- 1Department of Evolutionary Ecology, Leibniz Institute for Zoo-and Wildlife Research, Berlin, Germany
- 2Berlin-Brandenburg Institute of Advanced Biodiversity Research, Berlin, Germany
- 3Department of Reproduction Management, Leibniz Institute for Zoo-and Wildlife Research, Berlin, Germany
- 4Geography Department, Humboldt-University Berlin, Berlin, Germany
In urban areas with a high level of human disturbance, wildlife has to adjust its behavior to deal with the so called “landscape of fear.” This can be studied in risk perception during movement in relation to specific habitat types, whereby individuals trade-off between foraging and disturbance. Due to its high behavioral plasticity and increasing occurrence in urban environments, wild boar (Sus scrofa) is an excellent model organism to study adjustment to urbanization. With the help of GPS tracking, space use of 11 wild boar from Berlin's metropolitan region was analyzed: we aimed at understanding how animals adjust space use to deal with the landscape of fear in urban areas compared to rural areas. We compared use vs. availability with help of generalized linear mixed models. First, we studied landscape types selected by rural vs. urban wild boar, second, we analyzed distances of wild boar locations to each of the landscape types. Finally, we mapped the resulting habitat selection probability to predict hotspots of human-wildlife conflicts. A higher tolerance to disturbance in urban wild boar was shown by a one third shorter flight distance and by an increased re-use of areas close to the trap. Urban wild boar had a strong preference for natural landscapes such as swamp areas, green areas and deciduous forests, and areas with high primary productivity, as indicated by high NDVI (normalized difference vegetation index) values. The areas selected by urban wild boar were often located closely to roads and houses. The spatial distribution maps show that a large area of Berlin would be suitable for urban wild boar but not their rural conspecifics, with the most likely reason being a different perception of anthropogenic disturbance. Wild boar therefore showed considerable behavioral plasticity suitable to adjust to human-dominated environments in a potentially evolutionarily adaptive manner.
Introduction
The landscape of fear describes an animal's trade-off between access to food and predator avoidance on a spatial scale (Brown et al., 1999; Laundre et al., 2010, 2014). The concept includes that the landscape of fear represents relative levels of predation risk as peaks and valleys that reflect the level of fear in different parts of its area of use (Laundre et al., 2010). Disturbance of wildlife by people is particularly frequent in urban environments and can exceed disturbance by natural predators. It therefore has the potential to shape prey behavior and should incite avoidance of such environments (Frid and Dill, 2002; Ciuti et al., 2012; Rosner et al., 2014; Stoen et al., 2015). The number of mammals living in urban environments increases (Bateman and Fleming, 2012; Magle et al., 2012). Hence, urban environments can support wildlife and provide various food sources: natural food (Stillfried et al., 2017b) or anthropogenic, easily accessible food (Cahill et al., 2012; Murray et al., 2015; Theimer et al., 2015; Tryjanowski et al., 2015), both of which can contain a high amount of energy (Ottoni et al., 2009; Maibeche et al., 2015). The urban landscape of fear should be worse than the rural one because the threat increases with human proximity per se, a high traffic volume and additional predators such as domestic dogs and other companion animals (Frid and Dill, 2002; Baker et al., 2005; Hughes and Macdonald, 2013). In order to survive in urban landscapes with increased danger and disturbance, urban wildlife must become tolerant to harmless disturbance, hence urban wildlife adjusts behavior to secure sufficient shelter, breeding sites, and food (McKinney, 2002; Lowry et al., 2013). Urban wildlife needs to perceive spatio-temporal variation in risk (Valeix et al., 2012). The urban landscape of fear should correspond to landscape features such as roads, because of vehicle, and pedestrian traffic (Dowding et al., 2010; Bonnot et al., 2013; Lowry et al., 2013; Morelle et al., 2013; Murray and St Clair, 2015; Thurfjell et al., 2015; Gray et al., 2016), sealed built-up areas (= areas with a high density of housing; Bonnot et al., 2013; Magle et al., 2014; Beninde et al., 2015; Gray et al., 2016) and open areas with high human activity. Resource hotspots for food and shelter may often be found in more natural habitats as forests, parks, water bodies or swamp areas close to water (Beninde et al., 2015; Morelle et al., 2015) and in areas with a high vegetation density (Normalized Difference Vegetation Index, NDVI) (Bino et al., 2008). In order to identify resource selection of urban wildlife and their ability to perceive and respond to spatial variation in risk, the actually used landscape types within its specific habitat component is the appropriate scale for studying potential behavioral adjustments. For instance, it was demonstrated that urban bobcats and coyotes coped with human-related habitat resources (Tigas et al., 2002), while urban cougars (puma concolor) preferred natural patches (Kertson et al., 2011). Hence, behavioral adjustment in terms of resource selection to exploit and survive in novel habitats seems very likely, but remains poorly understood to date.
Medium-sized urban mammals such as foxes, badgers, or coyotes are increasingly studied (Gloor et al., 2001; Grinder and Krausman, 2001; Davison et al., 2008; Gehrt et al., 2009) but studies on large urban mammals are rare. Wild boar (Sus scrofa), a social mammal with a growing population throughout Europe (Massei et al., 2015; Keuling et al., 2016), is increasingly present in urban areas (Dinter, 1991; Cahill et al., 2003, 2012; Jansen et al., 2007; Podgorski et al., 2013). Wild boar frequently interfere with people, as they annoy garden owners (e.g., by rooting), can destroy agricultural crops (Amici et al., 2012; Ficetola et al., 2014; Jarolimek et al., 2014; Laznik and Trdan, 2014) and are potential transmitters of zoonotic diseases or diseases relevant to livestock (Fernández et al., 2006; Jansen et al., 2007; Chiari et al., 2015). Therefore, they are a useful model to study the ability of urban wildlife to perceive and respond to spatial variation in the landscape of fear. Moreover, studying wild boar assists local authorities to develop appropriate management tools to minimize human-wildlife conflicts. Despite numerous studies on wild boar demonstrated substantial variation in home range size and kinetics of movements in natural and agricultural landscapes (Dardaillon and Beugnon, 1987; Cousse et al., 1992; Janeau et al., 1995; Thurfjell et al., 2013; Jarolimek et al., 2014; Morelle et al., 2014, 2015), studies of urban wild boar are rare (Cahill et al., 2003, 2012; Podgorski et al., 2013; Stillfried et al., 2017a,b).
We conducted the first GPS data-based study of wild boar in urban and rural areas (metropolitan area Berlin, Germany). Our focus was the variation of space use within an urban and a rural landscape of fear. We hypothesized that urban wild boar adjusted their perception of risk, i.e., modulated their landscape of fear, in order to find sufficient food and shelter in a novel environment. Further, we hypothesized that the potential rewards in urban environment are higher for urban than for rural wild boar. Therefore, the modulation of the landscape of fear is stronger than the modulation of the natural landscape. To test this, we first analyzed flight initiation distance and response to trapping and predicted that urban wild boar have a higher tolerance toward human presence, indicated by a lower flight distance and a re-use of trapping locations (Prediction 1). Our next step was to design and test a set of candidate models to identify the most suitable habitat suitability model to predict the wild boar's presence due to its perception of the landscape of fear. The movement choices of wild boar were represented by use (recorded GPS locations) vs. availability (correlated random walks, CRWs) across 11 habitat types to fit binomial generalized linear mixed models. First we studied the selected habitat type and predicted that urban wild boar select natural landscape types in the city over urban landscape types (Prediction 2). Then we analyzed the distances of wild boar locations to each habitat type and predicted that urban wild boar are closer and more tolerant to human related landscape structures (Prediction 3). Finally, we mapped predicted probability of occurrence for rural and urban wild boar and predicted that a larger area within Berlin is suitable for urban than for rural wild boar (Prediction 4).
Materials and Methods
Study Area
The study was carried out in metropolitan area Berlin and the surrounding rural areas of the Federal state of Brandenburg, Germany (52°31′N, 13°24′E, Supplementary Figure 1). Twenty percent of Berlin is covered with forests. The biggest forest “Grunewald” is a mixed forest visited by ~1 million people annually BDF1 (Franusch, 2015). Although more than 1,000 wild boar are hunted every year in the Grunewald alone (Stillfried et al., 2017a), the number of wild boar remains high. Wild boar can be observed in most of Berlin's forests and adjacent built-up areas (personal observation MS). The forest areas are bordered by rural coniferous forests and agricultural areas (Supplementary Figure 1). All study areas are comparable in terms of providing similar natural and urban landscape structures accessible for wild boar. Despite wild boar are common in Berlin's forests since World War II; the urban wild boar, which invades built-up areas, is more recent (Dinter, 1991; Hespeler, 2007). To reduce human-wildlife conflicts hunting within built-up areas of Berlin was established in 2000 (Hespeler, 2007).
