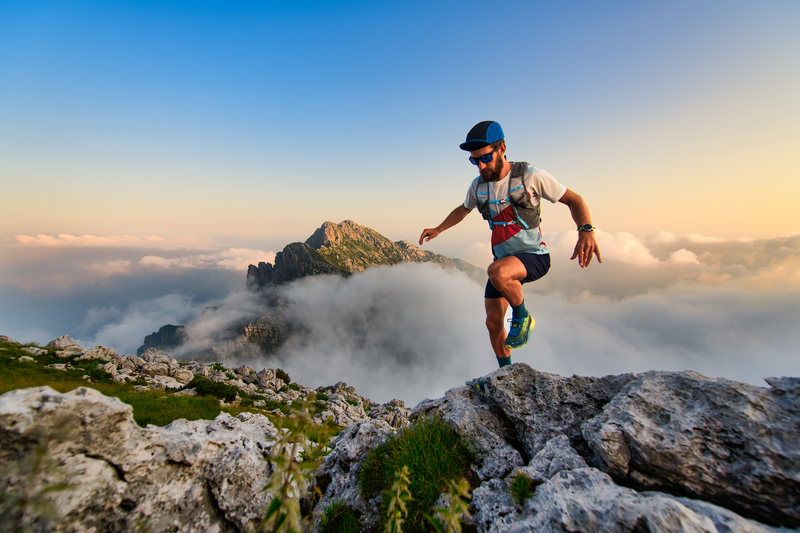
94% of researchers rate our articles as excellent or good
Learn more about the work of our research integrity team to safeguard the quality of each article we publish.
Find out more
ORIGINAL RESEARCH article
Front. Ecol. Evol. , 25 March 2015
Sec. Behavioral and Evolutionary Ecology
Volume 3 - 2015 | https://doi.org/10.3389/fevo.2015.00028
This article is part of the Research Topic The Development of Animal Personality View all 10 articles
The pace-of-life syndrome (POLS) hypothesis for animal personality proposes that variation among individuals in life-history strategies is associated with consistent differences in behavior. We tested predictions of this hypothesis in the superb fairy-wren, Malurus cyaneus, by investigating long-term individual differences in risk-related behaviors (latency to enter a novel artificial environment, exploration, activity and response to mirror image stimulation) and survival. We found consistent differences between individuals in these behaviors (adjusted repeatability of exploration of artificial novel environment = 0.37). Individual differences were consistent over several years and bi-variate analyses showed a significant among-individual correlation (“behavioral syndrome”) between exploration behavior at two life stages (young adult and old adult). Docility at the nestling stage predicted exploration behavior of juveniles. Behavioral traits measured in a risky context were correlated with one another, forming a behavioral syndrome of coping strategies ranging from “proactive” to “reactive.” Nestlings that were more active and exploratory in isolation were less docile during handling, while adults that entered the artificial environment fast were more exploratory, active, and aggressive in the artificial environment. Exploration behavior increased within individuals as they aged and when they were in poorer condition, consistent with expectations of more risk-prone behavior with lower residual reproductive value (RRV) (reduced “asset protection”). Risk-related behavior predicted the probability of apparent survival: more exploratory individuals were less likely to be present in the population 12 months later. Our findings suggest that, consistent with the predictions of the POLS hypothesis, individual variation in survival is associated with consistent individual differences in risk-related behavior that are maintained long-term and span developmental boundaries.
Animals differ in their pace of life, from those that are long-lived with slow reproductive rates, to those that “live fast and die young.” This “slow-fast” spectrum of life-history strategies has been well studied across species (Roff, 2002), but has only recently begun to attract attention as an explanation for behavioral differences among individuals within populations of the same species (Biro and Stamps, 2008; Smith and Blumstein, 2008; Reale et al., 2010). The trade-off between reproduction and survival that underpins the evolution of diverse life-history strategies among species may also be associated with the evolution of behavioral differences among individuals. Individuals that prioritize survival are expected to behave in ways that are more risk averse, while those that prioritize high reproductive rates are expected to engage in more risky behavior to maximize current reproductive success. The pace-of-life syndrome (POLS) hypothesis for animal personality predicts long term maintenance of consistent behavioral differences among individuals within populations, correlations between risk-related behaviors, and earlier mortality among risk-prone individuals (Reale et al., 2010).
If life-history strategies are associated with behavioral strategies, then individuals pursuing different life-history strategies should show consistent, long-term differences in behavior (Reale et al., 2010). If individuals diverge in their life-history trajectories from very early in life, then these behavioral differences may already be apparent in early life. Although the existence of consistent individual differences in behavior is well established in diverse traits across a large range of animal taxa (Bell et al., 2009), less is known about the extent to which such behavioral differences are maintained over the long term. A recent study on captive zebra finches (Taeniopygia guttata) found that nestling activity during begging predicted individual differences in adult activity levels (McCowan and Griffith, 2014). However, repeatability of behavior is often assessed over short time-scales: fewer than 10% of studies reviewed by Bell et al. (2009) spanned more than 1 year, and their meta-analysis showed that repeatability estimates were lower with long than short test intervals. Furthermore, repeatability differed among behavioral traits (Bell et al., 2009). The POLS hypothesis relates specifically to behavioral traits that promote particular life-history strategies (Reale et al., 2010), predicting long-term repeatability of behavioral traits such as boldness, aggression, exploration, and activity, where these are associated with shorter lifespans or increased risk of mortality.
If life-history strategy and risky behavior are linked (Reale et al., 2010), then suites of risk-related behaviors are predicted to be correlated at the between-individual level (a risk-related “behavioral syndrome”). Individuals pursuing a “fast” life-history strategy are predicted to express a variety of risk-related behaviors more than individuals pursuing a “slow” life-history strategy that behave to maximize their likelihood of survival. One context in which such correlations among traits are well established is in research on “coping strategy”—how animals differ in their behavioral responses to stress (Koolhaas et al., 1999; Coppens et al., 2010). Studies on a variety of laboratory, domestic, and more recently wild animals during captivity and handling have shown that individuals vary in a suite of correlated behaviors; “proactive” individuals are risk-taking, bold, aggressive, active, and fast-exploring, while “reactive” individuals are risk-averse, shy, less aggressive, less active, and slow-exploring (Koolhaas et al., 1999; Coppens et al., 2010).
Individuals following different life-history trajectories are expected to show increasing differences in residual reproductive value (RRV) as they age. Differences among individuals in RRV have been suggested as a cause for individual differences in risk-related behavior (Wolf et al., 2007). Specifically, individuals with high RRV are expected to be less likely to engage in risky behaviors to maximize current reproductive success (“asset protection”) than individuals with low RRV. Following the same logic, within-individual variation in RRV could explain behavioral plasticity within individuals over time. Since RRV generally declines with age (Roff, 2002), individuals may engage in more risky behavior as they age. Likewise, individuals may be more risk averse when they are in good condition and more risk prone when they are in poor condition.
The POLS hypothesis predicts that short-lived individuals should be more proactive (less risk-averse) than long-lived individuals, linking life-history strategy (fast-slow) with a behavioral syndrome (proactive-reactive) (Reale et al., 2010). In this scenario, the evolution and maintenance of behavioral diversity in a population is maintained by life-history trade-offs, since different life-history strategies can have similar lifetime fitness. In contrast, some behavioral polymorphisms may be maintained by disruptive viability selection on behavior, where individuals at the extremes of the behavioral continuum have higher survival than those expressing the behavior at average levels (Bergeron et al., 2013), or by fluctuating environments, where different behavioral types have higher survival in different years (Dingemanse et al., 2004). Two meta-analyses investigating the fitness consequences of animal personality found mixed evidence for a relationship between individual differences in behavior and survival (Biro and Stamps, 2008; Smith and Blumstein, 2008). This may be because of fluctuating selection (Dingemanse and Reale, 2013), and because different behavioral traits confer different costs and benefits in terms of survival and reproduction in different species. An explicit focus on the relationship between survival and behavioral responses to risk may enable a clearer test of the POLS hypothesis.
We investigated links between risk-related behaviors and survival in the superb fairy-wren (Malurus cyaneus), a small passerine native to south-eastern Australia. We measured a suite of behavioral traits in nestlings, and over several years in adults, in contexts likely to be perceived as associated with high mortality risk by wild birds—handling and captivity. We tested the following key predictions of the POLS hypothesis:
(1) Individuals show consistent differences in risk-related behavior.
(2) Individual differences in risky behavior are maintained long-term, including across the ontogenetic boundary from nest-bound to free-flying.
(3) Behavioral traits like exploration, boldness, and aggression expressed in a risky context are correlated.
(4) Low RRV is associated with increased risky behavior.
