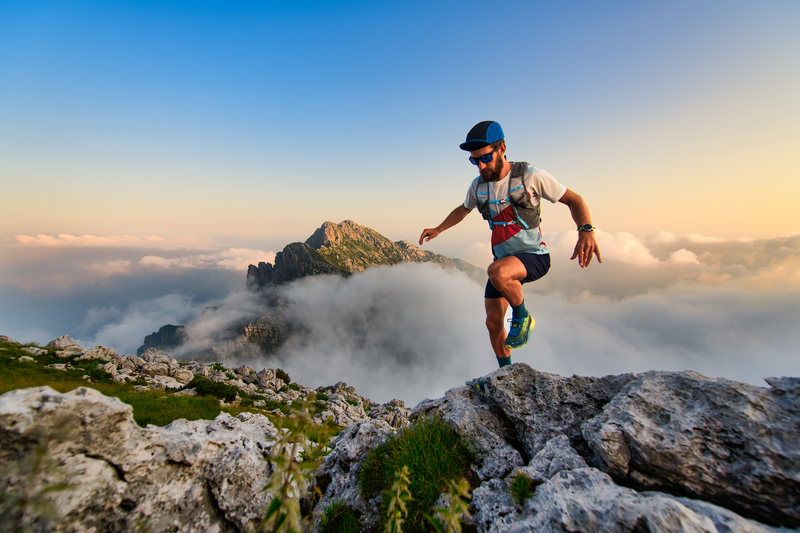
95% of researchers rate our articles as excellent or good
Learn more about the work of our research integrity team to safeguard the quality of each article we publish.
Find out more
ORIGINAL RESEARCH article
Front. Environ. Sci. , 10 January 2025
Sec. Environmental Economics and Management
Volume 12 - 2024 | https://doi.org/10.3389/fenvs.2024.1488526
This article is part of the Research Topic Advancing Carbon Reduction and Pollution Control Policies Management: Theoretical, Application, and Future Impacts View all 32 articles
In the context of global efforts to combat climate change, highlighted by the 2024 UN Climate Change Conference (COP29) and the growing global emphasis on low-carbon development, this study investigates the impact of China’s low-carbon city pilot policies on carbon emission intensity. Using data from 283 Chinese cities between 2005 and 2021, a multi-period difference-in-differences (DID) model is employed to analyze the effects of these policies. The study also explores the mediating mechanisms, moderating effects, and heterogeneity across cities. The main findings are as follows: (1) The low-carbon city pilot policies significantly reduce carbon emission intensity, with the impact becoming stronger and more stable over time. (2) The reduction in carbon intensity is partially mediated by enhanced carbon sink levels and industrial structure upgrades, although technological investment does not have a significant effect. (3) Environmental regulations negatively moderate the policy’s effectiveness, while fiscal freedom and population growth rates positively influence its impact. (4) The effects of the policy are heterogeneous across cities, driven by differences in economic levels, geographical locations, industrial bases, resource endowments, and population sizes. This paper provides valuable empirical insights and policy recommendations for China’s low-carbon transition and for achieving its carbon neutrality and peak emission targets.
In recent years, the greenhouse effect, driven by extensive carbon dioxide emissions, has become an increasingly urgent issue, drawing global attention to climate change. Since the Kyoto Protocol, effective mitigation of global warming has remained a central concern, with a growing international focus on reducing emissions. At the 2024 Global Low-Carbon Development Conference (COP29), countries reaffirmed their commitment to cutting emissions and promoting low-carbon development (Wang ZS. et al., 2023). As one of the world’s largest carbon emitters, China has taken proactive steps to address carbon emissions, placing climate change governance at the forefront of its agenda. It is expected that China will play a pivotal role in global efforts to reduce emissions, making the study of effective emission reduction policies crucial to advancing the country’s carbon reduction goals.
In 2020, President Xi Jinping introduced the “dual carbon” goals, outlining a strategic framework for achieving carbon neutrality and carbon peaking. These goals provide a blueprint for China’s economic development in the new era, integrating reduced carbon emissions with green transformation. As China transitions to a phase of high-quality economic growth, developing a low-carbon economy is seen as a crucial pathway to aligning economic progress with the “dual carbon” objectives. The 20th National Congress of the Communist Party of China emphasized the need for coordinated efforts to reduce carbon emissions, curb pollution, expand green initiatives, and promote sustainable economic growth. While China is diligently pursuing the goals of “carbon peaking” and “carbon neutrality,” significant challenges remain as of 2024. The gap between current progress and the “dual carbon” targets underscores the urgency of accelerating the effective implementation of low-carbon policies. China’s low-carbon initiatives are crucial not only for global climate governance but also for achieving high-quality economic development. The successful implementation of low-carbon policies requires a delicate balance between economic growth and environmental governance across the socio-economic system. Local governments play a key role in translating national policies into effective local strategies that drive low-carbon and green transformation. These strategies focus on raising industrial governance costs to incentivize enterprises to reduce emissions, while also encouraging residents to adopt energy-saving behaviors. The Ministry of Finance has highlighted the need to optimize policy frameworks and accelerate the creation of financial mechanisms tailored to China’s unique context, emphasizing the urgency of advancing low-carbon policies.
