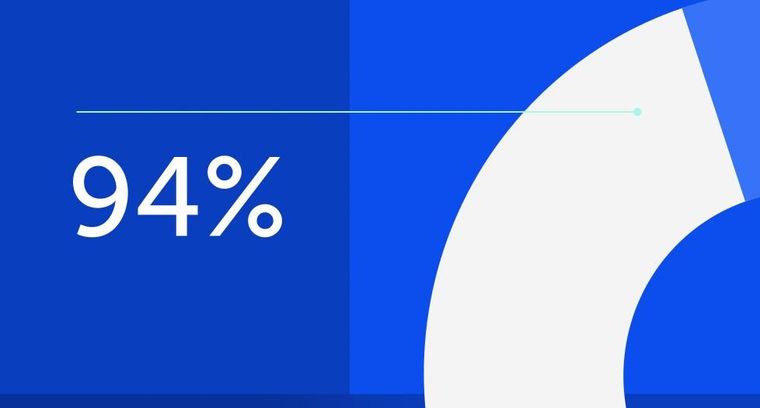
94% of researchers rate our articles as excellent or good
Learn more about the work of our research integrity team to safeguard the quality of each article we publish.
Find out more
ORIGINAL RESEARCH article
Front. Environ. Sci., 18 March 2025
Sec. Environmental Economics and Management
Volume 13 - 2025 | https://doi.org/10.3389/fenvs.2025.1492749
Three decades after the Earth Summit in Rio de Janeiro, the world continues to grapple with the intertwined challenges of environmental degradation and the pursuit of inclusive, sustainable economic growth. This study investigates three key aspects of green economic growth: First, it examines whether global green growth is converging or diverging across regions. Second, it explores the fundamental drivers of regional differences in green growth, focusing on trade openness, CO2 emissions, and the transition to renewable energy. Finally, the study delves into the complex, nonlinear relationships between economic development and environmental sustainability. Using innovative methodologies such as PS club clustering and log-t regression analysis, the study analyzes production-based CO2 productivity data from 134 countries spanning 1995 to 2022. Contrary to conventional assumptions, the results reveal significant divergence in global green economic growth, suggesting that different regions are experiencing varied levels of success in integrating environmental and economic goals. These findings highlight the need for region-specific policies, emphasizing tailored strategies that promote sustainable development. Policymakers should prioritize fostering trade openness, accelerating the renewable energy transition, and implementing stricter environmental regulations, while also encouraging international collaboration to bridge the gap between advanced and developing economies.
In the wake of recent global conferences such as COP28 and IPCC, sustainability and environmental concerns reached the heart of the global agenda (UNFCC, 2024). Environmental concerns are vital in policy discussions at these forums due to their relevance to sustainability (Tiwari et al., 2022). Industrialization, urbanization, and the race for economic growth have placed difficulties in the means of achieving these environmental goals. The recent targets of the United Nations Sustainable Development Goals that have replaced the Millennium Development Goals call for governments around the globe to address climate change issues (Awan et al., 2023). The notion of green growth (GG) has gradually gained distinction as a framework for pursuing economic development in an environmentally sustainable manner. Fundamentally, GG seeks to mitigate resource use and pollution due to economic growth by transitioning societies towards a low-carbon, efficient model of production and consumption (Antal & Van Den Bergh, 2016; Hickel and Kallis, 2020). GG includes nurturing innovation in cleaner technologies, investing in renewable energy and green investments, and promoting the conservation of ecosystem services and natural resources (Trinh et al., 2023; Xu et al., 2022; Miraz and Soo, 2024). Thus, GG aims to enhance economic growth while minimizing environmental damage caused during this process (Jouvet and de Perthuis, 2013).
The importance of climate change and sustainable development has attracted academia and researchers, however, for clear evaluation of various policies, a sustainable environment requires metrics for measurement. In this regard, carbon dioxide and ecological footprints are two broadly used indicators for environmental degradation (Moldan et al., 2012; Jahanger et al., 2023). Recently, various multidimensional indices have been introduced in the literature on environmental sustainability. On the one hand, several forecasting and long-term correlation studies identified empirical evidence on the performance of countries and the success of their policies. On the other hand, global efforts for promoting sustainable economic development that do not hurt the environment while achieving economic goals are evaluated in the literature. One such concept used in literature to measure global efforts toward achieving sustainability is convergence (Moldan et al., 2012; Xu et al., 2023).
The examination of global environmental convergence helps policymakers understand the patterns and dynamics of sustainability from an environmental perspective across countries (Ahmed et al., 2019). The concept of convergence refers to the idea that countries with initially lower levels of environmental performance tend to meet up with countries that have higher environmental performance (Ulucak, 2020; Ulucak, 2021). By studying convergence, governments get insights for policymaking into the factors that help mitigate environmental degradation and suggest policies that can promote sustainable development on a global scale (Hu, 2023).
To capture global environmental performance holistically, extant literature has developed and introduced various measures. These measure simple variables, for instance CO2 emissions and GDP per capita (Lozano and Gutierrez, 2008; Awan et al., 2022). These indices allow a more sophisticated apprehension of environmental sustainability and enable more accurate assessments of convergence. In addition, convergence literature is attractive to environmental economists and policymakers to reach the goals of the Kyoto Protocols and recent COP28 conference by understanding regional heterogeneities and regional quotas. This information about quotas and groups of countries facing the same challenges following similar convergence paths may add up their efforts and receive common policy benefits from global communities towards reaching the common goal of environmental protection (Tews et al., 2003; Akram and Ali, 2022).
Convergence examination of environmentally sustainable performance helps in identifying heterogeneity in convergence patterns across countries. It is useful to analyze whether convergence rates differ based on various factors. To this end, researchers divide the global sample into small samples and clubs. Such a method enables researchers to understand the exact challenges and opportunities in front of different countries or groups of countries in achieving environmental sustainability (Solarin, 2014). For instance, studies have found that the convergence rate in environmental performance may vary depending on a country’s income level, with low-income countries converging at a different rate than high-income countries (Tiwari et al., 2021).
Additionally, global environmental convergence analysis is useful in effective policy interventions to achieve the goal of promoting climate sustainability at the global level with the efficacy of intervention. In addition, by analyzing the factors that determine certain environmental performance indicators’ convergence, global-level efforts can be evaluated over time. This information can enlighten the design and implementation of policies that promote sustainable ecosystems and help economies achieve their environmental goals (Haider and Akram, 2019).
An important aspect of convergence literature is analyzing the factors driving the convergence of specific variables. In our case, what drives the convergence of GG, is also the objective of the study. Relevant literature for instance, Zhou et al. (2022), Song et al. (2019), Zheng et al. (2022), Cao et al. (2022), and Maiti (2022) analyzed the role of trade openness in driving GG. Similarly, Qiang and Jian (2020) and Ren et al. (2022) documented that green economic development is correlated with natural resource rent and institutional quality. Li et al. (2022) and Mensah et al. (2019) highlight the transformative effects of green technology and innovation on environmental sustainability and economic prosperity. Given the importance of various factors including carbon dioxide emissions and population size, this study attempts to fill the gap in the literature on what drives the convergence of GG in a global sample.
The study contributes to the existing body of literature by contributing in three different ways; firstly, it uses a novel measure of environmental performance to gauge global level figures for 134 countries from 1995 to 2022. Using such a large data set, the study compares the performance of global economies in terms of environmental performance (Shen et al., 2022). In this regards, the study used green economic growth as a proxy to measure environmental sustainability. Compared to simple CO2 emissions or per capita GDP, we used a more comprehensive measure of environmental sustainability which is production-based CO2 emissions to gauge GG. Secondly, we used the club convergence method introduced by Phillips and Sul (2007) which is a healthy methodology to identify clubs and their convergence. This method also involves estimating the chance of convergence between clubs (Blampied, 2021). Thirdly, this study examines various determinants of club convergence using GG as a proxy to measure environmental sustainability. By exploring macro-level factors that drive GG dynamics across the global sample, this research aims to identify policy suggestions for gearing up the global shift toward inclusive and green ways of development (Chen et al., 2020).
The remaining part of the manuscript will follow the order that in the next section we have presented a critical review of literature. This part explains the theoretical and empirical overview of the latest and important studies related to the research hypothesis of the present study. In the next Section 3, the manuscript sheds light on the data and methodology involved in the manuscript. Subsequently, in Section 4 the empirical findings are illustrated based on club convergence methodology. Lastly, our section on conclusion shows the important and main conclusion drawn based on empirical evidence followed by clear policy implications.
