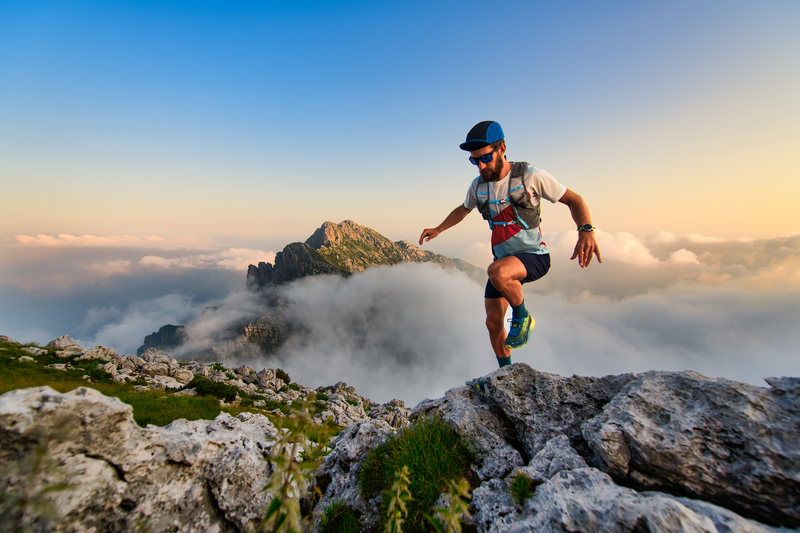
95% of researchers rate our articles as excellent or good
Learn more about the work of our research integrity team to safeguard the quality of each article we publish.
Find out more
ORIGINAL RESEARCH article
Front. Environ. Sci. , 26 November 2024
Sec. Environmental Policy and Governance
Volume 12 - 2024 | https://doi.org/10.3389/fenvs.2024.1491168
This article is part of the Research Topic Climate Risk and Green and Low-Carbon Transformation: Economic Impact and Policy Response View all 18 articles
Taking the “Green Credit Guidelines” (also known as “Green Credit Policy”, GCP) in 2012 as a quasi-natural experiment, this study adopts the panel datasets between 2010 and 2019 for Chinese manufacturing firms listed publicly to examine the effects of GCP on the labor productivity of Chinese heavily-polluting companies. The findings indicate that: (1) Upon introducing GCP, it can improve labor productivity of Chinese heavily-polluting companies, a result of the promotion effect of GCP on commercial credit and technological innovation. (2) The implementation of GCP can enhance the commercial credit of Chinese heavily-polluting enterprises and relieve its financing constraints to some degree. (3) The implementation of GCP can further the innovation activities of Chinese heavily-polluting companies and realize the re-test of the “Porter effect”. (4) The heterogeneity test indicates that GCP exerts a pronounced promoting influence on state-owned and large heavily-polluting enterprises in eastern China. Those conclusions are still valid after robustness tests. This research offers empirical evidence and policy wisdom for promoting the green conversion of manufacturing enterprises.
As a major green finance tool, green credit has progressively garnered the attention of the Chinese government, which promulgated a raft of policies and regulations to highlight the significance of finance in promoting green transformation development. For example, the “Opinions on Implementing Environmental Policies and Regulations to Prevent Credit Risks” published in 2007, emphasized an approach of environmental governance with the environmental sector as the primary and the financial sector as the secondary. “The Notice on the Issuance of Green Credit Guidelines” (call it green credit policy, GCP) released in 2012, defines the specific implementation methods for the green credit. Different from other environmental regulation policies characterized by administrative penalties, GCP is designed to guide heavily-polluting companies towards green innovation by allocating credit resources and accelerating their green transformation.
Given that GCP instructs financial institutions to give preference to cleaner companies when allocating credit funds, and to forbid or strictly regulate the issuance of loans to those with significant negative environmental impacts, this means that only enterprises that create severe environmental harm are subject to the “penalty effect” of the green loan. In this context, the question of how GCP promotes the green development of heavily-polluting industries has been widely concerned (Cai and Ye, 2020). Previous research has mostly looked at how GCP affect the micro-behavior of heavily -polluting enterprises, primarily from the perspective of financial constraints and technological innovation (Lu et al., 2021a; Lian et al., 2022; Cui et al., 2022; Ma et al., 2023) and there is some literature investigating the influence of GCP on the credit resources of financial institutions (Cui et al., 2022). However, few scholars have delved into the micro-effects of the GCP from the perspective of labor productivity. Existing researches of labor productivity mostly focus on the macro level (Zheng et al., 2019; Liu et al., 2021; Zhang and Kong, 2022), and pay little attention to the influencing factors of labor productivity at the micro enterprise level. The capital-labor ratio, which takes into account both labor and capital as the two primary factors influencing an enterprise’s ability to produce goods and services (Ma et al., 2020; Yao et al., 2023), is widely regarded as the key to improving enterprise labor productivity. Therefore, studying whether and how GCP affects the labor productivity of heavily-polluting companies remain an important practical issue (Wang and Wang, 2023).