China’s low-carbon city pilot program, launched in 2010, has expanded in three phases, covering numerous provinces and cities across the country. The policy aims to chart a “Chinese-style” path to low-carbon governance, focusing on industrial transformation and other strategic measures. While existing research has assessed the economic and social benefits of the policy, there is limited investigation into its impact on urban carbon intensity (Qiu et al., 2021; Chen et al., 2021; Liu and Yuan, 2023; Liu and Zhao, 2023). Most studies tend to focus on total carbon emissions or per capita emissions, often overlooking the complex interplay between economic development and environmental governance (Zhu et al., 2023a; Huang et al., 2021; Zhu et al., 2023b). Comprehensive and systematic studies on the policy’s “carbon reduction” effects remain scarce. Theoretically, it is crucial not only to assess whether the low-carbon city pilot policy effectively reduces urban carbon intensity but also to understand the mechanisms and factors driving these reductions. Key questions include: What economic and social factors influence the success of low-carbon policies? How do variations in cities' economic development, geographic locations, and resource endowments shape policy outcomes? As China’s economic growth slows, and the challenge of balancing carbon reductions with sustained growth becomes more pressing, addressing these questions is essential for reconciling the goals of high-quality economic development and the dual carbon targets. Gaining this understanding will be vital for policy development and the formulation of “carbon finance” strategies to support China’s green transition.
This study treats China’s low-carbon city pilot policy as a “quasi-natural experiment” and uses a multi-period difference-in-differences (DID) model to evaluate its impact on urban carbon intensity. It also examines the underlying mechanisms driving emission reductions, such as carbon sequestration and industrial upgrading, and explores how the policy’s effects vary across cities with different economic levels, geographic locations, and resource endowments. The primary objective of this study is to provide empirical evidence to optimize China’s low-carbon city pilot policies. In doing so, it aims to assist the government in balancing economic growth with environmental protection, while advancing the “dual carbon” goals and promoting a green economic transformation. The findings will not only enhance the implementation of low-carbon policies in China but also offer valuable insights for global low-carbon transitions. The novelty of this study lies in three key aspects: (1) examining the dynamic effects of carbon reduction, including both overall and stage-specific impacts, to provide data-driven insights for timely policy adjustments and prevent “free-riding” behavior in pilot cities; (2) analyzing the potential mediating and moderating mechanisms underlying the carbon reduction effects, which deepens the understanding of the policy’s internal workings; and (3) exploring the heterogeneity of policy effects by considering factors such as economic development, geographic location, and resource endowments, thereby offering insights for the formulation of tailored, local-level policies.
The remainder of this paper is organized as follows: Section 2 provides a comprehensive review of the literature on low-carbon policies, carbon reduction effects, and low-carbon city pilot initiatives. Section 3 explains the mechanisms of the pilot policies, with specific theoretical hypotheses addressing the direct effects, mediating effects, and moderating effects of the policies. Section 4 outlines the methodology, including the multi-period difference-in-differences (DID) model, data sources, and variable definitions. Section 5 presents the empirical results, covering baseline regressions, dynamic analysis, robustness checks, and an exploration of the policy’s mechanisms and its heterogeneity. Finally, Section 6 concludes with a summary of the key findings, policy implications, and a discussion of future directions for low-carbon policy development.
This study primarily explores the relationship between urban carbon emission intensity and low-carbon city pilot policies. Therefore, the existing literature is reviewed and summarized in relation to these two key areas.
Since the introduction of the low-carbon development concept in China, a variety of policies aimed at reducing carbon emissions have been implemented, with profound impacts on the economy, society, and environment. Research on the effects of these policies has expanded significantly, focusing on their economic, social, and ecological benefits. However, few studies have examined these dimensions in an integrated manner (Wang and Xiao, 2022; Wang ZS. et al., 2022; Shi et al., 2022).
The first area of research focuses on the “carbon market” and the carbon emissions trading system (ETS). Following the signing of the Kyoto Protocol, early studies concentrated on the trading mechanisms of carbon markets (Sandoff and Schaad, 2009). Classical market theory suggests that carbon markets are an effective tool for managing greenhouse gas emissions, offering flexibility and cost advantages (Jiang et al., 2016). As China’s carbon finance market has grown rapidly, its unique advantages—such as low-cost carbon markets and significant emission reduction potential—have become more evident (Zhang et al., 2013). Early studies, including those using CGE (Computable General Equilibrium) and multi-agent models, simulated the effects of emissions trading and confirmed the effectiveness of China’s carbon emissions trading system (Böhringer and Welsch, 2003; Suopajärvi et al., 2014; Li and Jia, 2016; Huang et al., 2023; Cai et al., 2016; Li et al., 2022). Zhang et al. (2017) empirically tested the significant emission reduction effects of carbon market policies using data from pilot cities between 2013 and 2014 (Zhang YJ. et al., 2017). Later studies using the difference-in-differences (DID) model with provincial panel data further confirmed that pilot policies significantly enhance carbon reduction outcomes (Tan et al., 2022; Xu et al., 2023; Song et al., 2023; Lu and Li, 2023; Jiang et al., 2024). To avoid bias in treatment group selection, some scholars applied propensity score matching (PSM) for empirical analysis (Xiao et al., 2023), while others addressed matching errors—due to overlapping provinces and years—using the synthetic control method, which optimally applies weights (Lin and Fu, 2023; Wang CZ. et al., 2024).
