- 1Land and Water Division, Commonwealth for Scientific and Industrial Research Organisation, Canberra, ACT, Australia
- 2Department of BioSciences, University of Melbourne, Melbourne, VIC, Australia
Overview: The Convergence of “Discrete” Disciplines
Biogeography and macroecology were, until recently, treated as separate disciplines with discrete aims and identities (e.g., Blackburn and Gaston, 2002; Wiens and Donoghue, 2004). This was largely based on the fact that biogeography is considered as the science of documenting and understanding spatial patterns of biodiversity, which tends to have an historical focus, while macroecology, in studying relationships between/among organisms and their environment, is generally considered to address contemporary questions (McIntosh, 1986; Blackburn and Gaston, 2002; Lomolino et al., 2010). But there has been a growing awareness that the two disciplines can, and do, converge, particularly at intermediate spatial and temporal scales (e.g., Ricklefs, 2007; Cavender-Bares et al., 2009; Jenkins and Ricklefs, 2011; Ricklefs and Jenkins, 2011).
With this convergence, it has become apparent that multidisciplinary studies can be instrumental in providing a deeper understanding of pertinent evolutionary questions (Saslis-Lagoudakis and Greve, 2011; Villalobos and Paknia, 2011). For example, past changes in environmental conditions (e.g., climate, plate tectonics), and the phylogenetic history of organisms, are two traditional components of biogeographic study, but these both also feed into explanations for current biotic patterns of distribution and abundance, i.e., macroecology (Jablonski, 2008; Wiens et al., 2010). Some authors have gone so far as to argue that important contemporary topics in macroecology can only be understood when historical and evolutionary (biogeographic) effects are considered (Ricklefs, 2004; Wiens and Donoghue, 2004). This commentary will discuss integration of macroecology and biogeography from the perspective of using intraspecific processes to inform interspecific patterns. In particular, I will argue that population genetics/genomics data can provide the glue to bond the two fields together to better inform macro-evolutionary research.
Species or Populations; Top-down or Bottom-up?
Macroecology and biogeography are, at heart, species-focused. Each uses analysis of species to explain patterns relating to distributions, diversity gradients (Adler et al., 2005; Hawkins et al., 2007), range size distributions (McPherson et al., 2004; Svenning and Skov, 2004; Beck et al., 2006; Morin and Chuine, 2006), and phylogenetic relationships (Winter et al., 2009; Cadotte et al., 2010; Capellini et al., 2010; Wiens et al., 2010). This essentially relates to the use of a top-down approach, whereby insights into complex systems are gained by breaking them down into their various sub-components (Friedman, 1974; Salmon, 1984). Bottom-up science, on the other hand, involves merging the sub-components together to build a complex, system-level understanding (Friedman, 1974; Salmon, 1984). The two approaches are often considered to be mutually exclusive, and yet, like the historical and ahistorical divisions of biogeography and macroecology, they essentially exist along a continuum of interpretation.
In this age of massive data gathering, particularly with respect to next-generation sequencing (NGS) of genetic data, there lies an opportunity for exploiting bottom-up approaches to address top-down questions, and thereby doing away entirely with unhelpful divisions among scientific disciplines. Indeed, the incorporation of molecular phylogenetic data into biogeographic approaches has informed knowledge about species distribution patterns and dispersal routes (Roncal et al., 2011; Saslis-Lagoudakis and Greve, 2011; Hernández et al., 2013), and important biogeographic insights have been gained by combining population genetic and landscape (i.e., phylogeographic) data with ecological niche modeling (e.g., Alvarado-Serrano and Knowles, 2013). Moreover, recent work has pushed for the unification of ecology and macroevolution (e.g., Rominger et al., 2015; Rosindell et al., 2015), and for the derivation of ecological inferences from population and/or community-level diversity (e.g., Pauls et al., 2014; Vellend et al., 2014). However, these approaches generally represent the use of species-level (i.e., phylogenetic) data in biogeography, and of population- or species-level data into macroecology. Thus far, little attention has focused on incorporating intraspecific (population-level) data into studies that include both historical (i.e., biogeography) and contemporary (i.e., macroecology) components (but see below). Such an approach would drive an understanding of evolutionary processes acting over both contemporary and historical scales across taxa into a systems-level understanding of patterns observed in complex systems (Figure 1).
