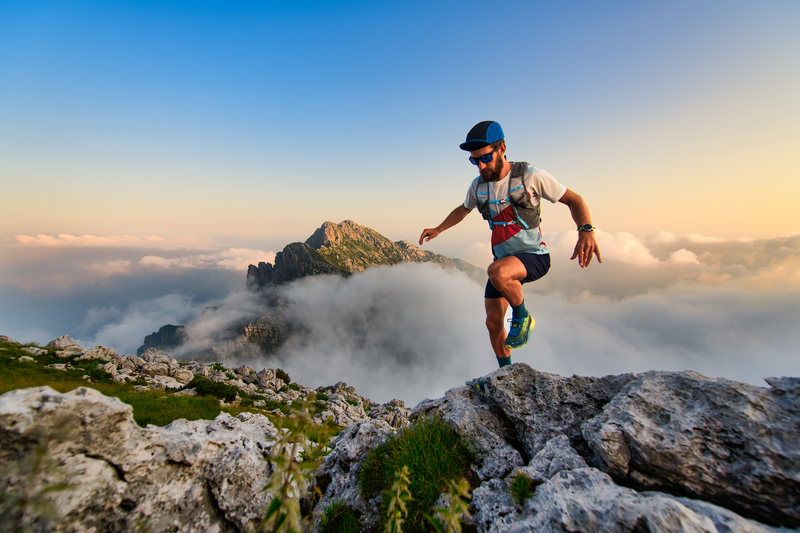
95% of researchers rate our articles as excellent or good
Learn more about the work of our research integrity team to safeguard the quality of each article we publish.
Find out more
GENERAL COMMENTARY article
Front. Epidemiol. , 10 February 2025
Sec. Occupational and Environmental Epidemiology
Volume 5 - 2025 | https://doi.org/10.3389/fepid.2025.1480372
This article is a commentary on:
Oil and gas development exposure and atrial fibrillation exacerbation: a retrospective study of atrial fibrillation exacerbation using Colorado's all payer claims dataset
A Commentary on
By McKenzie LM, Allshouse WB, Abrahams B and Tompkins C (2024). Front. Epidemiol. 4:1379271. doi: 10.3389/fepid.2024.1379271
McKenzie et al. (1) recently evaluated whether individuals with pre-existing atrial fibrillation (AF) or atrial flutter (AFl) living within 1 mile of an oil and gas (O&G) well were more likely to experience a healthcare encounter for AF/AFl during or after, compared to before, well development. The analysis used 2009–2017 data from Colorado's All Payer Claims Database (APCD) (2), representing nearly two-thirds of the state's insured residents.
The study was described as a quasi-experimental interrupted time series (ITS); however, it did not satisfy ITS design characteristics or statistical requirements, and to describe it as such ascribes rigor to the analysis that the structure of the data was unable to provide. The paper further detailed the use of survival analysis (SA), but again, the analysis did not include critical elements of this statistical approach.
Readers who have less familiarity with these statistical techniques may take these study results at face value, without understanding that the authors applied what are perceived as rigorous methods to data that were not suited for them. The methodological issues with this analysis, discussed below, should be addressed by the authors before the study is accepted as epidemiological evidence that nearby O&G activity increases the risk of AF/AFl.
ITS is the real-world analog to a randomized controlled trial (RCT) (3). As described in papers cited by McKenzie et al., ITS evaluates the impact of an intervention introduced to a specific population at a clearly defined point in time, effectively controlling for between-group differences and underlying time trends in the outcome (4, 5). The objective is to describe whether increased disease occurrence coincided with the start of the intervention (6). Results are typically reported as the average number or rate of health events before and after the intervention (7).