The concept of GG denotes the sustainable expansion of the economy in terms of environmental sustainability (Sandberg et al., 2019) and the efficient utilization of natural resources (Reilly, 2012). GG signifies a transformative approach to economic advancement that brings numerous benefits to both communities and ecosystems. One of its primary advantages lies in advancing environmental sustainability, promoting resource optimization, reduced emissions, and biodiversity preservation (D’Alessandro et al., 2020).
The overuse of fossil fuels and the energy needs resulting from industrial activity have caused considerable carbon dioxide (CO2) emissions into the atmosphere. Global warming and the ensuing rise in sea levels are mostly caused by these emissions (Keeling, 1973; Wuebbles and Jain, 2001; Quadrelli and Peterson, 2007; Heede, 2014; Li and Haneklaus, 2021). Recognizing the serious repercussions of greenhouse gases, global initiatives are underway to mitigate carbon emissions. It's increasingly vital for countries to explore avenues for curbing environmental degradation through sustainable development that benefits all (Li, Dong and Dong, 2022; Huang and Zhao, 2022).
It is revealed that positive changes in raw material efficiency reduce greenhouse gas emissions, whereas negative changes lead to an increase, particularly in the long term. Raw material efficiency for GG and environmentally relevant technologies decreases greenhouse gas emissions. The importance of raw material efficiency for GG is highlighted in studies conducted by Wang et al. (2020) and Gyamfi et al. (2021) for the G7 economies, Tufail et al. (2021) for the OECD countries, Adebayo et al. (2022) for newly industrialized countries, Shen et al. (2021) for China, and Bekun et al. (2019) for 16 EU countries. Bleischwitz (2010), for example, shows that although economic expansion raises greenhouse gas emissions over the long run, GG and raw material efficiency for technology connected to the environment reduce these emissions.
Moreover, a strong linkage exists between carbon emissions and innovation within green technology, energy, and environmental technology sectors. Conversely, proponents also posit that green technologies play a pivotal role in mitigating global pollution and advancing towards carbon neutrality (Ulucak, 2020; Dong et al., 2022).
The measurement methods for GG can be broadly classified into two main approaches. One such approach is the Malmquist-Luenberger (ML) index, pioneered by Caves et al. (1982). This index evaluates total factor productivity by employing input or output-oriented directional distance functions. Researchers like Zhang et al. (2024), Fang et al. (2021), and Ngo, T. Q. (2024) have utilized ML-based methodologies to derive green growth indices. Secondly, some methods integrate green growth by quantifying all environmental and resource costs and benefits, as highlighted by scholars such as Huang and Zhao (2022), Xu (2022), and Guo et al. (2017). Additionally, many studies in the literature focus on developing green indicator systems and parameters to gauge green growth effectiveness, including works by Sohag et al. (2019) and Song et al. (2019). Lastly, indices are formulated to assign weightings to specific indicators of green growth, as seen in the research by Kumar (2017), Maji (2019), and Zhao J. et al. (2022).
Green growth (GG), characterized by sustainable economic development that minimizes environmental degradation, is influenced by various factors ranging from trade openness and financial development to environmental regulations, technological innovations, natural resource use, and green technology. This literature review synthesizes key findings from recent studies to shed light on the diverse drivers of GG and their implications.
Zhou et al. (2022), Zheng et al. (2022), Cao et al. (2022), and Maiti (2022) are some of the recent studies that explore the relationship between trade openness, financial development, and green finance and green growth. These studies highlight the relationship between sustainable environmental practices and economic policy. For example, Zheng et al. (2022) show that rising levels of green credit promote the development of a high-quality green economy in China, with carbon emissions serving as intermediaries. The complex relationship between economic policies and environmental results is further highlighted by Song et al.'s (2019) observation of a non-linear U-shaped correlation between economic openness and green economic growth in China. Furthermore, through promoting technology and eco-innovations, financial development and venture capital investments can support green growth, as shown by the studies by Cao et al. (2022) and Maiti (2022).
The industrial structure and environmental laws are important factors that shape GG. In order to mitigate environmental deterioration and promote green economic development, it is important to implement strict laws and sustainable industrial practices, as highlighted by Zhao X. et al. (2022), Guo et al. (2017), Wang and Shao (2019), and Mahmood et al. (2022). Environmental rules, for instance, have a U-shaped effect on the expansion of the green economy in Chinese cities, according to Zhao J. et al. (2022), with spatial spillover and feedback effects influencing regional outcomes. Furthermore, Wang and Shao (2019) highlight the significance of both formal and informal laws in influencing environmental outcomes by demonstrating the nonlinear effects of environmental rules on green growth in Group 20 countries. Related to the above studies, Aziz and Bakoben (2024) bring to light some evidence to suggest that environmental decentralization has a negative impact on green growth in China.
Green growth is driven mainly by technological advances and advancements in green innovations. The transformative effects of green technology and innovation on environmental sustainability and economic success are highlighted by Li et al. (2022) and Mensah et al. (2019). Positive correlations between green energy, technical innovation, and green growth in China are found by Li et al. (2022), highlighting the role that technology breakthroughs play in promoting sustainable economic development. Similarly, Mensah et al. (2019) highlight the difficulties associated with energy production technologies while highlighting the contribution of industry and transportation-related innovations to green growth in OECD nations. Finally, Hu et al. (2024) maintain that digitalization benefits green economic growth by enhancing carbon productivity and environmental protection consciousness in China, while Wu et al. (2024) reveals that digital transformation can improve green competitive advantages in China.
Efficient resource management and energy transition are critical strategies for enhancing green growth. Xu (2022), Huang and Zhao (2022), Zhang et al. (2024), and Ngo (2024) investigate the intricate nexus between natural resource utilization and green economy. Xu (2022) emphasises that coal and gas are crucial for China’s green growth, despite negative associations with gas. While Zhang et al. (2024) highlight the detrimental consequences of natural resource availability on green growth and the possibility for energy transition to minimise negative effects, Geng et al. (2023) emphasize the long-run and continuous negative impact of natural resource consumption on green economic efficiency; Shang et al. (2023) point to the detrimental effects of energy resources (fossil fuels and renewable energy) on green economic in Asian economies, while Zhao et al. find evidence showing that energy consumption and GDP growth have negative effects on green economic in China. Huang and Zhao (2022) emphasise the importance of resource utilisation efficiency in supporting green growth in China. Furthermore, Ngo (2024) suggests encouraging green finance to mitigate the adverse impacts of resource availability on Vietnam’s overall green production parameters. The literature concludes by highlighting the complexity of green growth and the need to address a range of factors to achieve sustainable economic development with the least negative environmental effects. These factors include trade openness, financial development, environmental regulations, technological innovations, and the use of natural resources.
The convergence and divergence dynamics within sustainability and green economy have been intensely scrutinised in recent academic literature. Four key studies shed light on various aspects of this discourse. Shen et al. (2022) examine how green productivity is calculated, emphasizing the role of technological progress in driving GG in Africa. Eleftheriou et al. (2024) focus on the Sustainable Development Index (SDI), revealing distinct convergence clubs among countries with implications for ecological wellbeing. Sueyoshi and Wang (2020) explore convergence clubs in renewable energy innovations, highlighting factors such as income, emissions, and institutional quality. Finally, Pinar (2024) examines global convergence patterns in renewable energy innovations, underscoring the importance of environmental regulations and R&D investment. These studies collectively contribute to understanding convergence phenomena within the sustainability realm. While existing studies have extensively investigated convergence in various environmental factors (González-Álvarez et al., 2020; Zhang et al., 2021; Ulucak, 2021; Bigerna et al., 2021; Saba and Ngepah, 2022), research on convergence in production-based CO2 productivity remains insufficient. Additionally, despite the examination of convergence in GG, there is a significant vacuum in the literature regarding the drivers of GG convergence.
Shen et al. (2022) employed the Luenberger productivity measurement to incorporate carbon emissions into the measurement of green productivity. The study observed that the annual average green productivity growth in Africa is 1.51%. GG in Africa primarily stemmed from technological progress rather than efficiency changes, with three different convergence clubs identified for technological progress. Eleftheriou et al. (2024) utilized the Phillips and Sul methodology to examine convergence/divergence models of the Sustainable Development Index (SDI) across 137 countries. Their findings identified two convergence clubs: one comprising developing countries from Africa and Asia, and the other consisting of developed countries like the USA, Canada, and Australia. They concluded that the SDI encourages ecological wellbeing, with top-ranking countries characterized by minimal ecological impact and high human development.
