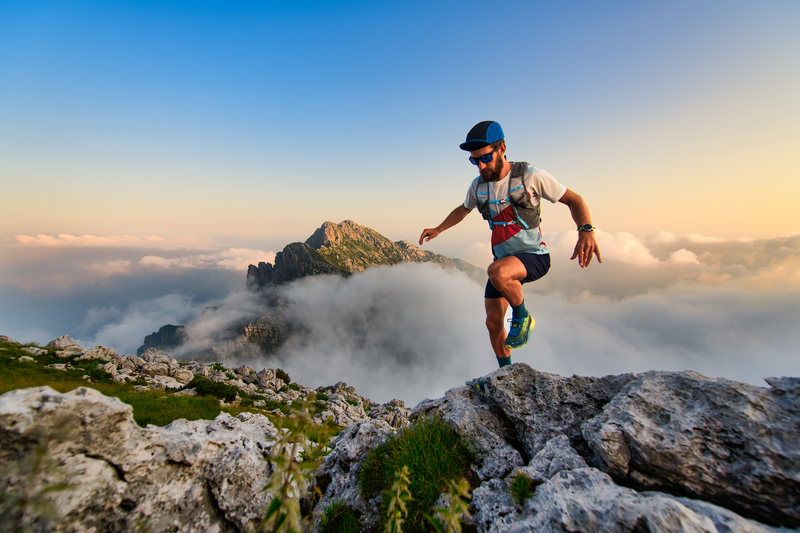
94% of researchers rate our articles as excellent or good
Learn more about the work of our research integrity team to safeguard the quality of each article we publish.
Find out more
REVIEW article
Front. Ecol. Evol. , 13 October 2016
Sec. Paleoecology
Volume 4 - 2016 | https://doi.org/10.3389/fevo.2016.00109
This article is part of the Research Topic Identifying Human Activity in Palaeoecological Records View all 7 articles
The amount of palaeoecological information available continues to grow rapidly, supporting improved descriptions of the dynamics of past ecosystems and enabling them to be seen from new perspectives. At the same time, there has been concern over whether palaeoecological enquiry needs to move beyond descriptive inference to a more hypothesis-focussed, or experimental approach. However, the extent to which conventional hypothesis-driven scientific frameworks can be applied to historical contexts (i.e., the past) is the subject of ongoing debate. In other disciplines concerned with human-environment interactions, including physical geography and archaeology, there has been growing use of generative simulation models, typified by agent-based approaches. Generative modeling encourages counter-factual questioning (“what if…?,”) a mode of argument that is particularly important in systems and time-periods, such as the Holocene, and now the Anthropocene, where the effects of humans and other biophysical processes are deeply intertwined. However, palaeoecologically focused simulation of the dynamics of the ecosystems of the past either seems to be conducted to assess the applicability of some model to the future or treats humans simplistically as external forcing factors. In this review we consider how generative simulation-modeling approaches could contribute to our understanding of past human-environment interactions. We consider two key issues: the need for null models for understanding past dynamics and the need to be able learn more from pattern-based analysis. In this light, we argue that there is considerable scope for palaeoecology to benefit from developments in generative models and their evaluation. We discuss the view that simulation is a form of experiment and by using case studies, consider how the many patterns available to palaeoecologists can support model evaluation in a way that moves beyond simplistic pattern-matching and how such models might also inform us about the data themselves and the processes generating them. Our emphasis is on how generative simulation might complement traditional palaeoecological methods and proxies rather than on a detailed overview of the modeling methods themselves.
Palaeoecologists are enjoying a data-rich era, with reconstructions using multiple proxies across large networks of sites now common, supported by advances in computational power, and informatics (Brewer et al., 2012). Large amounts of palaeoecological information, such as that stored in the NEOTOMA and the Global Charcoal databases, are available online, and can be interrogated using open-source software such as R (Blarquez et al., 2014; Goring et al., 2015). Likewise, the variety of proxies available to palaeoecologists has increased (Meadows, 2014), with, for example, ancient genomics providing new data and insights about the ecological dynamics of the ecosystems of the past (Orlando and Cooper, 2014; Hofman et al., 2015). The signatures of past changes and the processes generating them are assumed to be present in the spatial and temporal patterns embedded in these data and given the wealth of data available describing past ecosystems, palaeoecology is now awash, if not drowning, in “patterns” of all sorts. This wealth of data and patterns is allowing new avenues for palaeoecological research. For example, there is growing interest in the use of the information and knowledge gleaned from natural archives to inform understanding and management of contemporary ecosystem-service provisioning (Jeffers et al., 2015; Pearson et al., 2015).
Understanding the dynamics of feedback-driven ecological systems requires a pluralistic approach; in this pursuit the description of long-term ecosystem dynamics that underpins palaeoecology is a fundamental component, but is not sufficient of itself (Bowman et al., 2015). Models, and the intellectual practice of process-based modeling, have an important role to play in such efforts. Computational and data advances have allowed the development of detailed environmental models over increasingly finer and larger scales in space and time. Computer power is not, however, a panacea for the scaling and inferential challenges faced by (palaeo-) ecologists, nor does it negate the fundamental issues about representation that are central to all simulation. From the outset we acknowledge that models will always remain open to the criticism that they are incomplete, although as both Bryson et al. (2007) and Millington and Wainwright (2016) comment this incompleteness is true of all explanations and theories. Furthermore, purpose will remain the key determinant of how useful simulation might be in a given context and what form any such simulation should take; in short, not all questions require an explicit formal model, even if scientists are implicitly modeling all of the time. Alongside changes in computational power supporting more detailed representation, modelers have moved beyond seeing simulation models solely as predictive tools and now recognize their heuristic and exploratory value (Oreskes et al., 1994; Epstein, 2008). Importantly, there has been growing recognition that a simple confrontation of model predictions with observed data (so-called “pattern-matching”) is inadequate for model evaluation (O'sullivan and Perry, 2013). In response, environmental modelers have developed frameworks for making process-related inferences from complicated simulation models that go beyond simple pattern matching (single model vs. single dataset) and emphasize multiple hypothesis testing and the simultaneous evaluation of multiple model structures (McIntire and Fajardo, 2009; Grimm and Railsback, 2012). These frameworks can support the heuristic use of simulation models to explore palaeoecological questions, but to date there have been limited efforts to link these important developments in palaeoecological and human-environment models.
In this paper, we focus on how generative models can be used to strengthen the inferences made from palaeoecological data and the patterns embedded in them. We are concerned with the use of models to understand past human-environment interactions rather than the technical questions of how to develop a simulation model. Two recent reviews of modeling human-environment interactions in the Anthropocene help to fill this gap: Verburg et al. (2016) discuss, in general terms, the challenges of adequately representing human-environment interaction in coupled socio-ecological systems and Barton et al. (2016) describe in some detail the design and implementation of the MedLand Modeling Laboratory. Thus, we do not provide an exhaustive overview of the application of simulation models to palaeoecological questions (in fact the field is large enough that this is probably impossible in a single review); rather, we seek to highlight how recent advances in the computational tools available to ecological modelers can support better inference making from (simulation) models. In particular, we consider the view that models represent an alternative mode of experiment (Dowling, 1999; O'sullivan, 2004; Peck, 2004); this is a particularly relevant argument for historical sciences such as palaeoecology where direct manipulation of the system is impossible. We focus on how new frameworks for model selection and evaluation offer powerful frameworks within which in silico experimentation might be grounded and suggest that palaeoecological records provide an ideal test-bed for the application of these tools. Generative simulations models, including agent-based approaches, can be used to explore prehistoric human-environment interactions in ways that are currently under-explored; such approaches have been under-used to explore palaeoecological questions.
Many typologies have been proposed for ecological models, including some based on the underlying techniques used (e.g., mathematical vs. empirical vs. simulation) and others on the motivation behind the modeling exercise (e.g., prediction vs. heurism; Perry and Millington, 2008). Gerbault et al. (2014) distinguish between “discriminative” and “generative” simulation models; the former focus on finding patterns in data without explicit consideration of causality, and the latter with developing model-based representations of the underlying processes generating the patterns and structures that we observe (“story testing,” sensu Gerbault et al., 2014). Epstein (1999, 2006, 2008) has advocated for a generative approach in modeling social systems, using agent-based models (ABMs) to evaluate how complex social systems may be built up of, and evolve within, a set of relatively simple rules. This generative approach is important because interpretations of Holocene palaeoecological data must necessarily consider whether the signal has been perturbed, or is even dominated, by human action. In such contexts, models are tools designed to represent, and simplify more complicated or complex ecological systems and thus support surrogative reasoning (O'sullivan and Perry, 2013). Surrogative reasoning implies a feedback between model and understanding, with failure to close the reasoning loop resulting in “merely replicating field data in silico” (Premo, 2007, p. 30). Thus, models are not, at least in this context, of interest simply of themselves, but have value to the extent that they inform us about the system or phenomenon of interest. Lake (2015) argues that to be successful, experimental generative modeling needs to be grounded in theory (so moving primacy away from the data required for parameterization), and, by design, adopt an exploratory approach to model evaluation.