Animal Capture, Handling, and Data Collection
Wild boar were caught in collaboration with Berlin's and Brandenburg's foresters between 2013 and 2015 using two by two meter iron wild boar traps developed by Hinrich Zoller (University of Rostock, Germany). An overview about captured animals and duration can be found in Supplementary Figure 2). We hereby confirm that animal handling permits were approved by the respective animal welfare licensing committee of Berlin (“Landesamt für Gesundheit und Soziales”/LaGeSo: permit number: Reg 0383/12) and Brandenburg (“Landesamt für Umwelt, Gesundheit und Verbraucherschutz”: permit number: V3-2347-40-2012). All used methods were in accordance with these permits and the ASAB/ABS Guidelines for the Use of Animals in Research. A detailed description of animal capture and handling can be found in the Supplementary Information (Appendix 1).
Habitat Classification and Explanatory Variables
To derive landscape variables representing the metropolitan area of Berlin we used governmental, open-access land use maps from Berlin and the surrounding region of Brandenburg. The Berlin map is named “Umweltatlas Berlin/Stadtstruktur—Flächentypen differenziert 2010 (Umweltatlas)” and was downloaded from www.fisbroker.de. The Brandenburg map is named “Kartierung von Biotopen, gesetzlich geschützten Biotopen (§ 30 BNatSchG und § 18 BbgNatSchAG) und FFH-Lebensraumtypen im Land Brandenburg,” and the source is http://www.mlul.brandenburg.de/lua/gis/biotope_lrt.zip. Both maps contained 1,351 unique land use categories. We reclassified land use into 10 wild boar specific habitat classes to investigate space use of wild boar: deciduous and coniferous forest, green areas, agriculture, water shorelines and swamp, landscapes representing the urban landscape of fear industrial, houses with gardens, houses, roads and railways (see Map Supplementary Figure 1). Additionally we used the freely available normalized difference vegetation index maps (NDVI, Landsat, http://earthexplorer.usgs.gov/, downloaded on April 27th 2017). We used maps corresponding to the vegetation period (German Meteorological Service, www.dwd.de/EN) during our study years: Summer 2013 map date: August 2013, winter 2014 map date: February 2014, summer 2014 map date: July 2017, winter 2015 map date: March 2017. Thus, we could standardize the used NDVI across years, e.g., for summer recordings 3.5 month after the start of the vegetation period (see Supplementary Figure 2).
Data Preparation and Analysis
We tracked 13 GPS-collared wild boar, nine females and four males, between 2013 and 2015 (Supplementary Figure 2). Two females lost their collars after a few days and had to be excluded, resulting in data from 11 wild boar. The mean observation time was 6.0 ± 2 months as four of animals were prematurely shot by hunters and two were killed by cars. For the surviving animals the collars were removed using a drop-off transmitter function after 6–8 months according to our animal handling permits. In total we collected 78,293 GPS locations. Urban wild boar were those caught in Berlin and having the majority of GPS locations (>50%) within Berlin's administrative boundary (6 individuals), all other animals were classified as rural (5 individuals, Supplementary Figure 1).
The individual flight distance from people (Prediction 1) was recorded during field observations. Each animal was approached for a minimum of five times. The distance between observer and fleeing animal was estimated in 5 m steps. For wild boar which could not be visually observed, we estimated the flight distance to be a minimum of 100 m, using the strength of the VHF signal of the GPS collar as an indicator that the animal moved away when we came closer to resting sites. Actual distances might have well exceeded 100 m. To test the difference between urban and rural wild boar a Wilcoxon-Mann-Whitney was used. Mean flight distances and corresponding 95 % confidence interval, if available, were calculated with the exact and minimum (censored) estimates for urban and rural wild boar using a Kaplan–Meier survival analysis in Systat version 13 (Systat Software Inc., Richmond, VA, USA).
The tolerance to trapping (Prediction 1) was estimated for each individual using 150 and 1,000 m buffers around the trapping locations using the gBuffer function in the R-package rgeos (Bivand and Rundel, 2013). The percentage of points within one of these buffers was then calculated for the first month after trapping and for the time thereafter. Differences between urban and rural wild boar for each buffer size and both time intervals were analyzed (ANOVA) and visualized in R 3.3.3 (R Core Team, 2017).
To test for habitat preferences, we compared individual use of landscape types with the totally available landscape types reachable for an individual (Manly et al., 2002; Boyce, 2006; Johnson et al., 2006). Availability was derived by individually simulating CRWs. This established method uses the step length and turning angle from the original animal tracks and then simulate random trajectories within the study area based on these metrics (Kareiva and Shigesada, 1983; Bovet and Benhamou, 1988; Codling et al., 2008). We simulated five CRWs per individual and month within a buffer around the original tracks (sized was oriented at mean daily travel distance), using the adehabitatHR 0.4.14 R-package (Calenge, 2006). Large water bodies were eliminated from the buffer to ensure that the simulated locations were not set within lakes, since lakes were bordering the used areas of some individuals. The locations of the CRWs were then added to the wild boar data, resulting in a dataset with 469,758 locations. For each location we extracted the associated habitat class (10 categories described above) using the “over” function of the sp package (Pebesma and Bivand, 2005) in R.3.3.3. Second, we calculated the shortest distances to each landscape type, using the rgeos::gDistance function (Bivand et al., 2017). NDVI values for each location were extracted from a raster map with 30 m pixel size.
We analyzed the data using binomial generalized linear mixed model (GLMM) with observed wild boar tracks (1) or CRW (0) as binary response variable. The first model (Prediction 2) was specified using origin of wild boar (factor levels: rural or urban) in interaction with habitat class predictor (factor levels: 10 landscape types) as explanatory variables. The wild boar ID was included as random effect and a variable containing the wild boar ID and the month as nested random effect, because CRWs were created separately for each month. We chose a set of three candidate models (Supplementary Table 1A) with the full model containing all variables, a second model including only the landscape types without origin and an intercept only model. The candidate GLMMs were compared and ranked using Akaike's information criterion (AIC) and model weight. The best model was further evaluated by calculating the area under the curve (AUC; Rosset, 2004) using the dismo package (Hijmans et al., 2015) in R. Results of the best model were plotted using the “effects” package (Fox et al., 2014). Additionally, we plotted the percentage of locations within a habitat class for urban and rural wild boar and corresponding CRWs as mosaic plot.
In a second set of GLMMs, we used the same binary response and random factors as described above. Fixed effects were distances to each habitat class and the NDVI in interaction with origin (Prediction 3). The NDVI was in addition added as interaction term with season (vegetation = 1 vs. non-vegetation period = 0, Supplementary Figure 2). We assessed predictor correlations (Supplementary Figure 3) using Pearsons's product-moment correlation r and excluded correlating variables (|r| > 0.7). A correlation was found between agriculture and coniferous, agriculture and swamp and industrial. Therefore Coniferous and deciduous forests were merged, as well as water and swamp, while industrial was excluded, so that only 8 distances variables (plus NDVI) were used instead of 10.
Non-linearity was examined by plotting partial residual plots (Klar et al., 2008) of binomial generalized additive models (GAMs; Supplementary Figure 4; Hastie and Tibshirani, 1987; Austin, 2002; Wood, 2004). The model configuration was identic to the GLMMs, but we fitted smoothing splines with 3 knots for all dependent habitat variables and the NDVI (R-package mgcv 1.8-15). If variables were non-linear we included the quadratic term of the variables in the final models, which was the case for agriculture, green area, streets, houses and houses with garden. All explanatory habitat variables were centered and scaled using the function “scale” in R 3.3.3. We chose a set of four candidate models (Supplementary Table 1B) including: a full model including all distance variables and the NDVI; a landscape of fear model, including human related landscape variables (houses, house with garden, trails, street) and origin; a natural model, including non-urban variables (deciduous, green area, agriculture, water, NDVI); and an intercept only model. As described above, candidate models were compared and ranked using AICc and model weights (Wagenmakers and Farrell, 2004) and the AUC was calculated for the best model. Again, we plotted the effects for all variables of the best model, using the “effects” package. Final models for habitat class and distances were used to predict the presence of wild boar throughout our study region (Prediction 4). Wild boar presence was predicted using the “raster::predict” (Hijmans and Van Etten, 2014). The resulting maps were visualized using the viridis coloring scheme (Garnier, 2017). Probabilities for urban and rural wild boar were predicted separately and based on a raster stack with 100 m resolution. For the habitat class model the raster cell value coded the habitat class with the highest share. For the distance model, a raster stack (100 m resolution) containing one layer for each habitat class and the NDVI was used. Each cell value indicated the distance to the layer specific landscape type, except the NDVI layer which showed the NDVI values. The prediction (using the distance model) map for urban wild boar was overlapped with a map of Berlin which shows the amount of sealed area (Berlin, 2011) to visualize hotspots within the urban area. In addition, forest patches with a known wild boar occurrence were shaded in green. Conflict wild boar which were hunted by special city hunters (Stillfried et al., 2017a) were also plotted to compare the predicted suitability with true presence (using an independent dataset).