On basis of the panel datasets between 2010 and 2019 for Chinese manufacturing firms listed publicly, this research takes the GCP of 2012 as a quasi-natural experiment to investigate the effect of GCP on labor productivity of Chinese heavily-polluting enterprises. Firstly, to divide the treatment group and the control group, we created a comprehensive pollution index based upon the waste gas, waste water, and solid waste emissions from the Chinese manufacturing industry. Secondly, we employ the difference-in-difference model (DID) to explore the effect of GCP on labor productivity in Chinese heavily-polluting enterprises. Furthermore, we identify GCP’s mechanism in terms of commercial credit and innovation activities. Finally, we divided those sample enterprises into state-owned and non-state-owned, large-scale and small-scale, eastern, central, and western sub-samples according to ownership, scale, and region. Besides, we investigated the asymmetric effect of GCP on different types of companies.
The main achievements of this study are detailed below: (1) This study quantitatively studies the implementation impacts of GCP from the viewpoint of labor productivity, enriching the relevant research on GCP. (2) Through this research of the innovation compensation effect from GCP, this paper realizes the re-test of the “Porter effect”. (3) From the viewpoint of commercial credit, this study further reveals the impact from GCP on enterprise behavior, and extends the study on the micro-effects of environmental policy.
Regarding heavily-polluting enterprises, the execution of GCP can aggravate their financing difficulties in the short span (Yao et al., 2021; Zhou et al., 2022). Enterprises with serious financing constraints often find it difficult to obtain sufficient external funds, and their investment activities face serious financial constraints (Hadlock and Pierce, 2010; Lin and Pan, 2023). Aghion et al. (2014) found that credit expansion promoted enterprise productivity with high dependence on external financing, thus proving that credit constraints would hinder enterprise productivity.
As a financing channel different from credit financing, commercial credit is a credit agreement reached between enterprises and suppliers and customers based on trust (Ivashina et al., 2022). Commercial credit can not only effectively reduce the transaction cost of financing, but also provide stable cash flow for enterprises and ease the financing limitations faced by enterprises (Luo et al., 2021). Existing studies have confirmed the complementary connection between commercial credit and bank loans. Chang et al. (2019) found that banks regard commercial credit as reliable information about the financial status of enterprises, and the higher the commercial credit obtained by enterprises, the less difficult the financing of banks. Chai et al. (2022) discovered that the more net commercial credit an enterprise provides, the more bank loans it will get. In particular, Chinese private enterprises are more inclined to rely on commercial credit financing, while state-owned enterprises are not inclined to use informal finance even in financial crises (Lin and Chou, 2015). It can be seen that commercial credit can alleviate the financing constraints of heavily-polluting enterprises to a certain degree, and encourage them to increase human capital investment, ultimately promoting their labor productivity (Casino-Martínez et al., 2023).
Existing studies have also found that environmental regulation affects enterprises production activities by promoting their technological innovation, and finally has an important impact on enterprises labor productivity (Johnstone et al., 2017; Li et al., 2019; Wang et al., 2022; Xu et al., 2023; Zhang et al., 2023b). GCP, as an incentive environmental policy issued by the Chinese government, mainly affects enterprises production activities in the following two ways (see Figure 1).
Figure 1. The mechanism framework of green credit policy and labor productivity of Chinese heavily-polluting enterprises.
Firstly, green credit policy takes enterprises environmental performance as the evaluation criteria for credit eligibility, which will lead heavy-polluters to minimize manufacturing scale and invest greater costs in pollution control and emission mitigation activities (Zhu et al., 2021; Yu et al., 2023; Ma et al., 2023). Dang et al. (2022) found enterprises are more likely to face the situation of under-investment in human capital, which ultimately affects its labor productivity. Secondly, banks usually give green credit loans to businesses that have already invested in environmental governance or have the potential to do so (Zhang et al., 2021; An et al., 2021; Yin et al., 2023), which can effectively alleviate its financing constraints due to environmental governance investment. This can encourage heavily-polluting enterprises to purchase clean production equipment, introduce or independently research and develop green production technology (Hu et al., 2021; Zhang and Zhao, 2023), and be more likely to play an alternative role to traditional labor factors, which is not only conducive to shortening production hours, but also improve enterprises labor production efficiency (Chakraborty et al., 2023).