Generative modeling relies on disaggregated, process-based models in which the overall structure emerges from the activities of and interactions between individual elements of interest. Agent-based models (ABMs) typify this approach and have begun to be used across a broad range of the natural and social sciences (Heppenstall et al., 2012; Railsback and Grimm, 2012; Wurzer et al., 2015). In the ABM framework, the dynamics of systems are represented by considering the basic entities (the “agent”) and evaluating how interactions between these agents and their environment result in system-level (macroscopic) structure; in other words, it is “bottom-up.” In such models “agents” are entities that seek to fulfill some goal (e.g., capture resources, breed) and have some level of autonomy (that is their behavior is not hard-coded and may vary between individual agents). While agents may be individual organisms, they might equally represent households, wider family groups, settlements, or even entire tribes. Simulation models developed by ecologists to explore past human-environment interactions tend to have taken a rather different approach in which human agency is not directly represented but is instead mimicked by changes in parameterization (e.g., increased fire frequency or browsing) with the biophysical environment represented in detail (as per the case-studies described below). The flexible representation and emergent behavior possible with ABMs is especially important given that feedbacks between humans and ecosystems are reciprocal rather than uni-directional (Wainwright and Millington, 2010; Bowman et al., 2015). This point highlights the main weakness of a static representation of human-environment interactions, in that it fails to capture their reciprocal nature: as human action changes the landscapes they inhabit, so do human behaviors change in order to adapt to the new conditions (Wainwright, 2008).
Except for a few specific cases (Griffith et al., 2010), however, ABMs seem to have received little attention in ecologically focussed reconstructions of human-environment interactions. Conversely, the use of ABMs by archaeologists is growing (Cegielski and Rogers, 2016), and the most iconic prehistoric human-environment ABM—the Artifical Anasazi model—was developed by archaeological researchers (Axtell et al., 2002)1; in such models human decision-making is represented in detail but the biophysical environment is often less so. This difference in approach probably reflects the underlying differences in the foci and intellectual traditions of different disciplines2. Ultimately, understanding how humans and environments interact in the past is likely to require an explicit representation of human agency.
Dowling (1999, p. 261) makes it clear that the use of simulation models is, both epistemically and practically, a form of experiment:
A scientist running a computer simulation performs an experiment upon a theory. An abstract, mathematical model of a physical system is implemented on a concrete machine. Through that machine, the model can be manipulated as if it were a physical experimental target. The mathematical model can then be approached and analyzed using skills traditionally associated with experimental work: visual observation, “tinkering” with the machine, and intuition about the behavior of the concrete system.
This view of “simulation as experiment” is appealing for the historical sciences (sensu Cleland, 2001) because in such cases adopting the classical hypothetico-deductive scientific framework is infeasible (Biondi, 2014). Direct manipulation of the past is impossible, and the data describing the ecosystems of the past are usually spatio-temporally patchy and provide only indirect representations of the processes of interest. As a result, palaeoecology has relied heavily on pattern identification and diagnosis, but there is a limit to the inferences that can be made from pattern description alone (Birks, 1993; McIntire and Fajardo, 2009). A first concern with inference grounded in patterns is in the nature of the patterns themselves. For example, Blaauw (2012) highlights the risk of circularity in the diagnosis of pattern, especially in cases where multiple proxies are matched, or tuned against each other based on the assumption that events seen in them are synchronous. The problem posed by equifinality—that is, the same pattern can arise from many different processes—places a limit on the strength with which inferences about generating processes can be made from spatial or temporal patterns alone (Beven, 2006). A classic example of this problem in the palaeoecological literature is the long-standing debate over the mid-Holocene decline of Ulmus in northern Europe (Parker et al., 2002). Because this decline occurred around the time of the Mesolithic-Neolithic transition and associated agricultural expansion it is plausible that human activity played a role; on the other hand it is also plausible that a pathogen or regional drought or some combination of all three were responsible. Analysis of patterns alone cannot, of itself, distinguish between these causal explanations.
Generative simulation models provide tools for experimenting on the past and for testing hypotheses and counter-factual arguments (“how might the system have responded if…?,” Millington and Wainwright, 2016). As McIntire and Fajardo (2009) argue, making robust statements about the dynamics of systems to which we have only restricted access (in space and time) requires ecologists focussed on pattern analysis to adopt a more deductive framework. This argument is echoed in Lake's (2015) observation that to be successful generative modeling needs to be grounded in an experimental approach. Despite the appeal of a generative modeling approach to make more of palaeoecological data describing human-environment interaction, the approach seems under-used; instead, one of the main uses of palaeoecological information (such as pollen and charcoal records) by ecological modelers has been to “validate” their models (Birks, 1993; Anderson et al., 2006; Iglesias et al., 2015). Ultimately, these validations are used to justify, via induction, a model's extension to assessing the future. However, how much process-pattern links in the past will apply in a potentially “no-analogue” future is unclear, and hence using phenomenological representations of the past to predict the future is fraught with problems (Williams and Jackson, 2007; Haywood et al., 2011; Gustafson, 2013). This type of validation is also fraught where the types of circularity discussed by Blaauw (2012) may be present; if a model is built “knowing” what the interpretation of the palaeoecological data should be (albeit perhaps only implicitly), it is not surprising that validation via model-data confrontation is successful (echoing the concern of Premo, 2007, that modeling can reduce to the simple reproduction of field data). Finally, as Anderson et al. (2006) note, this validation-focussed approach is uni-directional in that the data inform the model but not the other way around; such a narrow application restricts what might be learned both from the data and the model.
At the heart of classical experimentation is the idea that the effect of process x in some system can be identified by manipulating it and holding all others constant. Thus, quantifying the effect of x requires a control that serves as a point of reference. This type of approach is problematic for natural systems (Diamond, 1983) and is effectively impossible for past ones (Cleland, 2001). However, developing simulations in which processes of interest are deliberately excluded provides a valuable null model that can act, in some ways, as a “control” (Lake, 2015). In their horizon-scan of 50 pressing questions for palaeoecologists, Seddon et al. (2014) identify both the need for a more experimental approach (their Q 49) and a closer consideration of the use of null models (their Q 25) as important. Although Seddon et al. (2014) emphasize statistical models in supporting those advances, the experimental use of simulation models can play an important role in both.
As an example of how simulation models can support the development of null models, consider the question “how much can fluctuations in proxy records be attributed to exogenous drivers as opposed to statistical variability?” or to turn this around, “what would proxy records look like if they were just stochastic time-series?.” Blaauw et al. (2010) show that patterns visually similar to those in “real” proxy records can arise from random walk processes (Figure 1). That a process-free algorithm can generate patterns difficult to distinguish from proxy records again evokes the perils of equifinality. Likewise, both Rhode et al. (2014) and Davies et al. (2016) show how changes in the temporal distribution of dated (e.g., 14C) records, which are often assumed to represent patterns in human occupation of the landscape (similar to those observed in the field), can emerge in the absence of any underlying change in human demography or behavior. A second context where neutral models are useful is in understanding the generation of landscape-level vegetation patterns. Succession-disturbance dynamics are affected by the spatial structure (composition and configuration of elements) of the landscapes in which they occur (Turner, 2010). Therefore, when developing representations of palaeoecological processes, it is not necessarily sufficient to consider just the composition of a landscape as established from pollen records; often the spatial pattern must also be examined. Understanding the implications of changes in landscape configuration is particularly important when trying to identify human activity, as prehistoric humans dynamically changed the processes shaping the landscape mosaic, and this change in landscape pattern alone may result in changes to ecological processes (Delcourt, 1987). The dynamic nature of landscape change is crucial and is a potential source of equifinality as, for example, the same outcome may not occur for the same change because of internal and external dynamic interactions. As we argued above, such multifaceted links between pattern-process are unlikely to be adequately captured in static representations of human-environment interactions. Detailed methods do exist to reconstruct landscape composition and structure from pollen records (e.g., the LRA, Sugita, 2007a,b; Sugita et al., 2010), but these are data-demanding, require extensive calibration against modern data and are taxa- and site-specific. A neutral landscape model (NLM) approach, in which a wide variety of landscape patterns are simulated but with the same statistical characteristics, can be used to test the potential influence of landscape pattern on past ecological processes (Etherington et al., 2015). Importantly for palaeoecological applications, NLMs can be constructed such that the known proportional composition of a landscape in a pollen catchment can be embedded within a broader unknown landscape pattern to examine the possible influence of patterns in the wider landscape (Figure 2). The Multiple Scenario Assessment (MSA) approach described by Bunting and Middleton (2009) is somewhat similar in that it starts with observed pollen records and then generates multiple candidate simulations of the landscape structure that might have produced them. Running repeated simulations on landscapes of the same composition, but with different spatial configurations, allows an experimental assessment of the importance of both initial conditions (enabling contingency and sensitivity issues to be evaluated) and space in ecosystem dynamics. Thus, using neutral models can provide a frame of reference for detailed palaeoecological records (a point emphasized by Barton et al., 2016), and with careful in silico experimentation partition the contribution of different drivers to observed dynamics.
