- 1Department of Ecology and Genetics, Uppsala University, Uppsala, Sweden
- 2Department of Ecosystem Science and Sustainability, Colorado State University, Fort Collins, CO, United States
- 3Department of Zoology and Physiology, University of Wyoming, Laramie, WY, United States
- 4Department of Biological Sciences, Virginia Tech, Blacksburg, VA, United States
- 5Louisiana Universities Marine Consortium, Chauvin, LA, United States
- 6Department of Plankton and Microbial Ecology, Leibniz Institute for Freshwater Ecology and Inland Fisheries, Stechlin, Germany
- 7Institute of Biochemistry and Biology, Potsdam University, Potsdam, Germany
- 8Department of Ecoscience, Aarhus University, Aarhus, Denmark
- 9Department of Water and Climate, Vrije Universiteit Brussel, Brussels, Belgium
- 10Department of Community and Ecosystem Ecology, Leibniz Institute of Freshwater Ecology and Inland Fisheries, Berlin, Germany
- 11Department of Lake Research, Helmholtz Center for Environmental Research, Magdeburg, Germany
- 12Environmental Sciences Division, Oak Ridge National Laboratory, Oak Ridge, TN, United States
- 13Biodiversity and Natural Resources Program, International Institute for Applied Systems Analysis, Laxenburg, Austria
- 14Department of Physical Geography, Utrecht University, Utrecht, Netherlands
- 157 Lakes Alliance, Belgrade Lakes, ME, United States
Free use and redistribution of data (i.e., Open Data) increases the reproducibility, transparency, and pace of aquatic sciences research. However, barriers to both data users and data providers may limit the adoption of Open Data practices. Here, we describe common Open Data challenges faced by data users and data providers within the aquatic sciences community (i.e., oceanography, limnology, hydrology, and others). These challenges were synthesized from literature, authors’ experiences, and a broad survey of 174 data users and data providers across academia, government agencies, industry, and other sectors. Through this work, we identified seven main challenges: 1) metadata shortcomings, 2) variable data quality and reusability, 3) open data inaccessibility, 4) lack of standardization, 5) authorship and acknowledgement issues 6) lack of funding, and 7) unequal barriers around the globe. Our key recommendation is to improve resources to advance Open Data practices. This includes dedicated funds for capacity building, hiring and maintaining of skilled personnel, and robust digital infrastructures for preparation, storage, and long-term maintenance of Open Data. Further, to incentivize data sharing we reinforce the need for standardized best practices to handle data acknowledgement and citations for both data users and data providers. We also highlight and discuss regional disparities in resources and research practices within a global perspective.
1 Introduction
Open Science practices are gaining ground in many scientific disciplines, thereby increasing the transparency, reproducibility, and accessibility of scientific research (Ramachandran et al., 2021; Tedersoo et al., 2021). In particular, Open Data - the free use and redistribution of data - has been a focus of the Open Science movement, resulting in broader data availability, standardized data repositories, data management plans, and data publication requirements from both funders and journals (Michener, 2015; Wilkinson et al., 2016; Clark et al., 2021). To increase the utility and equity of Open Data, the FAIR (Wilkinson et al., 2016) and CARE (Carroll et al., 2020) principles provide frameworks for making data Findable, Accessible, Interoperable, and Reusable (FAIR), while being conscious of power structures and respectful of Indigenous data sovereignty (CARE). Still, while substantial progress has been made in the movement towards Open Data, challenges for both data users and data providers may limit our ability to leverage the full potential of Open Data.
Aquatic sciences deal with specific Open Data challenges. For instance, the disciplines that make up this field, such as oceanography, limnology, ecohydrology, and catchment hydrology, are intricately connected to each other through the water cycle. However, they focus on different spatial and temporal scales and often use different tools, leading to differing data standards and decreased interoperability among the various scientific disciplines. Aquatic sciences deal with diverse data types (e.g., genetic data, species abundances, high-frequency sensor data), data structures, and repositories in which data are stored (Reichman et al., 2011; Jennings et al., 2017), which hinders standardization. Furthermore, interaction with surrounding (non-aquatic) environments (e.g., watersheds, atmosphere) is inherent to this field and complicates data sharing standards, requiring further harmonization of datasets and data management practices.
In this perspective paper, we summarize current challenges in Open Data within the aquatic science community, through literature study, the authors’ own experiences, and the survey responses of 174 aquatic science researchers in academia, government agencies, industry, and other sectors. Throughout this work, we focused on two primary groups, which often encompass the same individual researchers: data providers (who provide data for a specific end) and data users (who use data as an input for further analysis). We propose ways to address these current challenges, and note that implementing changes will take time, cooperation, and flexibility. However, we believe that continuing to advance Open Data practices in the aquatic sciences will foster transparency, expand inclusivity, and lead to impactful and interdisciplinary research.
