- 1Station of Experimental and Theoretical Ecology, Centre National de la Recherche Scientifique and Paul Sabatier University, Moulis, France
- 2INRA, UMR UAPV-INRA EMMAH, Centre PACA, Avignon, France
- 3Centre de Recherche en Écologie Expérimentale et Prédictive (Ecotron IleDeFrance), Ecole Normale Supérieure, CNRS, PSL Research University, UMS 3194, Saint-Pierre-lès-Nemours, France
- 4INRA, UR P3F, Centre Poitou-Charentes, Lusignan, France
- 5Plateforme Biochem-Env, UMR ECOSYS, INRA, AgroParisTech, Université Paris-Saclay, Versailles, France
- 6INRA, UAR 1275 EFPA, Centre de Nancy, Champenoux, France
- 7INRA, URFM, Centre PACA, Avignon, France
- 8Ecotron Européen de Montpellier, Centre National de la Recherche Scientifique, Montferrier-sur-Lez, France
- 9INRA, UR BEF, Centre de Nancy, Champenoux, France
Human activities have altered continental ecosystems worldwide and generated a major environmental crisis, prompting urgent societal questions on how to best produce goods while at the same time securing sustainable ecological services and raising needs to better understand and predict biodiversity and ecosystems dynamics under global changes. To tackle these questions, experimentation on ecosystems is necessary to improve our knowledge of processes and to propose scientifically sound management strategies. Experimental platforms able to manipulate key factors of global change and including state of the art observation methodologies are available worldwide but how to best integrate them has been rarely addressed. Here, we present and discuss the case of the national research infrastructure AnaEE France dedicated to the study of continental ecosystems and designed to congregate complementary experimental approaches in order to facilitate their access and use through a range of distributed and shared services. The conceptual design of AnaEE France includes five modules. Three modules gather experimental facilities along a gradient of experimental control ranging from highly controlled Ecotron facilities, semi-natural field mesocosms to in natura experimental sites covering major continental ecosystems (forests, croplands, grasslands, and lakes). In addition, AnaEE France also includes shared instruments that can be implemented in experiments and analytical platforms specifically dedicated to environmental biology. To promote reuse of data, generalize results and improve predictive models, AnaEE France further gathers modeling and information systems. The implementation of AnaEE France allowed for mutual synergies, improved the technical skills, stimulated new experiments and helped our scientific community to enter into the big data sharing era.
Introduction
Ecosystems provide key ecological services to human societies including provisioning services (e.g., biomass production) and the regulation of climate conditions and element cycles (Balmford and Bond, 2005; Cardinale et al., 2012). Human activities are the direct or indirect cause of various environmental pressures, including pollution, global warming, or the degradation of natural habitats (Vitousek et al., 1997; Pereira et al., 2010). Altogether, this has caused a rapid erosion of biodiversity and a major perturbation of most ecological systems and services, at the same time as increasing demands for food and energy and stronger competition for land and water use are expected in the near future (Howden et al., 2007; Ehrlich and Harte, 2015). Understanding ecological responses to global changes, and identifying possible mitigation or adaptation strategies are therefore becoming a crucial component of the research agenda (e.g., Olesen et al., 2011; Mooney et al., 2013).
Ecological studies indicate that living organisms are crucial drivers of ecosystem processes, hence pointing toward studies that address how biodiversity and ecosystems respond and eventually adapt (Loreau, 2010). To understand and predict ecosystem responses to a changing world, four scientific challenges of biodiversity research must be addressed. At the species level, we need first to understand phenotypic flexibility in response to environmental changes. When it comes to understand phenotypic variation, evolutionary theory begs for the simultaneous study of genetic factors, physiological trade-offs (i.e., the concurrent use of energy and resources by different traits) and developmental plasticity (i.e., the ability of a genotype to exhibit different phenotypes in different environments) since this is the only way to account for the interplay between genetic and non-genetic factors (e.g., Schlichting and Pigliucci, 1998; Vandenkoornhuyse et al., 2010). Second, studying the momentous impacts of biotic interactions on ecosystems dynamics entails detailed investigations of trophic and non-trophic interactions, which is a major challenge in the field of biodiversity science that attempts to predict the relationship between biodiversity and the functioning of ecosystems, including biogeochemical cycles (Hooper et al., 2005). Third, one fundamental aspect of living organisms is their ability to evolve by means of natural selection. Recent empirical studies in natural populations have shown that, provided genetic variability is sufficiently high, selection can sometimes be fast enough to interact with ecological processes (Post and Palkovacs, 2009). Thus, natural selection could alter the speed at which ecological systems respond to global changes if the genetic variation is not exhausted too quickly by such changes (Gonzalez et al., 2013). Fourth, how landscape features, such as habitat fragmentation (Legrand et al., 2017), interact with ecosystem dynamics, and especially with biogeochemical cycles, remains to be understood (Thompson et al., 2017).
Experimental approaches in ecology provide one of the best mean to achieve these goals (Schoener, 1983), although they have sometimes been criticized due to their lack of generality and limited spatial and temporal scales (e.g., Carpenter, 1996; Schindler, 1998). The use of experimental approaches in ecology and environmental sciences increased as a way to test predictions of the core theoretical concepts of population biology, population genetics, evolutionary biology, ecosystem science and food web theory, which arose in the 1960s (Begon et al., 1996). Now that modeling and analytical progresses lead to better and more accurate understanding and prediction of matter and energy processes through interdisciplinary approaches (e.g., Bashkin, 2002), a major focus in ecological sciences is on the production of quantitative, experimentally testable approaches using advances in our ability to characterize better the influence and cascading effects of heterogeneity at lower levels on higher levels of complexity (from genes to ecosystems, see Loreau, 2010). This challenge strongly urges the need for building novel, collaborative experimental infrastructures since no single effort will be able to provide us the necessary set of tools and data to solve interdisciplinary questions in our research community.
Up to now, most attempts to build generic experimental facilities have been strongly scale and approach specific, and little effort has been made to promote complementarities among experimental facilities in terms of replication, scales of approaches, levels of complexity, types of ecosystem, data management and modeling, as well as methods and tools for assessing biodiversity and ecosystem matter fluxes (Blanchfield et al., 2009; but see Stokstad, 2011). We propose here that the implementation of a network of experimental facilities cannot solely copy and paste from the success stories of observatory networks in our discipline, which have been reviewed elsewhere (e.g., Lindenmayer et al., 2012; Peters et al., 2014). Although complementary to observations, the logic behind experiments involves different constraints and opportunities, and thus calls for different solutions. The aim of this manuscript is therefore to provide a framework for the development of a network of experimental facilities open to a research community studying ecosystems' biology and ecology. This framework will be illustrated by the case of the Research Infrastructure (RI) AnaEE France, which is a set of open-access platforms offering services to experiment on, analyse, and model ecosystems (Mougin et al., 2015).
Current Networking Efforts in Environmental Sciences and Ecology
The large spatial and long temporal scales of global change impacts on ecological systems implies that results from site-based research must be scaled up in order to predict ecological processes operating on yearly to century time scales at a continent scale or even more (Peters et al., 2014). Local ecological observations have proved to be essential for studying diverse ecological phenomena such as plankton or terrestrial plant successions, cyclic predator-prey population dynamics or lake eutrophication (Magnuson, 1990). Some of the longest term observations started very early: for example, records in lake Suwa in Japan began in 1443 and those of the Anagara River in Siberia began in 1720 (Magnuson et al., 2000). However, long-term ecological research per se was initiated in the Rothamsted experimental farm in England in 1843 (Taylor, 1989), and it has flourished since then (reviewed by Clutton-Brock and Sheldon, 2010 for population studies). Most of these long term observations were not performed in coordinated networks until recently, preventing any straightforward comparisons across time and space by lack of harmonization and/or standardization of scientific practices (Peters et al., 2014). Efforts have been made in the last decades to develop dedicated observation networks (e.g., Lindenmayer et al., 2012). To achieve this goal, key milestones includes (1) the selection of existing or de novo construction of observational sites such that several ecosystem types can be studied and compared; (2) the standardization of existing methods to collect data across sites; and (3) the development of tools for prediction, databases, and a centralized policy management (Baker et al., 2000).