Figure 1. Sample fossil pollen and exogenous forcing factor records generated with Gaussian and Poisson random walks. Although these null records show some of the visual hallmarks of “real” proxy records [e.g., long-term shifts (proxy record O) and short-term spikes (proxy record D) in dominance] they are entirely process-free. Figure generated using R code provided in Blaauw et al. (2010).
Figure 2. Examples of neutral landscape models of prehistoric landscapes for a hypothetical pollen record indicating four landscape categories. (A,B) Different realizations of naturalistic landscapes in which landscape categories are an ordered sequence resulting from a natural environmental gradient. (C,D) Different realizations of human-influenced landscapes in which the original naturalistic gradient patterns have been modified by discrete patches representing localized human disturbance. In all cases the landscape category proportions within the hypothetical pollen catchment area (dotted line) are equally divided amongst the four categories, while the landscape proportions beyond the pollen catchment area vary individually as part of a broader but consistent spatial pattern to represent uncertainty about landscape patterns beyond the pollen catchment area.
The inferences made using any model will depend on its adequacy, which is a context-dependent quality. Most methods designed to assess model adequacy rely on the “confrontation” of a given model with some (independent) data (Beck, 1987; Mayer and Butler, 1993; Hilborn and Mangel, 1997; Mulligan and Wainwright, 2004). Putting to one side the fact that models are false by definition, Oreskes et al. (1994) argued that models cannot be verified (i.e., found “true”)3 simply by pattern matching; even if a model manages to perfectly (or even adequately) mimic some target data-set, other parameterizations, or models may perform equally well (i.e., there is a problem of under-determination). A second, but related, problem with model-data confrontation is that it tends to emphasize parameter uncertainty in a fixed model structure, whereas, in reality structural (epistemic) uncertainty (i.e., the way in which specific processes are represented in a model) is likely to be as acute, if not more so.
Partly in reaction to their concern over the ad hoc nature of the development of complex simulation models, Grimm et al. (2005) and Grimm and Railsback (2012) outline a framework that they term pattern-oriented modeling (POM). POM is based on the view that the patterns observed in complex systems (strictly, in the data describing them) are the fingerprints of the processes that generated them. In terms of model evaluation, these patterns act as filters that can be used to assess if a model is adequate in its parameterisation and/or its structure (Figure 3). Using multiple patterns is a key facet of POM; it is more difficult for a model to agree with multiple weak patterns than with a single strong one. Thus, for a model to be deemed adequate it will need to be able to reproduce a number of observed patterns. The POM approach is not concerned with isolating a single “true” model; rather it seeks to identify the set of models that have sufficient structural realism and adequate parameterization to meet specific targets. There are two compelling arguments for the use of a POM approach for palaeoecological data and models. First, as described above, a wealth of patterns describing (socio-) ecological systems of the past are now available, and, second, using multiple patterns to evaluate models is crucial in settings where the likelihood of either equifinality or trajectory divergence (i.e., the same parameter set generating a broad range of outcomes) is high, as it is in historical settings reconstructed via proxy data (Stiner, 2008; Bunting and Middleton, 2009; Janssen, 2009; Gerbault et al., 2014). Thrippleton et al. (2014) provide an example of the use of a POM framework to inform the parameterization of a dynamic vegetation model (LANDCLIM) that was used to explore successional change following the Taupō eruption of c. 232 CE (North Island of New Zealand). Horrocks and Ogden (1998) described two important patterns in the post-eruption succession: (1) conifer dominance in the period immediately after the eruption (in particular by Libocedrus bidwillii) and (2) a subsequent spread of Weinmannia racemosa in montane areas. These patterns were framed as quantitative criteria and a full parameter-space sweep conducted for two highly uncertain but critical life-history parameters—maximum growth rate and shade-tolerance—with only those parameterizations that met the two criteria retained. The parameterized model could reproduce a series of patterns seen in the pollen records and in the modern vegetation (e.g., vegetation composition and elevational zonation). If a model that has passed a POM assessment then generates previously unobserved patterns those can stimulate further empirical investigation and hypothesis testing (Wiegand et al., 2003; Grimm et al., 2005). An important challenge in the application of POM for palaeoecological models is that the state variables of models are not expressed in units similar to those of the proxies being used. For example, vegetation models may predict biomass or species abundance, but pollen records are expressed in concentrations that may or may not be easily converted to biomass or abundance. Developing palaeoecological models that produce virtual natural archives (see Barton et al., 2016) will be important if tools such as POM are to be more effectively used. Alongside the development of virtual records, ongoing advances in our ability to link proxy information to the underlying mechanisms generating it (e.g., Higuera et al., 2007; Dawson et al., 2016 provide examples with fossil pollen and charcoal, respectively) will also help to strength the inferences derived from a POM approach.
Figure 3. The pattern-oriented modeling (POM) framework (Grimm et al., 2005; Grimm and Railsback, 2012) is designed to help modelers implement models that contain sufficient structural detail and are adequately parameterised given the question being addressed. This evaluation is achieved by comparing a suite of model structures (different shapes in figure) and parameterisations (different colors in figure) and assessing them against a set of target patterns (the filters). POM does not seek to find the single “best” model; rather it inherently recognises that there may be a suite of adequate models (lower group of colored shapes) with different structures and parameterisations.
A key challenge in applying POM is in deciding what for any given model “adequate” actually means. Tools developed by statisticians to assess model adequacy, for example arising from multi-model inference (Burnham and Anderson, 2002), are now being applied to ecological simulation models (Hartig et al., 2011). Such tools facilitate a rigorous, robust and repeatable “tinkering with the machine” to use Dowling's (1999) phrase. For example, Approximate Bayesian Computation (ABC: Beaumont, 2010; ABC, Csilléry et al., 2010; Stumpf, 2014), which has been used to parameterize and select between population genomic models (e.g., Fagundes et al., 2007 use ABC to select between different models of human origin and migration from Africa), is beginning to be applied to complex ecological simulations (Morales et al., 2015; van der Vaart et al., 2015). In essence, ABC involves having some form of target data (a pattern, or more usually a suite of summary statistics describing multiple patterns) and then running many simulations with parameters sampled from broad uninformative (“prior”) distributions and model structure varied. Those simulations that are sufficiently close to the targets are retained and provide an updated (“posterior”) estimate of the parameters included in the model and also an indication as to the weight of support for alternative model structures (e.g., via Baye's factors, Beaumont, 2010). The simplest ABC estimation method is a reject-accept algorithm in which some threshold distance between model and observation is set and only those simulations within that tolerance retained or alternatively, the model is run until some pre-determined number of simulations fall within that threshold (see Figure 4). However, other more sophisticated approaches, such as sequential Monte Carlo filters in which the parameter space is searched in a biased way to focus on more informative parts of it, are likely more efficient for complicated simulation models (Stumpf, 2014). Again, the wealth of patterns available to palaeoecologists—coupled with the increasing accessibility and availability of high-performance computational infrastructure—makes ABC-type approaches relevant to model-based exploration of human-environment interactions in the past. The ability to filter different model structures is crucial given the critique that ABMs are prone to being overly complex, making it difficult to identify the processes and parameters that drive them and hence communicate their outcomes effectively (Lee et al., 2015).
Figure 4. A simple example of the ABC reject-accept approach. In this example, the “target” pattern is a population trajectory (A) arising from a discrete-time logistic population model with stochasticity in the growth term r. There are four parameters we wish to estimate (μ[r], σ[r], K, n0); to do so we simulate the population model 1 × 106 times, each time drawing values for the four unknown parameters from a broad uniform distribution (the “prior”; gray). For each simulation, we assess how close the trajectory is to the target using the summed squared difference across the entire series (red) and the Euclidean distance (blue) between 10 irregularly spaced observation points. We retain the 100 simulations closest to the observed pattern and from these estimate posterior distributions for each of the parameters (B–E). Vertical green lines are the true parameter values.