Results
Flight Distance and Reaction to Trapping (Prediction 1)
The flight distance of urban wild boar was significantly shorter than for rural wild boar (Wilcoxon-Mann-Whitney U-test, U = 1, exact p = 0.0065, n = 11). The mean flight distance of urban wild boar was 31.0 m (95% CI 16.4 m−45.5, n = 5, Kaplan–Meier survivorship analysis), whereas mean flight distances of rural wild boar was, at 92.5 m, almost three times higher (95% CI could not be computed because of censored data, n = 6). Urban wild boar used areas close to traps significantly more often than rural wild boar (Figure 1), especially within the first month after trapping: 7 ± 6% of locations of urban wild boar and 1 ± 5% of rural wild boar locations were within a 150 m buffer. 85 ± 5% of locations of urban wild boar were within a 1,000 m buffer and only 35 ± 10% of locations of rural wild boar (Figure 1).
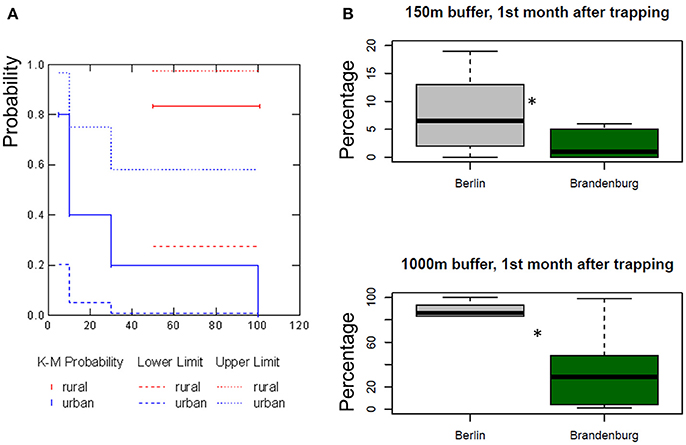
Figure 1. Survival plot for flight distance and reaction to trapping of wild boar in urban and rural areas in Berlin and Brandenburg between 2013 and 2015. (A) Probability for fleeing at a specific distance for urban wild boar (n = 6) is displayed in blue, upper and lower limits are displayed with a dashed line. The probability for flight distances for wild boar in rural areas (n = 5) are displayed in red, upper and lower probability limits are again displayed with dashed lines. The plot is the result of a Kaplan–Meier survival analysis. (B) Reaction to trapping was measured by the percentage of locations within a 150 m buffer and 1,000 m buffer around the trapping location within the first month after trapping. The boxplots show the median as well as 50% quantiles of analysis of the variances (ANOVA, 150 m: p = 0.0368, Df = 17; 1,000 m: p = 0.0128, Df = 17). The *indicates significant differences, indicated by a p-value below 0.05.
Use of Habitat Classes (Prediction 2)
The fitted models show that landscape types used by urban and rural wild boar locations differ from those of CRWs. Among the habitat class models, the full model was the best with a model weight of 1 (Table 1A; Supplementary Table 2) and an AUC of 0.71, indicating fair discriminative ability. The comparison of urban and rural wild boar shows that urban wild boar favor the landscape types deciduous forests (Δ rural−urban −0.18), house (Δ rural−urban −0.05), houses with garden (Δ rural−urban −0.49), industrial (Δ rural−urban −0.12) and green area (Δ rural−urban −0.07), while rural wild boar favor agricultural areas (Δ rural−urban 0.18), coniferous forests (Δ rural−urban 0.17) and swamps (Δ rural−urban −0.69). Streets (Δ rural−urban 0.0) and trails (Δ rural−urban 0.01) are similar.
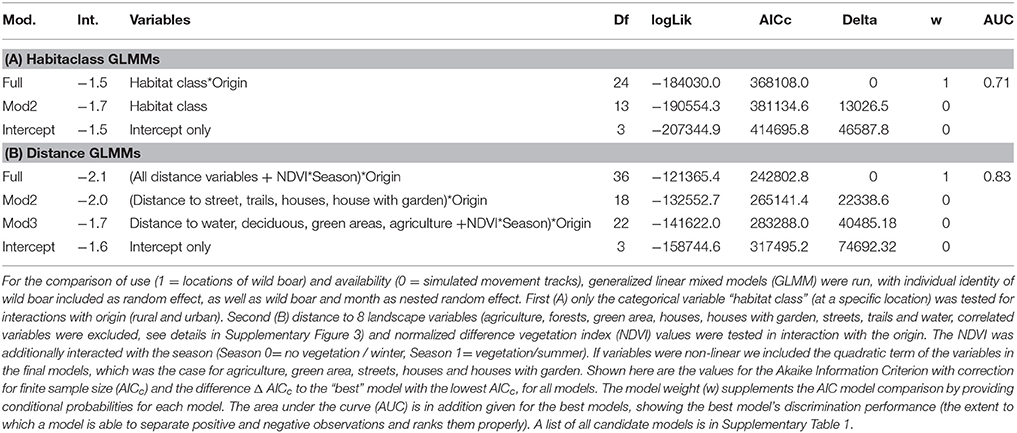
Table 1. Model selection table for the influence of landscape variables on habitat choice of wild boar in Berlin and Brandenburg between 2013 and 2015.
Overall, wild boar in both, urban and rural areas were in 60% of all records found in natural landscapes (forests and green areas) recoded, while the share of natural landscape types available for wild boar (CRWs) was only 30% (Figure 2B).
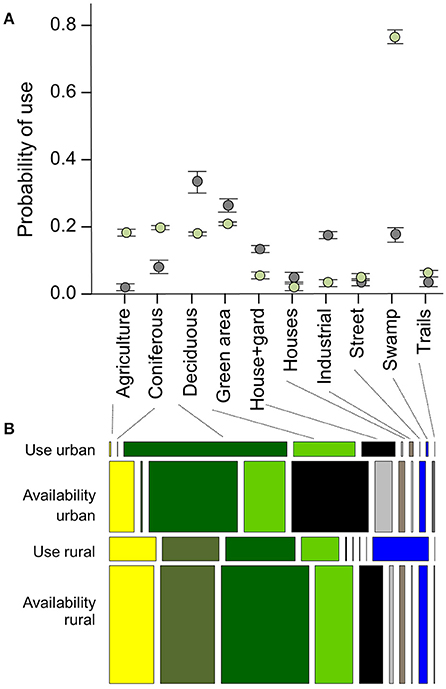
Figure 2. Use vs. availability of selected habitats in urban and rural wild boar from Berlin and Brandenburg between 2013 and 2015. (A) Effect plot of the generalized linear mixed models (GLMM; details see main text), showing the probability of use of the different habitat classes for urban (gray dots) vs. rural (green dots) wild boar. The default cutoff is 0.17 because we used five times more correlated random walks than wild boar locations. (B) Percentage of locations within a habitat category among urban and rural wild boar (original track locations = use) and correlated random walks (CRW = availability) are displayed. The width of each box shows the composition of habitat classes within one of the four groups, the height represents the total amount of locations. Since there were five times more CRW location than wild boar locations, the boxes are higher for the CRWs.
Distance to Habitat Structures (Prediction 3)
The full model showed the lowest AIC and a model weight of 1 (Table 1B; Supplementary Table 3) and an AUC of 0.83, indicating very good discrimination ability. Urban wild boar were found close to forest (0–500 m distance), houses (0–800 m) and streets (0–600 m) while rural wild boar were further away (forest: up to 2,500 m, houses: up to 2,000 m and streets: 1,000 m). Rural wild boar were closer to agricultural areas (500–2,000 m) while urban wild boar were up to 3,000 m away. Both, urban and rural wild boar were found close to green areas (0–1,400 m), houses with garden (0–2,000 m), and water (0–1,000 m), but the effect was stronger for urban wild boar. Trails were not preferred by wild boar. While urban wild boar select areas with high NDVI values all year round, rural wild boar select areas with a high NDVI especially in winter (Figure 3).
