prior to performing a SNP-trait association analysis, a detailed understanding of population structure and linkage disequilibrium is essential (Flint-Garcia et al., 2003). Therefore, we compared diploid and polyploid pipelines in terms of marker characterization and estimation of population genetic parameters.
The high degree of overlap between SNP loci identified by both pipelines suggested that the SNP calling step is not drastically affected by ploidy level. However, differences were observed in the genotype calling step, which affected the magnitude of the population parameters. The lower heterozygosity estimated by using diploid (0.34) rather than tetraploid (0.42) genotypes indicates that diploid standardization may cause an underestimation of the heterozygosity rates. Although heterozygosity is a populational parameter and therefore depends on the genetic background under analysis, the heterozygosity estimated in the tetraploid standardization is more in accordance with previous results reported for blueberry (Debnath, 2014; Tailor et al., 2017). Tetraploid highbush blueberry is primarily an outcrossing species with early-acting inbreeding depression (Krebs and Hancock, 1990). Therefore, higher levels of heterozygosity are indeed expected. Moreover, it is reasonable to assume a greater degree of heterozygosity in autopolyploid species in general, since more alleles at one locus are expected when compared to diploids (Gallais, 2003). High levels of heterozygosity have been reported in polyploid species due to its associated benefits, including buffering of deleterious mutations and heterosis (Comai, 2005).
In terms of population-based genomic association studies, it is well-known that population structure is one factor that can result in spurious associations, i.e., associations between a phenotype and markers that are not linked to any causative loci (Pritchard et al., 2000; Sillanpää, 2011). For diploid and tetraploid pipelines, the most likely number of groups in DAPC analyses were in accordance with the pedigree recorded in the population. Based on the QQ-plot results, we inferred that the first four principal components and the genomic relationship matrices in each parameterization were sufficient to account for sample structure confounders. However, it is noteworthy that this conclusion is limited to our breeding population. In more complex pedigrees, for example, the usage of relationship matrix for autotetraploids might impact the final results (Kerr et al., 2012; Amadeu et al., 2016).
LD is another population parameter that significantly affects GWAS results. Assuming that association analyses rely on non-random association between SNPs and causative genes, determining the extent of LD is important to define strategies in GWAS analyses. For both pipelines, we observed a rapid LD decay across the blueberry scaffolds. Accordingly, low LD is reported in other outcrossing species (Gupta et al., 2005). For practical purposes, short LD blocks require a higher number of individuals with records and higher marker density in order to identify causal variants (Goddard et al., 2016). Hence, the usage of a high number of individuals and a high throughput genotyping method was consistent with our research scenario. The LD pattern can also provide information about the genetic diversity in our breeding population. Assuming that the expectation of r2 can be expressed as a function of the effective population size (Ne), faster LD decay is expected as long as Ne increases (Flint-Garcia et al., 2003). Empirically, a short-range LD observed in our population suggests a large Ne value. This is in accordance with the breeding strategy at the University of Florida as parental selection has been performed in order to decrease the inbreeding depression, therefore maintaining genetic diversity (Cellon et al., 2018).
Polyploid studies considering the relative abundance of each allele at a particular locus in the genome allow the testing of more realistic genetic models. For example, the usage of allele dosage has impacted the construction of genetic linkage maps (Mollinari and Serang, 2015), the computation of observed and expected allele frequencies (Dufresne et al., 2014), and the inference of population structure and patterns of historical demography (Blischak et al., 2016). On the bases of genome-wide association studies, our results supported the importance of including allelic dosage to identify significant SNP-trait associations. By modeling tetraploid genotypes under a q-value threshold of 0.05, at least one SNP-trait association was detected for five traits in a blueberry breeding population, and no associations were detected for fruit size, pH, and soluble solids traits when the dosage effect was omitted.
In addition to the allelic dosage, we also tested different gene action models. It is noteworthy that the genotypic value of an individual is estimated differently in polyploid and diploid species. In autotetraploids, the higher number of alleles per locus reflects on different coefficients of dominance, increasing the range of genetic models to describe one-locus genotypic value (Gallais, 2003). In this study, dominance gene actions were addressed on the simplex and duplex dominance models. Simplex dominance represents the first order interaction among alleles and may be modeled regardless of the ploidy. Nevertheless, duplex dominance arises when heterozygotes are affected only if they have two unfavorable alleles; therefore, it is a model that can only be tested in polyploid systems. Duplex dominance interaction models were detected for associations under q-value threshold of 0.1 for flower bud density and firmness traits. Hence, our results reinforce the importance of considering an autotetraploid parameterization in blueberry.
We also tested “diploidized models” or “pseudodiploid models” using the tetraploid genotype calling, as they are widely-used in polyploid analyses due to straightforward implementation in diploid software (Li et al., 2014b; Biazzi et al., 2017). This parameterization disregards the allele dosage and all heterozygotes are grouped into the same genotypic class, which is at the midpoint between the two homozygotes (Rosyara et al., 2016; Slater et al., 2016). In diploid species, this is equivalent to the additive model (parameterized as {0,1,2} and assuming that the SNP effect is proportional to the dosage of the minor allele). In autotetraploids, this parameterization might be interpreted as a partial dominance model suggesting that any order of interaction between alleles reduces the genotypic value (Gallais, 2003; Slater et al., 2016). Our results showed that “diploidized models” were valid for scar diameter, fruit size, and soluble solids traits under a q-value threshold of 0.05. Interestingly, the standard assumption of additivity was not the most appropriate to describe the phenotypic variation observed in blueberry. Divergent results were described in autopolyploid potatoes, for which most of the QTLs were identified considering additive models (Rosyara et al., 2016). Based on our results, we might infer that non-additive effects have a key role in understanding the genetic architecture of blueberry fruit traits.
