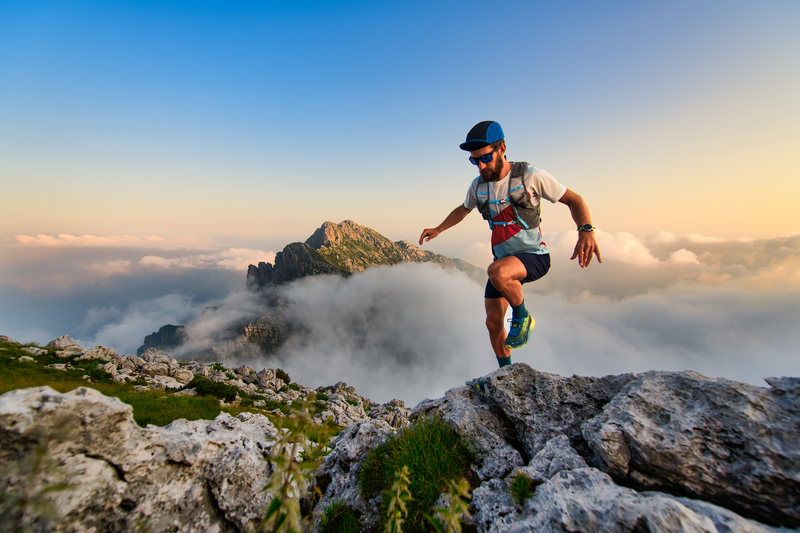
95% of researchers rate our articles as excellent or good
Learn more about the work of our research integrity team to safeguard the quality of each article we publish.
Find out more
ORIGINAL RESEARCH article
Front. Environ. Sci. , 21 February 2025
Sec. Environmental Economics and Management
Volume 13 - 2025 | https://doi.org/10.3389/fenvs.2025.1443405
This article is part of the Research Topic Urban Carbon Emissions and Anthropogenic Activities View all 18 articles
The application of digital technology and the emergence of new economic forms have accelerated economic and social dynamic circulation, and the digital economy industry has achieved positive results in enhancing regional carbon emission efficiency. Therefore, exploring the carbon footprint of the digital economy system and the new development model of “dual circulation” from the perspective of high-quality development is important to ensure its healthy development. This study is based on the theory of high-quality development. It uses panel models, spatial econometric models, and other methods for empirical analysis of the level of digital economy development and carbon emission efficiency in more than 25 provinces in China and also of their impact effects. The results indicated that under the post-epidemic situation, the digital economy level of various provinces in China has improved to varying degrees, especially in the Beijing, Tianjin, Hebei, and Pearl River Delta regions, where the improvement effect is significant. The carbon emission efficiency showed a decreasing trend from east to west in the spatial dimension. The digital economy was significantly positively correlated with carbon emission efficiency at the 1% level. In comparison, the negative effects of urbanization level and government macro intervention variables were significant at the 5% and 10% levels. The adjustment of industrial structure, energy technology, and development of the digital economy had significant spatial spillover effects and heterogeneity. When the digital economy improved carbon emission efficiency, a certain degree of peripheral inhibition was observed. From the perspective of high-quality development, the digital economy needs to focus on the “simultaneous realization and maintenance” of economic and ecological benefits and actively adjust the industrial structure and energy optimization based on regional differences.
Faced with the current trend of digital transformation and development in the world economy, its scale anddepth of development are also constantly deepening and expanding. As an important lever to enhance economic development and build new competitive advantages and new patterns, it has obvious advantages in leveraging China’s market advantages and domestic demand potential. The research and application of digital technology such as big data, artificial intelligence (AI), and cloud computing are continuously promoting technological innovation, and new economic formats are emerging one after another. The dynamic cycle system of economic development is also constantly being optimized (Yu and Zhu, 2023; Han et al., 2022). The report of the 20th National Congress of the Communist Party of China proposed accelerating the digital economy development (DED) and stimulating the deep and multi-domain development of digital technology through the deployment of economic network infrastructure (Hussain et al., 2022). Digital economy has become a new engine for promoting economic and social high-quality development (HQD). As an economic system based on digital technology, it covers a wide range of connotations. In the China Digital Economy Development Report (2022), the extent of industrial digitization in China exceeded 80% in 2021. As a new direction of industrial development, digital economy is closely related to carbon emissions (CEs) (Ren et al., 2022). According to relevant data, there are differences in the carbon footprint (CFP) of industrial activities in China in the 21st century, and the ecological deficit caused by the scarcity in productive land area is more obvious, making it difficult to compensate for the CFP of the industrial space (Hertwich, 2021). With the increasing severity of global climate change, reducing CEs and achieving sustainable economic development have become urgent issues that need to be addressed by countries and regions. The digital economy accelerates the penetration and integration of digital technology and digital elements into deeper and wider fields by continuously upgrading network infrastructure and information tools such as intelligent machines. It also promotes the transformation of the economic form from industrial economy to smart economy, thereby bringing about a change in the overall economic operation mode. In the context of carbon peak and carbon neutrality, high-energy-consuming industries urgently need to be transformed and upgraded, and residents’ consumption patterns need to be optimized urgently. Therefore, combining digital economy with green development is the only way and key technology to promote the “dual carbon” goal and high-quality economic development (Chen et al., 2024). In the 14th Five Year Plan and 2035 Vision Goal Outline, it is also proposed to that the intelligence level of cities be improved, digital management and operation of cities be promoted, and the concept of “low-carbon” cities be advanced (Saeed et al., 2023). Therefore, conducting research on China’s provincial level is in line with the requirements of China’s development strategy and an important part of adapting to future economic development. There are differences in industrial structure, resource endowment, and technological level among different provinces. At the provincial level, research can provide a scientific basis for the development of each province, improve resource utilization efficiency, and serve as a crucial strategic support for achieving China’s long-term carbon neutrality goals.