Among existing infrastructures, LTER (Long-Term Ecological Research) is funded since 1980 and gathers 24 long-term study sites in USA encompassing diverse continental and oceanic ecosystems (Baker et al., 2000). More recent initiatives include ICOS (Integrated Carbon Observation System, http://www.icos-infrastructure.eu/) dedicated to the monitoring of greenhouse gases budgets in 12 European countries since 2008 (Ciais et al., 2014), NEON (the National Ecological Observatory Network) designed to provide long-term ecological data on the US continental scale since 2012 (Kampe et al., 2010; Kao et al., 2012), and TERN (http://www.tern.org.au) contributing since 2008 to deliver observation data on all Australian ecosystems (Lindenmayer et al., 2012). Other networks aim at understanding specific ecosystem types such as GLEON (Global Lake Ecological Observatory Network; http://gleon.org), which is a grassroots network of limnologists, ecologists, information technology experts, and engineers who have a common goal of building a scalable, persistent network of lake ecology observatories (Weathers et al., 2013).
Most of the above mentioned coordinated networks are devoted to observational studies and do not include experimental designs and experimental sites, and most experimental approaches are not commonly conducted at the same spatial and temporal scales than observational programs (Pinto et al., 2014). Some long-term experiments have been done for example on ecosystems fragmentation (Brudvig et al., 2015) or forest dynamics (e.g., Magill et al., 2004), but they were usually not coordinated. A notable exception is the nutrient network experiment (NUTNET), which is a collaborative project at more than 40 grassland sites across North America, Europe, Australia, South America, Asia, and Africa, http://www.nutnet.umn.edu/home) to perform identical field experiments. NutNet is a unique effort to establish a general understanding of how fertilization (e.g., nitrogen or phosphorus runoff) and herbivory jointly control plant communities and ecosystem services (Stokstad, 2011). The case of the Experimental Lake Area (ELA) in Ontario should also be mentioned (see Blanchfield et al., 2009). Since it was created in 1968, more than 50 experiments were conducted at the ELA ranging in duration from several years to more than four decades. Through its ability to conduct whole-ecosystem experiments, this network has helped to understand many environmental concerns such as algal blooms associated with eutrophication, the effects of acid rains on lakes, the environmental impacts of aquaculture and dam development, or the effects on synthetic hormones on fish.
In parallel to this, laboratory experiments were performed since the beginning of modern ecological sciences (for example see Park, 1962), but most were uncoordinated among each other as well as with experiments done in semi-natural or natural conditions (Schoener, 1983). Laboratory or field experiments were designed for a single researcher-question approach and have been extremely successful, but they were not meant to be repeated by other researchers or to be used to respond to wider set of questions. Important initiatives that span multiple research questions exist and include for example the Silwood Park Ecotron dedicated to biodiversity research (Lawton, 1996), the Cedar Creek long-term experiment (Tilman et al., 2012), the Jena experiment (Roscher et al., 2005), the Harvard Forest laboratory (Stott, 1991), or the Landscape Earth Observatory (Pangle et al., 2015). Yet, until very recently, there was little attempt to coordinate experimental research in the field of ecological and environmental sciences, and few examples of coordinated experimental projects. Below, we focus on a new French project called AnaEE France (Analysis and Experimentation on Ecosystems), which was initiated in parallel to the European project called AnaEE in an attempt to improve synergies and global impacts of experimental research in our discipline (Chabbi and Loescher, 2017a).
Main Objectives and Implementation of Ecosystem Experimental Research Infrastructure
Ecologists working on ecosystems have only recently recognized the necessity of network-based approaches for the building of common instrumentation and facilities (Swanson and Sparks, 1990; Robertson et al., 2012; Peters et al., 2014). However, this approach has been rarely applied to experimental platforms (Chabbi and Loescher, 2017a). Clear advantages to gather a community around a distributed research infrastructure include (1) the improvement of integration and complementarities among experimental set-ups and instruments, and hence an increase in research efficiency, (2) a more inclusive and collaborative decision-making process to build new experimental facilities or abandon old fashioned ones, (3) the construction of information systems for the sharing of data and models, and (4) the optimization of funds. Here, we propose that the building of an experimental infrastructure in ecological sciences, such as our case study of AnaEE France, must respond to the three major challenges listed below and we suggest a method to do so based on the work done in France as well as the conclusions of a similar, ongoing integration process at the European level (Chabbi et al., 2017b).
Allowing for Integrative Study of Biodiversity and Ecosystem Functioning
Ecosystems are characterized by four main essential characteristics. First, their dynamics are typically difficult to predict (Scheffer and Carpenter, 2003; Pereira et al., 2010) due to, for example, tipping points, high sensitivity to initial conditions, or complex non-linear response curves. Second, most ecological processes are characterized by a strong spatial structure. Many living organisms have limited dispersal ability and display complex dispersal responses with respect to variation in the environment (Clobert et al., 2012). Thus, some ecological processes cannot be understood properly if the spatial structure is ignored (Peterson, 2000). Third, there is a strong functional heterogeneity among individuals, among species and among trophic levels which all have a major influence on the functioning and stability of ecosystems services (Loreau, 2010). For example, inter-individual and inter-specific heterogeneity in plastic responses to social and physical environments are currently pointed out has having major effect on ecosystem functioning (Jacob et al., 2015). Fourth, the interplay between the evolution of this biological diversity and ecological dynamics can be the key to predict the ecosystem state even on the short term (Post and Palkovacs, 2009; Schoener, 2011). For example, global changes shift the state of the environmental, which leads to changes in the shape and strength of selection on individual and species traits and feedbacks into ecological dynamics (Reiss et al., 2009). To account for these four essential properties, we acknowledge that integrated experimental set-ups should offer a high level of replication over multiple gradients of temporal and spatial scales and strong capacity to unravel complex biological processes.
Coping With Trade-Offs Imposed by the Size and Complexity of Experimental Units
Ecologists have developed experimental tools ranging from chemostats on a bench or complex climate chambers of Ecotrons to more or less complicated field set-ups. Such tools have been rarely taught in term of complementarities or in term of choosing the appropriate level of testability, replication, realism and multi-disciplinarity. Experimental approaches at small spatial and temporal scales (e.g., laboratory microcosms) offer a high degree of replication and environmental control, and are extremely powerful tools to validate general theories (Caswell, 1988), but they might lack realism and complexity (Figure 1, see Benton et al., 2007; Drake and Kramer, 2012). On the other hand, large scale, field experiments offer a high degree of natural complexity but are often poorly controlled and replicated (Osmond et al., 2004; Leuzinger et al., 2011; Haddad et al., 2017). More complex, larger experimental set-ups also often allow for more multidisciplinary research programs, with some large-scale field experiments often congregating a wider community of users from ecology, geosciences or even social sciences. In addition, it has been suggested that small-scale experimental approaches have a stronger internal validity (i.e., a high certainty to attribute causal effects to a given set of factors) but often lack external validity (i.e., a lower generalization capacity, De Boeck et al., 2015). In between these two extreme scales, mesocosm approaches (semi-natural experimental facilities) are an oft-used solution to manipulate a few biotic and/or abiotic factors while leaving some natural fluctuations operating on the system (Stewart et al., 2013). Technical and budgetary constraints impose a strong trade-off between replication power and biological complexity, and the environmental control and measurement capacity of experimental units (reviewed in Petersen et al., 1999; Stewart et al., 2013).