(5) Individual differences in risk-related behavior predict survival.
We studied a population ranging from 98 to 108 groups of superb fairy-wrens resident in a 28 hectare area (700 × 400 m) around the northern arm of the wetland at Serendip Sanctuary (38.00°S, 144.41°E), near Melbourne in south-eastern Australia. All work was conducted with the approval of the University of Melbourne Animal Ethics Committee (1212537.1), Victorian Department of Sustainability and Environment (10006026), and Australian Bird and Bat Banding Scheme (2073 and 1405). To allow individual recognition in the wild, all birds were banded with a unique combination of colored plastic leg-bands, and a numbered aluminum band supplied by the Australian Bird and Bat Banding Scheme. Banding at the site commenced in July 2009, and intensive monitoring of breeding and individual behavioral traits commenced in July 2011. Adults were captured with Ecotone mist-nets for banding, and we collected body measurements including mass (± 0.1 g), fat (furcular deposit scored from 0 = none visible, to 5 = bulging out from the cavity), and tarsus length (± 0.01 mm), and quantified individual behavioral differences in a novel artificial environment (see below) prior to release. We captured birds to quantify behavior in two sessions each year—pre-breeding (spring) and post-breeding (autumn). During the breeding season (September to January), we banded all nestlings as above, weighed them (± 0.1 g), and measured tarsus and fourth primary pin feather lengths (± 0.01 mm). During the 2011 breeding season, we banded nestlings approximately 7 days after hatching, and conducted a standardized test of nestling behavior on day 9 (see below). To reduce disturbance at the nest, from the 2012 breeding season we removed the nestlings only once from the nest, on day 8, for both the behavioral test and banding. The behavioral test was conducted first, except for adding a single plastic band to one leg of each nestling to distinguish individuals.
We re-sighted color-banded individuals approximately weekly during the breeding season to monitor survival, group membership, and nesting. We recorded group size and individual roles—breeding (dominant) females are identifiable as the only group member that builds nests and incubates eggs, and dominant males are typically the oldest male in the group and molt earliest into seasonal breeding plumage (Mulder and Magrath, 1994). All other group members were considered subordinates. In our population, more than half of dominant pairs had subordinate helpers (50–58% of 98–108 pairs in 2011–2013), and group sizes ranged up to 8 (mean ± s.e. = 2.8 ± 0.1). Among pairs with subordinates, 93–98% had male subordinates (mean ± s.e. = 1.5 ± 0.1, maximum 5 male subordinates) and 2–18% had female subordinates (mean ± s.e. = 1.2 ± 0.1, maximum 2 female subordinates). After finding nests, we checked them approximately every 3 days to record nesting details including the date first eggs were laid, clutch size (modal clutch size = 3 eggs), hatching and fledging, and for banding (see above) and behavioral tests (see below). Nestlings were aged based on hatch date (52 nestlings in nests checked during hatching), or inferred from known lay dates (300 nestlings in nests checked during laying), or estimated based on length of the fourth primary pin feather at banding. The latter was the best predictor of age in day 7–10 nestlings based on analysis of morphometric measurements of the 52 nestlings with known hatch date (β ± s.e. for fourth primary = 0.14 ± 0.05, p = 0.01, mass = −0.02 ± 0.14, p = 0.90, tarsus = 0.02 ± 0.12, p = 0.88, exposed feather on fourth primary = 0.01 ± 0.04, p = 0.88). We calculated the age of adult birds at the time of their tests as the difference between the test date and their hatch date, divided by 365. For birds first banded as adults, we estimated their minimum age at the time of the test using the mid-point of the breeding season (1 December) prior to the date they were first captured as their “hatch date” to give an estimate of their minimum age. The minimum age of adult birds was thus a continuous measure (in years) that distinguished age differences between tests conducted in the same year.
We quantified behavior at the nestling stage in a standardized test using early separation and handling stress, two stressors routinely used in developmental and physiological studies on birds and mammals (adapted from Fucikova et al., 2009; Brommer and Kluen, 2012). Nestlings were tested at approximately 8 or 9 days old (see above)—as late as possible in the 13-day nestling period to maximize responsiveness to handling while minimizing the risk of premature fledging. We removed nestlings from the nest and placed them in separate compartments (10 × 10 cm) of a shallow box for 60 s. The box was uncovered so that nestlings were exposed to the open, and visually (but not acoustically) isolated from one another. We video-recorded movements of the nest-mates during 60 s of isolation, and then the handling of each nestling in turn for a 30-s test of docility and 10-s assessment of breathing rate. During handling, the docility of each nestling was assessed by placing it on its back on the flat open palm of an observer's hand for 30 s, with the observer's thumb gently on the body to prevent the nestling righting itself (“back-test,” Hessing et al., 1994). Breathing rate was measured immediately after the back-test, by recording the movement of the nestling's chest associated with respiration for 10 s with the nestling held enclosed in the observer's hand in a more upright position (to minimize struggling that affected chest movements). Docility and breathing rate were then measured sequentially for the remaining nestlings, noting the order in which they were measured. To limit variation due to handling differences, nestlings were usually tested by one of two experienced observers (278 nestlings by MLH, 155 by TvA, 26 by others). For consistency, one person (Susan Ebeling) scored all nestling videos, with breathing rate scored while playing the video at half-speed to facilitate accurate counting of rapid breathing.
We quantified four behavioral variables over the course of the nestling test: (i) Isolation docility: number of seconds spent still in the compartment while isolated (of 60 s total). (ii) Exploration: the number of “zones” used in the compartment while isolated (of 9 in a 3 × 3 grid). (iii) Back-test docility: the number of seconds spent still during the 30-s back-test. (iv) Breaths: the number of breaths taken in a 10-s period immediately after the back-test. Some individuals (n = 25) had missing values due to technical problems with video recording, jumping out of the isolation compartment, or begging disrupting chest movement during the breath-test. We scored all four variables for 459 nestlings in 195 broods in three breeding seasons.
We quantified individual differences in behavior of adult birds in an artificial novel environment (similar to the novel environment test of Verbeek et al., 1994). Following processing in the field (above), birds were taken to onsite testing rooms in a cloth bag, and housed in a holding cage containing two perches, a water dispenser, and a tray on the floor with 10 mealworms. We kept birds in holding cages for at least 1 h to acclimatize. The time-course of hormonal stress responses is unknown in fairy-wrens, but a small sample of birds tested for corticosterone levels at the end of the test showed no relationship between corticosterone level and holding time (Jacques-Hamilton et al. in prep.). For animal welfare reasons, we did not keep birds in captivity overnight, as is done in some studies (Dingemanse et al., 2002), to reduce the likelihood of disrupting a complex social system. We minimized disturbance in holding cages with acoustic foam lining on the internal walls and roof of the cage and a cloth curtain drawn across the wire front. Immediately prior to testing, we transferred a bird to a cage (60 × 31 × 37 cm, with two perches and no food or water) connected to one of two test rooms by a 15 cm diameter round opening covered by one-way glass, permitting the bird to see into the lit test room. After 5 min of acclimatization, the glass door was raised by hand to allow the bird to enter the test room. The solid sides, floor and roof of the cage meant that the bird could not see the observer who raised the glass door. Birds would have been aware of the door being raised due to the associated sound and the increase in light-level in the cage (the glass door was slightly tinted).
The two test rooms (2.8 × 2.8 m, with sloping ceiling 1.95–2.28 m high; see Supplementary Material Figure S1 for schematic illustration of layout) each had two small video cameras (GoPro Hero and GoPro Hero2) attached to the ceiling in opposite corners of the room, with one of the cameras providing a live video feed to monitors in an adjacent “control” room between the two test rooms, to allow scoring of the bird's behavior. An observer used a custom-designed Filemaker Pro layout on an iPhone 4s to record the time and perch used whenever the bird moved among a total of 40 perching areas in the test room. Perching areas included six perches each on two tall (1.69 m) and one medium (1.34 m) wooden stands, four perches on one short (0.75 m) wooden stand, the tops and bases of the four stands, a ledge (11 cm wide × 39 cm long) below the hole via which they entered the room and, since birds also spent time on the floor, nine floor zones (0.9 × 0.9 m) demarcated with a chalked 3 × 3 grid.
