- 1Science and Conservation Division, Western Australian Department of Biodiversity, Conservation and Attractions, Bentley, WA, Australia
- 2School of Biological Sciences, The University of Western Australia, Crawley, WA, Australia
There is increasing awareness that the long-term success of ecological restoration efforts can be compromised if projected climate change is not effectively incorporated in restoration planning. We propose an approach that aims to support the decision-making process for seed provenance selection in ecological restoration when clear genetic-based guidelines for seed transfer are not available. The approach takes advantage of the increasing availability and refinement of user-friendly web-based GIS interfaces that allow non-experts to directly access biodiversity and environmental data, and build species distribution models. It offers an easily accessible desktop method that land managers and practitioners can use to gain insight: (1) on the overall spatial implications of projected climate change to their restoration project; (2) whether assisted gene flow through climate-adjusted provenance may be appropriate for a given species at a given restoration site; and (3) how far away and in which direction from the restoration site seeds should be collected from. This approach should be used in the early stages of the restoration project to help frame the decision-making process in a climate change context, and can also be used as a platform where other lines of evidence are integrated. We advocate that, in the context of rapid climate change, the climate-adjusted provenance is a promising approach to seed sourcing in ecological restoration, and we suggest its refinement in a way that hedges against uncertainty in climatic projections.
Introduction
Worldwide, ecological restoration is being used to mitigate environmental degradation, with global annual investment surpassing $US 2 trillion (Aitken and Whitlock, 2013; Williams et al., 2014). Concomitantly, there is increasing awareness of the threat anthropogenic climate change poses to this investment, and the need to explicitly consider and manage the risk through strategies such as the managed translocation of germplasm within (assisted gene flow) or outside (assisted migration) current species ranges (Erwin, 2009; Aitken and Whitlock, 2013; Lunt et al., 2013). This has prompted a discussion about seed provenance strategies that maximize the resilience of restoration plantings to the effects of projected climate change (Broadhurst et al., 2008; Sgrò et al., 2011; Breed et al., 2013; Prober et al., 2015; Hodgins and Moore, 2016).
Until recently, the most common seed-sourcing approach has been local provenance, where seeds are collected locally. This approach is based on evidence that local genotypes are often best adapted to local environmental conditions and outperform non-local genotypes in those conditions (e.g., Keller et al., 2000, although see Leimu and Fischer, 2008). However, strict adherence to local provenancing has been challenged for encouraging the establishment of populations that may not harbor sufficient genetic diversity to cope with rapid environmental change, and for potentially encouraging the selection of inbred or genetically depauperate seed sources in highly transformed and fragmented landscapes (Broadhurst et al., 2008; Sgrò et al., 2011; Breed et al., 2013).
The potential limitations of restricting seed collections to local provenance in an era of rapid environmental change have led to the development of alternative seed sourcing strategies that seek to maximize genetic variation. Composite provenancing attempts to mimic natural gene flow dynamics and uses mixed seed sources collected in a decreasing radius from the restoration site (Broadhurst et al., 2008). Admixture provenancing also uses mixed seed sources but has no spatial bias toward the restoration site (Breed et al., 2013). Predictive provenancing prescribes collecting seeds from areas that currently have climatic conditions matching those projected for the restoration site (Crowe and Parker, 2008; Bellon et al., 2011; Sgrò et al., 2011). This approach has been largely used in forestry and relies on species distribution models (SDMs) to identify seed provenance regions (e.g., Isaac-Renton et al., 2014). Finally, climate-adjusted provenancing advocates collecting seeds from multiple populations in the direction of the projected climate change, including local seeds (Prober et al., 2015). The latter two provenance strategies explicitly consider the directional nature of climate change. Furthermore, they both aim to increase the adaptive capacity of species to projected climate change at a restoration site using seeds from pre-adapted populations elsewhere in the species range.
Ideally, the identification of seed transfer zones for ecological restoration (whether to confirm local provenance or to identify adaptive capacity) is informed by knowledge of the species genetic adaptive capacities, derived from common garden trials, reciprocal transplant experiments (e.g., Mátyás, 1996), and even genomic screening (e.g., De Kort et al., 2014; Steane et al., 2014, 2017a). However, this information has been mostly obtained for commercial or dominant tree species (e.g., Gray et al., 2011; Isaac-Renton et al., 2014; Steane et al., 2017b). Only recently have efforts been made to establish genetic-based seed transfer zones for key species used in restoration, but information is generally limited for most species and regions (Clair et al., 2013; Durka et al., 2017; see also Table 1 in Bower et al., 2014).
A Spatially Explicit Approach to Support the Decision-Making Process for Seed Provenance Selection
We propose a spatially explicit desktop approach to support seed provenance selection in restoration projects when clear guidelines for genetic-based seed transfer zones are not available. Our approach provides a simple and readily accessible method that practitioners can use to gain insight: (1) on the overall spatial implications of projected climate change to their restoration project; (2) whether assisted gene flow through climate adjusted provenancing may be appropriate for a given species at a given restoration site; and (3) how far away and in which direction from the restoration site seeds should be collected.
The proposed approach requires geographic information system (GIS) software for visualization and preparation of spatial layers, and SDM software for estimation of species distributions under historical and projected climates. Examples of freely and openly available GIS software include GRASS GIS (https://grass.osgeo.org) and QGIS (http://www.qgis.org). Maxent (Phillips et al., 2006; Phillips and Dudík, 2008) is the most widely used software for SDM and is also freely and openly available (https://biodiversityinformatics.amnh.org/open_source/maxent/). The SDMtoolbox (Brown, 2014) is a GIS toolkit to be used with the commercial software ArcGIS (version 10.1 or higher; ESRI), and it embeds Maxent as well as several tools that facilitate the data preparation required for model building and post-modeling processing.