Although we did not have explicitly approached models addressing partial interactions among alleles, they are potential models to be further implemented in GWAS analyses. Overdominance is particularly more complex, since it can be explored by restricting interactions among alleles to different orders (Gallais, 2003). In this study, these genetic assumptions were implicitly considered in the general model. General model is a generic class that also encompasses other models with no genetic assumptions (Rosyara et al., 2016). Not surprisingly, this model was able to identify the highest number of significant trait-associations, with some overlap with the competing models.
However, considering a q-value threshold of 0.1, significant associations were identified by simplex and duplex models for soluble solids, flower bud density, and pH, but not by the general model. According to Rosyara et al. (2016), there is a trade-off between flexibility and power, because the general model requires a higher number of degrees of freedom, resulting in a lower statistical power.
The heritability estimate provided some insights into the results. Heritability is a population parameter that measures the degree of variation in a phenotypic trait that is due to genetic variation (Falconer and Mackay, 1996). Therefore, it is reasonable to expect a positive relation between heritability and ability to detect associations. In the current population, low to mid narrow-sense heritability was found for the traits, varying from 0.16 for flower bud density to 0.57 for scar diameter (for details, see Cellon et al., 2018). In line with this, individual markers explained a small portion of the phenotypic variation (less than 5%). These results suggest that all fruit-related traits analyzed herein are quantitative, which means that phenotypic variation depends on the cumulative actions of many genes with small effects and their interaction with environment.
Among the significant SNPs associated with blueberry fruit-related traits, some did not lie in protein-coding regions and others caused synonymous changes. In the majority of the GWAS studies in plants, significant associations were also detected for variants in introns, untranslated, or intergenic regions (Ingvarsson and Street, 2011). Many of these variants can be in LD with an untyped causal non-synonymous mutation or might cause changes in gene expression (Gilad et al., 2008). In the case of blueberry, the absence of a high-quality reference genome is an additional challenge for GWAS analysis and biological interpretation. The current available genome is very fragmented and many predicted genes are incomplete (Gupta et al., 2015). Hence, the biological significance of the associations found herein is still limited and speculative, but we point out some insights into the potential molecular mechanisms underlying the variation of each trait.
Larger fruits are a consumer-desired trait in the fresh blueberry market. Among the significant SNPs associated with fruit size, one caused a non-synonymous mutation in the putative gene encoding a chloroplast-located rhomboid-like protease. In A. thaliana, the lack of a rhomboid protease was associated with reduced fertility and aberrations in flower morphology (Knopf et al., 2012; Thompson et al., 2012). Changes in floral morphology and development can affect the fruit size and shape, as reported in tomato (Tanksley, 2004). However, to our knowledge, no study has reported the role of a rhomboid-like protease in fruit size variation. Another SNP associated with berry size occurred at a gene encoding a RING-type E3 ubiquitin ligase. Interestingly, a QTL for rice grain width and weight was also mapped in RING-type protein with E3 ubiquitin ligase activity (Song et al., 2007). Song et al. (2007) suggested that this protein negatively regulates cell division by targeting its substrate(s) to proteasome degradation, since its loss of function resulted in increased cell number and larger (wider) rice spikelet hull. Another interesting SNP was the one located in a gene encoding a xyloglucan endotransglucosylase. This enzyme catalyzes the molecular grafting between xyloglucan molecules in the plant cell-wall matrix, allowing expansive cell growth by restructuring the cell wall (Miedes et al., 2011; Ohba et al., 2011). In transgenic tomatoes with modified expression of a xyloglucan endotransglucosylase gene, fruit size was positively correlated with the expression level of this enzyme (Ohba et al., 2011).
The picking scar size also affects blueberry commercialization, as bigger scars increase perishability and pathogen penetration (Parra et al., 2007). Among the associations detected for scar diameter, the most interesting was the SNP detected under a q-value of 0.1, upstream of an auxin transporter 3, which controls cellular auxin influx. The major form of auxin IAA (Indole-3-acetic acid) is known to delay fruit abscission from the receptacle by reducing the sensitivity of cells in the abscission zone to ethylene (Blanusa et al., 2005; Kühn et al., 2016). The inhibition of polar auxin transport in grapevine fruitlets resulted in fruit drop (Kühn et al., 2016).
Soluble solid content and pH are important sensory quality factors affecting blueberry fruit flavor. Sweetness perception of fruits depends on the balance between sugars and acids (Cirilli et al., 2016; Farneti et al., 2017). For the sugar content, measured as the soluble solids content, two significant SNPs occurred at genes encoding proteins with a role in the ubiquitin-mediated protein degradation pathway. The attachment of ubiquitin molecules to selected proteins can have diverse regulatory functions, influencing the protein activity, abundance, trafficking, or localization (Stone, 2014). The ubiquitin-proteasomal degradation machinery is also involved in the regulation of sugar signaling pathways, which primarily targets the source-to-sink carbon partitioning (Rolland et al., 2006). The role of proteolysis in controlling sugar accumulation was also reported in tomato fruits (Ariizumi et al., 2011). For pH variation, no annotation was found for the predicted gene harboring the significant SNP, hindering biological insights at this point.
