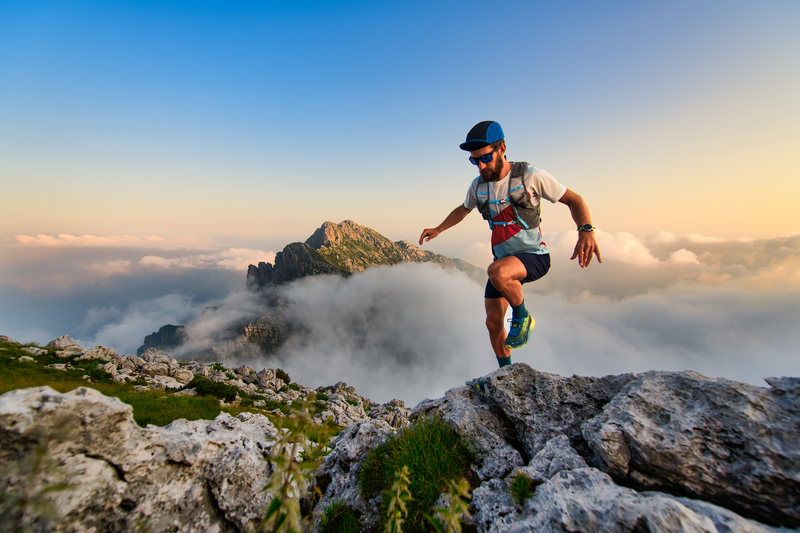
94% of researchers rate our articles as excellent or good
Learn more about the work of our research integrity team to safeguard the quality of each article we publish.
Find out more
ORIGINAL RESEARCH article
Front. Environ. Sci. , 12 February 2025
Sec. Environmental Economics and Management
Volume 13 - 2025 | https://doi.org/10.3389/fenvs.2025.1492874
Environmental pollution is a significant constraint on the sustainable development of manufacturing companies, while digital transformation has been proven to be an effective method for companies’ environmental governance. Government environmental information disclosure (GEID), as a crucial information governance tool, enhances the collaborative environmental governance capabilities of both government and external stakeholders of companies. Logically, this indicates that by emphasizing environmental regulation, GEID can promote corporate digital transformation. This study empirically analyzes the impact of GEID on the digital transformation of companies, using a sample of Chinese A-share listed manufacturing companies. The results indicate that higher local GEID levels significantly boost the digital transformation of manufacturing companies in the region, with a one-point increase in GEID resulting in a 0.31% rise in the degree of digital transformation. This conclusion remains robust across various endogeneity and robustness tests. Further analysis reveals that GEID primarily promotes digital transformation through mechanisms such as fostering companies’ green development strategies and green innovation, increasing R&D expenditure, and reducing financing constraints. Additionally, the study finds that the impact of GEID on digital transformation is especially significant in firms with poor environmental performance, low fixed asset ratios, non-state-owned enterprises, and during the post-2015 period. Despite heavy assets typically being seen as a barrier to digital transformation in heavily polluting industries, GEID still significantly promotes the digital transformation of asset-intensive enterprises. This study not only provides a new perspective on understanding the impact of GEID on corporate digital transformation but also offers empirical evidence for policymakers and corporate managers, thereby aiding enterprises in achieving sustainable development goals.
Digital transformation aims to enhance entities by leveraging information, computing, communication, and connectivity technologies, significantly impacting production, operations, and governance (Vial, 2019). For corporate environmental governance, digital transformation allows for more effective data collection and analysis, addressing resource waste, pollutant emissions, and customer preferences, ultimately optimizing resource allocation. Previous studies demonstrate that digital transformation fosters progress in green innovation (Martínez Falcó et al., 2024), pollution reduction (Zhao et al., 2024), environmental supply chain sustainability (Sarkis et al., 2021), energy efficiency, resource management (Kunkel and Matthess, 2020), energy transition (Stermieri et al., 2023), total factor productivity (Cheng et al., 2025), and overall environmental performance (Chen and Hao, 2022).
High energy consumption and emissions are prominent features of production processes (Zhang and Cheng, 2009), leading to environmental degradation and inhibiting green energy consumption (Qing et al., 2024). To address these challenges, technical change has emerged as a crucial approach for environmental governance and low-carbon transformation (Jiang et al., 2023). Despite this, the process of technological change is often impeded by market failures (Jaffe et al., 2005). Therefore, alongside technological governance, countries are focusing on environmental regulatory policies. These policies aim to design appropriate regulatory frameworks that incentivize innovation, thereby overcoming market failures and achieving a win-win outcome for both the environment and the economy (Porter and Van der Linde, 1995). However, traditional command-and-control environmental regulations lack flexibility and incur high administrative costs, leading to inefficiencies (Hahn and Stavins, 1991). Governmental disclosure of environmental supervision information, as an information-based tool, enhances public and investor access to corporate environmental data, increasing external pressure and fostering collaborative governance between stakeholders and the government. This approach has gained widespread attention and is central to global environmental initiatives (Attard et al., 2015). Moreover, the integration of information technology with environmental regulation, exemplified by smart city developments, has been shown to improve green economic efficiency (Chen et al., 2024).
On the other hand, pollutant emissions from the manufacturing industry constitute a significant portion of total emissions, particularly in China. According to the China Emission Accounts and Datasets (CEADs), national CO2 emissions in 2021 totaled 10,356.3 million tons, with the manufacturing sector contributing 3,482.2 million tons, accounting for 33.62% of the total emissions. The China Environmental Statistics Yearbook (2023) reports that in 2022, total sulfur dioxide emissions were 2.43 million tons, with manufacturing responsible for 1.23 million tons, constituting 50.62% of the total emissions. Additionally, total nitrogen oxide emissions were 8.95 million tons, with the manufacturing sector contributing 2.13 million tons, representing 23.80% of the total emissions. Given these figures, exploring the potential for government environmental information disclosure (GEID) to drive digital transformation in manufacturing enterprises is essential, as it could play a pivotal role in reducing emissions and enhancing environmental governance.
Existing research on digital transformation of companies primarily focuses on its economic and social outcomes. The driving and constraining factors of digital transformation are largely categorized into internal and external influences. Internal factors include elements such as effective corporate strategy (Mahmood et al., 2019), management commitment to digital transformation (Ko et al., 2022), digital human resources and knowledge management (Gilch and Sieweke, 2021), financial resources (Chwiłkowska-Kubala et al., 2023), asset intensity (Buck et al., 2023), and data security (Tsiavos et al., 2021). On the external side, industry characteristics (Bohnsack et al., 2018), customers and suppliers (Kraus et al., 2021), digital infrastructure (Borangiu et al., 2019), and government support policies (Bilal et al., 2024) are significant influencers. Despite these insights, there is a notable gap in the literature regarding the impact of GEID on digital transformation. This study seeks to address this gap, marking the first exploration of how GEID influences digital transformation processes in companies.
This study investigates the impact of GEID on the digital transformation of manufacturing companies, utilizing a sample of A-share listed manufacturing firms in China. The findings reveal that higher levels of local GEID are associated with greater degrees of digital transformation among manufacturing companies within those regions. To ensure the reliability of the research conclusions, this study employs local government fiscal transparency and the average level of GEID disclosure by other cities within the same province as instrumental variables to address endogeneity issues. Additionally, robustness checks were conducted by using alternative dependent variables and excluding samples from developed regions such as Beijing, Shanghai, Guangzhou, and Shenzhen, among others. The aforementioned conclusions remain consistent.
Further mechanism analysis reveals that GEID promotes the development of companies’ green strategy, increases R&D expenditure, and reduces financing constraints, thereby facilitating digital transformation. Considering that different enterprises face distinct opportunities and constraints, their processes of digital transformation exhibit heterogeneity (Ohlert et al., 2022). Therefore, we further conducted a heterogeneity analysis. The results indicate that the effect of GEID on promoting digital transformation is particularly significant in companies with poor environmental performance, low fixed asset ratios, non-state-owned companies, and since the initiation of the “Digital China” strategy in 2015. Moreover, this study finds that although the overall effect of GEID on promoting digital transformation is significant in heavily polluting industries, it is relatively weaker compared to non-heavily polluting industries. This is attributed to the higher fixed asset ratios in heavily polluting industries, which increase the difficulty of digital transformation. However, within heavily polluting industries, the effect of GEID on the digital transformation of asset-intensive companies remains stronger than in non-heavily polluting industries.
The potential contributions of our study are as follows: First, this study is the first to analyze the external drivers influencing corporate digital transformation from the perspective of government information disclosure. Existing research on the internal and external drivers or constraints of digital transformation has rarely addressed institutional factors at the government level (Jones et al., 2021; Nadkarni and Prügl, 2021). While Bilal et al. (2024) have initiated exploration into the role of government support policies, our study examines the impact of GEID as an external regulatory institutional factor on corporate digital transformation. This approach enriches the theoretical framework for understanding the drivers of digital transformation within the institutional contexts of varying intensities of government intervention.