Much of the discussion above could be related to nearly all ecological and environmental contexts. So, how do these arguments and approaches apply to the simulation of the dynamics of human-environment interactions in past environmental systems? Reconstructing environments from proxy information such as fossil pollen and charcoal requires a robust understanding of how those records are formed: where does the pollen preserved at a given site come from? from which taxa? what is the relative contribution of the local vs. the regional species pool? what is the relative importance of extrinsic (top-down) and intrinsic (bottom-up) forcing factors? And in the context of understanding how humans affected the processes described by these proxies questions of agency and social structure become central. In this section, we consider, how generative simulation modeling can inform our understanding of such questions, especially as they relate to human agency and decision-making. We do not review the methods themselves in depth—they have been thoroughly described elsewhere (Epstein, 2006; Heppenstall et al., 2012; O'sullivan and Perry, 2013; Wurzer et al., 2015)—rather our focus is on the types of inferences made from models in each of these examples.
In the context of understanding human-environment interactions, an obvious question is whether human activity was necessary to generate some observed pattern of interest. Because the presence of humans and their activities are often reconstructed indirectly (e.g., from abrupt changes in ecological conditions, or from changes in the distribution of specific materials/dates) a more specific question is “how likely are such patterns in the absence of humans?” Evaluating this question is not possible without explicit recourse to a model of some form, and as Barton et al. (2016 p. 38) comment “…the ability to conduct such contrafactual ecological dynamics (i.e., a Holocene world without humans) is a little discussed but uniquely important contribution of this kind of modeling that is impossible with the analysis of prehistoric empirical data alone.” Null simulation models provide a powerful way to evaluate such questions; a good example of this approach is provided by the random walk models of pollen records and associated forcing factors of Blaauw et al. (2010) described earlier (Figure 1). Likewise, Brantingham (2003) showed how an agent-based model with minimal (zero) representation of human agency and environmental structure can generate plausible patterns of lithic assemblages. In the specific context of human-environment interactions the “behaviourally neutral” model of Davies et al. (2016), which represents the formation and preservation of surface archaeological deposits (e.g., fire-pits and hearths) in arid Australia, is informative. In these landscapes the temporal density of surface deposits varies and this could be interpreted as evidence for changes in human presence/activity; in particular, the records exhibit occasional long gaps and an increase in density toward the present. Davies et al. (2016) used an agent-based model to evaluate how such records might be produced in the absence of human agency (the agents leave surface deposits at a constant rate and with no spatial structure). This simulation experiment, therefore, provides a null expectation against which to evaluate empirical data. The outcome of the experiment was to demonstrate that time-varying geomorphic processes reveal and preserve deposits and so, of themselves, generate spatial and temporal patterns. This model-derived outcome suggests that even though human activity was important in the landscapes that Davies et al. (2016) considered, and its intensity varied through time and space, directly linking this to the available patterns is not straightforward. This result does not mean that humans had no role in generating the observed pattern, but it does suggest that the a priori assumption that they are solely responsible for this pattern is questionable (as, for example, demonstrated by Wainwright (1994) in the case of post-depositional movement of artifacts at archaeological sites).
If human activity is established as an important driver of ecosystem change, then understanding the implications of their behavior for systems dynamics becomes central. As an aside, an interesting issue in this context is whether the appropriate null for human decision-making is the “zero intelligence agent” or the entirely rational and informed “Homo œconomicus” of classical economics (Bentley and Ormerod, 2012); most neutral models of human-environment interaction developed by non-economists have favored the former. Soon after human arrival in NZ in the late Thirteenth century CE (Wilmshurst et al., 2008) widespread deforestation took place as a result of anthropic fire. However, the motivation behind this event remains unclear, and cannot be elicited from palaeoecological information alone. Using a spatial simulation model, which incorporated successional change, fire and feedbacks between fire and vegetation age, Perry et al. (2012) showed that in the absence of human fire, the transformation was extremely unlikely (a null model of no humans) and would not have occurred if human ignitions were spatio-temporally random (a null model of uninformed ignition). However, their model experiments also suggest that fire-vegetation feedbacks made the transformation almost inevitable once started, suggesting that such dramatic changes might not have been intended even if anthropic fire was deliberate. Of course, the ability of these models to reproduce a suite of patterns does not “prove” that this is how these transformations unfolded, but it does generate a range of hypotheses amenable to experimental testing (e.g., testing whether the postulated fire-vegetation feedback mechanisms inherent in this explanation do exist). Furthermore, the model Perry et al. (2012) used is phenomenological rather than mechanistic, and so it is important to develop a process-based understanding of the underlying feedbacks if these dynamics are to be confirmed; neither proxies nor phenomenological models can generate such causal understanding. Developing simple representations of human behavior and agency is a powerful way of “generating inferences about how the world could have been, rather than about how the world is” (Premo, 2006, p. 108). The key point here is that neutral models and hypotheses can guide our understanding of what to expect if specific behaviors potentially responsible for generating observed patterns and trajectories are omitted from a model.
Crema et al. (2014) used a rejection-tolerance ABC approach to parameterize and select between three different models of cultural transmission as preserved in the archaeological record. In the apparent absence of the use of ABC to evaluate simulation models of past human-environment interactions this study provides a useful, and somewhat related example of the strengths of the approach. The specific context considered by Crema et al. (2014) is the temporal change in arrowhead form during the Neolithic (data from western Europe). Crema et al. consider three candidate models and their parameterization: (1) a model of unbiased transmission; (2) a model of conformist bias; and (3) a model of anti-conformist bias. The first of these three is a null model in that it assumes the probability of a variant being adopted is proportional to its current abundance; the other two models are biased either in favor of more (2) or less (3) widely used variants. The empirical data provide a target pattern, which is the dissimilarity in assemblage form between two successive periods. While the archaeological details are not relevant here, what is important is the ABC approach that Crema et al. (2014) adopt was able to parameterize the models adequately, but could not isolate a single “best” model, with both the unbiased and conformist model equally plausible. While this may seem inferentially unsatisfactory, it does quantify the risk of equifinality in the data in a way that an a priori assumption of the “best” model structure cannot4. The approach of Crema et al. (2014) is clearly applicable to a wide variety of palaeoecological settings where proxy records provide a range of summary statistics to inform the approach. The availability of multiple proxies is particularly useful for ABC because it provides potentially somewhat independent filters for the algorithm.
A common use of simulation models is to explore counterfactual (“what if…?”) scenarios, and there has been some use of this approach in understanding past human-environment interactions (Wainwright and Millington, 2010). Here we consider two contrasting examples: (i) the use of a dynamic vegetation model (LANDCLIM) supported by palaeoecological proxy data to explore the effects of land-use change and fire on vegetation in ecosystems in western Europe (Colombaroli et al., 2010; Henne et al., 2013) and (ii) the use of an agent-based model of landscape change (CybErosion) that directly represent human decision-making, as well as geomorphic and ecological processes (Wainwright, 2015). Our emphasis is not on a detailed description of the outcomes of these experiments per se, but rather on the way in which they were used and the types of inference developed from them.
Colombaroli et al. (2010) and Henne et al. (2013) used the LANDCLIM model to explore how changes in vegetation at Gouillé Rion (Swiss Alps) and Lago di Massacciucoli (Tuscany), over the Holocene might relate to shifts in climate and changes in human activity. The LANDCLIM model is a detailed representation of vegetation dynamics (succession and multiple disturbance types) at high spatial resolution (25 × 25 m); the model is described in detail by Schumacher et al. (2004). Interactions between disturbance and climate are dynamic and emerge from the model, but it does not directly include human behavior; rather Colombaroli et al. (2010), and Henne et al. (2013) mimic human actions by changes in parameterization (e.g., increased in fire frequency at given times). Colombaroli et al. (2010) and Henne et al. (2013) used model scenarios, supported by temperature reconstructions, to evaluate how the patterns seen in detailed multi-proxy palaeoecological records (pollen, plant remains, charcoal) might have arisen. For example, Henne et al. (2013) systematically explored the effects of browsing and fire by simulating three levels of each (nine experimental treatments in total). Both studies strongly suggest that the temporal shifts in vegetation seen in the proxy records are only likely to have occurred under increased human land activity. Wainwright (2015) used an agent-based model (CybErosion) that directly represents interactions between Mesolithic hunter-gatherers and Neolithic agriculturalists and their environment, including processes such as livestock husbandry and browse, fire, and erosion and the feedbacks between them in a semi-mechanistic way. Using this model, Wainwright (2015) explored three different scenarios in which human pressure on the landscape varied from low environmental pressure/low invasion rate/extensive agricultural production to high pressure/high invasion rate/intensive agricultural production. An important outcome of these experiments was that changes in landscape connectivity can result in periods of stability and instability [the stability-instability-connectivity (SIC) model] without such transitions being directly represented (i.e., it is “emergent,”) but the trajectories seen in the different scenarios suggest that these SIC dynamics can take a variety of forms.
