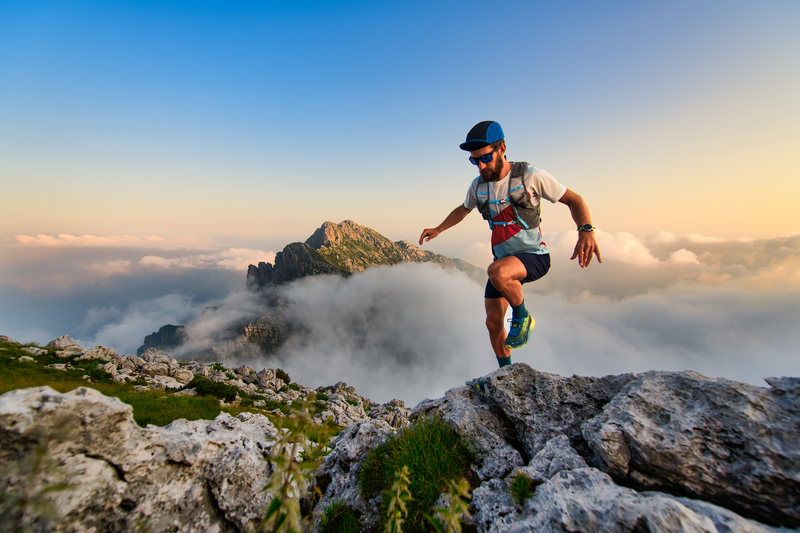
94% of researchers rate our articles as excellent or good
Learn more about the work of our research integrity team to safeguard the quality of each article we publish.
Find out more
ORIGINAL RESEARCH article
Front. Environ. Sci. , 29 October 2024
Sec. Soil Processes
Volume 12 - 2024 | https://doi.org/10.3389/fenvs.2024.1489070
This article is part of the Research Topic Regenerative Agriculture for Soil Health, Greenhouse Gas Mitigation, and Climate Action View all articles
Developing successful mitigation strategies for greenhouse gases (GHGs) from crop residue returned to the soil can be difficult due to an incomplete understanding of factors controlling their magnitude and direction. Therefore, this study investigates the effects of varying levels of wheat residue (WR) and nutrient management on GHGs emissions (CO2, N2O, and CH4) across three soil types: Alfisol, Vertisol, and Inceptisol. A combination of laboratory-based measurements and a variety of data analysis techniques was used to assess the GHG responses under four levels of WR inputs (0, 5, 10, and 15 Mg/ha; WR0, WR5, WR10, and WR15) and three levels of nutrient (NP0: no nutrient, NP1: nutrients (N and P) were added to balance the residue C/nutrient stoichiometry of C/N/P= 100: 8.3: 2.0 to achieve 30% stabilization of added residue C input at 5 Mg/ha (R5), and NP2: 3 × NP1). The results of this study clearly showed that averaged across residue and nutrient input, Inceptisol showed negative N2O flux, suggesting consumption which was supported by its high legacy phosphorus (19.7 mg kg⁻1), elevated pH (8.49), and lower clay content (13%), which reduced microbial activity, as indicated by lower microbial biomass carbon (MBC) and alkaline phosphatase (Alk-P) levels. N2O emissions were more responsive to nutrient inputs, particularly in Vertisol under high WR (15 Mg/ha) input, while CH4 fluxes were significantly reduced under high residue inputs, especially in Vertisol and Inceptisol. Alfisol exhibited the highest total carbon mineralization and GWP, with cumulative GWP being 1.2 times higher than Vertisol and 1.4 times higher than Inceptisol across residue and nutrient input. The partial least square (PLS) regression revealed that anthropogenic factors significantly influenced CO2 and N2O fluxes more than CH4. The anthropogenic drivers contributed 62% and 44% of the variance explained for N2O and CH4 responses. Our study proves that different biogeochemical mechanisms operate simultaneously depending on the stoichiometry of residue C and nutrients influencing soil GHG responses. Our findings provide insight into the relative contribution of anthropogenic and natural drivers to agricultural GHG emissions, which are relevant for developing process-based models and addressing the broader challenge of climate change mitigation through crop residue management.
Nitrous oxide (N₂O) and methane (CH₄) are two of the most significant greenhouse gases (GHGs) associated with agriculture, primarily due to their substantial global warming potentials 273 and 27 times higher than carbon dioxide (CO₂), respectively (Nabuurs et al., 2022). As global concerns about climate change intensify, understanding the sources and drivers of these emissions becomes increasingly important. Agricultural soils, which are vital for food production, are also major sources of N₂O and CH₄, driven by both natural factors and human activities (Gatica et al., 2020; Abalos et al., 2022). While there is consensus that both natural (e.g., soil type, climate) and anthropogenic (e.g., intensive agriculture, enhanced inputs) factors contribute to GHG emissions, the specific roles and relative magnitudes of these drivers are still subjects of active research and debate (Abalos et al., 2022; Wang C. et al., 2021).
Agricultural soils are currently the leading anthropogenic source of N₂O and CH₄ emissions, largely due to the intensification of high-input agricultural activities aimed at meeting the food demands of an expanding global population (Fontaine et al., 2004; Della Chiesa et al., 2019; Gatica et al., 2020). The extensive use of synthetic fertilizers, coupled with crop residue management practices, has significantly increased the fluxes of these gases from soils (Oertel et al., 2016; Wang X. D. et al., 2021; Abalos et al., 2022). However, natural factors such as soil type defined by long-term climate, topography, vegetation, and parent material (Dror et al., 2022) also play a critical role in modulating the magnitude of these emissions (Wang C. et al., 2021). The interaction between these natural factors and anthropogenic activities adds complexity to our understanding of GHG sources and emissions, particularly considering agricultural systems’ spatial and temporal variability.
The global agricultural landscape is diverse, with a wide range of soil types that differ in physical and chemical properties. This diversity is especially pronounced in India, where soil types vary significantly across regions due to the country’s varied climate, topography, and vegetation (Chandrakala et al., 2021). These soil types inherently possess different capacities for GHG emissions, influenced by factors such as soil texture, organic matter content, pH, and cation exchange capacity (Wang X. D. et al., 2021; Ren et al., 2024). Soil texture, an inherent property, influences GHG emission through its effects on soil porosity, aeration, moisture retention, and organic matter decomposition, affecting oxygen availability and thus changing the proportion of GHGs attributed to aerobic nitrification/methanotrophy and anaerobic denitrification/methanogenesis (Ball, 2013). For instance, clayey soils, with their higher water retention, tend to promote anaerobic conditions conducive to the production of N₂O and CH₄, while sandy soils, with better aeration, may release more CO₂ but lower amounts of N₂O and CH₄ (Oertel et al., 2016; Yu et al., 2019). N2O emission flux and production potential were higher in clay than in loam soil (Liu et al., 2023a).
Other soil parameters, such as pH (Šimek and Cooper, 2002), cation exchange capacity (CEC) (Li et al., 2021b), sorption capacity of soil particles for soil organic matter (Cui et al., 2023), and clay mineralogy (Rakhsh et al., 2017), further modulate GHG emissions. For instance, soil pH can influence the microbial processes that drive GHG emissions, with studies showing varying effects depending on the specific soil type and environmental conditions (Wang C. et al., 2021). The analyses of 50 years of published data sets showed that nitrous oxide emissions were less in acidic than in neutral or slightly alkaline soils (Šimek and Cooper, 2002). Contrarily, N2O emissions for field experiments showed that nitrous oxide emissions are generally lower for soils with elevated pH values (Pfülb et al., 2024). A lower soil pH of 5.7 in silt clay loam soil in a wet climate increased the emissions of N2O during denitrification by suppressing the reduction of N2O to N2 as compared to a soil pH of 7.0 in sandy loam soils in a drier climate (Hargreaves et al., 2021). In a laboratory study, soils of varying textures exhibited a negative nonlinear correlation between soil pH and the N2O/(N2+N2O) ratio (Khalifah and Foltz, 2024). Similarly, the CEC of soil, which is determined by its clay content and type, affects the soil’s ability to retain nutrients and organic matter, thereby influencing GHG fluxes (Li et al., 2021b). Soils with high CEC, such as those rich in smectitic clays, tend to retain more organic matter and nutrients, which can lead to higher GHG emissions under certain conditions. Conversely, soils with low CEC, such as those dominated by kaolinitic clays, may mineralize soil organic carbon more rapidly, resulting in different patterns of GHG emissions (Rakhsh et al., 2017). The NH4-N contents and AOA amoA and nirS gene copies were lower in the silty clay soil than in the sandy clay loam soil, which had a high clay content and cation exchange capacity. Consequently, the N2O emissions were higher in the sandy clay loam soil (low sand content and cation exchange capacity) than in the silty clay soil (high clay content and cation exchange capacity) (Yu et al., 2019). These inherent soil properties (natural factors), combined with the effects of agricultural practices (anthropogenic), create a complex web of interactions that determine the net GHG emissions from agricultural soils.