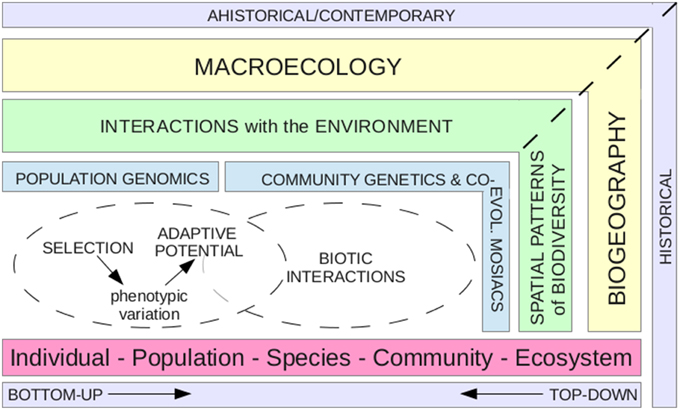
Figure 1. Population genomic data are relevant to systems-level insights. Historically, biogeography and macroecology have been divided on the basis that the former tries to understand spatial patterns of biodiversity, which tends to have an historical focus, while the latter, in studying relationships between organisms and their environment, is generally considered to address contemporary questions. This graphic promotes the idea of incorporating population genomic processes (e.g., selection acting on phenotypic variation to promote adaptive potential), with community genetics and co-evolutionary mosaics (i.e., interactions among populations and their communities, and biotic interactions among populations across regions) into biogeographic and macroecological research. This integrated viewpoint would allow bottom-up approaches to inform top-down questions, by feeding an understanding of processes within populations into an explanation for patterns observed at the system-level.
Population Genomic Data are Relevant to Systems-level Insights
Although biogeography and macroecology generally focus on understanding patterns at the meta-scale, incorporation of population-level data is necessary if these patterns are to inform us about how species have responded to environments in the past and enable us to predict how they will respond in the future (Passy, 2008; Hendriks et al., 2009). Recent modeling work has begun to take individual-based approaches toward testing large-scale patterns (e.g., Zurell et al., 2009; Buchmann et al., 2011; Fordham et al., 2014; Rosindell et al., 2015), even including processes such as adaptive potential into models of species distribution (Catullo et al., 2015). Others have argued for the incorporation of macroecological mechanisms, such as dispersal limitation, in biogeographic predictions (Gotelli et al., 2009). On the other hand, traditionally intraspecific landscape genomic studies are beginning to take comparative, multi-species approaches in recognition of the fact that general landscape effects on local adaptation and gene flow cannot be obtained from single-species studies (Thomassen et al., 2011; Manel and Holderegger, 2013). In the following paragraphs, I present three case studies that exemplify the ways in which population-level data can be used to facilitate understanding of macroecological and biogeographic patterns.
Hjalmarsson et al. (2015) used a comparative population genetic and phylogeographic approach to address a biogeographic and macroecological hypothesis that lentic (still water) species have higher dispersal rates that lotic (running water) species, as a result of the more ephemeral nature of the lentic habitat. These authors compared population genetic structure of 59 species of aquatic beetles from Madagascar, finding that lentic species have lower genetic structure (i.e., disperse more). The suggestion that lotic species are poorer dispersers has important implications for conservation of freshwater fauna as ephemeral habitats become increasingly isolated in the face of climate change (Hjalmarsson et al., 2015). By bringing a population focus to a macroecological question, this work demonstrates the usefulness of comparative intra-specific data in addressing macroevolutionary and biogeographical hypotheses.
Dexter et al. (2012) took a population genetics approach to look at alternative explanations for a community-level pattern of species compositions in the Amazon rainforest. Specifically, these authors identified clear genetic breaks within species of trees in the Inga genus that suggested population divergence had occurred tens to hundreds of thousands of years ago. Such a time scale effectively replaced a hypothesis of species sorting along an environmental gradient with one in which highly diverged species had come back into secondary contact (Dexter et al., 2012). By simultaneously studying biogeographic species patterns and population genetic data, these authors gained novel insights into the processes structuring patterns of spatial diversity. Other studies combining genetic with biogeographic and/or macroecological data have made similar advances in inferring the processes underlying spatial diversity (e.g., Bonada et al., 2009; Thiel-Egenter et al., 2011; Eldon et al., 2013).