While bearing in mind that these two case-studies come from different disciplinary perspectives (palaeoecology vs. geoarchaeology), it is informative to compare how they use in silico experiment to make inferences about past human-environment interactions. Colombaroli et al. (2010) and Henne et al. (2013) start with detailed palaeoecological reconstructions of two specific sites and their associated taxa, and seek to use the model to identify the mechanisms that may have generated the patterns observed in those records. Although they invoke human activity in the form of changes in fire regime and browsing, they do not directly represent it—rather humans are treated as “boundary conditions” with parameterization changed accordingly (e.g., fire frequency increased 10-fold to represent increasing human intensity in the landscape). This approach yields a detailed, and partially mechanistic, understanding of biophysical change in a specific landscape. On the other hand, Wainwright (2015) starts with the general observation that there are periods of both landscape stability and instability during the Neolithic in western Mediterranean Europe, and asks how they arise. He explores this question with an agent-based model (ABM) that explicitly represents human decision-making and biophysical change and evaluates the implications of a suite of assumptions, framed as scenarios describing different rates of human movement and agricultural intensity (Figure 5). While Wainwright (2015) does not do so, the types of virtual archive produced by process-oriented ABMs could be evaluated against proxy records such as fossil pollen (the caveats described earlier notwithstanding). This style of modeling demonstrates how feedbacks between humans and the environment can generate a rich range of dynamics (in this case by altering the nature of connectivity in the landscape), but it does not focus on a specific site or suite of taxa. It is important to emphasize that neither approach to modeling is inherently “better”—the usefulness of an approach depends on the purpose of the modeling activity—but, on the other hand, modelers cannot have it both ways; there will always be trade-offs between precision, realism and generality (Levins, 1966).
Figure 5. Output from the CybErosion model showing how vegetation and erosion emerge under three scenarios (A–C) about the nature of human-environment interactions, increasing in intensity and rapidity from left to right. Each grid cell is 100 × 100 m in size. Colours in the vegetation maps are as follows: light, medium, and dark green are grass, shrub and forest, respectively; blue areas are in active cultivation, and brown areas were formerly cultivated and are now bare. In the erosion maps (middle row), rates are scaled from high (white) through medium (brown) to low (black). Column (D) shows the temporal dynamics of the forest cover and erosion in the landscape (top and middle) and the relationship between landscape-level biomass and average net erosion (bottom). Figure from Wainwright (2015). Reproduced with the kind permission of John Wiley & Sons.
Increasing computational power and data availability are rapidly changing the way that simulation is practiced in the natural and social sciences (Lazer et al., 2009; Sellars et al., 2013; Gattiglia, 2015). However, technological increases will not solve all of the challenges associated with representation and scale with which ecologists struggle. In the specific area of modeling prehistoric human-environment interactions, we briefly consider two areas ripe for development from an ecological perspective: (i) the use of ABMs and (ii) improvements in the ways that model outcomes are communicated and interpreted.
We are not arguing that an ABM approach is the best option for all questions, and whether they will “make revolutionary advances within the overall archaeological research paradigm” as some have argued (Cegielski and Rogers, 2016, p. 284) remains to be seen. O'sullivan et al. (2012, p. 120) argue that there are three conditions where ABMs are likely to be useful: (i) the environment is heterogeneous in space and time, (ii) the agents interact in the decision-making process and (iii) the system is middle-numbered (that is too many elements to be open to purely deterministic analysis but too few for the laws of statistical physics to apply, Weaver, 1948). While these conditions may well be true of many settings where human-environment interactions are being represented, they are not universal. “Fast and frugal” models (Carpenter, 2003) still have an important role to play in (initial) explorations of system behavior (e.g., see Holdaway and Jacomb, 2000; Perry et al., 2014 in the context of hunting pressure required to drive moa to extinction). ABMs can also, but do not have to, be data-hungry and require extensive parameterisation and testing (especially if arguments about system properties such as “emergence” are to be made); for example the simplified version of the CybErosion ABM used by Wainwright (2015) still requires 35 parameters to be estimated (see his Table 5.2). In such cases, the POM approach supported by computational methods such as ABC have important roles to play. As with all areas of ecology the appropriate complexity (i.e., number of parameters and processes included) of a model is very much a function of the purpose of the modeling exercise (Levins, 1966; Evans et al., 2013). A final, important, question is whether the growing use of ABMs among those concerned with the ecological and social systems of the past will generate robust and testable theory or will simply generate a proliferation of empirically detailed but idiosyncratic models (a concern expressed by Grimm, 1999; O'sullivan et al., 2016).
A recurrent critique of palaeoecology has been its reliance on “story-telling” rather than “stronger” types of inference (Biondi, 2014) made in other areas of the natural sciences. There has been a long debate over the virtues, or otherwise, of how the historical sciences construct knowledge and this is beyond the scope of our review (but see, Cleland, 2001, 2011). However, it is becoming apparent that generative simulation models offer much more than shallow systems descriptions derived from quantitative syntheses of the data they produce (Winsberg, 2010); for example, there is growing interest in the view that simulation models are themselves narrative devices and their outcomes can be communicated in that way (Millington et al., 2012; McGlade, 2014). The use of simulation models in the context of understanding past human-environment interactions has the potential to mediate between the desire for strong and robust inferences and the historical nature of the data palaeoecologists use to make such inferences. Using models to develop “thick” descriptions (Millington and Wainwright, 2016) could take the form of narrative, or it could take the form of sophisticated visualization of the landscapes of the past (Caseldine et al., 2008; Edwards et al., 2015). Narrative explanations will require generative models that adequately capture feedbacks between social and ecological components of systems across multiple spatio-temporal scales (Verburg et al., 2016).
The “grand challenges” that palaeoecology and archaeology are engaged with (Biondi, 2014; Kintigh et al., 2014; Seddon et al., 2014) do not simply require more and bigger data, but also new ways to use and synthesize it. However, while simulation modeling has an important role to play in their resolution, this needs to be more than simply as a consumer of data for validation. As we have argued, generative models offer the ability for theory to inform empirical data and also a way to “experiment with theory” (Dowling, 1999). As with any informative experiment, the use of simulations as such should provide new insights and provoke new questions.
GP led the writing of the manuscript; all authors made substantial contributions to the development of the ideas presented here and commented critically on drafts of the manuscript.
The authors declare that the research was conducted in the absence of any commercial or financial relationships that could be construed as a potential conflict of interest.
This work was supported by Core Funding for Crown Research Institutes from the New Zealand Ministry of Business, Innovation and Employment's Science and Innovation Group. TE and GP were supported by the University of Auckland Faculty Research Development Fund 3702237. GP is grateful for the support of ARC grant DP140103591 and a University of Tasmania Visiting Scholar fellowship while this paper was written. JW acknowledges the support provided by a University of Auckland Foundation Distinguished Visitor Award. The ideas presented here have benefitted from discussions over the last few years with David O'sullivan, James Millington and Volker Grimm.
1. ^Available at: https://www.openabm.org/model/2222/version/2/view
2. ^It is worth noting that ecologists have used individual-based models (IBMs) since the 1960s, especially in the area of forest dynamics. The differences between IBM and ABM are largely semantic and reflect disciplinary traditions; both approaches have the same underlying bottom-up approach.
3. ^It is worth noting that Oreskes et al. use a natural language definition of verified that is distinct from what the term is usually taken to mean in a computer-science framework.
4. ^Although this outcome may also arise from Crema et al. using just a single summary statistic (i.e. pattern), rather than the multiple targets inherent in POM (Grimm and Railsback, 2012) and advocated in the technical ABC literature (Rasmussen and Hamilton, 2012).