Accurate estimates of the source effect on N₂O and CH₄ emissions from agricultural soils are vital due to the global importance of agriculture in food supply and GHG emissions (Li et al., 2021b; Wang X. D. et al., 2021; Shumba et al., 2023). Mitigation approaches must account for anthropogenic activities, especially given recent global soil management attempts to increase organic carbon, productivity, and GHG emissions (Della Chiesa et al., 2019; Gatica et al., 2020). Crop residue burning in North and Central India, especially rice and wheat, causes air pollution and GHG emissions (Golchin and Misaghi, 2024; Shakoor et al., 2022; Singh et al., 2020). Sustainable alternatives include returning residues to the soil to alleviate environmental issues. This recycles nutrients, promotes soil health, and retains water (Shakoor et al., 2022; Singh et al., 2020; Singh et al., 2014). While improving soil organic matter and crop nutrition may also increase GHG emissions such as NOx, CH₄, and CO₂ (Lenka et al., 2021; Lenka et al., 2022; Abalos et al., 2022; Li et al., 2022). Several theories have been proposed to explain responses of GHG fluxes caused by the combined inputs of mineral nutrients and residue, including changes in soil mineral nitrogen (nitrate N, NO3-N and ammoniacal N, NH4-N) (Fang et al., 2018; Li et al., 2021a), soil labile C, extracellular enzyme activity, especially alkaline phosphatase, microbial biomass carbon (Lenka et al., 2022), microbial nutrient mining, and microbial stoichiometry decomposition (Fang et al., 2018; Li et al., 2021a; Singh et al., 2024). Adding crop residues provides a source of organic carbon that can stimulate microbial activity in the soil (Li et al., 2018). This, in turn, can lead to increased decomposition rates of both the added residues and native soil organic matter (Lenka et al., 2019), potentially influencing GHG fluxes (CO2, CH4, and N2O) due to the increased mineralization and bio-geochemical processes regulating GHG fluxes (Fontaine et al., 2004; Li et al., 2021b). High residue and zero/low nutrient input create C-rich and nutrient-poor systems, leading to nutrient mining and immobilization of NO3-N and NH4-N due to microbial demand for nitrogen during decomposition (Fang et al., 2018; Lenka et al., 2022; Liu et al., 2023a), leading to fluctuations in their availability for processes driving GHG fluxes (denitrification/methanogenesis/methanotrophy). Contrarily, the combined application of mineral nutrients (N and P) along with C-rich residues may stimulate the growth and activity of microbial communities, with changes in microbial C: N: P stoichiometry during decomposition while expediting the fate of added residue C in stable pools of SOM with a constant C-to-nutrient ratio (Fang et al., 2019). Increased residue and nutrient application rates elevate nitrate (NO3-N) and ammoniacal nitrogen (NH4-N) levels, enhancing soil fertility (Singh et al., 2020). Crop residues contribute to higher labile carbon, which boosts microbial activity and nutrient cycling. That, in turn, promotes the production of enzymes like alkaline phosphatase, aiding phosphorus availability (Yang et al., 2021). Additionally, increased organic matter from residues raises MBC, further supporting microbial processes and soil health (Joshi et al., 2024; Ren et al., 2024; Singh et al., 2024; Wang C et al., 2021). Furthermore, although there have been a number of studies on the magnitude of soil GHG fluxes, the relative effect of source effect (anthropogenic vs natural) underlying GHG fluxes in response to varied input levels of crop residues and mineral nutrients (anthropogenic sources) under different soil types (natural effect) is still unclear and limited.
One of the important aspects of this study is the use of advanced statistical analysis and machine learning to disentangle the relative contributions of various factors to GHG emissions. Machine learning offers powerful tools for handling the complexity and variability inherent in agricultural systems (Joshi et al., 2024; Xu et al., 2024), allowing for the identification of patterns and relationships that may not be apparent through traditional statistical methods (Magazzino et al., 2024). By leveraging these techniques in our study, we hope to provide more accurate predictions of GHG emissions under different management scenarios, which can be used to guide policy and practice in agriculture. This study hypothesizes that greenhouse gas emissions and carbon mineralization are primarily driven by the interaction of residue and nutrient management with soil type, with anthropogenic factors playing a larger role than natural factors and specific soil and microbial properties serving as key predictors of these emissions. Our study addresses this need by investigating the effects of varying levels of crop residue and nutrient inputs on GHG emissions and carbon mineralization across three contrasting soil types (Vertisol, Alfisol, and Incetisol) in India. The objectives were to (1) assess the impact of different residue and nutrient management practices on GHG emissions and carbon mineralization, (2) quantify the relative contributions of natural and anthropogenic factors to GHG emissions using machine learning techniques, and (3) identify the predominant predictor variables that influence GHG emissions in residue-returned soils. To achieve the objectives of the study, we employed a combination of laboratory-based incubation experiments and advanced data analysis methods to analyze the complex interactions between soil properties, residue inputs, and nutrient management practices.
The surface soils were collected from 0 to 15 cm soil depth from three predominant soil types of India according to the USDA soil taxonomy 1) Alfisol (mixed hyperthermic VerticHaplustalfs) was collected from the farmer’s field (21.96°N latitude and 77.74°E longitude) in Betul district of Madhya Pradesh under maize-fallow cropping sequence, 2) Inceptisol (mixed hyperthermic Typic Haplustept) was collected from the farmer’s field (26.41°N latitude and 80.23°E longitude) in Kanpur district of Uttar Pradesh under rice-wheat cropping system, and 3) Vertisol (Isohyperthermic Typic Haplustert) was taken from the experimental farm (23.31°N latitude and 77.41°E longitude) of ICAR-Indian Institute of Soil Science under soybean-wheat cropping system. In the laboratory, air-dried soils, after carefully removing the recognizable gravels and debris (≥2 mm), were passed through a 2 mm sieve by gently breaking the clods along planes of weakness by hand, thus preserving soil aggregation. The wheat crop residue was collected after the harvest of wheat from an experimental plot under a long-term soybean-wheat cropping system at a recommended dose of fertilizer in the research farm of the Indian Institute of Soil Science. For the residue treatment, the wheat residues were pulverized after air drying and sieved to a size of 2 mm. An elemental analyzer (NC analyzer, Thermofisher, Flash 2,000 model) was used to determine total C and N concentrations in soil and residues. We used sulphuric acid-perchloric acid digestion and molybdenum antimony colorimetric estimation (Page et al., 1982) to determine total P concentration in soil and residue. The basic properties of soil and wheat residues are presented in Table 1.
Soil microcosms were prepared by taking 40 g of soil in a 100 mL beaker placed inside 461 mL glass jars with septum-fitted lids for gas sampling. After a 10-day pre-incubation at 70% moisture and room temperature, the experiment was conducted with varying residue and nutrient inputs at 80% field capacity. Wheat residues were applied at four levels: 0, 90, 180, and 270 mg (dry weight), which was equivalent to 0 (WR0), 5 (WR5), 10 (WR10), and 15 (WR15) Mg ha−1, respectively. The crop residues (<2 mm) were completely mixed with soil (<2 mm) for incubation based on a 10 cm incorporation depth in the field. After residue addition, the nutrient solution was added to all the WR treatments at three levels: 1) NP0: no nutrient, 2) NP1, nutrients (N and P) were added to balance the residue C/nutrient stoichiometry of C/N/P= 100: 8.3: 2.0 (Fang et al., 2020) to achieve 30% stabilization of added residue C input at 5 Mg/ha (R5), and 3) NP2 = 3 × NP1. Therefore, the nutrient addition altered nutrient stoichiometry in WR treatments, and the achieved stoichiometry in each treatment combination is shown in Supplementary Table S1. Nutrient solutions corresponding to nutrient levels were prepared using AR grade urea and potassium dihydrogen phosphate, and 10 M sodium hydroxide solution was used to modify the pH of the nutrient solution to 7. All treatments were replicated three times and incubated at 30°C incubation temperature at 80% FC moisture content. The incubation temperature was selected because the aboveground mean annual surface temperature is ca. 30°C in sub-tropical and semi-arid regions during different crop-growing seasons (Lenka et al., 2022). Triplicate empty glass jars were also incubated to account for the headspace GHGs. Field capacity (FC) was measured at matric potentials of −33 kPa using sieved (<2 mm) soil samples in pressure plate extractors from Soil Moisture Equipment Corp., Santa Barbara, CA, with FC moisture content at 0.14 m3m−3. Soil moisture was maintained through regular weighing and water addition to compensate for evaporation losses during gas sampling intervals.
The GHG fluxes from different treatments were measured in gas chromatography (Agilent Technologies model 7890A). Headspace gases were drawn from the incubation jars using a syringe and immediately transferred to a 10 mL evacuated glass at frequent intervals for 96 days of incubation. The CH4/CO2/N2O flux rate was calculated as the change in headspace N2O/CH4/CO2 concentration using the ideal gas law and molecular weight. Cumulative N2O, CH4, and CO2 emissions were determined by linear integration of daily fluxes. The global warming potential of CO2-equivalent was calculated by multiplying the cumulative N2O and CH4 emissions by their respective radiative forcing potentials using the following equation (Singh et al., 2024):
GWP (mg CO2 eq. kg−1 soil) = CH4 (mg kg−1 soil) × 27.2 + N2O (mg kg−1 soil) × 273 + CO2 (mg kg−1 soil) × 1
After the incubation period of 96 days, soil samples were analyzed for relevant soil properties such as soil minerals nitrogen (NO3-N and NH4-N), alkaline phosphatase, soil microbial biomass carbon (SMBC), and labile SOC following standard analytical procedures. The moisture content was determined gravimetrically using the oven-dry method in a part of the moist composite soil samples. Soil mineral nitrogen was estimated after extraction in 2M KCl, and subsequent analysis employed standard methods (Kempers, 1974). Labile SOC calculations utilized the potassium permanganate oxidation method (Blair et al., 1995; Islam et al., 2003) and measured alkaline phosphatase (alk-P) as an indicator of P demand (Alef and Nannipieri, 1995). Soil MBC was determined by a fumigation-extraction method, and a conversion factor of 0.45 was applied to determine MBC (Joergensen and Brookes, 1990).