Sueyoshi and Wang (2020) conducted a two-stage analysis using Data Envelopment Analysis (DEA) to construct sustainable development indices. They applied the Phillips and Sul algorithm for club convergence analysis and highlighted two convergence clubs in renewable energy innovations: one comprising more innovative countries and the other containing less innovative ones. Their conclusion suggested that countries with higher per capita income, lower CO2 emissions, higher R&D investment, better environmental regulations, and stronger institutional environments have a higher probability of being part of the innovative club. Pinar (2024) utilized the Phillips and Sul convergence algorithm to evaluate the convergence of renewable energy innovations across 90 countries. Determinants were explored through Probit and Logit regression analyses. Pinar (2024) revealed the absence of global convergence in renewable energy innovations, instead identifying two convergence clubs based on innovativeness. The study suggested that countries can enhance their likelihood of joining a more innovative club by increasing investments in R&D, improving environmental regulations, and enhancing institutional quality.
The literature is replete with unremitting debate on the application of convergence of renewable energy, renewable energy innovations, green productivity, SDI, and green economic efficiency among others. However, there is limited literature on the convergence of green economic growth and the drivers. This study contributes to the literature by (1) examining the global convergence path of green economic growth and (2) investigating the drivers of this convergence. This analysis is extended to regional analysis to understand the regional dynamics.
This study encompasses a panel of 134 countries spanning the years 1995–2022. The selection of countries was influenced by data availability, detailed in Supplementary Appendix Table A1 in the Appendix. Green economic growth, which is the dependent variable, is quantified using resources and environmental productivity. Specifically, we utilised production-based CO2 productivity (US dollars per kilogramme, 2015). This approach aligns with previous studies by Kasztelan (2017), Porada Rochoń (2021), Wu et al. (2022), and Kirikkaleli and Addai (2023). In exploring the drivers of club convergence, we incorporate economic growth, measured by per capita real GDP (in constant 2015 US dollars), drawing on the empirical insights of Fernandes et al. (2021) suggesting its contribution to green economy convergence. Additionally, trade openness is introduced, measured by the addition of imports and exports divided by gross domestic product is consistent with the approach of Shahbaz et al. (2014).
Given the empirical outcomes of Tariq et al. (2024) indicating the influence of environmental pollution (CO2 emissions per capita) on green economic growth, we include CO2 emissions in the model. Population size (total population) is integrated into the model based on the proposition of Nathaniel et al. (2021), highlighting its association with increased energy demand and environmental impacts. In order to evaluate the utilisation of renewable energy, we use renewable energy demand as a proportion of the overall final consumption of energy. This approach aligns with the findings of Ashfaq et al. (2024) concerning the critical role that the transition to renewable energy plays in fostering green economic growth. We used institutional quality measured by the average of control of corruption, regulatory quality, political stability, rule of law, government effectiveness, and voice and accountability. This metrics has been widely referenced by (Konara and Shirodkar, 2018; Elamer et al., 2020; Tunyi et al., 2020), providing insights into its impact on the green economy.
The descriptive statistics of green economic growth and its club convergence drivers are presented in Table 1. From 1995 to 2022, and among the 134 countries, the average value of green economic growth is 6.5761 for the full sample the full sample. This value is less than green economic growth in Club 1 and higher than green economic growth in Club 2. Furthermore, the average value of trade for the full sample is 84.75%, while for Club one is 89.60% and for Club 2 is 78.41%. Renewable energy has an average of 30.33% for the full sample, 33.98% for Club 1, and 25.68% for Club 2. Furthermore, Club 2 has the highest CO2 emissions, with an average of 5.95 metric tons per capita compared to 5.13 for the full sample and 4.48 for Club 1. In addition to the descriptive statistics, Supplementary Appendix Table A2 in the appendix presents the correlation statistics on the covariate. The key lesson from the analysis is that there is no multicollinearity problem, given that all the variables have a correlation coefficient of less than 0.8.
This study utilized the Phillips and Sul (2007) (PS) convergence econometric approach to ascertain convergence and identify clubs. The principal variable of interest, green economic growth, is represented as
Where
PS recommended eliminating the common factor in the following manner in order to impose restrictions on
The relative transition parameter (
In defining the null hypothesis, some assumptions are made on
We then use the log
The convergence methodology employed by PS relied on transition pathways derived from the green economic growth indicator. While proficient in delineating club convergence, it lacks the capacity to ascertain the underlying catalysts propelling the genesis of these clusters. Bartkowska and Riedl (2012), consequently introduced an ordered logit model aimed at discerning the determinants of these clusters. This model is premised on the assumption that the explained variable is ordinal, one or more of the explanatory variables are either ordinal, categorial or continuous, there is no multicollinearity, and the independent variables have homogenous effect at each cumulative split of the dependent variable (Bartkowska and Riedl, 2012). Our study aims to elucidate the factors that drive club formation. Initially, we employ the PS clustering methodology, followed by the application of the ordered logit model. This sequential analytical framework enables us to systematically identify the features motivating the emergence of these clubs, thereby enhancing scholarly insights into their formation dynamics.
Let
The relationship between the independent variables and the probability distribution over the C categories in the ordered logit regression model is outlined by a set of
For each
To measure the impact of specific factors on predicting club membership, we examine how each variable individually affects the likelihood. These assessments measure how much the probability of club membership changes when one variable shifts by one unit while holding all other variables at their average values. We employed the ordered probit model to ensure the robustness of our empirical results, following the econometric guidelines provided by Johnston (2020). This methodological choice serves to fortify the robustness of our analysis and instil confidence in the reliability of our conclusions.
Table 2 presents the results of the PS log(t) test conducted on a global scale, encompassing data from 134 countries. The table comprises two panels: A showcases the log(t) coefficients and associated t-statistics for the entire sample, both the original and merged club classifications, while Panel B provides the log(t) values specifically for the final club classification. The findings from the log(t) convergence test indicate an absence of convergence in green economic growth across the sampled countries. This conclusion is drawn from the coefficient of log(t) (−0.0936) and its associated t-statistics (−7.8996), which fall substantially below the critical threshold of −1.65. This divergence in green economic growth trends among the 134 countries suggests significant disparities. These results align with prior research highlighting variations in the green economy and environmental quality across nations (Porada Rochń, 2021; Haider et al., 2021).
Furthermore, PS underscores the opportunity to explore club convergence alongside analyzing convergence within the entire panel. Initially, the club clustering algorithm identified three convergence clubs across the full panel. Following PS’s guidance, a club convergence algorithm was subsequently deployed to mitigate the risk of overestimation. The results of the club merger tests indicate the potential combination of Club 1 + 2 and Club 2 + 3. Remarkably, the t-statistics for each club (Club 1: 8.458; Club 2: 1.813) surpasses the critical threshold of −1.65. Consequently, the empirical discoveries stemming from the club clustering algorithm affirm that the club convergence null hypothesis is accepted for both clubs.
Table 3 provides a comprehensive list of countries constituting each club formation. Furthermore, the relative transition curves for Clubs one and two are shown in Figures 1, 2, respectively, while Figure 3 illustrates the average transition paths for both clubs. Upon scrutinizing these curves, it becomes evident that the transition pathways for each club adhere to the theoretical framework of club convergence. According to this framework, members of each club have relative transition paths that converge towards different constants. A meticulous visual analysis of these data points suggests that while the green economy may vary among countries within each club, there is a notable tendency towards substantial convergence.
Converging of GG globally is crucial for addressing urgent environmental challenges such as deforestation, pollution, biodiversity loss, and climate change. This is the main objective of the Environmental Sustainability Goals (SDG) set forth by the United Nations in 2019. By transitioning to sustainable practices and reducing resource consumption, countries can collectively mitigate environmental degradation and preserve the Earth’s natural ecosystems for future generations. Since green economy convergence emphasizes the decoupling of economic growth from environmental degradation, its divergence or convergence implications are crucial.
The study’s findings about the global divergence of the green economy imply that diverse causes exert pressure on the green economies of different countries around the globe. Thus, universal laws pertaining to environmental sustainability might not work everywhere in the world. Because different countries have different starting conditions and different pathways towards the long-run equilibrium, it may be challenging to formulate common environmental policies. Hence, it is imperative to align international environmental accords and policies according to these club classifications (Pettersson et al., 2014; Stern, 2017). Furthermore, comparable strategies are unlikely to be successful in nations where associated variables differ, as stated by Ahmed et al. (2019). In addition, considering the country-specific drivers of the green economy implies the possibility of implementing tailored environmental policies at the national level, in addition to the common club-classification measures.