Finally, Branco et al. (2015) studied coastal and mountain populations of the Californian fungus, Suillus brevipes, using NGS to reveal unprecedented cryptic speciation and recent divergence of the isolated populations. Just 0.01% of single nucleotide polymorphisms (SNPs) were fixed between the two populations; despite this low divergence, phenotypic variation had begun to characterize the two populations, with a gene potentially involved in enhanced salt tolerance in acidic conditions fixed in the coastal, but not the mountain, populations (Branco et al., 2015). This suggests that a selection-based hypothesis might explain the biogeography and macroecology of the two fungal populations, thus demonstrating how comparative population genomic approaches can yield insight into larger scale diversification and ecological processes. Correlating genomic data with ecological variables is a powerful approach toward detecting the genes underlying adaptation across populations and subspecies (e.g., Cox et al., 2011; Hancock et al., 2011; Jones et al., 2012; Bergland et al., 2014). By identifying the genetic drivers of speciation and increasing understanding of which ecological factors are relevant for adaptation, population genomics can inform about what will become the biogeographic and macroecological patterns of the future.
Future Considerations
In a very general sense, it seems obvious that interactions at the level of phenotype and environment within and between species (i.e., microevolution), are prevalent drivers of distribution and abundance gradients that should be considered in biogeography and macroecology (Figure 1). But, how might a bottom-up approach (i.e., microevolution) explicitly inform these two fields in practice? In addition to the examples above, where population genetics or genomics data, have been applied to top-down questions, there are two other schools of thought that could contribute to such an endeavor: (i) community genetics; and (ii) co-evolutionary mosaics. Community genetics is a growing field that seeks to bridge community ecology and evolutionary biology by investigating how genetic diversity within populations of a single species may affect overall community composition (and it's flip side: how communities may shape the genetic diversity of their constituent species; Whitham et al., 2006). Concepts from community genetics have already been applied toward understanding the effects of genetic diversity on ecosystem function (Whitham et al., 2003; Schweitzer et al., 2004). Co-evolutionary mosaics refer to a different level of interaction. Rather than the interplay between population diversity and community function, the focus is toward the biotic interactions between species. In particular, the long-term dynamics of co-evolution underlie the co-evolutionary mosaics framework, with the idea that these interactions occur over a broad geographic range rather than just locally within populations (Thompson, 2005). In other words, the biotic interactions between species, and not just the species themselves, are considered as the raw material for the overall direction of co-evolution, and genes favored in local interactions can spread out into other populations to create regional patterns (Thompson, 2005).
These ideas form a good foundation for further incorporation of microevolutionary ideas into macroevolutionary research, and each rely on the generation of population genetic or genomic data to achieve an understanding of population variance. Marrying diversity data with population data pertinent to phenotypic trait variance and adaptive potential will also allow researchers to start building holistic population datasets that can be combined to create an ecosystem perspective. Generating this data will require coordinated efforts to apply NGS and other methods across a wide spectrum, encompassing bottom-up and top-down scales. Indeed, NGS databases for a number of organisms are accruing, such that comparing genetic data of multiple species to test hypotheses about the processes that govern large-scale community and ecosystem patterns is becoming feasible (Johnson and Boerlijst, 2002; Hohenlohe et al., 2010; Kelly and Palumbi, 2010).