All data underwent tests for normality and homogeneity of variance, with transformations applied as needed. Statistical analyses were performed using SPSS software (version 21.0, SPSS Inc., Chicago, IL, United States), setting a significance threshold of p = 0.05. The general linear model univariate ANOVA was employed, followed by Tukey’s HSD test for multiple comparisons of means. The relative effect of management variables (residue and nutrient input, soil moisture), post-harvest soil parameters (NO3-N, NH4-N, MBC, labile C, and alk-P) and inherited soil parameters (pH, CEC, clay, sand, silt, TC, TN, legacy P and K) on soil GHG emission (CO2/CH4/N2O) was evaluated using Pearson correlation (two-tailed significance) and partial least squares (PLS) regression models. The variable influence on projection (VIP) score of value >0.8 (Gómez-Gener et al., 2018) was used to identify the potential drivers of soil GHG emission. The PLS model fitting and cross-validation details are presented in the supplementary file. The model performance was analyzed using the root mean square error (RMSE), adjusted (R2) coefficient of determination, and p-value between observed and predicted values due to their robustness, simplicity, and widespread use. Finally, the effect size (VIP scores) were grouped into two broad categories: anthropogenic (WR input, the stoichiometry of C: N, C: P, N input, MBC, NO3-N, NH4-N, SMBC, labile C, alk- P) and natural drivers (pH, CEC, clay, sand, silt, TC, TN, legacy P, and K). All the graphs were constructed using Origin Pro, version 2024b (Origin Lab Corporation, Northampton, MA, United States).
Total C mineralization was significantly influenced by the main effects of soil type, wheat residue (WR) input, and the interactive effect of soil type and WR only (Supplementary Table S2; Figure 1). The total cumulative C mineralization ranged from 416.02 mg C kg−1 soil (WR15 + NP2 in Alfisol) to 27.33 mg C kg−1 soil in treatment WR0 + NP0 in Inceptisol over 96 days of incubation (Table 2). Averaged across nutrient and residue input, C mineralization was significantly the highest in Alfisol, 1.3 times Vertisol, and 1.4 times Inceptisol; however, the effect of Vertisol was comparable to Inceptisol. Wheat residue input significantly enhanced total cumulative C mineralization; the order was WR15 (317.46 mg C kg−1) > WR10 (204.85 mg C kg−1) > WR5 (118.67 mg C kg−1) > WR0 (41.80 mg C kg−1) averaged across nutrient input and soil types. High nutrient input increased the total cumulative C mineralization, but the effect was comparable to NP0 across soil type and WR input.
Figure 1. Effect of wheat residue (WR) input and soil type (Vertisol: Vert; Alfisol: Alf; Inceptisol: Incept) on total cumulative C mineralization (mg C kg−1 soil) averaged across nutrient input over 96 days of incubation. Vertical bars represent the mean ± standard error (n = 3). Different lower-case letters indicate significant differences among treatments at α < 0.05.
Table 2. Effect of varied wheat residue and nutrient input on cumulative soil GHG emission (CO2, CH4, and N2O) and global warming potential under different soil types over 96 days of incubation.
The cumulative N2O flux was significantly influenced by the main factors of wheat residue input, nutrient levels, and soil type (Table 2; Supplementary Table S2). Unlike total C mineralization, there was a significant three-factor interaction of wheat residue, nutrients, and soil type on the cumulative N2O flux over 96 days of incubation. For example, nutrient input (NP2, C/N/P = 100:8.3:2.0) had the highest N2O flux of 128.41 μg N kg−1 soil in the high-residue (WR15) input treatment Vertisol compared to the lowest value (−20.82 μg N kg−1 soil) in WR10 + NP0 (C/N/P > 100:8.3:2.0) under Inceptisol (Table 2; Figure 3). The negative flux indicates N2O consumption. The cumulative N2O flux over the 96-day incubation period was similar in the Alfisol (17.60 μg N kg−1 soil) and Vertisol (15.53 μg N kg−1 soil) across residue and nutrient input levels. Nutrient (NP) input significantly enhanced the cumulative N2O flux; the trend was NP2 > NP1 > NP0. However, the effect of wheat residue was inconsistent with WR15 ≈ WR0 > WR5 ≈ WR10 across nutrient input and soil type, indicating a nonlinear response of cumulative N2O emission with WR rate (Figure 2; Supplementary Figure S2). The negative N2O flux of cumulative N2O emission in Inceptisol at all WR levels (cf. WR0) indicates the sink capacity of the soil (Figure 3; Supplementary Figure S2).
Figure 2. A sunburst chart illustrating the percent share effect of wheat residue input (WR0, WR5, WR10, and WR15) and soil type (Vertisol: Vert; Alfisol: Alf; Inceptisol: Incept) on total cumulative N2O flux (µg N kg−1 soil) over 96 days of incubation.
Figure 3. Effect of wheat residue (WR0, WR5, WR10, and WR15) and nutrient (NP0, NP1, and NP2) input on total cumulative N2O flux (µg N kg−1 soil) under three soil types (Vertisol: Vert; Alfisol: Alf; Inceptisol: Incept) over 96 days of incubation. Vertical bars represent the mean ± standard error (n = 3). Different lower-case letters indicate significant differences among treatments at α < 0.05.
High input of wheat residue (WR15 and WR10) significantly decreased cumulative CH4 flux compared with WR0 and WR5, the order being WR5 (6.86 μg C kg−1 soil) > WR10 (2.99 μg C kg−1 soil) ≈ WR15 (0.13 μg C kg−1 soil) ≈ WR0 (0.05 μg C kg−1 soil) across nutrient management and soil type. The main factors of soil type and residue input significantly influenced the cumulative CH4 flux (Supplementary Table S2). In addition, a significant interaction of WR × soil type occurred; that is, high residue input (WR10 and WR15) only decreased cumulative CH4 flux in Vertisol and Inceptisol (Figure 4A). However, in Alfisol, cumulative CH4 flux increased with WR input to WR10 (@10 Mg/ha) and then declined at WR15. Significant interaction effect of soil type x NP (p = 0.053) showed different responses of soil type to increment in nutrient input (Supplementary Table S2); the response was linear in Alfisol, curvilinear in Vertisol, and nonlinear in Inceptisol (Figure 4B), across residue input. The cumulative CH4 flux ranged from 23.42 μg C kg−1 soil WR5 + NP0 (C/N/P > 100: 8.3: 2.0) in Inceptisol to −6.64 μg C kg−1 in treatment WR15 + NP0 (C/N/P > 100: 8.3: 2.0) under Vertisol, negative values indicate CH4 consumption from ambient (Table 2).
Figure 4. Interactive effect of (A) wheat residue (WR0, WR5, WR10, and WR15) and soil type (Vertisol: Vert; Alfisol: Alf; Inceptisol: Incept); (B) soil type and nutrient input (NP0, NP1, and NP2) on total cumulative CH4 flux (µg C kg−1 soil) over 96 days of incubation. Vertical bars represent the mean ± standard error (n = 3). Different lower-case letters indicate significant differences among treatments at α < 0.05.
The cumulative GWP was significantly the highest in the Alfisol, 1.2 times Vertisol, and 1.4 times Inceptisol across residues and nutrient inputs (Figure 5; Supplementary Figure S3). GWP increased significantly (p < 0.001) with the residue input in all soil types (Figure 5), and the trend followed the order WR15 (1,142.9 mg CO2-C eq. kg−1 soil) > WR10 (769.1 mg CO2-C eq. kg−1 soil) > WR5 (474.5 mg CO2-C eq. kg−1 soil) > WR0 (154.8 mg CO2-C eq. kg−1 soil) (Supplementary Figure S3). The interactive effect of WR × soil type x NP was significant (p = 0.047), and WR x soil type (p = 0.007) on cumulative GWP over 96 days of incubation (Supplementary Table S2). Therefore, the highest interactive effect was in the treatment WR15 (@15 Mg/ha) + NP2 (C/N/P = 100: 8.3: 2.0) in Alfisol (1,489.76 mg CO2-C eq. kg−1 soil) and the lowest in WR0 (no residue) + NP1 (C/N/P < 100: 8.3: 2.0) in Inceptisol (96.70 mg CO2-C eq. kg−1 soil) (Table 2).
Figure 5. Radar graph illustrating the effect of (a) wheat residue (WR0, WR5, WR10, and WR15) and soil type (Vertisol: Vert; Alfisol: Alf; Inceptisol: Incept) averaged across nutrient input on total cumulative CH4 flux (µg C kg−1 soil) over 96 days of incubation. The details of the statistical analysis are given in Table 1 and shown in Supplementary Figure S3.
The active soil organic carbon pool was measured as labile C and microbial biomass carbon (MBC). There was a significant interaction (p = 0.015) of wheat residue, nutrients, and soil type on the labile C after 96 days of incubation. The input of high residue (WR15) levels in Alfisol at NP1 and NP2 (528.87 mg C kg−1 soil) showed the highest labile C compared with zero residues (WR0) in Inceptisol at NP0 (142.26 mg C kg−1 soil) (Table 3). Nutrient input usually had no impact on labile C (Supplementary Table S2); however, the interaction of residue input and soil type was significant (Figure 6); that is, high residue input (WR15) in Alfisol significantly increased the labile C compared with Inceptisol and Vertisol, Alfisol was higher by 2.27 and 2.28 times compared with Inceptisol and Vertisol, respectively. Residue input increased the labile C with the effect of WR15 comparable to WR10 and WR5 comparable to WR0.
Table 3. Effect of varied wheat residue and nutrient input on relevant post incubation soil properties under different soil types over 96 days of incubation.
Figure 6. Interactive effect of wheat residue and soil type on (A) labile C (mg C kg−1 soil) and (B) microbial biomass carbon (MBC), (C) soil type, and nutrient on microbial biomass carbon (MBC, mg C kg−1 soil) over 96 days of incubation. Vertical bars represent the mean ± standard error (n = 3). Different lower-case letters indicate significant differences among treatments at α < 0.05.