2 Methods
To supplement the perspectives of the authors and existing published literature, we conducted a survey of data users and providers across various sectors in the aquatic sciences. Survey questions aimed to identify respondents’ challenges when dealing with Open Data, as well as the motivations and hurdles they face when implementing best practices in Open Data.
The survey was disseminated online via mailing lists, website posts, and newsletters to several widely known aquatic sciences communities (e.g., GLEON and CASS; for a full list of acronyms, see Supplementary Text S1). To reach a wider audience, it was additionally advertised on social media. The survey was conducted under approval from the Virginia Tech Institutional Review Board (IRB #23–611) and survey participants received a statement of informed consent that explained their rights as research subjects. We collected responses between 21 September and 17 October, 2023 and received 174 responses from the survey. The contacted networks, questions, and main outcomes of the survey are listed in the (Supplementary Text S2).
3 Challenges of open data
Challenges to the adoption of Open Data practices span multiple scales, with common themes identified between data users and data providers (Figure 1). Below (sections 3.1–3.7), we describe seven primary challenges that emerged across our personal experiences, in published literature, and in survey responses from aquatic science practitioners. These challenges are ordered by whether they can be mitigated on the level of individual publications (i.e., during a review process), require a consensus of the larger research community, or need to be addressed by a change in overall research policy (Figure 1). Problems and solutions identified in each challenge cannot be entirely separated, as higher-level challenges (e.g., on research policy) can influence and help address lower-level issues (e.g., on publication).
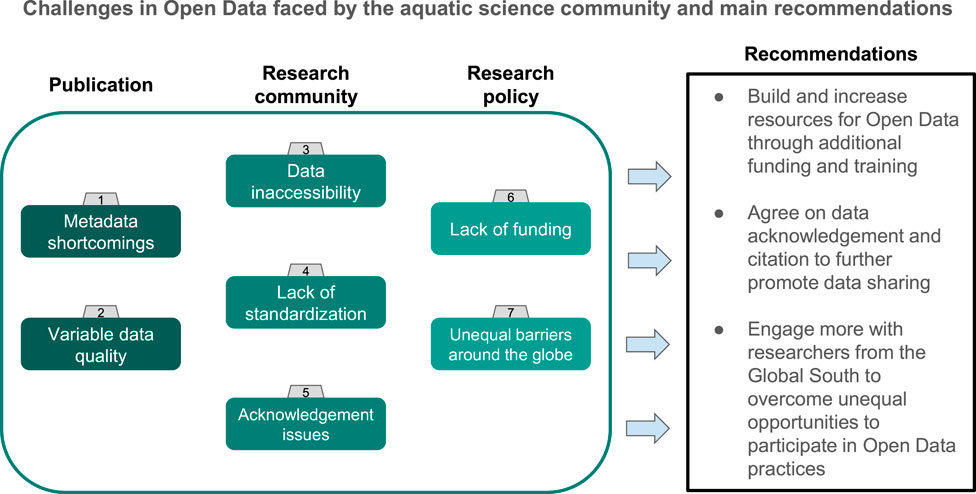
Figure 1. Main challenges faced by the aquatic science community, placed in a range from publication to research policy and recommendations to promote open and inclusive science. Each challenge is described in section 3, and the numbers in the figure refer to the subsections.
3.1 Metadata shortcomings: Incompleteness and low interoperability
Both data users and providers cited issues with metadata as a hurdle to Open Data (Figure 2). Users might find data difficult to understand due to lack of standardized documentation and formatting, challenging data integration and analysis, and incomplete or unclear metadata (Reichman et al., 2011). Indeed, inadequately captured information in metadata is a significant barrier for data retrieval (Löffler et al., 2021). Moreover, considerable efforts are required to compile and analyze data, because they are spread over heterogeneous repositories with varying metadata standards (e.g., Vlah et al., 2023), and use different terminology and scales (Reichman et al., 2011).