We quantified four behavioral variables over the course of the test. (i) Emergence speed: how quickly the bird emerged into the room after the door was raised, fast = <60 s, slow = 60+ s. Birds that did not enter the room naturally were forced to do so by tapping the cage after 3 min (in early tests after 1 or 2 min). (ii) Exploration: the number of unique perching areas the bird used in 5 min after first entering the test room. (iii) Activity: the total number of perching areas the bird used in 2 min after familiarization with the room (starting 6 min after it entered the room). Approximately eight min after the bird entered the room, the one-way glass door was lowered to expose the bird to a 39 × 35 cm mirror. Birds could see their reflections only if perched on the upper four perches of the two stands in front of the mirror, and for this subset of birds, we scored their reaction to their reflection in mirror. (iv) Mirror response: 1 = swooped the mirror, 2 = perched on the ledge in front of the mirror, 3 = pecked at the mirror. We scored movements for 5 min from when the mirror was exposed, or from when the bird first used one of the mirror perches if that was later in the initial 5-min period. At the end of the trial, the observer entered the test room, turned off the light, recaptured the bird by hand, and then released it at its capture location. On average, birds were released 2.65 ± 0.02 h (mean ± s.e.) after capture, and time off-territory did not significantly affect their apparent survival (β ± s.e. = −0.30 ± 0.28, p = 0.28). We completed 1093 tests on 678 birds between 24 January 2012 and 12 October 2014. Individuals were tested up to 5 times (N = 7 birds, 35 with 4 tests, 59 with 3 tests, 164 with 2 tests, 413 with 1 test) and with tests spanning more than 2 years for 20 birds.
We tested whether individuals showed consistent differences in their average behavior by estimating adjusted repeatability—the proportion of the total variation in the behavioral trait attributable to among-individual differences, while controlling for other factors to avoid the “pseudo-repeatability” that may arise if behavior is sampled unevenly with respect to environmental variables that influence behavior (Westneat et al., 2011; Dingemanse and Dochtermann, 2013). Alternative analytical approaches are shown in the Supplementary Material (“raw repeatability” and tests of similarity between first and second trials). We used generalized linear mixed models (GLMMs) to quantify adjusted repeatability, including bird, territory, and observer as random effects to assess the variance explained by these factors and a case-level variable to assess the residual variance. We specified territory as the home territory for all birds with known home territories (birds regularly re-sighted in the same area with the same group in our core area), and as the capture territory for birds without a known home territory (birds resident in territories around our core area that were captured in a core territory, or floaters/dispersers). As fixed effects, we included time held (time in holding cage prior to test, mean ± SD: 1.39 ± 0.39 h), time of day (in decimal format, 12.44 ± 2.20 h), test room (538 tests in room 1 and 555 in room 2), test sequence (up to 5 per bird, 1.59 ± 0.88), test interval (days since the bird's previous test (= 0 for the first test, following Dingemanse et al., 2002), 85.5 ± 149.4 days), season (819 tests in autumn/post-breeding and 274 tests in spring/pre-breeding), and year (486 tests in 2012, 377 in 2013, and 230 in 2014). All covariates were centered and standardized by subtracting the mean and dividing by the standard deviation. We included observer (the person who processed the bird through the test) as a random effect rather than a fixed effect because there were 21 observers over the course of the 3 years, and because we were not interested in differences between particular observers per se. To improve model convergence, we pooled observers who had run fewer than ten tests into the single group, “other,” so that the random effect had 12 levels.
We tested nestling behaviors during isolation and handling as predictors of behavioral differences in juveniles (less than 6 months of age) during artificial environment tests in the autumn following their hatch season using GLMMs, including home territory as a random effect to account for non-independence of birds from the same territory. We modeled error distributions as Poisson with a log link for exploration behavior, and binomial with a logit link for emergence, activity, and mirror response (all as binary responses). As predictors in the models, we included adjusted nestling behavioral scores of three of the four behaviors measured (excluding nestling exploration due to its high correlation with docility in isolation, see Results) and important test-covariates of the adult tests (see Results). Since nestling behaviors varied slightly between handlers, scores were centered and standardized by subtracting the handler-specific mean and dividing by the handler-specific standard deviation. Nestling breathing rate additionally increased with nestling age (1.16 ± 0.28, p < 0.001) and with time of day (0.26 ± 0.10, p = 0.008). In this case, we therefore used residuals from a model of breathing rate that controlled for handler, nestling age, and time of day. Order of testing did not affect any of the nestling behaviors. The analyses included 175 nestlings from 76 territories whose behavior had been tested in the artificial environment during the catching session after the breeding season in which they hatched, when they were 1.1–5.6 months old (mean = 2.6).
The analyses above identified docility during the back-test as the nestling behavior that best predicted juvenile exploration behavior (see Results). We therefore focused on this subset of behavioral measures to run a multi-variate analysis testing for behavioral correlations in tests across three life-stages, including tests on 186 nestlings, 316 “young” adults (up to 2 years from known hatch season, plus early tests of “old” birds), and 216 “old” adults (2+ years from estimated hatch date). Birds of unknown age that were not tested when they were at least 2 years old were excluded. The 186 nestlings were tested in the young adult stage (5 were also tested as old adults) and a further 53 birds were tested in both young and old adult stages. We quantified between-individual covariances (“behavioral syndromes”) between the three response variables (nestling docility and exploration in young adults and old adults) in a multi-variate generalized linear mixed model using Markov chain Monte Carlo methods (implemented with MCMCglmm in R3.0.2, sampling 1 in every 100 iterations after the first 3000 iterations to generate sample sizes of 10 000). We included a random intercept for bird and we set the within-individual covariances to zero (Scenario 4 in Table 2 of Dingemanse and Dochtermann, 2013).
We tested for correlations between the four behavioral traits at both between-individual (“behavioral syndromes”) and within-individual levels using a similar approach as above, partitioning the phenotypic variance in bivariate MCMCglmms. To quantify correlations, we fitted two variables as response variables into a single bi-variate mixed-effect model with a random intercept for bird. The within-individual variance was fixed to one for the binary variables. The variance-covariance matrix estimated by the model provided estimates of the covariance at both between-bird and within-bird levels. However, since all variables have non-normal error distributions, the estimates of within-individual covariances must be treated with caution (Dingemanse and Dochtermann, 2013). We also tested whether there were behavioral differences between individuals that used a perch with a view of the mirror or not.
We tested whether behavioral differences during the test were associated with individual differences in sex, size (tarsus length, in mm), mass, or age (minimum estimated age, in years). We again used GLMMs, testing whether sex, size, mass, or age predicted differences in each of the four behaviors. Since age and mass vary within as well as between birds, we used within-subject centering (van de Pol and Wright, 2009) to determine whether individual differences in behavior were associated with between- or within-individual differences in these traits. As covariates in these models, we included terms indicated as important by the analysis of adjusted repeatability: time of day, test room, year as fixed effects, and bird ID as a random effect. Using model parameters estimated with glmer in R, we ran a simulation with 1000 iterations to estimate effect sizes and 95% CIs (using the function sim in the R package arm). The analyses included only known-sex birds with no missing data for size, mass, and age (875 tests on 506 birds).
We tested whether behavioral differences among adults predicted their likelihood of surviving 12 months from their first test using a binomial mixed model with a logit link, including home territory as a random effect to control for the non-independence of birds sampled from the same territory. We focused on exploration behavior, since it was the behavioral trait with the highest repeatability (see Results), and considered year and sex as additional explanatory factors in the model. We did not use mark-recapture analysis because the probability of failing to resight an individual that was alive within the study population was extremely low due to intensive observations during the breeding seasons. The assumption that birds that disappeared from the study area had died was justified for males and for breeding females as these are highly philopatric (Mulder, 1995; Cockburn et al., 2008). However, young female superb fairy-wrens have longer natal dispersal distances than males (Mulder, 1995; Cockburn et al., 2008) and young females that disappeared from the study population may have died or emigrated (some emigrants were detected during annual surveys of the area surrounding the study population). We therefore repeated the analysis with a subset of the data excluding females that had not yet completed natal dispersal.
The behavior of superb fairy-wrens in an artificial novel environment showed considerable variation (Supplementary Material Figure S2 shows frequency distributions of behaviors), with a significant proportion of this variation due to consistent differences between individuals in their average behavior (Table 1 shows repeatability values adjusted for testing conditions). Between-bird differences explained 37% of the total variance in exploration behavior (adjusted repeatability on both link and original scales) while controlling for other factors. Emergence, activity, and mirror response had lower adjusted repeatability values than exploration behavior, with between-bird differences explaining only around 10% of the total variation in behavior (adjusted repeatability ranged from 6 to 14% on link and original scales, Table 1). Raw repeatability was similar to adjusted repeatability for exploration, and slightly higher than adjusted repeatability for the other three behavioral traits (Supplementary Material Table S1), and there were significant similarities between the behavior of individuals in their first and second tests (Supplementary Material Table S2).
Birds from different territories differed little in their exploration behavior (variance associated with territory much lower than that associated with bird; random effects in Table 1), but differed more in other behaviors, especially activity (variance associated with territory greater than that associated with bird). None of the variation in the measured behaviors was attributed to the effect of different observers carrying out the tests (observer random effect in Table 1).