The authors chose the ITS design because it allowed for control of potential confounders unavailable in the APCD. However, this feature of ITS hinges on the intervention beginning and ending at approximately the same time across the study population, similar to RCT participants. The intervention in this study was the development of a well within 1 mile of a home address, starting with the spud date and ending on the first day of production. This “during” drilling (i.e., intervention) period was highly variable across study participants, ranging from 3 to 844 days. Periods “before” and “after” the intervention were the same duration as the “during” period, but one before the spud date and the other after the first production date. Thus, follow-up periods including before, during, and after drilling for the nearly 1,200 study participants were as short as 9 days for some and over 2,500 days for others, staggered across the 9-year study period. While statistical approaches to analyze multiple baseline time series data exist, they cannot accommodate both staggered intervention start dates and widely varying follow-up time across the study population (8).
The authors do not explain why multi-failure SA was used, which is not a typical or recommended statistical method for ITS (9, 10). The study reported hazard ratios—the estimate of risk produced by SA—comparing the risk of AF/AFl encounters during vs. before drilling and after vs. before drilling.
SA requires calculating the observed person-time for each participant (11) based on the period of time he or she was “at risk” of a recorded AF/AFl encounter, which presumes presence in the APCD with no gaps in coverage. Person-time could almost certainly have been derived from beneficiary enrollment dates in the APCD; however, no description of this was included in the paper. Rather, it appears that at-risk and control patients were assumed to be present in the APCD for the entirety of their follow-up periods.
More concerning was the exclusion of half the at-risk patients from the analysis “to reduce errors from unknown losses to follow-up.” McKenzie et al. describe these participants in their text as “without a claim of any type preceding the before period and succeeding the after period,” and alternatively in their Figure 2 as “without evidence of presence in APCD through follow-up” (1). However, since an AF/AFl diagnosis between 2009 and 2017 (but before the end of follow-up) was required for study eligibility, and the same APCD enrollment file from which the authors obtained address, gender, and birth date also included enrollment dates, the reason for excluding these patients is unclear. Excluding those with no claims during follow-up from the at-risk population would effectively inflate hazard ratios by removing person-time from the denominator that did not add any AF/AFl events to the numerator.
Finally, the lack of covariate data in the APCD, while not required for ITS, is required for SA, particularly when participant follow-up time is variable and not temporally aligned. Although the study included controls, this could not account for factors that varied with time because the controls were matched to at-risk patients not by calendar year, but rather by region of residence in the state and year of first AF/AFl diagnosis in the APCD. In addition to seasonal trends and increasing AF prevalence during the 9-year study period (12), technological, regulatory, and economic factors likely impacted underlying trends in noise and air pollution exposure resulting from nearby drilling over time.
The question addressed in the study by McKenzie et al.—whether close proximity drilling exacerbates existing AF/AFl, and if so, whether certain demographic groups are particularly susceptible—is an important one that has not been previously addressed. The design of the study was novel, and the dataset used in their analysis is a rich source of claims data from a state with considerable O&G activity.
The authors state in their conclusion: “these findings support development of mitigation strategies and regulations to protect the health of populations living near O&G well sites.” The methodological issues described above, however, are significant and raise concerns about the validity and interpretation of the reported results. Given the importance of this topic, and potential implications for public health practitioners and policymakers, I encourage the authors to address these methodological issues in any further research they conduct on this topic.
JWH: Writing – original draft, Writing – review & editing.
The author(s) declare that no financial support was received for the research, authorship, and/or publication of this article.
JWH was employed by Shell Oil Company, and has consulted to oil and gas companies and trade associations.
All claims expressed in this article are solely those of the authors and do not necessarily represent those of their affiliated organizations, or those of the publisher, the editors and the reviewers. Any product that may be evaluated in this article, or claim that may be made by its manufacturer, is not guaranteed or endorsed by the publisher.