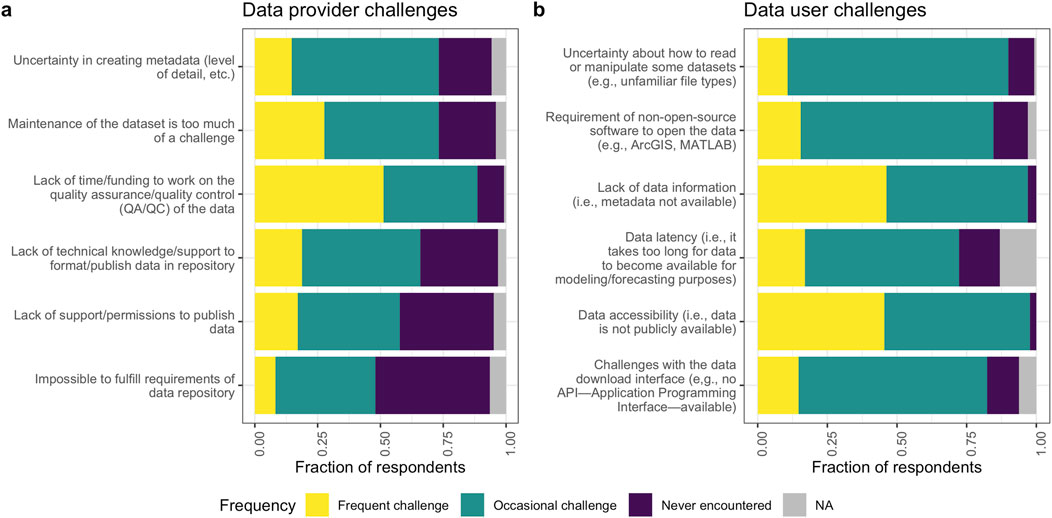
Figure 2. Challenges faced by (A) data providers and (B) data users, as represented in survey responses from aquatic science researchers (n = 174 respondents).
Generating complete metadata can be challenging for researchers, especially without an internationally-accepted standard in the aquatic sciences, though there are initiatives to create metadata standards, e.g., the Ecological Metadata Language (Jones et al., 2019), the European AquaINFRA project (Otsu et al., 2024), or NFDI in Germany (Koepler et al., 2021). Survey respondents expressed that a lack of training and awareness of available resources contributed to poor metadata quality. Multiple respondents suggested that templates for standardized metadata, workflows, and instructions for uncommon file types would improve metadata and Open Data practices. Metadata standards for environmental sciences exist, such as the Content Standard for Digital Geospatial Metadata (CSDGM), and NetCDF Climate and Forecast (CF) metadata conventions (Mayernik, 2016). However, each metadata standard has its own structure and vocabulary, which may hinder broad adoption of these metadata schemes. Researchers have worked to develop software applications (e.g., Morpho by NCEAS; dmdScheme; Krug and Petchey, 2021) to help scientists develop complete metadata, but the scale of knowledge and use of such resources remain unclear. As requirements for Open Data from journals and funding bodies are becoming the norm, it may be necessary for professional societies or other organizations to adopt a metadata standard and subsequently provide resources for researchers to use that standard.
Other studies have found similar perceptions of the need for more training in metadata curation. Emery et al. (2021) surveyed biological and environmental science instructors about the presence of data science skills in undergraduate education and reported insufficient background in data skills of instructors and students, and a lack of space in the curriculum. Similarly, a survey distributed to scientific researchers across numerous disciplines found that only 27.6% of respondents reported that they received assistance in metadata creation, and only 25.5% of respondents from academic institutions were satisfied with available metadata tools (Tenopir et al., 2020). There is apparently a lack of training in data management, including metadata creation, across all career stages. This could be remedied by increased training via graduate courses or professional society workshops as well as the employment of data scientists to support research projects.
3.2 Variable data quality and reusability
Open Data requirements are increasing without a concomitant increase in time and resources. This leads to concerns about the quality of openly published data; while data repositories usually require strict formats, the submitted data often does not undergo a thorough peer-review process (Peer et al., 2014), especially if data submission occurs separate from article submission. Therefore, it is possible that funder and journal data requirements are met with a lower quality of data, or data that is difficult to use. Unclear descriptions, metadata, formatting, or usage instructions can inhibit the use of data, even if publicly available (see sections 3.1 and 3.3). Good data management is inherently valuable to data providers for data re-usage and should be considered an integral part of data collection. More knowledge and discussion on ensuring data quality is important, and it is an argument for increased funding.
3.3 Open data inaccessibility
Openly-available data do not always translate to immediate access and utility. Unequal access to repositories (e.g., due to restrictive user agreements or membership requirements) or information about their existence, further complicate access to data even when published. These barriers to data availability are especially problematic when combining multiple data sources. One survey respondent noted that “wrangling data from multiple public sources is often the biggest challenge”. Our survey results and previously published literature (Savage and Vickers, 2009; Tedersoo et al., 2021) highlight that data users regularly need to contact authors or data providers to use data, with varying success rates. Extra time to contact authors to request data followed by delays in replies can slow down or even discourage re-use of data sources.
Technical barriers, such as requirements for specific software, use of advanced file types, or lack of step-by-step guidelines, can reduce the usability of the Open Data even if accessible. Both data providers and users indicated that a lack of technical know-how was an occasional challenge when creating or using datasets (Figure 2). Some of these issues could be mediated by using open-source software and providing clear usage instructions, though more education in data handling may also be required.