Microbial biomass C was significantly higher in the Vertisol than in Alfisol and Inceptisol and increased with residue and nutrient input (Figure 6; Table 3). However, the effect of residue levels (cf. WR0) was comparable in all three soil types (Figure 6B). Except Vertisol wheat residue input (cf. WR0) did not significantly increase the MBC in the other two soil types (Inceptisol and Alfisol). The highest effect was observed in treatment receiving WR10 NP0 (C/N/P > 100: 8.3: 2.0) in Vertisol (895.42 mg C kg−1 soil) and the lowest in WR10 NP1 (C/N/P > 100: 8.3: 2.0) in Inceptisol (79.18 mg C kg−1 soil) because of the significant interaction between soil type, WR and NP (Supplementary Table S2). There were no differences in MBC between nutrient inputs in all soil types; however, the significant interaction between soil type and nutrient input showed the highest MBC in Vertisol (cf. Inceptisol) at all nutrient levels (Figure 6C).
The soil nitrate (NO3-N) and ammonical N (NH4-N) account for mineral N. The main factors and interaction effects of soil type, residue, and nutrient input were significant except for WR × NP (Supplementary Table S2). The NO3-N ranged from 110.57 mg N kg−1 soil (WR0 + NP2 in Vertisol) to 6.80 mg N kg−1 soil (WR15 + NP0 in Alfisol) (Table 3). High residue and no nutrient input decreased the NO3-N concentration in all soil types (Supplementary Figure S4A). Among the soil types, Vertisol had 1.4 times higher NO3-N than Inceptisol and 2.4 times Alfisol (Supplementary Figure S4B). Similarly, NH4-N significantly responded to the interactive effect of residue and nutrient input and soil types (p < 0.0001) (Supplementary Table S2); that is, high residue input (WR15) in Vertisol at nutrient level NP2 and NP1 showed the highest concentration among all treatments compared to low residue input (WR0 and WR5) in Inceptisol at nutrient level NP0 and NP1 (Table 3), the mean values ranged from 1.63 to 104.89 mg N kg−1 soil. Similar to NO3-N, Vertisol had 2.8 times higher NH4-N than Inceptisol and 4.1 times Alfisol (Supplementary Figure S5).
Alkaline phosphatase (Alk-P) was estimated in this study because it is an essential extracellular enzyme that responds to different levels of residues and nutrient management, influencing nutrient stoichiometry C/N/P. Across soil types, residue input (@ 5, 10, and 15 Mg/ha) significantly increased the Alk-P compared with WR0; similarly, nutrient input (NP1 and NP2) showed a positive effect compared with NP0. There was a significant interaction of soil type, residue, and nutrient input (Table 3; Supplementary Table S2), with values ranging from 450.51 to 17.88 mg P-nitrophenol kg−1 soil h−1. Among soil types averaged across nutrient and residue input, Inceptisol showed the lowest Alk-P activity, 3.8 and 3.1 times less than Alfisol and Vertisol, respectively (Supplementary Figure S6).
The Spearman correlation analysis was carried out to explore the relationship between GHG emissions and the PLS model predictor variables. Pearson correlation (Supplementary Figure S7) between N2O and inherited soil properties showed a significant negative correlation (pH, available P, and a positive correlation with clay. Additionally, the correlation was very strong between N2O flux and the acquired soil properties due to soil management (MBC, NO3-N, Alk-P) with a positive correlation. Nutrient input shifting the nutrient stoichiometry (C: N and C: P) negatively correlated with N2O emission. However, varied nitrogen levels through nutrient input were positive on N2O.
However, fluxes of CH4 were negatively correlated with inherited soil properties (soil organic C, total N, available K, clay, and silt + clay) and negatively with sand and available P. MBC, NH4-N, and alk-P negatively affected the CH4 fluxes among the acquired soil properties. No significant correlation was observed between CH4 fluxes and residue and nutrient input. Soil CO2 fluxes were positively correlated with management factors (nutrient and residue input), the acquired soil properties (MBC, alk-P, and labile C), and negatively with NO3-N. However, only the soil pH negatively correlated with CO2 fluxes among the inherited soil properties.
Variable importance in projection (VIP) was used in the variable selection method that calculated scores to summarize the influence of individual variables on a PLS model. The PLS model for N2O emissions extracted nine latent factors from the data matrix that explained 99.26% of the cumulative predictor (X) variance and 59.18% of N2O (Y) responses (Supplementary Table S3). The PLS model extracted only 5 and 6 latent factors to estimate CH4 and CO2, respectively (Supplementary Tables S4, S5). The diagnostic plot (Supplementary Figure S8) and the relative importance of predictor variables (Figure 7) in the partial least square (PLS) model indicated that N2O emission was more responsive (values > 0.8) to management variables (nutrient input) and acquired soil properties (alk-P, NO3-N, MBC, labile C) than inherited soil properties. The ranking of the predictor variables (values > 0.8) important for the projection of N2O fluxes was as follows: alk-P > NO3-N > N input > C: N > C: P > inherited P > MBC > clay > inherited pH > labile C (Figure 7). The ranking of the predictor variables (values > 0.8) essential for the projection of CH4 fluxes was as follows: inherited soil P > clay > silt + clay > sand > inherited soil K > inherited soil N > MBC > NH4-N > inherited SOC > Alk-P > NO3-N > labile C > C: P. Similarly the relative variable importance for CO2 flux was WR > N input > labile C > NO3-N > alk-P > MBC > C: N > inherited soil pH (Figure 7). Indicating anthropogenic management (residue and nutrient input) factors and acquired soil properties from varied soil management influenced soil N2O and CO2 fluxes more than CH4 emission. The PLS model performance was significant (p < 0.001) for the estimation of all three GHGs; however, the model was most effective for the estimation of CO2 (r2 = 0.88), followed by N2O (r2 = 0.59) and CH4 (r2 = 0.35) (Supplementary Figures S8, S9, S10).
Figure 7. The relative importance of variables in regulating cumulative (A) N2O fluxes, (B) CH4 fluxes, (C) CO2 fluxes, and (D) the effect of anthropogenic versus natural drivers on GHG emissions. NO3-N: Nitrate N; NH4-N: ammoniacal N; alk-P: alkaline phosphatase; MBC: microbial biomass carbon; WR rate: wheat residue return rate; C: N: stoichiometry of residue C to N input/soil C to N; C: P: stoichiometry of residue C to P input/soil C to P.
Non-CO2 (N2O and CH4) GHG production and consumption in response to various soil management activities (anthropogenic drivers) indicated C and N turnover (Lenka et al., 2019; Chaves et al., 2021; Abalos et al., 2022; Li et al., 2022) in soil and its feedback to climate change. The results support our hypothesis that soil type (inherited soil properties) significantly regulated the response of GHG fluxes to varying residue and nutrient input. However, anthropogenic drivers (cf. natural) were the key determinant of total carbon mineralization (measured as CO2 fluxes) and N2O emissions only. Further, our study provides evidence that the predominant drivers varied across the GHGs, likely due to the availability of NO3-N and labile C in the soils incorporated with the crop residue, which explains the magnitude of GHGs and their correlation with key microbial variables (e.g., MBC, alkaline phosphatase activity, and nutrient stoichiometry).
The results of this study demonstrated that crop residue input was one of the major drivers of total carbon (C) mineralization (CO2 flux) in all three soils (Fang et al., 2018; Li et al., 2018), with the highest residue application (WR15) resulting in the most significant increase in carbon release. The order of C mineralization (WR15 > WR10 > WR5 > WR0) across all soil types highlights the direct relationship between organic matter availability and microbial decomposition processes (Li et al., 2021a). The additional carbon from increasing levels of wheat residue input likely provided a readily accessible energy source for soil microbes, accelerating the mineralization process. This observation is consistent with the substrate-induced respiration theory, where adding organic residues stimulates microbial activity, leading to increased carbon turnover (Fontaine et al., 2004; Lenka et al., 2019; Liu et al., 2023a). The accelerated C mineralization could be further attributed to crop residue-induced soil carbon (C) priming by stimulating microbial activity, which alters the decomposition of native soil organic carbon (SOC) (Lenka et al., 2019; Lenka et al., 2021; Salehin et al., 2024). This priming could be higher in high residue input (cf. low residue) (Fang et al., 2018). While nutrient input generally increased C mineralization, the effect was relatively minor compared to the influence of soil type and residue input. In soils with adequate nutrient levels (Ponce-Mendoza et al., 2010), additional nutrient inputs may not significantly enhance microbial activity beyond a certain threshold (Conde et al., 2005). The comparable effect of high nutrient input (NP2) to the NP0 treatment across soil types and residue levels suggests that nutrient availability was not the primary limiting factor for C mineralization in this study (Ma et al., 2020).