Alternatively, practitioners can make use of the increasing availability and ongoing refinement of user-friendly web-based GIS interfaces that allow non-experts to directly access biodiversity and environmental data and build SDMs. These include, for example, the Atlas of Living Australia (http://www.ala.org.au), the Biodiversity and Climate Change Virtual Lab (http://www.bccvl.org.au), and Lifemapper (http://lifemapper.org). These web-based GIS interfaces vary in their geographic coverage, type of environmental data available, modeling techniques and settings (see Table 1). Hence, the decision on whether to use desktop software or a web-based GIS interface, and which interface, depends on the available GIS and modeling skills, software, environmental data and geographic region, among others.
Geographic information systems can provide an effective platform for accessing and visualizing key spatial data relevant for provenance planning, including historical species distributions, historical, and projected climate layers, species habitat suitability (even if coarse) under current and projected climates, and other environmental factors that affect species distributions (e.g., soil, topography, habitat fragmentation). Nevertheless, the availability of embedded SDM tools on web-based GIS interfaces does not replace the direct involvement of professional modelers when fine-tuned results are required. This is because those interfaces can only afford limited ability to explore different data sets and model settings, and because of the inherent knowledge required for good modeling (Guisan et al., 2013).
The proposed approach focuses on a pool of plant species occurring at the restoration site and/or immediate vicinity and that can be used in revegetation, and uses the following three questions to determine which provenance approach is likely appropriate for each species (Figure 1).
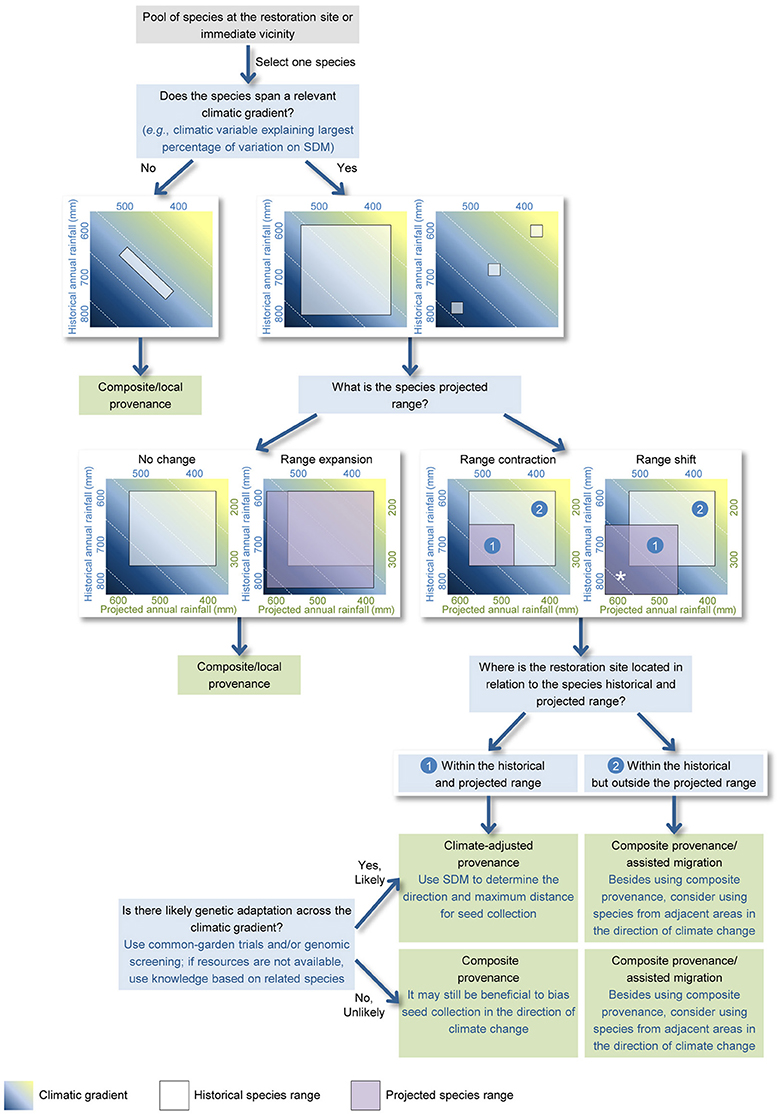
Figure 1. A spatially explicit approach to support the decision-making process for seed provenance selection in ecological restoration when clear guidelines for genetic-based seed transfer are not available. The approach relies on the sequential query of spatial data (including species distribution, and historical and projected climatic conditions) to determine which provenance approach is likely most suitable for a given species at a given restoration site. Three main questions used are: (1) Does the species span a relevant climatic gradient? (2) What is the species projected range under future climate conditions? (3) Where is the restoration site located in relation to the species historical and projected range? The proposed approach can also help understanding which species from adjacent warmer and drier/wetter areas can be suitable for the revegetation works. In such cases, non-local species that are predicted to have their range shifted toward the restoration site (marked with an asterisk *) could be potential candidates.
Does the Species Span a Relevant Climatic Gradient?
The size and spread of a species distribution can be assessed through either point data or estimated habitat suitability. If a widespread species spans a relevant climatic gradient (e.g., temperature of the warmest quarter, annual rainfall), then there is scope for assisted gene flow from warmer and drier/wetter environments.
How Is the Species Distribution Projected to Change?