Another key area of research focuses on the financial support for carbon emission policies. Financial reforms play a crucial role in the effective implementation of carbon emission governance. Early studies on the relationship between financial development and carbon emissions have yielded mixed results (Sadorsky and Renewable energy consumption, 2009; Shahbaz et al., 2013; Ganda, 2021; Liu et al., 2023). However, with the advancement of financial policies, green credit has become a central focus of research on financial support for carbon emission reduction. Studies have shown that green financial policies can reduce air and water pollution emissions (Zhang AL. et al., 2022) and directly curb CO₂ emissions (Tan and Chen, 2023), with some suggesting a U-shaped relationship between green credit development and technological innovation (Xu and Lin, 2025). As a key tool for financial institutions to fulfill their environmental responsibilities, green credit policies significantly impact energy efficiency, particularly in sectors constrained by green credit. In these sectors, energy intensity tends to decrease, although challenges arise due to a “financing constraints-innovation motivation weakening” cycle (Luo et al., 2023). Additionally, green financial policies, by reducing energy intensity and promoting technological innovation, produce increasing carbon reduction effects over time. This leads to positive spillover effects, although the synergy effect remains insignificant (Fan et al., 2024).
Finally, research on fiscal support for carbon emission policies has gained momentum. China’s fiscal policies, which support the goals of carbon peaking and carbon neutrality, emphasize the need for policies tailored to the country’s national conditions. Early studies on fiscal decentralization, such as those by He Qichun (2015), analyzed the relationship between fiscal decentralization and environmental pollution, finding a positive correlation (He, 2015). Research on transfer payments and local government competition further supports this view, suggesting that fiscal decentralization, coupled with government competition, can exacerbate carbon emissions (Zhang K. et al., 2017; Li, 2022). In contrast, studies on ecological governance and environmental expenditure indicate that fiscal and environmental policies can reduce emissions, though their effectiveness varies regionally (Wang SG. et al., 2024). Later research employing cost-estimation and system dynamics methods demonstrated that clean energy subsidy policies can improve energy consumption structures and reduce pollution intensity, although their effectiveness depends on program design (Zhang et al., 2023). Some studies have also found that energy-saving and emission reduction policies can have negative effects on corporate behavior, particularly in terms of environmental, social, and governance (ESG) performance, with this negative impact intensifying over time (Miao et al., 2023).
The Chinese government has implemented several low-carbon city pilot policies to promote carbon emission reductions. Research on these policies has primarily focused on areas such as green total factor productivity, technological innovation, and employment, with some studies examining low-carbon development pathways and carbon emission levels (Wang KL. et al., 2022; Ma et al., 2021; Wang CA. et al., 2023; Zhao et al., 2024). Most studies highlight the positive effects of these pilot policies, often using methods such as pathway analysis and single- and double-difference models. The widespread use of the difference-in-differences (DiD) model has provided empirical evidence that these pilot policies significantly improve air quality in the regions where they are implemented (Wolff, 2014). Carbon reduction effects are particularly prominent in western cities and regions with lower economic development levels (Zhang JG. et al., 2022). In contrast, carbon emissions in eastern regions have decreased rapidly, with northern regions experiencing higher reduction rates than southern ones (Wen et al., 2022). In terms of mediating effects, studies have found that pilot policies contribute to carbon emission reductions through mechanisms such as industrial structure upgrades, technological innovation, energy efficiency improvements, and the promotion of green innovation (Zhang H. et al., 2022; Pan et al., 2022; Zheng et al., 2023). Additionally, some scholars have explored co-reduction effects, noting that low-carbon cities often experience significant and heterogeneous reductions in both CO₂ and PM2.5 emissions. Improving public transport infrastructure has been identified as a key pathway for achieving these co-benefits (Yang et al., 2023). Moreover, when examining “dual pilot” cities—those participating in both low-carbon and eco-city initiatives—the synergistic carbon reduction effects are found to be stronger, though with a time lag (Li and Zhang, 2024).
Furthermore, some studies emphasize that the carbon reduction effects of low-carbon city pilot policies strengthen over time, though with delays. These reductions primarily occur through decreased energy consumption, lower energy intensity per unit of GDP, industrial decarbonization, and enhanced green technological innovation (Wang and Li, 2023; Zhao et al., 2023). Research also highlights the heterogeneous effects of fiscal pressures and incentives on carbon reduction outcomes (Yang and Peng, 2022). Current studies on low-carbon city pilot policies predominantly involve simulation models, such as those evaluating government subsidies for voluntary carbon reduction, cost-benefit models, and industry-specific analyses. These studies suggest that voluntary carbon reduction strategies have optimal solutions, with innovation gains and government subsidies serving as key drivers of success (Cheng et al., 2015). Other research has explored the relationship between carbon taxes and low-carbon pilot policies, using supply chain game models to demonstrate that changes in pilot proportions are positively correlated with optimal carbon reductions, while carbon taxes and reduction subsidies are negatively correlated under constant carbon reduction (Cao and Zhang, 2018). Additionally, dynamic stochastic general equilibrium (DSGE) models have been applied to simulate the environmental and corporate effects of pilot policies within China’s current fiscal system. These models suggest that while pilot policies may lead to environmental deterioration due to crowding-out effects, they can also optimize corporate financial structures and incentivize enterprises to implement energy-saving measures on their own (Cai et al., 2019). Some studies from the perspective of the green economy argue that although low-carbon city pilot policies drive significant carbon reductions, their effects on green development are not sustained in the long term (Guan and Liu, 2022).