Final Remarks
A highly significant output of biogeographic and macroecological research is the understanding of relationships between organisms and their environments as drivers of spatial diversity. In today's world, the relevance of this information to conservation approaches cannot be over-stated. Yet, the mechanisms underlying meta-patterns represent an assemblage of processes operating across the landscape at a finer scale; in general, population patterns ultimately form species patterns (Figure 1). In order to truly understand the limits of species distributions, we must understand the development of life history traits and evolutionary potential among taxa, as well as the interactions within and among species, as it is these that define the optimal limits in which organisms reside, both now and in the future. Population genomic data can provide key insights into phenotypic trait variation and adaptive potential that may promote future species responses to environmental change, as well as tell us how groups of related taxa have responded across historical and contemporary scales to reach their current distribution extents. Concepts from community genetics and co-evolutionary mosaics can also provide us with a framework to associate genetic diversity within populations to greater regional landscape patterns. Crucial to exploring all of these possibilities, and hence for ultimately predicting future biogeography and macroecology, is knowledge of population genetic parameters across multiple populations and species. By incorporating a more holistic view that includes the use of bottom-up genomic approaches to get at the finer-scale processes that underlie broad-scale patterns, scientists can better bridge the gap toward a comprehensive understanding of macro-evolutionary research.
Conflict of Interest Statement
The author declares that the research was conducted in the absence of any commercial or financial relationships that could be construed as a potential conflict of interest.
Acknowledgments
I would like to thank Craig Moritz for enlightening discussion around community genetics and co-evolutionary mosaics. I would also like to thank Craig, along with Peter Alan Ritchie and Kevin C. Burns, for critically reviewing an earlier draft of this manuscript.
References
Adler, P. B., White, E. P., Lauenroth, W. K., Kaufman, D. M., Rassweiler, A., and Rusak, J. A. (2005). Evidence for a general species-time-area relationship. Ecology 86, 2032–2039. doi: 10.1890/05-0067
Alvarado-Serrano, D. F., and Knowles, L. L. (2013). Ecological niche models in phylogeographic studies: applications, advances and precautions. Mol. Ecol. Res. 14, 233–248. doi: 10.1111/1755-0998.12184
Beck, J., Kitching, I. J., and Linsenmair, K. E. (2006). Measuring range sizes of southeast-Asian hawkmoths (Lepidoptera: Sphingidae): effects of scale, resolution and phylogeny. Global Ecol. Biogeogr. 15, 339–348. doi: 10.1111/j.1466-822X.2006.00230.x
Bergland, A. O., Behrman, E. L., O'Brien, K. R., Schmidt, P. S., and Petrov, D. A. (2014). Genomic evidence of rapid and stable adaptive oscillations over seasonal time scales in Drosophila. PLoS Genet. 10:e1004775. doi: 10.1371/journal.pgen.1004775
Blackburn, T. M., and Gaston, K. J. (2002). Macroecology is distinct from biogeography. Nature 418, 723. doi: 10.1038/418723b
Bonada, N., Múrria, C., Zamora-Muñoz, C., El Alami, M., Poquet, J. M., Punti, T., et al. (2009). Using community and population approaches to understand how contemporary and historical factors have shaped species distribution in river ecosystems. Global Ecol. Biogeogr. 18, 202–213. doi: 10.1111/j.1466-8238.2008.00434.x
Branco, S., Gladieux, P., Ellison, C. E., Kuo, A., LaButti, K., Lipzen, A., et al. (2015). Genetic isolation between two recently diverged populations of a symbiotic fungus. Mol. Ecol. 24, 2747–2758. doi: 10.1111/mec.13132
Buchmann, C. M., Schurr, F. M., Nathan, R., and Jeltsch, F. (2011). An allometric model of home range formation explains the structuring of animal communities exploiting heterogeneous resources. Oikos 120, 106–118. doi: 10.1111/j.1600-0706.2010.18556.x
Cadotte, M. W., Jonathan Davies, T., Regetz, J., Kembel, S. W., Cleland, E., and Oakley, T. H. (2010). Phylogenetic diversity metrics for ecological communities: integrating species richness, abundance and evolutionary history. Ecol. Lett. 13, 96–105. doi: 10.1111/j.1461-0248.2009.01405.x