Average behaviors did not change significantly across the sequence of repeated tests, or with the duration of the interval between repeated tests, the time the bird was held before the test started, or between autumn and spring (Table 1). Exploration behavior decreased with time of day, was lower in room 2 than room 1, and was higher in 2013 than in 2012 and higher again in 2014. Activity also decreased over the course of the day, but was unaffected by other test variables. Emergence was faster in test room 2 and in 2014, while birds were less likely to approach the mirror in room 2, with no effects of other variables.
Nestlings that were more docile during the back-test were less exploratory as juveniles in the artificial environment in the autumn following their hatch season, when they were less than 6 months old (Figure 1, Table 2). Other measures of nestling behavior did not predict juvenile exploration behavior, and nestling behaviors did not predict other measures of juvenile behavior (Table 2).
Figure 1. Nestling docility predicts juvenile exploration. Nestlings that were more docile during the back-test (number of seconds not moving) were less exploratory (number of unique perches) during their first 5 min in an artificial novel environment as juveniles (less than 6 months old). Point size is proportional to sample size in each category. Nestling back-test docility remained a significant predictor of juvenile exploration behavior when three nestlings that were docile for less than 10 s were excluded [β (CI) = −0.09 (−0.17, −0.01); compared to Table 5].
Between-individual covariances across life-stages were strong between exploration scores of young and old adults, but not with nestling docility (Table 3).
The four traits measured in the novel artificial environment were positively correlated with one another between individuals: birds that were on average more exploratory than others were on average also more likely to emerge fast into the test room, be active, and approach the mirror (Table 4). Within-bird correlations between exploration and the three binary traits were very similar to between-bird correlations (except for correlations with emergence, Table 4)—in those trials where an individual was more exploratory, it was more likely to be active and to approach the mirror.
The subsample of individuals for which a mirror response could be scored was not biased with respect to exploration behavior: there was no between-individual correlation between whether a bird used a perch with a view of the mirror and exploration (0.01, 95% CI = −0.28, 0.23). The within-bird correlation was negative (−0.43, 95% CI = −0.66, −0.09), indicating that, if anything, the mirror response was less likely to be scoreable during tests when an individual was more exploratory than during those when it was less exploratory.
Nestling behaviors measured during isolation and handling (Supplementary Material Figure S3 shows distributions) were also mostly correlated with one another. Not surprisingly, nestlings that were more docile during the 60-s period of isolation explored fewer zones in their compartment (Spearman r = −0.68, n = 459, p < 0.001). Nestlings that were more docile in isolation were also more docile during the back-test (Spearman r = 0.19, n = 459, p < 0.001). However, the subsequent breathing rate of nestlings was not correlated with their docility in isolation (Spearman r = 0.06, n = 459, p = 0.22) or during the back-test (Spearman r = 0.03, n = 459, p = 0.47).
Males and females behaved similarly in the test room, and individual differences in size did not predict differences in any of the measured behaviors (sex and tarsus length effects in Table 5). The effect of size on exploration also did not differ between the sexes (interaction β = 0.08, 95% CI = −0.04, 0.20). Birds with an older mean test age were less likely to emerge into the room fast or approach the mirror, and birds with a higher mean test mass were less likely to emerge into the room fast or be active (bird mean effects, Table 5). Within-bird increases in age were associated with increases in exploration behavior, while increases in mass were associated with decreases in both exploration and activity (test-specific deviation from bird mean (dev) effects in Table 5). In general, among-bird differences in behavior continued to explain a significant amount of the variation in behavior when these bird characteristics were accounted for (95% CIs for estimates of variance associated with bird random effect did not overlap zero, Table 5).
Exploration and activity both decreased with within-individual increases in mass (test-specific deviation from bird mean age and mass (dev) in Table 5), suggesting that these correlated behaviors are somewhat condition-dependent. We tested this by assessing effects on exploration of the amount of stored fat birds carried (bird condition) and of recent minimum temperature (environmental conditions: daily minimum averaged over the preceding week). Substituting fat score for mass (including all other terms in the model in Table 5, except age) indicated that birds tested with higher fat scores on average, had slightly lower exploration scores than birds with lower average fat scores [effect of Fat (bird mean) = −0.09, 95% CI = −0.17, −0.03]. Within-bird changes in fat score across tests had no effect on exploration behavior [effect of Fat (dev) = −0.01, 95% CI = −0.06, 0.03, but note overlap with 95% CI of between-bird effect]. Birds tested when average minimum temperatures were higher tended to be less exploratory than birds tested when average minimum temperatures were lower [effect of minimum temperature (bird mean) = −0.07, 95% CI = −0.14, 0.01], while increases in minimum temperatures across tests within-bird were also associated with decreases in exploration behavior [effect of minimum temperature (dev) = −0.07, 95% CI = −0.11, −0.03].
Within-bird increases in age are statistically confounded with habituation effects and year differences, but the former appeared to better explain increases in exploration behavior. Comparing the effects separately in three different models suggested a better fit (ΔAIC > 2) of the model including within- and between-bird variation in Age (AIC = 5474.7) than models with test sequence and test interval (AIC = 5479.5) or Year (AIC = 5486.5; all models included bird and territory as random effects and start time and room as fixed effects, as in Table 1). Furthermore, habituation effects should be strongest when test intervals are short, but exploration behavior instead tended to increase with longer test intervals (β = 0.042, 95% CI = 0.004, 0.085) as well as with test sequence (β = 0.08, 95% CI = 0.04, 0.12). Within-bird increases in age are also statistically confounded with Year [the positive effect of Year in Table 1 became marginally negative in the model including Age (dev) in Table 5]. However, examining changes in exploration scores of cohorts of known-aged birds over the 3 years of testing (Figure 2) suggested that within-bird increases in exploration were not driven by higher exploration scores in 2014 tests than in 2013 and 2012 tests (the 2009 and 2012 cohorts showed no increase between test years).
Figure 2. Variation in exploration behavior with age in five cohorts. Exploration scores (number of unique perches) in tests over the three test years for a subset of birds including a cohort of old birds (banded as adults at the start of the study in 2009) and four cohorts of known-aged birds (banded as nestlings in the 2009, 2011, 2012, and 2013 breeding seasons).
Mortality (or emigration) was more likely among birds that were more exploratory in the artificial environment test (Figure 3, β ± s.e. = −0.58 ± 0.24, p = 0.02, in a logistic model controlling for a tendency for higher apparent survival among males 0.50 ± 0.26, p = 0.06, n = 382 birds from 116 territories). On average, 78.8% of males and 71.4% of females were still present in the population 12 months from their first test date (n = 383 birds from 116 territories first tested before 30 September 2013). Apparent survival did not differ between years (−0.30 ± 0.29, p = 0.30), and there was no evidence of heterogeneity in selection acting on exploration behavior since the effect of exploration on apparent survival did not differ significantly between years (−0.18 ± 0.52, p = 0.73) or between the sexes (0.43 ± 0.50, p = 0.39). Excluding females that had not yet completed natal dispersal showed a similar trend for higher apparent survival of birds that were less exploratory in the test (−0.48 ± 0.26, p = 0.07, n = 326 birds from 115 territories).
Figure 3. Exploration behavior predicts apparent survival. Individuals that were more exploratory in the artificial environment were less likely to be present in the study population 12 months following the test. See text for results of logistic regression analysis of exploration as a continuous variable (here grouped into thirds for illustration) and controlling for non-independence of birds from the same territory. “Breeders” include only the subset of individuals that have completed natal dispersal, when disappearance from the site is more likely to be due to death alone, rather than a combination of death and long-distance dispersal (most likely for young females).
We found support for several predictions of the pace-of-life hypothesis for personality in superb fairy-wrens. Individuals showed consistent and long term differences in risk-related behavior. Behavioral traits expressed during handling and captivity were correlated to form a behavioral syndrome, consistent with a proactive-reactive continuum of coping strategies. Proactive birds were more exploratory in a novel artificial environment, and more likely to emerge into the room fast, to be active, and to approach their reflection in a mirror. Nestlings that were less docile during handling were subsequently more exploratory in a novel environment as juveniles. More exploratory birds had lower apparent survival rates, consistent with the POLS prediction that individuals with short lifespans are less risk-averse.
Superb fairy-wrens showed consistent individual differences in the way they behaved when confronted with a novel artificial environment, a context likely to be perceived as dangerous by wild birds. The repeatability of exploration behavior was typical of behavioral traits measured in other studies (Radj = 0.37, the average in the meta-analysis by Bell et al., 2009), while the repeatability of emergence into the test room (“boldness”), activity, and mirror response (“aggression”) were lower. These latter traits were all binary—tests and/or scoring methods that do not polarize individuals into two behavioral “types” may allow more powerful estimates of repeatability. Our findings are similar to a large number of studies on a variety of taxa demonstrating consistent individual differences in behavior during an “open field” test (Reale et al., 2007).