In summary, the academic literature on policies affecting carbon emissions is extensive, but the findings remain complex and inconclusive. Studies on carbon market trading systems generally present optimistic results, while research on green credit and fiscal support policies yields more mixed outcomes. Most studies on low-carbon city pilot policies focus on their economic and social benefits, with relatively few directly addressing their impact on carbon emissions. There are also several areas where existing research could be improved. For example, future studies could refine the construction of dependent variables to better integrate both economic and environmental factors in assessing carbon reduction effects. Additionally, more work is needed to explore the specific pathways and moderating mechanisms that influence policy effectiveness. Finally, the impact of factors such as city economic development levels, resource endowments, and population distribution on policy outcomes remains underexplored. This study seeks to address these gaps by providing a more comprehensive analysis of low-carbon city pilot policies, contributing valuable insights into their effectiveness and offering a more nuanced understanding of the factors that drive carbon reduction.
Low-carbon city pilot policies are designed to achieve substantial reductions in carbon emissions by integrating various mechanisms, including government performance evaluations, corporate production constraints, and public engagement with low-carbon initiatives. These policies play a vital role in advancing China’s transition to low-carbon and green cities. The effectiveness of these policies is closely tied to several factors. First, government performance is a key determinant of policy effectiveness. An accountability system that assesses government action ensures that policies produce tangible and measurable results, rather than becoming mere formalities that fail to drive real emission reductions. Second, corporate behavior is significantly influenced by environmental regulations and local government policies. In response to low-carbon mandates, businesses are incentivized to adopt cleaner technologies, innovate industrial processes, and upgrade equipment, all of which directly reduce emissions. This process not only contributes to emissions reductions but also fosters a culture of sustainability and innovation within industries. Lastly, public participation is essential to achieving low-carbon objectives. Initiatives like carbon credit systems for residents, along with public awareness campaigns promoting energy-saving and emission-reduction practices, encourage individuals to adopt low-carbon lifestyles, thus contributing to the overall reduction in urban emissions. In summary, the success of low-carbon city pilot policies hinges on the collaboration between government, enterprises, and the public. By aligning these key stakeholders, the policy can effectively reduce urban carbon emissions, making it an essential component of China’s broader low-carbon transition.
Hypothesis 1. The implementation of low-carbon city pilot policies significantly reduces urban carbon dioxide emissions.
Building upon existing literature, this study proposes that the effectiveness of low-carbon city pilot policies in reducing carbon emissions can be mediated through three key mechanisms: enhancing carbon sink levels, fostering technological innovation, and upgrading industrial structures. First, low-carbon city pilot policies can reduce carbon intensity by improving carbon sink capacity. Carbon sequestration plays a critical role in carbon reduction strategies. The policies focus on increasing the capacity for carbon absorption, such as by expanding green areas and forests, which not only mitigate the urban carbon footprint but also create additional environmental and social benefits. The development of green spaces enhances the city’s reputation as a “natural oxygen bar,” attracting tourism and improving the quality of life for residents. Second, these policies support emission reductions through technological innovation. Technological advancement is a major driver of emission reductions, particularly in energy-intensive industries. Investments in new technologies can reduce carbon intensity, promote low-carbon economic growth, and lower pollution levels. Upgrades in energy efficiency, such as improved fossil fuel combustion and renewable energy integration, can significantly cut CO₂ emissions. Additionally, innovations in production processes may lead to the establishment of “self-sustaining” systems that actively reduce greenhouse gases during manufacturing, further driving down emissions. Third, industrial structure upgrades are essential for sustained reductions in carbon emissions. Low-carbon city pilot policies promote structural shifts toward low-carbon industries, such as renewable energy sectors (wind, solar, and photovoltaic energy), which are less energy-intensive. This shift reduces reliance on carbon-heavy industries. Moreover, the development of low-emission, low-energy tertiary industries (such as finance, technology, and logistics) ensures that economic growth is aligned with environmental sustainability, creating a balanced, low-carbon economic model. In summary, low-carbon city pilot policies facilitate carbon emission reductions through three primary pathways: enhancing carbon sink capacity, promoting technological innovation, and upgrading industrial structures.
Hypothesis 2. Low-carbon city pilot policies reduce carbon emissions through three mechanisms: enhancing carbon sink levels, fostering technological innovation, and upgrading industrial structures.