Moreover, recent research shows that green credit policies (GCP) have a significant crowding-out effect on the labor demand of high-polluting enterprises, and this effect is primarily achieved by enhancing the productivity of these enterprises (Jiang and Jiang, 2023). Although companies may face financing challenges in the short term, in the long run, GCP could have a positive impact on labor demand by promoting efficiency improvements. Another study further confirmed that GCP significantly enhances total factor productivity in enterprises by promoting the fulfillment of corporate social responsibility and increasing R&D investment, particularly showing notable effects in large enterprises and state-owned enterprises (Ge et al., 2024). These findings highlight the potential of GCP in enhancing labor productivity by promoting enterprises to leverage technological innovation and improve production efficiency.
On the basis of the above discussion, this article puts forth these hypotheses:
H1: GCP implementation can significantly increase the labor productivity in Chinese heavily-polluting businesses.
H2a: The commercial credit of Chinese companies heavily involved in pollution can be notably improved by introducing green credit policies.
H2b: The implementation of green credit policy can improve the labor efficiency of Chinese heavily-polluting enterprises by promoting their commercial credit.
H3a: The implementation of GCP can notably spurs the technological innovation of Chinese heavily-polluting enterprises.
H3b: The labor efficiency of Chinese heavily-polluting enterprises can be increased by promoting their technological innovation through the implementation of GCP.
Firstly, according to the China Environmental Protection Statistical Yearbook in 2011, following the research of Chai et al. (2022), we calculate the variation coefficient of each industrial pollutant, which is the ratio of the standard deviation of each industrial pollutant emission and its mean value (
We conduct dimensionally standardized treatment on each industrial pollutant and multiply the corresponding weight (
where
Taking the arithmetic mean value of L and H to quantify the pollution extent of different industries. The manufacturing industry is classified according to its arithmetic mean value. Industries with a pollution degree higher than the arithmetic mean value are defined as heavily-polluting industries, while those with a pollution degree lower than the arithmetic mean value are tagged as non-heavy polluting industries. Founded on this, we designate the heavily-polluting sector marked as the treatment group and the non-heavy polluting sector marked as the control group, as depicted in Table 1.
Secondly, we select the panel datasets between 2010 and 2019 for Chinese manufacturing firms listed publicly as the initial samples, then process them as follows to obtain all samples in this paper: (1) Excluded all ST and *ST samples; (2) Excluded samples marked by serious data gaps; (3) Excluded the initial samples with an asset-liability ratio greater than 1; (4) Winsorized the first and last 1% of all continuous variables and finally obtained a total of 10,841 observations for 1,255 enterprises. The dataset consisted of 360 heavily-polluting companies and 895 non-heavily-polluting ones, sourced from the CSMAR database.
We adopt the two-way fixed effects model to investigate the effect of GCP on the labor productivity of Chinese heavily-polluting enterprises. It provides more consistent parameter estimates than random effects models (Bell et al., 2019); it can make better use of time-series information from panel data than difference-in-difference models (Fredriksson and Oliveira, 2019); and it does not need to rely on strong assumptions about instrumental variable assumptions compared to instrumental variable approaches (Milner et al., 2018). Two-way fixed effects models not only effectively control for the invariant properties of both the firm and time dimensions, thereby reducing omitted variable bias and endogeneity problems, but also fully exploit the time-series and cross-sectional information in panel data to improve the explanatory power and predictive accuracy of the model (Fredriksson and Oliveira, 2019; Gauthier, 2021). The regression equation is as Equation 4:
where
Referencing the research of Wang and Wei (2017), we adopt the operating income to workforce ratio to determine labor productivity in enterprises.
Referring to the studies by Hasan and Du (2023) and Zhang et al. (2023b), the annual number of green patents granted is selected to assess corporate technological innovation. We also use the quotient of the combined values of payables, notes payable, and prepaid accounts against total assets to measure an enterprise’s commercial credit.
In this paper, we add individual-level control variables, such as firm size (Li et al., 2019), debt level (Yao et al., 2023), firm’s return on total assets (ROA) (Zhou et al., 2022), and enterprise ownership (Zhu et al., 2021). To reduce the impact of outliers on empirical results, in this paper each continuous variable is winsorized up or down by 1%. Table 2 outlines the names and corresponding definitions of each variable.
The results of Table 3 showed that before the policy was implemented, the mean value and median value of the firm’s labor productivity in the treated group are 1.406 and 0.890, respectively; while after the policy was implemented, the mean and median of companies’ labor productivity in the treated group are 1.617 and 1.053, respectively. The mean difference of the treated group is −0.211, statistically significant at a 5% level, and the median Wilcoxon rank sum test z value of the treated group is 21.827, showing significance at 1% level. This indicates that the labor productivity of Chinese heavily-polluting companies increased after the policy was implemented, and the above results confirm the assumption of this paper.