We found no overall effect of increasing experience with the test on behavior (sequence and interval effects in Table 1), probably because most birds were not tested more than once within the same catching session (spring or autumn). These widely spaced intervals between tests (76% of repeat-tests were conducted more than 3 months after the previous test) thus seemed to minimize effects of prior experience. Other studies have found habituation to the test environment associated with exploration behavior in repeat tests either increasing (when test intervals were short, Dingemanse et al., 2012) or decreasing (Boon et al., 2007) and, within a species, individuals may show substantial variation in short-term habituation patterns (Biro, 2012).
Birds from the same territory were somewhat similar in their activity and mirror response, but not in exploration behavior (territory explained a similar amount of the phenotypic variation to bird in activity and mirror response, but virtually none in exploration; Tables 1, 5). Behavioral similarity among birds from the same territory could be driven by differential settlement patterns (certain behavioral types attracted to certain territories), phenotypic plasticity within individuals to match their behavior with their territory (for example, driven by variation among territories in resources, predation risk, or levels of cover), or genetically-based behavioral similarity among kin (if territory residents are related). In contrast, the social niche hypothesis suggests birds from the same territory would show divergent behaviors associated with role division (Dingemanse and Araya-Ajoy, 2015). The difference in territory effects on behavioral traits of superb fairy-wrens suggests differential effects of these mechanisms. These mechanisms are also likely to affect the sexes differently in superb fairy-wrens. Differential settlement patterns may be relevant to females, since they undertake long-distance natal dispersal to find breeding vacancies, and established female breeders share the territory mostly with relatives (groups form by delayed dispersal of offspring). In contrast, males are highly philopatric with the majority spending their entire life on or close to their natal territory (Mulder, 1995; Cockburn et al., 2008), but male breeders are often unrelated to members of their group due to high rates of extra-pair mating (Mulder et al., 1994; Bain et al., 2014). In this analysis, birds assigned to the same territory included birds resident on the territory and birds not resident in our core area that were captured on the territory (no known territory, so either resident nearby or dispersing through our core area). If these categories of birds differ in their captive exploration scores, this could also contribute to the lack of similarity in this trait between birds from the same territory. Teasing apart the contributions of genetic, environmental, and social effects to individual differences in behavioral traits will help to explain behavioral similarities and differences among birds from the same territory.
The relationship between individual differences in behavior expressed in artificial novel environments and in the wild is a topic of current interest (Niemela and Dingemanse, 2014). In this study, we used the artificial novel environment test to quantify individual differences in response to risk. Some studies have shown positive correlations between exploration of artificial environments and exploration behavior in the wild (for example, Wilson and McLaughlin, 2007; Herborn et al., 2010; Minderman et al., 2010; Bijleveld et al., 2014). Furthermore, research on great tits (Parus major) has shown that exploration of an artificial novel environment is related to natal dispersal (Dingemanse et al., 2003), extra-pair mating (Van Oers et al., 2008), nest defense (Hollander et al., 2008; Cole and Quinn, 2014), and territorial defense (Amy et al., 2010). Further work is needed in superb fairy-wrens to determine whether individual differences in behavioral traits assessed in the captive test predict variation in similar traits in the wild, and other aspects of life history.
Individual differences in risk-related behavior were fairly consistent over the long-term in superb fairy-wrens, with exploration behavior showing significant repeatability over long test intervals. Nestling docility predicted exploration behavior of juveniles (less than 6 months old), and there was significant between-individual covariation in the exploration behavior of young adults (first 2 years) and old adults (more than 2 years since hatching). However, the between-individual covariation between nestling docility and young adult exploration behavior did not differ from zero (Table 3). This may have been because the different test required to assess behavioral responses to risk in the non-mobile nestling stage gave a subtly different assessment of risk-aversion. However, it may also be that early environmental factors influenced juveniles differently, shifting their life-history trajectories and leading to changes in their relative levels of risk-aversion.
Many taxa have ontogenetic boundaries separating life-stages that may differ dramatically, for example, in constraints such as whether individuals are stationary or mobile and in priorities (for example, growth or reproduction). Surprisingly few studies have taken advantage of the opportunity these changed life history contexts provide to improve our understanding of animal personality (Wilson and Krause, 2012a). Although behavior might be expected to shift in association with changes in environments, constraints, or priorities, several studies across a range of taxa have found that consistent individual differences in behavior can be maintained across such ontogenetic boundaries, even if average behaviors change (Niemela et al., 2012; Sprenger et al., 2012; Wilson and Krause, 2012b). The findings of a recent study on captive zebra finches were similar to ours, showing that nestling activity during begging predicted adult activity levels (McCowan and Griffith, 2014).
We found correlations between behavioral traits measured in a risky context that were consistent with superb fairy-wrens displaying a proactive-reactive stress coping strategy similar to that identified in a range of other species (Koolhaas et al., 1999). Compared to reactive birds, proactive birds were more likely to enter the test room fast (“bold”), more exploratory, and more likely to be active and to approach their reflection in a mirror (“aggressive”). These between-individual covariances among traits were similar to the covariances among traits varying within individuals across tests (except for emergence; Table 4). Nestlings that were less docile in the back-test were also less docile and more exploratory in isolation, and less docile nestlings were more exploratory as juveniles, though the single test meant that we could not distinguish within- and between-individual covariances at the nestling stage.
The proactive-reactive behavioral axis has been described in terms of “fight or flight” vs. “freeze” responses to stress (Coppens et al., 2010). We indeed found that, in the high-threat context of an artificial novel environment, behavioral responses of superb fairy-wrens were rather polarized (often better modeled as binary variables, Supplementary Material Figure S2). Some birds were very active, but most were not, even sometimes freezing for a short while, especially in response to the mirror. While assessing variation in response to risk in less threatening situations might reveal more subtle differences between individuals, the artificial environment test highlighted stark differences in the way individual superb fairy-wrens responded to stress, and a behavioral syndrome of correlated traits.
We found no sex- or size-related differences in behavior during the captive test, even though female superb fairy-wrens typically pursue a “faster” life-history strategy than males. Females usually disperse to breed in their first year, whereas males often remain on their natal territory as subordinate helpers for one or more years (female helpers much less common than male helpers in our population, see Study System; also Mulder, 1995) and males must usually survive beyond 3 or 4 years of age to sire offspring (Dunn and Cockburn, 1999). Size is related to life-history strategy in some species, but it is not known whether this is the case in superb fairy-wrens, although male size does not predict within- or extra-pair mating success (Dunn and Cockburn, 1999).
We found mixed support for the proposal that between-individual differences in RRV cause behavioral differences among individuals (asset protection, Wolf et al., 2007). Consistent with the hypothesis, heavier birds (expected to be more risk averse due to higher RRV) were less likely to emerge into the test room fast or be active. However, older birds (expected to be more risk prone due to lower RRV) were instead less likely to emerge into the test room fast or approach the mirror, and between-individual variation in age and mass did not predict exploration behavior. Few other studies have separated between- and within-individual effects of age or mass on risky behavior. Red knots (Calidris canutus) showed a negative between-individual correlation between mass and exploratory behavior in captivity (Bijleveld et al., 2014), also consistent with expectations of asset protection.
If variation in RRV underpins variation in risky behavior (Wolf et al., 2007), then within-individual changes in RRV may also explain behavioral variation within individuals. Consistent with this, superb fairy-wrens became more exploratory and active in the artificial environment when they were in poorer condition (weighed less or had less stored fat) or when environmental conditions were harsher (lower recent minimum temperatures), and they also became more exploratory as they aged. Large fat stores can have costs as well as benefits in birds (for example, in terms of flying efficiency and vulnerability to predation, Witter and Cuthill, 1993). However, fairy-wrens do not often fly long distances and tend to maintain low fat levels—in our sample, only 11% of captures had fat scores above 3 (out of 5). The fact that the effect on behavior of reduced fat was similar to that of reduced minimum temperature was consistent with the interpretation that low fat levels indicate poor condition. The effect of age on exploration was inevitably statistically confounded with year and test sequence, but increases in exploration seemed more likely to be attributable to changes with age than to habituation because the effect was larger when test intervals were longer (habituation effects are expected to be greatest with short test intervals, Dingemanse et al., 2012).