Referring to Table 4, the convergence log(t) test results for each global region are delineated. The table is partitioned into two panels: Panel A presents the initial club classification, merged clubs, as well as the coefficients and associated t-statistics of log(t) for the entire sample within each region. Panel B delineates the final club classifications. Our analysis encompasses four continents: Africa, Asia, Europe, and Latin America, which also includes North America and the Caribbean. The respective t-statistics of −40.3704, −1.9909, and −10.7441, all markedly falling below −1.65, suggest a lack of evidence supporting conditional or relative convergence across the entire samples for Africa, Europe, Latin America, and the Caribbean. Consequently, the null hypothesis of full panel convergence is rejected for each region.
However, as the t-statistic of 4.4805 is greater than −1.65, the estimates of the log(t) convergence tests for Asia show evidence of conditional/relative convergence. As a result, the null hypothesis of full panel convergence is accepted. Furthermore, if the estimate of log(t) is larger than or equal to 2, it is also possible to determine the size of the corresponding log(t) coefficients, which, in accordance with PS, represent convergence patterns or decay rates. The full sample of the aforementioned regions (Africa: −0.6764; Asia: 0.2084; Europe: 0.0284; Latin America, North America, and Caribbean: −0.2503) has a coefficient of less than 2, as indicated in Table 4, indicating that there is a divergence in green economic growth for each of the four regions.
An examination of possible convergence club was subsequently done. The algorithm uncovers four convergence clubs for Africa at first, but after using a club merging technique to prevent overestimating the clubs, the final club classification was reduced to three, as shown in Panel B of Table 4. Club 1 comprises two countries, while Club 2 and Club 3 consist of twenty and eleven countries, respectively. Table 5 presents the list of countries included in each final club classification for Africa. With decay rates of 36.9%, 17.8%, and 13% for clubs 1, 2, and 3, respectively. Table 4 indicates that there is conditional convergence among the clubs, with each log(t) coefficient being significant and having a t-test greater than −1.65. Based on their relative log(t) coefficients, club 1 and club 2 appear to have the highest rates of convergence. The empirical results support the findings of Arogundade et al. (2023) and Ulucak et al. (2020), who indicated that three convergence clubs exist in the region despite finding divergence in environmental quality in Africa.
Now turning to the findings of the Asian region. The entire sample for the Asian continent shows indications of convergence, in contrast to the results of the world sample and the African continent. Given that the log(t) t-statistic of 4.4805 is greater than −1.65, the null hypothesis for entire sample convergence is accepted. This demonstrates that, at a decay rate of 20.84%, the green economies of Asian countries do, in fact, converge to the same steady state. The club clustering algorithm identified a single club that is representative of the full sample. For the region, the club merging technique did not need to be used because the final club categorization consisted of just one club.
The club clustering algorithm results in a final club categorization of one for European countries, whereas the club clustering algorithm initially identified three clustering clubs. An examination of the results indicates the existence of convergence (since the t-test for club one is 4.463 > −1.65). The empirical findings corroborate the conclusions drawn by Yıldırım et al. (2021), who observed no disparities in environmental quality across 16 European countries. Furthermore, they align with the discoveries of Ulucak and Apergis (2018), who identified three convergence clubs among EU countries demonstrating convergence in environmental quality.
Lastly, the club clustering’s empirical results for Latin, North America, and the Caribbean indicate a divergence since the log(t) t-statistic of −10.7441 is less than −1.65 (the null hypothesis for entire sample convergence is rejected). This confirms the divergence in green economic growth of the Latin, North America, and Caribbean countries. The club merging technique was implemented to mitigate the risk of overestimating club memberships, resulting in a reduction of the final club classification to two, as illustrated in Panel B of Table 4. Club one comprises 17 countries, whereas Club 2 consists of 7 countries. The list of countries within each final club classification for the region is provided in Table 5. Notably, with decay rates of 28.2% and −0.45% for Clubs 1 and 2, respectively, Club 1 exhibits the highest rate of convergence based on its relative log(t) coefficients.
Following the proposition of Quah (1990) and Carlino and Mills, (1993), Carlino and Mills, (1996) that stationarity tests can be used to assess stochastic convergence. These studies argue that stochastic convergence occurs when a series is trend stationary. On the other hand, the presence of a unit root indicates that the shock is permanent, leading to the divergence of the series from the sample mean. Due to this, our study applied the stationarity test to assess whether there is convergence or divergence in green economic growth among the selected sample. We adopted the Fourier ADF unit root test since the traditional stationarity test (ADF and PP) cannot address structural breaks. As suggested by Enders and Lee (2012), the FADF unit root test exhibits superior power performance as it accounts for structural break and nonlinearity.
As presented in Figure 4, FADF test statistics for 48 countries are statistically significant since the p-values are less than a 5% significance level (For a list of these countries, see Supplementary Appendix Table A1 in the Appendix). Hence, green economic growth for these countries is stationary at a 5% significance level. Put differently, these countries' green economic growth converges to the World average of green economic growth. However, since the p-values of the remaining countries are not significant, we can conclude that there is a divergence in green economic growth. In other words, these countries' green economic growth does not converge to the World average of green economic growth.
Table 6 displays the average marginal effects on club membership probability, derived from both an ordered logit and probit model. It's crucial to acknowledge that while the size of the estimates may not inherently provide a straightforward economic interpretation, assessing the significance of explanatory variables in predicting club membership is vital before delving into economic implications. This evaluation is conducted by calculating the marginal effects of predicted probabilities, clarifying how a one-unit rise in an explanatory variable impacts the probability of membership in each club while keeping all other variables fixed at their respective sample averages (Potoski and Prakash, 2005; Bartkowska and Riedl, 2012; Von Lyncker and Thoennessen, 2017). Comprehension of the sign and magnitude of estimation coefficients is critical, as they indicate whether a variable amplifies or diminishes the likelihood of convergence into a particular club. To streamline our analysis, we primarily rely on empirical estimations from the ordered logit model.
The estimated results from Table 6 unveil intriguing insights. A 1% increase in real GDP correlates with a significant reduction in the probability of converging into Club 1, specifically by 0.842%. This finding suggests that elevated GDP growth often accompanies heightened energy consumption, which, in turn, exacerbates reliance on carbon-intensive fossil fuels such as coal, oil, and natural gas. Such dependence on carbon-centric energy sources may hinder the transition to sustainable practices and renewable energy, consequently impeding efforts to achieve convergence within the green economy.
Conversely, while the impact of real GDP growth on the probability of converging into Club 2 is statistically insignificant, there exists a marginal increase. A 1% rise in real GDP elevates the probability of converging into Club 2 by 0.452%. This finding prompts the speculation that economic growth could potentially foster innovation and technical advancement, leading to the development of novel strategies and methodologies that promote environmental sustainability.
To expedite the convergence of the green economy, nations experiencing faster GDP growth rates might consider allocating greater resources towards research and development initiatives in critical areas such as sustainable agriculture, waste management, renewable energy, and green transportation. Such strategic investments could catalyze transformative progress towards a more sustainable and environmentally resilient future.
Furthermore, the estimated coefficient of the squared term of real GDP indicates a positive and significant relationship for Club 1 and negative and significant relationship for Club 2. This infers that the nature of the relationship between the economy and the club membership of green economic convergence is characterized to be nonlinear (convex for Club one and concave for Club 2).
Trade openness exerts a positive and statistically significant influence on Club 1, while demonstrating a negative and statistically significant impact on Club 2. To be precise, a 1% increase in trade openness enhances the likelihood of converging into Club one by 0.2%, whereas it diminishes the probability of converging into Club two by 0.55%. We believe this is because trade liberalisation makes it easier for countries to share information, best practices, and environmentally beneficial technologies. Similarly, countries can obtain green technologies, expertise, and innovations created elsewhere through international commerce, which speeds up the adoption of sustainable practices and fosters convergence towards green economic objectives.
Furthermore, the estimated coefficient of CO2 emissions reduces the probability of club membership of Club 1 by 0.76% and increases the probability of joining Club 2 by 0.72%, if CO2 emissions increase by 1%. A possible explanation for the negative impact on Club 2 convergence could be because CO2 emissions contribute to air pollution, climate change, and ecosystem disruption in the environment. By reducing natural resources, endangering biodiversity, and jeopardizing ecosystem services necessary for sustainable development, this degradation weakens the basis of a green economy. However, we believe that the positive impact of Club two membership could be because countries may become increasingly aware of the need to address the climate change impact of CO2 emission. This acknowledgment may inspire initiatives and regulations meant to further sustainability and the shift to a green economy.