Flower bud density can be useful to estimate potential yield in the next harvest (Salvo et al., 2012). Among the significant associations with this trait, we found SNPs leading to missense mutations. One missense mutation occurred at the gene encoding for a heat shock protein (hsp83-90). In Ipomoea nil (formely Pharbitis nil, the Japanese morning glory), hsp83 was upregulated upon exposure to a photoperiod that induces flowering (Felsheim and Das, 1992). The heat shock protein Hsp90 was also reported to act as an environmental signal sensor regulating flowering time (Sangster et al., 2007) and flower development (Margaritopoulou et al., 2016). Another missense variant was found at a gene encoding for a protein kinase U-box domain-containing. The U-box domain has a ubiquitin ligase activity and the kinase motif suggests that this protein participates in signal transduction cascades via phosphorylation. The potential ortholog in Arabidopsis thaliana (At1g16760) is expressed during the pollen stage (Wang et al., 2008).
Fruit firmness is a trait of commercial importance as it directly affects fruit quality, shelf life, and transportability (MacLean and NeSmith, 2011); therefore, it is a key target for blueberry breeding. In this work, we identified associations only when we used a less stringent q-value threshold of 0.1; two missense variants were detected. One of the SNPs causing missense mutations was located at a putative ubiquitin-like-specific cysteine proteinase. Recent studies have shown the role of proteolysis in the regulation of fruit ripening in tomato (Wang et al., 2014, 2017). Particularly, a vacuolar cysteine proteinase (SlVPE3) was shown to affect the accumulation of numerous ripening-related proteins, acting as a post-transcriptional regulator (Wang et al., 2017). Moreover, Salentijn et al. (2003) found cysteine proteinases differentially expressed between firm and soft strawberry cultivars. The other missense variant associated with firmness was located in a SAM-MTase. SAM-MTases are ubiquitous enzymes that catalyze the transfer of methyl groups from S-adenosyl methionine (SAM) to a myriad of compounds (e.g., DNA, RNA, proteins, sterols, pectin, lignin, flavonoids, phenylpropanoids, and alkaloids) and also act in the biosynthesis pathway of ethylene and polyamines. Many of those compounds have an important role in fruit ripening (Moffatt and Weretilnyk, 2001; Roje, 2006; Teyssier et al., 2008; Singh et al., 2010; MacLean and NeSmith, 2011; Paul et al., 2012; Van de Poel et al., 2013; Zhang et al., 2015).
Two of the major challenges faced in this study were the absence of a high-quality genome assembly for blueberry and the allelic dosage calling. We expect that the improvement of genome contiguity might impact the reads alignment quality, providing a more accurate SNP calling and a more precise location of the markers associated with traits. Dosage calling has also been recognized to be a major challenge in genomic studies of polyploid species (Bourke et al., 2018), and it is an area that when fully developed could contribute significantly to association studies in autopolyploids. Population structure is another issue that could be affecting the current results. Controlling for population structure is a standard procedure in GWAS analyses, as we did by using the Q+K model; however, it reduces the statistical power to detect associations when phenotypes strongly correlate with relatedness (Reif et al., 2010; Brachi et al., 2011; Würschum et al., 2012; Ogut et al., 2015; Han et al., 2016; Klasen et al., 2016).
Our results suggested that blueberry fruit quality traits have a complex genetic basis. Therefore, the traditional implementation of marker-assisted selection using our GWAS results seems limited at this point. However, we emphasize that new associations with higher effects could be detected in future GWAS analyses using a complete genome assembly, higher marker density, and more accurate dosage calling method. Alternatively, genomic selection is a promising approach for prediction of complex traits and it is an opportunity for future studies.
Altogether, in this study we demonstrated that simplifying tetraploid data as a diploid can have significant consequences in some population genetic parameters and in the ability to detect marker-trait associations. The absence of associations detected by the conventional additive gene action model suggests that non-additive effects might play a key role in understanding the genetic architecture of blueberry fruit traits. Some of the significant SNPs were detected within and around biologically plausible candidate genes. The encoded proteins may act on pathways that affect the traits as suggested by studies in other plant species. However, better gene prediction and functional validation of these genes will further improve our understanding of the variation of fruit-related traits in blueberry.
Phenotypic and genotypic datasets used for diploid and tetraploid analyses are available from the Dyrad Digital Repository (accession number doi: 10.5061/dryad.kd4jq6h).
PM and JO designed the study. CC and JO conducted the field experiment and collected the phenotypic data. CC performed the DNA extraction. MR performed the SNP calling and filtering. LF, JB, and IdB performed the data analyses and interpretation. JB, LF, IdB, and PM wrote the paper. MR and MK provided analytical expertise and edited the manuscript. PM supervised the whole study. All authors read and approved the final version of the manuscript for publication.
This work was funded by the UF royalty fund generated by the licensing of blueberry cultivars. CC was partially supported, while at the University of Florida, by the National Institute of Food and Agriculture, United States Department of Agriculture, under award number 2014-67013-22418 to PM and JO. IdB was supported by CAPES (Coordenação de Aperfeiçoamento de Pessoal de Nível Superior) [PSDE scholarship: 88881.131685/2016-01].
JO and CC were affiliated to the University of Florida when the study started and by the time the manuscript was submitted they were employed by Driscoll's Inc., and Duda Farm Fresh Foods, respectively.
The remaining authors declare that the research was conducted in the absence of any commercial or financial relationships that could be construed as a potential conflict of interest.