Instead, the physical and chemical properties of the soil and the quantity of organic residue appeared to play more critical roles. These findings align with previous research that highlights the importance of soil types and residue C inputs in driving microbial activity and subsequent carbon mineralization rates (Fang et al., 2019; Lenka et al., 2019; Liu et al., 2023b; Singh et al., 2024). The addition of wheat residue (15 Mg/ha) with nutrient input (NP2) led to higher cumulative C mineralization in Alfisol compared to Inceptiosl in treatment WR0 plus NP0 over the incubation period, likely because (i) nutrient input improved the C: N imbalance of soil microorganisms due to the high C: N ratio of wheat residue input (79.54: 1.00), thus eliminating the N limitation of microorganisms (Fang et al., 2020; Song et al., 2022) as a result, microorganisms in NP2 increased C mineralization compared to NP0, which was used to satisfy energy requirements when accessing nutrients. (ii) The lower pH (5.70) and high sand content (53%) (Table 1) in Alfisol accelerated the use of total C (residue plus soil) by microorganisms by enhancing their activity, driven by better soil structure, aeration, and nutrient availability (Song et al., 2022). The result was also supported by higher labile C (Table 3) in Alfisol receiving high residue and nutrient input. The strong positive correlation between C mineralization (CO2 fluxes) and labile C (Supplementary Figure S7) provided the required energy source for higher microbial activity (Fang et al., 2020; Lenka et al., 2022) compared to Vertisol and Inceptisol. Our results demonstrated that Alfisols having higher sand content (Table 1) have greater macropores and oxygen supply (Pathak et al., 2013; Chandrakala et al., 2021), promoting more active microbial communities that can efficiently decompose organic residues. In contrast, the lower mineralization rates observed in Vertisols and Inceptisols may be due to their higher clay content, which can limit microbial access to organic substrates by physically protecting organic matter within soil aggregates (Chowdhury et al., 2021; Lenka et al., 2021; Ren et al., 2024). This significant interaction of WR and soil types indicated the inherent properties of the soil modulate the effectiveness of residue inputs in promoting C mineralization. In soils with favorable conditions (e.g., Alfisols), residue additions can lead to a marked increase in microbial activity and carbon mineralization. However, in soils with less favorable conditions (e.g., Inceptisols), the same residue inputs may not be as effective due to limitations in microbial activity or substrate accessibility (Lenka et al., 2019). The partial least square regression analysis demonstrated that anthropogenic management practices (residue and nutrient inputs) and acquired soil properties substantially impact carbon mineralization (CO2 fluxes) compared to natural soil factors. The PLS analysis indicated that wheat residue input (WR) and nutrient input were the most influential variables, followed by labile carbon, NO3-N, and microbial biomass carbon (MBC). The model shows that anthropogenic factors account for 72% of the variance in C mineralization. In contrast, natural factors, such as soil type, contribute only 28% (Figure 7). This finding underscores the critical role of targeted management practices in regulating soil carbon dynamics (Fontaine et al., 2004), highlighting the potential to optimize carbon mineralization through appropriate residue and nutrient management strategies considering the basic soil characteristics.
Our experiment showed that Vertisol (cf. Incetisol) exhibited a significant increase in denitrification activity when higher levels of residue (WR15) and nutrients (NP2) were applied (Table 2; Figure 2). This could be attributed to i) the enhanced availability of substrates for microbial nitrification and denitrification processes (Abalos et al., 2022; Lenka et al., 2022) ii) the high clay content (Table 1) in Vertisol (Gebremichael et al., 2022) iii) enhanced microbial biomass carbon (MBC) and nitrate (NO3-N) concentrations (Table 3) (Li et al., 2021a), iv) high soil pH (Khalifah and Foltz, 2024), and less available soil P (Gebremichael et al., 2022) (Table 1) and v) high alkaline phosphatase activity (Yang et al., 2021). The presence of smectite and vermiculite clay types in Vertisols (Rakhsh et al., 2017; Fang et al., 2018; Fang et al., 2019) is related to higher mineral-associated carbon (Fang et al., 2019), creating an ideal environment for the processes of ammonification and nitrification, resulting in high concentrations of NH4-N, NO3-N, and N2O losses compared to Inceptisol. Further, Inceptisol demonstrated negative N2O flux, indicating consumption, possibly due to a significant amount of legacy phosphorus (19.7 mg kg-1), a high soil pH (8.49), and less clay content (13%) compared to Vertisol and Alfisol soils (Table 1). These characteristics of Inceptisol contributed to reduced microbial activity, as evidenced by lower levels of MBC and alkaline phosphatase. The results supported the previous finding of the association of soil legacy P limitation on enhanced N2O emission (Li et al., 2021a; Gebremichael et al., 2022) in Vertisol (cf. Inceptisol). The addition of nutrients (N and P) changed the stoichiometry of nutrients (carbon/nitrogen/phosphorus), eliminating the P limitation, with increased P levels leading to lower emissions of N2O, emphasizing the importance of nutrient management in controlling greenhouse gas release. However, the dominant influence of nitrogen over phosphorus on N2O production plays a crucial role in determining the overall impact of phosphorus when residues and NP nutrients are applied together. Because the relative effect of soil legacy P estimated through PLS model analysis was lesser than soil NO3-N and N input (Figure 7). Excessive residue without sufficient nutrient input resulted in nitrogen immobilization (Table 3). This immobilization led to a decrease in N2O emissions. Conversely, when nutrient inputs were balanced, it stimulated microbial activity, resulting in increased emissions. The results of our study support the conclusions of previous researchers that maintaining a high ratio of carbon, nitrogen, and phosphorus (C: N: P) in the soil promoted the growth and activity of microbial biomass, resulting in the conversion of nitrogen from its mineral form to its organic form, which reduced the risk of nitrogen losses through denitrification and leaching (Lenka et al., 2019; Lenka et al., 2022; Li et al., 2021a; Li et al., 2021b). Consequently, a residue input will preserve the nitrogen (N) in its organic state, making it gradually accessible for microbial and plant development. The nonlinear relationship between N2O fluxes and residue input indicated the interaction of carbon and nitrogen availability, soil microbial activity, and the physical characteristics of the soil (Fang et al., 2020; Singh et al., 2024; Xu et al., 2024). The observed interaction between residue and nutrient input with soil type suggests that the impact of residue carbon input on reducing soil N2O emissions was influenced by both the C/N/P stoichiometry and the fundamental soil properties, such as clay percentage, pH, and phosphorus content (Supplementary Figure S7). The strong link between N2O emissions and acquired soil parameters, such as MBC, NO3-N, and alkaline phosphatase (Alk-P), further highlighted the crucial role of soil management in affecting N2O fluxes.
The PLS regression analysis reaffirmed our findings and offered valuable insights into the variables that affect soil nitrous oxide (N2O) emissions (Figure 7; Supplementary Figure S8) (Shah et al., 2024; Xu et al., 2024). The PLS model, which accounted for a significant amount of the variation in N2O emissions, emphasized the influential impact of management factors and acquired soil qualities compared to inherent soil characteristics. The elevated VIP scores for parameters such as alkaline phosphatase (alk-P), nitrate-nitrogen (NO3-N), nitrogen input, and MBC suggested that these factors drive N2O emissions. The high ranking of alk-P indicates that nutritional stoichiometry, specifically the availability of phosphorus (Li et al., 2021a; Gebremichael et al., 2022), substantially influences the microbial activities that produce N2O fluxes. This is reinforced by the significance of NO3-N, which plays a direct role in nitrification and denitrification, the primary biological processes responsible for N2O production (Weitz et al., 2001; Khalifah and Foltz, 2024). Consequently, the presence of limited phosphorus in Vertisols (3.7 mg kg−1) and Alfisol (8.5 mg kg-1) (compared to Incetisol: 19.7 mg kg−1) and the high concentration of nitrate nitrogen (NO3-N) greatly increased the emission of nitrous oxide (N2O) in both soils (Figure 2). The importance of nitrogen input has been identified as another crucial component, emphasizing how the management of nutrients directly impacts the release of N2O by influencing nitrogen availability in the soil (Wang C. et al., 2021; Lenka et al., 2022; Singh et al., 2024).
The model also recognized the importance of nutrient stoichiometry (C: N and C: P), essential for maintaining a balance between carbon and nitrogen cycling. This stoichiometry directly affects the efficiency of N2O production or reduction processes (Liu et al., 2021; Lenka et al., 2022; Song et al., 2022). The VIP scores indicated that the influence of inherited soil properties, such as clay content and soil pH, on N2O emissions was lesser than the impact of management practices and the consequently acquired soil attributes. The relative contribution of the anthropogenic drivers is more significant than natural, with a share of 62% of the total N2O variance in the PLS model. Our study indicated that the effects of soil type on N2O emissions could be counteracted by effective management strategies, including those that promote microbial activity and increase nutrient availability. Optimizing residue and nutrient management strategies will be crucial in efficiently reducing N2O emissions in various soil types.
Higher organic C and N availability from high residue input (WR10 and WR15) likely suppressed methanogenesis and enhanced oxidation of CH4, resulting in CH4 consumption (Choudhary et al., 2024). This suggestion is consistent with a substantial reduction in the cumulative CH4 flux in high wheat residue (WR10 and WR15) compared to low residue inputs (WR0 and WR5). The effect was more pronounced in Vertisol, followed by Alfisol and Inceptisol. The negative correlation (though insignificant) between CO2 and CH4 emissions (Supplementary Figure S7) aligned with the previous finding of a positive relationship between CH4 uptake and high CO2 emission in the upland ecosystems (Li et al., 2022; Wu et al., 2024). Across soils, the nonlinear response of CH4 emissions to residue input suggested that while low and moderate residue input might stimulate CH4 production, the excessive residue could lead to a saturation point or shift in the microbial activity that reduces CH4 emissions (Wang X. D. et al., 2021; Singh et al., 2024). Additionally, the Pearsons correlation analysis also found that CH4 fluxes were negatively correlated (Supplementary Figure S7) with several inherited (legacy) soil properties, such as soil organic carbon, total nitrogen, available potassium, and clay content, indicating a greater edaphic (natural) control on CH4 emission/uptake. The Vertisol was rich in clay content compared with Alfisol and Inceptisol (Table 1) and low in legacy P, showing significantly higher cumulative CH4 uptake over the incubation period (Figure 4) (Cui et al., 2023; Shumba et al., 2023). Among the acquired properties induced by varied residue and nutrient input, MBC, NH4-N, and alkaline phosphatase negatively correlated with CH4 fluxes. Our study observed high residue input (WR10 and WR15) resulted in higher MBC, NH4-N, and alkaline phosphatase activity related to less CH4 fluxes (high CH4 oxidation) in the treatments (Lai et al., 2017; Fang et al., 2018; Yang et al., 2021; Shah et al., 2024). The correlations analysis suggested that inherent soil characteristics and management-induced changes can significantly influence CH4 emissions. Notably, there was no significant correlation between CH4 fluxes and direct residue or nutrient inputs, suggesting that the impact of these management practices on CH4 emissions is mediated through changes in soil properties rather than direct effects. Moreover, the interaction between soil type and nutrient input (p = 0.053) revealed that soil types respond differently to increments in nutrient input across varying residue levels (Nguyen et al., 2013; Liu et al., 2021; Singh et al., 2024). Alfisol exhibited a linear response, Vertisol a curvilinear response, and Inceptisol a nonlinear response to nutrient additions, highlighting the complexity of nutrient-soil interactions and their influence on CH4 fluxes. Overall, our results suggest that Vertsiols were CH4 sinks (−2.33 μg C kg−1 soil) compared to Alfisol (2.70 μg C kg−1 soil) and Inceptiosl (7.16 μg C kg−1 soil) across varied levels of residue and nutrient input. As estimated through the PLS model analysis (Joshi et al., 2024), the relative effect size of the studied variables on CH4 emission further supported our results. The best-fitting PLS model identified that soil CH4 flux responded strongly to inherited (legacy) soil properties with a predominant effect of the soil phosphorus, clay, silt + clay content, potassium, and total nitrogen (Figure 7) (Yu et al., 2017). The relative contribution of anthropogenic and natural factors are 44% and 56%, respectively, to the total variation in CH4 flux estimation. This contrasts with CO2 and N2O emissions, where anthropogenic management factors (residue and nutrient input) and acquired soil properties played a more significant role. Our results confirmed previous results that edaphic variables had greater control than anthropogenic factors on CH4 fluxes at the global scale (Gatica et al., 2020). The lower predictive power of the PLS model for CH4 fluxes (r2 = 0.35) compared to CO2 (r2 = 0.88) and N2O (r2 = 0.59) suggests that CH4 emissions are governed by more complex or less direct factors that the model does not fully capture (Xu et al., 2024). This finding underscores the need for a more nuanced understanding of the interactions between soil management practices and CH4 fluxes, particularly across different soil types.