Capellini, I., Venditti, C., and Barton, R. A. (2010). Phylogeny and metabolic scaling in mammals. Ecology 91, 2783–2793. doi: 10.1890/09-0817.1
Catullo, R. A., Ferrier, S., and Hoffmann, A. A. (2015). Extending spatial modelling of climate change responses beyond the realized niche: estimating, and accommodating, physiological limits and adaptive evolution. Global Ecol. Biogeogr. 24, 1192–1202. doi: 10.1111/geb.12344
Cavender-Bares, J., Kozak, K. H., Fine, P. V. A., and Kembel, S. W. (2009). The merging of community ecology and phylogenetic biology. Ecol. Lett. 12, 693–715. doi: 10.1111/j.1461-0248.2009.01314.x
Cox, K., Vanden Broek, A., Van Calster, H., and Mergeay, J. (2011). Temperature-related natural selection in a wind-pollinated tree across regional and continental scales. Mol. Ecol. 20, 2724–2738. doi: 10.1111/j.1365-294X.2011.05137.x
Dexter, K. G., Terborgh, J. W., and Cunningham, C. W. (2012). Historical effects on beta diversity and community assembly in Amazonian trees. Proc. Natl Acad. Sci. U.S.A. 109, 7787–7792. doi: 10.1073/pnas.1203523109
Eldon, J., Price, J. P., Magnacca, K., and Price, D. K. (2013). Patterns and processes in complex landscapes: testing alternative biogeographical hypotheses through integrated analysis of phylogeography and community ecology in Hawai'i. Mol. Ecol. 22, 3613–3628. doi: 10.1111/mec.12326
Fordham, D. A., Brook, B. W., Moritz, C., and Nogués-Bravo, D. (2014). Better forecasts of range dynamics using genetic data. Trends Ecol. Evol. 29, 436–443. doi: 10.1016/j.tree.2014.05.007
Friedman, M. (1974). Explanation and scientific understanding. J. Philos. 61, 5–19. doi: 10.2307/2024924
Gotelli, N. J., Anderson, M. J., Arita, H. T., Chao, A., Colwell, R. K., Connolly, S. R., et al. (2009). Patterns and causes of species richness: a general simulation model for macroecology. Ecol. Lett. 12, 873–886. doi: 10.1111/j.1461-0248.2009.01353.x
Hancock, A. M., Brachi, B., Faure, N., Horton, M. W., Jarymowycz, L. B., Sperone, F. G., et al. (2011). Adaptation to climate across the Arabidopsis thaliana genome. Science 334, 83–86. doi: 10.1126/science.1209244
Hawkins, B. A., Diniz-Filho, J. A., Jaramillo, C. A., and Soeller, S. A. (2007). Climate, niche conservatism, and the global bird diversity gradient. Am. Nat. 170, S16–S27. doi: 10.1086/519009
Hendriks, A. J., Willers, B. J. C., Lenders, H. J. R., and Leuven, R. S. E. W. (2009). Towards a coherent allometric framework for individual home ranges, key population patches and geographic ranges. Ecography 32, 929–942. doi: 10.1111/j.1600-0587.2009.05718.x
Hernández, C. E., Rodríguez-Serrano, E., Avaria-Llautureo, J., Inostroza-Michael, O., Morales-Pallero, B., Boric-Bargetto, D., et al. (2013). Using phylogenetic information and the comparative method to evaluate hypotheses in macroecology. Methods Ecol. Evol. 4, 401–415. doi: 10.1111/2041-210X.12033
Hjalmarsson, A. E., Bergsten, J., and Monaghan, M. T. (2015). Dispersal is linked to habitat use in 59 species of water beetles (Coleoptera: Adephaga) on Madagascar. Ecography 38, 732–739. doi: 10.1111/ecog.01138
Hohenlohe, P. A., Bassham, S., Etter, P. D., Stiffler, N., Johnson, E. A., and Cresko, W. A. (2010). Population genomics of parallel adaptation in threespine stickleback using sequenced RAD tags. PLoS Genet. 6:e1000862. doi: 10.1371/journal.pgen.1000862
Jablonski, D. (2008). Species selection: theory and data. Ann. Rev. Ecol. Evol. Syst. 39, 501–524. doi: 10.1146/annurev.ecolsys.39.110707.173510
Jenkins, D. G., and Ricklefs, R. E. (2011). Biogeography and ecology: two views of one world. Phil. Trans. R. Soc. B 366, 2331–2335. doi: 10.1098/rstb.2011.0064
Johnson, C. R., and Boerlijst, M. C. (2002). Selection at the level of the community: the importance of spatial structure. Trends Ecol. Evol. 17, 83–90. doi: 10.1016/S0169-5347(01)02385-0
Jones, F. C., Grabherr, M. G., Chan, Y. F., Russell, P., Mauceli, E., Johnson, J., et al. (2012). The genomic basis of adaptive evolution in threespine sticklebacks. Nature 484, 55–61. doi: 10.1038/nature10944
Kelly, R. P., and Palumbi, S. R. (2010). Genetic structure among 50 species of the Northeastern Pacific Rocky intertidal community. PLoS ONE 5:e8594. doi: 10.1371/journal.pone.0008594
Lomolino, M. V., Riddle, B. R., Whittaker, R. J., and Brown, J. H. (2010). Biogeography, 4th Edn. Sunderland: Sinauer Associates, Inc.