The Supplementary Material for this article can be found online at: https://www.frontiersin.org/articles/10.3389/fevo.2018.00107/full#supplementary-material
Adams, K. L., and Wendel, J. F. (2005). Polyploidy and genome evolution in plants. Curr. Opin. Plant Biol. 8, 135–141. doi: 10.1016/j.pbi.2005.01.001
Amadeu, R. R., Cellon, C., Olmstead, J. W., Garcia, A. A., Resende, M. F., and Muñoz, P. R. (2016). AGHmatrix: R package to construct relationship matrices for autotetraploid and diploid species: a blueberry example. Plant Genome 9. doi: 10.3835/plantgenome2016.01.0009
Annicchiarico, P., Nazzicari, N., Li, X., Wei, Y., Pecetti, L., and Brummer, E. C. (2015). Accuracy of genomic selection for alfalfa biomass yield in different reference populations. BMC Genomics 16:1020. doi: 10.1186/s12864-015-2212-y
Ariizumi, T., Higuchi, K., Arakaki, S., Sano, T., Asamizu, E., and Ezura, H. (2011). Genetic suppression analysis in novel vacuolar processing enzymes reveals their roles in controlling sugar accumulation in tomato fruits. J. Exp. Bot. 62, 2773–2786. doi: 10.1093/jxb/erq451
Bian, Y., Ballington, J., Raja, A., Brouwer, C., Reid, R., Burke, M., et al. (2014). Patterns of simple sequence repeats in cultivated blueberries (Vaccinium section Cyanococcus spp.) and their use in revealing genetic diversity and population structure. Mol. Breed. 34, 675–689. doi: 10.1007/s11032-014-0066-7
Biazzi, E., Nazzicari, N., Pecetti, L., Brummer, E. C., Palmonari, A., Tava, A., et al. (2017). Genome-wide association mapping and genomic selection for alfalfa (Medicago sativa) forage quality traits. PLoS ONE 12:e0169234. doi: 10.1371/journal.pone.0169234
Blanusa, T., Else, M. A., Atkinson, C. J., and Davies, W. J. (2005). The regulation of sweet cherry fruit abscission by polar auxin transport. Plant Growth Regul. 45, 189–198. doi: 10.1007/s10725-005-3568-9
Blischak, P. D., Kubatko, L. S., and Wolfe, A. D. (2016). Accounting for genotype uncertainty in the estimation of allele frequencies in autopolyploids. Mol. Ecol. Resour. 16, 742–754. doi: 10.1111/1755-0998.12493
Bourke, P. M., Voorrips, R. E., Visser, R. G. F., and Maliepaard, C. (2018). Tools for genetic studies in experimental populations of polyploids. Front. Plant Sci. 9:513. doi: 10.3389/fpls.2018.00513
Brachi, B., Morris, G. P., and Borevitz, J. O. (2011). Genome-wide association studies in plants: the missing heritability is in the field. Genome Biol. 12:232. doi: 10.1186/gb-2011-12-10-232
Cellon, C., Amadeu, R. R., Olmstead, J. W., Mattia, M. R., Ferrão, L. F. V., and Munoz, P. R. (2018). Estimation of genetic parameters and prediction of breeding values in an autotetraploid blueberry breeding population with extensive pedigree data. Euphytica 214:87. doi: 10.1007/s10681-018-2165-8
Cingolani, P., Platts, A., Wang le, L., Coon, M., Nguyen, T., Wang, L., et al. (2012). A program for annotating and predicting the effects of single nucleotide polymorphisms, SnpEff: SNPs in the genome of Drosophila melanogaster strain w1118; iso-2; iso-3. Fly 6, 80–92. doi: 10.4161/fly.19695
Cirilli, M., Bassi, D., and Ciacciulli, A. (2016). Sugars in peach fruit: a breeding perspective. Hortic. Res. 3:15067. doi: 10.1038/hortres.2015.67
Comai, L. (2005). The advantages and disadvantages of being polyploid. Nat. Rev. Genet. 6, 836–846. doi: 10.1038/nrg1711
Debnath, S. C. (2014). Structured diversity using EST-PCR and EST-SSR markers in a set of wild blueberry clones and cultivars. Biochem. Syst. Ecol. 54, 337–347. doi: 10.1016/j.bse.2014.03.018
Dufresne, F., Stift, M., Vergilino, R., and Mable, B. K. (2014). Recent progress and challenges in population genetics of polyploid organisms: an overview of current state-of-the-art molecular and statistical tools. Mol. Ecol. 23, 40–69. doi: 10.1111/mec.12581
Falconer, D. S., and Mackay, T. F. C. (1996). Quantitative Genetics. New York, NY: Longman Scientific and Technical.
Farneti, B., Khomenko, I., Grisenti, M., Ajelli, M., Betta, E., Algarra, A. A., et al. (2017). Exploring blueberry aroma complexity by chromatographic and direct-injection spectrometric techniques. Front. Plant Sci. 8:617. doi: 10.3389/fpls.2017.00617
Felsheim, R. F., and Das, A. (1992). Structure and expression of a heat-shock protein 83 gene of Pharbitis nil. Plant Physiol. 100, 1764–1771. doi: 10.1104/pp.100.4.1764
Flint-Garcia, S. A., Thornsberry, J. M., and Buckler, E. S. (2003). Structure of linkage disequilibrium in plants. Annu. Rev. Plant Biol. 54, 357–374. doi: 10.1146/annurev.arplant.54.031902.134907
Fort, A., Ryder, P., McKeown, P. C., Wijnen, C., Aarts, M. G., Sulpice, R., et al. (2016). Disaggregating polyploidy, parental genome dosage and hybridity contributions to heterosis in Arabidopsis thaliana. New Phytol. 209, 590–599. doi: 10.1111/nph.13650
Galitski, T., Saldanha, A. J., Styles, C. A., Lander, E. S., and Fink, G. R. (1999). Ploidy regulation of gene expression. Science 285, 251–254. doi: 10.1126/science.285.5425.251
Gallais, A. (2003). Quantitative Genetics and Breeding Methods in Autopolyploid Plants. Paris: INRA Editions.