Building on the previous literature, this study proposes that the effectiveness of low-carbon city pilot policies in reducing carbon intensity can be moderated by three key factors: environmental regulation, fiscal freedom, and population growth rate. First, environmental regulation plays a crucial role in enhancing the effectiveness of low-carbon city pilot policies. As the primary enforcers of environmental standards, governments can strengthen the impact of low-carbon policies by implementing stricter regulations. Stringent environmental policies encourage businesses to transition from high-carbon industries to cleaner, more sustainable production methods. In response, companies may invest in upgrading their technologies to comply with emission standards or collaborate with environmental firms to outsource their carbon reduction efforts. These actions contribute to more significant reductions in carbon emissions, making environmental regulation a key moderating factor. Second, a city’s fiscal freedom can moderate the effectiveness of low-carbon city pilot policies. In cities with limited fiscal freedom, local governments may face pressures to relax environmental standards in order to attract investment, which can result in the relocation of polluting industries and an overall increase in regional carbon emissions. Conversely, cities with greater fiscal freedom benefit from a more competitive environment, where local governments with more financial resources can invest in green technologies, environmental projects, and stricter emission reduction policies. This creates an environment where emission reductions are more effective due to increased government investment and stronger enforcement of environmental regulations. Finally, the rate of population growth in a city can also influence the success of low-carbon city pilot policies. Rapid population growth increases both production- and consumption-related carbon emissions. However, it also presents opportunities for emission reductions, as a growing population drives demand for more energy-efficient technologies and services. Furthermore, a larger population results in increased human capital, which lowers the marginal cost of green and low-carbon transformations for businesses. Additionally, the growing demand for services in the tertiary sector (e.g., finance, technology) can support the development of low-carbon industries and drive further sustainable growth. In summary, environmental regulation, fiscal freedom, and population growth rate all serve as moderating factors that shape the effectiveness of low-carbon city pilot policies in reducing carbon emissions.
Hypothesis 3. Environmental regulation, fiscal freedom, and population growth rate moderate the effectiveness of low-carbon city pilot policies.
This section outlines the methodology employed in this study, including the technical roadmap, model construction, data description, and variable definitions. The methodology is designed to assess the impact of low-carbon city pilot policies on carbon dioxide emission intensity (CDEI) across Chinese cities. The section begins with a presentation of the technical approach, followed by the specifications of the model, data sources, and definitions of key variables used in the analysis.
The methodology adopted in this study employs a multi-period difference-in-differences (DID) model. This model is well-suited to estimate the causal effects of the low-carbon city pilot policies on carbon emission intensity (CDEI) by exploiting the variation in policy implementation across different cities over time. The data used in the analysis includes panel data from 283 prefecture-level cities in China, covering the period from 2005 to 2021. These data are sourced from reputable databases, including CSMAR, the “China Carbon Accounting Database,” and the “China City Statistical Yearbook.” Variable selection focuses on key factors related to carbon emissions, economic performance, and the implementation of low-carbon policies. The central variable of interest is the interaction between the low-carbon city pilot policy and carbon emission intensity (CDEI), which serves as the main dependent variable. The analysis includes several models to capture the effects of the policy, such as the baseline model (Equation 1) to estimate the overall impact of the policy on CDEI. In addition, mediation models (Equations 2 and 3) are developed to explore the mechanisms through which the policy affects emission intensity, with particular emphasis on factors such as carbon sink enhancement, technological innovation, and industrial structure upgrades. A moderation model (Equations 4 and 5) is also included to test the moderating effects of external factors, such as economic development, fiscal policies, and environmental regulations, on the effectiveness of the low-carbon city pilot policies. The empirical analysis follows a structured process, beginning with the estimation of the baseline DID model to assess the primary impact of the low-carbon city pilot policies. Subsequent steps include robustness checks and dynamic analysis to ensure the reliability and consistency of the results across different model specifications and data subsets. A comprehensive series of diagnostic tests is conducted to verify the validity of the findings, including tests for parallel trends, model stability, and potential endogeneity concerns. The technical roadmap for this study is presented in Figure 1, which illustrates the flow of the analysis, from model design and data collection to the final empirical results.
Figure 1. Research technical roadmap. Note: This figure illustrates the technical roadmap of the research, outlining the major steps and methodologies employed in the study. It begins with the identification of research objectives and the formulation of hypotheses, followed by data collection and pre-processing. The core analytical techniques, such as regression models, dynamic effect tests, and robustness checks, are then applied. The results are subsequently analyzed for causal relationships, with further tests for heterogeneity and mediation effects to ensure the robustness of the findings. The final step involves interpretation and policy implications based on the results.
This study evaluates the impact of China’s low-carbon city pilot policies on carbon emission intensity (CDEI) using a multi-period difference-in-differences (DID) model, which treats the policy implementation as a “quasi-natural experiment.” The low-carbon city pilot policy was introduced in three phases: 2010, 2013, and 2017, with the goal of progressively consolidating achievements in low-carbon city construction and promoting the adoption of low-carbon development models. By using panel data, we can observe changes in carbon emissions before and after the policy adoption within the same city, and compare cities that adopted the policy with those that did not at the same time points. To capture the impact of these policies, we use a DID model, with clustered robust standard errors at the city level, and control for both city-specific and time-specific fixed effects. The key dependent variable is carbon emission intensity (CDEI), and the model tests the effects of the low-carbon city pilot policy on CDEI over time, considering potential mediating and moderating factors.