Table 3. Changes in enterprise labor productivity of the treated group before and after green credit policy.
The data from Table 4 reveals that the average of the firm’s labor productivity is 1.221, while the median productivity stands at 0.799, and the standard deviation is 2.080. The labor productivity ranges from nearly 0 to over 80, highlighting substantial variability among the sampled companies. The mean value of enterprise size is 8.312 and the median value is 8.167, suggesting that the size distribution in the sample is relatively concentrated. The average of enterprise ownership is 0.383, indicating that 38.3% of the sample is privately owned.
As indicated by the result in Table 5, the greater part of the control variables have a significant connection to the firm’s labor productivity of Chinese heavily-polluting enterprises, and the correlation coefficients are no more than 0.5, indicating that the probability of multicollinearity between variables is small, and the choice of control variables in this paper is reasonable to a certain extent.
It can be seen from Table 6, before adding control variables, the coefficient of column (1) is 0.317, statistically significant with a p-value of less than 0.05. This result implies that GCP can significantly boost the labor productivity of heavily-polluting companies and also verify the existence of the “strong” Porter Hypothesis in heavily-polluting industry. This aligns with the results reported by Cui et al. (2022), thereby confirming H1. After including the inclusion of control variables, the coefficient for column (2) remains at 0.321, with a 95% confidence level. Thus, the paper’s base regression outcome proves study.
To investigate the dynamic impact of GCP over time and to validate the parallel trend hypothesis of the differential model, we adopt the event study approach proposed by Jacobson et al. (1993) for testing the parallel trend. According to Figure 2, the estimated coefficient of the firm’s labor productivity was basically insignificant before 2012, affirming the validity of the parallel trend test. While the estimated coefficient of the firm’s labor productivity is significantly positive after 2012, which indicates a substantial and positive impact of the GCP on enhancing labor productivity in heavily-polluting manufacturing firms, albeit with a time lag. The above results show that the conclusions of baseline regression analysis are robust.
Referring to Ferrara et al. (2012), we construct the new treatment and control groups generated by random sampling. Based on Model (1), regression analysis was repeated for 1,000 times, as shown in Figure 3. We find that the distribution of the estimated coefficient with a normal distribution, centered around a mean value of 0.0001, alongside a nearly universal P-value exceeding 0.1. It aligns well with the placebo test’s anticipation, which indicates that there are no non-observed factors interfering with the estimated results, and the above empirical results passed the placebo test.
This section modifies the treatment group’s and control group’s classification criteria to verify the robustness of the aforementioned empirical finding. According to the China Securities Regulatory Commission’s 2012 publication, “Guidance on Industry Classification of Listed Companies”, businesses with the industry codes C17, C19, C22, C25, C26, C28, C29, C30, C31, and C32 are categorized as belonging to the control group. The coefficient of column (1) in Table 7 is 0.289 before controlling factors are added, which is significant at the 5% level. Even with controlling factors included, the column (2) coefficient remains positive at the 5 percent level, which further supports the robustness of the regression results.
The robustness of results may be compromised by bias in the sample selection process. In this study, the propensity score matching (PSM) approach is employed, and a total of 5,156 samples are obtained after propensity score matching according to 1:2 nearest neighbor. Then, a regression analysis yields the results detailed in Table 7. After controlling the fixed effects of enterprise and time, the coefficient of the column (3) without controlling control variables is 0.461, significant at the 5% level. After incorporating control factors, the coefficient of the column (4) landed at 0.481, which still remains statistically significant at the 5% level, suggesting that our findings in this study are robust.
After incorporating control variables alongside fixed effects of enterprise and industry, following the research of Baron and Kenny (1986) and Wen et al. (2004), the results of the mediating influence are shown in Table 8.
According to the results in Table 6, the total influence of GCP on the firm’s labor productivity is 0.321. After controlling the effect of commercial credit, the direct influence of GCP on the firm’s labor productivity is 0.291 (see in column (2) in Table 8). Depending on the research of Baron and Kenny (1986) and Wen et al. (2004), the commercial credit mediating influence accounts for 0.029 (0.291*2.447), about 9.03% (0.029/0.321) of the total effect. It shows that commercial credit plays a partial mediating role between GCP and the labor productivity of heavily-polluting businesses, and the H2a was verified. The above findings indicate that GCP helps to exert the environmental governance effect of financial resources by guiding the judicious distribution of bank resources among enterprises, and thus enhancing the labor productivity of heavily-polluting enterprises, which verifies H2b.