Second, this study pioneers the exploration into how governmental environmental information governance impacts corporate digital transformation, filling a critical gap concerning the impact of GEID on aspects at the corporate level that are not directly related to green governance. Existing studies have primarily focused on areas directly related to environmental governance, such as environmental performance (Lin et al., 2021),total factor energy efficiency (Cheng et al., 2025), corporate location decisions (Lu and Li, 2020), and green innovation (Xu et al., 2024). However, given that environmental regulatory policies have the potential to fundamentally transform a country’s energy and economic structure, it is imperative to evaluate these policies from a comprehensive, economy-wide perspective (Jiang et al., 2024). This study specifically expands the scope of research on the economic consequences of GEID at the corporate level.
Third, this study reveals the heterogeneous effects of GEID on company digital transformation, particularly highlighting the differences in environmental pressures at both the individual company level and the industry level. This aspect of the research enhances our understanding of the mechanisms behind GEID and provides theoretical support and empirical evidence for more targeted policy formulation. This contribution not only broadens the depth of existing research but also offers important references for environmental governance and corporate management practices.
The structure of this study is as follows: The first section is the introduction, the second section covers theoretical analysis and research hypotheses, the third section details the research design, the fourth section presents the research results, and the final section provides the conclusion.
The disclosure of government environmental information essentially enables environmental regulatory agencies to timely acquire data on pollutant emissions from companies identified as pollution sources, and to monitor, analyze, assess, and disclose this information. To meet these requirements, companies must invest in digital pollutant emission monitoring systems. For instance, China’s “Measures for the Disclosure of Environmental Information (Trial)” which came into effect in 2008, mandates that environmental regulatory agencies proactively disclose certain governmental environmental information within their jurisdiction. This includes information on environmental quality status, environmental statistics and survey data, and the list of enterprises with pollutant emissions exceeding national or local standards or local government-assigned total emission control quotas. The “Measures for the Disclosure of Environmental Information (Trial)” also stipulates that environmental information subject to proactive disclosure should be made public within 20 working days from the date of its formation or alteration. Therefore, the GEID requires environmental regulatory agencies to timely collect data on pollutant emissions, particularly from enterprises, and process and publish this information. Such timely collection and publication of pollutant information are challenging without the use of online environmental monitoring systems and other information and communication technologies (ICT) (Schlæger and Zhou, 2019). In fact, ICT itself is a significant driver for local governments to carry out environmental governance information (Mol, 2009). Furthermore, the ICT nature of GEID imposes digital transformation requirements on the polluting companies, demanding the collection of real-time data on production and emissions for monitoring, analysis, and processing by regulatory agencies, thus promoting the companies’ digital transformation. In reality, the driving forces behind company digital transformation can also stem from external stakeholders (Jones et al., 2021). For example, Hanelt et al. (2021) found that consumers are increasingly incorporating technology into their daily routines and personal interactions, which drives the need for communication between consumers and companies and, consequently, promotes the digital transformation of companies.
The disclosure of government environmental information plays a pivotal role in fostering the development of company green strategy and subsequently encourages companies to leverage digital transformation for environmental governance. Corporate governance refers to the institutional arrangements designed to determine and control the strategic direction and performance of organizations, with the core objective being to ensure the effectiveness of strategic management (Davis et al., 1997). External stakeholders can participate in corporate governance through various institutional mechanisms (Hillman and Dalziel, 2003). As an information-based instrument, GEID empowers the public, investors, and other stakeholders to access data on corporate pollutant emissions and environmental governance practices. This transparency facilitates stakeholder collaboration in environmental governance, thereby intensifying external pressure on corporate environmental management (Attard et al., 2015). Given the issue of government-enterprise collusion in environmental governance (Wang et al., 2024), the role of information-based instruments in mobilizing stakeholder participation becomes particularly crucial. For example, Li et al. (2024) found that public participation in monitoring can effectively deter local governments and enterprises from colluding, which significantly increases the level of effort both parties invest in the green transition.
Therefore, The legitimacy pressure exerted by stakeholders directly influences corporate governance and the formulation of green development strategies (Gago and Antolin, 2004), which, in turn, prompts companies to invest in digital transformation for enhanced environmental governance. For instance, Fan et al. (2024) found that rising public environmental concern significantly influences the advancement of corporate digital transformation. Therefore, the collaborative environmental governance driven by the disclosure of government environmental information advances the construction of company green strategy and, consequently, promotes digital transformation.
The disclosure of government environmental information also helps to alleviate company financing constraints, which is a critical factor influencing digital transformation (Chwiłkowska-Kubala et al., 2023) and environmental governance. For example, Chen and Li (2023) found that when funding assistance does not exceed a certain threshold, companies tend to prefer green funding assistance. This is because the emission reduction efficiency and profits of enterprises receiving green funding are higher than those of enterprises investing in green technology alone. By reducing information asymmetry between companies and capital providers, the disclosure can effectively lower the investment risk for capital providers, reduce the required risk premium, and consequently decrease company financing costs and constraints (Fazzari et al., 1987). Existing research indicates that the disclosure of corporate environmental information can reduce information asymmetry between investors and companies, thereby lowering financing costs, including both debt (Raimo et al., 2021) and equity (Fonseka et al., 2019). Thus, the financing advantages brought about by GEID facilitate the capital investment needed for digital transformation, thereby supporting companies in their digitalization efforts.
The disclosure of government environmental information also promotes company research and development (R&D) innovation, which is a crucial factor ensuring the success of digital transformation (Gilch and Sieweke, 2021). As previously mentioned, the disclosure reduces information asymmetry between companies and external stakeholders, thereby increasing the cost of rent-seeking by exposing the behaviors of local governments and polluters. Both governmental regulatory actions and company pollution activities become more susceptible to public scrutiny. Considering that green and technological innovations have significantly improved resource and energy efficiency (Yang et al., 2024), the context of governmental environmental information disclosure further incentivizes companies to invest in R&D to enhance their pollution control capabilities and stimulate product innovation, rather than resorting to collusion with government entities. This strategy mitigates the potential negative impacts of environmental regulations on corporate environmental performance, consistent with the Porter Hypothesis, which posits that stringent environmental regulations can drive innovation, yielding both economic and environmental benefits (Porter and Van der Linde, 1995). Moreover, Brown and Martinsson (2019) indicate that information transparency encourages R&D innovation activities within companies. As a result, firms are more likely to achieve digital transformation through innovation, thereby fulfilling environmental regulatory requirements. Based on the above analysis, this study proposes Research Hypothesis 1.
Hypothesis 1. Higher levels of environmental information disclosure by local governments promote the digital transformation of local manufacturing companies.
Psychological research indicates that negative information has a greater impact on humans than positive information and is more likely to elicit emotional responses. These bias leads society to focus more intently on and scrutinize negative information (Baumeister et al., 2001). In the context of corporate environmental information disclosure, most companies are eager to present themselves as “green”, “sustainable”, or “socially responsible” by making overly positive and optimistic corporate social responsibility (CSR) disclosures, while failures, setbacks, and mistakes are usually withheld (Jahn and Brühl, 2019). In contrast, local governments, driven by the need to meet stakeholder demands, are more willing than enterprises to disclose negative environmental information about companies, such as details on environmental emergencies (Li et al., 2017). Consequently, GEID amplifies the environmental pressures on companies with poor environmental performance.
Legitimacy theory posits that corporate actions and decisions must conform to societal, cultural, and legal norms to gain social acceptance and support (Suchman, 1995). As concerns about environmental pollution and carbon dioxide emissions have grown globally, environmental regulations have become increasingly stringent. Companies with poor environmental performance face mounting regulatory pressures from governments, society, and other stakeholders. This pressure compels companies to adopt environmental governance measures to comply with environmental regulations, achieve legitimacy, and avoid fines and litigation. Digital technologies such as the Internet of Things (IoT), big data analytics, and artificial intelligence (AI) can monitor and analyze resource usage in real-time during production processes. By optimizing production flows and improving resource utilization efficiency, companies can significantly reduce energy consumption and waste emissions (Porter and Heppelmann, 2014), thereby enhancing corporate environmental performance (Chen and Hao, 2022). Therefore, we hypothesize that the effect of GEID on promoting digital transformation is particularly pronounced in companies with poor environmental performance. Based on this reasoning, we propose Research Hypothesis 2:
Hypothesis 2. The effect of local government environmental information disclosure in promoting the digital transformation of manufacturing companies is especially significant for those with poor environmental performance.
Asset-intensive enterprises possess long-lived and high-cost fixed assets, making them typically difficult to adjust and retrofit (Wang, 2018; Woodward, 1997). Their digital transformation involves complex production equipment and processes, which require higher technical, investment, and safety considerations (Gao et al., 2019). Buck et al. (2023) conducted interviews with elite informants at seven leading asset-intensive companies in the Australian mining industry and found that the digital transformation progress of asset-intensive companies lags significantly behind that of asset-light ones.