3.4 Lack of standardization
Aquatic sciences deal with diverse environments (e.g., oceans and groundwater), data sources (e.g., laboratory and field data), scales (microscales to global circulations), and data types (e.g., genetic and chemical). Therefore, standardization can only be achieved to a moderate degree. Even when assessing similar data types, published data are often provided in widely variable formats and across a broad array of repositories. Should the aquatic sciences move toward harmonization and standardization of published data? Some respondents noted that harmonization among repositories may ease data publishing and re-use. For example, one respondent remarked that harmonized data submission systems would be helpful so that providers “do not need to study or read the instructions for each repository.” Given the challenges of utilizing data from multiple sources, several respondents argued in favor of consolidating data into larger, all-encompassing databases.
Despite the aforementioned diversity in data types, integration of aquatic ecosystems with surrounding environments and of different types of measurements within water bodies is fundamental within the aquatic sciences. As such, initiatives to standardize data or at least promote interoperability are ongoing within or involving the aquatic sciences. Some non-exhaustive examples are EOSC (https://open-science-cloud.ec.europa.eu/), ILTER (Mirtl et al., 2018), ISIMIP (e.g., Hempel et al., 2013), Macrosheds (Vlah et al., 2023), NFDI (https://www.nfdi.de/), and SBDI (https://biodiversitydata.se/).
While harmonizing and consolidating datasets could potentially make Open Data practices easier for data users and providers, these “mega-datasets” have the potential to exacerbate other challenges of Open Data. Other respondents raised concerns that easing data reuse decreases the frequency with which data users interact with data providers, which may increase misinterpretation of data. One survey respondent argued strongly that centralization efforts would not lead to collaborative research without users and providers first agreeing on the purpose of centralization. It is up for debate whether easing access to data would inherently encourage collaboration, exchange, and acknowledgement between data users and providers.
3.5 Authorship and acknowledgment issues
Despite both data users and providers acknowledging the value of Open Data, there is some disagreement on how the two groups should interact after data publication. Data providers pointed out that a lot of effort goes into creating high quality datasets, and some are consequently reluctant to share data openly. Instead, those survey respondents preferred direct contact with data users and occasionally expected inclusion in author lists. The main reasons cited for this stance were the use of data without proper citation or acknowledgement, improper use of data, and a need to be involved in publications for continued employment or career advancement. Indeed, data providers are regularly not or incorrectly cited (Kratz and Strasser, 2015), and survey respondents suggested standardized formats or guidance on how to use and cite shared data correctly.
It is sometimes unclear how to acknowledge contributors and publishers of data, which can lead to a lack of trust and engagement in the future. For example, one respondent noted that “the more accessible the data is, the less likely data requesters are to collaborate”. There is no standard on how to collaborate with data providers when using Open Data. Respondents commented “it should be standard practice to include data providers in the early stages of a study”, and “data ownership needs to be recognized”. On the other hand, some responses strongly advocated for sharing data “without any expectation in return”, arguing that data created from public resources should be available without limitations.
Guidance on how to acknowledge Open Data is often provided by the data providers themselves, suggesting a certain phrasing in the acknowledgement section or a publication/dataset to cite. It is also becoming common practice to attach persistent identifiers (e.g., Digital Object Identifiers, DOIs) to datasets and to include a license (e.g., Creative Commons). The DOIs guarantee a stable link to the data and ease citation (CODATA-ICSTI Task Group on Data Citation Standards and Practices, 2013; Damerow et al., 2021), while licenses formalize how the data can be reused and should be acknowledged. For groups that may not have explicitly written or standardized data usage protocols, such as indigenous communities or citizen scientists, additional care should be taken to ensure appropriate data quality, acknowledgement, and conduct (Bowser et al., 2020; Jennings et al., 2023).
If Open Data is to be the future of aquatic sciences, a consensus on acknowledgement must be reached. Such consensus should ideally cover all aspects of Open Science, including not only Open Data, but also open-source software. Working towards a common understanding and expectation for data sharing, use, and acknowledgment between data providers and users is vital to further support Open Data practices. Furthermore, proper acknowledgement of Open Data is instrumental in showing the benefit of monitoring programs, and thereby securing their funding.
3.6 Lack of funding
Collecting Open Data and assuring and maintaining their quality requires time, expertise, and funding. While data providers in the survey acknowledged the importance of making their data freely available and their willingness to do so, they also identified the lack of support from funders as a frequent challenge (50% of respondents; Figure 2A). Without financial or technical support, creating and maintaining Open Data products becomes overly burdensome and time-consuming for data providers. Proposed solutions from respondents included additional funds, compensation for the time it takes to prepare data for sharing, and hiring data scientists for support. Many other issues encountered during data creation (e.g., improving user-friendliness of data sharing interfaces, data maintenance) could also be partially resolved through additional funding. Lack of training in data curation, however, is a more systematic problem with the potential to be addressed through undergraduate education (Emery et al., 2021).