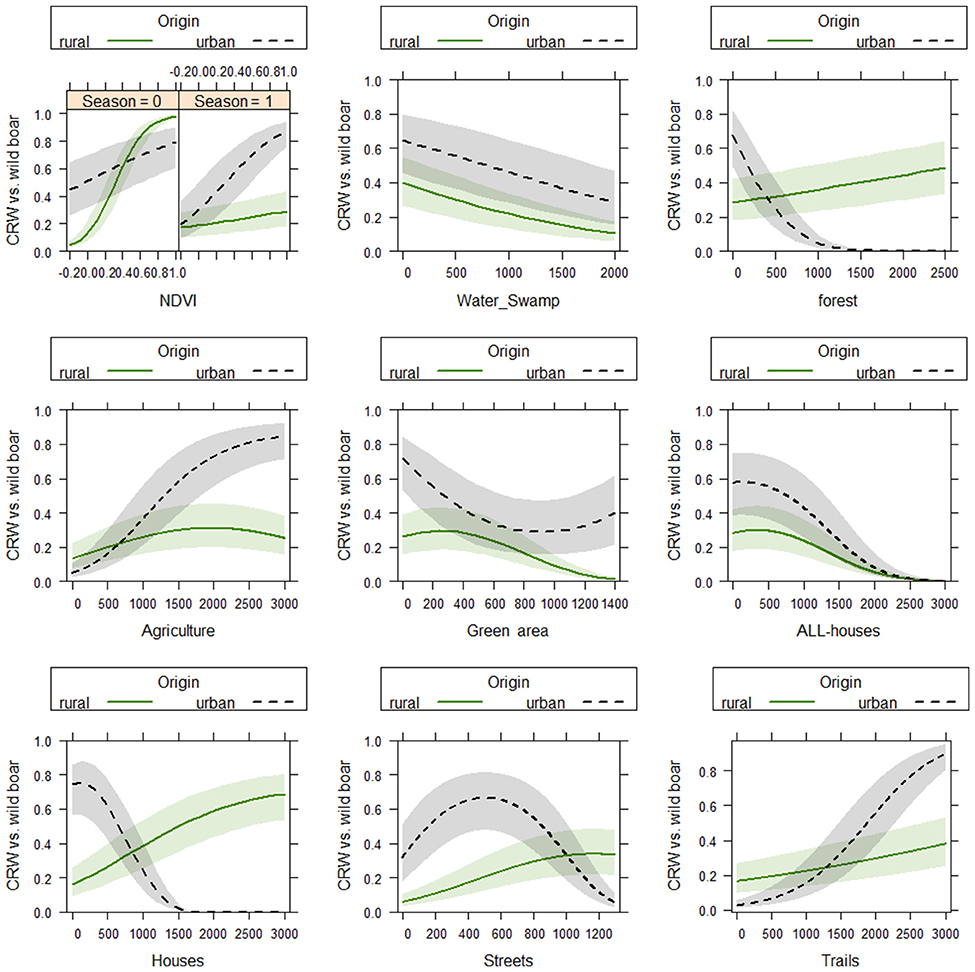
Figure 3. Effects plot of generlized mixed models, testing the impact of distance to different landscape types and NDVI on habitat use of urban vs. rural wild boar in Berlin and Brandenburg between 2013 and 2015. For the comparison of use (1 = locations of WILD BOAR) and availability (0 = simulated movement tracks), generalized linear mixed models (GLMM) were run. The distance to 8 landscape variables (agriculture, forests, green area, houses, houses with garden, streets, trails, and water) and normalized difference vegetation index (NDVI) values were tested in interaction with the origin (urban, dashed black line and rural, solid green line). The NDVI was additionally entered as interaction term with the season (Season 0 = no vegetation/winter, Season 1 = vegetation /summer). The default cutoff is 0.17 because we used five times more correlated random walks than wild boar locations.
Prediction Maps (Prediction 4)
The prediction maps for both, the habitat class and distance to habitats show strong differences between rural and urban wild boar (Figures 4A,B). Both maps indicate that a larger amount of areas within the city can be used by urban wild boar, while only a few patches, mostly close to large waterbodies and within the urban forests are suitable for rural wild boar. Moreover, urban wild boar were not restricted to the edges of nature-close habitats, but could invade the urban landscape types much further. The overlap of the prediction map and map of the large forest patches that hold genetically distinct wild boar populations (Stillfried et al., 2017a) shows that the predicted areas within Berlin are actually used by wild boar (see Figure 5).
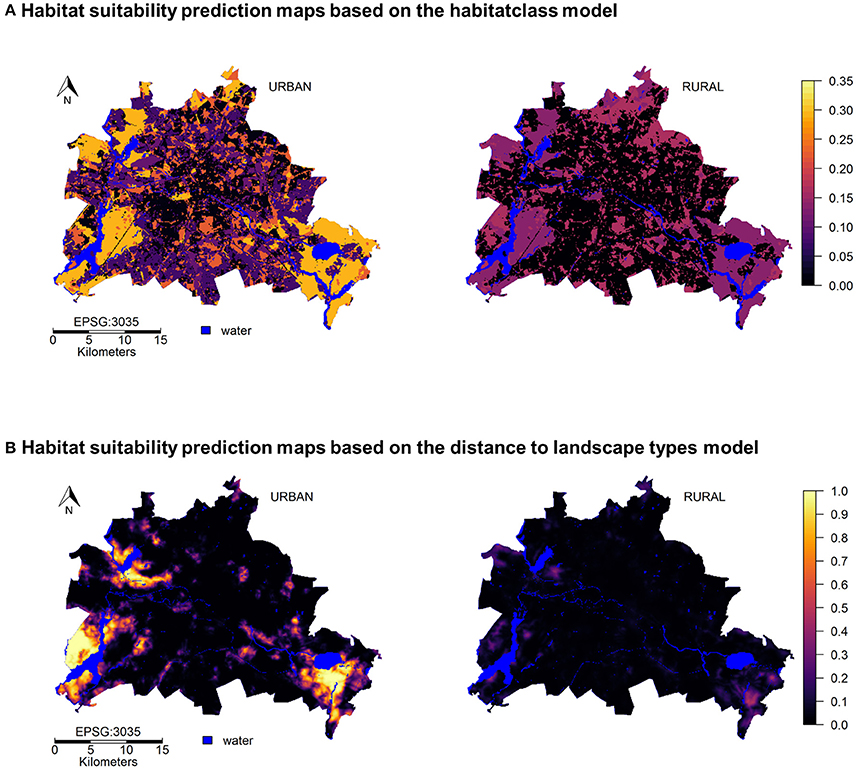
Figure 4. Prediction maps showing suitable habitats within Berlin from the perspective of urban and rural wild boar. (A) Habitat suitability from predicted from GLMMs with habitat class. (B) Habitat suitability predicted from GLMMS with distance variables and NDVI.
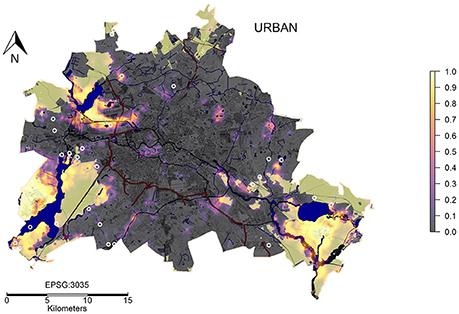
Figure 5. Prediction map and urban structure A prediction map (Figure 4B) for urban wild boar space use (transparency 50%) was overlapped with a map showing sealed surfaces. In addition, waterbodies were displayed in blue. Areas of urban forestry departments, where wild boar presence is known, was stained light green. The white circles are locations where wild boar were hunted by city hunters (for more details see Stillfried et al., 2017a) and show presence of wild boar outside the forest areas.
Discussion
Response to Trapping and Flight Distance (Prediction 1)
Our study provides scientific evidence that a large mammalian herbivore trades-off fear caused by human disturbance with the need to access food in urban areas. We approved our first prediction that urban wild boar show a higher tolerance toward human presence, indicated by a lower flight distance and a reuse of trapping locations. During the first month, over 90% of all locations of urban wild boar were within a 1,000 m distance to the trapping location, but for rural wild boar only 25% of the locations. The avoidance in rural wild boar could be a result of larger home ranges in rural areas. However, the increased presence within 150 m buffers of urban wild boar shows a difference between urban and rural wild boar. These findings can be a result of habituation to humans, which is in line with observations of urban wild boar from other cities (Licoppe et al., 2013). In our study only urban wild boar were recaptured or observed re-entering a traps, which supports the assumption that trapping locations can be used as an example for a landscape of fear (Laundre et al., 2010) where animals experienced interaction with humans. These areas were only avoided by rural wild boar because they are less tolerant to anthropogenic disturbance. The significantly lower flight distance of urban wild boar is an even stronger hint for behavioral adjustment to anthropogenic disturbance by urban wild boar.
Studies about flight distances in comparable urban mammals such as urban foxes which are also present in Berlin (Börner et al., 2009) have not been conducted yet. But Foxes or raccoons are well-known to occur in many urban areas worldwide and are well-adapted to human presence (Bateman and Fleming, 2012). Consequently, behavioral adjustment to novel environments might be common across generalist mammals, but the underlying mechanisms remain poorly understood to date.