Moreover, company digital transformation usually encompasses both process digital transformation and product and service digital transformation (Duraivelu, 2022). The product and service digital transformation typically demands lower technical and investment costs; hence it often precedes process digital transformation in manufacturing companies (Blichfeldt and Faullant, 2021). Additionally, asset-intensive enterprises rarely interact directly with end consumers, face a limited number of clients, and possess relatively stable organizational structures (Gebauer et al., 2020). They focus more on competitive production processes, further delaying their overall digital transformation progress.
Although, as previously mentioned, GEID aids in promoting company digital transformation, its impact varies significantly between asset-light and asset-intensive enterprises. The costs, risks, and technical challenges associated with digital transformation are particularly burdensome for asset-intensive companies, which can inhibit the positive effects of GEID on their transformation efforts. In contrast, asset-light enterprises are better positioned to leverage the impetus provided by such disclosures. Based on this reasoning, we propose Research Hypothesis 3.
Hypothesis 3. The effect of local government environmental information disclosure in promoting the digital transformation of manufacturing companies is especially significant for asset-light ones.
Due to differences in property relations between state-owned companies (SOEs) and non-state-owned ones (non-SOEs) with the government, there exists a problem of selective enforcement by environmental regulatory agencies. This leads to varying levels of environmental pressure on SOEs and non-SOEs under similar conditions. For instance, Chen et al. (2011) found that the China Securities Regulatory Commission (CSRC) considers whether companies violating the rules have a state-owned background and the strength of that background when investigating and punishing non-compliance. After controlling for the degree of violation, SOEs are punished less severely than private companies, and the higher the hierarchy of the SOE in question, the less severe the punishment.
Zhu et al. (2022) also examined corporate environmental governance behaviors in the context of the new Environmental Protection Law in China and found that environmental regulations have a greater impact on the environmental governance behaviors of non-SOEs compared to SOEs. GEID, as an environmental information-based instrument, serves as an effective complement to formal environmental regulatory tools. However, there may also be issues of selective disclosure of corporate environmental information, particularly concerning environmental violations, which imposes greater social environmental regulatory pressure on non-SOEs. Therefore, it can be inferred that the governance impact of GEID on non-SOEs will exceed its impact on SOEs. Based on this reasoning, we propose Research Hypothesis 4.
Hypothesis 4. The effect of local government environmental information disclosure in promoting the digital transformation of manufacturing companies is especially significant for non-state-owned ones.
Government policy support is a crucial driver for company digital transformation. Wang et al. (2023), using firm-level data from China, found that government subsidies and tax incentives positively influence company digital transformation. Additionally, the government’s efforts in building digital infrastructure, providing digital human resources, and constructing digital collaboration ecosystems all facilitate corporate digital transformation by reducing associated costs (Chen et al., 2021).
The overarching design of the “Digital China” initiative can be traced back to 2015, when China’s top leader, President Xi Jinping, formally proposed advancing the construction of “Digital China” during the opening ceremony of the second World Internet Conference. Since then, the “Digital China” strategy has been continuously promoted, with various fiscal, financial, investment, and technological support policies being introduced. Within this “Digital China” context, the extent of local GEID not only increases company sensitivity to environmental requirements but also, when combined with digital construction efforts, promotes digital transformation in environmental compliance and operational efficiency. Accordingly, we propose Research Hypothesis 5.
Hypothesis 5. The effect of local government environmental information disclosure in promoting the digital transformation of manufacturing companies is especially significant since 2015.
Overall, the research framework for this study is illustrated in Figure 1. Based on Research Hypothesis 1, the study initially examines the impact of GEID on corporate digital transformation. It further investigates the mechanisms of action involving the development of corporate green strategies, promotion of R&D expenditure, and reduction of financing constraints. In line with Research Hypotheses 2 through 5, we also explore the moderating effects of corporate environmental performance, fixed asset ratio, ownership structure, and the post-2015 period on the relationship between GEID and digital transformation.
As previously mentioned, the manufacturing sector is a significant contributor to major pollutant emissions in society. Therefore, this study examines the impact of GEID on the digital transformation of manufacturing companies, using a sample of publicly listed manufacturing companies on China’s A-share market. The primary independent variable in this study, GEID, is derived from PITI index compiled by the Institute of Public and Environmental Affairs (IPE) and the Natural Resources Defense Council (NRDC). This index is available for the years 2008 to20191, thus the study period is limited to these years.
To ensure data quality and consistency, the following samples were excluded: (1) companies flagged as Special Treatment (ST) or Particular Transfer (PT) during 2008–2019; (2) company-year samples for the year of the initial public offering (IPO); (3) industries with fewer than 10 samples; and (4) samples with missing values for any of the dependent, independent, or control variables. After applying these criteria, the final dataset comprises 12,254 samples from 1,850 companies covering the period from 2008 to 2019.
The dependent variable in this study is the company’s digital transformation (DT). According to Fan et al. (2024), we use the digital transformation index from the China Stock Market and Accounting Research (CSMAR) database to measure the extent of company’s digital transformation. The CSMAR database constructs a digital transformation index for all A-share listed companies in China. This index encompasses five dimensions: artificial intelligence (AI) technology, blockchain technology, cloud computing technology, big data technology, and digital technology applications. Specifically, the dimension of AI technology includes 15 keywords such as artificial intelligence, business intelligence, image understanding, investment decision support systems, intelligent data analysis, intelligent robots, machine learning, deep learning, semantic search, biometric technology, facial recognition, voice recognition, identity verification, autonomous driving, and natural language processing. The blockchain technology dimension includes 8 keywords; the cloud computing technology dimension includes 13 keywords; the big data technology dimension includes 9 keywords; and the digital technology applications dimension includes 34 keywords.
The CSMAR database analyzes the textual information in the annual reports of listed companies, particularly the management’s discussion and analysis section. It searches, matches, and counts the frequency of the aforementioned keywords across the five dimensions. The word frequencies for AI technology, blockchain technology, cloud computing technology, big data technology, and digital technology applications are then aggregated to form a comprehensive digital transformation index. A higher index value indicates a higher degree of digital transformation in the company. To ensure the normal distribution of the digital transformation variable, we log-transform the index value by taking the natural logarithm after adding one.
The independent variable in this study is government environmental information disclosure (GEID). This is measured using the Pollution Information Transparency Index (PITI) from the “Urban Pollution Source Regulatory Information Disclosure Index Report”, jointly compiled by the Institute of Public and Environmental Affairs (IPE) and the Natural Resources Defense Council (NRDC). The report evaluates the transparency of pollution source regulatory information disclosure by environmental regulatory agencies of 120 cities (113 cities prior to 2012) from 2008 to 2019. The evaluation criteria include five primary indicators and eight secondary indicators, assessing information disclosure in terms of “Regulatory Information”, “Self-Monitoring”, “Interactive Responses”, “Emission Data”, and “Environmental Impact Assessment (EIA) Information”.
In this study, we use the annual PITI score of each city as the corresponding value for GEID in that city. The range of PITI values is 0–100 points. A higher PITI score indicates a higher level of transparency in environmental information disclosure by the government in that city. The PITI index has been widely used in research on governmental environmental information transparency at the city level in China (Pan et al., 2023; Yu and Jin, 2022; Zhong et al., 2021).
Drawing on existing literature, we selected a series of firm-level characteristic variables, including firm size (SIZE), leverage (LEV), proportion of fixed assets (PFA), cash holdings (CASH), profitability (ROA), environmental performance (EP), investment opportunities (TOBINQ), R&D intensity (RD), ownership structure (SOE), and listing age (AGE). The specific definitions of the variables are shown in Table 1. Among them, environmental performance is represented by the environmental score in the ESG rating of listed companies, sourced from the China National Research Data Service (CNRDS) database. This rating system integrates international ESG disclosure standards (ISO 26000, GRI Standards, SASB Standards) and relevant Chinese ESG disclosure policies, including four secondary indicators (climate change, pollution control, circular economy, environmental risks) and seven tertiary indicators.
Additionally, considering that command-and-control environmental regulations may also impact company digital transformation, we control for local government environmental regulatory intensity (ENVREG). Following the methodology of Chen et al. (2018), we collected annual government work reports from various cities and counted the frequency of environment-related keywords, log-transforming this frequency after adding one. The keywords include environmental protection, pollution, energy consumption, emission reduction, pollution discharge, ecology, green, low carbon, air, chemical oxygen demand, sulfur dioxide, carbon dioxide, PM10, and PM2.5. Higher values indicate stronger local government environmental regulatory intensity. Given that enhancing company competitiveness is a primary rationale for promoting digital transformation (Mihu et al., 2023), we also control for industry competition (HHI). Finally, we include industry and year fixed effects. Unless otherwise specified, the values for all the aforementioned control variables are sourced from China’s CSMAR database. Definitions for the main variables can be found in Table 1.
Table 2 provides the descriptive statistics for the main variables. To mitigate the impact of outliers, all continuous variables are winsorized at the 1% and 99% levels.