Garcia, A. A., Mollinari, M., Marconi, T. G., Serang, O. R., Silva, R. R., Vieira, M. L., et al. (2013). SNP genotyping allows an in-depth characterisation of the genome of sugarcane and other complex autopolyploids. Sci. Rep. 3:3399. doi: 10.1038/srep03399
Garrison, E., and Marth, G. (2012). Haplotype-based variant detection from short-read sequencing. arXiv [Preprint]. arXiv1207.3907.
Gilad, Y., Rifkin, S. A., and Pritchard, J. K. (2008). Revealing the architecture of gene regulation: the promise of eQTL studies. Trends Genet. 24, 408–415. doi: 10.1016/j.tig.2008.06.001
Goddard, M. E., Kemper, K. E., MacLeod, I. M., Chamberlain, A. J., and Hayes, B. J. (2016). Genetics of complex traits: prediction of phenotype, identification of causal polymorphisms and genetic architecture. Proc. R. Soc. B. 283:20160569. doi: 10.1098/rspb.2016.0569
Götz, S., García-Gómez, J. M., Terol, J., Williams, T. D., Nagaraj, S. H., Nueda, M. J., et al. (2008). High-throughput functional annotation and data mining with the Blast2GO suite. Nucleic Acids Res. 36, 3420–3435. doi: 10.1093/nar/gkn176
Grandke, F., Singh, P., Heuven, H. C., De Haan, J. R., and Metzler, D. (2016). Advantages of continuous genotype values over genotype classes for GWAS in higher polyploids: a comparative study in hexaploid chrysanthemum. BMC Genomics 17:672. doi: 10.1186/s12864-016-2926-5
Guo, M., Davis, D., and Birchler, J. A. (1996). Dosage effects on gene expression in a maize ploidy series. Genetics 142, 1349–1355.
Gupta, P. K., Rustgi, S., and Kulwal, P. L. (2005). Linkage disequilibrium and association studies in higher plants: present status and future prospects. Plant Mol. Biol. 57, 461–485. doi: 10.1007/s11103-005-0257-z
Gupta, V., Estrada, A. D., Blakley, I., Reid, R., Patel, K., Meyer, M. D., et al. (2015). RNA-Seq analysis and annotation of a draft blueberry genome assembly identifies candidate genes involved in fruit ripening, biosynthesis of bioactive compounds, and stage-specific alternative splicing. Gigascience 4, 1–22. doi: 10.1186/s13742-015-0046-9
Han, S., Utz, H. F., Liu, W., Schrag, T. A., Stange, M., Würschum, T., et al. (2016). Choice of models for QTL mapping with multiple families and design of the training set for prediction of Fusarium resistance traits in maize. Theor. Appl. Genet. 129, 431–444. doi: 10.1007/s00122-015-2637-3
Hancock, J. F., Lyrene, P., Finn, C. E., Vorsa, N., and Lobos, G. A. (2008). “Blueberries and cranberries,” in Temperate Fruit Crop Breeding, ed J. F. Hancock (Dordrecht: Springer), 115–150.
Ingvarsson, P. K., and Street, N. R. (2011). Association genetics of complex traits in plants. New Phytol. 189, 909–922. doi: 10.1111/j.1469-8137.2010.03593.x
Jackson, S., and Chen, Z. J. (2010). Genomic and expression plasticity of polyploidy. Curr. Opin. Plant Biol. 13, 153–159. doi: 10.1016/j.pbi.2009.11.004
Jiao, Y., Wickett, N. J., Ayyampalayam, S., Chanderbali, A. S., Landherr, L., Ralph, P. E., et al. (2011). Ancestral polyploidy in seed plants and angiosperms. Nature 473, 97–100. doi: 10.1038/nature09916
Jombart, T., and Ahmed, I. (2011). adegenet 1.3-1: new tools for the analysis of genome-wide SNP data. Bioinformatics 27, 3070–3071. doi: 10.1093/bioinformatics/btr521
Kerr, R. J., Li, L., Tier, B., Dutkowski, G. W., and McRae, T. A. (2012). Use of the numerator relationship matrix in genetic analysis of autopolyploid species. Theor. Appl. Genet. 124, 1271–1282. doi: 10.1007/s00122-012-1785-y
Klasen, J. R., Barbez, E., Meier, L., Meinshausen, N., Bühlmann, P., Koornneef, M., et al. (2016). A multi-marker association method for genome-wide association studies without the need for population structure correction. Nat. Commun. 7:13299. doi: 10.1038/ncomms13299
Knopf, R. R., Feder, A., Mayer, K., Lin, A., Rozenberg, M., Schaller, A., et al. (2012). Rhomboid proteins in the chloroplast envelope affect the level of allene oxide synthase in Arabidopsis thaliana. Plant J. 72, 559–571. doi: 10.1111/j.1365-313X.2012.05090.x
Krebs, S. L., and Hancock, J. F. (1990). Early-acting inbreeding depression and reproductive success in the highbush blueberry, Vaccinium corymbosum L. Theor. Appl. Genet. 79, 825–832. doi: 10.1007/BF00224252
Kühn, N., Serrano, A., Abello, C., Arce, A., Espinoza, C., Gouthu, S., et al. (2016). Regulation of polar auxin transport in grapevine fruitlets (Vitis vinifera L.) and the proposed role of auxin homeostasis during fruit abscission. BMC Plant Biol. 16:234. doi: 10.1186/s12870-016-0914-1