Within different economic circles, the impact of DED on CEs varies, and the spatial boundary effects that it presents also differ to some extent. Among them, the development of digital industries, digital innovation capabilities, and digital inclusive finance are important factors regarding the impact of the digital economy on urban CEs. The digital economy has driven the development of information and communication technology, while remote sensing technology, Internet of Things devices, and online data analysis tools have improved the efficiency of economic activities. Digital transformation may lead to changes in energy consumption patterns, which can help automate CE trading markets. The relationship between the digital economy and emission efficiency depends on multiple factors, especially the application of digital technology and the policy environment in which it operates. To maximize the use of the digital economy to improve emission efficiency, it is necessary to strategically plan and adjust energy use and emissions, while encouraging responsible technology use and innovation. Related studies have shown that there is significant spatial heterogeneity in the development of China’s digital economy, and the development pattern has shifted from a “multi-point” sporadic distribution to a “clustered” aggregation form. However, the gap in development levels among cities has not improved (Wang and Zhong, 2023). The digital economy, with the Internet and big data as its main components, has become an important booster for China’s HQD. The integration of digital technology provides an opportunity for economic green and low-carbon transformation (LCT), and carbon emission efficiency (CEE) and CFP are related to factors such as energy structure, industrial upgrading, and technological innovation. Exploring the relationship between DED and CE is of great practical significance in handling the difficulties posed by global climate change to the dual circulation pattern. Most studies only concentrate on the CE effect of energy consumption (EC) in the digital industry, which tends to overlook the technology spillover situation in the digital industry, making it difficult to present actual results. In this context, this study combines theoretical analysis with empirical testing to explore the empirical impacts of DED on CE through theoretical model design and econometric data collection. This study innovatively utilizes the perspective of HQD to enrich the empirical content of the relationship between the two. In addition, the refinement of variable indicator data and the consideration of mediating variables from multiple perspectives can also provide theoretical support and policy recommendations for achieving sustainable HQD.
Digital economy is an essential endogenous driving force for driving urban economic development. Yu et al. discussed panel data of Chinese cities from 2011 to 2019 and examined the impact of DED level on carbon reduction. Digital economy had an inhibitory effect on the development of CE, showing significant heterogeneity, and it could effectively intervene in CE by regulating green energy efficiency (Yu et al., 2022). Zhong et al. conducted a research on the relationship between agricultural CE and economic HQD using panel data, spatial Durbin model (SDM), and mediation effect (ME) models. At present, the carbon intensity level of agriculture in China is still relatively high, and adjustment of agricultural technology could effectively play an intermediary role. Therefore, the application and DED in agricultural technology should be strengthened in the later stage (Zhong et al., 2022). Tan et al. focused on the ME of industrial structure upgrading (ISU) on the correlation between digital economy and low-carbon sustainable development (LCSD) and tested it using urban regression models. ISU could provide an external positive environment for the DED and reduce CE (Tan et al., 2024). In response to the LCT of the manufacturing economy, T. Wu combined qualitative and quantitative analyses, using theoretical and empirical methods to perform an analysis on 30 provinces in China. Digital economy could reduce CE, and its integration with technology-intensive manufacturing could effectively leverage the multiplier effect of carbon reduction (Wu et al., 2023). Wang et al. explored the relationship between digital economy and urban LCSD from a climate perspective using panel data and fixed-effects (FE) models. Promoting digital economy could effectively promote urban LCSD, and the flow of innovative factors was an important influencing factor. The influence of digital economy in urban LCSD has increased (Wang et al., 2022). Cui et al. innovatively analyzed the relationship between productive capital stock and CE from the perspective of China’s information and communication technology calculation. Wireless communication in information technology could indirectly reduce CE through DE, and a non-linear connection was observed between DE and CE. The degree of influence mechanism was related to the type of wireless communication (Cui et al., 2023). The framework of the Environmental Kuznets Curve assumption, the relationship between CE and natural resource exploitation, globalization index, economic growth, and population aging presented an inverted U-shaped relationship. Strengthening the sustainable development goals under multiple objectives had important practical significance. Shang et al. used the Tapio decoupling model to explore the relationship between carbon dioxide and driving factors, and they employed parallel deep learning algorithms to design a perceptual neural network. They constructed a partial least squares regression model to analyze the driving factors. The results indicated that the variable importance of urbanization rate in predicting the output had a strong inhibitory effect on CFP growth, and the marginal effect relationship between CFP and economic growth had stages (Shang and Luo, 2021). Regarding the EC and CEs of 5G mobile networks, Li et al. developed a data-driven framework and coordinated the working status of 5G batteries using DeepEnergy energy-saving methods and deep reinforcement learning neural networks. The results indicated that this method had great potential in reducing CEs, and its integration with solar energy systems could further promote the development of energy-efficient telecommunications (Li et al., 2023). Based on the perspective of ecological environment improvement research, Wang et al. explored the impact and mechanism of green finance on the economic and social green development space under panel data from 30 provinces and cities in China (2011–2020) through FEs and moderation effects models. The results indicated that the development of green finance in China has significantly expanded the green development space of the economy and society. The improvement of the ecological environment played an important intermediary and regulatory role, which could achieve high-quality and sustainable development of the economy and society (Wang et al., 2023).