Given that the main independent variable, GEID, is a regional-level variable with relatively small annual variation, using a fixed effects model may amplify the measurement error of this variable. Research by Hill et al. (2020) also indicates that fixed effects models are not suitable for estimating coefficients of variables with minimal within-group variation or slow temporal changes. Therefore, this study employs an OLS regression model as the basis to construct Model (1) for testing Hypothesis 1:
The subscripts i, t, and c denote the company, year, and city, respectively. The dependent variable DTi,t represents the level of digital transformation for company i in year t; the independent variable GEIDc,i,t specifies the degree of government environmental information disclosure in the city c where company i is located during year t. Control variables include firm-level characteristics, regional factors (such as local government environmental regulatory intensity), and industry-level factors (such as industry concentration), as previously described. “Industry” and “Year” represent industry and year fixed effects, respectively.
Table 3 reports the results of the baseline regression based on Model 1. Column (1) of Table 2 displays the regression result including only the independent variable GEID, along with industry and year fixed effects. Column (2) includes all control variables but excludes industry and year fixed effects. Column (3) incorporates all independent variables, control variables, as well as industry and year fixed effects. The values of Variance Inflation Factor (VIF) in Columns (1)–(3) are below 10, indicating no multicollinearity issues among the independent variables. The results show that the coefficient of GEID is significantly positive, suggesting that higher levels of government environmental information disclosure are associated with a greater degree of digital transformation in companies. In terms of the specific magnitude of impact, since corporate digital transformation (DT) is the natural logarithm of the original value plus one, a one-point increase in the GEID value leads to a 0.31% increase in the original value of DT. This result validates Hypothesis 1, confirming that GEID has facilitated the digital transformation of manufacturing firms.
From Table 3, we also observe that the intensity of government environmental regulations (ENVREG) significantly promotes company digital transformation. This aligns with the findings of Yu et al. (2023), who discovered that regional environmental regulations significantly enhance the digital transformation of energy companies. Additionally, the coefficient for industry competition (HHI) is significantly negative, suggesting that firms in more competitive industries are more likely to pursue digital transformation to gain a competitive advantage. This finding is consistent with the results of Leão and da Silva (2021). Furthermore, our analysis reveals that the coefficient for the proportion of fixed assets (PFA) is significantly negative. This suggests that asset-intensive companies face higher costs for digital transformation, thereby constraining their ability to undertake such initiatives, consistent with the findings of Buck et al. (2023).
Moreover, the coefficient for R&D intensity (RD) is significantly positive, affirming that company digital transformation necessitates robust innovation capabilities (Gilch and Sieweke, 2021). The coefficient for ownership structure (SOE) is significantly negative, indicating that non-SOE companies are more likely to pursue digital transformation. Similarly, the coefficient of environmental pressure (EP) is significantly negative, suggesting that companies facing greater external environmental pressures are more inclined towards digital transformation. In addition, we find that company size (SIZE) and leverage (LEV) both have a significantly positive impact on company digital transformation.
The baseline regression model in this study primarily controls for the influence of the intensity of urban environmental regulation, which is a regional macroeconomic factor. As observed in column (3) of Table 3, the local government environmental regulatory intensity where a firm is located significantly affects the firm’s digital transformation. Considering that a firm’s digital transformation may also be influenced by the level of economic development and the level of digital economy development in its location, we further introduce two control variables in column (4) of Table 3: the city’s economic development level and the city’s digital economy development level. The city’s economic development level (GDP) is expressed in terms of per capita GDP in thousands of RMB. The city’s digital economy development level (DIGE) is measured based on the availability of relevant data at the city level, encompassing two main aspects: internet development and digital financial inclusion. The measurement of urban internet development includes several indicators: the number of broadband internet users per hundred people, the proportion of employees in computer services and software industries relative to urban unit employees, per capita telecommunication business volume, and the number of mobile phone users per hundred people. The raw data for these indicators are sourced from the “China City Statistical Yearbook”. As for the measurement of urban digital financial development, the China Digital Financial Inclusion Index is utilized, which is jointly compiled by the Digital Finance Research Center of Peking University and Ant Financial Group. The comprehensive index of digital economy development is obtained through principal component analysis, which standardizes and reduces the dimensionality of the data from the aforementioned five indicators.
Column (4) of Table 3 shows that both the GDP and DIGE variables significantly influence firms’ digital transformation, further supporting Hypothesis 1 of this study. However, compared to column (3) of Table 3, the inclusion of the GDP and DIGE control variables increases the model’s R2 by only 0.0018, which corresponds to an increase of merely 0.48%. This indicates that the additional explanatory power for the dependent variable is minimal. Furthermore, the maximum Variance Inflation Factor (VIF) for the variables in column (4) is 11.52, exceeding the threshold value of 10. This could be due to the fact that the ENVREG has already absorbed the variance of the GDP and DIGE variables, indicating a degree of multicollinearity. Therefore, to ensure the robustness of the research conclusions, the variables of GDP and DIGE will not be controlled for in subsequent analyses.
Additionally, this study presents a scatter plot of GEID versus DT in Figure 2, where DT values represent the group-wise averages of GEID. Figure 2 demonstrates a positive correlation between GEID and DT, consistent with Hypothesis 1.
Although GEID is a city-level variable, and it is unlikely that digital transformation behaviors at the firm level would influence city-level government environmental information disclosure, the baseline regression model in this study may suffer from omitted variable bias, potentially leading to endogeneity issues. To address this, we employ two instrumental variables to mitigate the potential endogeneity problem.
First, we utilize the city’s fiscal transparency (FT) as an instrumental variable for GEID. Since both local fiscal agency and environmental regulatory agency are subject to the administrative influence of their respective local government, the degree of information disclosure by these agencies is correlated. However, the transparency of fiscal agency does not directly impact the digital transformation of companies in the locality. Therefore, using city-level fiscal transparency as an instrumental variable for GEID is appropriate. The data on fiscal transparency are sourced from the “China Municipal Government Fiscal Transparency Report”, which has been published annually since 2013 by the Public Economics, Finance, and Governance Research Center at the School of Public Policy and Management, Tsinghua University.
Second, following the methodology proposed by Chong et al. (2013), we use the average GEID of other cities within the same province (AVERAGEGEID) as an instrumental variable for the GEID of the city where the company is located. Given the characteristics of China’s administrative system, cities within the same province exhibit certain similarities in environmental information governance. However, the GEID of other cities within the same province does not directly impact the digital transformation of companies in the focal city. Therefore, the average GEID of other cities within the same province meets the requirements for being an instrumental variable. Moreover, GEID, as an information-based instrument, is generally considered an informal environmental regulation (Jamalpuria, 2013). However, it may also be influenced by formal environmental regulations. To account for this, we additionally use both the intensity of local government environmental regulation and the average GEID of other cities within the same province as instrumental variables for GEID in the endogeneity testing.
Table 4 presents the results of the 2SLS tests using instrumental variables. The p-values of the Sargan statistic for all models exceed 0.05, suggesting that there is no overidentification problem with the instrumental variables. Furthermore, the First-stage F-statistic values for each type of instrumental variable significantly surpass 10, indicating that there is no weak instrument problem. Therefore, the instrumental variables used in this study are appropriate.
Columns (1) and (2) in Table 4 display the first-stage and second-stage results, respectively, when using local fiscal transparency (FT) as an instrumental variable. From Column (1), we observe that the coefficient for local is significantly positive, indicating a positive correlation between FT and GEID. In Column (2), IVGEID represents the predicted value of GEID calculated from the first-stage regression. The coefficient for IVGEID remains significantly positive, suggesting that GEID indeed facilitates company digital transformation, thereby passing the endogeneity test with the instrumental variable.
Column (3) in Table 4 illustrates the first-stage results using the average GEID of other cities within the same province as the instrumental variable. The coefficient for AVERAGEGEID is significantly positive, indicating a positive correlation with GEID. Additionally, the coefficient for the local government environmental regulation intensity is also significantly positive, suggesting a correlation with GEID as well. Column (4) presents the second-stage regression results when using AVERAGEGEID as the sole instrumental variable. The coefficient for IVGEID remains significantly positive, confirming that the average GEID of other cities within the same province, used as an instrumental variable, still supports the hypothesis that GEID facilitates company digital transformation. Column (5) shows the second-stage results when both the average GEID of other cities within the same province and the local government environmental regulation intensity are used as instrumental variables. The coefficient for IVGEID continues to be significantly positive, further validating Hypothesis 1 that GEID contributes to company digital transformation.
The data used to measure the Dt variable in this article is sourced from the CSMAR database. This data is derived by mining and analyzing the frequency of keywords related to digital transformation found in the annual reports of listed companies, particularly within the management’s discussion and analysis section. To ensure the robustness of our research results, we further utilize an annual index from the CSMAR database that specifically categorizes management’s orientation towards digital innovation (DT_ma1) to measure corporate digital transformation. This index is solely based on keywords extracted from the management’s discussion and analysis section of the annual reports. Additionally, we refine our approach by limiting the digital transformation keywords to those related to production and environmental protection. These keywords only include “Industrial Internet”, “Internet of Things”, “smart robotics”, “smart environmental protection”, “smart grid”, “smart energy”, and “green computing”. These are used to construct a new digital transformation variable, DT_ma2. Both variables, DT_ma1 and DT_ma2, are processed by adding one to the keyword frequency count and then applying a natural logarithm transformation. We conducted the regression again using the dependent variables DT_ma1 and DT_ma2, and the results are presented in columns 1 and 2 of Table 5.