Anderson, N. J., Bugmann, H., Dearing, J. A., and Gaillard, M.-J. (2006). Linking palaeoenvironmental data and models to understand the past and to predict the future. Trends Ecol. Evol. 21, 696–704. doi: 10.1016/j.tree.2006.09.005
Axtell, R. L., Epstein, J. M., Dean, J. S., Gumerman, G. J., and Swedlung, A. C. (2002). Population growth and collapse in a multiagent model of the Kayenta Anasazi in Long House Valley. Proc. Natl. Acad. Sci. U.S.A. 99, 7275–7279. doi: 10.1073/pnas.092080799
Barton, C. M., Ullah, I. I. T., Bergin, S. M., Sarjoughian, H. S., Mayer, G. R., Bernabeu-Auban, J. E., et al. (2016). Experimental socioecology: integrative science for anthropocene landscape dynamics. Anthropocene 13, 34–45. doi: 10.1016/j.ancene.2015.12.004
Beaumont, M. A. (2010). Approximate Bayesian computation in evolution and ecology. Annu. Rev. Ecol. Evol. Syst. 41, 379–406. doi: 10.1146/annurev-ecolsys-102209-144621
Beck, M. B. (1987). Water quality modeling: a review of the analysis of uncertainty. Water Resour. Res. 23, 1393–1442. doi: 10.1029/WR023i008p01393
Bentley, A., and Ormerod, P. (2012). “Agents, intelligence, and social atoms,” in Creating Consilience: Reconciling Science and the Humanities, eds M. Collard and E. Slingerland (Oxford: Oxford University Press), 205–222.
Beven, K. (2006). A manifesto for the equifinality hypothesis. J. Hydrol. 320, 18–36. doi: 10.1016/j.jhydrol.2005.07.007
Biondi, F. (2014). Paleoecology - grand challenge. Front. Ecol. Evol. 2:50. doi: 10.3389/fevo.2014.00050
Birks, H. J. B. (1993). Quaternary palaeoecology and vegetation science— current contributions and possible future developments. Rev. Palaeobot. Palynol. 79, 153–177. doi: 10.1016/0034-6667(93)90045-V
Blaauw, M. (2012). Out of tune: the dangers of aligning proxy archives. Quat. Sci. Rev. 36, 38–49. doi: 10.1016/j.quascirev.2010.11.012
Blaauw, M., Bennett, K. D., and Christen, J. A. (2010). Random walk simulations of fossil proxy data. Holocene 20, 645–649. doi: 10.1177/0959683609355180
Blarquez, O., Vannière, B., Marlon, J. R., Daniau, A.-L., Power, M. J., Brewer, S., et al. (2014). Paleofire: an R package to analyse sedimentary charcoal records from the global charcoal database to reconstruct past biomass burning. Comput. Geosci. 72, 255–261. doi: 10.1016/j.cageo.2014.07.020
Bowman, D. M. J. S., Perry, G. L. W., and Marston, J. B. (2015). Feedbacks and landscape-level vegetation dynamics. Trends Ecol. Evol. 30, 255–260. doi: 10.1016/j.tree.2015.03.005
Brantingham, P. J. (2003). A neutral model of stone raw material procurement. Am. Antiq. 68, 487–509.
Brewer, S., Jackson, S. T., and Williams, J. W. (2012). Paleoecoinformatics: applying geohistorical data to ecological questions. Trends Ecol. Evol. 27, 104–112. doi: 10.1016/j.tree.2011.09.009
Bryson, J. J., Ando, Y., and Lehmann, H. (2007). Agent-based modelling as scientific method: a case study analysing primate social behaviour. Philos. Trans. R. Soc. B 362, 1685–1699. doi: 10.1098/rstb.2007.2061
Bunting, M. J., and Middleton, R. (2009). Equifinality and uncertainty in the interpretation of pollen data: the multiple scenario approach to reconstruction of past vegetation mosaics. Holocene 19, 799–803. doi: 10.1177/0959683609105304
Burnham, K. P., and Anderson, D. R. (2002). Model Selection and Multimodel Inference: A Practical Information-Theoretic Approach, 2nd Edn. New York, NY: Springer.
Carpenter, S. R. (2003). “The need for fast-and-frugal models,” in Models in Ecosystem Science, eds C. D. Canham, J. J. Cole, and W. K. Lauenroth (Princeton, NJ: Princeton University Press), 455–460.
Caseldine, C., Fyfe, R., and Hjelle, K. (2008). Pollen modelling, palaeoecology and archaeology: virtualisation and/or visualisation of the past? Veg. Hist. Archaeobot. 17, 543–549. doi: 10.1007/s00334-007-0093-y
Cegielski, W. H., and Rogers, J. D. (2016). Rethinking the role of agent-based modeling in archaeology. J. Anthropolog. Archaeol. 41, 283–298. doi: 10.1016/j.jaa.2016.01.009
Cleland, C. E. (2001). Historical science, experimental science, and the scientific method. Geology 29, 987–990. doi: 10.1130/0091-7613(2001)029<0987:hsesat>2.0.co;2
Cleland, C. E. (2011). Prediction and explanation in historical natural science. Br. J. Philos. Sci. 62, 551–582. doi: 10.1093/bjps/axq024
Colombaroli, D., Henne, P. D., Kaltenrieder, P., Gobet, E., and Tinner, W. (2010). Species responses to fire, climate and human impact at tree line in the Alps as evidenced by palaeo-environmental records and a dynamic simulation model. J. Ecol. 98, 1346–1357. doi: 10.1111/j.1365-2745.2010.01723.x
Crema, E. R., Edinborough, K., Kerig, T., and Shennan, S. J. (2014). An approximate Bayesian computation approach for inferring patterns of cultural evolutionary change. J. Archaeol. Sci. 50, 160–170. doi: 10.1016/j.jas.2014.07.014
Csilléry, K., Blum, M. G. B., Gaggiotti, O. E., and Olivier François, O. (2010). Approximate Bayesian Computation (ABC) in practice. Trends Ecol. Evol. 25, 410–418. doi: 10.1016/j.tree.2010.04.00
Davies, B., Holdaway, S. J., and Fanning, P. C. (2016). Modelling the palimpsest: an exploratory agent-based model of surface archaeological deposit formation in a fluvial arid Australian landscape. Holocene 26, 450–463. doi: 10.1177/0959683615609754
Dawson, A., Paciorek, C. J., McLachlan, J. S., Goring, S., Williams, J. W., and Jackson, S. T. (2016). Quantifying pollen-vegetation relationships to reconstruct ancient forests using 19th-century forest composition and pollen data. Quat. Sci. Rev. 137, 156–175. doi: 10.1016/j.quascirev.2016.01.012
Delcourt, H. R. (1987). The impact of prehistoric agriculture and land occupation on natural vegetation. Trends Ecol. Evol. 2, 39–44. doi: 10.1016/0169-5347(87)90097-8
Edwards, K. J., Fyfe, R. M., Hunt, C. O., and Schofield, J. E. (2015). Moving forwards? Palynology and the human dimension. J. Archaeolog. Sci. 56, 117–132. doi: 10.1016/j.jas.2015.02.010
Epstein, J. M. (1999). Agent-based computational models and generative social science. Complexity 4, 41–60.
Epstein, J. M. (2006). Generative Social Science: Studies in Agent-Based Computational Modeling. Princeton, NJ: Princeton University Press.
Etherington, T. R., Holland, E. P., and O'sullivan, D. (2015). NLMpy: a PYTHON software package for the creation of neutral landscape models within a general numerical framework. Methods Ecol. Evol. 6, 164–168. doi: 10.1111/2041-210X.12308
Evans, M. R., Grimm, V., Johst, K., Knuuttila, T., de Langhe, R., Lessells, C. M., et al. (2013). Do simple models lead to generality in ecology? Trends Ecol. Evol. 28, 578–583. doi: 10.1016/j.tree.2013.05.022
Fagundes, N. J. R., Ray, N., Beaumont, M., Neuenschwander, S., Salzano, F. M., Bonatto, S. L., et al. (2007). Statistical evaluation of alternative models of human evolution. Proc. Natl. Acad. Sci. U.S.A. 104, 17614–17619. doi: 10.1073/pnas.0708280104
Gattiglia, G. (2015). Think big about data: archaeology and the big data challenge. Arch. Inf. 38, 113–124. doi: 10.11588/ai.2015.1.26155
Gerbault, P., Allaby, R. G., Boivin, N., Rudzinski, A., Grimaldi, I. M., Pires, J. C., et al. (2014). Storytelling and story testing in domestication. Proc. Natl. Acad. Sci. U.S.A. 111, 6159–6164. doi: 10.1073/pnas.1400425111
Goring, S., Dawson, A., Simpson, G. L., Ram, K., Graham, R. W., Grimm, E. C., et al. (2015). Neotoma: a programmatic interface to the neotoma paleoecological database. Open Q. 1. doi: 10.5334/oq.ab
Griffith, C. S., Long, B. L., and Sept, J. M. (2010). HOMINIDS: an agent-based spatial simulation model to evaluate behavioral patterns of early Pleistocene hominids. Ecol. Modell. 221, 738–760. doi: 10.1016/j.ecolmodel.2009.11.009
Grimm, V. (1999). Ten years of individual-based modelling in ecology: what have we learned and what could we learn in the future? Ecol. Modell. 115, 129–148.