Li, H., and Durbin, R. (2009). Fast and accurate short read alignment with Burrows–Wheeler transform. Bioinformatics 25, 1754–1760. doi: 10.1093/bioinformatics/btp324
Li, X., Han, Y., Wei, Y., Acharya, A., Farmer, A. D., Ho, J., et al. (2014a). Development of an alfalfa SNP array and its use to evaluate patterns of population structure and linkage disequilibrium. PLoS ONE 9:e84329. doi: 10.1371/journal.pone.0084329
Li, X., Wei, Y., Acharya, A., Jiang, Q., Kang, J., and Brummer, E. C. (2014b). A saturated genetic linkage map of autotetraploid alfalfa (Medicago sativa L.) developed using genotyping-by-sequencing is highly syntenous with the Medicago truncatula genome. G3 4, 1971–1979. doi: 10.1534/g3.114.012245
Lu, F., Lipka, A. E., Glaubitz, J., Elshire, R., Cherney, J. H., Casler, M. D., et al. (2013). Switchgrass genomic diversity, ploidy, and evolution: novel insights from a network-based SNP discovery protocol. PLoS Genet. 9:e1003215. doi: 10.1371/journal.pgen.1003215
Lyrene, P. M., Vorsa, N., and Ballington, J. R. (2003). Polyploidy and sexual polyploidization in the genus Vaccinium. Euphytica 133, 27–36. doi: 10.1023/A:1025608408727
MacLean, D. D., and NeSmith, D. S. (2011). Rabbiteye blueberry postharvest fruit quality and stimulation of ethylene production by 1-methylcyclopropene. Hortscience 46, 1278–1281.
Margaritopoulou, T., Kryovrysanaki, N., Megkoula, P., Prassinos, C., Samakovli, D., Milioni, D., et al. (2016). HSP90 canonical content organizes a molecular scaffold mechanism to progress flowering. Plant J. 87, 174–187. doi: 10.1111/tpj.13191
Miedes, E., Zarra, I., Hoson, T., Herbers, K., Sonnewald, U., and Lorences, E. P. (2011). Xyloglucan endotransglucosylase and cell wall extensibility. J. Plant Physiol. 168, 196–203. doi: 10.1016/j.jplph.2010.06.029
Moffatt, B. A., and Weretilnyk, E. A. (2001). Sustaining S-adenosyl-l-methionine-dependent methyltransferase activity in plant cells. Physiol. Plant 113, 435–442. doi: 10.1034/j.1399-3054.2001.1130401.x
Mollinari, M., and Serang, O. (2015). “Quantitative SNP genotyping of polyploids with MassARRAY and other platforms,” in Plant Genotyping Methods in Molecular Biology (Methods and Protocols), ed J. Batley (New York, NY: Humana Press), 215–241.
Ogut, F., Bian, Y., Bradbury, P. J., and Holland, J. B. (2015). Joint-multiple family linkage analysis predicts within-family variation better than single-family analysis of the maize nested association mapping population. Heredity 114, 552–563. doi: 10.1038/hdy.2014.123
Ohba, T., Takahashi, S., and Asada, K. (2011). Alteration of fruit characteristics in transgenic tomatoes with modified expression of a xyloglucan endotransglucosylase/hydrolase gene. Plant Biotechnol. J. 28, 25–32. doi: 10.5511/plantbiotechnology.10.0922a
Ortiz, R., Vorsa, N., Bruederle, L. P., and Laverty, T. (1992). Occurrence of unreduced pollen in diploid blueberry species, Vaccinium sect. Cyanococcus. Theor. Appl. Genet. 85, 55–60. doi: 10.1007/BF00223844
Osborn, T. C., Pires, J. C., Birchler, J. A., Auger, D. L., Chen, Z. J., Lee, H. S., et al. (2003). Understanding mechanisms of novel gene expression in polyploids. Trends Genet. 19, 141–147. doi: 10.1016/S0168-9525(03)00015-5
Parra, R., Lifante, Z. D., and Valdés, B. (2007). Fruit size and picking scar size in some blueberry commercial cultivars and hybrid plants grown in SW Spain. Int. J. Food Sci. Technol. 42, 880–886. doi: 10.1111/j.1365-2621.2006.01299.x
Paterson, A. H. (2005). Polyploidy, evolutionary opportunity, and crop adaptation. Genetica 123, 191–196. doi: 10.1007/s10709-003-2742-0
Paul, V., Pandey, R., and Srivastava, G. C. (2012). The fading distinctions between classical patterns of ripening in climacteric and non-climacteric fruit and the ubiquity of ethylene—an overview. J. Food Sci. Technol. 49, 1–21. doi: 10.1007/s13197-011-0293-4
Pritchard, J. K., Stephens, M., Rosenberg, N. A., and Donnelly, P. (2000). Association mapping in structured populations. Am. J. Hum. Genet. 67, 170–181. doi: 10.1086/302959
Pumphrey, M., Bai, J., Laudencia-Chingcuanco, D., Anderson, O., and Gill, B. S. (2009). Nonadditive expression of homoeologous genes is established upon polyploidization in hexaploid wheat. Genetics 181, 1147–1157. doi: 10.1534/genetics.108.096941
Qu, L., and Hancock, J. F. (1995). Nature of 2n gamete formation and mode of inheritance in interspecific hybrids of diploid Vaccinium darrowi and tetraploid V. corymbosum. Theor. Appl. Genet. 91, 1309–1315. doi: 10.1007/BF00220946