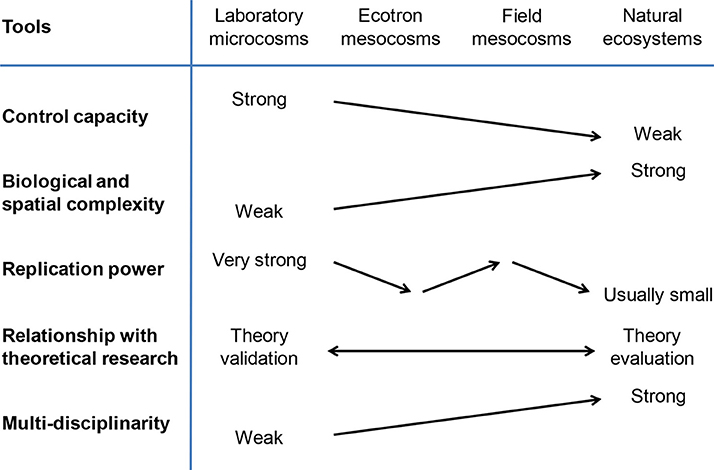
Figure 1. The design of an experimental set-up typically involves several trade-offs. Here, experimental set-ups were arranged by size from small laboratory microcosms, highly controlled laboratory mesocosms inside Ecotrons, field mesoscosms and natural ecosystems. Control capacity describes the level of environmental control that the experimental set-up can offer and it typically decreases from enclosed, laboratory systems to natural ecosystems (Petersen et al., 1999). Complexity defines the number of species, trophic levels or ecosystem types, as well as the spatial complexity in the environment, and it typically increases from laboratory to natural systems. Replication power is related to the maximum number of experimental units and, given budgetary and technical constraints, it decreases with the spatial scale and technological complexity of the experimental set-up. Internal validation defines the extent to which the experimental set-up can be used to test for a given theory and it trade-offs with theory evaluation as spatial scale increases (De Boeck et al., 2015). Multidisciplinarity is the extent to which several disciplines can work together in the experimental set-up, and it increases on average with spatial scale (Stewart et al., 2013).
Altogether, this implies (1) that not a single experimental set-up can optimize all components and we will need new experimental tools characterized by a capacity to somehow escape from technical and budgetary constraints (Haddad, 2012), and that (2) the optimization of each component of the experimental set-up should be carefully thought given the ecosystem type, research question, and available technologies. More generally the novelty of experimental approaches will lie in both their possibility to recreate ill investigated ecosystems as well as enabling to study complex biotic and abiotic interactions with a high level of replication. Such experimental set ups are very costly and can only be programmed and managed by an entire scientific community. These new types of experimental set-ups will be key for speeding up research on ecosystems and addressing key scientific challenges.
Allowing for Feedback Loops Between Theory and Experimentation
Many theories have flourished in ecology during the last decades, but most have only been partially tested and some are even untested. Theory validation has been attempted in chemostats, Ecotrons, or in semi-natural conditions, but theory evaluation (i.e., assessment of the part of variance explained in the natural systems variation) still remains rare (Figure 1, but see Schmid et al., 2002; Tilman et al., 2014). It is therefore urgent to organize experimental set-ups in such a way that they can be complementary and compatible with models and allow more efficient model-data interactions.
A Proposed Method
Challenges to develop experimental approaches spanning multiple scales, covering a range of processes and complexity, and tightly coupled with ecological models are multiple. A research infrastructure can cope with these challenges more easily than independent site-based approaches if it provides an efficient access to cutting edge experimental facilities and modeling tools, and if it facilitates the scientific process by offering fluent links between data collected in different experiments and models. We propose a method based on six key ingredients to achieve these overarching goals:
1. A selection of front edge resources including experimentation, analytical and data-models platforms. The core elements of the research infrastructure (RI) should include experimentation platforms ranging from highly controlled experimental setups to long term experiments in natural ecosystems but also analytical tools (dedicated to data acquisition) and information systems dedicated to data management, model implementation and experimentation-data-model integration. Each experimentation platform must present outstanding characteristics and a strong originality (see for example, Legrand et al., 2012; Verdier et al., 2014). In addition, each experimentation platform must be supported by a strong and skilled staff to perform high quality research. The RI will have the responsibility to manage the life cycle of all platforms by promoting new ideas, facilitating the construction of new platforms and evaluating their quality and management.
2. A standardized and centralized access policy to the platforms. Access to each platform must follow standardized procedures similar to the open-access policy recently adopted for data access and services should be open to both the academic and private sectors. The RI should be the appropriate entity to decide for shared procedures dedicated to project submission, pricing policy and data dissemination.
3. A standardized catalog of resources, data and models. The complex management and operation of the RI requires to describe each of its component using standardized metadata. This description includes the platforms themselves, the results of the experiments performed in each platform, and the models. The RI should provide strict metadata guidelines, make them semantically consistent and expose them in efficient querying interface for the discovery and access to the RI resources, data and models.
4. Harmonization of measurements and methods. In order to facilitate the handling of data of different origins and to ensure their comparability, the infrastructure should define a policy for the acquisition, processing and qualification of measurements. It is important to share and harmonize protocols. Harmonization implies to identify equipment, instruments and data, and may include a standardization of the protocols, for example standard procedures to use specific sensors. In particular, a procedure to design and describe experimental protocols must be adopted and shared.
5. Promoting data access and reuse. The infrastructure data policy has to contribute to the development of an open-science through the sharing and reuse of data from ecosystem studies. Intellectual property rules and data sets' identifiers must guarantee that most data produced by the RI platforms will be accessible and citable by the international research community. Data can be directly delivered by a database interface proper to the infrastructure, through web services or in modeling environments that integrate data in modeling activities.
6. Contributing to the agenda of the scientific community. The RI should attract a scientific community that shares an interest on the use of experimental platforms and their management. It is therefore a central place to foster discussions on a range of topics such as cutting edge science which can be done with the infrastructure, experimentation-model coupling, implementation of new technologies and data synthesis (see for example this review dedicated to fragmentation experiments produced by AnaEE France, Haddad et al., 2017).
The Case Study of AnaEE France
The RI AnaEE France was built according to the above methodology in order to join efforts from various French research organizations to upgrade and integrate existing experimental tools on ecosystems in France. During the last 25 years, we developed independently some experimental facilities in France to study various types of continental ecosystems. These facilities were usually built by single research teams without considering of their overall complementarities. The construction of AnaEE France thus involved a first step to define criteria for selecting among existing platforms followed by a second step to define a general and complementary organization and propose a central management plan. We discuss below each of these steps and conclude by showing the added values of the RI for the research community.
Selection and Organization of the Resources
Experimental facilities in AnaEE France focused on continental ecosystems (aquatic and terrestrial) and were selected for their originality, large community of users, and open access to the international research community. They were also chosen on the basis of their complementary tools and approaches to offer opportunities for scaling up and down from in vitro to in natura approaches within a unique infrastructure and with the same access rules, and to allow feedbacks between models and experiments. In order to compare data by using a common framework, and to use comparable measures and standards across platforms, we anticipated the need for a common set of analytical platforms and a common procedure for collecting data using the same instruments with a mobile laboratory. Thus, the distributed and coordinated network of experimental platforms of AnaEE France was associated with a selection of some analytical and modeling platforms.