Population size contributes negatively to the probability of club membership of Club one by 0.3% and 0.03% to club membership of Club 2. The intuition behind this empirical outcome is that the need for natural resources like energy, land, and water rises as the population grows quickly. This increased demand has the potential to undermine efforts towards sustainable resource management and the convergence of the green economy by causing overexploitation, deforestation, habitat damage, and resource depletion. Similarly, natural resources contribute negatively to the probability of Club 1 membership by 0.04%. However, it increases Club 2 membership by 0.12%. For the negative impact of natural resources, we believe that natural resource exploitation, particularly when done in an unsustainable way, can result in habitat destruction, biodiversity loss, and environmental deterioration; all of which compromise the environmental sustainability required for the convergence of the green economy. However, the positive impact of club membership can be because countries with abundant natural resources, including those with geothermal, wind, or solar power, can use these resources to generate renewable energy and promote convergence of the green economy.
The estimate of renewable energy transition is negative and statistically significant, indicating that renewable energy reduces the probability of club membership of Club 1 and Club 1 by 0.003% and 0.12%, respectively. This suggests that the adoption of renewable energy might not necessarily translate to green economic growth convergence among countries. This is because some countries might have potential challenges like financial constraints, transition challenges, and technological limitations towards the adoption of renewable energy transition. Similarly, the coefficient of institutional governance reveals that it decreases the probability of club membership for Club 1 and 2 by 0.21% and 0.1%, respectively.
The study further examines the regional analysis on the drivers of club convergence of green economy. The empirical outcomes suggest that the relationship between economic growth and green economic growth convergence is characterized to be nonlinear. However, the nature of this nonlinearity differs across regions. For instance, it is convex for Africa and Europe, while the relationship is concave for Asia and Latin, North America, and Caribbean. Trade contributes positively to club membership of Club one across all the different regions. However, it negatively impacts the probability of Club two membership across all the regions.
CO2 emissions reduce the probability of club membership of Club one in Latin, North America, and Caribbean, Europe, and Asia. While it increases the club membership of Club one in Africa. Nonetheless, CO2 emissions increase the probability of club membership of Club 2 Latin, North America, and Caribbean, Europe, and Asia. Whereas the impact in Africa is negative. With the exception of Europe, population contributes positively to the probability of Club one membership. However, it contributes negatively to Club two membership in Africa, Asia, Latin, North America, and Caribbean, while it contributes positively to Europe. Furthermore, the estimated coefficient natural resources contribute negatively to club1 membership in Asia, Europe, and Latin, North America, and Caribbean, while it impacts positively in Africa.
Renewable energy transition is negative and statistically significant, suggesting that renewable energy reduces the probability of club membership of Club one in Asia, Europe, and Latin, North America, and Caribbean, while it impacts positively the probability of club membership in Africa. However, renewable energy transition is positive and significant on the probability of club membership of Club two in Asia, Europe, and Latin, North America, and Caribbean, but the impact is negative for countries in Africa. The empirical outcome of institutional governance contributes negatively to the probability of Club one membership in Africa and Latin, North America, and Caribbean, while it has positive and significant impact on probability of Club one membership in Asia and Europe. Nonetheless, institutional governance increase the probability of Club two membership in Africa and Latin, North America, and Caribbean, whereas, it has a significant and negative impact on Club two membership in Asia and Europe.
Two challenges remain for the world 20 years after the first Rio Summit: expanding economic opportunities for all in the context of a growing global population and managing environmental concerns that, if ignored, might make it more difficult for us to take advantage of this potential. GG represents the intersection of these two difficulties, and it involves seizing the chance to integrate the two. Hence, the literature is replete with unremitting debate on factors inimical to ecosystem and human survival. To foster sustainable development, the United Nations (2012) has advocated for various global actions, including the reinforcement of environmental protection against human-induced activities. This recommendation holds particular significance for policymakers. In crafting policies aimed at balancing economic growth with environmental considerations, it is essential to grasp global convergence patterns. Such understanding is paramount for advancing towards a green economy, as nations facing similar levels of environmental degradation can collaborate more effectively. By implementing shared environmental protection measures, countries can mitigate risks to human survival posed by environmental threats. Therefore, it is crucial to investigate how green economy is converging globally in order to formulate effective environmental protection and economic growth strategies for the various countries that make up the globe.
In examining the global convergence in green economic growth, we use the production-based CO2 productivity (US dollars per kilogram, 2015) of 134 countries from 1995 to 2022. Green economic growth provides a flexible and pragmatic method for attaining measurable progress across different dimensions of economic and environmental pillars, while considering the full account of social impact of greening the growth dynamics of these economies. This study examines the convergence characteristics of green economic growth in the 134 countries using the PS club clustering algorithm and the log t regression test. This method is better than earlier methods as it can accommodate the expected transitional heterogeneity in the panel while remaining robust to the stationarity features of the variable under consideration. In addition, the club convergence system can identify any instance of disequilibrium in the convergence and functions as a nonlinear model with time-varying properties.
Our empirical findings indicate a divergence in green economic growth within the global sample. However, upon applying the club clustering technique, we identified two convergence clubs: Club 1 comprising 99 countries and Club 2 with 42 countries. Expanding our analysis to regional levels, the results of the log(t) convergence tests reveal a lack of evidence for conditional or relative convergence across the full samples of Africa, Europe, Latin America, North America, and the Caribbean. Nonetheless, we observed club convergence within the Asian region. Additionally, our analysis revealed three convergence clubs in Africa, two in Latin America, North America, and the Caribbean, while Asia and Europe each exhibited a single club. These findings suggest that all countries within each region converge towards the same equilibrium, underscoring regional patterns of convergence in green economic growth.
In contrast to prior research on club convergence within the green economy, this empirical study not only explores the characteristics of such convergence but also contributes to the literature by delving into the potential drivers of identified club memberships. This is accomplished through an analysis of the determinants of club formation at both global and regional levels. The findings from the ordered logit and probit models suggest that the relationship between the economy and club membership in green economic convergence is nonlinear and exhibits a convex pattern for Club 1, while displaying a concave pattern for Club 2. Other variables like trade openness, CO2 emission, population growth, natural resource rent, renewable energy transition, and institutional governance are found to be crucial drivers of club membership of green economic growth both at the world level and regional levels.
The study’s outcomes underscore several significant policy implications: Firstly, fostering global collaboration and partnerships is imperative to address worldwide environmental challenges and promote sustainable development. Secondly, enhancing multilateral frameworks such as the United Nations Framework Convention on Climate Change (UNFCCC) and the United Nations Environment Program (UNEP) is crucial for facilitating knowledge sharing, technology transfer, and capacity building in green economy initiatives. Thirdly, advocating for urgent climate action and the effective implementation of the Paris Agreement through the reinforcement of national climate commitments (Nationally Determined Contributions, or NDCs) and the formulation of long-term decarbonization strategies is essential. This entails promoting increased ambition among countries, setting precise emission reduction targets, and implementing policies and measures to transition towards low-carbon and resilient economies. Fourth, supporting developing countries in their transition to a green economy by providing technology transfer, financial assistance, and capacity-building support is vital. Leveraging climate finance, green bonds, and investment funds can aid in funding renewable energy projects, sustainable infrastructure, and adaptation measures in vulnerable regions.
While our study aimed to address certain limitations in the current research, there is still potential for further improvement in future studies. It could be beneficial for future studies to incorporate alternative measures of green economic growth in order to enhance the current body of knowledge in this field.
This study offers valuable insights into the convergence dynamics of green economic growth and the drivers of this convergence. However, the study has a couple of limitations which could be looked at in future research: (1) The study only used the resources and environmental productivity and production-based CO2 productivity. Future studies could look at other green growth measures like the demand-based CO2 productivity; and (2) variables like economic growth, trade, renewable energy transition, natural resources, population growth, C02 emissions, and institutional quality were considered as the drivers. However, other variables like investments in green economy, green FDI, and financial sector have been argued to be a green growth driver, and could be looked at in future literature.
The raw data supporting the conclusions of this article will be made available by the authors, without undue reservation.
SA: Conceptualization, Data curation, Formal Analysis, Investigation, Methodology, Project administration, Software, Writing–original draft, Writing–review and editing. MB: Project administration, Supervision, Validation, Writing–review and editing.
The author(s) declare that no financial support was received for the research, authorship, and/or publication of this article.