Response patterns to climate change indicated by SDMs include no change, range expansion, range contraction, and complete range shift. When species ranges are predicted to contract or shift, a provenance approach that incorporates existing genetic adaptation from warmer and drier/wetter conditions may be appropriate (Crowe and Parker, 2008; Sgrò et al., 2011; Prober et al., 2015). SDMs can also provide guidance on how far from the restoration site seeds should be collected. The choice of climate change severity scenario and projection year will greatly influence the SDM-suggested distances. Long-term climatic projections have a high degree of uncertainty and represent a greater mismatch with the short-term climatic conditions plants may endure in the early, more vulnerable, stages of their life cycle (i.e., seedling and sapling stages; Gray et al., 2011; Isaac-Renton et al., 2014). For this reason, it is generally recommended that projection timeframes of 10–30 years be used (Gray et al., 2011; Aitken and Bemmels, 2016). It has also been recommended that the choice of climate change severity scenario should be dependent on the projects' approach to risk (Snover et al., 2013), as explained in Section Dealing with Uncertainty. We recommend that the maximum distance for seed collection should not exceed the distance between the restoration site and sites with current climatic conditions similar to those projected for the former (Figure 1).
Where Is the Restoration Site Located in Relation to the Species Historical and Projected Range?
Clear understanding of the location of the restoration site in relation to the historical and projected species range is a simple and yet key element for provenance planning. If the restoration site is located at the center or cooler boundary of the species distribution, then assisted gene flow incorporating genetic adaptation from warmer and drier/wetter areas may facilitate persistence under future climates. In contrast, if the restoration site is located at the species warmer, contracting range boundary and/or outside the projected range, then a local/composite provenance is the only appropriate option. In this situation, there are no pre-adapted populations from which to source seed from, and thus adaptation depends largely on variation within the local population (Davis and Shaw, 2001). In these circumstances, restoration of the historical community assemblage may not be an adequate goal, and consideration should be given to selection of different species from adjacent warmer and drier/wetter areas that occupy similar habitat conditions to that of the restored site (Figure 1). This process of purposefully moving species to facilitate or mimic natural range expansion as a direct management response to climate change is known as assisted migration. In this case, SDMs can be used to understand whether those (non-local) species are likely to have their range shifted toward the restoration site (marked with an * on Figure 1) under future climatic conditions.
Integrating Other Lines of Evidence
Consideration of genetic adaptive variation is most important when a species spans a regional climatic gradient, when its range is predicted to contract or shift, and when restoration sites are within the historical and projected range. When genetic adaptive variation data are not available, nor are the resources, in terms of funding or time, to undertake such investigations, knowledge developed on related species could be used (Byrne et al., 2013; Aitken and Bemmels, 2016). Mbogga et al. (2010) and Isaac-Renton et al. (2014) also suggest that field-collected or remotely sensed-data of climate-driven plant health decline (especially relevant for trees) could be used to advocate for a provenance approach that increases the resilience of restoration plantings through assisted gene flow. In contrast, if the populations of a widespread species are isolated by topographic or environmental features (e.g., mountain ranges, granite outcrops in a matrix of a different geology; Tapper et al., 2014), then a more cautious approach is needed. This might include consideration of the likely role of those features on the partitioning of genetic diversity between populations.
Habitat fragmentation is another important factor to be considered when planning provenance selection. Seed quality and seed quantity may be compromised in small remnant populations because of inbreeding and reduced pollinator activity (Young et al., 1996). For this reason, collection of genetic material should seek, as much as possible, large, healthy populations (Hancock et al., 2016; Harrison et al., 2017).
Key known ecological interactions should also be taken into account when climate-adjusted provenance or assisted migration of species from adjacent warmer areas is being considered (Lunt et al., 2013). The possible role of ecological interactions (e.g., competition, trophic associations, and mutualisms) in limiting the success of assisted migration interventions has been discussed (e.g., McLachlan et al., 2007). For example, species may not establish in areas unless obligatory mutualists, such as pollinators (e.g., Pemberton, 2010) or mycorrhizal fungi important for plant germination and growth (e.g., Schwartz et al., 2006), occur in, or are moved to, that same area. On the other hand, predation or disease at the recipient site may also prevent plant establishment.
Dealing with Uncertainty
Decisions on provenance selection in an era of extensive land transformation and rapid climate change are typically challenged by uncertainty. In SDM, uncertainty can originate from several sources, including parameterization of the models, climate projections, and quality and resolution of the species presence and environmental data (Barry and Elith, 2006; Heikkinen et al., 2006). Some sources of SDM uncertainty can be minimized through implementation of good modeling practices. These include data cleaning for elimination of spurious and misidentified records, selection of non-correlated predictors and analysis of their fitted response functions, consideration of the sampling bias, and use of sensitivity analyses to evaluate the impact of model calibration decisions (Elith and Leathwick, 2009). However, other sources of uncertainty, such as the inherent uncertainty of climate change projections, are irreducible, but can be explicitly dealt with using established methods (Littell et al., 2011). For example, Snover et al. (2013) provide a structured approach and general guidelines for selecting climate-change scenarios for ecological impact assessment and conservation decision making, which can be applied to provenance selection. Importantly, these authors recommended that the choice of climate change severity scenario should be dependent on the projects' approach to risk. A risk-adverse approach that seeks to minimize climate risk may use a high severity climate scenario (the largest negative effects are taken into account so to prevent the worst case outcomes); whereas a low or intermediate severity scenario might be more appropriate for a risk-tolerant approach (Snover et al., 2013). Genetic risks also need to be evaluated through a genetic risk framework (Byrne et al., 2011), and taken into account in this decision-making process. Ensemble analysis is another example of an approach to deal with uncertainty in climate projections, and which characterizes and maps the consensus across different global climate models (Littell et al., 2011). Finally, Lee-Yaw et al. (2016) also suggest combining SDMs and reciprocal transplantation experiments to iteratively reduce the uncertainty on both approaches.