Existing experimental platforms were first ranked along a control axis (Figure 2) leading to three categories of platforms including controlled environment facilities (Ecotrons), semi-controlled field mesocosms in which some environmental and biotic factors could be manipulated and field experiments with in natura ecosystems. Each experimental platform must allow for the simultaneous manipulation and monitoring of ecosystem processes through a multi-disciplinary approach (see Mougin et al., 2015 for more technical details on each platform type). Experimental platforms were selected based on their ability to manipulate a range of distinct, representative ecosystems types including forests, grasslands, croplands and aquatic ecosystems (Mougin et al., 2015). In addition, we included analytical platforms offering tools to describe the most relevant biotic and abiotic conditions (including metabarcoding, Yang et al., 2014) and we designed a new e-service dedicated to data management and modeling. Modeling services were based on platforms hosting models and modules, offering model coupling facilities, direct access to the data and statistical tools (sensitivity analysis, parameter estimation, error assessment, output visualization). The modular structure of the proposed RI allowed for a better internal organization, but synergies between modules were promoted through regular workshops and meetings (see below). A full list of the services is summarized in Mougin et al. (2015) and is also available on a dedicated web site (http://www.anaee-france.fr/).
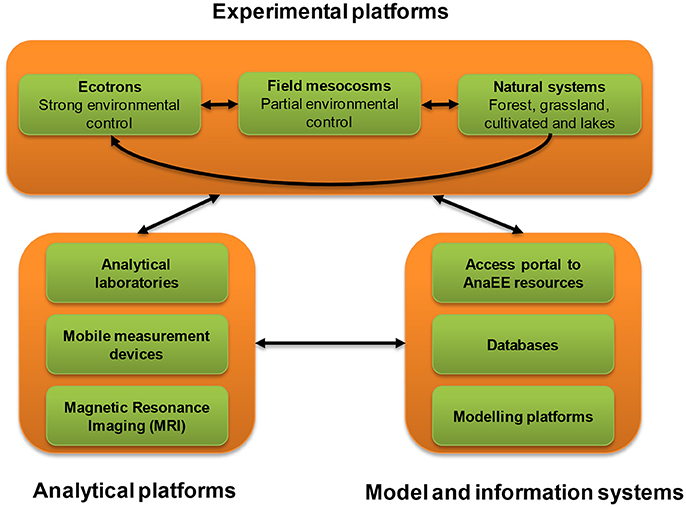
Figure 2. General organization of the experimental facilities along a gradient of size of the experimental set-ups complemented by analytical facilities, modeling platforms and information systems. The degree of control of environmental conditions diminishes from Ecotrons to field experiments (see Figure 1).
General Organization, Types of Services, and Access Policy
The first goal of the construction of the infrastructure was to build a coherent set of experimental, analytical and data-model platforms with respect to the different key conditions defined in sections Main objectives and Implementation of ecosystem experimental research infrastructure. The second goal was to ensure a smooth infrastructure management and an open access policy. We first defined the three main kinds of AnaEE France services, which include (1) access to experimental and analytical facilities, instruments, and modeling platforms; (2) access to archived samples and collections of biological resources; and (3) access to data sets. The two first kinds of services require matching the demands with the offers in terms of technical, financial and logistic needs, while the data access is regulated by a general license defining the rights and duties associated with the use of data. To implement the data access policy, users are asked to register and commit to properly cite the source and authorship of the downloaded data sets. We developed a single access point with updated information on services and fees, open calls for projects, and a review process to evaluate the scientific and technical relevance of proposed projects (see Figure 3). The general policy for access fees (i.e., the economic model of the RI) is to ask users to cover part of the running costs of the services for projects coordinated by academics and to charge additional fees corresponding to manpower and renewal of instruments for projects coordinated by private partners. Approved users are requested to sign an agreement with AnaEE France including information on data and patent protection, copyright and ownership. A core part of the general policy for access rules and fees is mandatory to all services but additional specificities can be included by each platform. Each platform has a dedicated panel of reviewers with peer-reviewers in charge of selecting projects and scheduling the access to the platform. The novelty of this approach is not only about the common rules related to access policy and data management but stands in the construction of unique web portal an data base dedicated to project submission and implementation (see Figure 4).
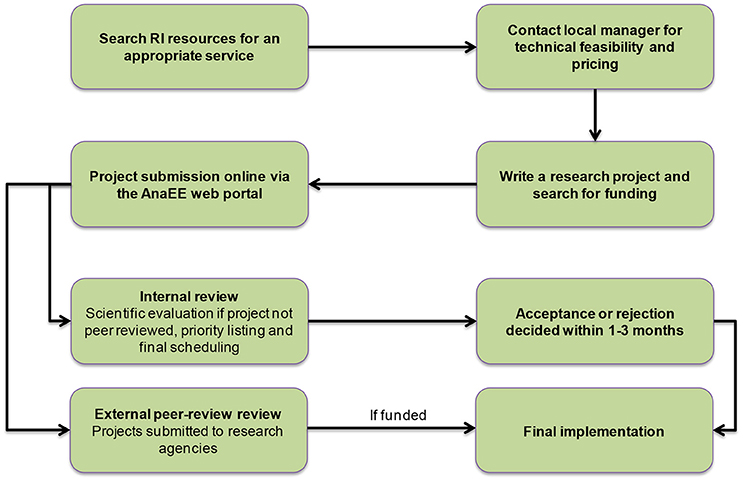
Figure 3. Project submission procedure. The flow chart illustrates the standard project submission review and selection which includes either external review for funding or internal review when funding is not based on external, peer-review.
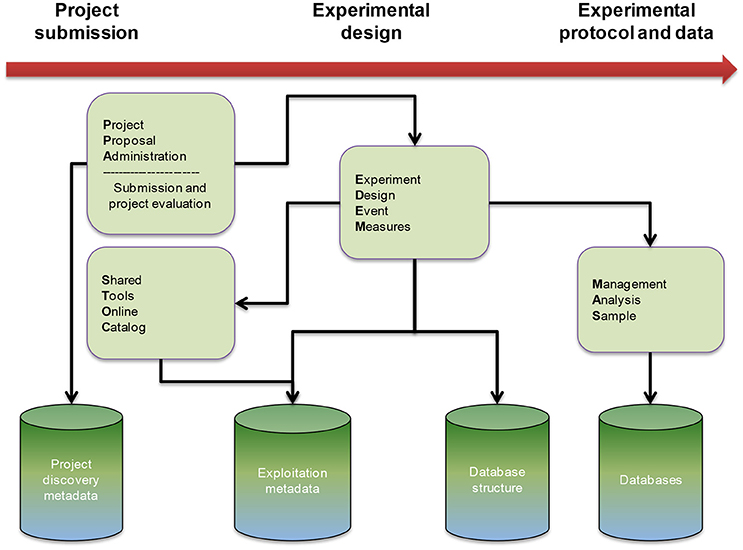
Figure 4. Information system for the administration of AnaEE RI including dedicated web applications for project submission (Project Proposal Administration), project implementation (Experiment Design Event Measures) and data management (Management Analysis Sample). The diagram shows the flow path from project submission to data production and storage, and associated databases dedicated to project and exploitation metadata, database structure and raw data.
Information Systems
The AnaEE France information system is based on a distributed architecture gathering information about databases located in different centers, some modeling platforms and a portal for metadata and access to the resources (Figure 5). The databases information system was designed to store data, to make them available and to manage access rights. Two complementary systems were developed in parallel for the management of data generated by experimental platforms, including one dedicated to long-term experiments (i.e., decades) conducted with in natura platforms (accessible for instance at https://si-acbb.inra.fr for experiments on agrosystems) and one dedicated to short-term (i.e., months or years) research projects operated with Ecotrons and field mesocosms. Since the goal of the information systems for long term experiments is to deliver core data, tools were developed for on line data stream, the standardization of variables and metadata across platforms, data querying and the management of rights. For short-term experiments, a dedicated web application, called ISIA (Information System for Infrastructure Administration), was designed and implemented (see Figure 4). The aim of ISIA is to collect the information of the whole experimental cycle within a single environment and database including the project submission, the experimental design, the experimental protocols and the raw data. To identify all data available in the information systems, a web portal was developed. Thanks to semantically rigorous annotation, data can be found either from predefined filters or through open queries. To increase the functionalities of the modeling platforms and promote data reuse, web services were designed to transfer data from the information systems to the modeling platforms.