Habitat Selection in a Landscape of Fear (Prediction 2)
Our second prediction that urban wild boar select natural landscape types could also be confirmed by our data and is in line with results from urban wild boar in Barcelona (Castillo-Contreras et al., 2018). Beninde et al. (2015) reviewed that especially green areas are important for an increased biodiversity in cities. Green areas are also strongly selected by urban wild boar which fits to observations from the study area that many private and public areas are damaged by wild boar when they are rooting (Supplementary Figure 1). Damage of grasslands by wild boar is also recorded for other areas (Bueno et al., 2009; Laznik and Trdan, 2014). In contrast to rural wild boar in our study area, urban wild boar do not use agricultural areas, even though agriculture is available in close proximity to the used habitats (Supplementary Figure 1, Figure 2). Agricultural areas usually provide attractive foraging opportunities for wild boar (Herrero et al., 2006; Amici et al., 2012; Frackowiak et al., 2013; Ficetola et al., 2014). But wild boar are known to be opportunistic omnivores whose diet, in any particular instance, is largely determined by the relative availability of different food types (Schley and Roper, 2003). Urban wild boar in Berlin use more deciduous forests which provide higher energetic food resources than coniferous forests which are the main forest type in Brandenburg (Stillfried et al., 2017b). Therefore agricultural areas function as additional food resources in Brandenburg which are not selected in Berlin due to the availability to alternative high energetic natural food items (Stillfried et al., 2017b).
Distance to Human Related Landscape Types (Prediction 3)
In the third prediction, we expected urban wild boar to tolerate close proximity to human related habitat structures which is strongly supported by our results. We demonstrated that rural wild boar avoided a crucial component of the landscape of fear (Laundre et al., 2010, 2014; Gallagher et al., 2017) as they were further away from houses, but used houses with garden similar to urban wild boar. We infer that rural wild boar occasional make use of areas close to houses with garden at the edge of villages (Lowry et al., 2013), while urban wild boar modulate the perception of harmless anthropogenic risks and invade urban areas much further. Thus, urban wild boar demonstrated that they can cope with an urban landscape of fear by an intelligent adjustment to human presence (and disturbance), similar to urban peccaries (Tayassu tajacu) foraging in housing areas (Bellantoni and Krausman, 1993). Similar patterns of spatial behavioral adjustment were observed in urban bobcats and coyotes (Tigas et al., 2002; George and Crooks, 2006). As houses in urban areas are densely build a behavioral adjustment toward tolerance of anthropogenic harmless risks was crucial for urban wild boar to successfully invade urban areas and to become urban dwellers. Another hint that urban wild boar perceive the urban landscape of fear differently than rural wild boar is shown by their use of forest. Urban forests which are intensely used by urban wild boar in Berlin are particularly famous for their substantial numbers of visitors (BDF, 20152; Franusch, 2015) which use the forest for recreational activities and therefore are part of the landscape of fear (Frid and Dill, 2002; Ciuti et al., 2012). A modulated perception of a landscape of fear enables wildlife to utilize new habitats such as highly frequented forests (recreational value) providing a high value to society and thus are frequently visited by humans. In urban natural landscapes numerous domestic dogs are walked. A study from California showed, that activity of urban bobcats and coyotes was decreased at sites with high recreational activities and presence of domestic dogs and urban deer were harder to detect in areas with high human activity (George and Crooks, 2006). Especially in our study region groups of up to 10 dogs are present frequently in the forests (Andreas Constien, forester in the Grunewald, personal communication and own observation). Due to preadaptation wild boar will recognize domestic dogs as predators (Nores et al., 2008) belonging to their urban landscape of fear. In rural environments domestic dogs are used in wild boar hunting to this date (Sodeikat and Pohlmeyer, 2003). Consequently, all domestic dogs will be perceived as persisting threat and areas with a high density of dogs represent a landscape of fear. Moreover, as people frequently unleash their dogs within urban forests far from roads (personal observation), areas close to major roads may probably be perceived safer by urban wild boar. This is another example how urban wild boar modulated the landscape of fear: Usually roads provide a landscape of fear and are avoided but in this case are safe for urban wild boar because the disturbance by cars is predictable. Urban wild boar can be found close to roads while rural wild boar avoided areas close to roads similar to other wildlife (Grinder and Krausman, 2001; Dowding et al., 2010; Poessel et al., 2014; Stillfried et al., 2015; Thurfjell et al., 2015). Hence, the cognitive ability of wild boar to assess risk related road proximity and adjust their behavior accordingly seems very likely.
Water bodies in both urban and rural forests are used by many people for recreational activities such as swimming during the summer. Observations of single individuals suggest that rural wild boar avoid these patches during the summer because of the urban landscape of fear (Frid and Dill, 2002; Ciuti et al., 2012; Rosner et al., 2014; Stoen et al., 2015). During the winter when the number of drive hunts in the rural forests is high, they select areas close to water bodies as hiding places (personal observations). In opposite, urban wild boar were located close to recreational urban beaches inside reed beds year-round, as the presented data show. Further studies accounting for such temporal effects are necessary to clarify indicated seasonal differences in wild boar behavior comprehensively.
Habitat Suitability (Prediction 4)
Prediction 4 which claims that a larger area is suitable for urban wild boar than for rural wild boar within Berlin, can also be confirmed. The maps show that urban wild boar are expected to invade the urban environment much further than rural ones. We assume this to happen due to modulation of the urban landscape of fear and a resulting trade-off between access to food and avoidance of anthropogenic disturbance. Urban wild boar therefore fit the description of urban utilizers and urban dwellers successfully living in urban areas (Fischer et al., 2015). To fit rural wild boar into this concept we have to expand the urban utilizer concept by intraspecific variability, as they have to be classified urban avoiders (McKinney, 2002; Fischer et al., 2015). The underlying processes are unkown, as we cannot disentangle genetic, population ecological, cognitive or personality (Dingemanse et al., 2010) driven responses to the environment. Modulation of a perceived urban landscape of fear seems likely. Regarding management issues, the differentiation between urban and rural wild boar provides an excellent tool to show possible travel routes. Main travel routes are through the forests or along the forest boarders and along waterways. The fact, that conflict wild boar which were hunted by city hunters occur within hotspots of the predicted maps, proofs, that the maps are useful to show present and future wild boar hotspots.
Reflection of the Methods
The analysis of habitat selection with help of CRWs allowed us to study not only those habitats which were actually used by wild boar but to show in addition the available surroundings which were not selected. This resource selection function (Boyce et al., 2002) could alternatively have been assessed with step selection function which compare original steps with random steps (Thurfjell et al., 2014) instead of CRWs. Since both methods were shown to provide similar results (Duchesne et al., 2015), we assume that our results are a good reflection of wild boar's resource selection. Thereby we were for example able to show that urban wild boar do not select agricultural areas although they are available for them. For further studies it would be interesting to investigate wild boar tracks with a higher resolution (for example 5 min intervals or less) which would enable to analyze small scaled movement within the urban environment such as road crossing behavior. Regarding the concept of the landscape of fear, our results are based on interpretations of animal behavior. To fully investigate the impact of the landscape of fear, it would be crucial to include measurements of internal factors such stress and body condition into further analyses.
Conclusion
With our study, we showed how individuals of large social mammalian omnivores such as wild boar encounter urbanization. These individuals live among humans within highly anthropogenic environments such as major capitals while their rural conspecifics avoid the very same areas. We measured how urban wild boar deal with the human presence and human associated landscape structures such as roads and houses (landscape of fear) in urban areas by analyzing habitat selection of GPS-collared wild boar. The wild boar is a suitable model to improve our knowledge about the adjustment of large mammals to urban areas in general which is of interest worldwide. At the same time our analyses help to gain an understanding of the special characteristics of wild boar in Berlin. Thereby our analyses provide important data which can be used for management, for example by showing wild boar hotspots within Berlin. Our results show how wild boar deal to trade-off between the human induced landscape of fear and the need to forage by modulating their risk perception: They tolerate human presence and use human associated habitat classes. Wild boar therefore showed considerable intraspecific behavioral plasticity suitable to adjust to human-dominated environments in a potentially evolutionarily adaptive manner.
Author Contributions
SO and SK-S: conceived the study; FG, JP, and KR: performed wild boar anesthesia; MS and KB: collected data; MW and PG: created spatial land cover maps; MS, PG, HH, and SK-S: performed the statistical analyses; MS, PG, HH, SO, and SK-S wrote the manuscript. All authors read and commented on the manuscript.
Conflict of Interest Statement
The authors declare that the research was conducted in the absence of any commercial or financial relationships that could be construed as a potential conflict of interest.