We advocate that climate-adjusted provenancing provides an approach to enhancing adaptation of restoration plantings to future climates that maximizes the benefits of mixing seed sources to increase adaptive genetic variation whilst minimizing risk. This approach explicitly considers the directional nature of climate change (in contrast to the composite and admixture provenances). Furthermore, it capitalizes on naturally occurring genetic adaptation to warmer and drier/wetter conditions that may be present in the species local and regional gene pools along the climatic gradient (e.g., McLean et al., 2014; Steane et al., 2014, 2017a; Prober et al., 2015). Moreover, it is less affected by SDM uncertainty than the climate-predictive provenance, being able to hedge against uncertainty in SDMs projections. This is because it can use SDMs to establish the direction and spatial distances from the restoration site seeds should be collected, but does not rely on those models to explicitly identify provenance zones that match future climates. Equally important, the climate-adjusted provenance approach can be implemented in an adaptive management framework (Williams, 2011). This, on the one hand, can weigh the uncertainty present in each case on the spatial distances for seed collection and, on the other hand, empirically test the use of seeds from different non-local populations in experimental plantings along with local sources (Harrison et al., 2017).
A Case Study—Ecological Restoration of Riparian Habitat in South-Western Australia
The recent decline of the invasive European blackberry (Rubus anglocandicans) in the lower-mid Warren catchment (34°30′29.00″S, 116°05′17.50″E) of south-western Australia due to a newly described root rot pathogen, Phytophthora bilorbang (Aghighi et al., 2014), has left large river sections denuded of riparian understory. This pathogen is not well known and its long-term interactions with European blackberry stands are not yet fully understood. The region has high conservation value, with most native forest maintained within conservation reserves, and thus, an inter-agency restoration effort was undertaken to avoid re-invasion of the riparian habitat (http://www.warrencc.org.au/Projects/warren-donnelly-rivers-restoration-biodiversity-project/).
The exposure to climate change of five key species selected for the restoration plantings (Astartea leptophylla, Banksia seminuda, Callistachys lanceolata, Lepidosperma effusum, and Taxandria linearifolia) were investigated using SDMs. Spatial data were compiled and prepared using the commercial software ArcGIS (ESRI). Species data were compiled from two biodiversity online databases (NatureMap https://naturemap.dpaw.wa.gov.au/ and Atlas of Living Australia http://www.ala.org.au/). We primarily used location records from NatureMap, which gathers data from Western Australian datasets, including the Western Australia Herbarium. We further used Atlas of Living Australia to source location records from non-Western Australian botanical collections. Data were scrutinized to remove misidentified, suspected inaccurate or duplicate records. Environmental predictors included historical (1961–1990) and projected (2050) bioclimatic (Yates et al., 2010), topo-hydrographic (Geoscience Australia, 2009), and remnant vegetation extent (Western Australian Department of Agriculture and Food, 2011) layers. The incorporation of non-collinear, fine-scale topo-hydrographic variables that depict the species requirements for explicit environmental resources can improve predictive ability of models (e.g., McLane and Aitken, 2011). Five bioclimatic variables considered most relevant to plant distributions in southwestern Australia (Yates et al., 2010) were used. These included annual precipitation, precipitation of the driest quarter, mean temperature of the warmest quarter, mean temperature of the wettest quarter, and isothermality. Projected climate data considered three climate change severity scenarios (low-, medium-, and high-severity), which are based on three emission scenarios (B1, A1B, and A1F1) and three climate sensitivities (low, medium, and high), and encompass the range of uncertainties in future climate for southwestern Australia (Yates et al., 2010).
The SDMs were conducted using the freely available software Maxent (Phillips et al., 2006; Phillips and Dudík, 2008). The geographic extent of the models was constrained to the focal and immediately neighboring biogeographic regions occupied by each species, and was the same for the five species. To account for the spatial bias in survey effort, the records density of all plant species belonging to the study species' botanical families (Cyperaceae, Fabaceae, Myrtaceae, and Proteaceae) was used as a sampling effort bias layer (Kramer-Schadt et al., 2013). For selection of the best predictors, variables with a Pearson's pairwise correlation coefficient >|0.80| were not included in the same model and were tested separately. Predictors were selected based on the percentage of variation explained, performance in the Jackknife gain tests, and whether the response curves were ecologically meaningful.
The SDMs showed that the five species: (1) are widespread over a relatively large region and span a regional climatic gradient; (2) their ranges are likely to contract over time in a south and westwards direction, with an average ±SE of range decline of 15 ± 3, 39 ± 2, and 67 ± 6%, for the low, medium, and high severity climate change scenarios; 39 ± 1, 55 ± 1, and 75 ± 6%, when habitat loss was taken into consideration; (3) species range contractions are primarily driven by changes in annual precipitation (which is projected to decline by 4, 15, and 20% by 2050 under the low, medium, and high severity scenarios; Yates et al., 2010); and (4) the restoration sites are within the historical and projected distributions.
These results, together with the significant climatic changes that have already occurred in south-western Australia since the 1970s, with a 15–20% decline in annual rainfall (Bates et al., 2008), indicate that a climate-adjusted provenance could be appropriate. This assumes the species are likely to exhibit genetic adaptive capacity, which is currently being investigated (Hopley and Byrne, unpublished data). Catchments can work as natural geographic barriers to gene flow for riparian species dependent on stream flow for seed dispersal (Nilsson et al., 2010), thus the use of seeds from other catchments is not being considered. Figure 2 presents the study area, the results of the SDMs for the five plant species, and the implications for provenance of one selected species, B. seminuda. The current and projected species range boundaries for this species were drawn based on a visual assessment of the habitat suitability map. Alternatively, suitability thresholds that convert the suitability values (varying between 0 and 1) into presence-absence maps (0/1) could have been used (Liu et al., 2005).