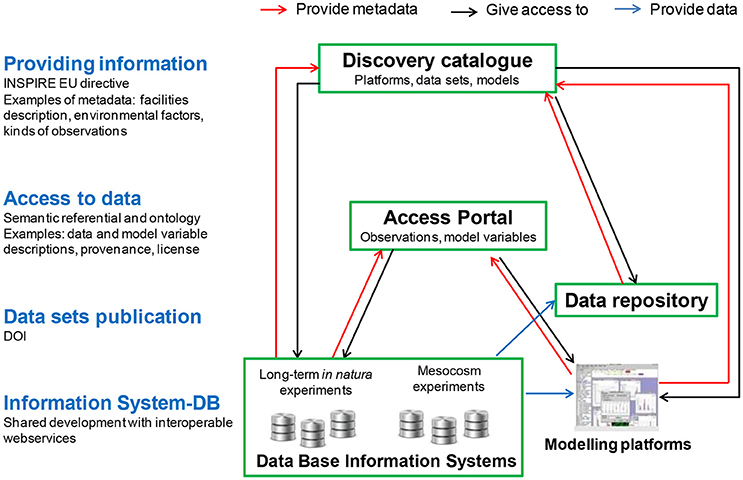
Figure 5. The distributed architecture of the AnaEE-F information system includes a discovery catalog to access metadata information about platforms, datasets, or models, a portal to access metadata about observations or model variables including a semantic referential and an ontology, and a data repository to store digital object identifies (DOI) of data sets from information systems of in natura and mesocosm experiments. Data sets from experiments are linked with model factories to enable model parameterisation or data assimilation.
Metadata
The annotation of resources using metadata (data about data) is a key to promote their discovery and reuse. When based on common standards, it directly contributes to build an efficient interoperability between raw data, models and experiments. Metadata is used in three main mechanisms including discovery (identification of the data sources that contain a given information), exploration (evaluation of the match between data and users' needs) and exploitation (use and access conditions of the data sources). Exploitation of metadata are usually managed within the data information systems, whereas discovery metadata are most frequently managed in a dedicated environment allowing compatibility with international standards and the harvesting by different catalogs (e.g., GEOBON at a global scale in the field of biodiversity research). We used two international metadata standards developed for environmental sciences and ecology: the geospatial metadata standard ISO19115/19139 compatible with the EU INSPIRE directive (Anonymous, 2013), and the Ecological Metadata Language (EML, Fegraus et al., 2005). Currently, the description of all resources of AnaEE France (http://w3.avignon.inra.fr/geonetwork_anaee) follows uses of the GeoNetwork (http://geonetwork-opensource.org/) software.
In addition to these standards, there are international initiatives to develop metadata standards such as OGC's Sensor Web Enablement (SWE) to implement data flow from sensors to web interface and a series of Metadata Language (e.g., SensorML) to describe sensors and associated data sets. However, these standards do not fix the vocabulary and semantic links between concepts, and there exist several initiatives led by different groups of ecologists, agronomists or geoscientists to develop thesaurus and ontologies. To fulfill our specific needs, we therefore developed our own semantic referential tools by using vocabularies derived from different existing thematic thesauri (GEMET, EnvThes, AGROVOC, TAXREF, etc.) and by collecting the vocabularies used in the different distributed platforms of the infrastructure. These terms were then gathered in an AnaEE France Thesaurus, which is supported by the VOCBENCH software and shared with the whole AnaEE community. The AnaEE thesaurus is publicly accessible through the AgroPortal semantic repository (http://agroportal.lirmm.fr/; Jonquet et al., 2016). In order to provide an accurate description of data resources and to allow their interoperability with modeling platforms, existing databases and models were semantically annotated using ontologies. We used the OBOE ontology (https://github.com/NCEAS/oboe/; Madin et al., 2007; Schildhauer et al., 2016) developed for scientific observations and measurements in ecology as the core element of our ontology. Extensions to OBOE are developed for the specific needs of AnaEE France and others thematic ontologies are also used such as wgs84_pos for spatially-located objects to describe their spatial patterns and SSN-SensorML to describe sensors and methods. The AnaEE ontology is developed using the Protégé collaborative software and will be accessible through AgroPortal as well.
Harmonization
Current monitoring, measurements and experimental protocols in AnaEE France are not standardized due to various historical, logistical or monitoring constraints. Substantial improvements are expected on the short-term in order to improve data traceability and facilitate comparisons across experiments. Harmonization of data and protocol description is an outcome of the use of the shared information systems, which provide an integrated, semantically correct and shared description of each data set. Standardization of protocols and measurements, including accuracy assessment, curation procedure and the use of shared instruments will be a key objective to improve data quality and make them comparable across datasets. For example, methodological developments are implemented on different platforms to improve methods as for soil moisture sensor calibration or soil gas sampling techniques. These studies can take advantage of the variety of sites contexts and newly improved methods can then be more quickly deployed in the different platforms.
Community Building Activities
AnaEE France involves a large technical and scientific community dedicated to the platform management (about 300 permanent staff) and a wide community of users (about 300–400 projects per year). The high technical skill, the variety of experimental approaches and the range of scientific domains addressed by the AnaEE France community give it the legitimacy to play a leading role in the scientific community on topics related to the experimentation on ecosystems and methodological developments. In AnaEE France, significant effort has been therefore paid for community building activities. Working groups on methodological issues most relevant for the development of the infrastructure have been established. These groups address topics about measurements and experimental protocol standardization, the development of new instruments or experimental set-ups, or the use of model organisms and ecosystems. For example, working groups were established to address some of them as for instance the review of concepts and methods to measure biodiversity, dedicated sensors, and tools to analyse and model biodiversity data.
The RI also raises opportunities to develop both training and teaching based on technologies and data available in all platforms. In AnaEE France, the infrastructure was used to organize and offer some training on best techniques or practices, and to foster or develop experiment-oriented teaching. Outreach activities were also developed for the general public. By their very nature, research infrastructures offer also other possibilities of developing synthesis works such as comparisons of ecosystem indicators or reviews of management practices. In the field of ecosystem science, an infrastructure can help developing general measures of the state of the environment such as CO2 storage capacities, or tools to characterize and measure biodiversity. These tools can be made available to scientists as well as to public agencies and policy makers.
Performance Indicators and Some Examples
The RI AnaEE France was officially started at the end of 2011 and we have been producing summary statistics since then, which may indicate trends in the performance of experimental research tools over the last four years (Table 1). Since the beginning, financial and human support by our funding agencies has been steady (annual budget around 10 M€ and full-time equivalent manpower of about 145 persons), and most of the resources from the RI have been invested into the upgrading of existing platforms and the construction of new platforms (22 services including 3 newly constructed). At the same time, the leverage effect on regional and national funding programmes for associated platforms was important and in constant increase (from 2 to 5 M€) and the revenues secured from user fees and external projects increased from 0.2 to 1.4 M€ due to some average increase in occupancy rate, a new fee policy and an increase in the number of funded projects. A fairly high number of research projects was hosted each year with fluctuations caused by inter-annual changes in the average duration of each project. A reasonable number (20%) of projects involved foreign laboratories. In addition, we have importantly increased the use and re-use of data and models generated by each service (10 times increase after 4 years). This was accompanied by an increase in the number of publications including technical publications, and in the performance statistics of training activities. Current efforts will continue to raise the number of private sectors projects, improve further harmonizing of measurements and methods, and deploy the newly developed information systems.