Green development is an important part of the transformation and upgrading of other enterprises. Gao et al. analyzed the energy-saving and CE reduction ability of the digital economy from the perspective of technological structure transformation using a bidirectional FE model and an ME model. The improvement of green technology efficiency could reduce EC (Gao and Peng, 2023). Patterson et al. analyzed CE using machine learning techniques and found that reducing the EC of machine learning is of great significance in reducing the total CE (Patterson et al., 2022). Müller L J et al. analyzed the CFP raw materials and found that capturing CO2 carbon sources can significantly reduce their emissions and that accelerating the optimization of CFP indicator evaluation methods can analyze CO2 under different energy demands (Müller et al., 2020). Valls-Val K et al. believed that from the perspective of CE evaluation content, CFP should strengthen the analysis of the impact of different key elements on it, further improve the emission coefficient database, and develop reasonable processing tools, which was a long and arduous task (Valls-Val and Bovea, 2021). Wood et al. believed that the formulation of strategies for CFP control needs to consider trade composition and driving factors, among which greenhouse gases are crucial for analyzing the industrial and agricultural sectors (Wood et al., 2020). Mi et al. analyzed the CFP of 10 households with different income groups in China using multi-regional input–output analysis and found that CFP showed regional differences and that economic growth can improve carbon inequality (Mi et al., 2020). Shahbaz et al. considered the potential relationship between digital economy and energy transition and used panel data from 72 countries between 2003 and 2019 to study the impact of the digital economy on renewable EC and power generation structure. They analyzed the mediating role of government governance, indicating that the digital economy stimulated the transition to renewable energy by enhancing government governance capabilities (Shahbaz et al., 2022). The market-oriented trading mechanism is an important means for the Chinese government to control environmental pollution. Xuan et al. used a differential model to explore the impact of CE trading policies on CE reduction. The results indicated that CE trading policies, economic development, technological research level, and opening up to the outside world could significantly reduce CE intensity (Xuan et al., 2020). Adams et al. found that when analyzing the relationship between the economy and EC of countries with high geopolitical risks from 1996 to 2017, the uncertainty index had a co-integration relationship between EC, economic growth, geopolitical risks, economic policy uncertainty, and CE. There was a one-way causal relationship between CE and geopolitical risks, making significant adjustments to energy policies to better adapt to economic policy uncertainty and geopolitical risks (Adams et al., 2020).
Most previous studies demonstrated that the evaluation of CFP requires the support of a large amount of data, and there are differences in the standards for CE selection among different industries and regions. CFP is closely related to carbon reduction, and it is difficult to accurately evaluate CFP solely through macro-surveys conducted by DE. Therefore, the research mainly focuses on carbon reduction, indirectly demonstrating the impact of CFP. This paper analyzes the correlation between digital economy and carbon reduction efficiency (CRE) from multiple aspects and considers the impact of various mediating variables on urban CFP, which can effectively provide the reference value for urban HQD.
Digital economy has accelerated the development of information-related industries and also promoted digital technology penetration and integration in traditional industries, causing a huge improvement in the degree of economic intelligence. On the one hand, digital technology relies on intelligent information technology to improve scientific decision-making efficiency, while reducing CE while improving energy utilization efficiency. For example, the dynamic assessment of market data by digital technology platforms can facilitate a more comprehensive analysis of the trajectory and supply–demand dynamics of the energy economy. This, in turn, enables the formulation of macro-level policies that regulate energy market transactions (Luo et al., 2023). On the other hand, the upgrading and transformation of traditional industries through digital technology can reduce the clustering of high pollution emission industries, stimulate the vertical flow of production factors, and improve resource allocation efficiency. The enhancement of green consumption concepts can also help reduce the CE intensity. The advent of the post-pandemic era has caused damage to China’s domestic production and business activities, and coupled with the severe external global economic background, the concept of a new development pattern of dual circulation has emerged. The impact mechanism of DED and CEE from the perspective of HQD is shown in Figure 1.
From the perspective of direct impacts, the digital economy industry has obvious environmentally friendly characteristics, which make people’s work and production activities no longer limited to specialized time and space, and low-carbon lifestyles are gradually becoming a new fashion. The integration of digital economy and traditional industries can reduce production costs and develop economies of scale and scope, to a certain extent reduce waste of resource investment, and create a good economic development environment (Zhu et al., 2022). From the perspective of indirect impacts, digital economy can reduce CEE through industrial energy structure adjustment and optimization, energy utilization intensity reduction, technological progress, and other aspects. Specifically, the application and development of AI and Internet of Things technology have changed the traditional mode of industrial economic operation, and their design of resource allocation efficiency and restructuring of industrial organization has enhanced the EC structure. The reduction in the use of fossil fuels and non-renewable energy, as well as the development and utilization of clean energy and new energy industries, can effectively improve CEE and reduce the irreversible damage of energy to the environment. The technological innovation wave triggered by digital economy can effectively stimulate the transformation of EC structure (Xu et al., 2024a; Gazman, 2023). Given the CE spatial spillover effects (SSE), the acceleration of information technology circulation speed and the flow of production factors by digital economy can greatly achieve the balanced development of CEE at the spatial level.
This paper adopts the entropy weight approach to calculate indicator data. The entropy value is inversely proportional to the amount of information it contains. Moreover, the method is easy to carry out and can reduce data evaluation errors caused by human factors. According to the positive and negative attributes of the indicators, different index calculation methods are designed, as shown in Equation 1.
In Equation 1,
In Equation 2,
In Equation 3,
The selected digital economy indicator data are all from the National Statistical Yearbook and the statistical yearbooks of various local provinces. Parts of the industrial data are taken from the China Industrial Economic Statistical Yearbook. Missing data are filled in using interpolation (Kirikkaleli and Oyebanji, 2022).
This study designs a panel regression model to empirically model the relationship between selected DED data and CEE, as shown in Equation 4.
In Equation 4,
In the process of mechanism verification, an ME model is constructed to discuss the influence mechanism of digital economy on CEE. The model setting is shown in Equation 5.
In Equation 5,
In Equation 6,
Panel data refer to taking multiple cross-sections on a time series and simultaneously selecting sample observations on the cross-sections. It can reflect the heterogeneity of data in time and space to a certain extent, and it can better estimate dynamic behavior compared to simple time-series data. The stationarity of sequence variables is an important basis for affecting model performance, and directly conducting regression analysis on non-stationary variables may lead to spurious regression problems. The study hypothesizes that the cross-sectional sequence of panel data has different unit root processes. The study takes 30 provinces other than Xizang from 2000 to 2021 as the research object, and the data are from “China Statistical Yearbook,” “China Urban Statistical Yearbook,” and “China Financial Statistical Yearbook.” A few missing values are filled with multiple imputation. The outliers in the experimental data have been removed. To verify whether the selected variables have a high correlation, the study uses the coefficient of variance inflation for multicollinearity testing. The results are shown in Table 4.