The baseline model is designed to estimate the overall effect of the low-carbon city pilot policy on carbon emission intensity (CDEI). It is specified as follows:
Where: CDEIit is the carbon dioxide emission intensity for city i in year t α is the intercept. β is the coefficient of interest, representing the net or total effect of the low-carbon city pilot policy on emission intensity. A significantly negative β indicates that the policy significantly reduces carbon emission intensity. DIDit is a binary indicator for policy implementation, taking the value one if the policy was implemented in city i at year t, and 0 otherwise. γ represents the effect size of control variables on carbon emission intensity. Xit is the vector of time-varying control variables. μi and λt are city and time fixed effects, respectively. εit is the error term.
The mediation model is designed to investigate the pathways through which the low-carbon city pilot policy affects carbon emission intensity. The model adopts an improved causal stepwise regression method, following the approach by Wen Zhonglin et al. (2014), and tests the mediation effects using the Bootstrap method (Wen et al., 2004).
First, the baseline model (Equation 1) is used to estimate the total effect of policy implementation on CDEI. Then, a second equation is introduced to test the effect of policy implementation on the mediating variable:
Where: Mechit represents the mediating variable through which the policy impacts CDEI. β1 is the effect of the policy on the mediator. γ1 represents the effect size of control variables on the mediator.
Finally, the mediating variable is added to the baseline model to estimate both the direct effect of the policy on carbon emission intensity and the indirect effect through the mediator:
Where: β2 is the direct effect of the policy on emission intensity. δ is the effect of the mediating variable on carbon emission intensity. The interaction term β1*δ represents the indirect effect of the policy on carbon emission intensity via the mediator.
The moderation model examines how external factors, such as economic development, environmental regulations, and fiscal autonomy, moderate the effectiveness of low-carbon city pilot policies in reducing carbon emission intensity. A positive moderating effect is indicated when both the main effect of the policy (DID) and the interaction effect between the policy and the moderator are significant and have the same sign, while a negative moderating effect occurs when the signs are opposite. The moderation model is specified as follows:
First, the moderator variable is added to the baseline model to test the impact of both the policy and the moderator on carbon emission intensity:
Where: Zit represents the moderating variable. θ is the coefficient for the moderating variable.
Next, an interaction term between the policy and the moderating variable is introduced to test if the policy effect is moderated by the external factor:
Where: DIDit × Zit represents the moderating effect of the policy, testing whether the interaction between policy implementation and the moderator affects carbon emission intensity. β3 is the coefficient of the moderating effect.
This study uses panel data from 283 prefecture-level cities in China, spanning from 2005 to 2021. The data is sourced from reputable databases, including the CSMAR database, the China Carbon Accounting Database, and the China City Statistical Yearbook. To account for international currency fluctuations, Foreign Direct Investment (FDI) is converted to Chinese yuan using the annual average exchange rate between the United States dollar and the Chinese yuan. Nominal indicators, such as FDI and regional GDP, are adjusted to real terms using the GDP deflator, with 2005 as the base year. To mitigate potential measurement issues such as heteroscedasticity, logarithmic transformations are applied to key variables such as Carbon Dioxide Emission Intensity (CDEI), Pollution Control Capacity (PCC), Environmental Regulation (EL), and Population Density (PD). Data from autonomous prefectures are included, but regions such as Hong Kong, Macao, Taiwan, and Tibet are excluded from the analysis due to their distinct economic and administrative characteristics. Missing values in the dependent variable are filled using the moving average method, and missing data for other variables are interpolated where necessary. Descriptive statistics for each variable are presented in Table 1, offering an overview of the key characteristics and distribution of the data.
Carbon Dioxide Emission Intensity (CDEI): Unlike traditional carbon emission measures such as total CO₂ emissions or per capita CO₂ emissions, CDEI is defined as the logarithm of the ratio of total CO₂ emissions to regional GDP. This measure reflects carbon emissions per unit of GDP, providing insights into both environmental and economic impacts. For robustness checks, Per Capita CO₂ Emissions (PCCDE) is used as a supplementary variable, calculated as the logarithm of the ratio of total CO₂ emissions to the year-end population.
Implementation of Low-Carbon City Pilot Policies (DID): This is an interaction term between policy implementation and time, represented as a dummy variable. If a city adopts the policy, treat = 1; otherwise, treat = 0. The “period” variable equals 1 after the policy implementation and 0 before. The second batch of pilot cities began in 2013, with 2012 serving as the baseline for robustness testing. For cities that undergo multiple rounds of policy implementation, the earliest implementation date is used by default.
Carbon Sink Level (CSL): Measured by the ratio of green coverage area in built-up areas to the total land area of built-up areas. Increasing carbon sequestration is essential for reducing CO₂ emissions, as it enhances the capacity of urban areas to absorb carbon.
Technological Investment (TI): Measured by the ratio of scientific and technological investment to fiscal expenditure. Technological investment helps firms adopt cleaner technologies, thereby reducing CO₂ emissions through more efficient production methods.
Industrial Structure Upgrading (ISU): Measured by the ratio of GDP from the tertiary sector (services) to total regional GDP. The transition to service-based economies often promotes cleaner, more efficient industries, thus reducing carbon emissions.
Environmental Regulation (ER): Measured by the comprehensive utilization rate of industrial fixed waste. Environmental regulations, as proposed in the Porter Hypothesis, can stimulate technological innovation, improve resource efficiency, and reduce carbon emissions.