Examples of remarkable, recent studies conducted in AnaEE France include experiments on climate change usually difficult to perform within the realm of a single laboratory. For example, collaborations between in natura and Ecotrons platforms make it possible to conduct short-term climate simulation experiments on intact pieces of soil-plant ecosystems extracted from long-term study sites. Using this approach, Roy et al. (2016) recently uncovered that elevated atmospheric CO2 concentrations predicted for the future should compensate the negative effect of a summer drought stress on grasslands, and therefore influences the resilience to warming of this ecosystem. Such extreme weather events are also predicted to increase extinction risks of numerous species on earth, but large scale experimental demonstration are missing and it remains unknown if species can compensate climate warming by means of dispersal (Sinervo et al., 2010). Recently, an experiment performed in one AnaEE France platform (see Legrand et al., 2012) allowing joint manipulations of climate conditions and habitat fragmentation was able to show that neither dispersal nor acclimation can prevent the rapid extinction of lizard populations by 2050 with a further 2.5°C increase in mean temperature (Bestion et al., 2015a,b).
Conclusion and Perspectives
There are still too few attempts to network together relevant experimental facilities from the same country in the scientific fields of biodiversity, agronomy and ecology. Learning from grand challenges in ecological research, we propose guidelines for the construction and operation of such a research infrastructure. In the case of AnaEE France, experimental set-ups were selected from a range of control capacity and a capacity to handle a representative set of ecosystem types. The infrastructure included analytical and modeling platforms, and dedicated information systems. Standardized methods and practices, solutions for data storing and access, modeling platforms, and training activities were developed to increase the quality of all services and promote synergies among existing platforms. Experimental set-ups and services usually have a limited lifetime. By organizing the life cycle of platforms together with the financial bodies, it is expected that a long term sustained and optimized effort in progressing in the understanding of ecosystems and the management of ecological services will be initiated in France. Now in its fifth year of life, AnaEE France is fulfilling its initial objectives and the number of projects and researchers using these services is encouraging.
Experimental infrastructures are not the only tools which have to be developed in a coordinated way to optimize research in the ecology-environment domain. Long term observations of ecosystems and socio-ecosystems are other important infrastructures, and well-established observational networks do exist. Links between experimental and observational infrastructures should be established or strengthened if they already exist because they will generate synergies and enable better dialog between observational and experimental approaches in our field. Such an effort is a key objective to address pressing questions about the state and future of ecosystems.
Author Contributions
JC, AnC, J-FL, AbC, TC, ML, CM, CP, JR, and LS-A conceived the infrastructure. JC, AnC, J-FL, and AbC conceived the general structure of the AnaEE-France infrastucture with the help of TC, ML, CM, CP, JR, and LS-A. JC, AnC, J-FL, and LG wrote the manuscript. TC, ML, CM, CP, JR, and LS-A helped reviewing different versions of the manuscript.
Conflict of Interest Statement
The authors declare that the research was conducted in the absence of any commercial or financial relationships that could be construed as a potential conflict of interest.
Acknowledgments
The authors acknowledge CNRS, INRA, Université Grenoble Alpes and colleagues from AnaEE France for their support. Cécile Callou and Aurélien Maire coordinated the construction of the ISIA software and helped draw Figure 4 in this paper. AnaEE France is a project supported under the program Investissements d'Avenir launched by the French government and implemented by ANR with the reference ANR-11-INBS-0001.
References
Anonymous (2013). INSPIRE Metadata Implementing Rules: Technical Guidelines based on EN ISO 19115 and EN ISO 19119, V 1.3. European Commission Joint Research Centre.
Baker, K. S., Benson, B. J., Henshaw, D. L., Blodgett, D., Porter, J. H., and Stafford, S. G. (2000). Evolution of a multisite network information system: the LTER information management paradigm. BioScience 50, 963–978. doi: 10.1641/0006-3568(2000)050[0963:EOAMNI]2.0.CO;2
Balmford, A., and Bond, W. (2005). Trends in the state of nature and their implications for human well-being. Ecol. Lett. 8, 1218–1234. doi: 10.1111/j.1461-0248.2005.00814.x
Begon, M., Harper, J. L., and Townsend, C. R (1996). Ecology. Individuals, Populations and Community. New York, NY: Blackwell Science.
Benton, T. G., Solan, M., Travis, J. M., and Sait, S. M (2007). Microcosm experiments can inform global ecological problems. Trends Ecol. Evol. 22, 516–521. doi: 10.1016/j.tree.2007.08.003
Bestion, E., Clobert, J., and Cote, J. (2015b). Intraspecific variation of dispersal response to climate change. Ecol. Lett. 18, 1226–1233. doi: 10.1111/ele.12502
Bestion, E., Teyssier, A., Richard, M., Clobert, J., and Cote, J. (2015a). Live fast, die young: experimental evidence of population extinction risk due to climate change. PLoS Biol. 13:e1002281 doi: 10.1371/journal.pbio.1002281
Blanchfield, P. J., Paterson, M. J., Shearer, J. A., and Schindler, D. W. (2009). Johnson and Vallentyne's legacy: 40 years of aquatic research at the Experimental Lakes Area. Can. J. Fish. Aquat. Sci. 66, 1831–1836. doi: 10.1139/F09-148
Brudvig, L. A., Damschen, E. I., Haddad, N. M., Levey, D. J., and Tewksbury, J. J. (2015). The influence of habitat fragmentation on multiple plant-animal interactions and plant reproduction. Ecology 96, 2669–2678. doi: 10.1890/14-2275.1
Cardinale, B. J., Duffy, J. E., Gonzalez, A., Hooper, D. U., Perrings, C., Venail, P., et al. (2012). Biodiversity loss and its impact on humanity. Nature 486, 59–67. doi: 10.1038/nature11148
Carpenter, S. R. (1996). Microcosm experiments have limited relevance for community and ecosystem ecology. Ecology 77, 677–680. doi: 10.2307/2265490
Caswell, H. (1988). Theory and models in ecology: a different perspective. Ecol. Model. 43, 33–44. doi: 10.1016/0304-3800(88)90071-3
Chabbi, A., and Loescher, H. W. (2017a). Terrestrial Ecosystem Research Infrastructures: Challenges and Opportunities. London: CRC Press; Taylor & Francis group.
Chabbi, A., Loescher, H. W., and Tye, M. R. (2017b). “Integrated experimental research infrastructures: a paradigm shift to face an uncertain world and innovate for social benefit,” in Ecosystem Research Infrastructures: The Need to Address Global Change and Associated Challenges, eds A. Chabbi, and H. W. Loescher (London: CRC Press; Taylor & Francis group), 3–26.
Ciais, P., Dolman, A. J., Bombelli, A., Duren, R., Peregon, A., Rayner, P. J., et al. (2014). Current systematic carbon-cycle observations and the need for implementing a policy-relevant carbon observing system. Biogeosciences 11, 3547–3602. doi: 10.5194/bg-11-3547-2014
Clobert, J., Baguette, M., Benton, T. G., and Bullock, J. M. (2012). Dispersal Ecology and Evolution. Oxford: Oxford University Press.