The results in Table 4 indicate that the variance inflation factor values of each variable do not exceed 10 and the maximum VIF value among the variables does not exceed 3. This indicates that there is no multicollinearity among the control variables and a certain correlation exists between them and CEE. The ADF test is used to test the stationarity of sequence variables affecting CEs, and the results are shown in Table 5.
If there is a unit root process in the sequence, it will be non-stationary and lead to spurious regression in regression analysis. In Table 5, Ln-CEE is the explanatory variable, while Ln-DE, Ln-DI, Ln-ID, Ln-Pup, Ln-URB, Ln-GI, Ln-L-open, and Ln-FS are dependent variables. The results in the table indicate that the critical values of ADF tests for different variables are stable at 5% and 10%, indicating that the corresponding variable difference sequence is a stationary sequence. Subsequently, the regression model proposed in the study is subjected to the Breusch–Pagan LM test, and the dependence of cross-sectional data is analyzed. The results are shown in Table 6.
In Table 6, the LM test results indicate that the variable data do not reject the null hypothesis of structural mutation unit root process at the 5% significance level, indicating that there is no cross-sectional dependence in the data. At the same time, when conducting the Gregory–Hansen co-integration test on the ADF statistic, the results indicate that there is no structural mutation co-integration at the 1% significance level, and the statistic value is −8.7526, indicating that there is indeed a certain co-integration relationship between CE and influencing factors. This study first analyzes the digital economy composite index of 30 Chinese provinces from 2000 to 2021, as displayed in Figure 2.
The digital economy levels in various provinces have shown varying degrees of improvement after the epidemic, especially in areas such as Beijing, Tianjin, and Hebei (BTH) and the Pearl River Delta (PRD). The results indicate that the digital economy index ranges from 0.2 to 0.3 in Guangdong and Jiangsu, from 0.1 to 0.2 in Sichuan and Shandong, and from 0.3 to 0.4 in Henan. The digital economy improvement in Zhejiang Province is also quite significant. The digital economy level in the western region is still relatively low. Figure 3 shows the CEE results for each province.
In Figure 3, CEE shows a reduced tendency in the spatial dimension from east to west. Among them, the CE rate in western regions such as Xinjiang and Gansu has changed from the range of (0.25) in 2000 to the range of (25.50) in 2021. In terms of the time dimension, the CEE of most cities has improved, with significant growth observed in the eastern regions dominated by Jiangsu and Zhejiang and the southwestern regions dominated by Sichuan and Chongqing. The CE rates in Shanghai and Tianjin have exceeded 100, with a significant increase. The study conducts panel regression and robustness tests on the relationship between digital economy and CE efficiency and examines them from two dimensions of the digital economy. The results are shown in Table 7.
Table 7 shows a positive correlation between digital economy and CRE at 1%, with a regression value of 310.289 and the t-value of 9.876. There are also differences in regression analysis between the dimensions of DI, ID, and CRE, with DI significantly improving CRE compared to ID (259.783 > 210.659). On CV, the regression values of Pop, URB, and GI dimensions on the impact of CRE are negative. The URB and GI variables have significant negative effects at 5% and 10%, indicating that population growth and urbanization development rate will accelerate EC to a certain extent, and unreasonable macro interventions may affect the achievement of CRE. Other variables, such as higher FS, indicate a more significant positive effect of CRE, with regression values and t-values reaching 16.334 and 2.146, respectively. The effect of the L-open level on CRE does not exhibit any significant characteristics. The robustness result is the result of replacing the self-variable and control variable with the previous year’s profit growth rate as the dependent variable and then conducting regression analysis on the self-variable and control variable. The robustness test results indicate that the regression results between the digital economy and CE reduction efficiency are consistent with the variable regression results, indicating the feasibility of the results.
To ensure the accuracy of the Hausman test, lagged data on the DED level are utilized as a tool to perform endogeneity robust analysis on variables. Table 8 contains the details of further testing of the model.
In Table 8, the P of the regression results on KP-LM are all less than 0.005, indicating no identification error. The results of KP-WF show that the values above the 10% critical value are all greater than 15, showing no weak instrumental variable matter. The above results indicate that the selected model is effective. Table 9 presents the results of robustness testing on the digital economy variables.
The results in the second and third columns of Table 9 indicate that under the selected instrumental variables, digital economy still shows a positive effect with CRE at the 1% level. Under the least squares method, the regression results show that digital economy and CE have an impact at 5% efficiency. This study calculates the efficiency of CE measurement and replaces the independent and dependent variables with the digital economy innovation and entrepreneurship index based on the per capita real GDP, and the results are shown in the fifth and sixth columns. The replacement of the dependent and independent variables does not exhibit an obvious effect on the regression between digital economy and CEE, with regression values of 0.052 and 0.715 and a significant positive correlation at the 1% level. Differently, compared to the outcomes in the fifth column, the dependent variable, after being replaced, the regression value of Pop is positive, and the government’s macroeconomic regulation shows a negative correlation regression effect. This indicates that government macroeconomic regulation can create a certain economic environment for social innovation and entrepreneurship. The seventh column shows the regression results after excluding certain administrative data. The regression value between digital economy and carbon reduction is 197.321. The above results indicate that the regression results have good robustness.
Based on the rationality of the above benchmark content, this study conducts regression tests using AIS and rationalization as mediating variables, as shown in Table 10.