Acknowledgments
We thank Andreas Burchardt, Andreas Constien, Egbert Gleich, Erica Grant, Bodo Janitza, Uwe Peschke, Marion Schmolke, and Heiko Wessendorf for their help with trapping wild boar, the Leibniz Centre for Agricultural Landscape Research (ZALF) and Hinrich Zoller (University of Rostock) for providing traps, Anne Berger and Knut Eichhorn for the use of telemetry devices, Matthias Busch, and Ilja Heckmann for assistance with data analyses and National Geographic, the “Stiftung Naturschutz” and the Leibniz Institute for Zoo & Wildlife Research for funding. PG was funded by the German Federal Ministry of Education and Research BMBF within the Collaborative Project “Bridging in Biodiversity Science—BIBS” (funding number 01LC1501A-H). The publication of this article was funded by the open Access Fund of the Leibniz Association.
Supplementary Material
The Supplementary Material for this article can be found online at: https://www.frontiersin.org/articles/10.3389/fevo.2017.00157/full#supplementary-material
Footnotes
1. ^Available online at: https://www.berlin.de/tourismus/nachrichten/3688703-1721038-grunewald-ist-waldgebiet-des-jahres-2015 (Accessed).
2. ^Available online at: https://www.berlin.de/tourismus/nachrichten/3688703-1721038-grunewald-ist-waldgebiet-des-jahres-2015 (Accessed).
References
Amici, A., Serrani, F., Rossi, C. M., and Primi, R. (2012). Increase in crop damage caused by wild boar (Sus scrofa L.): the “refuge effect”. Agron. Sus. Develop. 32, 683–692. doi: 10.1007/s13593-011-0057-6
Austin, M. P. (2002). Spatial prediction of species distribution: an interface between ecological theory and statistical modelling. Ecol. Modell. 157, 101–118. doi: 10.1016/S0304-3800(02)00205-3
Baker, P. J., Bentley, A. J., Ansell, R. J., and Harris, S. (2005). Impact of predation by domestic cats Felis catus in an urban area. Mamm. Rev. 35, 302–312. doi: 10.1111/j.1365-2907.2005.00071.x
Bateman, P. W., and Fleming, P. A. (2012). Big city life: carnivores in urban environments. J. Zool. 287, 1–23. doi: 10.1111/j.1469-7998.2011.00887.x
Bellantoni, E. S., and Krausman, P. R. (1993). Habitat use by collared peccaries in an urban-environment. Southwest. Nat. 38, 345–351. doi: 10.2307/3671613
Beninde, J., Veith, M., and Hochkirch, A. (2015). Biodiversity in cities needs space: a meta-analysis of factors determining intra-urban biodiversity variation. Ecol. Lett. 18, 581–592. doi: 10.1111/ele.12427
Bino, G., Levin, N., Darawshi, S., Van Der Hal, N., Reich-Solomon, A., and Kark, S. (2008). Accurate prediction of bird species richness patterns in an urban environment using Landsat-derived NDVI and spectral unmixing. Int. J. Remote Sens. 29, 3675–3700. doi: 10.1080/01431160701772534
Bivand, R., and Rundel, C. (2013). rgeos: Interface to Geometry Engine-Open Source (GEOS). R Package version 0.3–2.
Bivand, R., Rundel, C., Pebesma, E., Stuetz, R., Hufthammer, K. O., and Bivand, M. R. (2017). Package “rgeos”. Available online at: ftp://ftp.sam.math.ethz.ch/sfs/Software/CRAN/web/packages/rgeos/rgeos.pdf
Bonnot, N., Morellet, N., Verheyden, H., Cargnelutti, B., Lourtet, B., Klein, F., et al. (2013). Habitat use under predation risk: hunting, roads and human dwellings influence the spatial behaviour of roe deer. Eur. J. Wildl. Res. 59, 185–193. doi: 10.1007/s10344-012-0665-8
Börner, K., Schneider, R., and Wittstatt, U. (2009). Untersuchungen zur Populationsökologie des Rotfuchses (Vulpes vulpes L.) in Berlin. Beiträge zur Jagd und Wildforschung 34, 307–313.
Bovet, P., and Benhamou, S. (1988). Spatial-analysis of animals movements using a correlated random-walk model. J. Theor. Biol. 131, 419–433. doi: 10.1016/S0022-5193(88)80038-9
Boyce, M. S. (2006). Scale for resource selection functions. Divers. Distrib. 12, 269–276. doi: 10.1111/j.1366-9516.2006.00243.x
Boyce, M. S., Vernier, P. R., Nielsen, S. E., and Schmiegelow, F. K. (2002). Evaluating resource selection functions. Ecol. Modell. 157, 281–300. doi: 10.1016/S0304-3800(02)00200-4
Brown, J. S., Laundre, J. W., and Gurung, M. (1999). The ecology of fear: optimal foraging, game theory, and trophic interactions. J. Mammal. 80, 385–399. doi: 10.2307/1383287
Bueno, C. G., Alados, C. L., Gómez-García, D., Barrio, I. C., and García-González, R. (2009). Understanding the main factors in the extent and distribution of wild boar rooting on alpine grasslands. J. Zool. 279, 195–202. doi: 10.1111/j.1469-7998.2009.00607.x
Cahill, S., Llimona, F., Cabaneros, L., and Calomardo, F. (2012). Characteristics of wild boar (Sus scrofa) habituation to urban areas in the Collserola Natural Park (Barcelona) and comparison with other locations. Anim. Biodivers. Conserv. 35, 221–233.
Cahill, S., Llimona, F., and Gracia, J. (2003). Spacing and nocturnal activity of wild boar Sus scrofa in a Mediterranean metropolitan park. Wildl. Biol. 9, 3–13.
Calenge, C. (2006). The package “adehabitat” for the R software: A tool for the analysis of space and habitat use by animals. Ecol. Modell. 197, 516–519. doi: 10.1016/j.ecolmodel.2006.03.017
Castillo-Contreras, R., Carvalho, J., Serrano, E., Mentaberre, G., Fernández-Aguilar, X., Colom, A., et al. (2018). Urban wild boars prefer fragmented areas with food resources near natural corridors. Sci. Total Environ. 615, 282–288. doi: 10.1016/j.scitotenv.2017.09.277
Chiari, M., Ferrari, N., Bertoletti, M., Avisani, D., Cerioli, M., Zanoni, M., et al. (2015). Long-term surveillance of aujeszky's disease in the alpine wild boar (Sus scrofa). Ecohealth 12, 563–570. doi: 10.1007/s10393-015-1064-x
Ciuti, S., Northrup, J. M., Muhly, T. B., Simi, S., Musiani, M., Pitt, J. A., et al. (2012). Effects of humans on behaviour of wildlife exceed those of natural predators in a landscape of fear. PLoS ONE 7:e50611. doi: 10.1371/journal.pone.0050611
Codling, E. A., Plank, M. J., and Benhamou, S. (2008). Random walk models in biology. J. R. Soc. Interface 5, 813–834. doi: 10.1098/rsif.2008.0014
Cousse, S., Janeau, G., and Spitz, F. (1992). “Telemetry on free-ranging wild boars (Sus scrofa L.): temporal and spatial structure of daily movements,” in Wildlife Telemetry. Remote Monitoring and Tracking of Animals, eds I. G. Priede and S. W. Swift (New York, NY: Hellis Horwood), 693–697. Available online at: http://prodinra.inra.fr/record/105532
Dardaillon, M., and Beugnon, G. (1987). The influence of some environmental characteristics on the movements of wild boar Sus-Scrofa. Biol. Behav. 12, 82–92.
Davison, J., Huck, M., Delahay, R. J., and Roper, T. J. (2008). Urban badger setts: characteristics, patterns of use and management implications. J. Zool. 275, 190–200. doi: 10.1111/j.1469-7998.2008.00424.x
Dingemanse, N. J., Kazem, A. J. N., Réale, D., and Wright, J. (2010). Behavioural reaction norms: animal personality meets individual plasticity. Trends Ecol. Evol. 25, 81–89. doi: 10.1016/j.tree.2009.07.013
Dinter, U. (1991). Das Raum-Zeitverhalten von Schwarzwild im Grunewald in den Sommermonaten unter Besonderer Berücksichtigung Menschlicher Störungen. Dissertation, Ludwig-Maximilian-Universität.