Clutton-Brock, T., and Sheldon, B. C. (2010). Individuals and populations: the role of long-term, individual-based studies of animals in ecology and evolution. Trends Ecol. Evol. 25, 562–573. doi: 10.1016/j.tree.2010.08.002
De Boeck, H. J., Vicca, S., Roy, J., Nijs, I., Milcu, A., Kreyling, J., et al. (2015). Global changes experiments: challenges and opportunities. Bioscience 65, 922–931. doi: 10.1093/biosci/biv099
Drake, J. M., and Kramer, A. M. (2012). Mechanistic analogy: how microcosms explain nature. Theor. Ecol. 5, 433–444. doi: 10.1007/s12080-011-0134-0
Ehrlich, P. R., and Harte, J. (2015). Opinion: to feed the world in 2050 will require a global revolution. Proc. Nat. Acad. Sci. U.S.A. 112, 14743–14744. doi: 10.1073/pnas.1519841112
Fegraus, E. F, Andelman, S., Matthew, B. J., and Schildhauer, M. (2005). Maximizing the value of ecological Data with Structured Metadata: An Introduction to Ecological Metadata Language (EML) and Principles for Metadata Creation. Bull. Ecol. Soc. Am. 86, 158–168. doi: 10.1890/0012-9623(2005)86[158:MTVOED]2.0.CO;2
Gonzalez, A., Ronce, O., Ferrire, R., and Hochberg, M. E. (2013). Evolutionary rescue: an emerging focus at the intersection between ecology and evolution. Philos. Trans. R. Soc. B 368:20120404. doi: 10.1098/rstb.2012.0404
Haddad, N. M. (2012). Connecting ecology and conservation through experiment. Nat. Methods 9, 794–795. doi: 10.1038/nmeth.2107
Haddad, N. M., Holt, R. D., Fletcher, R. J., Jr, Loreau, M., and Clobert, J. (2017). Connecting models, data, and concepts to understand fragmentation's ecosystem-wide effects. Ecography, 40, 1–8. doi: 10.1111/ecog.02974
Hooper, D. U., Chapin, F. S., Ewel, J. J., Hector, A., Inchausti, P., Lavorel, S., et al. (2005). Effects of biodiversity on ecosystem functioning: a consensus of current knowledge. Ecol. Monogr. 75, 3–35. doi: 10.1890/04-0922
Howden, S. M., Soussana, J.-F., Tubiello, F. N., Chhetri, N., Dunlop, M., and Meinke, H. (2007). Adapting agriculture to climate change. Proc. Nat. Acad. Sci. U.S.A. 104, 19691–19696. doi: 10.1073/pnas.0701890104
Jacob, S., Bestion, E., Legrand, D., Clobert, J., and Cote, J. (2015). Habitat matching and spatial heterogeneity of phenotypes: implications for metapopulation and metacommunity functioning. Evol. Ecol. 29, 851–871. doi: 10.1007/s10682-015-9776-5
Jonquet, C., Toulet, A., Arnaud, E., Aubin, S., Dzalé-Yeumo, E., Emonet, V., et al. (2016). “Reusing the NCBO BioPortal technology for agronomy to build AgroPortal,” in 7th International Conference on Biomedical Ontologies, ICBO'16, Demo Session (Corvallis, OR).
Kampe, T. U., Johnson, B. R., Kuester, M., and Keller, M. (2010). NEON: the first continental-scale ecological observatory with airbone remote sensing of vegetation canopy biochemistry and structure. J. Appl. Remote Sens. 4:0433510. doi: 10.1117/1.3361375
Kao, R. H., Gibson, C. M., Gallery, R. E., Meier, C. L., Barnett, D. T., Docherty, K. M., et al. (2012). NEON terrestrial field observations: designing continental-scale, standardized sampling. Ecosphere 3, 1–17. doi: 10.1890/ES12-00196.1
Lawton, J. H. (1996). The Ecotron facility at Silwood Park: the value of “big bottle” experiments. Ecology 77, 665–669. doi: 10.2307/2265488
Legrand, D., Guillaume, O., Baguette, M., Cote, J., Trochet, A., Calvez, O., et al. (2012). The Metatron: an experimental system to study dispersal and metaecosystems for terrestrial organisms. Nat. Methods 9, 828–830. doi: 10.1038/nmeth.2104
Legrand, D., Cote, J., Fronhofer, E. A., Holt, R. D., Ronce, O., Schtickzelle, N., et al. (2017). Eco-evolutionary dynamics in fragmented landscapes. Ecography 40, 9–25. doi: 10.1111/ecog.02537
Leuzinger, S., Luo, Y., Beir, C., Dieleman, W., Vicca, S., and Körner, C. (2011). Do lobal change experiments overestimate impacts on terrestrial ecosystems? Trends Ecol. Evol. 26, 236–241. doi: 10.1016/j.tree.2011.02.011
Lindenmayer, D. B., Likens, G. E., Andersen, A., Bowman, D., Bull, C. M., Burns, E., et al. (2012). Value of long-term ecological studies. Austral Ecol. 37, 745–757. doi: 10.1111/j.1442-9993.2011.02351.x
Loreau, M. (2010). From Populations to Ecosystems: Theoretical Foundations for a New Ecological Synthesis. Princeton, NJ: Princeton University Press.
Madin, J., Bowers, S., Schildhauer, M., Krivov, S., Pennington, D., and Villa, F. (2007). An ontology for describing and synthesizing ecological observation data. Ecol. Informat. 2, 279–296. doi: 10.1016/j.ecoinf.2007.05.004
Magill, A. H., Aber, J. D., Currie, W. S., Nadelhoffer, K. J., Martin, M. E., McDowell, W. H., et al. (2004). Ecosystem response to 15 years of chronic nitrogen additions at the Harvard Forest LTER, Massachusetts, USA. Forest Ecol. Mgmt 196, 7–28. doi: 10.1016/j.foreco.2004.03.033
Magnuson, J. J., Robertson, D. M., Benson, B. J., Wynne, R. H., Livingstone, D. M., Arai, T., et al. (2000). Historical trends in lake and river ice cover in the Northern Hemisphere. Science 289, 1743–1746. doi: 10.1126/science.289.5485.1743
Magnuson, J. T. (1990). Long-term ecological research and the invisible present. BioScience 40, 495–501. doi: 10.2307/1311317
Mooney, H. A., Duraiappah, A., and Larigauderie, A. (2013). Evolution of natural and social science interactions in global change research programs. Proc. Nat. Acad. Sci. U.S.A. 110, 3665–3672. doi: 10.1073/pnas.1107484110
Mougin, C., Azam, D., Caquet, T., Cheviron, N., Dequiedt, S., Le Galliard, J.-F., et al. (2015). A coordinated set of ecosystem research platforms open to international research in ecotoxicology, AnaEE France. Environ. Sci. Pollut. Res. 22, 16215–16228. doi: 10.1007/s11356-015-5233-9
Olesen, J. E., Trnka, M., Kersebaum, K. C., Skjelvåg, A. O., Seguin, B., Peltonen-Sainio, P., et al. (2011). Impacts and adaptation of European crop production systems to climate change. Eur. J. Agron. 34, 96–112. doi: 10.1016/j.eja.2010.11.003
Osmond, B., Ananyev, G., Berry, J., Langdon, C., Kolber, Z., Lin, G., et al. (2004). Changing the way we think about global change research: scaling up in experimental ecosystem science. Global Change Biol. 10, 393–407. doi: 10.1111/j.1529-8817.2003.00747.x
Pangle, L. A., DeLong, S. B., Abramson, N., Adams, J., Barron-Gafford, G. A., Breshears, D. D., et al. (2015). The landscape evolution observatory: a large-scale controllable infrastructure to study coupled earth-surface processes. Geomorphology 244, 190–203. doi: 10.1016/j.geomorph.2015.01.020
Park, T. (1962). Beetles, competition, and populations: an intricate ecological phenomenon is brought into the laboratory and studied as an experimental model. Science 138, 1369–1375. doi: 10.1126/science.138.3548.1369
Pereira, H. M., Leadley, P. W., Proença, V., Alkemade, R., Scharlemann, J. P., Fernandez-Manjarrés, J. F., et al. (2010). Scenarios for global biodiversity in the 21st century. Science 330, 1503–1509. doi: 10.1126/science.1196624
Peters, D. P. C., Loescher, H. W., SanClements, M. D., and Havstad, K. M. (2014). Taking the pulse of a continent: expanding site-based research infrastructure for regional- to continental-scale ecology. Ecosphere 5, 1–23. doi: 10.1890/ES13-00295.1
Petersen, J. E., Cornwell, J. C., and Kemp, W. (1999). Implicit scaling in the experimental aquatic ecosystems. Oikos 85, 3–18. doi: 10.2307/3546786
Peterson, G. D. (2000). Scaling ecological dynamics: self-organization, hierarchical structure, and ecological resilience. Clim. Change 44, 291–309. doi: 10.1023/A:1005502718799
Pinto, R., de Jonge, V. N., Marques, P. W., and Joao, C. (2014). Linking biodiversity indicators, ecosystem functioning, provision of services and human well-being in estuarine systems: application of a conceptual framework. Ecol. Indic. 36, 644–655. doi: 10.1016/j.ecolind.2013.09.015
Post, D. M., and Palkovacs, E. P. (2009). Eco-evolutionary feedbacks in community and ecosystem ecology: interactions between the ecological theatre and the evolutionary play. Philos. Trans. R. Soc. B Biol. Sci. 364, 1629–1640. doi: 10.1098/rstb.2009.0012
Reiss, J., Bridle, J. R., Montoya, J. M., and Woodward, G. (2009). Emerging horizons in biodiversity and ecosystem functioning research. Trends Ecol. Evol. 24, 505–514. doi: 10.1016/j.tree.2009.03.018
Robertson, G. P., Collins, S. L., Foster, D. R., Brokaw, N., Ducklow, H. W., Gragson, T. L., et al. (2012). Long-term ecological research in a human-dominated world. Bioscience 62, 342–353. doi: 10.1525/bio.2012.62.4.6
Roscher, C., Temperton, V. M., Scherer-Lorenzen, M., Schmitz, M., Schumacher, J., Schmid, B. Schulze, et al. (2005). Overyielding in experimental grassland communities – irrespective of species pool or spatial scale. Ecol. Lett. 8, 419–429. doi: 10.1111/j.1461-0248.2005.00736.x
Roy, J., Picon-Cochard, C., Augusti, A., Benot, M.-L., Thiery, L., Darsonville, O., et al. (2016). Elevated CO2 maintains grassland net carbon uptake under a future heat and drought extreme. Proc. Natl. Acad. Sci. U.S.A. 113, 6224–6229. doi: 10.1073/pnas.1524527113
Scheffer, M., and Carpenter, S. R. (2003). Catastrophic regime shifts in ecosystems: linking theory to observation. Trends Ecol. Evol. 18, 648–656. doi: 10.1016/j.tree.2003.09.002
Schildhauer, M., Jones, M. B., Bowers, S., Madin, J., Krivov, S., Pennington, D., et al. (2016). OBOE: the Extensible Observation Ontology, Version 1.1. KNB Data Repository.