As shown in Table 10, digital economy and AIS have an obvious effect at the 1% level, and the CEE changes significantly. The impact coefficients of digital economy and CEE have decreased, but the significance of RSP on CEE is significantly smaller than that of AIS. The possible reason for this result may be that when AIS occupies a partial mediating position, the updating and upgrading of the EC industry requires a relatively slow process, so the CEE effect demonstrated by rational resource allocation is slower. Table 11 conducts an ME test from the perspective of energy.
Table 11 has a significant inactive relation between digital economy and ES, and a decrease in coal consumption can enhance CRE. The larger EI represents a higher degree of digital economy effect and a smaller carbon reduction impact, showing a more significant negative correlation. The adjustment of ES and EI can have a certain mediating effect, but the DED may lead to an increase in the energy input. Table 12 shows the mediating effects under TP.
The TP results indicate that the digital economy driving effect on technology is obvious. TP has a negative effect on CRE at the 5% level, which may be related to the conversion cycle of technological achievements. Overall, the significant positive effect of the DED on carbon reduction is still quite evident.
As shown in Table 13, this study roughly divides the sample data into three parts based on geographical space: eastern, central, and western, and conducts heterogeneity testing.
In Table 13, digital economy is significantly correlated with CEE in all three regions at the 1% level, with regression values of 336.012, 325.167, and 355.661, respectively. The most influential region is the eastern area, followed by the central range. The Pop variable and URB have a negative impact on CEE in the east, while they perform slightly worse in the west. The regression value between L-open and central CEE is negative at the 1% level (−1.688). Subsequently, the SSE of digital economy and CEE are analyzed, and the spatial dependency relationship of indicator data is analyzed by introducing a weight matrix approach. Figure 4 shows the MSP of CEE.
As shown in Figure 4, most provinces are located in high- or low-concentration areas, with a good spatial correlation. The Moran index has changed from a dispersed trend to a gathering trend toward the origin, indicating that the CEE between provinces is decreasing. It should be noted that the CEE in the Yangtze River Delta regions such as Jing and Hu is still relatively high. Subsequently, regression tests are conducted using the SDM, as shown in Table 14.
Table 14 indicates that the spatial lag coefficients of CEE under three matrices are −3.165, −3.164, and 3.029. This indicates that digital economy exhibits peripheral inhibition when enhancing CEE. Under the EDM, there is an active relationship between digital economy and CEE at the 1% level. From the perspective of CV, Pop and URB exhibit significant SSE. The spatial coefficient between government macroeconomic regulation and L-open is not significant. Regions with similar economic levels have a positive correlation between CEE and the economic level.
While promoting the process of globalization, digital economy also constructs the development format of global information technology and economic system structure. As an endogenous driving force for economy development, it effectively promotes the rationalization and ISU, reducing the hindrance of spatial distance to production efficiency. This study is based on the HQD perspective and analyzes the CE reduction and CFP impact mechanism under DE. The digital economy level in various Chinese provinces has improved to varying degrees after the epidemic, especially that in BTH, PRD, and other regions are more obvious. The coefficient of influence between digital economy and CEE has decreased, but the significance of RSP on CEE is significantly smaller than that of AIS. The reason may be that when AIS plays a partial mediating role, the upgrading of the EC industry requires a relatively slow process, so the CEE effect demonstrated by rational resource allocation is slower. The adjustment of ES and EI can have a certain mediating effect, but the DED may lead to an increase in the energy input. The regression results show that in various provinces of China, the digital economy is significantly positively correlated with CE reduction efficiency at the 1% level. This result is similar to the research findings by Zhong et al. (2022) and Wu et al. (2023). Zhong believes that the digital economy can effectively reduce agricultural carbon intensity by improving agricultural technology. The ME under technological regulation is consistent with the research results of energy technology regulation on CEE (Zhong et al., 2022). Wu believes that the integration of technology-intensive manufacturing can effectively leverage the multiplier effect of CE reduction. The research found that there is heterogeneity in the development of digital economy and CE in various provinces of China (Wu et al., 2023). The CEE of the digital economy in the three regions of East, Central, and West is significantly correlated at the 1% level, with regression values of 336.012, 325.167, and 355.661, respectively. The regression value between the degree of openness to the outside world and the CEE of the central region has a significant negative effect at the 1% level (−1.688). The SSE results show that the CEE between provinces is decreasing. When the digital economy improves CEE, there is a surrounding decrease, and the two have a significant positive impact relationship (at the 1% level) under the economic distance matrix. The CEE in eastern China has significantly increased, which is similar to the results of Shahbaz et al. (2022) on the relationship between digital economy and energy transition. There are differences in the energy structure among different regions, which are related to the conversion efficiency and energy emission efficiency of regional economic activities.
Compared to the research of Gao and Peng (2023) that focused on resource-based cities and the heterogeneity results of Yu et al. (2022) that emphasized spatial differences and SSE, this study has a richer content. In addition, this study suggests that the level of urbanization and macro government intervention may accelerate EC and affect CEE. In contrast, Adams et al. (2020) focused on the relationship between different factors (such as geopolitical risks and economic policy uncertainty) and EC and CEE. Tan et al. (2024) found that the rationalization of industrial structure is directly related to the development of digital economy and the decrease in the consumption level, but there is no specific comparison of the strength of the impact of industrial structure rationalization and industrial structure upgrading. This is different from the research that directly points out that the impact of industrial structure rationalization is smaller than that of industrial structure rationalization. The pilot program of low-carbon cities in China has promoted the digital transformation of manufacturing enterprises in pilot cities, and it has significant heterogeneity in enterprises, industries, and regions. It can promote digital transformation by strengthening science and technology fiscal expenditures and alleviating financing constraints (Zhao et al., 2023). Xu et al. (2024b) constructed a performance ranking technique based on ideal solution similarity to analyze the development status of new urbanization. They believed that the new urbanization policies significantly suppressed CE in pilot cities, and economic and population urbanization were important influencing factors, showing an inverted U-shaped relationship with CE. It is important to note that there is regional heterogeneity between these mechanisms. Therefore, when formulating carbon reduction strategies, it is essential to consider the specific local conditions. (Xu et al., 2024b).