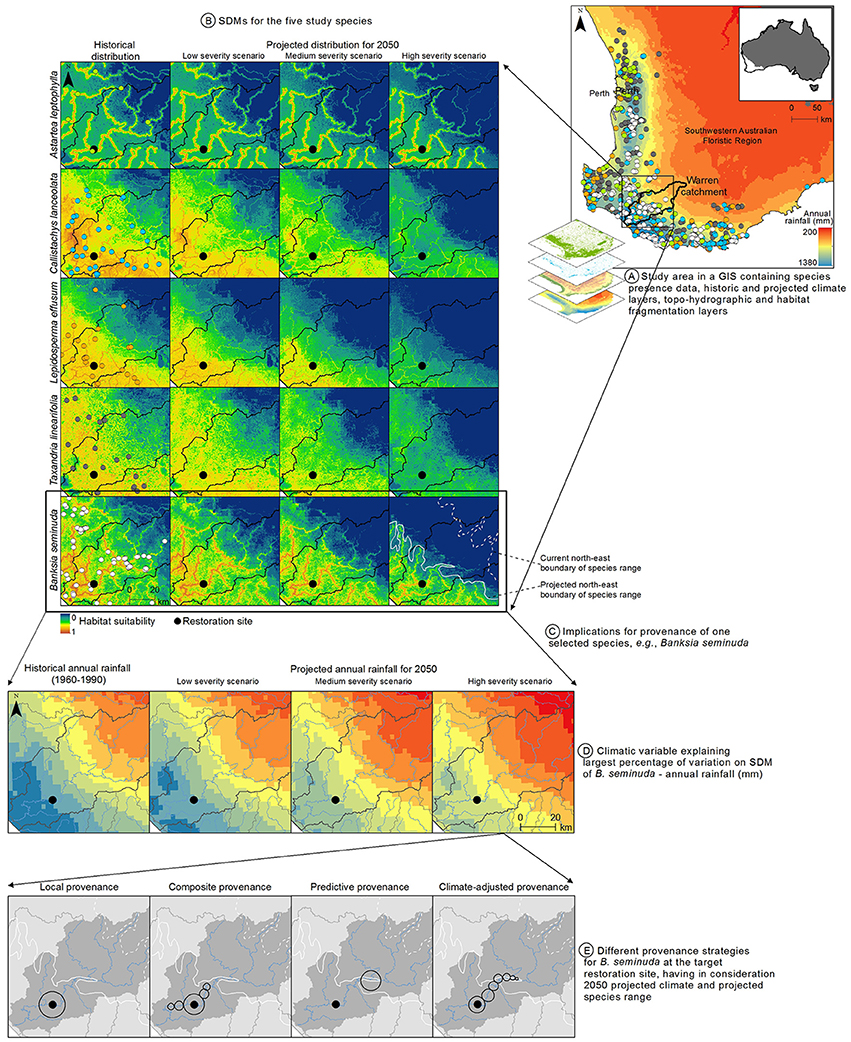
Figure 2. Illustration of the proposed approach using the case study in the Warren catchment of southwestern Australia. (A) Study area in a GIS containing the species distribution and environmental predictor data. (B) SDMs for the five species selected for restoration plantings under historical and 2050 projected climatic conditions (low, medium, and high-severity scenarios); models not taking land transformation into account are shown here for better visualization of the effects of projected climate change on species range; map colors represent habitat suitability, with warmer colors indicating higher habitat suitability and cooler colors indicating lower habitat suitability. (C) Implications for provenance of one of the species, Banksia seminuda, at the restoration site (represented with a black dot); the dashed and solid white lines represent the historical and projected north-east boundary range of B. seminuda, respectively. (D) Historical and 2050 projected annual rainfall (climatic variable explaining most variation on the SDM for B. seminuda) for the Warren catchment (100 mm classes). The annual rainfall at the restoration site is projected to decline from 1,195 mm to 1,147, 1,016, and 956 mm under the low, medium, and high severity climate change scenarios, respectively (see Yates et al., 2010 for descriptions of climate change scenarios). (E) Different approaches for provenancing B. seminuda for the given restoration site, taking into consideration projected rainfall and the projected species range. Circles represent native populations used for seed collection. The size of the circles represent the relative quantities of seeds from each population in the final seed mix.
Final Remarks
Inaction toward incorporating climate change in ecological restoration in the present may come at a high cost in the future, potentially compromising the ability of restored plant communities to cope with climate change. We propose an approach that aims to support the decision-making process for seed provenance selection in ecological restoration when clear genetic-based guidelines for seed transfer are not available. The approach takes advantage of the increasing availability and refinement of user-friendly web-based GIS interfaces that allow non-experts to directly access biodiversity and environmental data, and build SDMs. It offers an easily accessible desktop method that practitioners can use to gain insight: (1) on the overall spatial implications of projected climate change on their restoration project; (2) whether assisted gene flow through climate-adjusted provenancing may be appropriate for a given species at a given restoration site; and (3) how far away and in which direction from the restoration site seeds should be collected from. This approach should be used in the early stages of the restoration project to help frame the decision-making process in a climate change context. It should also be used as a platform where other lines of evidence are integrated. These include information about species genetic adaptive variation, climate-driven health decline, key ecological interactions, topographic or environmental features potentially leading to the partitioning of genetic diversity between populations, and habitat fragmentation. We advocate that, in the context of rapid climate change, climate-adjusted provenancing is a promising approach to seed sourcing in ecological restoration, and we suggest its refinement in a way that hedges against uncertainty in climatic projections.
Author Contributions
CR, MB, CY contributed with ideas and wrote the manuscript; CR undertook the data analysis and spatial modeling and led the writing.
Conflict of Interest Statement
The authors declare that the research was conducted in the absence of any commercial or financial relationships that could be construed as a potential conflict of interest.