1. McKenzie LM, Allshouse WB, Abrahams B, Tompkins C. Oil and gas development exposure and atrial fibrillation exacerbation: a retrospective study of atrial fibrillation exacerbation using Colorado's all payer claims dataset. Front Epidemiol. (2024) 4:1379271. doi: 10.3389/fepid.2024.1379271
2. Center for Improving Value in Health Care. CO APCD Info. Available online at: https://civhc.org/get-data/co-apcd-info/ (Accessed August 12, 2024)
3. Kontopantelis E, Doran T, Springate DA, Buchan I, Reeves D. Regression based quasi-experimental approach when randomisation is not an option: interrupted time series analysis. BMJ. (2015) 350:h2750. doi: 10.1136/bmj.h2750
4. Bernal JL, Cummins S, Gasparrini A. Interrupted time series regression for the evaluation of public health interventions: a tutorial. Int J Epidemiol. (2017) 46(1):348–55. doi: 10.1093/ije/dyw098
5. Lopez Bernal J, Cummins S, Gasparrini A. The use of controls in interrupted time series studies of public health interventions. Int J Epidemiol. (2018) 47(6):2082–93. doi: 10.1093/ije/dyy135
6. Wagner A, Soumerai S, Zhang F, Ross-Degnan D. Segmented regression analysis of interrupted time series studies in medication use research. J Clin Pharm Ther. (2002) 27(4):299–309. doi: 10.1046/j.1365-2710.2002.00430.x
7. Habib N, Steyn P, Boydell V, Cordero J, Nguyen M, Thwin S, et al. The use of segmented regression for evaluation of an interrupted time series study involving complex intervention: the CaPSAI project experience. Health Serv Outcomes Res Methodol. (2021) 21(2):188–205. doi: 10.1007/s10742-020-00221-9
8. Biglan A, Ary D, Wagenaar AC. The value of interrupted time-series experiments for community intervention research. Prev Sci. (2000) 1(1):31–49. doi: 10.1023/A:1010024016308
9. Hategeka C, Ruton H, Karamouzian M, Lynd LD, Law MR. Use of interrupted time series methods in the evaluation of health system quality improvement interventions: a methodological systematic review. BMJ Glob Health. (2020) 5(10):e003567. doi: 10.1136/bmjgh-2020-003567
10. Ewusie JE, Soobiah C, Blondal E, Beyene J, Thabane L, Hamid JS. Methods, applications and challenges in the analysis of interrupted time series data: a scoping review. J Multidiscip Healthc. (2020) 13:411–23. doi: 10.2147/JMDH.S241085
11. Sainani KL. Introduction to survival analysis. PM&R. (2016) 8:580–5. doi: 10.1016/j.pmrj.2016.04.003
Keywords: atrial fibrillation, survival analysis, interrupted time series, oil and gas development, Colorado (USA)
Citation: Wendt Hess J (2025) Commentary: Oil and gas development exposure and atrial fibrillation exacerbation: a retrospective study of atrial fibrillation exacerbation using Colorado's all payer claims dataset. Front. Epidemiol. 5:1480372. doi: 10.3389/fepid.2025.1480372
Received: 13 August 2024; Accepted: 13 January 2025;
Published: 10 February 2025.
Edited by:
Morteza Abdullatif Khafaie, Ahvaz Jundishapur University of Medical Sciences, IranReviewed by:
Morufu Olalekan Raimi, Federal University, NigeriaCopyright: © 2025 Wendt Hess. This is an open-access article distributed under the terms of the Creative Commons Attribution License (CC BY). The use, distribution or reproduction in other forums is permitted, provided the original author(s) and the copyright owner(s) are credited and that the original publication in this journal is cited, in accordance with accepted academic practice. No use, distribution or reproduction is permitted which does not comply with these terms.
*Correspondence: Judy Wendt Hess, ZXBpc2VydmljZXNAcHJvdG9uLm1l
Disclaimer: All claims expressed in this article are solely those of the authors and do not necessarily represent those of their affiliated organizations, or those of the publisher, the editors and the reviewers. Any product that may be evaluated in this article or claim that may be made by its manufacturer is not guaranteed or endorsed by the publisher.
Research integrity at Frontiers
Learn more about the work of our research integrity team to safeguard the quality of each article we publish.