From columns (1) and (2) of Table 5, it can be observed that the coefficient of GEID remains significantly positive. This indicates that Hypothesis 1 still holds true even when the method for measuring the dependent variable is altered.
Considering that the impact of current GEID on company digital transformation may not immediately influence the current year’s digital transformation investments, there is a possibility of a time lag. Therefore, we conduct a regression analysis using the one-year-ahead value of company digital transformation as the dependent variable. The results are presented in Column (3) of Table 5. From Column (3) of Table 5, we observe that the regression coefficient of GEID remains significantly positive. This indicates that the current GEID continues to have a significant positive effect on the digital transformation of companies in the subsequent period, thereby further validating Hypothesis 1.
Beijing, Shanghai, Guangzhou, and Shenzhen are the most developed cities in China and attract a significant number of companies to register their headquarters. Some manufacturing companies establish their headquarters in these four cities while situating their production bases in other locations. Consequently, such companies may be influenced by the GEID of both their headquarters and production base locations. To ensure the robustness of our results, we exclude firms registered in Beijing, Shanghai, Guangzhou, and Shenzhen and re-run the regression analysis. The results are presented in Columns (4) and (5) of Table 5.
In Column (4), the dependent variable represents the projected value of company digital transformation 1 year into the future, whereas in Column (5), it denotes the current value of digital transformation. From both columns, we observe that the coefficient for GEID remains significantly positive, indicating that Hypothesis 1 continues to hold.
Based on the theoretical analysis presented earlier, GEID reduces information asymmetry between company and external stakeholders, facilitating the latter’s participation in corporate environmental co-governance. This, in turn, aids company digital transformation, which is beneficial for environmental governance (Chen and Hao, 2022). To explore this logic chain, we first examine whether GEID promotes the development of corporate green strategy, subsequently influencing digital transformation. Moreover, green innovation itself is also considered a strategy to achieve environmental protection and economic growth (Taklo et al., 2021). Therefore, we further examine whether green innovation serves as a mediating variable in GEID’s promotion of corporate digital transformation.
Additionally, given that innovative knowledge management capabilities are crucial for the success of company digital transformation (Gilch and Sieweke, 2021), we further investigate whether GEID fosters R&D expenditure, thereby supporting digital transformation. Moreover, financial resources are also a critical factor in ensuring successful digital transformation (Chwiłkowska-Kubala et al., 2023). Theoretically, GEID reduces information asymmetry between company and external stakeholders, potentially alleviating financing constraints. Therefore, we also examine whether GEID can reduce companies’ financing constraints, thereby promoting digital transformation.
Hence, this study investigates the mechanisms through which GEID promotes corporate digital transformation. Specifically, it examines three channels: the development of corporate green strategy and green innovation, the promotion of R&D expenditure, and the reduction of financing constraints. For the mediating variable of corporate green strategy (GS), we utilize Python’s regular expression lazy matching algorithm to analyze the textual information in the “Outlook for Future Development” section of listed companies’ annual reports. Keywords such as “green”, “environmental”, “sustainable”, “environment”, and “low-carbon” are matched with the keyword “strategy” without sentence breaks. The occurrence count of the matched green strategy keywords is incremented by one and then log-transformed, with higher values indicating a stronger emphasis on corporate green strategy development. The mediating variable for corporate green innovation (GI) is quantified by taking the logarithm of one plus the number of green invention patent applications submitted by the firm. These green patents are defined in accordance with the IPC Green Inventory. For the mediating variable representing R&D expenditure (RD), as defined earlier, it is calculated as the annual R&D expenditure divided by operating revenue.
According to Hadlock and Pierce (2010) and Li et al. (2023), we employ the FC index as a measure of corporate financing constraint. The data utilized for this calculation are sourced from the China Stock Market and Accounting Research (CSMAR) database. The calculation process involves two primary steps: first, we standardize three variables—firm size, firm age, and cash dividend payout ratio—on an annual basis, and then rank the listed companies in ascending order based on the mean values of these standardized variables. The financing constraint dummy variable QUFC is determined by using the upper and lower tertiles as cut-off points; companies above the 66th percentile are classified as having low financing constraints (QUFC = 0), while those below the 33rd percentile are classified as having high financing constraints (QUFC = 1). Second, we perform a Logit regression as specified in Models 2, 3 to fit the probability (P) of financing constraints occurring for each firm annually. This probability (P) is defined as the financing constraint index Fc, which ranges from 0 to 1, with higher Fc values indicating more severe financing constraints.
The definitions of Size and Lev are consistent with those previously mentioned; CasℎDiv denotes the cash dividends distributed by the company in the current year; Mb refers to the market-to-book ratio, calculated as market value divided by book value; NWC represents net working capital, defined as working capital minus cash equivalents and short-term investments; Ebit stands for earnings before interest and taxes; and Ta signifies total assets.
To test the mediation effect, this study employs both the Sobel and Bootstrap methods. The Sobel test involves constructing two regression models: one regresses the independent variable on the mediator, and the other regresses both the independent variable and the mediator on the dependent variable. Path coefficients and their standard errors from these models are used to calculate the Z-value; a significant Z-value indicates a significant mediation effect. The Bootstrap method, similar to the Sobel test, involves repeated sampling to construct a 95% bias-corrected confidence interval. If this interval does not include zero, the mediation effect is considered significant. We conduct mediation effect tests using the Bootstrap method with 1,000 resamples.
Table 6 provides a comprehensive analysis of the mediation effects in the context of GEID’s influence on digital transformation. Initially, Column (1) reveals a significantly positive coefficient for GEID on the mediator variable GS, indicating that GEID positively impacts the development of corporate green strategies. This is further supported in Column (2), where the effects of GEID and GS on the dependent variable DT are examined. The positive coefficient for GEID, combined with a significant Sobel Z-value and a Bootstrap bias-corrected confidence interval that excludes zero, confirms the mediating role of corporate green strategy in GEID’s impact on digital transformation. This finding is consistent with the results of Xu et al. (2024). Similarly, Columns (3) and (4) demonstrate that green innovation (GI) acts as a mediator in the GEID-digital transformation relationship. Columns (5) and (6) highlight the mediation effect of R&D expenditure (RD), showing that GEID promotes corporate R&D spending, with the Sobel and Bootstrap tests validating significant indirect effects. Lastly, Columns (7) and (8) focus on the mediation effect of financial constraint (FC), where a significantly negative coefficient for GEID indicates its role in reducing financial constraints. Both the Sobel and Bootstrap tests again confirm significant indirect effects, reinforcing the mediation role of financial constraint.
To test hypotheses 2 through 5, we extended Model (1) by adding interaction terms, specifically GEID with company environmental performance (GEID*EP), fixed asset ratio (GEID*PFA), ownership structure (GEID*SOE), and post-2015 indicator (GEID*Year 2015). The definitions of EP, PFA, and SOE are consistent with those previously mentioned. The post-2015 variable Year 2015 is a dummy variable that takes the value of 1 for observations from the year 2015 onwards, and 0 otherwise. The regression results are presented in Table 7.
Column (1) of Table 7 shows a significantly negative coefficient for the interaction term GEID*EP, indicating that the effect of GEID on digital transformation is more pronounced for companies with poorer environmental performance, thereby validating Hypothesis 2. Column (2) presents a significantly negative coefficient for the interaction term GEID*PFA, suggesting that the effect of GEID on digital transformation is more significant for companies with a lower proportion of fixed assets, thus supporting Hypothesis 3. Column (3) reveals a significantly negative coefficient for the interaction term GEID*SOE, indicating that the effect of GEID on digital transformation is more substantial for non-state-owned companies, validating Hypothesis 4. Finally, Column (4) shows a significantly positive coefficient for the interaction term GEID*Year 2015, suggesting that the effect of GEID on digital transformation is particularly significant in the years 2015 and onwards, thereby supporting Hypothesis 5.
It is noteworthy that in 2015, the Chinese government revised the Environmental Protection Law, a policy that enhanced the environmental information disclosure requirements for local governments. This legislative change can be regarded as an exogenous shock. By using the interaction between the GEID variable and the Year 2015 variable in a difference-in-differences (DID) model, we can assess its impact. Column 4 of Table 7 essentially presents the results of this DID model, providing further validation for Hypothesis 1 of this study.