Dowding, C. V., Harris, S., Poulton, S., and Baker, P. J. (2010). Nocturnal ranging behaviour of urban hedgehogs, Erinaceus europaeus, in relation to risk and reward. Anim. Behav. 80, 13–21. doi: 10.1016/j.anbehav.2010.04.007
Duchesne, T., Fortin, D., and Rivest, L.-P. (2015). Equivalence between step selection functions and biased correlated random walks for statistical inference on animal movement. PLoS ONE 10:e0122947. doi: 10.1371/journal.pone.0122947
Fernández, N., Kramer-Schadt, S., and Thulke, H.-H. (2006). Viability and risk assessment in species restoration: planning reintroductions for the wild boar, a potential disease reservoir. Ecol. Soc. 11:6. Available online at: http://www.ecologyandsociety.org/vol11/iss1/art6/
Ficetola, G. F., Bonardi, A., Mairota, P., Leronni, V., and Padoa-Schioppa, E. (2014). Predicting wild boar damages to croplands in a mosaic of agricultural and natural areas. Curr. Zool. 60, 170–179. doi: 10.1093/czoolo/60.2.170
Fischer, J. D., Schneider, S. C., Ahlers, A. A., and Miller, J. R. (2015). Categorizing wildlife responses to urbanization and conservation implications of terminology. Conserv. Biol. 29, 1246–1248. doi: 10.1111/cobi.12451
Fox, J., Weisberg, S., Friendly, M., Hong, J., Andersen, R., Firth, D., et al. (2014). Effect displays for linear, generalized linear, and other models. J. Stat. Softw. 8, 1–27.
Frackowiak, W., Gorczyca, S., Merta, D., and Wojciuch-Ploskonka, M. (2013). Factors affecting the level of damage by wild boar in farmland in north-eastern Poland. Pest Manag. Sci. 69, 362–366. doi: 10.1002/ps.3368
Frid, A., and Dill, L. (2002). Human-caused disturbance stimuli as a form of predation risk. Conserv. Ecol. 6, 16. doi: 10.5751/ES-00404-060111
Gallagher, A. J., Creel, S., Wilson, R. P., and Cooke, S. J. (2017). Energy landscapes and the landscape of fear. Trends Ecol. Evol. 32, 88–96. doi: 10.1016/j.tree.2016.10.010
Gehrt, S. D., Anchor, C., and White, L. A. (2009). Home range and landscape use of coyotes in a metropolitan landscape: conflict or coexistence? J. Mammal. 90, 1045–1057. doi: 10.1644/08-MAMM-A-277.1
George, S. L., and Crooks, K. R. (2006). Recreation and large mammal activity in an urban nature reserve. Biol. Conserv. 133, 107–117. doi: 10.1016/j.biocon.2006.05.024
Gloor, S., Bontadina, F., Hegglin, D., Deplazes, P., and Breitenmoser, U. (2001). The rise of urban fox populations in Switzerland. Mamm. Biol 66, 155–164.
Gray, M., Wilmers, C. C., Reed, S. E., and Merenlender, A. M. (2016). Landscape feature-based permeability models relate to puma occurrence. Landsc. Urban Plan. 147, 50–58. doi: 10.1016/j.landurbplan.2015.11.009
Grinder, M. I., and Krausman, P. R. (2001). Home range, habitat use, and nocturnal activity of coyotes in an urban environment. J. Wild. Manag. 65, 887–898. doi: 10.2307/3803038
Hastie, T., and Tibshirani, R. (1987). Generalized additive-models - some applications. J. Am. Stat. Assoc. 82, 371–386. doi: 10.1080/01621459.1987.10478440
Herrero, J., García-Serrano, A., Couto, S., Ortuño, V. M., and García-González, R. (2006). Diet of wild boar Sus scrofa L. and crop damage in an intensive agroecosystem. Eur. J. Wildl. Res. 52, 245–250. doi: 10.1007/s10344-006-0045-3
Hespeler, B. (2007). Leitlinie Jagd im urbanen Raum von Berlin. Senatsverwaltung fuer Stadt und Umwelt, Berliner Forsten.
Hijmans, R. J., Phillips, S., Leathwick, J., and Elith, J. (2015). dismo: Species distribution Modeling. R Package Version 1.0-12. Vienna: R Foundation for Statistical Computing. Available online at: http://cranr-project.org
Hijmans, R. J., and Van Etten, J. (2014). raster: Geographic Data Analysis and Modeling. R package version 2, 15.
Hughes, J., and Macdonald, D. W. (2013). A review of the interactions between free-roaming domestic dogs and wildlife. Biol. Conserv. 157, 341–351. doi: 10.1016/j.biocon.2012.07.005
Janeau, G., Cousse, S., Cargnelutti, B., and Spitz, F. (1995). Role of daily movements in the sociospatial organization of wild boar populations (Sus-Scrofa L). Rev. Ecol. Terre 50, 35–48.
Jansen, A., Luge, E., Guerra, B., Wittschen, P., Gruber, A. D., Loddenkemper, C., et al. (2007). Leptospirosis in urban wild boars, Berlin, Germany. Emerg. Infect. Dis. 13, 739–742. doi: 10.3201/eid1305.061302
Jarolimek, J., Vanek, J., Jezek, M., Masner, J., and Stoces, M. (2014). The telemetric tracking of wild boar as a tool for field crops damage limitation. Plant Soil Environ. 60, 418–425.
Johnson, C. J., Nielsen, S. E., Merrill, E. H., McDonald, T. L., and Boyce, M. S. (2006). Resource selection functions based on use-availability data: theoretical motivation and evaluation methods. J. Wild. Manag. 70, 347–357. doi: 10.2193/0022-541X(2006)70[347:RSFBOU]2.0.CO;2
Kareiva, P. M., and Shigesada, N. (1983). Analyzing insect movement as a correlated random-walk. Oecologia 56, 234–238. doi: 10.1007/BF00379695
Kertson, B. N., Spencer, R. D., Marzluff, J. M., Hepinstall-Cymerman, J., and Grue, C. E. (2011). Cougar space use and movements in the wildland-urban landscape of western Washington. Ecol. Appl. 21, 2866–2881. doi: 10.1890/11-0947.1
Keuling, O., Strauss, E., and Siebert, U. (2016). Regulating wild boar populations is “somebody else's problem”! - Human dimension in wild boar management. Sci. Tot. Environ. 554, 311–319. doi: 10.1016/j.scitotenv.2016.02.159
Klar, N., Fernandez, N., Kramer-Schadt, S., Herrmann, M., Trinzen, M., Buttner, I., et al. (2008). Habitat selection models for European wildcat conservation. Biol. Conserv. 141, 308–319. doi: 10.1016/j.biocon.2007.10.004
Laundré, J. W., Hernández, L., López Medina, P., Campanella, A., López-Portillo, J., González-Romero, A., et al. (2014). The landscape of fear: the missing link to understand top-down and bottom-up controls of prey abundance? Ecology 95, 1141–1152. doi: 10.1890/13-1083.1
Laundre, J. W., Hernandez, L., and Ripple, W. J. (2010). The landscape of fear: ecological implications of being afraid. Open Ecol. J. 3, 1–7. doi: 10.2174/1874213001003030001
Laznik, Z., and Trdan, S. (2014). Evaluation of different soil parameters and wild boar (Sus scrofa L.) grassland damage. Ital. J. Anim. Sci. 13, 7. doi: 10.4081/ijas.2014.3434
Licoppe, A., Prévot, C., Bovy, C., and Casaer, J. (2013). Wild Boar/Feral Pig in (peri-) Urban Areas. Brussels: International Survey Report as an Introduction to the Workshop: Managing Wild Boar in Human-Dominated Landscapes. International Union of Game Biologists-Congress (IUGB).
Lowry, H., Lill, A., and Wong, B. B. M. (2013). Behavioural responses of wildlife to urban environments. Biol. Rev. 88, 537–549. doi: 10.1111/brv.12012
Magle, S. B., Hunt, V. M., Vernon, M., and Crooks, K. R. (2012). Urban wildlife research: past, present, and future. Biol. Conserv. 155, 23–32. doi: 10.1016/j.biocon.2012.06.018
Magle, S. B., Simoni, L. S., Lehrer, E. W., and Brown, J. S. (2014). Urban predator-prey association: coyote and deer distributions in the Chicago metropolitan area. Urban Ecosyst. 17, 875–891. doi: 10.1007/s11252-014-0389-5
Maibeche, Y., Moali, A., Yahi, N., and Menard, N. (2015). Is diet flexibility an adaptive life trait for relictual and peri-urban populations of the endangered primate Macaca Sylvanus? PLoS ONE 10:e0118596. doi: 10.1371/journal.pone.0118596
Manly, B. F., McDonald, L., Thomas, D., McDonald, T. L., and Erickson, W. P. (2002). Resource Selection by Animals: Statistical Design and Analysis for Field Studies, 2nd Edn. Springer Netherlands.