While any effects of sex, size, and age on behavior are likely to be directional, effects of mass could involve feedback loops. Positive feedback between individual characteristics that are labile and behavior can promote stable behavioral differences among individuals, whereas negative feedback can erode individual differences (Wolf et al., 2013; Sih et al., 2015). Thus, if risk-averse behavior increased RRV while risk-prone behavior decreased RRV, positive feedback would maintain individual differences in behavior over time. In contrast, if risk-averse behavior decreased RRV, for example if cautious behavior when food was scarce resulted in mass loss, then this negative feedback would erode individual differences in behavior over time. The relatively long-term stability of behavioral differences in superb fairy-wrens suggests that risk-averse behavior had a positive effect on RRV (at least under the environmental conditions characterizing the period of our study). Investigating the effect of risky behavior in the field on subsequent mass, and the effect of mass on subsequent risky behavior would help to determine the nature of any feedback relationships between these labile state and behavior traits in superb fairy-wrens.
We found that more exploratory birds had lower apparent survival than less exploratory birds (Figure 3). We found no evidence of heterogeneity in selection on exploration behavior with respect to sex or year. However, such effects might be more likely when conditions differ between years, and we did not detect overall differences in apparent survival between years. If viability selection on exploration behavior is indeed directional in our population, then the maintenance of behavioral variation would depend on fecundity selection acting in the opposite direction, such that fast explorers have higher annual productivity, or an earlier peak in fecundity, than slow explorers.
Few other studies have investigated the relationship between survival and consistent individual differences in behavior under risk (Smith and Blumstein, 2008). Similar to our finding in superb fairy-wrens, juvenile European rabbits (Oryctolagus cuniculus) appeared to experience directional viability selection, since those that were more exploratory were less likely to survive (Rödel et al., 2015). Likewise, female North American red squirrels (Tamiasciurus hudsonicus) that were more active in an open field test were less likely to survive until the following spring (Boon et al., 2008). In this species, behavioral variation seemed to be maintained by fitness trade-offs, as females that engaged in more risky behavior in the field were more likely to bequeath their territory to their offspring, a practice that increased offspring fitness (Boon et al., 2008). In contrast, the relationship between novel environment exploration and survival differed between the sexes and between years in great tits in one population (Dingemanse et al., 2004), but not in another (Quinn et al., 2009). In two other studies, less exploratory individuals had shorter lifespans than more exploratory individuals (Banks et al., 2002; Cavigelli and McClintock, 2003).
Death and emigration are not distinguishable in many systems, and many studies reporting links between personality and survival have assessed survival on the basis of local re-sighting or re-trapping (Dingemanse et al., 2004; Boon et al., 2008; Bijleveld et al., 2014; Rödel et al., 2015), thus quantifying “apparent survival,” as we did. In the superb fairy-wren system, death is far more likely than emigration to be the cause of disappearance from the site, except in the case of young females at the natal dispersal stage. A 19-year study on superb fairy-wrens found that 72% of males spent their entire life on their natal territory (often inheriting the dominant position there) and, of those that dispersed, 95% settled on a territory immediately neighboring their natal territory (Cockburn et al., 2008). Male disappearance is thus far more likely due to death than undetected dispersal. Natal dispersal distances of females are longer than males and most young females leave the local area, but are thought to suffer high mortality, resulting in the strongly male-biased sex-ratios that characterize superb fairy-wren populations (Mulder, 1995). The weaker effect of exploration behavior on survival when non-breeding females are excluded (clear vs. filled circles in Figure 3) suggests that fast-exploring females may be more likely to attempt long distance dispersal. An association between personality traits and dispersal has been found in other studies, including in great tits and bluebirds, Sialia spp. (Dingemanse et al., 2003; Duckworth and Badyaev, 2007). For example, there is significant genetic covariance between exploration behavior and local dispersal distances in great tits (Dingemanse et al., 2003; Korsten et al., 2013). Although the precise mechanism is not known, it has been suggested to be mediated by an association between exploration behavior in the novel environment test with exploratory behavior in the field rather than the suite of “proactive” behavioral traits (Korsten et al., 2013).
Alternative mechanisms could explain the relationship between individual differences in exploration behavior and survival (Montiglio et al., 2014). Individuals that engage in more risky behavior may increase their exposure to extrinsic causes of mortality such as predators or pathogens, and suffer higher mortality as a consequence of their risky behavior. Alternatively, intrinsic differences in lifespan among individuals may drive short-lifespan birds to engage in more risky behavior to ensure early reproductive success. Comparative studies have shown that variation in age at first reproduction is linked to metabolic rates and exploration behavior in rodents (Careau et al., 2009), while variation in lifespan across species is linked to rates of telomere shortening in birds and mammals (Haussmann et al., 2003). Resolving the nature of the relationship between individual differences in risky behavior and survival in superb fairy-wrens will require further investigation of the mechanisms involved, such as assessing individual differences in willingness to approach predators, or in intrinsic aging processes.
MH, ND, MM, and RM conceived and designed the work. MH, TA, and AK conducted the behavioral tests on nestling and adult birds. MH analyzed the data and wrote the article. All authors contributed to interpretation of the data, and revised and commented on drafts of the article.
The authors declare that the research was conducted in the absence of any commercial or financial relationships that could be construed as a potential conflict of interest.
We would like to thank the dedicated teams of volunteers for their efforts in the field, monitoring and catching superb fairy-wrens: K. Allen, M. Belpinati, L. Berthold, E. Capp, A. Domalik, J. Jacobs, K. King, H. Knoper, V. Koos, E. Poslinska, M. Proudman, K. Romano, J. Steele, A. Schreiber, and E. Silva. Thanks to S. Ebeling for scoring nestling behavior from videos, and to M. Yewers and E. McLeod for their early work establishing the study population and developing personality tests. Many thanks also to Y. Araya-Ajoy for training and advice on multivariate statistical analysis in R. This research was supported by funding from the Australian Research Council (Discovery Project grant DP110103120 to RM and ND) and from the Australia and Pacific Science Foundation (APSF1204 to MH).
The Supplementary Material for this article can be found online at: http://www.frontiersin.org/journal/10.3389/fevo.2015.00028/abstract
Amy, M., Sprau, P., de Goede, P., and Naguib, M. (2010). Effects of personality on territory defence in communication networks: a playback experiment with radio-tagged great tits. Proc. Biol. Sci. 277, 3685–3692. doi: 10.1098/rspb.2010.0598
PubMed Abstract | Full Text | CrossRef Full Text | Google Scholar
Bain, G. C., Hall, M. L., and Mulder, R. A. (2014). Territory configuration moderates the frequency of extra-group mating in superb fairy-wrens. Mol. Ecol. 23, 5619–5627. doi: 10.1111/mec.12959