The authors declare that the research was conducted in the absence of any commercial or financial relationships that could be construed as a potential conflict of interest.
All claims expressed in this article are solely those of the authors and do not necessarily represent those of their affiliated organizations, or those of the publisher, the editors and the reviewers. Any product that may be evaluated in this article, or claim that may be made by its manufacturer, is not guaranteed or endorsed by the publisher.
The Supplementary Material for this article can be found online at: https://www.frontiersin.org/articles/10.3389/fenvs.2025.1492749/full#supplementary-material
1Non convergent group
Adebayo, T. S., Onifade, S. T., Alola, A. A., and Muoneke, O. B. (2022). Does it take international integration of natural resources to ascend the ladder of environmental quality in the newly industrialized countries? Resour. Policy 76, 102616. doi:10.1016/j.resourpol.2022.102616
Ahmed, Z., Wang, Z., and Ali, S. (2019). Investigating the non-linear relationship between urbanization and CO 2 emissions: an empirical analysis. Air Qual. Atmos. heal. 12, 945–953. doi:10.1007/s11869-019-00711-x
Akram, V., and Ali, J. (2022). Do countries converge in natural resources rents? Evidence from club convergence analysis. Resour. Policy 77, 102743. doi:10.1016/j.resourpol.2022.102743
Antal, M., and Van Den Bergh, J. C. (2016). Green growth and climate change: conceptual and empirical considerations. Clim. Policy 16 (2), 165–177. doi:10.1080/14693062.2014.992003
Arogundade, S., Hassan, A., Akpa, E., and Mduduzi, B. (2023). Closer together or farther apart: are there club convergence in ecological footprint? Environ. Sci. Pollut. Res. 30 (6), 15293–15310. doi:10.1007/s11356-022-23203-5
Ashfaq, S., Liangrong, S., Waqas, F., Gulzar, S., Mujtaba, G., and Nasir, R. M. (2024). Renewable energy and green economic growth nexus: insights from simulated dynamic ARDL. Gondwana Res. 127, 288–300. doi:10.1016/j.gr.2023.08.014
Awan, A., Bilgili, F., and Bahadur, D. (2023). Household fuel choices and consumption intensity in Pakistan: evidence from HIES data 2001 – 2019. Environ. Sci. Pollut. Res. 1–16. doi:10.1007/s11356-023-27227-3
Awan, A., Bilgili, F., and Rahut, D. B., (2022). Energy poverty trends and determinants in Pakistan: empirical evidence from eight waves of HIES 1998–2019. Renew. Sustain. Energy Rev. 158, 112157. doi:10.1016/j.rser.2022.112157
Aziz, G., and Bakoben, H. B. M. (2024). Environmental decentralization and green economic growth: do renewable energy development play any role? Energy Strategy Rev. 54, 101459. doi:10.1016/j.esr.2024.101459
Bartkowska, M., and Riedl, A. (2012). Regional convergence clubs in Europe: identification and conditioning factors. Econ. Model. 29 (1), 22–31. doi:10.1016/j.econmod.2011.01.013
Bekun, F. V., Gyamfi, B. A., Onifade, S. T., and Agboola, M. O. (2021). Beyond the environmental Kuznets Curve in E7 economies: accounting for the combined impacts of institutional quality and renewables. J. Clean. Prod. 314, 127924. doi:10.1016/j.jclepro.2021.127924
Bigerna, S., Bollino, C. A., and Polinori, P. (2021). Convergence in renewable energy sources diffusion worldwide. J. Environ. Manag. 292, 112784. doi:10.1016/j.jenvman.2021.112784
Blampied, N. (2021). Economic growth, environmental constraints and convergence: the declining growth premium for developing economies. Ecol. Econ. 181, 106919. doi:10.1016/j.ecolecon.2020.106919
Bleischwitz, R. (2010). International economics of resource productivity–Relevance, measurement, empirical trends, innovation, resource policies. Int. Econ. Econ. Policy 7, 227–244. doi:10.1007/s10368-010-0170-z
Cao, J., Law, S. H., Samad, A. R. B. A., Mohamad, W. N. B. W., Wang, J., and Yang, X. (2022). Effect of financial development and technological innovation on green growth—analysis based on spatial Durbin model. J. Clean. Prod. 365, 132865. doi:10.1016/j.jclepro.2022.132865
Carlino, G. A., and Mills, L. (1996). Testing neoclassical convergence in regional incomes and earnings. regional Sci. urban Econ. 26 (6), 565–590. doi:10.1016/s0166-0462(96)02137-0
Carlino, G. A., and Mills, L. O. (1993). Are US regional incomes converging? a time series analysis. J. monetary Econ. 32 (2), 335–346. doi:10.1016/0304-3932(93)90009-5
Caves, D. W., Christensen, L. R., and Diewert, W. E. (1982). The economic theory of index numbers and the measurement of input, output, and productivity. Econ. J. Econ. Soc. 50, 1393–1414. doi:10.2307/1913388
Chen, G., Yang, Z., and Chen, S. (2020). Measurement and convergence analysis of inclusive green growth in the yangtze river economic belt cities. Sustainability 12, 2356. doi:10.3390/su12062356
D’Alessandro, S., Cieplinski, A., Distefano, T., and Dittmer, K. (2020). Feasible alternatives to green growth. Nat. Sustain. 3 (4), 329–335. doi:10.1038/s41893-020-0484-y
Dong, K., Wang, B., Zhao, J., and Taghizadeh-Hesary, F. (2022). Mitigating carbon emissions by accelerating green growth in China. Econ. Analysis Policy 75, 226–243. doi:10.1016/j.eap.2022.05.011
Elamer, A. A., Ntim, C. G., and Abdou, H. A. (2020). Islamic governance, national governance, and bank risk management and disclosure in MENA countries. Bus. Soc. 59, 914–955. doi:10.1177/0007650317746108
Eleftheriou, K., Nijkamp, P., and Polemis, M. L. (2024). Club convergence of sustainable development: fresh evidence from developing and developed countries. Econ. Change Restruct. 57 (2), 32. doi:10.1007/s10644-024-09617-w
Enders, W., and Lee, J. (2012). The flexible Fourier form and Dickey–Fuller type unit root tests. Econ. Lett. 117 (1), 196–199. doi:10.1016/j.econlet.2012.04.081
Fang, C., Cheng, J., Zhu, Y., Chen, J., and Peng, X. (2021). Green total factor productivity of extractive industries in China: an explanation from technology heterogeneity. Resour. Policy 70, 101933. doi:10.1016/j.resourpol.2020.101933
Fernandes, C. I., Veiga, P. M., Ferreira, J. J., and Hughes, M. (2021). Green growth versus economic growth: do sustainable technology transfer and innovations lead to an imperfect choice? Bus. Strategy Environ. 30 (4), 2021–2037. doi:10.1002/bse.2730
Geng, Q., Wang, Y., and Wang, X. (2023). The impact of natural resource endowment and green finance on green economic efficiency in the context of COP26. Resour. Policy 80, 103246. doi:10.1016/j.resourpol.2022.103246
González-Álvarez, M. A., Montañés, A., and Olmos, L. (2020). Towards a sustainable energy scenario? A worldwide analysis. Energy Econ. 87, 104738. doi:10.1016/j.eneco.2020.104738
Guo, L., Qu, Y., and Tseng, M. L. (2017). The interaction effects of environmental regulation and technological innovation on regional green growth performance. J. Clean. Prod. 162, 894–902. doi:10.1016/j.jclepro.2017.05.210
Gyamfi, B. A., Ozturk, I., Bein, M. A., and Bekun, F. V. (2021). An investigation into the anthropogenic effect of biomass energy utilization and economic sustainability on environmental degradation in E7 economies. Biofuels, Bioprod. Biorefining 15 (3), 840–851. doi:10.1002/bbb.2206
Haider, S., and Akram, V. (2019). Club convergence analysis of ecological and carbon footprint: evidence from a cross-country analysis. Carbon Manag. 10, 451–463. doi:10.1080/17583004.2019.1640135
Haider, S., Malik, S. M., Nadeem, B., Sadiq, N., and Ghaffari, A. S. (2021). Impact of population growth on the natural resources of Cholistan desert. PalArch’s J. Archaeol. Egypt/Egyptology 18 (10), 1778–1790.