After controlling the effect of technological innovation, the direct influence of GCP on the firm’s labor productivity is 0.271 (see in column (4) in Table 8). Based on the research of Baron and Kenny (1986) and Wen et al. (2004), the impact of technological innovation as a mediator is 0.011 (0.271*0.04), about 3.43% (0.011/0.321) of the total effect. It verifies that technological innovation serves a partial mediating function in the influence of GCP on companies’ labor productivity, and the H3a is verified. Those findings indicate that GCP, as an environmental policy tool, can stimulate the internal motivation of enterprise technological innovation, thus increasing the labor productivity of Chinese heavily-polluting companies, which retested the “Porter effect” and the H3b is verified.
Given China’s institutional background, state-owned enterprises (SOE) and private enterprises face de facto unequal competition. Compared with private enterprises, SOE give more emphasis to achieving their social and environmental responsibilities. Furthermore, SOE generally have better access to financial support from banks and financial institutions compared to private enterprises. According to the result of column (1) and (2) in Table 9, there are significant different impacts of GCP on the labor productivity of SOE and POE (see column (1) and column (2)). Specifically, GCP positively impacts labor productivity in SOE, showing significance at the 5% level. While GCP positively affects the SOE’s labor productivity, it is not significant. It has been verified that GCP’s influence on labor productivity of heavily-polluting manufacturing companies is more obvious in SOE.
Varying significantly in operating efficiency, internal control, and funding capacity, enterprises across different sizes showcase stark disparities. “ Constrained by GCP, small enterprises have to halt green production activities due to financial limitations, leading to a vicious circle of “financing difficulties-green transformation difficulties”. This study defines enterprises above the industry median size as large companies (LC) and those below as small companies (SC). Table 9 columns (3) and (4) depict the varied effects of GCP on firms’ labor productivity across scales. The regression coefficient in column (3) is positively significant at the 5 percent level, in contrast to the negative and non-significant regression coefficient in column (4). This finding implies that GCP has a greater effect on labor productivity in heavily polluting manufacturing companies when compared to smaller businesses.
In contrast to central China and western China, the economic development level and financial market environment of Eastern China are relatively better, and the government and enterprises focus more on environmental protection. Therefore, enterprises in eastern China are less likely to be restricted by green credit policy because their environmental performance does not meet the standards. Based upon the province (city) where the firm is registered, we examined the heterogeneous impact of GCP on labor productivity from eastern China, central China, and western China. The results of column (5) to (7) in Table 9 show that GCP has a significant regional heterogeneity in its promotion of the enterprise’s labor productivity. Specifically, the coefficient for the fifth column is 0.420, indicating significance at the 5% level. The sixth and seventh columns’ coefficients, which are 0.247 and −0.059, respectively, are not significant. It indicates that GCP can only promote the firm’s labor productivity in Eastern China.
This research adopts panel datasets between 2010 and 2019 for Chinese manufacturing firms listed publicly. Its objective was to explore how GCP affects the labor productivity of heavily-polluting enterprises in China, along with the micro-mechanisms involved. Then, the analytical results indicate that: (1) The implementation of GCP has raised the labor productivity of Chinese heavily-polluting enterprises, which means GCP can foster a win-win scenario where environmental conservation and economic expansion coexist harmoniously. (2) By introducing GCP, the financial hurdles for heavily-polluting enterprises in China intensify, thus stimulating those enterprises to actively seek external commercial credit and obtain more financial support. (3) The implementation of GCP can stimulate innovation activities among Chinese heavily-polluting firms, and has produced the “Porter effect”. (4) The heterogeneity test shows that GCP’s stronger influence on state-owned and large heavily-polluting enterprises in eastern China.
Following the outcomes and conclusions of the empirical study, this dissertation put forward policy recommendations as follows:
It is essential for government departments to boost their monitoring of commercial banks, so that they can efficiently screen enterprises for environmental risks. For example, regularly conduct compliance reviews of banks’ environmental risk management to ensure they adhere to GCP. At the same time, provide training and technical support to banks to help them enhance their capabilities in environmental risk assessment and green project evaluation.
Banks implement differentiated credit policies for enterprises. For enterprises that perform poorly environmentally, banks should strictly control the credit lines. At the same time, they should reduce credit constraints on enterprises with good environmental performance. Such measures can force enterprises with poor environmental performance to undertake green technological innovation.
For enterprises that possess strong commercial credit financing ability, the financing constraint influence of GCP is weakened, and enterprises still lack the motivation to improve their environmental performance. Therefore, the government needs to enhance the environmental protection policies for these enterprises, establish a mandatory environmental information disclosure system, and effectively promote their green transformation.