Grimm, V., and Railsback, S. F. (2012). Pattern-oriented modelling: a “multi-scope” for predictive systems ecology. Philos. Trans. R. Soc. B 367, 298–310. doi: 10.1098/rstb.2011.0180
Grimm, V., Revilla, E., Berger, U., Jeltsch, F., Mooij, W. M., Railsback, S. F., et al. (2005). Pattern-oriented modeling of agent-based complex systems: lessons from ecology. Science 310, 987–991. doi: 10.1126/science.1116681
Gustafson, E. J. (2013). When relationships estimated in the past cannot be used to predict the future: using mechanistic models to predict landscape ecological dynamics in a changing world. Landsc. Ecol. 28, 1429–1437. doi: 10.1007/s10980-013-9927-4
Hartig, F., Calabrese, J. M., Reineking, B., Wiegand, T., and Huth, A. (2011). Statistical inference for stochastic simulation models – theory and application. Ecol. Lett. 14, 816–827. doi: 10.1111/j.1461-0248.2011.01640.x
Haywood, A. M., Ridgwell, A., Lunt, D. J., Hill, D. J., Pound, M. J., Dowsett, H. J., et al. (2011). Are there pre-Quaternary geological analogues for a future greenhouse warming? Philos. Trans. R. Soc. A 369, 933–956. doi: 10.1098/rsta.2010.0317
Henne, P., Elkin, C., Colombaroli, D., Samartin, S., Bugmann, H., Heiri, O., et al. (2013). Impacts of changing climate and land use on vegetation dynamics in a Mediterranean ecosystem: insights from paleoecology and dynamic modeling. Landsc. Ecol. 28, 819–833. doi: 10.1007/s10980-012-9782-8
Heppenstall, A. J., Crooks, A. T., See, L. M., and Batty, M. (eds.). (2012). Agent-Based Models of Geographical Systems. Berlin: Springer.
Higuera, P., Peters, M., Brubaker, L., and Gavin, D. (2007). Understanding the origin and analysis of sediment-charcoal records with a simulation model. Quat. Sci. Rev. 26, 1790–1809. doi: 10.1016/j.quascirev.2007.03.010
Hilborn, R., and Mangel, M. (1997). The Ecological Detective: Confronting Models with Data. Princeton, NJ: Princeton University Press.
Hofman, C. A., Rick, T. C., Fleischer, R. C., and Maldonado, J. E. (2015). Conservation archaeogenomics: ancient DNA and biodiversity in the Anthropocene. Trends Ecol. Evol. 30, 540–549. doi: 10.1016/j.tree.2015.06.008
Holdaway, R. N., and Jacomb, C. (2000). Rapid extinction of the Moas (Aves: Dinornithiformes): model, test, and implications. Science 287, 2250–2254. doi: 10.1126/science.287.5461.2250
Horrocks, M., and Ogden, J. (1998). The effects of the Taupo tephra eruption of c. 1718 BP on the vegetation of Mt Hauhungatahi, Central North Island, New Zealand. J. Biogeogr. 25, 649–660.
Iglesias, V., Yospin, G. I., and Whitlock, C. (2015). Reconstruction of fire regimes through integrated paleoecological proxy data and ecological modeling. Front. Plant Sci. 5:785. doi: 10.3389/fpls.2014.00785
Jeffers, E. S., Nogué, S., and Willis, K. J. (2015). The role of palaeoecological records in assessing ecosystem services. Quat. Sci. Rev. 112, 17–32. doi: 10.1016/j.quascirev.2014.12.018
Kintigh, K., Altschul, J., Beaudry, M., Drennan, R., Kinzig, A., Kohler, T., et al. (2014). Grand challenges for archaeology. Am. Antiq. 79, 5–24. doi: 10.7183/0002-7316.79.1.5
Lake, M. W. (2015). “Explaining the past with ABM: on modelling philosophy,” in Agent-Based Modeling and Simulation in Archaeology, eds G. Wurzer, K. Kowarik, and H. Reschreiter (Cham: Springer International Publishing), 3–35.
Lazer, D., Pentland, A., Adamic, L., Aral, S., Barabási, A.-L., Brewer, D., et al. (2009). Computational social science. Science 323, 721–723. doi: 10.1126/science.1167742
Lee, J.-S., Filatova, T., Ligmann-Zielinska, A., Hassani-Mahmooei, B., Stonedahl, F., Lorscheid, I., et al. (2015). The complexities of agent-based modeling output analysis. J. Artif. Soc. Soc. Simulat. 18:4. doi: 10.18564/jasss.2897
McGlade, J. (2014). Simulation as narrative: contingency, dialogics, and the modeling conundrum. J. Archaeol. Method Theory 21, 288–305. doi: 10.1007/s10816-014-9201-3
McIntire, E. J. B., and Fajardo, A. (2009). Beyond description: the active and effective way to infer processes from spatial patterns. Ecology 90, 46–56. doi: 10.1890/07-2096.1
Meadows, M. E. (2014). Recent methodological advances in Quaternary palaeoecological proxies. Prog. Phys. Geogr. 38, 807–817. doi: 10.1177/0309133314540690
Millington, J. D. A., O'sullivan, D., and Perry, G. L. W. (2012). Model histories: narrative explanation in generative simulation modelling. Geoforum 43, 1025–1034. doi: 10.1016/j.geoforum.2012.06.017
Millington, J. D. A., and Wainwright, J. (2016). Mixed qualitative-simulation methods: understanding geography through thick and thin. Prog. Hum. Geogr. doi: 10.1177/0309132515627021. [Epub ahead of print].
Morales, J. M., Mermoz, M., Gowda, J. H., and Kitzberger, T. (2015). A stochastic fire spread model for north Patagonia based on fire occurrence maps. Ecol. Modell. 300, 73–80. doi: 10.1016/j.ecolmodel.2015.01.004
Mulligan, M., and Wainwright, J. (2004). “Modelling and model building,” in Environmental Modelling: Finding Simplicity in Complexity, eds J. Wainwright and M. Mulligan (London, UK: John Wiley and Sons), 7–68.
Oreskes, N., Shrader-Frechette, K., and Belitz, K. (1994). Verification, validation, and confirmation of numerical models in the earth sciences. Science 263, 641–646.
Orlando, L., and Cooper, A. (2014). Using ancient DNA to understand evolutionary and ecological processes. Annu. Rev. Ecol. Evol. Syst. 45, 573–598. doi: 10.1146/annurev-ecolsys-120213-091712
O'sullivan, D. (2004). Complexity science and human geography. Trans. Inst. Br. Geogr. 29, 282–295. doi: 10.1111/j.0020-2754.2004.00321.x
O'sullivan, D., Evans, T., Manson, S., Metcalf, S., Ligmann-Zielinska, A., and Bone, C. (2016). Strategic directions for agent-based modeling: avoiding the YAAWN syndrome. J. Land Use Sci. 11, 177–187. doi: 10.1080/1747423X.2015.1030463
O'sullivan, D., Millington, J. D. A., Perry, G. L. W., and Wainwright, J. (2012). “Agent-based models – because they're worth it?,” in Agent-Based Models for Geographical Systems, eds A. Heppenstall, A. Crooks, L. M. See, and M. Batty (New York, NY: Springer), 109–123.
O'sullivan, D., and Perry, G. L. W. (2013). Spatial Simulation: Exploring Pattern and Process. Chichester: John Wiley & Sons.