Schindler, D. W. (1998). Replication versus realism: the need for ecosystem-scale experiments. Ecosystems 1, 323–334. doi: 10.1007/s100219900026
Schlichting, C. D., and Pigliucci, M. (1998). Phenotypic Evolution: A Reaction Norm Perspective. Sunderland: Sinauer Associates Incorporation.
Schmid, B., Hector, A., Huston, M. A., Inchausti, P., Nijs, I., Leadley, P. W., et al. (2002). “The design and analysis of biodiversity experiments,” in Biodiversity and Ecosystem Functioning. Synthesis and Perspectives, eds M. Loreau, S. Naeem, and P. Inchausti (Oxford: Oxford University Press), 61–75.
Schoener, T. W. (1983). Field experiments on interspecific competition. Am. Nat. 122, 240–285. doi: 10.1086/284133
Schoener, T. W. (2011). The newest synthesis: understanding the interplay of evolutionary and ecological dynamics. Science 331, 426–429. doi: 10.1126/science.1193954
Sinervo, B., Méndez–de–la–Cruz, F., Miles, D. B., Heulin, B., Bastiaans, E., Villagran–Santa Cruz, M. Jr., et al. (2010). Rapid erosion of lizard biodiversity at global scales: altered thermal niche and global change. Science 328, 894–899. doi: 10.1126/science.1184695
Stewart, R. I. A., Dossena, M., Bohan, D. A., Jeppesen, E., Kordas, R. L., Ledger, M. E., et al. (2013). Mesocosm experiments as a tool for ecological climate change research. Adv. Ecol. Res. 48, 71–181. doi: 10.1016/B978-0-12-417199-2.00002-1
Stokstad, E. (2011). Open-source ecology takes root across the world. Science 334, 308–309. doi: 10.1126/science.334.6054.308
Swanson, F. J., and Sparks, R. E. (1990). Long-term ecological research and the invisible place. BioScience 40, 502–508. doi: 10.2307/1311318
Taylor, L. R. (1989). “Objective and experiment in long-term research,” in Long Term Studies in Ecology, ed G. E. Likens (New York, NY: Springer Verlag). 20–70.
Thompson, P. L., Rayfiled, B., and Gonzalez, A. (2017). Loss of habitat an connectivity erodes species diversity, ecosystem functioning, and stability in metacommunity networks. Ecography 40, 98–108. doi: 10.1111/ecog.02558
Tilman, D., Isbell, F., and Cowles, J. N. (2014). Biodiversity and ecosystem functioning. Annu. Rev. Ecol. Evol. Syst. 45, 471–493. doi: 10.1146/annurev-ecolsys-120213-091917
Tilman, D., and Reich, P. B. (2012). Biodiversity impacts ecosystem productivity as much as resources, disturbances, or herbivory. Proc. Natl. Acad. Sci. 26, 10394–10397. doi: 10.1073/pnas.1208240109
Vandenkoornhuyse, P., Dufresne, A., Quaiser, A., Gouesbet, G., Binet, F., Francez, A. J., et al. (2010). Integration of molecular functions at the ecosystemic level: breakthroughs and future goals of environmental genomics and post-genomics. Ecol. Lett. 13, 776–791. doi: 10.1111/j.1461-0248.2010.01464.x
Verdier, B., Jouanneau, I., Simonnet, B., Rabin, C., Van Dooren, T. J. M., Delpierre, N., et al. (2014). Climate and atmosphere simulator for experiments on ecological systems in changing environments. Environ. Sci. Technol. 48, 8744–8753. doi: 10.1021/es405467s
Vitousek, P. M., Mooney, H. A., Lubchenco, J., and Melillo, J. M. (1997). Human domination of Earth's ecosystems. Science 277, 494–499. doi: 10.1126/science.277.5325.494
Weathers, K. C., Hanson, P. C., Arzberger, P., Brentrup, J., Brookes, J., Carey, C. C., et al. (2013). The Global Lake Ecological Observatory Network (GLEON): the evolution of grassroots network science. Limnol. Oceanogr. Bull. 22, 71–73.
Keywords: research infrastructure, experimentation, continental ecosystems, global changes, environmental sciences, open-access platforms, modeling, data management
Citation: Clobert J, Chanzy A, Le Galliard J-F, Chabbi A, Greiveldinger L, Caquet T, Loreau M, Mougin C, Pichot C, Roy J and Saint-André L (2018) How to Integrate Experimental Research Approaches in Ecological and Environmental Studies: AnaEE France as an Example. Front. Ecol. Evol. 6:43. doi: 10.3389/fevo.2018.00043
Received: 19 January 2017; Accepted: 03 April 2018;
Published: 20 April 2018.
Edited by:
Urs Feller, Universität Bern, SwitzerlandReviewed by:
Bjorn J. M. Robroek, École Polytechnique Fédérale de Lausanne, SwitzerlandPhilippe Rocca-Serra, University of Oxford, United Kingdom
Copyright © 2018 Clobert, Chanzy, Le Galliard, Chabbi, Greiveldinger, Caquet, Loreau, Mougin, Pichot, Roy and Saint-André. This is an open-access article distributed under the terms of the Creative Commons Attribution License (CC BY). The use, distribution or reproduction in other forums is permitted, provided the original author(s) and the copyright owner are credited and that the original publication in this journal is cited, in accordance with accepted academic practice. No use, distribution or reproduction is permitted which does not comply with these terms.
*Correspondence: Jean Clobert, amVhbi5jbG9iZXJ0QHNldGUuY25ycy5mcg==
†These authors have contributed equally to this work.