Normalizing the non-CO2 (CH4 and N2O) GHG emission to CO2 emissions gives a single metric that helps identify the best management practices and soil type relevant to climate change mitigation and feedback. Among the three soil types examined, Alfisol exhibited the highest cumulative GWP, 1.2 times greater than Vertisol and 1.4 times greater than Inceptisol across all residue and nutrient input levels. That suggests that Alfisol may be more prone to greenhouse gas (GHG) emissions due to its inherent properties when subjected to residue and nutrient management practices. Though high residue input (WR10 and WR15) generally decreased CH4 and N2O emission, the increasing trend of GWP with higher residue input levels (WR15 > WR10 > WR5 > WR0) is likely due to the increased availability of organic carbon from the residue, which accelerates microbial activity microbial biomass carbon and leads to higher CO2 emissions, the primary contributor to GWP, that aligned with previous studies (Li et al., 2022; Singh et al., 2024). The response of GWP to residue and nutrient input was modulated by soil type (p = 0.047), further emphasizing the complexity of the factors that influence GWP in soils (Rakhsh et al., 2017; Li et al., 2021a). Across all treatments, the integrated application of WR15 plus NP2 had the highest cumulative GWP of 1,489.76 mg CO2-C eq. kg−1 soil in Alfisol. In contrast, the lowest GWP (96.70 mg CO2-C eq. kg−1 soil) was recorded in the treatment with no residue input (WR0) and moderate nutrient input (NP1) in Inceptisol. These results underscore the need for tailored residue and nutrient management strategies to mitigate GWP in agricultural soils. While residue inputs are essential for maintaining soil health and fertility, their impact on GHG emissions and GWP must be carefully managed, especially in soils like Alfisol, which are more susceptible to high GWP. The study suggests that reducing residue input or optimizing nutrient management could effectively reduce GWP in such soils, thereby contributing to more sustainable agricultural practices. Additionally, wheat residue return to soil compared with residue burning could emit fewer GHGs (Deshpande et al., 2023) considering the potential benefits of wheat residue return on improving soil health, soil organic carbon, and crop yield, plus the associated co-benefits in improving essential ecosystem services (Singh et al., 2020; Li et al., 2022; Zhang et al., 2023; Ma et al., 2024).
The study highlights the importance of understanding the interactions between residue management, nutrient inputs, and soil type in influencing GWP. By adopting management practices that consider these interactions, it may be possible to reduce the climate impact of agricultural soils while maintaining their productivity and ecological function (Lenka et al., 2017; Wang X. D et al., 2021; Li et al., 2022).
Crop residue returned soils are crucial in sequestering SOC and GHG emission mitigation. This study provides new knowledge to enhance the understanding of different drivers and their effect on GHG emission and C mineralization in response to the varied levels of crop residue and nutrient inputs in soils with different inherent properties (e.g., clay, sand, pH, legacy P). High residue input significantly increased C mineralization, and the effects of nutrient input were comparable to those of no nutrient input. Vertisol (cf. Incetisol and Alfisol) exhibited a significant increase in N2O emission at high residue (WR15) and nutrient (NP2) input because of high clay and greater soil NO3-N and alkaline phosphatase activity. Higher wheat residue inputs (WR10 and WR15) significantly reduced cumulative CH4 flux, with the strongest effect in Vertisol, followed by Alfisol and Inceptisol. GWP was the highest in Alfisol, followed by Vertisol and Inceptisol, with labile carbon and microbial biomass significantly impacted by residue and nutrient inputs key for regulating soil microbial activity and nutrient cycling. The PLS model analysis further revealed that N2O and CO2 emissions were more influenced by anthropogenic management practices and acquired soil properties, while CH4 fluxes were more responsive to inherited soil characteristics. This study was conducted in controlled lab conditions, where wheat residues were mixed into the soil. However, real field conditions, including episodic events like rainfall and crop, may trigger additional N2O emissions. Future research should explore field studies to capture these factors fully. These findings should be explicitly considered to improve process-based models to predict better C and N dynamics and their responses to integrated residue and nutrient management, which have implications for GHG mitigation and soil organic carbon sequestration in global agroecosystems.
The original contributions presented in the study are included in the article/Supplementary Material, further inquiries can be directed to the corresponding authors.
DS: Formal Analysis, Investigation, Methodology, Writing–original draft. SL: Conceptualization, Funding acquisition, Methodology, Project administration, Writing–original draft, Writing–review and editing. RK: Formal Analysis, Software, Writing–review and editing. SY: Conceptualization, Formal Analysis, Methodology, Writing–original draft. MS: Software, Writing–original draft. AS: Investigation, Software, Writing–original draft. DY: Formal Analysis, Methodology, Writing–original draft. MC: Formal Analysis, Resources, Writing–review and editing. NL: Formal Analysis, Writing–original draft, Writing–review and editing. TA: Project administration, Resources, Writing–original draft. PJ: Data curation, Investigation, Writing–original draft. VG: Formal Analysis, Investigation, Writing–original draft.
The author(s) declare that financial support was received for the research, authorship, and/or publication of this article. This research was financially supported by the Science and Engineering Research Board, POWER Fellowship (Grant No. SPF/2020/000022), and the instrument from National Agricultural Science Fund of the Indian Council of Agricultural Research, New Delhi (Grant No. NASF/CA-7019/2018-19).
The corresponding author (SL) express sincere gratitude to USDA-Foreign Agrcicultural Service for garnting the Sciemtific Excahnge Program Fellowship on Climate Smart Agriculture. We gratefully acknowledge the editor’s and reviewer’s invaluable contributions in shaping this research.
The authors declare that the research was conducted in the absence of any commercial or financial relationships that could be construed as a potential conflict of interest.
All claims expressed in this article are solely those of the authors and do not necessarily represent those of their affiliated organizations, or those of the publisher, the editors and the reviewers. Any product that may be evaluated in this article, or claim that may be made by its manufacturer, is not guaranteed or endorsed by the publisher.
The Supplementary Material for this article can be found online at: https://www.frontiersin.org/articles/10.3389/fenvs.2024.1489070/full#supplementary-material
Abalos, D., Recous, S., Butterbach-Bahl, K., De Notaris, C., Rittl, T. F., Topp, C. F. E., et al. (2022). A review and meta-analysis of mitigation measures for nitrous oxide emissions from crop residues. Sci. Total Environ. 828, 154388. doi:10.1016/j.scitotenv.2022.154388
Ball, B. C. (2013). Soil structure and greenhouse gas emissions: a synthesis of 20 years of experimentation. Eur. J. Soil Sci. 64, 357–373. doi:10.1111/ejss.12013
Blair, G. J., Lefroy, R. D., and Lisle, L. (1995). Soil carbon fractions based on their degree of oxidation, and the development of a carbon management index for agricultural systems. Aust. J. Agric. Res. 46, 1459–1466. doi:10.1071/AR9951459
Chandrakala, M., Lakhsman, K., and Maske, S. P. (2021). Indian soils: characteristics, distribution, potentials and constraints. Chron. Bioresour. Manag. 4, 121–127.