Publicly available datasets were analyzed in this study. This data can be found here: Data will be made available on request.
YL: Conceptualization, Resources, Supervision, Writing–review and editing. SZ: Formal Analysis, Methodology, Writing–original draft. PZ: Conceptualization, Methodology, Supervision, Writing–review and editing. HL: Conceptualization, Formal Analysis, Funding acquisition, Methodology, Writing–review and editing. XD: Conceptualization, Formal Analysis, Methodology, Writing–original draft, Writing–review and editing.
The author(s) declare that financial support was received for the research, authorship, and/or publication of this article. This research was supported by the National Natural Science Foundation of China (Nos. 72404086, 72203059), the National Natural Science Foundation of Hunan Province (Nos. 2023JJ40336), the Humanities and Social Science Project of the Ministry of Education (Nos. 22YJCZH078), and the Research Foundation of Education Bureau of Hunan Province (Nos. 23B0198, 22B0195).
The authors declare that the research was conducted in the absence of any commercial or financial relationships that could be construed as a potential conflict of interest.
All claims expressed in this article are solely those of the authors and do not necessarily represent those of their affiliated organizations, or those of the publisher, the editors and the reviewers. Any product that may be evaluated in this article, or claim that may be made by its manufacturer, is not guaranteed or endorsed by the publisher.
Aghion, P., Hemous, D., and Kharroubi, E. (2014). Cyclical fiscal policy, credit constraints, and industry growth. J. Monetary Econ. 62, 41–58. doi:10.1016/j.jmoneco.2013.12.003
An, S., Li, B., Song, D., and Chen, X. (2021). Green credit financing versus trade credit financing in a supply chain with carbon emission limits. Eur. J. Operational Res. 292 (1), 125–142. doi:10.1016/j.ejor.2020.10.025
Baron, R. M., and Kenny, D. A. (1986). The moderator–mediator variable distinction in social psychological research: conceptual, strategic, and statistical considerations. J. personality Soc. Psychol. 51 (6), 1173–1182. doi:10.1037/0022-3514.51.6.1173
Bell, A., Fairbrother, M., and Jones, K. (2019). Fixed and random effects models: making an informed choice. Qual. and quantity 53, 1051–1074. doi:10.1007/s11135-018-0802-x
Cai, W., and Ye, P. (2020). How does environmental regulation influence enterprises’ total factor productivity? A quasi-natural experiment based on China’s new environmental protection law. J. Clean. Prod. 276, 124105. doi:10.1016/j.jclepro.2020.124105
Casino-Martínez, A., López-Gracia, J., and Mestre-Barberá, R. (2023). The regulatory environment and financial constraints of private firms in the European Union. Glob. Finance J. 55, 100798. doi:10.1016/j.gfj.2022.100798
Chai, S., Zhang, K., Wei, W., Ma, W., and Abedin, M. Z. (2022). The impact of green credit policy on enterprises' financing behavior: evidence from Chinese heavily-polluting listed companies. J. Clean. Prod. 363, 132458. doi:10.1016/j.jclepro.2022.132458
Chakraborty, P., Chakrabarti, A. S., and Chatterjee, C. (2023). Cross-border environmental regulation and firm labor demand. J. Environ. Econ. Manag. 117, 102753. doi:10.1016/j.jeem.2022.102753
Chang, K., Zeng, Y., Wang, W., and Wu, X. (2019). The effects of credit policy and financial constraints on tangible and research and development investment: firm-level evidence from China's renewable energy industry. Energy policy 130, 438–447. doi:10.1016/j.enpol.2019.04.005
Cui, X., Wang, P., Sensoy, A., Nguyen, D. K., and Pan, Y. (2022). Green credit policy and corporate productivity: evidence from a quasi-natural experiment in China. Technol. Forecast. Soc. Change 177, 121516. doi:10.1016/j.techfore.2022.121516
Dang, T. V., Wang, Y., and Wang, Z. (2022). The role of financial constraints in firm investment under pollution abatement regulation. J. Corp. Finance 76, 102252. doi:10.1016/j.jcorpfin.2022.102252
Ferrara, E. L., Chong, A., and Duryea, S. (2012). Soap operas and fertility: evidence from Brazil. American Econo. J. Appli. Econo. 4 (4), 1–13.