Our previous analysis demonstrated the positive impact of GEID on company digital transformation, particularly pronounced in firms with poorer environmental performance, which face greater environmental pressure. Given the significant differences in environmental pressure between heavily polluting and non-heavily polluting industries, we further investigate whether the effect of GEID on company digital transformation varies between these industry categories. To examine industry-specific heterogeneity effects, we extend Model (1) by including an interaction term between GEID and the dummy variable for heavily polluting industries (HPI). The classification of heavily polluting industries follows the 2012 revision of the “Guidelines for Industry Classification of Listed Companies” by the China Securities Regulatory Commission, the 2008 “Classification of Industries for Environmental Protection Verification of Listed Companies” by the Ministry of Environmental Protection, and the “Guidelines for Environmental Information Disclosure of Listed Companies”. These classifications encompass industries such as steel, cement, electrolytic aluminum, coal, metallurgy, chemicals, petrochemicals, building materials, paper, brewing, pharmaceuticals, fermentation, textiles, and tanning. If a company’s industry belongs to one of these categories, HPI is set to 1; otherwise, it is 0. The results of this heterogeneity analysis are presented in Columns (1) to (3) of Table 8.
Column (1) of Table 8 presents the full-sample regression results including the interaction term GEID*HPI, indicating a significantly negative coefficient for GEID*HPI. This suggests that the positive impact of GEID on company digital transformation is particularly pronounced in non-heavily polluting industries. Columns (2) and (3) of Table 8 display the subsample regression results for heavily polluting and non-heavily polluting industries, respectively. Although GEID significantly promotes digital transformation in heavily polluting industries, the Chow test indicates that its effect is smaller compared to non-heavily polluting industries.
Considering our previous conclusion that the positive influence of GEID on digital transformation is more pronounced in companies with weaker environmental performance—those facing more substantial environmental pressure—why is this effect not evident in heavily polluting industries? One possible explanation is that the digital transformation of fixed assets involves complex production equipment and processes, requiring higher technical and investment costs, as well as safety considerations (Gao et al., 2019). These factors directly constrain the digital transformation process of asset-intensive enterprises (Buck et al., 2023), as also suggested by the baseline regression results in Table 3, which indicate that Pfa significantly hinders corporate digital transformation2. Therefore, we posit that the higher proportion of fixed assets in heavily polluting industries is a crucial factor delaying their digital transformation process, thereby weakening the effect of GEID.
To examine whether there is a significant difference in the proportion of fixed assets between heavily polluting and non-heavily polluting industries, we conducted both T-tests and Wilcoxon tests. The results are presented in Table 9.
As observed in Table 9, the mean and median values of PFA for companies in heavily polluting industries are significantly higher than those in non-heavily polluting industries. Conversely, for DT, the mean and median values are significantly higher for companies in non-heavily polluting industries. These findings validate the reasons for the differences in the effect of GEID on company digital transformation between heavily and non-heavily polluting industries.
Given the fundamental logic of our research hypotheses—that GEID promotes corporate environmental governance and that digital transformation aids environmental governance—we posit that GEID positively impacts company digital transformation. Therefore, under equal fixed asset ratios, the effect of GEID on digital transformation should be more pronounced in heavily polluting industries. To further examine this, we incorporate the interaction term among GEID, PFA, and HPI (GEID*PFA*HPI) into Model (1).
Column (4) of Table 8 presents the full-sample regression results for the GEID*PFA*HPI interaction term, showing a significantly positive coefficient. This indicates that, under equal fixed asset ratios, GEID’s positive impact on digital transformation remains more significant in heavily polluting industries. Columns (5) and (6) present the subsample regression results for heavily and non-heavily polluting industries, respectively. The results reveal that, while the impact of GEID on promoting digital transformation is inhibited by PFA in both industry types3, the Chow test shows that this inhibitory effect is significantly smaller in heavily polluting industries. In other words, GEID has a more substantial effect on digital transformation for companies with the same fixed asset ratio in heavily polluting industries than in non-heavily polluting industries. Alternatively, this can be interpreted as the positive impact of GEID on the digital transformation of asset-intensive companies being more prominent in heavily polluting industries. The results in Columns (4) to (6) of Table 8, considering the fixed asset ratio in depth, further validate the positive impact of GEID on company digital transformation.
Company digital transformation is crucial for effective environmental governance. Government environmental information disclosure aids this process by fostering collaborative governance, reducing information asymmetry, and alleviating financing constraint. Using data from A-share listed manufacturing companies in China from 2008 to 2019, our study finds that stronger local GEID significantly promotes company digital transformation. This effect remains robust under various endogeneity and robustness checks. Further analysis shows that the disclosure primarily facilitates digital transformation through constructed green strategy, increased R&D expenditure, and reduced financing constraint. The positive impact is notably stronger in companies with poor environmental performance, low fixed asset ratios, non-state-owned, and during the post-2015 period. Moreover, although heavy asset intensity is a significant constraint on company digital transformation, the effect of GEID on the digital transformation of asset-intensive enterprises is still more pronounced in heavily polluting industries compared to non-heavily polluting industries. Overall, this study robustly demonstrates the significant influence of GEID as an information governance tool in promoting company digital transformation and green development. The findings enrich the theoretical research on digital transformation and environmental governance while providing empirical evidence to inform government environmental policy and corporate strategic adjustments. According to the above analysis, we propose the following policy recommendations.
Firstly, the design of government environmental policies should consider the complex and multifaceted economic, environmental, and social impacts that may result from regulatory measures. While this study primarily focuses on GEID, it uncovers its significant impact on company digital transformation, illustrating the interconnected nature of environmental policies and technological advancement. Therefore, policymakers should adopt a holistic approach, analyzing potential outcomes from multiple dimensions. This involves considering how such policies can not only achieve their primary environmental objectives but also drive technological innovation and business transformation. By doing so, they can aim for a strategy that achieves “one stone, two birds” or even “one stone, multiple birds”, where a single policy initiative generates a range of positive outcomes, such as enhanced competitiveness, improved sustainability, and increased economic resilience. This comprehensive analysis will help ensure that policies are not only effective in addressing environmental concerns but also beneficial in fostering broader economic and technological progress.
Secondly, governments can strategically utilize environmental information disclosure as a vital instrument not only for enhancing environmental governance but also for driving company digital transformation. By mandating transparency and accountability in environmental practices, governments can incentivize companies to adopt digital technologies that improve their operational efficiency and reduce their environmental footprint. This dual approach not only strengthens regulatory compliance but also fosters innovation and technological adoption within industries. Moreover, by aligning environmental objectives with digital transformation goals, governments can create an ecosystem that encourages sustainable business practices and technological advancement, thereby enhancing sustainable competitiveness in the digital economy era.
Finally, information governance tools must consider the diversity at both industry and firm levels. This study underscores that different firm and industry characteristics shape the impact of GEID on digital transformation. Therefore, the design of these tools should involve tailoring strategies that address these specific moderating factors. By recognizing the unique needs and characteristics of different industries and firm types, including ownership structures and asset compositions, policymakers can create more effective governance frameworks. This approach can facilitate more impactful digital transformation efforts, thereby enhancing sustainability and competitiveness across various sectors.
Although this study involves enterprises in heavily polluting industries within the manufacturing sector, our research indicates that these companies are typically characterized as asset-intensive. The challenges and costs associated with the digital transformation of newly invested fixed assets compared to existing fixed assets are substantially different. Newly invested fixed assets usually incorporate digitalization requirements during the investment decision-making process, resulting in relatively lower costs and difficulties. Conversely, digitally upgrading existing fixed assets presents significant challenges. Therefore, an in-depth analysis of digital transformation in heavily polluting industries should consider these two distinct types of digital investment decisions. However, due to limitations in current empirical research, including this study’s data sources, it is not possible to distinguish between these two types of digital transformation decisions. Therefore, future research could employ categorized case studies to conduct an in-depth analysis of the differentiated and multifaceted corporate motivations driving the digital transformation of newly invested versus existing fixed assets.
The raw data supporting the conclusions of this article will be made available by the authors, without undue reservation.
SX: Data curation, Writing–original draft. XC: Conceptualization, Investigation, Writing–review and editing.
The author(s) declare that financial support was received for the research, authorship, and/or publication of this article. This study was supported by the National Natural Science Foundation of China (Grant 72064024) and the Project of Jiangxi Social Science (Grant 19GL32).
The authors declare that the research was conducted in the absence of any commercial or financial relationships that could be construed as a potential conflict of interest.
All claims expressed in this article are solely those of the authors and do not necessarily represent those of their affiliated organizations, or those of the publisher, the editors and the reviewers. Any product that may be evaluated in this article, or claim that may be made by its manufacturer, is not guaranteed or endorsed by the publisher.