Heede, R. (2014). Tracing anthropogenic carbon dioxide and methane emissions to fossil fuel and cement producers, 1854–2010. Clim. change 122 (1), 229–241. doi:10.1007/s10584-013-0986-y
Hickel, J., and Kallis, G. (2020). Is green growth possible? New polit. Econ. 25 (4), 469–486. doi:10.1080/13563467.2019.1598964
Hu, J. (2023). Green productivity growth and convergence in Chinese agriculture. J. Environ. Plan. Manag. 67, 1775–1804. doi:10.1080/09640568.2023.2180350
Huang, L., and Zhao, W. (2022). The impact of green trade and green growth on natural resources. Resour. Policy 77. doi:10.1016/j.resourpol.2022.102749
Jahanger, A., Hossain, M. R., Awan, A., Sunday Adebayo, T., and Zubair Chishti, M. (2023). Linking tourist’s footprint and environmental tragedy through transportation, globalization and energy choice in BIMSTEC region: directions for a sustainable solution using novel GMM-PVAR approach. J. Environ. Manage. 345, 118551. doi:10.1016/j.jenvman.2023.118551
Johnston, C. (2020). Essays in labor economics and econometric methods. Berkeley: University of California.
Jouvet, P. A., and de Perthuis, C. (2013). Green growth: from intention to implementation. Int. Econ. 134, 29–55. doi:10.1016/j.inteco.2013.05.003
Kasztelan, A. (2017). Green growth, green economy and sustainable development: terminological and relational discourse. Prague Econ. Pap. 26 (4), 487–499. doi:10.18267/j.pep.626
Keeling, C. D. (1973). Industrial production of carbon dioxide from fossil fuels and limestone. Tellus 25 (2), 174–198. doi:10.3402/tellusa.v25i2.9652
Kirikkaleli, D., and Addai, K. (2023). The asymmetric and long-run effect of demand-based CO2 emissions productivity on production-based CO2 emissions in the UK. Energy and Environ. doi:10.1177/0958305x231204958
Kumar, P. (2017). Innovative tools and new metrics for inclusive green economy. Curr. Opin. Environ. Sustain. 24, 47–51. doi:10.1016/j.cosust.2017.01.012
Li, B., and Haneklaus, N. (2021). The role of renewable energy, fossil fuel consumption, urbanization and economic growth on CO2 emissions in China. Energy Rep. 7, 783–791. doi:10.1016/j.egyr.2021.09.194
Li, J., Dong, K., and Dong, X. (2022). Green energy as a new determinant of green growth in China: the role of green technological innovation. Energy Econ. 114, 106260. doi:10.1016/j.eneco.2022.106260
Lozano, S., and Gutierrez, E. (2008). Non-parametric frontier approach to modelling the relationships among population, GDP, energy consumption and CO2 emissions. Ecol. Econ. 66 (4), 687–699. doi:10.1016/j.ecolecon.2007.11.003
Mahmood, N., Zhao, Y., Lou, Q., and Geng, J. (2022). Role of environmental regulations and eco-innovation in energy structure transition for green growth: evidence from OECD. Technol. Forecast. Soc. Change 183, 121890. doi:10.1016/j.techfore.2022.121890
Maiti, M. (2022). Does development in venture capital investments influence green growth? Technol. Forecast. Soc. Change 182, 121878. doi:10.1016/j.techfore.2022.121878
Maji, I. K. (2019). Impact of clean energy and inclusive development on CO2 emissions in sub-Saharan Africa. J. Clean. Prod. 240, 118186. doi:10.1016/j.jclepro.2019.118186
Mensah, C. N., Long, X., Dauda, L., Boamah, K. B., Salman, M., Appiah-Twum, F., et al. (2019). Technological innovation and green growth in the organization for economic cooperation and development economies. J. Clean. Prod. 240, 118204. doi:10.1016/j.jclepro.2019.118204
Miraz, M., and Soo, T. (2024). Factors affecting the green economy: the mediating role of foreign direct investment. J. Econ. Stud. doi:10.1108/JES01-2024-0012
Moldan, B., Janoušková, S., and Hák, T. (2012). How to understand and measure environmental sustainability: indicators and targets. Ecol. Indic. 17, 4–13. doi:10.1016/j.ecolind.2011.04.033
Nathaniel, S. P., Yalciner, K., and Bekun, F. V. (2021). Assessing the environmental sustainability corridor: linking natural resources, renewable energy, human capital, and ecological footprint in BRICS. Res. Pol. 70, 101924. doi:10.1016/j.resourpol.2020.101924
Ngo, T. Q. (2024). Natural resource rents, clean energy, and green total factor productivity. Evidence from Vietnam in pre-post Covid era. Resour. Policy 88, 104401. doi:10.1016/j.resourpol.2023.104401
Pettersson, F., Maddison, D., Acar, S., and Söderholm, P. (2014). Convergence of carbon dioxide emissions: a review of the literature. Int. Rev. Environ. Resour. Econ. 7 (2), 141–178. doi:10.1561/101.00000059
Phillips, P. C., and Sul, D. (2007). Transition modeling and econometric convergence tests. Econometrica 75 (6), 1771–1855. doi:10.1111/j.1468-0262.2007.00811.x
Phillips, P. C., and Sul, D. (2009). Economic transition and growth. J. Appl. Econ. 24 (7), 1153–1185. doi:10.1002/jae.1080
Pinar, M. (2024). Convergence in renewable energy innovation and factors influencing convergence club formation. Renew. Energy 220, 119607. doi:10.1016/j.renene.2023.119607
Porada Rochoń, M. (2021). Convergence in green growth as the key to fighting climate change, 1990–2019. Energies 14 (24), 8324. doi:10.3390/en14248324
Potoski, M., and Prakash, A. (2005). Green clubs and voluntary governance: ISO 14001 and firms' regulatory compliance. Am. J. political Sci. 49 (2), 235–248. doi:10.2307/3647674
Qiang, Q., and Jian, C. (2020). Natural resource endowment, institutional quality and China's regional economic growth. Resour. Policy 66, 101644. doi:10.1016/j.resourpol.2020.101644
Quadrelli, R., and Peterson, S. (2007). The energy–climate challenge: recent trends in CO2 emissions from fuel combustion. Energy policy 35 (11), 5938–5952. doi:10.1016/j.enpol.2007.07.001
Quah, D. (1990). Permanent and transitory movements in labor income: an explanation for “excess smoothness” in consumption. J. Political Econ. 98 (3), 449–475. doi:10.1086/261690
Reilly, J. M. (2012). Green growth and the efficient use of natural resources. Energy Econ. 34, S85–S93. doi:10.1016/j.eneco.2012.08.033
Ren, S., Hao, Y., and Wu, H. (2022). The role of outward foreign direct investment (OFDI) on green total factor energy efficiency: does institutional quality matters? Evidence from China. Resour. Policy 76, 102587. doi:10.1016/j.resourpol.2022.102587
Saba, C. S., and Ngepah, N. (2022). Convergence in renewable energy consumption and their influencing factors across regions: evidence from convergence algorithm approach. Environ. Sci. Pollut. Res. 29 (40), 61412–61445. doi:10.1007/s11356-022-19731-9
Sandberg, M., Klockars, K., and Wilén, K. (2019). Green growth or degrowth? Assessing the normative justifications for environmental sustainability and economic growth through critical social theory. J. Clean. Prod. 206, 133–141. doi:10.1016/j.jclepro.2018.09.175
Shahbaz, M., Nasreen, S., Ling, C. H., and Sbia, R. (2014). Causality between trade openness and energy consumption: what causes what in high, middle and low income countries. Energy policy 70, 126–143. doi:10.1016/j.enpol.2014.03.029
Shang, Y., Lian, Y., Chen, H., and Qian, F. (2023). The impacts of energy resource and tourism on green growth: evidence from Asian economies. Resour. Policy 81, 103359. doi:10.1016/j.resourpol.2023.103359
Shen, A., Shao, A., Chen, J., and Cai, J. (2022). The club convergence of green productivity across African countries. Environ. Sci. Pollut. Res. 29, 4722–4735. doi:10.1007/s11356-021-15790-6
Shen, Y., Su, Z. W., Malik, M. Y., Umar, M., Khan, Z., and Khan, M. (2021). Does green investment, financial development and natural resources rent limit carbon emissions? A provincial panel analysis of China. Sci. Total Environ. 755, 142538. doi:10.1016/j.scitotenv.2020.142538
Sohag, K., Taşkın, F. D., and Malik, M. N. (2019). Green economic growth, cleaner energy and militarization: evidence from Turkey. Resour. Policy 63, 101407. doi:10.1016/j.resourpol.2019.101407
Solarin, S. A. (2014). Convergence of CO2 emission levels: evidence from African countries. J. Econ. Res. 19, 65–92.