3.7 Unequal barriers around the globe
The majority of publications originate from the Global North (e.g., Dangles et al., 2022; Potter and Pearson, 2023) and it is therefore easy to overlook data issues in other parts of the world. While our survey indeed reached fewer participants outside North America and Europe (16%), it is important to acknowledge that certain issues scale with financial restrictions and a lower representation in scientific publishing. In the past decades, a barrier towards Open Science has been building in developing countries due to what is termed “parachute science”, where researchers from high-income countries collect and use data from low-income countries and publish their findings without engaging or acknowledging local researchers (Stefanoudis et al., 2021). While there are great benefits to making data openly available, Open Data practices may unintentionally reinforce this harmful conduct of data use without local engagement.
A lower availability of resources for research in developing countries is reflected in less data being collected and reduced publishing opportunities. Moreover, the scarcer funding opportunities are even less likely to cover long-term data maintenance, which is a common occurrence worldwide (Lindenmayer, 2018), and further compound Open Data issues in low income regions. Restricted resources underscore the need for universities and institutes to be acknowledged for data collection and sharing, so that the benefit of the investment is shown (see section 3.5). While calls for more data availability in the Global South are frequent (e.g., Chambers et al., 2017; Loch and Riechers, 2021; Kirschke et al., 2023) - for good scientific reasons - it may be that the current conditions of Open Data practices, such as challenges explained in the above sections, are actively withholding development in this direction. This reinforces the need to regulate data acknowledgement for both data users and providers, and it underlines the necessity of including data providers from low income countries in discussions about how to resolve this issue.
4 Final remarks
Open Data is an increasingly important topic in scientific communities and education (Ramachandran et al., 2021). Many researchers are convinced this is the way forward, where data collected by researchers becomes available for all (e.g., Powers and Hampton, 2019). Open Data has been shown to result in novel and timely studies in aquatic science studies (e.g., Hanson et al., 2016; Rose et al., 2016) and makes research more inclusive and transparent (Soranno et al., 2015; Hampton et al., 2015). However, the right conditions and possibilities for open publishing need to be fostered at a global scale.
Recommendations in the practice of Open Data within the aquatic sciences have been outlined here (Figure 1):
- Improve data usability (data accessibility and standardization, and metadata quality) to transform data products from a mere collection of numbers to FAIR data.
- Ensure data providers–particularly those working outside academia–are rewarded in a way that maintains open access to data, benefits both data users and providers, and is agreed upon by all parties.
- Increase funding to facilitate better Open Data practices, increase quality and reusability of Open Data, and avoid loss of data sources.
- Get a truly global representation of these issues and include data users and providers from low- and middle-income countries in discussions on how to move forward.
While impressive progress toward Open Data and Open Science has been made within the aquatic sciences in the past years, data users and providers still face challenges. Continued discussion and demonstration of the benefits of openly sharing data is paramount to ensure further improvement in the years to come.
Data availability statement
The original contributions presented in the study are included in the article/Supplementary Material, further inquiries can be directed to the corresponding author.
Ethics statement
The studies involving humans were approved by Virginia Tech Institutional Review Board (IRB #23-611). The studies were conducted in accordance with the local legislation and institutional requirements. The participants provided their written informed consent to participate in this study.
Author contributions
JM: Conceptualization, Methodology, Project administration, Writing–original draft, Writing–review and editing. CB: Conceptualization, Methodology, Project administration, Writing–original draft, Writing–review and editing. AL: Conceptualization, Methodology, Writing–original draft, Writing–review and editing. FO: Conceptualization, Methodology, Writing–original draft, Writing–review and editing. SC-G: Conceptualization, Methodology, Writing–original draft, Writing–review and editing. H-PG: Conceptualization, Methodology, Writing–original draft, Writing–review and editing. RL: Conceptualization, Methodology, Writing–original draft, Writing–review and editing. RF: Conceptualization, Methodology, Writing–original draft, Writing–review and editing. KM: Conceptualization, Methodology, Writing–original draft, Writing–review and editing. LN: Conceptualization, Methodology, Writing–original draft, Writing–review and editing. RP: Conceptualization, Methodology, Writing–original draft, Writing–review and editing. KS: Conceptualization, Methodology, Writing–original draft, Writing–review and editing. DW: Conceptualization, Methodology, Writing–original draft, Writing–review and editing.