1The PITI index stopped being disclosed after 2019
2In Column (3) of Table 3, the coefficient for PFA is significantly negative, with a T-value of −18.75, which is the highest absolute value among all variables in the model
3In Columns (5) and (6) of Table 8, the coefficients for GEID*PFA are significantly negative
Attard, J., Orlandi, F., Scerri, S., and Auer, S. (2015). A systematic review of open government data initiatives. Gov Inf Q 32 (4), 399–418. doi:10.1016/j.giq.2015.07.006
Baumeister, R. F., Bratslavsky, E., Finkenauer, C., and Vohs, K. D. (2001). Bad is stronger than good. Rev general 5 (4), 323–370. doi:10.1037/1089-2680.5.4.323
Bilal, M., Xicang, Z., Jiying, W., Sohu, J. M., and Akhta, S. (2024). Navigating the manufacturing revolution: dentifying the digital transformation antecedents. Manag Decis 62 (6), 1775–1805. doi:10.1108/MD-09-2023-1722
Blichfeldt, H., and Faullant, R. (2021). Performance effects of digital technology adoption and product service innovation – a process-industry perspective. Technovation 105, 102275. doi:10.1016/j.technovation.2021.102275
Bohnsack, R., Hanelt, A., Marz, D., and Marante, C. (2018). Same, same, but different!? A systematic review of the literature on digital transformation. Acad Manag Proc 2018, 16262. doi:10.5465/ambpp.2018.16262abstract
Borangiu, T., Trentesaux, D., Thomas, A., Leitão, P., and Barata, J. (2019). Digital transformation of manufacturing through cloud services and resource virtualization. Comput 108, 150–162. doi:10.1016/j.compind.2019.01.006
Brown, J. R., and Martinsson, G. (2019). Does transparency stifle or facilitate innovation? Manag Sci 65 (4), 1600–1623. doi:10.1287/mnsc.2017.3002
Buck, C., Clarke, J., Torres de Oliveira, R., Desouza, K. C., and Maroufkhani, P. (2023). Digital transformation in asset-intensive organisations: the light and the dark side. J Knowl 8 (2), 100335. doi:10.1016/j.jik.2023.100335
Chen, C.-L., Lin, Y.-C., Chen, W.-H., Chao, C.-F., and Pandia, H. (2021). Role of government to enhance digital transformation in small service business. Sustainability 13 (3), 1028. doi:10.3390/su13031028
Chen, D., Jiang, D., Liang, S., and Wang, F. (2011). Selective enforcement of regulation. China J Account Res 4 (1), 9–27. doi:10.1016/j.cjar.2011.04.002
Chen, P., and Hao, Y. (2022). Digital transformation and corporate environmental performance: the moderating role of board characteristics. Corp Soc Responsib Environ Manag 29 (5), 1757–1767. doi:10.1002/csr.2324
Chen, Y., Chen, S., and Miao, J. (2024). Does smart city pilot improve urban green economic efficiency: ccelerator or inhibitor. Environ Impact Assess Rev 104, 107328. doi:10.1016/j.eiar.2023.107328
Chen, Y., and Li, L. (2023). Differential game model of carbon emission reduction decisions with two types of government contracts: green funding and green technology. J Clean Prod 389, 135847. doi:10.1016/j.jclepro.2023.135847
Chen, Z., Kahn, M. E., Liu, Y., and Wang, Z. (2018). The consequences of spatially differentiated water pollution regulation in China. J 88, 468–485. doi:10.1016/j.jeem.2018.01.010
Cheng, Z., Zhang, Q., and Li, X. (2025). Can environmental information disclosure improve total-factor energy efficiency? J Environ Plan Manag 68 (1), 104–131. doi:10.1080/09640568.2023.2238893
Chong, T. T.-L., Lu, L., and Ongena, S. (2013). Does banking competition alleviate or worsen credit constraints faced by small-and medium-sized enterprises? Evidence from China. J Bank 37 (9), 3412–3424. doi:10.1016/j.jbankfin.2013.05.006
Chwiłkowska-Kubala, A., Cyfert, S., Malewska, K., Mierzejewska, K., and Szumowski, W. (2023). The impact of resources on digital transformation in energy sector companies. The role of readiness for digital transformation. Technol Soc 74, 102315. doi:10.1016/j.techsoc.2023.102315
Davis, J. H., Schoorman, F. D., and Donaldson, L. (1997). Toward a stewardship theory of management. Acad Manag Rev 22 (1), 20–47. doi:10.5465/amr.1997.9707180258
Duraivelu, K. (2022). Digital transformation in manufacturing industry–A comprehensive insight. Mater Today Proc 68, 1825–1829. doi:10.1016/j.matpr.2022.07.409
Fan, J., Xiao, D., Xun, M., and Wang, C. (2024). Informal environmental regulation and enterprises digital transformation: a study based on the perspective of public environmental concerns. Ecol Indic 163, 112142. doi:10.1016/j.ecolind.2024.112142
Fazzari, S., Hubbard, R. G., and Petersen, B. C. (1987). Financing constraints and corporate investment. USA: National Bureau of Economic Research Cambridge, Mass.
Fonseka, M., Rajapakse, T., and Tian, G. L. (2019). The effects of environmental information disclosure and energy types on the cost of equity: evidence from the energy industry in China. Abacus 55 (2), 362–410. doi:10.1111/abac.12157
Gago, R. F., and Antolin, M. N. (2004). Stakeholder salience in corporate environmental strategy. Corp Gov. 4 (3), 65–76. doi:10.1108/14720700410547512
Gao, S., Hakanen, E., Töytäri, P., and Rajala, R. (2019). Digital transformation in asset-intensive businesses: essons learned from the metals and mining industry. Proc Hawaii Int Conf Syst Sci.
Gebauer, H., Fleisch, E., Lamprecht, C., and Wortmann, F. (2020). Growth paths for overcoming the digitalization paradox. Bus 63 (3), 313–323. doi:10.1016/j.bushor.2020.01.005
Gilch, P. M., and Sieweke, J. (2021). Recruiting digital talent: the strategic role of recruitment in organisations’ digital transformation. Ger J Hum Resour Manag 35 (1), 53–82. doi:10.1177/2397002220952734
Hadlock, C. J., and Pierce, J. R. (2010). New evidence on measuring financial constraints: oving beyond the KZ index. Rev Stud 23 (5), 1909–1940. doi:10.1093/rfs/hhq009
Hahn, R. W., and Stavins, R. N. (1991). Incentive-based environmental regulation: a new era from an old idea. Ecol LQ 18, 1. doi:10.15779/Z38BC12
Hanelt, A., Bohnsack, R., Marz, D., and Antunes Marante, C. (2021). A systematic review of the literature on digital transformation: insights and implications for strategy and organizational change. J 58 (5), 1159–1197. doi:10.1111/joms.12639
Hill, T. D., Davis, A. P., Roos, J. M., and French, M. T. (2020). Limitations of fixed-effects models for panel data. Sociol Perspect 63 (3), 357–369. doi:10.1177/0731121419863785
Hillman, A. J., and Dalziel, T. (2003). Boards of directors and firm performance: ntegrating agency and resource dependence perspectives. Acad Manag Rev 28 (3), 383–396. doi:10.5465/amr.2003.10196729
Jaffe, A. B., Newell, R. G., and Stavins, R. N. (2005). A tale of two market failures: technology and environmental policy. Ecol Econ 54 (2), 164–174. doi:10.1016/j.ecolecon.2004.12.027
Jahn, J., and Brühl, R. (2019). Can bad news be good? On the positive and negative effects of including moderately negative information in CSR disclosures. J Bus Res 97, 117–128. doi:10.1016/j.jbusres.2018.12.070
Jamalpuria, A. (2013). On information dissemination as an informal environmental regulation. Environ Dev Econ 18 (6), 749–772. doi:10.1017/S1355770X13000223
Jiang, H.-D., Dong, K., Qing, J., and Teng, Q. (2023). The role of technical change in low-carbon transformation and crises in the electricity market: a CGE analysis with R&D investment. Energy Econ 125, 106897. doi:10.1016/j.eneco.2023.106897
Jiang, H.-D., Pradhan, B. K., Dong, K., Yu, Y.-Y., and Liang, Q.-M. (2024). An economy-wide impacts of multiple mitigation pathways toward carbon neutrality in China: a CGE-based analysis. Energy Econ 129, 107220. doi:10.1016/j.eneco.2023.107220
Jones, M. D., Hutcheson, S., and Camba, J. D. (2021). Past, present, and future barriers to digital transformation in manufacturing: a review. J Manuf Syst 60, 936–948. doi:10.1016/j.jmsy.2021.03.006
Ko, A., Fehér, P., Kovacs, T., Mitev, A., and Szabó, Z. (2022). Influencing factors of digital transformation: management or IT is the driving force? Int J Sci 14 (1), 1–20. doi:10.1108/IJIS-01-2021-0007
Kraus, S., Jones, P., Kailer, N., Weinmann, A., Chaparro-Banegas, N., and Roig-Tierno, N. (2021). Digital transformation: an overview of the current state of the art of research. Open 11 (3), 21582440211047576. doi:10.1177/21582440211047576
Kunkel, S., and Matthess, M. (2020). Digital transformation and environmental sustainability in industry: utting expectations in Asian and African policies into perspective. Environ Sci Policy 112, 318–329. doi:10.1016/j.envsci.2020.06.022
Leão, P., and da Silva, M. M. (2021). Impacts of digital transformation on firms' competitive advantages: a systematic literature review. Strateg Change 30 (5), 421–441. doi:10.1002/jsc.2459