Fiscal Freedom Degree (FFD): Measured by the ratio of general budgetary revenue to general budgetary expenditure. High fiscal freedom provides local governments with more resources to invest in low-carbon initiatives. Low fiscal freedom may lead to fiscal pressures that could undermine the effectiveness of emission reduction policies.
Population Growth Rate (PGR): Measured by the natural population growth rate. As population growth can drive increased energy consumption and carbon emissions, it serves as a proxy for potential future emissions. It may also influence the scale of green transformations in urban areas.
Foreign Direct Investment (FDI): Measured by the ratio of actual foreign investment utilization to regional GDP. Increased foreign investment often brings advanced technology and capital that could influence carbon emissions.
Government Support Level (GSL): Measured by the ratio of general budgetary expenditure to regional GDP. Higher government expenditure may reflect greater investment in low-carbon technologies and environmental projects.
Economic Level (EL): Measured by the logarithm of total urban GDP per capita. Economic growth can reduce carbon emissions through economies of scale and technological innovations that drive energy efficiency.
Financial Development (FD): Measured by the ratio of financial loans to regional GDP. The development of the financial sector may facilitate investments in sustainable technologies and businesses that contribute to lower emissions.
Population Density (PD): Measured by the logarithm of the ratio of total population to the total land area. High population density can lead to more concentrated emissions due to higher levels of consumption and waste generation.
Pollution Control Capacity (PCC): Measured by the rate of harmless treatment of domestic waste. This variable captures the potential role of pollution control measures in reducing CO₂ emissions, as effective waste management can complement carbon reduction efforts.
This study employs a dynamic Difference-in-Differences (DID) model, which represents a methodological advancement over static models typically used in policy impact studies. The multi-period DID design captures both short-term and long-term effects of the low-carbon city pilot policy, offering a more comprehensive view of its impact on carbon emission intensity. This approach is essential for understanding the policy’s effectiveness over time, considering potential lag effects and changes in policy enforcement. Moreover, this study integrates mediation and moderation analysis, providing deeper insights into how the policy affects carbon emissions through mediators such as carbon sinks, technological innovation, and industrial structure upgrades. It also examines how external factors, such as environmental regulations and fiscal freedom, may moderate the effectiveness of the policy. By exploring these pathways and moderating factors, this study contributes to understanding not just the direct impact of low-carbon policies, but also the mechanisms that drive their effectiveness. Additionally, the study considers heterogeneity in policy impacts across cities with different economic development levels, geographical locations, and resource endowments. This regional variation is critical for tailoring low-carbon strategies to specific urban contexts, ensuring more targeted and effective policies. In conclusion, by addressing gaps in the existing literature and adopting a robust and dynamic methodological approach, this study provides valuable empirical insights into optimizing China’s low-carbon city pilot policies and advancing sustainable urban development.
The regression results examining the impact of the low-carbon city pilot policy on urban carbon emission intensity are presented in Table 2. The coefficients of the interaction terms for the core explanatory variables are negative and statistically significant at the 1% level, indicating a significant inhibitory effect of the policy on urban carbon emission intensity. In column (6), the coefficient of the DID term is significantly negative (−0.0482), suggesting that the policy implementation reduces carbon emission intensity by 4.82%. This result highlights the substantial emission reduction benefits of the policy, driven primarily by effective policy incentives and improvements in urban environmental governance. Specifically, the phased implementation of the pilot policy has reduced emissions through governmental compliance monitoring, restricting industrial practices, and promoting public awareness of low-carbon lifestyles. These findings show that the policy has successfully met its initial objective of emission reduction, illustrating the effectiveness of combining administrative measures with public engagement. No significant differences are observed across the models, except for variations in coefficient magnitudes, confirming the robustness of the baseline regression results.
Regarding control variables, government support significantly increases carbon emission intensity, likely reflecting that much of the government spending in many cities is directed towards industries, such as manufacturing, which are associated with higher emissions. In contrast, economic development level, fiscal freedom, and technological investment significantly reduce carbon emission intensity. Regions with higher economic development levels often serve as financial and commercial hubs, thus avoiding the concentration of highly polluting industries. Similarly, higher fiscal freedom allows local governments to resist relaxing environmental standards for attracting investment, preventing the entry of polluting firms. Additionally, greater technological investment enables firms to upgrade technologies, phase out outdated production processes, and innovate energy systems, driving industrial transformation and emission reduction. Other control variables are significant in columns (2) and (4), where time and individual fixed effects are excluded, but lose significance in column (6) with the inclusion of fixed effects. This suggests that the impact of these variables is likely influenced by unobserved factors. After controlling for fixed effects, their significance disappears, emphasizing the importance of a two-way fixed-effects model to account for unobserved heterogeneity and reduce estimation bias, thereby improving result reliability.