Chaves, B., Redin, M., Giacomini, S. J., Schmatz, R., Léonard, J., Ferchaud, F., et al. (2021). The combination of residue quality, residue placement and soil mineral N content drives C and N dynamics by modifying N availability to microbial decomposers. Soil Biol. biochem. 163, 108434. doi:10.1016/j.soilbio.2021.108434
Choudhary, R., Lenka, S., Yadav, D. K., Lenka, N. K., Kanwar, R. S., Sarkar, A., et al. (2024). Impact of crop residue, nutrients, and soil moisture on methane emissions from soil under long-term conservation tillage. Soil Syst. 8, 88–19. doi:10.3390/soilsystems8030088
Chowdhury, S., Bolan, N., Farrell, M., Sarkar, B., Sarker, J. R., Kirkham, M. B., et al. (2021). Role of cultural and nutrient management practices in carbon sequestration in agricultural soil. 1st ed. Elsevier Inc. doi:10.1016/bs.agron.2020.10.001
Conde, E., Cardenas, M., Ponce-Mendoza, A., Luna-Guido, M. L., Cruz-Mondragón, C., and Dendooven, L. (2005). The impacts of inorganic nitrogen application on mineralization of C-labelled maize and glucose, and on priming effect in saline alkaline soil. Soil Biol. biochem. 37, 681–691. doi:10.1016/j.soilbio.2004.08.026
Cui, P., Chen, Z., Fan, F., Yin, C., Song, A., Li, T., et al. (2023). Soil texture is an easily overlooked factor affecting the temperature sensitivity of N2O emissions. Sci. Total Environ. 862, 160648. doi:10.1016/j.scitotenv.2022.160648
Della Chiesa, T., Piñeiro, G., and Yahdjian, L. (2019). Gross, background, and net anthropogenic soil nitrous oxide emissions from soybean, corn, and wheat croplands. J. Environ. Qual. 48, 16–23. doi:10.2134/jeq2018.07.0262
Deshpande, M. V., Kumar, N., Pillai, D., Krishna, V. V., and Jain, M. (2023). Greenhouse gas emissions from agricultural residue burning have increased by 75 % since 2011 across India. Sci. Total Environ. 904, 166944. doi:10.1016/j.scitotenv.2023.166944
Dror, I., Yaron, B., and Berkowitz, B. (2022). The human impact on all soil-forming factors during the anthropocene. ACS Environ. Au 2, 11–19. doi:10.1021/acsenvironau.1c00010
Fang, Y., Nazaries, L., Singh, B. K., and Singh, B. P. (2018). Microbial mechanisms of carbon priming effects revealed during the interaction of crop residue and nutrient inputs in contrasting soils. Glob. Chang. Biol. 24, 2775–2790. doi:10.1111/gcb.14154
Fang, Y., Singh, B. P., Collins, D., Armstrong, R., Van Zwieten, L., and Tavakkoli, E. (2020). Nutrient stoichiometry and labile carbon content of organic amendments control microbial biomass and carbon-use efficiency in a poorly structured sodic-subsoil. Biol. Fertil. Soils 56, 219–233. doi:10.1007/s00374-019-01413-3
Fang, Y., Singh, B. P., Cowie, A., Wang, W., Arachchi, M. H., Wang, H., et al. (2019). Balancing nutrient stoichiometry facilitates the fate of wheat residue-carbon in physically defined soil organic matter fractions. Geoderma 354, 113883. doi:10.1016/j.geoderma.2019.113883
Fontaine, S., Bardoux, G., Abbadie, L., and Mariotti, A. (2004). Carbon input to soil may decrease soil carbon content. Ecol. Lett. 7, 314–320. doi:10.1111/j.1461-0248.2004.00579.x
Gatica, G., Fernández, M. E., Juliarena, M. P., and Gyenge, J. (2020). Environmental and anthropogenic drivers of soil methane fluxes in forests: global patterns and among-biomes differences. Glob. Chang. Biol. 26, 6604–6615. doi:10.1111/gcb.15331
Gebremichael, A. W., Wall, D. P., O’Neill, R. M., Krol, D. J., Brennan, F., Lanigan, G., et al. (2022). Effect of contrasting phosphorus levels on nitrous oxide and carbon dioxide emissions from temperate grassland soils. Sci. Rep. 12, 2602–2613. doi:10.1038/s41598-022-06661-2
Golchin, A., and Misaghi, M. (2024). Investigating the effects of climate change and anthropogenic activities on SOC storage and cumulative CO2 emissions in forest soils across altitudinal gradients using the century model. Sci. Total Environ. 943, 173758. doi:10.1016/j.scitotenv.2024.173758
Gómez-Gener, L., Gubau, M., von Schiller, D., Marcé, R., and Obrador, B. (2018). Effect of small water retention structures on diffusive CO2 and CH4 emissions along a highly impounded river. Inl. Waters 8, 449–460. doi:10.1080/20442041.2018.1457846
Hargreaves, P. R., Baker, K. L., Graceson, A., Bonnett, S. A. F., Ball, B. C., and Cloy, J. M. (2021). Use of a nitrification inhibitor reduces nitrous oxide (N2O) emissions from compacted grassland with different soil textures and climatic conditions. Agric. Ecosyst. Environ. 310, 107307. doi:10.1016/j.agee.2021.107307
Islam, K. R., Stine, M. A., Gruver, J. B., Samson-Liebig, S. E., and Weil, R. R. (2003). Estimating active carbon for soil quality assessment: a simplified method for laboratory and field use. Am. J. Altern. Agric. 18, 3–17. doi:10.1079/ajaa2003003
Joergensen, R. G., and Brookes, P. C. (1990). Ninhydrin-reactive nitrogen measurements of microbial biomass in 0.5 m K2SO4 soil extracts. Soil Biol. biochem. 22, 1023–1027. doi:10.1016/0038-0717(90)90027-W
Joshi, D. R., Clay, D. E., Clay, S. A., Moriles-Miller, J., Daigh, A. L. M., Reicks, G., et al. (2024). Quantification and machine learning based N2O–N and CO2–C emissions predictions from a decomposing rye cover crop. Agron. J. 116, 795–809. doi:10.1002/agj2.21185
Kempers, A. J. (1974). Determination of sub-microquantities of ammonium and nitrates in soils with phenol, sodium nitroprusside and hypochlorite. Geoderma 12, 201–206. doi:10.1016/0016-7061(74)90068-8
Khalifah, S., and Foltz, M. E. (2024). The ratio of denitrification end-products were influenced by soil pH and clay content across different texture classes in Oklahoma soils. Front. Soil Sci. 4, 1–9. doi:10.3389/fsoil.2024.1342986
Lai, R., Arca, P., Lagomarsino, A., Cappai, C., Seddaiu, G., Demurtas, C. E., et al. (2017). Manure fertilization increases soil respiration and creates a negative carbon budget in a Mediterranean maize (Zea mays L.)-based cropping system. Catena 151, 202–212. doi:10.1016/j.catena.2016.12.013
Lenka, S., Choudhary, R., Lenka, N. K., Saha, J. K., Amat, D., Patra, A. K., et al. (2022). Nutrient management drives the direction and magnitude of nitrous oxide flux in crop residue-returned soil under different soil moisture. Front. Environ. Sci. 10, 857233. doi:10.3389/fenvs.2022.857233
Lenka, S., Lenka, N. K., Singh, A. B., Singh, B., and Raghuwanshi, J. (2017). Global warming potential and greenhouse gas emission under different soil nutrient management practices in soybean–wheat system of central India. Environ. Sci. Pollut. Res. 24, 4603–4612. doi:10.1007/s11356-016-8189-5
Lenka, S., Malviya, S. K., Lenka, N. K., Sahoo, S., Bhattacharjya, S., Jain, R. C., et al. (2021). Manure addition influences the effect of tillage on soil aggregation and aggregate associated carbon in a Vertisol of central India. J. Environ. Biol. 41, 1585–1593. doi:10.22438/JEB/41/6/SI-221
Lenka, S., Trivedi, P., Singh, B., Singh, B. P., Pendall, E., Bass, A., et al. (2019). Effect of crop residue addition on soil organic carbon priming as influenced by temperature and soil properties. Geoderma 347, 70–79. doi:10.1016/j.geoderma.2019.03.039
Li, Y., Chang, S. X., Tian, L., and Zhang, Q. (2018). Conservation agriculture practices increase soil microbial biomass carbon and nitrogen in agricultural soils: a global meta-analysis. Soil Biol. biochem. 121, 50–58. doi:10.1016/j.soilbio.2018.02.024
Li, Z., Reichel, R., and Brüggemann, N. (2021a). Effect of C:N:P stoichiometry on soil nitrous oxide emission and nitrogen retention. J. Plant Nutr. Soil Sci. 184, 520–529. doi:10.1002/jpln.202000416
Li, Z., Reichel, R., Xu, Z., Vereecken, H., and Brüggemann, N. (2021b). Return of crop residues to arable land stimulates N2O emission but mitigates NO3− leaching: a meta-analysis. Agron. Sustain. Dev. 41, 66. doi:10.1007/s13593-021-00715-x
Li, Z., Zhang, Q., Li, Z., Qiao, Y., Du, K., Tian, C., et al. (2022). Effects of straw mulching and nitrogen application rates on crop yields, fertilizer use efficiency, and greenhouse gas emissions of summer maize. Sci. Total Environ. 847, 157681. doi:10.1016/j.scitotenv.2022.157681
Liu, J., Hou, H., and Zhang, W. (2023a). Fungi contribute more to N2O emissions than bacteria in two paddy soils with different textures. Eur. J. Soil Biol. 115, 103476. doi:10.1016/j.ejsobi.2023.103476
Liu, J., Qiu, T., Peñuelas, J., Sardans, J., Tan, W., Wei, X., et al. (2023b). Crop residue return sustains global soil ecological stoichiometry balance. Glob. Chang. Biol. 29, 2203–2226. doi:10.1111/gcb.16584
Liu, X., Peñuelas, J., Sardans, J., Fang, Y., Wiesmeier, M., Wu, L., et al. (2021). Response of soil nutrient concentrations and stoichiometry, and greenhouse gas carbon emissions linked to change in land-use of paddy fields in China. Catena 203, 105326. doi:10.1016/j.catena.2021.105326
Ma, B., Karimi, M. S., Mohammed, K. S., Shahzadi, I., and Dai, J. (2024). Nexus between climate change, agricultural output, fertilizer use, agriculture soil emissions: novel implications in the context of environmental management. J. Clean. Prod. 450, 141801. doi:10.1016/j.jclepro.2024.141801
Ma, S., Chen, G., Tian, D., Du, E., Xiao, W., Jiang, L., et al. (2020). Effects of seven-year nitrogen and phosphorus additions on soil microbial community structures and residues in a tropical forest in Hainan Island, China. Geoderma 361, 114034. doi:10.1016/j.geoderma.2019.114034
Magazzino, C., Cerulli, G., Haouas, I., Unuofin, J. O., and Sarkodie, S. A. (2024). The drivers of GHG emissions: a novel approach to estimate emissions using nonparametric analysis. Gondwana Res. 127, 4–21. doi:10.1016/j.gr.2023.10.004
Nabuurs, G.-J., Mrabet, R., Abu Hatab, A., Bustamante, M., Clark, H., Havlík, P., et al. (2022). IPCC sixth assessment report. Mitigation of climate change, Chapter 7: agriculture, forestry and other land uses. doi:10.1017/9781009157926.009
Nguyen, B. T., Trinh, N. N., and Bach, Q. V. (2013). Emissions of CH4 and N2O under different tillage systems from double-cropped paddy fields in southern China. PLoS One 8, e65277. doi:10.1016/j.apsoil.2020.103531
Oertel, C., Matschullat, J., Zurba, K., Zimmermann, F., and Erasmi, S. (2016). Greenhouse gas emissions from soils—a review. Chem. Erde 76, 327–352. doi:10.1016/j.chemer.2016.04.002
Page, A. L., Millar, R. H., and Keeney, D. R. (1982). Methods of soil analysis, Part 2. Madison WI, USA: American Society of Agronomy and Soil Sciences Society of America. Ponnamperuma.