Qu, L., Hancock, J., and Whallon, J. (1998). Evolution in an autopolyploid group displaying predominantly bivalent pairing at meiosis: genomic similarity of diploid Vaccinium darrowi and autotetraploid V. corymbosum (Ericaceae). Am. J. Bot. 85, 698–703. doi: 10.2307/2446540
Reif, J. C., Liu, W., Gowda, M., Maurer, H. P., Möhring, J., Fischer, S., et al. (2010). Genetic basis of agronomically important traits in sugar beet (Beta vulgaris L.) investigated with joint linkage association mapping. Theor. Appl. Genet. 12, 1489–1499. doi: 10.1007/s00122-010-1405-7
Renny-Byfield, S., and Wendel, J. F. (2014). Doubling down on genomes: polyploidy and crop plants. Am. J. Bot. 101, 1711–1725. doi: 10.3732/ajb.1400119
Roje, S. (2006). S-Adenosyl-L-methionine: beyond the universal methyl group donor. Phytochemistry 67, 1686–1698. doi: 10.1016/j.phytochem.2006.04.019
Rolland, F., Baena-Gonzalez, E., and Sheen, J. (2006). Sugar sensing and signaling in plants: conserved and novel mechanisms. Annu. Rev. Plant Biol. 57, 675–709. doi: 10.1146/annurev.arplant.57.032905.105441
Rosyara, U. R., De Jong, W. S., Douches, D. S., and Endelman, J. B. (2016). Software for genome-wide association studies in autopolyploids and its application to potato. Plant Genome 9, 1–10. doi: 10.3835/plantgenome2015.08.0073
Salentijn, E. M. J., Aharoni, A., Schaart, J. G., Boone, M. J., and Krens, F. A. (2003). Differential gene expression analysis of strawberry cultivars that differ in fruit-firmness. Physiol. Plant. 118, 571–578. doi: 10.1034/j.1399-3054.2003.00138.x
Salvo, S., Muñoz, C., Ávila, J., Bustos, J., Ramirez-Valdivia, M., Silva, C., et al. (2012). An estimate of potential blueberry yield using regression models that relate the number of fruits to the number of flower buds and to climatic variables. Sci. Hortic. 133, 56–63. doi: 10.1016/j.scienta.2011.10.020
Sangster, T. A., Bahrami, A., Wilczek, A., Watanabe, E., Schellenberg, K., McLellan, C., et al. (2007). Phenotypic diversity and altered environmental plasticity in Arabidopsis thaliana with reduced Hsp90 levels. PLoS ONE 2:e648.doi: 10.1371/journal.pone.0000648
Schindelin, J., Arganda-Carreras, I., Frise, E., Kaynig, V., Longair, M., Pietzsch, T., et al. (2012). Fiji: an open-source platform for biological-image analysis. Nat. Methods 9, 676–682. doi: 10.1038/nmeth.2019
Schulz, D. F., Schott, R. T., Voorrips, R. E., Smulders, M. J., Linde, M., and Debener, T. (2016). Genome-wide association analysis of the anthocyanin and carotenoid contents of rose petals. Front. Plant Sci. 7:1798. doi: 10.3389/fpls.2016.01798
Sharpe, R. H., and Darrow, G. M. (1959). Breeding blueberries for the Florida climate. Proc. Fla. State Hort. Soc. 72, 308–311.
Sillanpää, M. J. (2011). Overview of techniques to account for confounding due to population stratification and cryptic relatedness in genomic data association analyses. Heredity 106, 511–519. doi: 10.1038/hdy.2010.91
Singh, R., Rastogi, S., and Dwivedi, U. N. (2010). Phenylpropanoid metabolism in ripening fruits. Compr. Rev. Food Sci. Food Saf. 9, 398–416. doi: 10.1111/j.1541-4337.2010.00116.x
Slater, A. T., Cogan, N. O., Forster, J. W., Hayes, B. J., and Daetwyler, H. D. (2016). Improving genetic gain with genomic selection in autotetraploid potato. Plant Genome 9, 1–15. doi: 10.3835/plantgenome2016.02.0021
Slater, A. T., Wilson, G. M., Cogan, N. O., Forster, J. W., and Hayes, B. J. (2013). Improving the analysis of low heritability complex traits for enhanced genetic gain in potato. Theor. Appl. Genet. 127, 809–820. doi: 10.1007/s00122-013-2258-7
Song, X. J., Huang, W., Shi, M., Zhu, M. Z., and Lin, H. X. (2007). A QTL for rice grain width and weight encodes a previously unknown RING-type E3 ubiquitin ligase. Nat. Genet. 39, 623–630. doi: 10.1038/ng2014
Spoelhof, J. P., Soltis, P. S., and Soltis, D. E. (2017). Pure polyploidy: closing the gaps in autopolyploid research. J. Syst. Evol. 55, 340–352. doi: 10.1111/jse.12253
Stone, S. L. (2014). The role of ubiquitin and the 26S proteasome in plant abiotic stress signaling. Front. Plant Sci. 5:135. doi: 10.3389/fpls.2014.00135
Storey, J. D., and Tibshirani, R. (2003). Statistical significance for genome-wide experiments. Proc. Natl. Acad. Sci. U.S.A. 100, 9440–9445. doi: 10.1073/pnas.1530509100
Tailor, S., Bykova, N. V., Igamberdiev, A. U., and Debnath, S. C. (2017). Structural pattern, and genetic diversity in blueberry (Vaccinium) clones and cultivars using EST-PCR and microsatellite markers. Genet. Resour. Crop Evol. 64, 1–12. doi: 10.1007/s10722-017-0497-1