Manel, S., and Holderegger, R. (2013). Ten years of landscape genetics. Trends Ecol. Evol. 28, 614–621. doi: 10.1016/j.tree.2013.05.012
McIntosh, R. P. (1986). The Background of Ecology: Concept and Theory. Cambridge: Cambridge University Press.
McPherson, J. M., Jetz, W., and Rogers, D. J. (2004). The effects of species' range sizes on the accuracy of distribution models: ecological phenomenon or statistical artefact? J. Appl. Ecol. 41, 811–823. doi: 10.1111/j.0021-8901.2004.00943.x
Morin, X., and Chuine, I. (2006). Niche breadth, competitive strength and range size of tree species: a trade-off based framework to understand species distribution. Ecol. Lett. 9, 185–195. doi: 10.1111/j.1461-0248.2005.00864.x
Passy, S. I. (2008). Continental diatom biodiversity, in stream benthos declines as more nutrients become limiting. Proc. Natl Acad. Sci. U.S.A. 105, 9663–9667. doi: 10.1073/pnas.0802542105
Pauls, S. U., Alp, M., Bálint, M., Bernabò, P., Čiampor, F. Jr., and Čiamporová-Zat´ovič, Z. (2014). Integrating molecular tools into freshwater ecology: developments and opportunities. Freshw. Biol. 59, 1559–1576. doi: 10.1111/fwb.12381
Ricklefs, R. E. (2004). A comprehensive framework for global patterns in biodiversity. Ecol. Lett. 7, 1–15. doi: 10.1046/j.1461-0248.2003.00554.x
Ricklefs, R. E. (2007). History and diversity: explorations at the intersection of ecology and evolution. Am. Nat. 170, S56–S70. doi: 10.1086/519402
Ricklefs, R. E., and Jenkins, D. G. (2011). Biogeography and ecology: towards the integration of two disciplines. Phil. Trans. R. Soc. B 366, 2438–2448. doi: 10.1098/rstb.2011.0066
Rominger, A. L., Goodman, K. R., Lim, J. Y., Armstrong, E. E., Becking, L. E., Bennett, G. M., et al. (2015). Community assembly on isolated islands: macroecology meets evolution. Global Ecol. Biogeogr. doi: 10.1111/geb.12341. [Epub ahead of print].
Roncal, J., Blach-Overgaard, A., Borchsenius, F., Balslev, H., and Svenning, J.-C. (2011). A dated phylogeny complements macroecological analysis to explain the diversity patterns in Geonoma (Arecaceae). Biotropica 43, 324–334. doi: 10.1111/j.1744-7429.2010.00696.x
Rosindell, J., Harmon, L. J., and Etienne, R. S. (2015). Unifying ecology and macroevolution with individual-based theory. Ecol. Lett. 18, 472–482. doi: 10.1111/ele.12430
Salmon, W. (1984). Scientific Explanation and the Causal Structure of the World. Princeton, NJ: Princeton University Press.
Saslis-Lagoudakis, C. H., and Greve, M. (2011). Hot topics in biogeography and ecology. Front. Biogeogr. 3, 18–19.