Song, X., Zhou, Y., and Jia, W. (2019). How do economic openness and R&D investment affect green economic growth? evidence from China. Resour. Conservation Recycl. 146, 405–415. doi:10.1016/j.resconrec.2019.03.050
Stern, D. I. (2017). The environmental Kuznets curve after 25 years. J. Bioeconomics 19, 7–28. doi:10.1007/s10818-017-9243-1
Sueyoshi, T., and Wang, D. D. (2020). Rank dynamics and club convergence of sustainable development for countries around the world. J. Clean. Prod. 250, 119480. doi:10.1016/j.jclepro.2019.119480
Tariq, M., Xu, Y., Ullah, K., and Dong, B. (2024). Toward low-carbon emissions and green growth for sustainable development in emerging economies: do green trade openness, eco-innovation, and carbon price matter? Sustain. Dev. 32 (1), 959–978. doi:10.1002/sd.2711
Tews, K., Busch, P. O., and Jörgens, H. (2003). The diffusion of new environmental policy instruments 1. Eur. J. political Res. 42 (4), 569–600. doi:10.1111/1475-6765.00096
Tiwari, A. K., Kocoglu, M., Banday, U. J., and Awan, A. (2022). Hydropower, human capital, urbanization and ecological footprints nexus in China and Brazil: evidence from quantile ARDL. Environ. Sci. Pollut. Res. 29, 68923–68940. doi:10.1007/s11356-022-20320-z
Tiwari, A. K., Nasir, M. A., Shahbaz, M., and Raheem, I. D. (2021). Convergence and club convergence of CO2 emissions at state levels: a nonlinear analysis of the USA. J. Clean. Prod. 288, 125093. doi:10.1016/j.jclepro.2020.125093
Trinh, H. H., McCord, M., Lo, D., and Squires, G. (2023). Do green growth and technological innovation matter to infrastructure investments in the era of climate change? Global evidence. Appl. Econ. 55 (35), 4108–4129. doi:10.1080/00036846.2022.2125493
Tufail, M., Song, L., Adebayo, T. S., Kirikkaleli, D., and Khan, S. (2021). Do fiscal decentralization and natural resources rent curb carbon emissions? Evidence from developed countries. Environ. Sci. Pollut. Res. 28 (35), 49179–49190. doi:10.1007/s11356-021-13865-y
Tunyi, A. A., Ehalaiye, D., Gyapong, E., and Ntim, C. G. (2020). The value of discretion in Africa: evidence from acquired intangible assets under IFRS 3. Int. J. Acc. 55, 2050008. doi:10.1142/s1094406020500080
Ulucak, R. (2020). How do environmental technologies affect green growth? Evidence from BRICS economies. Sci. Total Environ. 712, 136504. doi:10.1016/j.scitotenv.2020.136504
Ulucak, R. (2021). A revisit to the relationship between financial development and energy consumption: is globalization paramount? Energy 227, 120337. doi:10.1016/j.energy.2021.120337
Ulucak, R., and Apergis, N. (2018). Does convergence really matter for the environment? An application based on club convergence and on the ecological footprint concept for the EU countries. Environ. Sci. Policy 80, 21–27. doi:10.1016/j.envsci.2017.11.002
Ulucak, R., Kassouri, Y., Çağrı İlkay, S., Altıntaş, H., and Garang, A. P. M. (2020). Does convergence contribute to reshaping sustainable development policies? Insights from Sub-Saharan Africa. Ecol. Indic. 112, 106140. doi:10.1016/j.ecolind.2020.106140
UNFCC (2024). UN climate change conference. Available online at: https://unfccc.int/cop28/blogs.
Von Lyncker, K., and Thoennessen, R. (2017). Regional club convergence in the EU: evidence from a panel data analysis. Empir. Econ. 52, 525–553. doi:10.1007/s00181-016-1096-2
Wang, L., Vo, X. V., Shahbaz, M., and Ak, A. (2020). Globalization and carbon emissions: is there any role of agriculture value-added, financial development, and natural resource rent in the aftermath of COP21? J. Environ. Manag. 268, 110712. doi:10.1016/j.jenvman.2020.110712
Wang, X., and Shao, Q. (2019). Non-linear effects of heterogeneous environmental regulations on green growth in G20 countries: evidence from panel threshold regression. Sci. Total Environ. 660, 1346–1354. doi:10.1016/j.scitotenv.2019.01.094
Wu, R., Ma, T., and Schröder, E. (2022). The contribution of trade to production-based carbon dioxide emissions. Struct. Change Econ. Dyn. 60, 391–406. doi:10.1016/j.strueco.2021.12.005
Wu, S., Cheng, P., and Yang, F. (2024). Study on the impact of digital transformation on green competitive advantage: the role of green innovation and government regulation. PLoS One 19 (8), e0306603–e0306614. doi:10.1371/journal.pone.0306603
Wuebbles, D. J., and Jain, A. K. (2001). Concerns about climate change and the role of fossil fuel use. Fuel Process. Technol. 71 (1-3), 99–119. doi:10.1016/s0378-3820(01)00139-4
Xu, J., Zhao, J., She, S., and Liu, W. (2022). Green growth, natural resources and sustainable development: evidence from BRICS economies. Resour. Policy 79, 103032. doi:10.1016/j.resourpol.2022.103032
Xu, K., Mei, R., Liang, L., and Sun, W. (2023). Regional convergence analysis of sustainable innovation efficiency in European Union countries. J. Environ. Manag. 325, 116636. doi:10.1016/j.jenvman.2022.116636
Xu, X. (2022). The impact of natural resources on green growth: the role of green trade. Resour. Policy 78, 102720. doi:10.1016/j.resourpol.2022.102720
Yıldırım, D. Ç. Y., Yıldırım, S., Erdoğan, S., Demirtaş, I., Couto, G., and Castanho, R. A. (2021). Time-varying convergences of environmental footprint levels between European countries. Energies 14 (1813), 1813. doi:10.3390/en14071813
Zhang, F., Wang, Q., and Li, R. (2024). Linking natural resource abundance and green growth: the role of energy transition. Resour. Policy 91, 104898. doi:10.1016/j.resourpol.2024.104898
Zhang, Y., Jin, W., and Xu, M. (2021). Total factor efficiency and convergence analysis of renewable energy in Latin American countries. Renew. Energy 170, 785–795. doi:10.1016/j.renene.2021.02.016
Zhao, J., Shahbaz, M., and Dong, K. (2022a). How does energy poverty eradication promote green growth in China? The role of technological innovation. Technol. Forecast. Soc. Change 175, 121384. doi:10.1016/j.techfore.2021.121384
Zhao, X., Mahendru, M., Ma, X., Rao, A., and Shang, Y. (2022b). Impacts of environmental regulations on green economic growth in China: new guidelines regarding renewable energy and energy efficiency. Renew. Energy 187, 728–742. doi:10.1016/j.renene.2022.01.076
Zheng, W., Zhang, L., and Hu, J. (2022). Green credit, carbon emission and high quality development of green economy in China. Energy Rep. 8, 12215–12226. doi:10.1016/j.egyr.2022.09.013
Keywords: convergence, PS club clustering, sustainable development, green economic growth, world
Citation: Arogundade S and Biyase M (2025) Is the world becoming a “greener” place? empirics of green economic growth convergence and its determinants. Front. Environ. Sci. 13:1492749. doi: 10.3389/fenvs.2025.1492749
Received: 07 September 2024; Accepted: 17 February 2025;
Published: 18 March 2025.
Edited by:
Mobeen Ur Rehman, Keele University, United KingdomCopyright © 2025 Arogundade and Biyase. This is an open-access article distributed under the terms of the Creative Commons Attribution License (CC BY). The use, distribution or reproduction in other forums is permitted, provided the original author(s) and the copyright owner(s) are credited and that the original publication in this journal is cited, in accordance with accepted academic practice. No use, distribution or reproduction is permitted which does not comply with these terms.
*Correspondence: Sodiq Arogundade, YXJvZ3VuZGFkZXNvZGlxOEBnbWFpbC5jb20=
Disclaimer: All claims expressed in this article are solely those of the authors and do not necessarily represent those of their affiliated organizations, or those of the publisher, the editors and the reviewers. Any product that may be evaluated in this article or claim that may be made by its manufacturer is not guaranteed or endorsed by the publisher.
Research integrity at Frontiers
Learn more about the work of our research integrity team to safeguard the quality of each article we publish.