Funding
The author(s) declare that financial support was received for the research, authorship, and/or publication of this article. J. P. M. was funded by the European Union’s Horizon 2020 research and innovation programme, grant agreement number 101017861 (SMARTLAGOON). C. C. B was supported by the NSF award OIA-2019528. S. C. G. was funded by BOEM cooperative agreement M19AAC00015. K. S and L. G. T. N were funded from European Union’s Horizon 2021 research and innovation programme under the Marie Sklodowska-Curie grant agreement No.956623, MSCA-ITN-ETN-European Training Network (inventWater Project), and H.-P. G from the European Union’s Horizon 2020 research and innovation program under the Marie Skłodowska-Curie grant agreement No 722518 (MANTEL project). A. S. L. L. acknowledges support for her Ph.D. from the U.S. National Science Foundation (NSF; DGE-1840995 and DEB-1753639), the Institute for Critical Technology and Applied Science (ICTAS), and the College of Science Roundtable at Virginia Tech. R. M. P. was supported by the U.S. Department of Energy (DOE), Office of Energy Efficiency and Renewable Energy, Water Power Technologies Office, and Environmental Sciences Division at Oak Ridge National Laboratory (ORNL). ORNL is managed by UT-Battelle, LLC, for the U.S. DOE under contract DE-AC05-00OR22725. R. S. L. F. was funded by VUB Research. This work was conceived at the 2023 virtual meeting of the Global Lake Ecological Observatory Network (GLEON).
Acknowledgments
We would like to thank Ashley Trudeau and César Ordóñez for their contributions in earlier stages of this project. We additionally express our gratitude to everyone who filled out the survey. This work was conceived at the 2023 virtual meeting of the Global Lake Ecological Observatory Network (GLEON).
Conflict of interest
The authors declare that the research was conducted in the absence of any commercial or financial relationships that could be construed as a potential conflict of interest.
Publisher’s note
All claims expressed in this article are solely those of the authors and do not necessarily represent those of their affiliated organizations, or those of the publisher, the editors and the reviewers. Any product that may be evaluated in this article, or claim that may be made by its manufacturer, is not guaranteed or endorsed by the publisher.
Supplementary material
The Supplementary Material for this article can be found online at: https://www.frontiersin.org/articles/10.3389/fenvs.2024.1497105/full#supplementary-material
References
Bowser, A., Cooper, C., De Sherbinin, A., Wiggins, A., Brenton, P., Chuang, T.-R., et al. (2020). Still in need of norms: the state of the data in citizen science. Citiz. Sci. Theory Pract. 5 (1), 18. doi:10.5334/cstp.303
Carroll, S. R., Garba, I., Figueroa-Rodríguez, O. L., Holbrook, J., Lovett, R., Materechera, S., et al. (2020). The CARE principles for indigenous data governance. Data Sci. J. 19 (November), 43. doi:10.5334/dsj-2020-043
Chambers, L. E., Barnard, P., Poloczanska, E. S., Hobday, A. J., Keatley, M. R., Allsopp, N., et al. (2017). Southern hemisphere biodiversity and global change: data gaps and strategies. Austral Ecol. 42 (1), 20–30. doi:10.1111/aec.12391
Clark, M. P., Luce, C. H., AghaKouchak, A., Berghuijs, W., David, C. H., Duan, Q., et al. (2021). Open science: open data, open models, and open publications? Water Resour. Res. 57 (4), e2020WR029480. doi:10.1029/2020WR029480
CODATA-ICSTI Task Group on Data Citation Standards and Practices (2013). Out of cite, out of mind: the current state of practice, policy, and Technology for the citation of data. Data Sci. J. 12 (0), CIDCR1–CIDCR75. doi:10.2481/dsj.OSOM13-043
Damerow, J. E., Varadharajan, C., Boye, K., Brodie, E. L., Burrus, M., Dana Chadwick, K., et al. (2021). Sample identifiers and metadata to support data management and reuse in multidisciplinary ecosystem sciences. Data Sci. J. 20 (1), 11. doi:10.5334/dsj-2021-011
Dangles, O., Struelens, Q., Ba, M.-P., Bonzi-Coulibaly, Y., Charvis, P., Emmanuel, E., et al. (2022). Insufficient yet improving involvement of the Global South in top sustainability science publications. PLOS ONE 17 (9), e0273083. doi:10.1371/journal.pone.0273083