PubMed Abstract | Full Text | CrossRef Full Text | Google Scholar
Banks, P. B., Norrdahl, K., and Korpimaki, E. (2002). Mobility decisions and the predation risks of reintroduction. Biol. Conserv. 103, 133–138. doi: 10.1016/S0006-3207(01)00110-0
Bell, A. M., Hankison, S. J., and Laskowski, K. L. (2009). The repeatability of behaviour: a meta-analysis. Anim. Behav. 77, 771–783. doi: 10.1016/j.anbehav.2008.12.022
Bergeron, P., Montiglio, P. O., Reale, D., Humphries, M. M., Gimenez, O., and Garant, D. (2013). Disruptive viability selection on adult exploratory behaviour in eastern chipmunks. J. Evol. Biol. 26, 766–774. doi: 10.1111/jeb.12081
PubMed Abstract | Full Text | CrossRef Full Text | Google Scholar
Bijleveld, A. I., Massourakis, G., van der Marel, A., Dekinga, A., Spaans, B., van Gils, J. A., et al. (2014). Personality drives physiological adjustments and is not related to survival. Proc. Biol. Sci. 281:20133135. doi: 10.1098/rspb.2013.3135
PubMed Abstract | Full Text | CrossRef Full Text | Google Scholar
Biro, P. A. (2012). Do rapid assays predict repeatability in labile (behavioural) traits? Anim. Behav. 83, 1295–1300. doi: 10.1016/j.anbehav.2012.01.036
Biro, P. A., and Stamps, J. A. (2008). Are animal personality traits linked to life-history productivity? Trends Ecol. Evol. 23, 361–368. doi: 10.1016/j.tree.2008.04.003
PubMed Abstract | Full Text | CrossRef Full Text | Google Scholar
Boon, A. K., Reale, D., and Boutin, S. (2007). The interaction between personality, offspring fitness and food abundance in North American red squirrels. Ecol. Lett. 10, 1094–1104. doi: 10.1111/j.1461-0248.2007.01106.x
PubMed Abstract | Full Text | CrossRef Full Text | Google Scholar
Boon, A. K., Reale, D., and Boutin, S. (2008). Personality, habitat use, and their consequences for survival in North American red squirrels Tamiasciurus hudsonicus. Oikos 117, 1321–1328. doi: 10.1111/j.0030-1299.2008.16567.x
Brommer, J. E., and Kluen, E. (2012). Exploring the genetics of nestling personality traits in a wild passerine bird: testing the phenotypic gambit. Ecol. Evol. 2, 3032–3044. doi: 10.1002/ece3.412
PubMed Abstract | Full Text | CrossRef Full Text | Google Scholar
Careau, V., Bininda-Emonds, O. R. P., Thomas, D. W., Reale, D., and Humphries, M. M. (2009). Exploration strategies map along fast-slow metabolic and life-history continua in muroid rodents. Funct. Ecol. 23, 150–156. doi: 10.1111/j.1365-2435.2008.01468.x
Cavigelli, S. A., and McClintock, M. K. (2003). Fear of novelty in infant rats predicts adult corticosterone dynamics and an early death. Proc. Natl. Acad. Sci. U.S.A. 100, 16131–16136. doi: 10.1073/pnas.2535721100
PubMed Abstract | Full Text | CrossRef Full Text | Google Scholar
Cockburn, A., Osmond, H. L., Mulder, R. A., Double, M. C., and Green, D. J. (2008). Demography of male reproductive queues in cooperatively breeding superb fairy-wrens Malurus cyaneus. J. Anim. Ecol. 77, 297–304. doi: 10.1111/j.1365-2656.2007.01335.x
PubMed Abstract | Full Text | CrossRef Full Text | Google Scholar
Cole, E. F., and Quinn, J. L. (2014). Shy birds play it safe: personality in captivity predicts risk responsiveness during reproduction in the wild. Biol. Lett. 10:20140178. doi: 10.1098/rsbl.2014.0178
PubMed Abstract | Full Text | CrossRef Full Text | Google Scholar
Coppens, C. M., de Boer, S. F., and Koolhaas, J. M. (2010). Coping styles and behavioural flexibility: towards underlying mechanisms. Philos. Trans. R. Soc. Lond. B Biol. Sci. 365, 4021–4028. doi: 10.1098/rstb.2010.0217
PubMed Abstract | Full Text | CrossRef Full Text | Google Scholar
Dingemanse, N. J., and Araya-Ajoy, Y. G. (2015). Interacting personalities: behavioural ecology meets quantitative genetics. Trends Ecol. Evol. 30, 88–97. doi: 10.1016/j.tree.2014.12.002
PubMed Abstract | Full Text | CrossRef Full Text | Google Scholar
Dingemanse, N. J., Both, C., Drent, P. J., and Tinbergen, J. M. (2004). Fitness consequences of avian personalities in a fluctuating environment. Proc. Biol. Sci. 271, 847–852. doi: 10.1098/rspb.2004.2680
PubMed Abstract | Full Text | CrossRef Full Text | Google Scholar
Dingemanse, N. J., Both, C., Drent, P. J., van Oers, K., and van Noordwijk, A. J. (2002). Repeatability and heritability of exploratory behaviour in great tits from the wild. Anim. Behav. 64, 929–938. doi: 10.1006/anbe.2002.2006
PubMed Abstract | Full Text | CrossRef Full Text | Google Scholar
Dingemanse, N. J., Both, C., van Noordwijk, A. J., Rutten, A. L., and Drent, P. J. (2003). Natal dispersal and personalities in great tits (Parus major). Proc. Biol. Sci. 270, 741–747. doi: 10.1098/rspb.2002.2300
PubMed Abstract | Full Text | CrossRef Full Text | Google Scholar
Dingemanse, N. J., Bouwman, K. M., van de Pol, M., van Overveld, T., Patrick, S. C., Matthysen, E., et al. (2012). Variation in personality and behavioural plasticity across four populations of the great tit Parus major. J. Anim. Ecol. 81, 116–126. doi: 10.1111/j.1365-2656.2011.01877.x
PubMed Abstract | Full Text | CrossRef Full Text | Google Scholar
Dingemanse, N. J., and Dochtermann, N. A. (2013). Quantifying individual variation in behaviour: mixed- effect modelling approaches. J. Anim. Ecol. 82, 39–54. doi: 10.1111/1365-2656.12013
PubMed Abstract | Full Text | CrossRef Full Text | Google Scholar
Dingemanse, N. J., and Reale, D. (2013). “What is the evidence that natural selection maintains variation in animal personalities?,” in Animal Personalities: Behaviour, Physiology, and Evolution, eds C. Carere and D. Maestripieri (Chicago: The University of Chicago Press), 201–220.
Duckworth, R. A., and Badyaev, A. V. (2007). Coupling of dispersal and aggression facilitates the rapid range expansion of a passerine bird. Proc. Natl. Acad. Sci. U.S.A. 104, 15017–15022. doi: 10.1073/pnas.0706174104
PubMed Abstract | Full Text | CrossRef Full Text | Google Scholar
Dunn, P. O., and Cockburn, A. (1999). Extrapair mate choice and honest signaling in cooperatively breeding superb fairy-wrens. Evolution 53, 938–946. doi: 10.2307/2640733
Fucikova, E., Drent, P. J., Smits, N., and van Oers, K. (2009). Handling stress as a measurement of personality in great tit nestlings (Parus major). Ethology 115, 366–374. doi: 10.1111/j.1439-0310.2009.01618.x
PubMed Abstract | Full Text | CrossRef Full Text | Google Scholar
Gelman, A. (2008). Scaling regression inputs by dividing by two standard deviations. Stat. Med. 27, 2865–2873. doi: 10.1002/sim.3107
PubMed Abstract | Full Text | CrossRef Full Text | Google Scholar
Haussmann, M. F., Winkler, D. W., O'Reilly, K. M., Huntington, C. E., Nisbet, I. C. T., and Vleck, C. M. (2003). Telomeres shorten more slowly in long-lived birds and mammals than in short-lived ones. Proc. Biol. Sci. 270, 1387–1392. doi: 10.1098/rspb.2003.2385
PubMed Abstract | Full Text | CrossRef Full Text | Google Scholar
Herborn, K. A., MacLeod, R., Miles, W. T. S., Schofield, A. N. B., Alexander, L., and Arnold, K. E. (2010). Personality in captivity reflects personality in the wild. Anim. Behav. 79, 835–843. doi: 10.1016/j.anbehav.2009.12.026
Hessing, M. J. C., Hagelso, A. M., Schouten, W. G. P., Wiepkema, P. R., and van beek, J. A. (1994). Individual behavioral and phyiological strategies in pigs. Physiol. Behav. 55, 39–46. doi: 10.1016/0031-9384(94)90007-8
PubMed Abstract | Full Text | CrossRef Full Text | Google Scholar
Hollander, F. A., van Overveld, T., Tokka, I., and Matthysen, E. (2008). Personality and nest defence in the great tit (Parus major). Ethology 114, 405–412. doi: 10.1111/j.1439-0310.2008.01488.x
Koolhaas, J. M., Korte, S. M., De Boer, S. F., Van Der Vegt, B. J., Van Reenen, C. G., Hopster, H., et al. (1999). Coping styles in animals: current status in behavior and stress-physiology. Neurosci. Biobehav. Rev. 23, 925–935. doi: 10.1016/S0149-7634(99)00026-3
PubMed Abstract | Full Text | CrossRef Full Text | Google Scholar
Korsten, P., van Overveld, T., Adriaensen, F., and Matthysen, E. (2013). Genetic integration of local dispersal and exploratory behaviour in a wild bird. Nat. Commun. 4:2362. doi: 10.1038/ncomms3362
PubMed Abstract | Full Text | CrossRef Full Text | Google Scholar
McCowan, L., and Griffith, S. C. (2014). Nestling activity levels during begging behaviour predicts activity level and body mass in adulthood. PeerJ 2:e566. doi: 10.7717/peerj.566