Acknowledgments
We thank the Warren Catchment Council for implementing the restoration program that inspired this project, John Scott for assistance with climate modeling, and Terry MacFarlane, Juliet Wege, and Ryonen Butcher for assistance with species information. We are also thankful to Chantal Huijbers, Lee Belbin, Aimee Stewart, and Catherine Jarnevich for their assistance in providing information about the web-based GIS interfaces mentioned in the manuscript. Finally, we thank two reviewers for their thoughtful comments on earlier versions of the manuscript. The project was funded by the Australian Government through the Biodiversity Fund (project no. LSP-944784-1088).
References
Aghighi, S., Fontanini, L., Yeoh, P. B., Hardy, G. E. S. J., Burgess, T. I., and Scott, J. K. (2014). A conceptual model to describe the decline of European blackberry (Rubus anglocandicans), a weed of national significance in Australia. Plant Dis. 98, 580–589. doi: 10.1094/PDIS-11-13-1124-FE
Aitken, S. N., and Bemmels, J. B. (2016). Time to get moving: assisted gene flow of forest trees. Evol. Appl. 9, 271–290. doi: 10.1111/eva.12293
Aitken, S. N., and Whitlock, M. C. (2013). Assisted gene flow to facilitate local adaptation to climate change. Annu. Rev. Ecol. Evol. Syst. 44, 367–388. doi: 10.1146/annurev-ecolsys-110512-135747
Barry, S., and Elith, J. (2006). Error and uncertainty in habitat models. J. Appl. Ecol. 43, 413–423. doi: 10.1111/j.1365-2664.2006.01136.x
Bates, B., Hope, P., Ryan, B., Smith, I., and Charles, S. (2008). Key findings from the Indian Ocean climate initiative and their impact on policy development in Australia. Clim. Change 89, 339–354. doi: 10.1007/s10584-007-9390-9
Belbin, L. (2011). “The Atlas of living Australia's spatial portal,” in Proceedings of the Environmental Information Management Conference 2011 (EIM2011) (Santa Barbara, CA: University of California), 39–43.
Belbin, L., and Williams, K. J. (2016). Towards a national bio-environmental data facility: experiences from the Atlas of living Australia. Int. J. Geogr. Inf. Sci. 30, 108–125. doi: 10.1080/13658816.2015.1077962
Bellon, M. R., Hodson, D., and Hellin, J. (2011). Assessing the vulnerability of traditional maize seed systems in Mexico to climate change. Proc. Natl. Acad. Sci. U.S.A. 108, 13432–13437. doi: 10.1073/pnas.1103373108
Bower, A. D., Clair, J. B. S., and Erickson, V. (2014). Generalized provisional seed zones for native plants. Ecol. Appl. 24, 913–919. doi: 10.1890/13-0285.1
Breed, M., Stead, M., Ottewell, K., Gardner, M., and Lowe, A. (2013). Which provenance and where? seed sourcing strategies for revegetation in a changing environment. Conserv. Genet. 14, 1–10. doi: 10.1007/s10592-012-0425-z
Broadhurst, L. M., Lowe, A., Coates, D. J., Cunningham, S. A., McDonald, M., Vesk, P. A., et al. (2008). Seed supply for broadscale restoration: maximizing evolutionary potential. Evol. Appl. 1, 587–597. doi: 10.1111/j.1752-4571.2008.00045.x
Brown, J. L. (2014). SDMtoolbox: a python-based GIS toolkit for landscape genetic, biogeographic and species distribution model analyses. Methods Ecol. Evol. 5, 694–700. doi: 10.1111/2041-210X.12200
Byrne, M., Prober, S., McLean, L., Steane, D. A., Stock, W. D., Potts, B. M., et al. (2013). Adaptation to Climate in Widespread Eucalypt Species. National Climate Change Adaptation Research Facility, Gold Coast.
Byrne, M., Stone, L., and Millar, M. A. (2011). Assessing genetic risk in revegetation. J. Appl. Ecol. 48, 1365–2664. doi: 10.1111/j.1365-2664.2011.02045.x
Cavner, J. A., Stewart, A. M., Grady, C. J., and Beach, J. H. (2012). An innovative web processing services based GIS architecture for global biogeographic analyses of species distributions. OSGeo J. 10:11.
Clair, J. B. S., Kilkenny, F. F., Johnson, R. C., Shaw, N. L., and Weaver, G. (2013). Genetic variation in adaptive traits and seed transfer zones for Pseudoroegneria spicata (bluebunch wheatgrass) in the northwestern United States. Evol. Appl. 6, 933–948. doi: 10.1111/eva.12077
Crowe, K. A., and Parker, W. H. (2008). Using portfolio theory to guide reforestation and restoration under climate change scenarios. Clim. Change 89, 355–370. doi: 10.1007/s10584-007-9373-x
Davis, M. B., and Shaw, R. G. (2001). Range shifts and adaptive responses to quaternary climate change. Science 292, 673–679. doi: 10.1126/science.292.5517.673
De Kort, H., Mergeay, J., Vander Mijnsbrugge, K., Decocq, G., Maccherini, S., Kehlet Bruun, H. H., et al. (2014). An evaluation of seed zone delineation using phenotypic and population genomic data on black alder Alnus glutinosa. J. Appl. Ecol. 51, 1218–1227. doi: 10.1111/1365-2664.12305
Durka, W., Michalski, S. G., Berendzen, K. W., Bossdorf, O., Bucharova, A., Hermann, J., et al. (2017). Genetic differentiation within multiple common grassland plants supports seed transfer zones for ecological restoration. J. Appl. Ecol. 54, 116–126. doi: 10.1111/1365-2664.12636
Elith, J., and Leathwick, J. R. (2009). Species distribution models: ecological explanation and prediction across space and time. Annu. Rev. Ecol. Evol. Syst. 40, 677–697. doi: 10.1146/annurev.ecolsys.110308.120159
Erwin, K. L. (2009). Wetlands and global climate change: the role of wetland restoration in a changing world. Wetlands Ecol. Manage. 17, 71–84. doi: 10.1007/s11273-008-9119-1
Geoscience Australia (2009). 1 second SRTM Level 2 Derived Digital Elevation Models (DEM-H supply) Version 1.0.