Emery, N. C., Crispo, E., Supp, S. R., Farrell, K. J., Kerkhoff, A. J., Bledsoe, E. K., et al. (2021). Data science in undergraduate life science education: a need for instructor skills training. BioScience 71 (12), 1274–1287. doi:10.1093/biosci/biab107
Hampton, S. E., Anderson, S. S., Bagby, S. C., Gries, C., Han, X., Hart, E. M., et al. (2015). The tao of open science for ecology. Ecosphere 6 (7), 1–13. doi:10.1890/ES14-00402.1
Hanson, P. C., Weathers, K. C., and Kratz, T. K. (2016). Networked Lake science: how the Global Lake Ecological observatory network (GLEON) works to understand, predict, and communicate lake ecosystem response to global change. Inland Waters 6 (4), 543–554. doi:10.1080/IW-6.4.904
Hempel, S., Frieler, K., Warszawski, L., Schewe, J., and Piontek, F. (2013). A trend-preserving bias correction – the ISI-mip approach. Earth Syst. Dyn. 4 (2), 219–236. doi:10.5194/esd-4-219-2013
Jennings, E., De Eyto, E., Laas, A., Pierson, D., Mircheva, G., Naumoski, A., et al. (2017). The NETLAKE metadatabase-A tool to support automatic monitoring on lakes in Europe and beyond. Limnol. Oceanogr. Bull. 26 (4), 95–100. doi:10.1002/lob.10210
Jennings, L., Anderson, T., Martinez, A., Sterling, R., Chavez, D. D., Garba, I., et al. (2023). Applying the “CARE principles for indigenous data governance” to ecology and biodiversity research. Nat. Ecol. and Evol. 7 (10), 1547–1551. doi:10.1038/s41559-023-02161-2
Jones, M. B., O’Brien, M., Mecum, B., Boettiger, C., Schildhauer, M., Maier, M., et al. (2019). Ecological Metadata Language (EML). KNB Data Repos. doi:10.5063/F11834T2
Kirschke, S., Van Emmerik, T. H. M., Nath, S., Schmidt, C., and Wendt-Potthoff, K. (2023). Barriers to plastic monitoring in freshwaters in the Global South. Environ. Sci. and Policy 146 (August), 162–170. doi:10.1016/j.envsci.2023.05.011
Koepler, O., Schrade, T., Neumann, S., Stotzka, R., Wiljes, C., Blümel, I., et al. (2021). Sektionskonzept Meta(Daten), Terminologien Und Provenienz Zur Einrichtung Einer Sektion Im Verein Nationale Forschungsdateninfrastruktur (NFDI) e. V.’ Zenodo. doi:10.5281/zenodo.5619089
Kratz, J. E., and Strasser, C. (2015). Researcher perspectives on publication and peer review of data. PLOS ONE 10 (2), e0117619. doi:10.1371/journal.pone.0117619
Krug, R. M., and Petchey, O. L. (2021). Metadata made easy: develop and use domain-specific metadata schemes by following the dmdScheme approach. Ecol. Evol. 11 (14), 9174–9181. doi:10.1002/ece3.7764
Lindenmayer, D. (2018). Why is long-term ecological research and monitoring so hard to do? (And what can Be done about it). Aust. Zool. 39 (4), 576–580. doi:10.7882/AZ.2017.018
Loch, T. K., and Riechers, M. (2021). Integrating indigenous and local knowledge in management and research on coastal ecosystems in the Global South: a literature review. Ocean and Coast. Manag. 212 (October), 105821. doi:10.1016/j.ocecoaman.2021.105821
Löffler, F., Wesp, V., König-Ries, B., and Klan, F. (2021). Dataset search in biodiversity research: do metadata in data repositories reflect scholarly information needs? PLOS ONE 16 (3), e0246099. doi:10.1371/journal.pone.0246099
Mayernik, M. S. (2016). Research data and metadata curation as institutional issues. J. Assoc. Inf. Sci. Technol. 67 (4), 973–993. doi:10.1002/asi.23425
Michener, W. K. (2015). Ten simple rules for creating a good data management plan. PLOS Comput. Biol. 11 (10), e1004525. doi:10.1371/journal.pcbi.1004525
Mirtl, M., Borer, E. T., Djukic, I., Forsius, M., Haubold, H., Hugo, W., et al. (2018). Genesis, goals and achievements of long-term ecological research at the global scale: a critical review of ILTER and future directions. Sci. Total Environ. 626 (June), 1439–1462. doi:10.1016/j.scitotenv.2017.12.001
Otsu, K., Pesquer, L., and Garcia, X. (2024) “Key role of AquaINFRA interactive platform integrated in blue research infrastructures,”. Vienna, Austria. doi:10.5194/egusphere-egu24-206
Peer, L., Green, A., and Stephenson, E. (2014). Committing to data quality review. Int. J. Digital Curation 9 (1), 263–291. doi:10.2218/ijdc.v9i1.317
Potter, R. W. K., and Pearson, B. C. (2023). Assessing the global ocean science community: understanding international collaboration, concerns and the current state of ocean basin research. Npj Ocean. Sustain. 2 (1), 14. doi:10.1038/s44183-023-00020-y