Tamayo-Ordóñez, M. C., Espinosa-Barrera, L. A., Tamayo-Ordóñez, Y. J., Ayil-Gutiérrez, B., and Sánchez-Teyer, L. F. (2016). Advances and perspectives in the generation of polyploid plant species. Euphytica 209, 1–22. doi: 10.1007/s10681-016-1646-x
Tanksley, S. D. (2004). The genetic, developmental, and molecular bases of fruit size and shape variation in tomato. Plant Cell 16(Suppl.), S181–S189. doi: 10.1105/tpc.018119
Teyssier, E., Bernacchia, G., Maury, S., How Kit, A., Stammitti-Bert, L., Rolin, D., et al. (2008). Tissue dependent variations of DNA methylation and endoreduplication levels during tomato fruit development and ripening. Planta 228, 391–399. doi: 10.1007/s00425-008-0743-z
Thompson, E. P., Llewellyn Smith, S. G., and Glover, B. J. (2012). An Arabidopsis rhomboid protease has roles in the chloroplast and in flower development. J. Exp. Bot. 63, 3559–3570. doi: 10.1093/jxb/ers012
Uitdewilligen, J. G., Wolters, A.-M. A., Bjorn, B., Borm, T. J. A., Visser, R. G. F., and van Eck, H. J. (2015). Correction: A next-generation sequencing method for genotyping-by-sequencing of highly heterozygous autotetraploid potato. PLoS ONE 8:e0141940. doi: 10.1371/journal.pone.0141940
USDA (2016). United States Department of Agriculture: Fruit and Tree Nut Data. Avaiable online at: https://data.ers.usda.gov.
Van de Poel, B., Bulens, I., Oppermann, Y., Hertog, M. L., Nicolai, B. M., Sauter, M., et al. (2013). S-adenosyl-l-methionine usage during climacteric ripening of tomato in relation to ethylene and polyamine biosynthesis and transmethylation capacity. Physiol. Plant. 148, 176–188. doi: 10.1111/j.1399-3054.2012.01703.x
VanRaden, P. M. (2008). Efficient methods to compute genomic predictions. J. Dairy Sci. 91, 4414–4423. doi: 10.3168/jds.2007-0980
Wang, W., Cai, J., Wang, P., Tian, S., and Qin, G. (2017). Post-transcriptional regulation of fruit ripening and disease resistance in tomato by the vacuolar protease SlVPE3. Genome Biol. 18:47. doi: 10.1186/s13059-017-1178-2
Wang, Y., Wang, W., Cai, J., Zhang, Y., Qin, G., and Tian, S. (2014). Tomato nuclear proteome reveals the involvement of specific E2 ubiquitin-conjugating enzymes in fruit ripening. Genome Biol. 15:548. doi: 10.1186/s13059-014-0548-2
Wang, Y., Zhang, W. Z., Song, L. F., Zou, J. J., Su, Z., and Wu, W. H. (2008). Transcriptome analyses show changes in gene expression to accompany pollen germination and tube growth in Arabidopsis. Plant Physiol. 148, 1201–1211. doi: 10.1104/pp.108.126375
Würschum, T., Liu, W., Gowda, M., Maurer, H. P., Fischer, S., Schechert, A., et al. (2012). Comparison of biometrical models for joint linkage association mapping. Heredity 108, 332–340. doi: 10.1038/hdy.2011.78
Keywords: autopolyploid, allelic dosage, SNP calling, genetic association, gene action, breeding, Vaccinium
Citation: Ferrão LFV, Benevenuto J, de Bem Oliveira I, Cellon C, Olmstead J, Kirst M, Resende MFR Jr and Munoz P (2018) Insights Into the Genetic Basis of Blueberry Fruit-Related Traits Using Diploid and Polyploid Models in a GWAS Context. Front. Ecol. Evol. 6:107. doi: 10.3389/fevo.2018.00107
Received: 31 January 2018; Accepted: 02 July 2018;
Published: 24 July 2018.
Edited by:
Hans D. Daetwyler, La Trobe University, AustraliaReviewed by:
Peter Bradbury, United States Department of Agriculture, United StatesCopyright © Ferrão, Benevenuto, de Bem Oliveira, Cellon, Olmstead, Kirst, Resende, and Munoz. This is an open-access article distributed under the terms of the Creative Commons Attribution License (CC BY). The use, distribution or reproduction in other forums is permitted, provided the original author(s) and the copyright owner(s) are credited and that the original publication in this journal is cited, in accordance with accepted academic practice. No use, distribution or reproduction is permitted which does not comply with these terms.
*Correspondence: Patricio Munoz, cC5tdW5vekB1ZmwuZWR1
†These authors have contributed equally to this work.
Disclaimer: All claims expressed in this article are solely those of the authors and do not necessarily represent those of their affiliated organizations, or those of the publisher, the editors and the reviewers. Any product that may be evaluated in this article or claim that may be made by its manufacturer is not guaranteed or endorsed by the publisher.
Research integrity at Frontiers
Learn more about the work of our research integrity team to safeguard the quality of each article we publish.