Gray, L. K., Gylander, T., Mbogga, M. S., Chen, P. Y., and Hamann, A. (2011). Assisted migration to address climate change: recommendations for aspen reforestation in western Canada. Ecol. Appl. 21, 1591–1603. doi: 10.1890/10-1054.1
Guisan, A., Tingley, R., Baumgartner, J. B., Naujokaitis-Lewis, I., Sutcliffe, P. R., Tulloch, A. I. T., et al. (2013). Predicting species distributions for conservation decisions. Ecol. Lett. 16, 1424–1435. doi: 10.1111/ele.12189
Hallgren, W., Beaumont, L., Bowness, A., Chambers, L., Graham, E., Holewa, H., et al. (2016). The Biodiversity and climate change virtual laboratory: where ecology meets big data. Environ. Model. Softw. 76, 182–186. doi: 10.1016/j.envsoft.2015.10.025
Hancock, N., Harris, R., Broadhurst, L., and Hughes, L. (2016). Climate-Ready Revegetation. A Guide for Natural Resource Managers. Sydney, NSW: Macquarie University Available online at: http://anpc.asn.au/resources/climate_ready_revegetation
Harrison, P. A., Vaillancourt, R. E., Harris, R. M. B., and Potts, B. M. (2017). Integrating climate change and habitat fragmentation to identify candidate seed sources for ecological restoration. Restor. Ecol. 25, 524–531. doi: 10.1111/rec.12488
Heikkinen, R. K., Luoto, M., Araújo, M. B., Virkkala, R., Thuiller, W., and Sykes, M. T. (2006). Methods and uncertainties in bioclimatic envelope modelling under climate change. Prog. Phys. Geogr. 30, 751–777. doi: 10.1177/0309133306071957
Hodgins, K. A., and Moore, J. L. (2016). Adapting to a warming world: ecological restoration, climate change, and genomics. Am. J. Bot. 103, 590–592. doi: 10.3732/ajb.1600049
Isaac-Renton, M. G., Roberts, D. R., Hamann, A., and Spiecker, H. (2014). Douglas-fir plantations in Europe: a retrospective test of assisted migration to address climate change. Glob. Chang. Biol. 20, 2607–2617. doi: 10.1111/gcb.12604
Keller, M., Kollmann, J., and Edwards, P. J. (2000). Genetic introgression from distant provenances reduces fitness in local weed populations. J. Appl. Ecol. 37, 647–659. doi: 10.1046/j.1365-2664.2000.00517.x
Kramer-Schadt, S., Niedballa, J., Pilgrim, J. D., Schröder, B., Lindenborn, J., Reinfelder, V., et al. (2013). The importance of correcting for sampling bias in MaxEnt species distribution models. Divers. Distrib. 19, 1366–1379. doi: 10.1111/ddi.12096
Lee-Yaw, J. A., Kharouba, H. M., Bontrager, M., Mahony, C., Csergő, A. M., Noreen, A. M. E., et al. (2016). A synthesis of transplant experiments and ecological niche models suggests that range limits are often niche limits. Ecol. Lett. 19, 710-722. doi: 10.1111/ele.12604
Leimu, R., and Fischer, M. (2008). A meta-analysis of local adaptation in plants. PLoS ONE 3:e4010. doi: 10.1371/journal.pone.0004010
Littell, J. S., McKenzie, D., Kerns, B. K., Cushman, S., and Shaw, C. G. (2011). Managing uncertainty in climate-driven ecological models to inform adaptation to climate change. Ecosphere 2, 1–19. doi: 10.1890/ES11-00114.1
Liu, C., Berry, P. M., Dawson, T. P., and Pearson, R. G. (2005). Selecting thresholds of occurrence in the prediction of species distributions. Ecography 28, 385–393. doi: 10.1111/j.0906-7590.2005.03957.x
Lunt, I. D., Byrne, M., Hellmann, J. J., Mitchell, N. J., Garnett, S. T., Hayward, M. W., et al. (2013). Using assisted colonisation to conserve biodiversity and restore ecosystem function under climate change. Biol. Conserv. 157, 172–177. doi: 10.1016/j.biocon.2012.08.034
Mátyás, C. (1996). Climatic adaptation of trees: rediscovering provenance tests. Euphytica 92, 45–54. doi: 10.1007/BF00022827
Mbogga, M. S., Wang, X., and Hamann, A. (2010). Bioclimate envelope model predictions for natural resource management: dealing with uncertainty. J. Appl. Ecol. 47, 731–740. doi: 10.1111/j.1365-2664.2010.01830.x
McLachlan, J. S., Hellmann, J. J., and Schwartz, M. W. (2007). A framework for debate of assisted migration in an era of climate change. Conserv. Biol. 21, 297–302. doi: 10.1111/j.1523-1739.2007.00676.x
McLane, S. C., and Aitken, S. N. (2011). Whitebark pine (Pinus albicaulis) assisted migration potential: testing establishment north of the species range. Ecol. Appl. 22, 142–153. doi: 10.1890/11-0329.1
McLean, E. H., Prober, S. M., Stock, W. D., Steane, D. A., Potts, B. M., Vaillancourt, R. E., et al. (2014). Plasticity of functional traits varies clinally along a rainfall gradient in Eucalyptus tricarpa. Plant Cell Environ. 37, 1440–1451. doi: 10.1111/pce.12251
Nilsson, C., Brown, R. L., Jansson, R., and Merritt, D. M. (2010). The role of hydrochory in structuring riparian and wetland vegetation. Biol. Rev. 85, 837–858. doi: 10.1111/j.1469-185X.2010.00129.x
Pemberton, R. W. (2010). Biotic resource needs of specialist orchid pollinators. Bot. Rev. 76, 275–292. doi: 10.1007/s12229-010-9047-7