Fredriksson, A., and Oliveira, G. M. D. (2019). Impact evaluation using Difference-in-Differences. RAUSP Manag. J. 54, 519–532. doi:10.1108/rausp-05-2019-0112
Gauthier, G. (2021). On the use of two-way fixed effects models for policy evaluation during pandemics. Econo. Med. 6, 10949. doi:10.48550/arXiv.2106.10949
Ge, S., Luo, X., Li, Y., and Zheng, L. (2024). The impact of green credit policy on total factor productivity of enterprises. Int. Rev. Econ. and Finance 95, 103480. doi:10.1016/j.iref.2024.103480
Hadlock, C. J., and Pierce, J. R. (2010). New evidence on measuring financial constraints: moving beyond the KZ index. Rev. financial Stud. 23 (5), 1909–1940. doi:10.1093/rfs/hhq009
Hasan, M. M., and Du, F. (2023). Nexus between green financial development, green technological innovation and environmental regulation in China. Renew. Energy 204, 218–228. doi:10.1016/j.renene.2022.12.095
Hu, G., Wang, X., and Wang, Y. (2021). Can the green credit policy stimulate green innovation in heavily polluting enterprises? Evidence from a quasi-natural experiment in China. Energy Econ. 98, 105134. doi:10.1016/j.eneco.2021.105134
Ivashina, V., Laeven, L., and Moral-Benito, E. (2022). Loan types and the bank lending channel. J. Monetary Econ. 126, 171–187. doi:10.1016/j.jmoneco.2021.11.006
Jacobson, L. S., LaLonde, R. J., and Sullivan, D. G. (1993). Earnings losses of displaced workers. The American economic review., 685–709.
Jiang, P., and Jiang, H. (2023). Green finance policy and labor demand. Econ. Lett. 225, 111065. doi:10.1016/j.econlet.2023.111065
Johnstone, N., Managi, S., Rodríguez, M. C., Haščič, I., Fujii, H., and Souchier, M. (2017). Environmental policy design, innovation and efficiency gains in electricity generation. Energy Econ. 63, 106–115. doi:10.1016/j.eneco.2017.01.014
Li, H. L., Zhu, X. H., Chen, J. Y., and Jiang, F. T. (2019). Environmental regulations, environmental governance efficiency and the green transformation of China's iron and steel enterprises. Ecol. Econ. 165, 106397. doi:10.1016/j.ecolecon.2019.106397
Lian, Y., Gao, J., and Ye, T. (2022). How does green credit affect the financial performance of commercial banks? evidence from China. J. Clean. Prod. 344, 131069. doi:10.1016/j.jclepro.2022.131069
Lin, B., and Pan, T. (2023). Financing decision of heavy pollution enterprises under green credit policy: based on the perspective of signal transmission and supply chain transmission. J. Clean. Prod. 412, 137454. doi:10.1016/j.jclepro.2023.137454
Lin, T. T., and Chou, J. H. (2015). Trade credit and bank loan: evidence from Chinese firms. Int. Rev. Econ. and Finance 36, 17–29. doi:10.1016/j.iref.2014.11.004
Liu, M., Tan, R., and Zhang, B. (2021). The costs of “blue sky”: environmental regulation, technology upgrading, and labor demand in China. J. Dev. Econ. 150, 102610. doi:10.1016/j.jdeveco.2020.102610
Lu, J., Yan, Y., and Wang, T. X. (2021a). A study on the micro effects of green credit policy - based on the perspective of technological innovation and resource reallocation. China Ind. Econ. 01, 174–192. doi:10.19581/j.cnki.ciejournal.2021.01.010
Luo, S., Yu, S., and Zhou, G. (2021). Does green credit improve the core competence of commercial banks? Based on quasi-natural experiments in China. Energy Econ. 100, 105335. doi:10.1016/j.eneco.2021.105335
Ma, Y., Sha, Y., Wang, Z., and Zhang, W. (2023). The effect of the policy mix of green credit and government subsidy on environmental innovation. Energy Econ. 106512. doi:10.1016/j.eneco.2023.106512
Ma, Y., Zhang, Q., and Yin, H. (2020). Environmental management and labor productivity: the moderating role of quality management. J. Environ. Manag. 255, 109795. doi:10.1016/j.jenvman.2019.109795
Milner, A., Aitken, Z., Kavanagh, A., LaMontagne, A. D., Pega, F., and Petrie, D. (2018). Combining fixed effects and instrumental variable approaches for estimating the effect of psychosocial job quality on mental health: evidence from 13 waves of a nationally representative cohort study. J. Public Health 40 (2), 426–434. doi:10.1093/pubmed/fdx070
Wang, W. T., and Wei, S. G. (2023). How does the gender structure affect firm productivity?. Finan. Trade. Econo. 06, 130–146. (in Chinese)
Wang, C., and Wang, L. (2023). Green credit and industrial green total factor productivity: the impact mechanism and threshold effect tests. J. Environ. Manag. 331, 117266. doi:10.1016/j.jenvman.2023.117266
Wang, H., Qi, S., Zhou, C., Zhou, J., and Huang, X. (2022). Green credit policy, government behavior and green innovation quality of enterprises. J. Clean. Prod. 331, 129834. doi:10.1016/j.jclepro.2021.129834
Wen, Z., and Ye, B. (2004). Analyses of mediating effects: the development of methods and models. Advan. psycholo. Sci. 22 (5), 731.