Parker, A. G., Goudie, A. S., Anderson, D. E., Robinson, M. A., and Bonsall, C. (2002). A review of the mid-Holocene elm decline in the British Isles. Prog. Phys. Geogr. 26, 1–45. doi: 10.1191/0309133302pp323ra
Pearson, S., Lynch, A. J. J., Plant, R., Cork, S., Taffs, K., Dodson, J., et al. (2015). Increasing the understanding and use of natural archives of ecosystem services, resilience and thresholds to improve policy, science and practice. Holocene 25, 366–378. doi: 10.1177/0959683614558650
Peck, S. L. (2004). Simulation as experiment: a philosophical reassessment for biological modeling. Trends Ecol. Evol. 19, 530–534. doi: 10.1016/j.tree.2004.07.019
Perry, G. L. W., and Millington, J. D. A. (2008). Spatial modelling of succession-disturbance dynamics in forest ecosystems: concepts and examples. Perspect. Plant Ecol. Evol. Syst. 9, 191–210. doi: 10.1016/j.ppees.2007.07.001
Perry, G. L. W., Wheeler, A. B., Wood, J. R., and Wilmshurst, J. M. (2014). A high-precision chronology for the rapid extinction of New Zealand moa (Aves, Dinornithiformes). Quat. Sci. Rev. 105, 126–135. doi: 10.1016/j.quascirev.2014.09.025
Perry, G. L. W., Wilmshurst, J. M., McGlone, M. S., McWethy, D. B., and Whitlock, C. (2012). Explaining fire-driven landscape transformation during the initial burning period of New Zealand's prehistory. Glob. Chang. Biol. 18, 1609–1621. doi: 10.1111/j.1365-2486.2011.02631.x
Premo, L. S. (2006). Agent-based models as behavioral laboratories for evolutionary anthropological research. Ariz. Anthropol. 17, 91–113.
Premo, L. S. (2007). “Exploratory agent-based models: towards an experimental ethnoarchaeology,” in Digital Discovery: Exploring New Frontiers in Human Heritage (CAA 2006), eds J. T. Clark, E. M. Clark, and E. M. Hagemeister (Budapest: Archeolingua Press), 29–36.
Railsback, S. F., and Grimm, V. (2012). Agent-Based and Individual-Based Modeling: A Practical Introduction. Princeton, NJ: Princeton University Press.
Rasmussen, R., and Hamilton, G. (2012). An approximate Bayesian computation approach for estimating parameters of complex environmental processes in a cellular automata. Environ. Modell. Softw. 29, 1–10. doi: 10.1016/j.envsoft.2011.10.005
Rhode, D., Brantingham, P. J., Perreault, C., and Madsen, D. B. (2014). Mind the gaps: testing for hiatuses in regional radiocarbon date sequences. J. Archaeol. Sci. 52, 567–577. doi: 10.1016/j.jas.2014.02.022
Schumacher, S., Bugmann, H., and Mladenoff, D. J. (2004). Improving the formulation of tree growth and succession in a spatially explicit landscape model. Ecol. Modell. 180, 175–194. doi: 10.1016/j.ecolmodel.2003.12.055
Seddon, A. W. R., Mackay, A. W., Baker, A. G., Birks, H. J. B., Breman, E., Buck, C. E., et al. (2014). Looking forward through the past: identification of 50 priority research questions in palaeoecology. J. Ecol. 102, 256–267. doi: 10.1111/1365-2745.12195
Sellars, S., Nguyen, P., Chu, W., Gao, X., Hsu, K., and Sorooshian, S. (2013). Computational earth science: big data transformed into insight. Eos. Trans. Am. Geophys. Union 94, 277–278. doi: 10.1002/2013EO320001
Stiner, M. C. (2008). “Taphonomy,” in Encyclopedia of Archaeology, ed D. M. Pearsall (New York, NY: Academic Press), 2113–2119.
Stumpf, M. P. H. (2014). Approximate Bayesian inference for complex ecosystems. F1000Prime Rep. 6:60. doi: 10.12703/P6-60
Sugita, S. (2007a). Theory of quantitative reconstruction of vegetation I: pollen from large sites REVEALS regional vegetation composition. Holocene 17, 229–241. doi: 10.1177/0959683607075837
Sugita, S. (2007b). Theory of quantitative reconstruction of vegetation II: all you need is LOVE. Holocene 17, 243–257. doi: 10.1177/0959683607075838
Sugita, S., Parshall, T., Calcote, R., and Walker, K. (2010). Testing the landscape reconstruction algorithm for spatially explicit reconstruction of vegetation in northern Michigan and Wisconsin. Quat. Res. 74, 289–300. doi: 10.1016/j.yqres.2010.07.008
Thrippleton, T., Dolos, K., Perry, G. L. W., Groeneveld, J., and Reineking, B. (2014). Simulating long-term vegetation dynamics using a forest landscape model: the post-Taupo succession on Mt Hauhungatahi, North Island, New Zealand. N.Z. J. Ecol. 38, 26–38.
Turner, M. G. (2010). Disturbance and landscape dynamics in a changing world. Ecology 91, 2833–2849. doi: 10.1890/10-0097.1
van der Vaart, E., Beaumont, M. A., Johnston, A. S. A., and Sibly, R. M. (2015). Calibration and evaluation of individual-based models using Approximate Bayesian Computation. Ecol. Modell. 312, 182–190. doi: 10.1016/j.ecolmodel.2015.05.020
Verburg, P. H., Dearing, J. A., Dyke, J. G., Leeuw, S., van der Seitzinger, S., Steffen, W., et al. (2016). Methods and approaches to modelling the Anthropocene. Glob. Environ. Change 39, 328–340. doi: 10.1016/j.gloenvcha.2015.08.007
Wainwright J. (1994). Erosion of archaeological sites: results and implications of a site simulation model. Geoarchaeology 9, 173–201.
Wainwright, J. (2008). Can modelling enable us to understand the rôle of humans in landscape evolution? Geoforum 39, 659–674. doi: 10.1016/j.geoforum.2006.09.011
Wainwright, J. (2015). “Stability and instability in Mediterranean landscapes: A geoarchaeological perspective,” in Monitoring and Modelling Dynamic Environments, eds A. P. Dykes, M. Mulligan, and J. Wainwright (Chichester: John Wiley & Sons, Ltd.), 99–120. doi: 10.1002/9781118649596.ch5
Wainwright, J., and Millington, J. D. A. (2010). Mind, the gap in landscape-evolution modelling. Earth Surf. Proc. Landforms 35, 842–855. doi: 10.1002/esp.2008
Wiegand, T., Jeltsch, F., Hanski, I., and Grimm, V. (2003). Using pattern-oriented modeling for revealing hidden information: a key for reconciling ecological theory and application. Oikos 100, 209–222. doi: 10.1034/j.1600-0706.2003.12027.x
Williams, J. W., and Jackson, S. T. (2007). Novel climates, no-analog communities, and ecological surprises. Front. Ecol. Environ. 5, 475–482. doi: 10.1890/1540-9295(2007)5[475:NCNCAE]2.0.CO;2
Wilmshurst, J. M., Anderson, A. J., Higham, T. G. F., and Worthy, T. H. (2008). Dating the late prehistoric dispersal of Polynesians to New Zealand using the commensal Pacific rat. Proc. Natl. Acad. Sci. U.S.A. 105, 7676–7680. doi: 10.1073/pnas.0801507105
Winsberg, E. (2010). Science in the Age of Computer Simulation. Chicago, IL: Chicago University Press.
Wurzer, G., Kowarik, K., and Reschreiter, H. (eds.). (2015). Agent-Based Modeling and Simulation in Archaeology. Cham: Springer International Publishing. Available online at: http://link.springer.com/10.1007/978-3-319-00008-4 (Accessed May 9, 2016).
Keywords: agent-based models, pattern-oriented modeling, generative simulation models, equifinality, inference
Citation: Perry GLW, Wainwright J, Etherington TR and Wilmshurst JM (2016) Experimental Simulation: Using Generative Modeling and Palaeoecological Data to Understand Human-Environment Interactions. Front. Ecol. Evol. 4:109. doi: 10.3389/fevo.2016.00109
Received: 30 May 2016; Accepted: 24 August 2016;
Published: 13 October 2016.
Edited by:
Simon Haberle, Australian National University, AustraliaReviewed by:
Juan Carlos Berrio, University of Leicester, UKCopyright © 2016 Perry, Wainwright, Etherington and Wilmshurst. This is an open-access article distributed under the terms of the Creative Commons Attribution License (CC BY). The use, distribution or reproduction in other forums is permitted, provided the original author(s) or licensor are credited and that the original publication in this journal is cited, in accordance with accepted academic practice. No use, distribution or reproduction is permitted which does not comply with these terms.
*Correspondence: George L. W. Perry, Z2VvcmdlLnBlcnJ5QGF1Y2tsYW5kLmFjLm56
Disclaimer: All claims expressed in this article are solely those of the authors and do not necessarily represent those of their affiliated organizations, or those of the publisher, the editors and the reviewers. Any product that may be evaluated in this article or claim that may be made by its manufacturer is not guaranteed or endorsed by the publisher.
Research integrity at Frontiers
Learn more about the work of our research integrity team to safeguard the quality of each article we publish.