Pathak, P., Sudi, R., Wani, S. P., and Sahrawat, K. L. (2013). Hydrological behavior of Alfisols and Vertisols in the semi-arid zone: implications for soil and water management. Agric. Water Manag. 118, 12–21. doi:10.1016/j.agwat.2012.11.012
Pfülb, L., Elsgaard, L., Dörsch, P., Fuß, R., and Well, R. (2024). Impact of liming and maize residues on N2O and N2 fluxes in agricultural soils: an incubation study. Biol. Fertil. Soils. doi:10.1007/s00374-024-01825-w
Ponce-Mendoza, A., Ceballos-Ramírez, J. M., Gutierrez-Micelli, F., and Dendooven, L. (2010). Emission of nitrous oxide and carbon dioxide from semi-arid tropical soils in Chiapas México. Rev. Bras. Ciência do Solo 34, 1617–1628. doi:10.1590/s0100-06832010000500015
Rakhsh, F., Golchin, A., Beheshti Al Agha, A., and Alamdari, P. (2017). Effects of exchangeable cations, mineralogy and clay content on the mineralization of plant residue carbon. Geoderma 307, 150–158. doi:10.1016/j.geoderma.2017.07.010
Ren, F., Zhang, R., Sun, N., Li, Y., Xu, M., Zhang, F., et al. (2024). Patterns and driving factors of soil organic carbon sequestration efficiency under various manure regimes across Chinese croplands. Agric. Ecosyst. Environ. 359, 108723. doi:10.1016/j.agee.2023.108723
Salehin, S. M. U., Rajan, N., Mowrer, J., Casey, K. D., Somenahally, A. C., and Bagavathiannan, M. (2024). Greenhouse gas emissions during decomposition of cover crops and poultry litter with simulated tillage in 90-day soil incubations. Soil Sci. Soc. Am. J., 1–21. doi:10.1002/saj2.20730
Shah, A., Huang, J., Han, T., Khan, M. N., Tadesse, K. A., Daba, N. A., et al. (2024). Impact of soil moisture regimes on greenhouse gas emissions, soil microbial biomass, and enzymatic activity in long-term fertilized paddy soil. Environ. Sci. Eur. 36, 120. doi:10.1186/s12302-024-00943-4
Shakoor, A., Dar, A. A., Arif, M. S., Farooq, T. H., Yasmeen, T., Shahzad, S. M., et al. (2022). Do soil conservation practices exceed their relevance as a countermeasure to greenhouse gases emissions and increase crop productivity in agriculture? Sci. Total Environ. 805, 150337. doi:10.1016/j.scitotenv.2021.150337
Shumba, A., Chikowo, R., Corbeels, M., Six, J., Thierfelder, C., and Cardinael, R. (2023). Long-term tillage, residue management and crop rotation impacts on N2O and CH4 emissions from two contrasting soils in sub-humid Zimbabwe. Agric. Ecosyst. Environ. 341, 108207. doi:10.1016/j.agee.2022.108207
Šimek, M., and Cooper, J. E. (2002). The influence of soil pH on denitrification: progress towards the understanding of this interaction over the last 50 years. Eur. J. Soil Sci. 53, 345–354. doi:10.1046/j.1365-2389.2002.00461.x
Singh, D., Lenka, S., Lenka, N. K., Trivedi, S. K., Bhattacharjya, S., Sahoo, S., et al. (2020). Effect of reversal of conservation tillage on soil nutrient availability and crop nutrient uptake in soybean in the vertisols of central India. Sustain 12, 6608. doi:10.3390/su12166608
Singh, D., Lenka, S., Lenka, N. K., Yadav, D. K., Yadav, S. S., Kanwar, R. S., et al. (2024). Residue management and nutrient stoichiometry control greenhouse gas and global warming potential responses in Alfisols. Sustain 16, 3997. doi:10.3390/su16103997
Singh, R. C., Lenka, S., and Singh, C. D. (2014). Conservation tillage and manure effect on soil aggregation, yield and energy requirement for wheat (Triticum aestivum) in vertisols. Indian J. Agric. Sci. 84, 267–271. doi:10.56093/ijas.v84i2.38047
Song, X., Liu, X., Liang, G., Li, S., Li, J., Zhang, M., et al. (2022). Positive priming effect explained by microbial nitrogen mining and stoichiometric decomposition at different stages. Soil Biol. biochem. 175, 108852. doi:10.1016/j.soilbio.2022.108852
Wang, C., Amon, B., Schulz, K., and Mehdi, B. (2021). Factors that influence nitrous oxide emissions from agricultural soils as well as their representation in simulation models: a review. Agronomy 11, 770. doi:10.3390/agronomy11040770
Wang, X. D., He, C., Cheng, H. Y., Liu, B. Y., Li, S. S., Wang, Q., et al. (2021). Responses of greenhouse gas emissions to residue returning in China’s croplands and influential factors: a meta-analysis. J. Environ. Manage. 289, 112486. doi:10.1016/j.jenvman.2021.112486
Weitz, A. M., Linder, E., Frolking, S., Crill, P. M., and Keller, M. (2001). N2O emissions from humid tropical agricultural soils: effects of soil moisture, texture and nitrogen availability. Soil Biol. biochem. 33, 1077–1093. doi:10.1016/S0038-0717(01)00013-X
Wu, J., Chen, L., Zhang, H., Zhao, X., Cheng, X., Zhang, K., et al. (2024). Soil methane uptake is tightly linked to carbon dioxide emission in global upland ecosystems. Agric. Ecosyst. Environ. 373, 109127. doi:10.1016/j.agee.2024.109127
Xu, X., Xiao, C., Dong, Y., Zhan, L., Bi, R., Song, M., et al. (2024). Machine learning algorithms realized soil stoichiometry prediction and its driver identification in intensive agroecosystems across a north-south transect of eastern China. Sci. Total Environ. 906, 167488. doi:10.1016/j.scitotenv.2023.167488
Yang, T., Lupwayi, N., Marc, S. A., Siddique, K. H. M., and Bainard, L. D. (2021). Anthropogenic drivers of soil microbial communities and impacts on soil biological functions in agroecosystems. Glob. Ecol. Conserv. 27, e01521. doi:10.1016/j.gecco.2021.e01521
Yu, L., Huang, Y., Zhang, W., Li, T., and Sun, W. (2017). Methane uptake in global forest and grassland soils from 1981 to 2010. Sci. Total Environ. 607–608, 1163–1172. doi:10.1016/j.scitotenv.2017.07.082
Yu, Y., Zhao, C., Zheng, N., Jia, H., and Yao, H. (2019). Interactive effects of soil texture and salinity on nitrous oxide emissions following crop residue amendment. Geoderma 337, 1146–1154. doi:10.1016/j.geoderma.2018.11.012
Keywords: machine learning, predictor variable, greenhouse gasses, residue, soil type, mitigation
Citation: Singh D, Lenka S, Kanwar RS, Yadav SS, Saha M, Sarkar A, Yadav DK, Vassanda Coumar M, Lenka NK, Adhikari T, Jadon P and Gami V (2024) Drivers of greenhouse gas emissions in agricultural soils: the effect of residue management and soil type. Front. Environ. Sci. 12:1489070. doi: 10.3389/fenvs.2024.1489070
Received: 31 August 2024; Accepted: 14 October 2024;
Published: 29 October 2024.
Edited by:
Rui Yang, Chinese Academy of Agricultural Sciences, ChinaReviewed by:
Yuan Li, Lanzhou University, ChinaCopyright © 2024 Singh, Lenka, Kanwar, Yadav, Saha, Sarkar, Yadav, Vassanda Coumar, Lenka, Adhikari, Jadon and Gami. This is an open-access article distributed under the terms of the Creative Commons Attribution License (CC BY). The use, distribution or reproduction in other forums is permitted, provided the original author(s) and the copyright owner(s) are credited and that the original publication in this journal is cited, in accordance with accepted academic practice. No use, distribution or reproduction is permitted which does not comply with these terms.
*Correspondence: Sangeeta Lenka, c2FuZ2VldGEubGVua2FAaWNhci5nb3YuaW4=; Narendra Kumar Lenka, bmtsZW5rYTc0QGdtYWlsLmNvbQ==
Disclaimer: All claims expressed in this article are solely those of the authors and do not necessarily represent those of their affiliated organizations, or those of the publisher, the editors and the reviewers. Any product that may be evaluated in this article or claim that may be made by its manufacturer is not guaranteed or endorsed by the publisher.
Research integrity at Frontiers
Learn more about the work of our research integrity team to safeguard the quality of each article we publish.