However, it should be noted that in the results of this study, the CEE of the Yangtze River Delta region, including Beijing and Shanghai, is still relatively high. To fully tap into the potential of CE reduction, three countermeasures are proposed for research. First, it is imperative to accelerate the industrialization of the digital economy, promote the integration of green industries and traditional economic sectors, and facilitate carbon reduction through the implementation of sustainable development principles. Second, in response to regional development differences, it is necessary to actively adjust the industrial structure, accelerate industrial upgrading, regulate high CFP industries, and pay attention to the simultaneous realization and maintenance of economic and ecological benefits. Furthermore, the circulation and allocation of enterprise resources must be actively promoted. Third, it is essential to prioritize the optimization of energy structure, the enhancement of energy efficiency, and the completion of a green transformation through strategic investment in technological innovation and the transformation of achievements.
In the context of the dual carbon goals, the analysis of the spatiotemporal characteristics of the digital economy and CEE can clearly demonstrate the basic situation of China’s DED and CEE, providing some reference for relevant departments to formulate targeted goals and policies for the development of the digital economy and for emission reduction. The impact of the digital economy on CEE and its SSE can also provide some reference for some regions to improve CEE through the development of the digital economy. It should be noted that the research mainly discusses the impact and mechanism of the macro-level digital economy on CE. With the gradual improvement in the classification standards for the digital economy industry, it is necessary to objectively measure the impact of CE on different digital economy industries, construct a more comprehensive and perfect indicator system, and increase the richness of data acquisition. This is an important aspect that needs to be improved in future research.
From the perspective of HQD, digital economy needs to focus on environmental protection factors and emphasize the synergistic progress of economic and ecological benefits. The CFP level of industries in different regions is often correlated to their land-use structure. The adjustment of traditional ES can reduce the CFP of regional units, and the digital industry may cause an increase in the energy in the product service life cycle, an increase in the number of digital devices, and the use of information technology resources, causing a significant increase in the growth rate of CFP. The current energy source for electricity production is still traditional energy sources such as oil and coal. The demand for computing power urgently needs to handle the issue of EC. Emphasizing the digitalization and green synergy of digital economy is an important aspect of building the “dual carbon” goal. To fully tap into the potential of carbon reduction, this study proposes three strategies: to accelerate the DED industrialization, promote the integration of green industries and traditional economy, and use the LCSD concept to help reduce CE. The second is to actively adjust the industrial structure, accelerate industrial upgrading, regulate high-CFP industries in response to regional development differences, pay attention to the “simultaneous realization and maintenance” of economic and ecological benefits, and actively promote the circulation and allocation of enterprise resources. The third is to focus on the adjustment of ES, optimize energy efficiency, and complete the green transformation through technological innovation investment and achievement transformation. Most previous research studies have shown that the assessment of CFP requires much data support, and there are differences in the standards for selecting CE among different industries and regions. The relationship between CFP and carbon reduction is closely related, and it is difficult to accurately assess CFP solely through macro surveys of the digital economy. The research results and existing literature both agree that the digital economy has a positive effect on improving CEE. However, the study analyzes the specific impact of spatial differences, urbanization, government intervention, and industrial structure, which covers a wider and more specific range of factors and provides a reference for policy formulation and analysis of the impact on CEE. By analyzing the digital economy and CRE from multiple perspectives and considering the impact of various mediating variables on urban CFP, this study can effectively provide reference values for high-quality urban development.
The CEE of each province has a decreasing trend in spatial dimensions from the east to the west. In terms of the time dimension, the CEE of most cities has improved, with significant growth observed in the eastern regions dominated by Jiangsu and Zhejiang and the southwestern regions dominated by Sichuan and Chongqing. The regression results demonstrate a significant active correlation between digital economy and CRE at the 1% level, with a regression value of 310.289. The efficiency of DI in improving CER is significantly better than that of ID (259.783 > 210.659). The regression values of Pop, URB, and GI dimensions on the impact of CRE are negative, with URB and GI variables having significant negative effects at 5% and 10%, respectively. This indicates that population growth and the urbanization development rate will accelerate EC to a certain extent, and unreasonable macro interventions may affect the achievement of CRE. However, overall, there are spatial differences (eastern > central > western) and SSEs between the development of the digital economy and CE reduction efficiency, and the CEE among provinces is decreasing.
There are two shortcomings in this research. One is that it is based solely on static simulation analysis to explore the relationship between the development of the digital economy and CE without conducting any long-term dynamic analysis of the relationship between the two. The second is that the selection of indicator data is not comprehensive enough, including the failure to further refine and analyze the industrial digitalization indicators of the secondary industry. It is, thus, recommended that subsequent research studies consider the addition of dynamic modules to explore the impact of the digital economy on the development of total factor productivity. Furthermore, the effects of carbon tax policies, CE trading policies, and other factors on economic structure emissions reduction should be considered. Concurrently, a more comprehensive and detailed indicator system is being constructed to investigate the long-term impact trends of the digital economy and CE.
The original contributions presented in the study are included in the article/supplementary material; further inquiries can be directed to the corresponding author.
YL: investigation, supervision, and writing–review and editing. BC: investigation and writing–original draft. LG: investigation and writing–original draft. JK: investigation and writing–original draft.
The author(s) declare that financial support was received for the research, authorship, and/or publication of this article. This research was supported by the Science and Technology Bureau of Yulin City (1504-CXY2022110) and the Education Department of Shaanxi Province (23JK0751).
The authors declare that the research was conducted in the absence of any commercial or financial relationships that could be construed as a potential conflict of interest.
All claims expressed in this article are solely those of the authors and do not necessarily represent those of their affiliated organizations, or those of the publisher, the editors and the reviewers. Any product that may be evaluated in this article, or claim that may be made by its manufacturer, is not guaranteed or endorsed by the publisher.