Phillips, S. J., Anderson, R. P., and Schapire, R. E. (2006). Maximum entropy modeling of species geographic distributions. Ecol. Modell. 190, 231–259. doi: 10.1016/j.ecolmodel.2005.03.026
Phillips, S. J., and Dudík, M. (2008). Modeling of species distributions with maxent: new extensions and a comprehensive evaluation. Ecography 31, 161–175. doi: 10.1111/j.0906-7590.2008.5203.x
Prober, S. M., Byrne, M., McLean, E. H., Steane, D. A., Potts, B. M., Vaillancourt, R. E., et al. (2015). Climate-adjusted provenancing: a strategy for climate-resilient ecological restoration. Front. Ecol. Evol. 3:65. doi: 10.3389/fevo.2015.00065
Schwartz, M. W., Hoeksema, J. D., Gehring, C. A., Johnson, N. C., Klironomos, J. N., Abbott, L. K., et al. (2006). The promise and the potential consequences of the global transport of mycorrhizal fungal inoculum. Ecol. Lett. 9, 501–515. doi: 10.1111/j.1461-0248.2006.00910.x
Sgrò, C. M., Lowe, A. J., and Hoffmann, A. A. (2011). Building evolutionary resilience for conserving biodiversity under climate change. Evol. Appl. 4, 326–337. doi: 10.1111/j.1752-4571.2010.00157.x
Snover, A. K., Mantua, N. J., Littell, J. S., Alexander, M. A., McClure, M. M., and Nye, J. (2013). Choosing and using climate-change scenarios for ecological-impact assessments and conservation decisions. Conserv. Biol. 27, 1147–1157. doi: 10.1111/cobi.12163
Steane, D. A., McLean, E., Potts, B. M., Prober, S. M., Stock, W. D., Stylianou, V. M., et al. (2017a). Evidence for adaptation and acclimation in a widespread eucalypt of semi-arid Australia. Biol. J. Linn. Soc. 121, 484–500. doi: 10.1093/biolinnean/blw051
Steane, D. A., Potts, B. M., McLean, E., Collins, L., Holland, B. R., Prober, S. M., et al. (2017b). Genome scans across three eucalypts indicate that adaptation is a genome wide phenomenon. Genome Biol. Evol. 9, 253–265. doi: 10.1093/gbe/evw290
Steane, D. A., Potts, B. M., McLean, E., Prober, S. M., Stock, W. D., Vaillancourt, R. E., et al. (2014). Genome-wide scans detect adaptation to aridity in a widespread forest tree species. Mol. Ecol. 23, 2500–2513. doi: 10.1111/mec.12751
Stockwell, D. R. B., Beach, J. H., Stewart, A., Vorontsov, G., Vieglais, D., and Pereira, R. S. (2006). The use of the GARP genetic algorithm and Internet grid computing in the Lifemapper world atlas of species biodiversity. Ecol. Modell. 195, 139–145. doi: 10.1016/j.ecolmodel.2005.11.016
Tapper, S.-L., Byrne, M., Yates, C. J., Keppel, G., Hopper, S. D., Van Niel, K., et al. (2014). Prolonged isolation and persistence of a common endemic on granite outcrops in both mesic and semi-arid environments in south-western Australia. J. Biogeogr. 41, 2032–2044. doi: 10.1111/jbi.12343
Western Australian Department of Agriculture and Food (2011). Current Extent of Native Vegetation - Western Australia. Available online at: https://catalogue.data.wa.gov.au/dataset/native-vegetation-extent
Williams, A. V., Nevill, P. G., and Krauss, S. L. (2014). Next generation restoration genetics: applications and opportunities. Trends Plant Sci. 19, 529–537. doi: 10.1016/j.tplants.2014.03.011
Williams, B. K. (2011). Adaptive management of natural resources - framework and issues. J. Environ. Manage. 92, 1346–1353. doi: 10.1016/j.jenvman.2010.10.041
Williams, N., Stewart, A., and Papadopoulos, P. (2017). Virtualizing Lifemapper software infrastructure for biodiversity expedition. Concurrency Comput. Pract. Exp. 29:e4137. doi: 10.1002/cpe.4137
Yates, C. J., McNeill, A., Elith, J., and Midgley, G. F. (2010). Assessing the impacts of climate change and land transformation on Banksia in the South West Australian floristic region. Divers. Distrib. 16, 187–201. doi: 10.1111/j.1472-4642.2009.00623.x
Keywords: climate-adjusted provenancing, climate change adaptation, conservation planning, ecological restoration, seed provenance, seed sourcing, species distribution models
Citation: Ramalho CE, Byrne M and Yates CJ (2017) A Climate-Oriented Approach to Support Decision-Making for Seed Provenance in Ecological Restoration. Front. Ecol. Evol. 5:95. doi: 10.3389/fevo.2017.00095
Received: 25 April 2017; Accepted: 28 July 2017;
Published: 11 August 2017.
Edited by:
Marco A. Molina-Montenegro, University of Talca, ChileReviewed by:
Ian Sajid Acuña Rodriguez, Center for Advanced Studies in Arid Zones (CEAZA), ChileKaty Morgan, University of New Orleans, United States
Copyright © 2017 Ramalho, Byrne and Yates. This is an open-access article distributed under the terms of the Creative Commons Attribution License (CC BY). The use, distribution or reproduction in other forums is permitted, provided the original author(s) or licensor are credited and that the original publication in this journal is cited, in accordance with accepted academic practice. No use, distribution or reproduction is permitted which does not comply with these terms.
*Correspondence: Cristina E. Ramalho, Y3Jpc3RpbmEucmFtYWxob0B1d2EuZWR1LmF1