Schweitzer, J. A., Bailey, J. K., Rehill, B. J., Martinsen, G. D., Hart, S. C., Lindroth, R. L., et al. (2004). Genetically based trait in a dominant tree affects ecosystem processes. Ecol. Lett. 7, 127–134. doi: 10.1111/j.1461-0248.2003.00562.x
Svenning, J. C., and Skov, F. (2004). Limited filling of the potential range in European tree species. Ecol. Lett. 7, 565–573. doi: 10.1111/j.1461-0248.2004.00614.x
Thiel-Egenter, C., Alvarez, N., Holderegger, R., Tribsch, A., Englisch, T., Wohlgemuth, T., et al. (2011). Break zones in the distributions of alleles and species in alpine plants. J. Biogeogr. 38, 772–782. doi: 10.1111/j.1365-2699.2010.02441.x
Thomassen, H. A., Fuller, T., Buermann, W., Milá, B., Kieswetter, C. M., Jarrín-V, P., et al. (2011). Mapping evolutionary process: a multi-taxa approach to conservation prioritization. Evol. Appl. 4, 397–413. doi: 10.1111/j.1752-4571.2010.00172.x
Thompson, J. N. (2005). The Geographic Mosaic of Coevolution. Chicago, IL: University of Chicago Press.
Vellend, M., Lajoie, G., Bourret, A., Múrria, C., Kembel, S. W., and Garant, D. (2014). Drawing ecological inferences from coincident patterns of population- and community-level biodiversity. Mol. Ecol. 23, 2890–2901. doi: 10.1111/mec.12756
Villalobos, F., and Paknia, O. (2011). Biogeography and ecology: two lenses in one telescope. Front. Biogeogr. 3, 5–7.
Whitham, T. G., Bailey, J. K., Schweitzer, J. A., Schuster, S. M., Bangert, R. K., LeRoy, C. J., et al. (2006). A framework for community and ecosystem genetics: from genes to ecosystems. Nat. Rev. 7, 510–523. doi: 10.1038/nrg1877
Whitham, T. G., Young, W. P., Martinsen, G. D., Gehring, C. A., Schweitzer, J. A., Shuster, S. M., et al. (2003). Community and ecosystem genetics: a consequence of the extended phenotype. Ecology 84, 559–573. doi: 10.1890/0012-9658(2003)084[0559:CAEGAC]2.0.CO;2
Wiens, J. J., Ackerly, D. D., Allen, A. P., Anacker, B. L., Buckley, L. B., Cornell, H. V., et al. (2010). Niche conservatism as an emerging principle in ecology and conservation biology. Ecol. Lett. 13, 1310–1324. doi: 10.1111/j.1461-0248.2010.01515.x
Wiens, J. J., and Donoghue, M. J. (2004). Historical biogeography, ecology and species richness. Trends Ecol. Evol. 19, 639–644. doi: 10.1016/j.tree.2004.09.011
Winter, M., Schweiger, O., Klotz, S., Nentwig, W., Andriopoulos, P., Arianoutsou, M., et al. (2009). Plant extinctions and introductions lead to phylogenetic and taxonomic homogenization of the European flora. Proc. Natl Acad. Sci. U.S.A. 106, 21721–21725. doi: 10.1073/pnas.0907088106
Keywords: adaptive potential, biogeography, integration, macroecology, population genomics, phenotypic plasticity
Citation: McGaughran A (2015) Integrating a Population Genomics Focus into Biogeographic and Macroecological Research. Front. Ecol. Evol. 3:132. doi: 10.3389/fevo.2015.00132
Received: 21 May 2015; Accepted: 09 November 2015;
Published: 26 November 2015.
Edited by:
Kevin C. Burns, Victoria University of Wellington, New ZealandReviewed by:
Peter Alan Ritchie, Victoria University of Wellington, New ZealandCopyright © 2015 McGaughran. This is an open-access article distributed under the terms of the Creative Commons Attribution License (CC BY). The use, distribution or reproduction in other forums is permitted, provided the original author(s) or licensor are credited and that the original publication in this journal is cited, in accordance with accepted academic practice. No use, distribution or reproduction is permitted which does not comply with these terms.
*Correspondence: Angela McGaughran, ang.mcgaughran@gmail.com