Adams, S., Adedoyin, F., Olaniran, E., and Bekun, F. (2020). Energy consumption, economic policy uncertainty and carbon emissions; causality evidence from resource rich economies. Econ. Anal. Policy 68, 179–190. doi:10.1016/j.eap.2020.09.012
Addai, K., Serener, B., and Kirikkaleli, D. (2022). Empirical analysis of the relationship among urbanization, economic growth and ecological footprint: evidence from Eastern Europe. Environ. Sci. Pollut. R. 29 (19), 27749–27760. doi:10.1007/s11356-021-17311-x
Chen, Y., Ji, X., and Zhao, G. (2024). Does digital infrastructure construction impact urban carbon emission reduction? Evidence from China's smart city construction. Environ. Sci. Pollut. R. 31 (27), 39481–39496. doi:10.1007/s11356-024-33799-5
Cui, H., Cao, Y., Feng, C., and Zhang, C. (2023). Multiple effects of ICT investment on carbon emissions: evidence from China. Environ. Sci. Pollut. R. 30 (2), 4399–4422. doi:10.1007/s11356-022-22160-3
Dabbous, A., and Tarhini, A. (2021). Does sharing economy promote sustainable economic development and energy efficiency? Evidence from OECD countries. J. Innov. Knowl. 6 (1), 58–68. doi:10.1016/j.jik.2020.11.001
Gao, W., and Peng, Y. (2023). Energy saving and emission reduction effects of urban digital economy: technology dividends or structural dividends? Environ. Sci. Pollut. R. 30 (13), 36851–36871. doi:10.1007/s11356-022-24780-1
Gazman, V. D. (2023). A new criterion for the ESG model. Green Low-Carbon Econ. 1 (1), 22–27. doi:10.47852/bonviewGLCE3202511
Han, D., Ding, Y., Shi, Z., Shi, Z., and He, Y. (2022). The impact of digital economy on total factor carbon productivity: the threshold effect of technology accumulation. Environ. Sci. Pollut. R. 29 (37), 55691–55706. doi:10.1007/s11356-022-19721-x
Hertwich, E. G. (2021). Increased carbon footprint of materials production driven by rise in investments. Nat. Geosci. 14 (3), 151–155. doi:10.1038/s41561-021-00690-8
Hussain, M., Mir, G. M., Usman, M., Ye, C., and Mansoor, S. (2022). Analysing the role of environment-related technologies and carbon emissions in emerging economies: a step towards sustainable development. Environ. Technol. 43 (3), 367–375. doi:10.1080/09593330.2020.1788171
Jiang, S., Chishti, M. Z., Rjoub, H., and Syed, R. (2022). Environmental R&D and trade-adjusted carbon emissions: evaluating the role of international trade. Environ. Sci. Pollut. R. 29 (42), 63155–63170. doi:10.1007/s11356-022-20003-9
Khan, A. J., Hameed, W. U., Ahmed, T., Uqbal, J., Aplin, M. J., and Leahy, S. (2024). Green behaviors and innovations: a green hrm perspective to move from traditional to sustainable environmental performance. Employ. Responsib. Rights J. 36 (2), 231–248. doi:10.1007/s10672-023-09443-8
Kirikkaleli, D., and Oyebanji, M. O. (2022). Consumption-based carbon emissions, trade, and globalization: an empirical study of Bolivia. Environ. Sci. Pollut. R. 29 (20), 29927–29937. doi:10.1007/s11356-022-18495-6
Li, J., Chen, L., Chen, Y., and He, J. (2022). Digital economy, technological innovation, and green economic efficiency—empirical evidence from 277 cities in China. Manag. Decis. Econ. 43 (3), 616–629. doi:10.1002/mde.3406
Li, T., Yu, L., Ma, Y., Duan, T., Huang, W., Zhou, Y., et al. (2023). Carbon emissions of 5G mobile networks in China. Nat. Sustain. 6 (12), 1620–1631. doi:10.1038/s41893-023-01206-5
Luo, S., Yimamu, N., Li, Y., Wu, H., Irfan, M., and Hao, Y. (2023). Digitalization and sustainable development: how could digital economy development improve green innovation in China? Bus. Strateg. Environ. 32 (4), 1847–1871. doi:10.1002/bse.3223
Mi, Z., Zheng, J., Meng, J., Ou, J., Hubacek, K., Liu, Z., et al. (2020). Economic development and converging household carbon footprints in China. Nat. Sustain. 3 (7), 529–537. doi:10.1038/s41893-020-0504-y
Müller, L. J., Kätelhön, A., Bringezu, S., McCoy, S., Suh, S., Edwards, R., et al. (2020). The carbon footprint of the carbon feedstock CO2. Energ. Environ. Sci. 13 (9), 2979–2992. doi:10.1039/D0EE01530J
Patterson, D., Gonzalez, J., Hölzle, U., Le, Q., Liang, C., Munguia, L. M., et al. (2022). The carbon footprint of machine learning training will plateau, then shrink. Computer. 55 (7), 18–28. doi:10.1109/MC.2022.3148714
Ren, S., Li, L., Han, Y., Hao, Y., and Wu, H. (2022). The emerging driving force of inclusive green growth: does digital economy agglomeration work? Bus. Strateg. Environ. 31 (4), 1656–1678. doi:10.1002/bse.2975
Saeed, A., Ali, B. A., Javed, M., Atiqur, R., Swapan, T., and Talukdar, S. (2023). Mapping and evaluating sustainable and unsustainable urban areas for ecological management towards achieving low-carbon city: an empirical study of Asir Region, Saudi Arabia. Environ. Sci. Pollut. R. 30, 65916–65932. doi:10.1007/s11356-023-27129-4
Shahbaz, M., Wang, J., Dong, K., and Zhao, J. (2022). The impact of digital economy on energy transition across the globe: the mediating role of government governance. Renew. Sust. Energ. Rev. 166, 112620. article 112620. doi:10.1016/j.rser.2022.112620
Shang, M., and Luo, J. (2021). The tapio decoupling principle and key strategies for changing factors of Chinese urban carbon footprint based on cloud computing. Int. J. Env. Res. Pub. He. 18 (4), 2101. article 2101. doi:10.3390/ijerph18042101