PubMed Abstract | Full Text | CrossRef Full Text | Google Scholar
Minderman, J., Reid, J. M., Hughes, M., Denny, M. J. H., Hogg, S., Evans, P. G. H., et al. (2010). Novel environment exploration and home range size in starlings Sturnus vulgaris. Behav. Ecol. 21, 1321–1329. doi: 10.1093/beheco/arq151
Montiglio, P. O., Garant, D., Bergeron, P., Messier, G. D., and Reale, D. (2014). Pulsed resources and the coupling between life-history strategies and exploration patterns in eastern chipmunks (Tamias striatus). J. Anim. Ecol. 83, 720–728. doi: 10.1111/1365-2656.12174
PubMed Abstract | Full Text | CrossRef Full Text | Google Scholar
Mulder, R. A. (1995). Natal and breeding dispersal in a co-operative, extra-group-mating bird. J. Avian Biol. 26, 234–240. doi: 10.2307/3677324
Mulder, R. A., Dunn, P. O., Cockburn, A., Lazenby-Cohen, K. A., and Howell, M. J. (1994). Helpers liberate female fairy-wrens from constraints on extra-pair mate choice. Proc. R. Soc. Lond. B Biol. Sci. 255, 223–229. doi: 10.1098/rspb.1994.0032
Mulder, R. A., and Magrath, M. J. L. (1994). Timing of prenuptial molt as a sexually selected indicator of male quality in superb fairy-wrens (Malurus cyaneus). Behav. Ecol. 5, 393–400. doi: 10.1093/beheco/5.4.393
Nakagawa, S., and Schielzeth, H. (2010). Repeatability for Gaussian and non-Gaussian data: a practical guide for biologists. Biol. Rev. Camb. Philos. Soc. 85, 935–956. doi: 10.1111/j.1469-185X.2010.00141.x
PubMed Abstract | Full Text | CrossRef Full Text | Google Scholar
Niemela, P. T., and Dingemanse, N. J. (2014). Artificial environments and the study of “adaptive” personalities. Trends Ecol. Evol. 29, 245–247. doi: 10.1016/j.tree.2014.02.007
PubMed Abstract | Full Text | CrossRef Full Text | Google Scholar
Niemela, P. T., Vainikka, A., Hedrick, A. V., and Kortet, R. (2012). Integrating behaviour with life history: boldness of the field cricket, Gryllus integer, during ontogeny. Funct. Ecol. 26, 450–456. doi: 10.1111/j.1365-2435.2011.01939.x
Quinn, J. L., Patrick, S. C., Bouwhuis, S., Wilkin, T. A., and Sheldon, B. C. (2009). Heterogeneous selection on a heritable temperament trait in a variable environment. J. Anim. Ecol. 78, 1203–1215. doi: 10.1111/j.1365-2656.2009.01585.x
PubMed Abstract | Full Text | CrossRef Full Text | Google Scholar
Reale, D., Garant, D., Humphries, M. M., Bergeron, P., Careau, V., and Montiglio, P. O. (2010). Personality and the emergence of the pace-of-life syndrome concept at the population level. Philos. Trans. R. Soc. B Biol. Sci. 365, 4051–4063. doi: 10.1098/rstb.2010.0208
PubMed Abstract | Full Text | CrossRef Full Text | Google Scholar
Reale, D., Reader, S. M., Sol, D., McDougall, P. T., and Dingemanse, N. J. (2007). Integrating animal temperament within ecology and evolution. Biol. Rev. Camb. Philos. Soc. 82, 291–318. doi: 10.1111/j.1469-185X.2007.00010.x
PubMed Abstract | Full Text | CrossRef Full Text | Google Scholar
Rödel, H. G., Zapka, M., Talke, S., Kornatz, T., Bruchner, B., and Hedler, C. (2015). Survival costs of fast exploration during juvenile life in a small mammal. Behav. Ecol. Sociobiol. 69, 205–217. doi: 10.1007/s00265-014-1833-5
Sih, A., Mathot, K. J., Moirón, M., Montiglio, P. O., Wolf, M., and Dingemanse, N. J. (2015). Animal personality and state–behaviour feedbacks: a review and guide for empiricists. Trends Ecol. Evol. 30, 50–60. doi: 10.1016/j.tree.2014.11.004
PubMed Abstract | Full Text | CrossRef Full Text | Google Scholar
Smith, B. R., and Blumstein, D. T. (2008). Fitness consequences of personality: a meta-analysis. Behav. Ecol. 19, 448–455. doi: 10.1093/beheco/arm144
PubMed Abstract | Full Text | CrossRef Full Text | Google Scholar
Sprenger, D., Dingemanse, N. J., Dochtermann, N. A., Theobald, J., and Walker, S. P. W. (2012). Aggressive females become aggressive males in a sex-changing reef fish. Ecol. Lett. 15, 986–992. doi: 10.1111/j.1461-0248.2012.01819.x
PubMed Abstract | Full Text | CrossRef Full Text | Google Scholar
van de Pol, M. V., and Wright, J. (2009). A simple method for distinguishing within- versus between-subject effects using mixed models. Anim. Behav. 77, 753–758. doi: 10.1016/j.anbehav.2008.11.006
Van Oers, K., Drent, P. J., Dingemanse, N. J., and Kempenaers, B. (2008). Personality is associated with extrapair paternity in great tits, Parus major. Anim. Behav. 76, 555–563. doi: 10.1016/j.anbehav.2008.03.011
PubMed Abstract | Full Text | CrossRef Full Text | Google Scholar
Verbeek, M. E. M., Drent, P. J., and Wiepkema, P. R. (1994). Consistent individual differences in early exploratory behaviour of male great tits. Anim. Behav. 48, 1113–1121. doi: 10.1006/anbe.1994.1344
PubMed Abstract | Full Text | CrossRef Full Text | Google Scholar
Westneat, D. F., Hatch, M. I., Wetzel, D. P., and Ensminger, A. L. (2011). Individual variation in parental care reaction norms: integration of personality and plasticity. Am. Nat. 178, 652–667. doi: 10.1086/662173
PubMed Abstract | Full Text | CrossRef Full Text | Google Scholar
Wilson, A. D. M., and Krause, J. (2012a). Metamorphosis and animal personality: a neglected opportunity. Trends Ecol. Evol. 27, 529–531. doi: 10.1016/j.tree.2012.07.003
PubMed Abstract | Full Text | CrossRef Full Text | Google Scholar
Wilson, A. D. M., and Krause, J. (2012b). Personality and metamorphosis: is behavioral variation consistent across ontogenetic niche shifts? Behav. Ecol. 23, 1316–1323. doi: 10.1093/beheco/ars123
Wilson, A. D. M., and McLaughlin, R. L. (2007). Behavioural syndromes in brook charr, Salvelinus fontinalis: prey-search in the field corresponds with space use in novel laboratory situations. Anim. Behav. 74, 689–698. doi: 10.1016/j.anbehav.2007.01.009
Witter, M. S., and Cuthill, I. C. (1993). The ecological costs of avian fat storage. Philos. Trans. R. Soc. Lond. B Biol. Sci. 340, 73–92. doi: 10.1098/rstb.1993.0050
PubMed Abstract | Full Text | CrossRef Full Text | Google Scholar
Wolf, M., van Doorn, G. S., Leimar, O., and Weissing, F. J. (2007). Life-history trade-offs favour the evolution of animal personalities. Nature 447, 581–584. doi: 10.1038/nature05835
PubMed Abstract | Full Text | CrossRef Full Text | Google Scholar
Wolf, M., van Doorn, G. S., Leimar, O., and Weissing, F. J. (2013). “The evolution of animal personality,” in Animal Personalities: Behaviour, Physiology, and Evolution, eds C. Carere and D. Maestripieri (Chicago: The University of Chicago Press), 252–275. doi: 10.7208/chicago/9780226922065.003.0010
Keywords: animal personality, life-history strategy, survival rate, individual variation, risk-taking
Citation: Hall ML, van Asten T, Katsis AC, Dingemanse NJ, Magrath MJL and Mulder RA (2015) Animal personality and pace-of-life syndromes: do fast-exploring fairy-wrens die young? Front. Ecol. Evol. 3:28. doi: 10.3389/fevo.2015.00028
Received: 24 December 2014; Accepted: 05 March 2015;
Published: 25 March 2015.
Edited by:
Ann Valerie Hedrick, University of California Davis, USAReviewed by:
Peter L. Hurd, University of Alberta, CanadaCopyright © 2015 Hall, van Asten, Katsis, Dingemanse, Magrath and Mulder. This is an open-access article distributed under the terms of the Creative Commons Attribution License (CC BY). The use, distribution or reproduction in other forums is permitted, provided the original author(s) or licensor are credited and that the original publication in this journal is cited, in accordance with accepted academic practice. No use, distribution or reproduction is permitted which does not comply with these terms.
*Correspondence: Michelle L. Hall, School of BioSciences, University of Melbourne, Building 147, Melbourne, VIC 3010, AustraliaaGFsbC5tQHVuaW1lbGIuZWR1LmF1
Disclaimer: All claims expressed in this article are solely those of the authors and do not necessarily represent those of their affiliated organizations, or those of the publisher, the editors and the reviewers. Any product that may be evaluated in this article or claim that may be made by its manufacturer is not guaranteed or endorsed by the publisher.
Research integrity at Frontiers
Learn more about the work of our research integrity team to safeguard the quality of each article we publish.