Xu, Y., Dong, Z., and Wu, Y. (2023). The spatiotemporal effects of environmental regulation on green innovation: evidence from Chinese cities. Sci. Total Environ. 876, 162790. doi:10.1016/j.scitotenv.2023.162790
Yao, S., Pan, Y., Sensoy, A., Uddin, G. S., and Cheng, F. (2021). Green credit policy and firm performance: what we learn from China. Energy Econ. 101, 105415. doi:10.1016/j.eneco.2021.105415
Yao, W., Zhang, Y., Ma, J., and Cui, G. (2023). Does environmental regulation affect capital-labor ratio of manufacturing enterprises: evidence from China. Int. Rev. Financial Analysis 86, 102485. doi:10.1016/j.irfa.2023.102485
Yin, X., Chen, D., and Ji, J. (2023). How does environmental regulation influence green technological innovation? Moderating effect of green finance. J. Environ. Manag. 342, 118112. doi:10.1016/j.jenvman.2023.118112
Yu, H., Wang, J., Hou, J., Yu, B., and Pan, Y. (2023). The effect of economic growth pressure on green technology innovation: do environmental regulation, government support, and financial development matter? J. Environ. Manag. 330, 117172. doi:10.1016/j.jenvman.2022.117172
Zhang, D., and Kong, Q. (2022). Credit policy, uncertainty, and firm R&D investment: a quasi-natural experiment based on the Green Credit Guidelines. Pacific-Basin Finance J. 73, 101751. doi:10.1016/j.pacfin.2022.101751
Zhang, M., Yan, T., Gao, W., Xie, W., and Yu, Z. (2023b). How does environmental regulation affect real green technology innovation and strategic green technology innovation? Sci. Total Environ. 872, 162221. doi:10.1016/j.scitotenv.2023.162221
Zhang, M., and Zhao, Y. (2023). Does environmental regulation spur innovation? Quasi-natural experiment in China. World Dev. 168, 106261. doi:10.1016/j.worlddev.2023.106261
Zhang, S., Wu, Z., Wang, Y., and Hao, Y. (2021). Fostering green development with green finance: an empirical study on the environmental effect of green credit policy in China. J. Environ. Manag. 296, 113159. doi:10.1016/j.jenvman.2021.113159
Zheng, T., Zhao, Y., and Li, J. (2019). Rising labour cost, environmental regulation and manufacturing restructuring of Chinese cities. J. Clean. Prod. 214, 583–592. doi:10.1016/j.jclepro.2018.12.328
Zhou, X. Y., Caldecott, B., Hoepner, A. G., and Wang, Y. (2022). Bank green lending and credit risk: an empirical analysis of China's Green Credit Policy. Bus. Strategy Environ. 31 (4), 1623–1640. doi:10.1002/bse.2973
Keywords: green credit policy, labor productivity, commercial credit, technological innovation, heavily-polluting enterprises
Citation: Lan Y, Zhang S, Zhou P, Li H and Dong X (2024) Green credit policy and firm’s labor productivity: evidence from Chinese heavily-polluting enterprises. Front. Environ. Sci. 12:1491168. doi: 10.3389/fenvs.2024.1491168
Received: 04 September 2024; Accepted: 11 November 2024;
Published: 26 November 2024.
Edited by:
Jinyu Chen, Central South University, ChinaReviewed by:
Jingxing Liu, Huaqiao University, ChinaCopyright © 2024 Lan, Zhang, Zhou, Li and Dong. This is an open-access article distributed under the terms of the Creative Commons Attribution License (CC BY). The use, distribution or reproduction in other forums is permitted, provided the original author(s) and the copyright owner(s) are credited and that the original publication in this journal is cited, in accordance with accepted academic practice. No use, distribution or reproduction is permitted which does not comply with these terms.
*Correspondence: Xuesong Dong, Y29kZW5hbWVkeHMyMDIyQDE2My5jb20=
Disclaimer: All claims expressed in this article are solely those of the authors and do not necessarily represent those of their affiliated organizations, or those of the publisher, the editors and the reviewers. Any product that may be evaluated in this article or claim that may be made by its manufacturer is not guaranteed or endorsed by the publisher.
Research integrity at Frontiers
Learn more about the work of our research integrity team to safeguard the quality of each article we publish.