Sharma, M., Kumar, A., Luthra, S., Joshi, S., and Upadhyay, A. (2022). The impact of environmental dynamism on low-carbon practices and digital supply chain networks to enhance sustainable performance: an empirical analysis. Bus. Strateg. Environ. 31 (4), 1776–1788. doi:10.1002/bse.2983
Tan, L., Yang, Z., Irfan, M., Ding, C. J., Hu, M., and Hu, J. (2024). Toward low-carbon sustainable development: exploring the impact of digital economy development and industrial restructuring. Bus. Strateg. Environ. 33 (3), 2159–2172. doi:10.1002/bse.3584
Valls-Val, K., and Bovea, M. D. (2021). Carbon footprint in Higher Education Institutions: a literature review and prospects for future research. Clean. Technol. Envir. 23 (9), 2523–2542. doi:10.1007/s10098-021-02180-2
Wang, L., Chen, L., and Li, Y. (2022). Digital economy and urban low-carbon sustainable development: the role of innovation factor mobility in China. Environ. Sci. Pollut. R. 29 (32), 48539–48557. doi:10.1007/s11356-022-19182-2
Wang, X., and Zhong, M. (2023). Can digital economy reduce carbon emission intensity? Empirical evidence from China’s smart city pilot policies. Environ. Sci. Pollut. R. 30 (18), 51749–51769. doi:10.1007/s11356-023-26038-w
Wang, Z., Teng, Y. P., Wu, S., and Chen, H. (2023). Does green finance expand China’s green development space? Evidence from the ecological environment improvement perspective. Systems 11 (7), 369. doi:10.3390/systems11070369
Wood, R., Neuhoff, K., Moran, D., Simas, M., Grubb, M., and Stadler, K. (2020). The structure, drivers and policy implications of the European carbon footprint. Clim. Policy. 20 (Suppl. 1), S39–S57. doi:10.1080/14693062.2019.1639489
Wu, T., Peng, Z., Yi, Y., and Chen, J. (2023). The synergistic effect of digital economy and manufacturing structure upgrading on carbon emissions reduction: evidence from China. Environ. Sci. Pollut. R. 30 (37), 87981–87997. doi:10.1007/s11356-023-28484-y
Xu, A., Song, M., Wu, Y., Luo, Y., Zhu, Y., and Qiu, K. (2024a). Effects of new urbanization on China's carbon emissions: a quasi-natural experiment based on the improved PSM-DID model. Technol. Forecast Soc. 200, 123164. doi:10.1016/j.techfore.2023.123164
Xu, C., Zhao, W., Li, X., Cheng, B., and Zhang, M. (2024b). Quality of life and carbon emissions reduction: does digital economy play an influential role? Clim. Policy. 24 (3), 346–361. doi:10.1080/14693062.2023.2197862
Xuan, D., Ma, X., and Shang, Y. (2020). Can China’s policy of carbon emission trading promote carbon emission reduction? J. Clean. Prod. 270, 122383. doi:10.1016/j.jclepro.2020.122383
Yu, H., and Zhu, Q. (2023). Impact and mechanism of digital economy on China’s carbon emissions: from the perspective of spatial heterogeneity. Environ. Sci. Pollut. R. 30 (4), 9642–9657. doi:10.1007/s11356-022-22552-5
Yu, Z., Liu, S., and Zhu, Z. (2022). Has the digital economy reduced carbon emissions? analysis based on panel data of 278 cities in China. Int. J. Env. Res. Pub. He. 19 (18), 11814. doi:10.3390/ijerph191811814
Zhao, S., Zhang, L., An, H., Peng, L., Zhou, H., and Hu, F. (2023). Has China's low-carbon strategy pushed forward the digital transformation of manufacturing enterprises? Evidence from the low-carbon city pilot policy. Environ. Impact Asses. 102, 107184. doi:10.1016/j.eiar.2023.107184
Zhong, R., He, Q., and Qi, Y. (2022). Digital economy, agricultural technological progress, and agricultural carbon intensity: evidence from China. Int. J. Env. Res. Pub. He. 19 (11), 6488. doi:10.3390/ijerph19116488
Keywords: digital economic system, carbon emissions, panel model, spatial spillover effects, industrial structure, carbon footprint
Citation: Li Y, Chen B, Guo L and Kang J (2025) Analysis of the impact of the digital economy system on carbon emissions and carbon footprint from the perspective of high-quality development. Front. Environ. Sci. 13:1443405. doi: 10.3389/fenvs.2025.1443405
Received: 04 June 2024; Accepted: 16 January 2025;
Published: 21 February 2025.
Edited by:
Mobeen Ur Rehman, Keele University, United KingdomReviewed by:
Mohamed R. Abonazel, Cairo University, EgyptCopyright © 2025 Li, Chen, Guo and Kang. This is an open-access article distributed under the terms of the Creative Commons Attribution License (CC BY). The use, distribution or reproduction in other forums is permitted, provided the original author(s) and the copyright owner(s) are credited and that the original publication in this journal is cited, in accordance with accepted academic practice. No use, distribution or reproduction is permitted which does not comply with these terms.
*Correspondence: Youhui Li, bGl5b3VodWkxOTkwMTAxOEAxNjMuY29t
Disclaimer: All claims expressed in this article are solely those of the authors and do not necessarily represent those of their affiliated organizations, or those of the publisher, the editors and the reviewers. Any product that may be evaluated in this article or claim that may be made by its manufacturer is not guaranteed or endorsed by the publisher.
Research integrity at Frontiers
Learn more about the work of our research integrity team to safeguard the quality of each article we publish.