Massei, G., Kindberg, J., Licoppe, A., Gacić, D., Šprem, N., Kamler, J., et al. (2015). Wild boar populations up, numbers of hunters down? A review of trends and implications for Europe. Pest Manag. Sci. 71, 492–500. doi: 10.1002/ps.3965
McKinney, M. L. (2002). Urbanization, biodiversity, and conservation. Bioscience 52, 883–890. doi: 10.1641/0006-3568(2002)052[0883:UBAC]2.0.CO;2
Morelle, K., Lehaire, F., and Lejeune, P. (2013). Spatio-temporal patterns of wildlife-vehicle collisions in a region with a high-density road network. Nat. Conserv. Bulgaria 5, 53–73. doi: 10.3897/natureconservation.5.4634
Morelle, K., Lehaire, F., and Lejeune, P. (2014). Is wild boar heading towards movement ecology? A review of trends and gaps. Wild. Biol. 20, 196–205. doi: 10.2981/wlb.00017
Morelle, K., Podgorski, T., Prevot, C., Keuling, O., Lehaire, F., and Lejeune, P. (2015). Towards understanding wild boar Sus scrofa movement: a synthetic movement ecology approach. Mamm. Rev. 45, 15–29. doi: 10.1111/mam.12028
Murray, M., Cembrowski, A., Latham, A. D. M., Lukasik, V. M., Pruss, S., and St Clair, C. C. (2015). Greater consumption of protein-poor anthropogenic food by urban relative to rural coyotes increases diet breadth and potential for human-wildlife conflict. Ecography 38, 1235–1242. doi: 10.1111/ecog.01128
Murray, M. H., and St Clair, C. C. (2015). Individual flexibility in nocturnal activity reduces risk of road mortality for an urban carnivore. Behav. Ecol. 26, 1520–1527. doi: 10.1093/beheco/arv102
Nores, C., Llaneza, L., and Alvarez, M. A. (2008). Wild boar Sus scrofa mortality by hunting and wolf Canis lupus predation: an example in northern Spain. Wild. Biol. 14, 44–51. doi: 10.2981/0909-6396(2008)14[44:WBSSMB]2.0.CO;2
Ottoni, I., De Oliveira, F. F. R., and Young, R. J. (2009). Estimating the diet of urban birds: the problems of anthropogenic food and food digestibility. Appl. Anim. Behav. Sci. 117, 42–46. doi: 10.1016/j.applanim.2008.11.002
Pebesma, E. J., and Bivand, R. S. (2005). Classes and methods for spatial data in R. R News 5, 9–13.
Podgorski, T., Bas, G., Jedrzejewska, B., Soennichsen, L., Sniezko, S., Jedrzejewski, W., et al. (2013). Spatiotemporal behavioral plasticity of wild boar (Sus scrofa) under contrasting conditions of human pressure: primeval forest and metropolitan area. J. Mammal. 94, 109–119. doi: 10.1644/12-MAMM-A-038.1
Poessel, S. A., Burdett, C. L., Boydston, E. E., Lyren, L. M., Alonso, R. S., Fisher, R. N., et al. (2014). Roads influence movement and home ranges of a fragmentation-sensitive carnivore, the bobcat, in an urban landscape. Biol. Conserv. 180, 224–232. doi: 10.1016/j.biocon.2014.10.010
R Core Team (2017). R: A Language and Environment for Statistical Computing. Vienna: R Foundation for Statistical Computing. Available online at: http://www.R-project.org/
Rosner, S., Mussard-Forster, E., Lorenc, T., and Muller, J. (2014). Recreation shapes a “landscape of fear” for a threatened forest bird species in Central Europe. Landsc. Ecol. 29, 55–66. doi: 10.1007/s10980-013-9964-z
Rosset, S. (2004). “Model selection via the AUC,” in Proceedings of the Twenty-First International Conference on Machine Learning (Banff, AB: ACM), 89.
Schley, L., and Roper, T. J. (2003). Diet of wild boar Sus scrofa in Western Europe, with particular reference to consumption of agricultural crops. Mamm. Rev. 33, 43–56. doi: 10.1046/j.1365-2907.2003.00010.x
Sodeikat, G., and Pohlmeyer, K. (2003). Escape movements of family groups of wild boar Sus scrofa influenced by drive hunts in Lower Saxony, Germany. Wild. Biol. 9, 43–49.
Stillfried, M., Belant, J. L., Svoboda, N. J., Beyer, D. E., and Kramer-Schadt, S. (2015). When top predators become prey: black bears alter movement behaviour in response to hunting pressure. Behav. Process. 120, 30–39. doi: 10.1016/j.beproc.2015.08.003
Stillfried, M., Fickel, J., Börner, K., Wittstatt, U., Heddergott, M., Ortmann, S., et al. (2017a). Do cities represent sources, sinks or isolated islands for urban wild boar population structure? J. Appl. Ecol. 54, 272–281. doi: 10.1111/1365-2664.12756
Stillfried, M., Gras, P., Busch, M., Börner, K., Kramer-Schadt, S., and Ortmann, S. (2017b). Wild inside: urban wild boar select natural, not anthropogenic food resources. PLoS ONE 12:e0175127. doi: 10.1371/journal.pone.0175127
Støen, O. G., Ordiz, A., Evans, A. L., Laske, T. G., Kindberg, J., Fröbert, O., et al. (2015). Physiological evidence for a human-induced landscape of fear in brown bears (Ursus arctos). Physiol. Behav. 152, 244–248. doi: 10.1016/j.physbeh.2015.09.030
Theimer, T. C., Clayton, A. C., Martinez, A., Peterson, D. L., and Bergman, D. L. (2015). Visitation rate and behavior of urban mesocarnivores differs in the presence of two common anthropogenic food sources. Urban Ecosyst. 18, 895–906. doi: 10.1007/s11252-015-0436-x
Thurfjell, H., Ciuti, S., and Boyce, M. S. (2014). Applications of step-selection functions in ecology and conservation. Mov. Ecol. 2:4. doi: 10.1186/2051-3933-2-4
Thurfjell, H., Spong, G., and Ericsson, G. (2013). Effects of hunting on wild boar Sus scrofa behaviour. Wild. Biol. 19, 87–93. doi: 10.2981/12-027
Thurfjell, H., Spong, G., Olsson, M., and Ericsson, G. (2015). Avoidance of high traffic levels results in lower risk of wild boar-vehicle accidents. Landsc. Urban Plan. 133, 98–104. doi: 10.1016/j.landurbplan.2014.09.015
Tigas, L. A., Van Vuren, D. H., and Sauvajot, R. M. (2002). Behavioral responses of bobcats and coyotes to habitat fragmentation and corridors in an urban environment. Biol. Conserv. 108, 299–306. doi: 10.1016/S0006-3207(02)00120-9
Tryjanowski, P., Skórka, P., Sparks, T. H., Biadun, W., Brauze, T., Hetmanski, T., et al. (2015). Urban and rural habitats differ in number and type of bird feeders and in bird species consuming supplementary food. Environ. Sci. Pollut. Res. 22, 15097–15103. doi: 10.1007/s11356-015-4723-0
Valeix, M., Hemson, G., Loveridge, A. J., Mills, G., and Macdonald, D. W. (2012). Behavioural adjustments of a large carnivore to access secondary prey in a human-dominated landscape. J. Appl. Ecol. 49, 73–81. doi: 10.1111/j.1365-2664.2011.02099.x
Wagenmakers, E.-J., and Farrell, S. (2004). AIC model selection using Akaike weights. Psychon. Bull. Rev. 11, 192–196. doi: 10.3758/BF03206482
Keywords: behavioral adjustment, GPS-telemetry, habitat selection, human wildlife conflict, ungulate, correlated random walk, space use, urbanization
Citation: Stillfried M, Gras P, Börner K, Göritz F, Painer J, Röllig K, Wenzler M, Hofer H, Ortmann S and Kramer-Schadt S (2017) Secrets of Success in a Landscape of Fear: Urban Wild Boar Adjust Risk Perception and Tolerate Disturbance. Front. Ecol. Evol. 5:157. doi: 10.3389/fevo.2017.00157
Received: 12 September 2017; Accepted: 22 November 2017;
Published: 06 December 2017.
Edited by:
Federico Morelli, Czech University of Life Sciences Prague, CzechiaReviewed by:
Hannes J. König, Leibniz-Zentrum für Agrarlandschaftsforschung (ZALF), GermanyJari Niemelä, University of Helsinki, Finland
Copyright © 2017 Stillfried, Gras, Börner, Göritz, Painer, Röllig, Wenzler, Hofer, Ortmann and Kramer-Schadt. This is an open-access article distributed under the terms of the Creative Commons Attribution License (CC BY). The use, distribution or reproduction in other forums is permitted, provided the original author(s) or licensor are credited and that the original publication in this journal is cited, in accordance with accepted academic practice. No use, distribution or reproduction is permitted which does not comply with these terms.
*Correspondence: Milena Stillfried, stillfried@izw-berlin.de