Li, C., Gu, X., Li, Z., and Lai, Y. (2024). Government-enterprise collusion and public oversight in the green transformation of resource-based enterprises: a principal-agent perspective. Syst Eng 27 (2), 417–429. doi:10.1002/sys.21731
Li, C., Wang, Y., Zhou, Z., Wang, Z., and Mardani, A. (2023). Digital finance and enterprise financing constraints: tructural characteristics and mechanism identification. J Bus Res 165, 114074. doi:10.1016/j.jbusres.2023.114074
Li, Q., Ruan, W., Shao, W., and Huang, G. (2017). Information disclosure in an environmental emergency. Disaster Prev Manag Int J 26 (2), 134–147. doi:10.1108/DPM-06-2016-0125
Lin, Y., Huang, R., and Yao, X. (2021). Air pollution and environmental information disclosure: an empirical study based on heavy polluting industries. J Clean Prod 278, 124313. doi:10.1016/j.jclepro.2020.124313
Lu, J., and Li, H. (2020). The impact of government environmental information disclosure on enterprise location choices: heterogeneity and threshold effect test. J Clean Prod 277, 124055. doi:10.1016/j.jclepro.2020.124055
Mahmood, F., Khan, A. Z., and Khan, M. B. (2019). Digital organizational transformation issues, challenges and impact: a systematic literature review of a decade. Univ J 12 (2). doi:10.34091/AJSS.12.2.03
Martínez Falcó, J., Sánchez-García, E., Marco-Lajara, B., and Akram, U. (2024). Digital transformation and green innovation performance: unraveling the role of green knowledge sharing and top management environmental awareness. Internet Res. ahead-of-print(ahead-of-print). doi:10.1108/INTR-11-2023-1016
Mihu, C., Pitic, A. G., and Bayraktar, D. (2023). Drivers of digital transformation and their impact on organizational management. Stud Bus Econ 18 (1), 149–170. doi:10.2478/sbe-2023-0009
Mol, A. P. (2009). Environmental governance through information: China and Vietnam. Singap J Trop Geogr 30 (1), 114–129. doi:10.1111/j.1467-9493.2008.00358.x
Nadkarni, S., and Prügl, R. (2021). Digital transformation: a review, synthesis and opportunities for future research. Manag Rev Q 71, 233–341. doi:10.1007/s11301-020-00185-7
Ohlert, C., Giering, O., and Kirchner, S. (2022). Who is leading the digital transformation? Understanding the adoption of digital technologies in Germany. New Technol Work Employ 37 (3), 445–468. doi:10.1111/ntwe.12244
Pan, A., Qin, Y., Li, H., Zhang, W., and Shi, X. (2023). Can environmental information disclosure attract FDI? Evidence from PITI project. J Clean Prod 403, 136861. doi:10.1016/j.jclepro.2023.136861
Porter, M. E., and Heppelmann, J. E. (2014). How smart, connected products are transforming competition. Harv Bus Rev 92 (11), 64–88.
Porter, M. E., and Van der Linde, C. (1995). Toward a new conception of the environment-competitiveness relationship. J 9 (4), 97–118. doi:10.1257/jep.9.4.97
Qing, L., Yao, Y., Sinisi, C.-I., Salman, A., Jaradat, M., Spinu, A. E., et al. (2024). Do trade openness, environmental degradation and oil prices affect green energy consumption? Energy Strategy Rev 52, 101342. doi:10.1016/j.esr.2024.101342
Raimo, N., Caragnano, A., Zito, M., Vitolla, F., and Mariani, M. (2021). Extending the benefits of ESG disclosure: the effect on the cost of debt financing. Corp Soc Responsib Environ Manag 28 (4), 1412–1421. doi:10.1002/csr.2134
Sarkis, J., Kouhizadeh, M., and Zhu, Q. S. (2021). Digitalization and the greening of supply chains. Manag Data Syst 121 (1), 65–85. doi:10.1108/IMDS-08-2020-0450
Schlæger, J., and Zhou, J. (2019). Digital environmental monitoring in urban China. Green urban, 131–149. doi:10.1007/978-981-13-0740-9_7
Stermieri, L., Kober, T., Schmidt, T. J., McKenna, R., and Panos, E. (2023). Quantifying the implications of behavioral changes induced by digitalization on energy transition: a systematic review of methodological approaches. Energy Res Soc Sci 97, 102961. doi:10.1016/j.erss.2023.102961
Suchman, M. C. (1995). Managing legitimacy: strategic and institutional approaches. Acad Manag Rev 20 (3), 571–610. doi:10.5465/amr.1995.9508080331
Taklo, S. K., Tooranloo, H. S., and Shahabaldini parizi, Z. (2021). Green innovation: a systematic literature review. J Clean Prod 279 (10), 122474. doi:10.1016/j.jclepro.2020.122474
Tsiavos, V., and Kitsios, F. (2021). Technology as driver, enabler and barrier of digital transformation: a review. European, mediterranean, and middle eastern conference on information systems.
Vial, G. (2019). Understanding digital transformation: review and a research agenda. The Journal of Strategic Information Systems 28 (2), 118–144. doi:10.1016/j.jsis.2019.01.003
Wang, B., Ma, Y., Luo, B., Shi, D., and Jiang, S. (2024). Environmental supervision distance, government-enterprise collusion and corporate environmental information disclosure. Int Rev Econ 96, 103710. doi:10.1016/j.iref.2024.103710
Wang, S., Li, X., Li, Z., and Ye, Y. (2023). The effects of government support on enterprises' digital transformation: vidence from China. Manag Decis Econ 44 (5), 2520–2539. doi:10.1002/mde.3831
Wang, Y. (2018). Life Cycle Cost Management of Fixed Assets in Chinese Power Grid Enterprises. 2018 3rd International Conference on Education. E-learning and Management Technology (EEMT 2018) (Atlantis Press), 307–311.
Woodward, D. G. (1997). Life cycle costing—Theory, information acquisition and application. Int. J. Proj. Manag., 15(6), 335–344. doi:10.1016/S0263-7863(96)00089-0
Xu, S., Dong, M., and Chen, X. (2024). Information transparency of government environmental supervision and corporate green innovation in Chinese highly polluting sectors. Environ Dev Sustain. doi:10.1007/s10668-024-05701-3
Yang, Y., Subhani, G., Sakina, S., and Zhou, Y. (2024). Natural resource and energy efficiency in BRICS countries: an green innovation capabilities really change the game? Gondwana Res. doi:10.1016/j.gr.2024.03.014
Yu, F., Zhang, Q., and Jiang, D. (2023). The impact of regional environmental regulations on digital transformation of energy companies: he moderating role of the top management team. Manag Decis Econ 44 (6), 3152–3165. doi:10.1002/mde.3868
Yu, W., and Jin, X. (2022). Does environmental information disclosure promote the awakening of public environmental awareness? Insights from Baidu keyword analysis. J Clean Prod 375, 134072. doi:10.1016/j.jclepro.2022.134072
Zhang, X.-P., and Cheng, X.-M. (2009). Energy consumption, carbon emissions, and economic growth in China. Ecol Econ 68 (10), 2706–2712. doi:10.1016/j.ecolecon.2009.05.011
Zhao, S., Zhang, L., Peng, L., Zhou, H., and Hu, F. (2024). Enterprise pollution reduction through digital transformation? Evidence from Chinese manufacturing enterprises. Technol Soc 77, 102520. doi:10.1016/j.techsoc.2024.102520
Zhong, S., Li, J., and Zhao, R. (2021). Does environmental information disclosure promote sulfur dioxide (SO2) remove? New evidence from 113 cities in China. J Clean Prod 299, 126906. doi:10.1016/j.jclepro.2021.126906
Keywords: government environmental information disclosure, manufacturing companies, heavily polluting industries, digital transformation, China
Citation: Xu S and Chen X (2025) Government environmental information disclosure and digital transformation of manufacturing companies: evidence from China. Front. Environ. Sci. 13:1492874. doi: 10.3389/fenvs.2025.1492874
Received: 09 September 2024; Accepted: 27 January 2025;
Published: 12 February 2025.
Edited by:
Pierre Glynn, Arizona State University, United StatesReviewed by:
Yufeng Chen, Zhejiang Normal University, ChinaCopyright © 2025 Xu and Chen. This is an open-access article distributed under the terms of the Creative Commons Attribution License (CC BY). The use, distribution or reproduction in other forums is permitted, provided the original author(s) and the copyright owner(s) are credited and that the original publication in this journal is cited, in accordance with accepted academic practice. No use, distribution or reproduction is permitted which does not comply with these terms.
*Correspondence: Xia Chen, eGlhY2hlbkBqanUuZWR1LmNu
Disclaimer: All claims expressed in this article are solely those of the authors and do not necessarily represent those of their affiliated organizations, or those of the publisher, the editors and the reviewers. Any product that may be evaluated in this article or claim that may be made by its manufacturer is not guaranteed or endorsed by the publisher.
Research integrity at Frontiers
Learn more about the work of our research integrity team to safeguard the quality of each article we publish.