Powers, S. M., and Hampton, S. E. (2019). Open science, reproducibility, and transparency in ecology. Ecol. Appl. 29 (1), e01822. doi:10.1002/eap.1822
Ramachandran, R., Bugbee, K., and Murphy, K. (2021). From open data to open science. Earth Space Sci. 8 (5), e2020EA001562. doi:10.1029/2020EA001562
Reichman, O. J., Jones, M. B., and Schildhauer, M. P. (2011). Challenges and opportunities of open data in ecology. Science 331 (6018), 703–705. doi:10.1126/science.1197962
Rose, K. C., Weathers, K. C., Hetherington, A. L., and Hamilton, D. P. (2016). Insights from the Global Lake Ecological observatory network (GLEON). Inland Waters 6 (4), 476–482. doi:10.1080/IW-6.4.1051
Savage, C. J., and Vickers, A. J. (2009). Empirical study of data sharing by authors publishing in PLoS journals. PLOS ONE 4 (9), e7078. doi:10.1371/journal.pone.0007078
Soranno, P. A., Cheruvelil, K. S., Elliott, K. C., and Montgomery, G. M. (2015). It’s good to share: why environmental scientists’ ethics are out of date. BioScience 65 (1), 69–73. doi:10.1093/biosci/biu169
Stefanoudis, P. V., Licuanan, W. Y., Morrison, T. H., Talma, S., Veitayaki, J., and Woodall, L. C. (2021). Turning the tide of parachute science. Curr. Biol. 31 (4), R184–R185. doi:10.1016/j.cub.2021.01.029
Tedersoo, L., Küngas, R., Oras, E., Köster, K., Eenmaa, H., Leijen, Ä., et al. (2021). Data sharing practices and data availability upon request differ across scientific disciplines. Sci. Data 8 (1), 192. doi:10.1038/s41597-021-00981-0
Tenopir, C., Rice, N. M., Allard, S., Baird, L., Borycz, J., Christian, L., et al. (2020). Data sharing, management, use, and reuse: practices and perceptions of scientists worldwide. PLOS ONE 15 (3), e0229003. doi:10.1371/journal.pone.0229003
Vlah, M. J., Spencer, R., Bernhardt, E. S., Slaughter, W., Gubbins, N., DelVecchia, A. G., et al. (2023). MacroSheds: a synthesis of long-term biogeochemical, hydroclimatic, and geospatial data from small watershed ecosystem studies. Limnol. Oceanogr. Lett. 8 (3), 419–452. doi:10.1002/lol2.10325
Keywords: open data, aquatic sciences, open science, data management, data collection, data sharing, fair principles
Citation: Mesman JP, Barbosa CC, Lewis ASL, Olsson F, Calhoun-Grosch S, Grossart H-P, Ladwig R, La Fuente RS, Münzner K, Nkwalale LGT, Pilla RM, Suresh K and Wain DJ (2024) Challenges of open data in aquatic sciences: issues faced by data users and data providers. Front. Environ. Sci. 12:1497105. doi: 10.3389/fenvs.2024.1497105
Received: 16 September 2024; Accepted: 02 December 2024;
Published: 17 December 2024.
Edited by:
Diego Copetti, National Research Council of Italy, ItalyReviewed by:
Caterina Bergami, National Research Council (CNR), ItalyCopyright © 2024 Mesman, Barbosa, Lewis, Olsson, Calhoun-Grosch, Grossart, Ladwig, La Fuente, Münzner, Nkwalale, Pilla, Suresh and Wain. This is an open-access article distributed under the terms of the Creative Commons Attribution License (CC BY). The use, distribution or reproduction in other forums is permitted, provided the original author(s) and the copyright owner(s) are credited and that the original publication in this journal is cited, in accordance with accepted academic practice. No use, distribution or reproduction is permitted which does not comply with these terms.
*Correspondence: Jorrit P. Mesman, am9ycml0Lm1lc21hbkBlYmMudXUuc2U=
†ORCID: Jorrit P. Mesman, orcid.org/0000-0002-4319-260X; Carolina C. Barbosa, orcid.org/0000-0002-6393-5730; Abigail S. L. Lewis, orcid.org/0000-0001-9933-4542; Freya Olsson, orcid.org/0000-0002-0483-4489; Stacy Calhoun-Grosch, orcid.org/0000-0002-1426-8003; Hans-Peter Grossart, orcid.org/0000-0002-9141-0325; Robert Ladwig, orcid.org/0000-0001-8443-1999; R. Sofia La Fuente, orcid.org/0000-0002-9665-672X; Karla Münzner, orcid.org/0000-0002-7568-8095; Lipa Nkwalale, orcid.org/0009-0004-3832-3056; Rachel M. Pilla, orcid.org/0000-0001-9156-9486; Keerthana Suresh, orcid.org/0000-0003-1930-9318; Danielle J. Wain, orcid.org/0000-0001-5091-102X
‡Present address: Abigail S. L. Lewis, Smithsonian Environmental Research Center, Edgewater, MD, United States