The Difference-in-Differences (DID) method is a classic approach for policy evaluation that addresses endogeneity issues arising from omitted variables. However, it relies on the assumption of parallel trends between the treatment and control groups. If this assumption is violated, pre-policy trend differences may bias the estimated results, affecting the accuracy of policy impact evaluations. To test this assumption, this study adopts the event study methodology and introduces interaction terms for the years before and after policy implementation. The test model is specified as follows:
Where, DIDitk represents the interaction term for the kth year before or after policy implementation, and βk represents the impact of the policy on urban carbon emission intensity in the kth year. Other variables remain unchanged. When k < 0, if βk is not significant, the parallel trends assumption holds. When k > 0, if βk is significant, it indicates dynamic effects following policy implementation. To visualize the results of the model (Equation 6), confidence interval plots for the parallel trends test are used. Before policy implementation, the confidence intervals for all periods should include zero, indicating no significant difference from zero, confirming the parallel trends assumption. After policy implementation, if the confidence intervals exclude zero, it signals significant dynamic effects; otherwise, it suggests no long-term impact.
To mitigate potential multicollinearity among the interaction terms, data for the current period in the treated areas are excluded, and data beyond six periods before and after the policy are merged into the sixth period. The parallel trends graph in Figure 2 shows that the coefficients for the six periods before policy implementation are positive but not significantly different from zero, indicating no significant difference in carbon emission intensity trends between the treatment and control groups prior to policy implementation, thus confirming the parallel trends assumption. For the six periods after policy implementation, the coefficients are significantly different from zero, indicating a notable dynamic effect of the policy. Furthermore, the coefficients are negative, suggesting that the policy significantly reduces carbon emission intensity in the treatment group. The absolute values of the coefficients initially increase, then decrease, and eventually stabilize, reflecting the lagged effect of the policy. As the policy is implemented, its effect gradually emerges and stabilizes over time, exerting a significant and sustained inhibitory impact on carbon emissions.
Figure 2. Parallel trend test graph. Note: This graph presents the confidence intervals used for testing the parallel trend assumption in a Difference-in-Differences (DID) analysis. If the confidence interval includes zero, it suggests that the pre-treatment trends of the treatment and control groups are similar, supporting the parallel trend assumption. If the confidence interval does not include zero, it indicates a significant difference in the trends between the two groups before the treatment, violating the parallel trend assumption.
To examine variations in policy effects across different implementation batches, we define the first batch as the period from 2010 to 2012 (DID1), the second batch from 2013 to 2016 (DID2), and the third batch from 2017 to 2021 (DID3). The dynamic effects test results for these three stages are presented in Table 3. The results in column 2 of Table 3 show that the policy effect in the first batch is not statistically significant, though it exhibits an inhibitory tendency. This could be due to the lagged nature of policy implementation, where the effects take time to materialize, and the relatively short duration of the first batch, which limited its visibility. In contrast, the policy effects in the second and third batches are significantly negative, indicating that the low-carbon city pilot policy effectively reduced carbon emission intensity during these phases. However, the inhibitory effect in the third batch is weaker than in the second batch, possibly due to the shorter duration of enforcement or the larger number of cities involved in the third batch. This larger group of cities may have caused disparities in policy execution, with some cities engaging in “free-riding” behavior, which weakened the overall policy impact. From a policy perspective, these findings provide several key insights. First, the observed lag in the policy’s effects underscores the importance of sustained efforts and monitoring during the early stages of policy implementation. Second, the stable and significant inhibitory effects in the second batch highlight the need for balancing the scale and scope of policy implementation. Finally, addressing the issue of free-riding in later batches may require strengthening accountability mechanisms and tailoring policy measures to better align with local conditions, ensuring more effective execution.
To ensure the robustness of the estimated policy effects and eliminate potential biases from omitted variables or unobservable factors, placebo tests were conducted from both temporal and spatial perspectives. First, in the time placebo test, we examined whether the observed policy effects were due to systematic differences in time trends between the treatment and control groups, rather than the actual implementation of the low-carbon city pilot policy. Following the event-study framework, we artificially advanced the policy implementation by 2, 3, 4, and 5 years, creating counterfactual scenarios. The results, presented in Table 3, show that none of the artificially advanced policies were significant at the 5% or 10% levels. This indicates that the counterfactual policy effects are insignificant, supporting the conclusion that there are no systematic differences in time trends between the groups. Thus, the observed inhibitory effects can be attributed to the actual implementation of the low-carbon city pilot policy, rather than spurious correlations or pre-existing trends. Second, a city placebo test was conducted to address the potential influence of unobservable city-level factors or omitted variables. Unlike traditional placebo tests using dummy treatment groups, our approach randomly assigned the DID term to cities, accounting for both temporal and spatial differences. Figure 3 shows the results from 1,000 random draws, with fake regression coefficients on the left and fake p-values on the right. The placebo coefficients are tightly clustered around zero and are statistically insignificant, contrasting sharply with the significant coefficients in the baseline regression. This indicates that the placebo test successfully rules out the possibility of unobserved city-level factors or model misspecification biasing the estimated policy effects. In conclusion, the time and city placebo tests strongly confirm the robustness of the estimated impact of the low-carbon city pilot policy on carbon emission intensity.
Figure 3. Results of city placebo test. Note: This figure shows the results of the placebo test conducted at the city level. It evaluates whether the estimated treatment effect on carbon dioxide emission intensity is driven by random variation in the data rather than the actual policy intervention. The placebo test is applied by assigning the treatment to cities that did not receive the policy intervention. If the treatment effect is significantly different from zero in the placebo test, it would suggest that the observed effect may not be causal. Conversely, if the effect is close to zero and statistically insignificant, it supports the validity of the treatment effect estimated in the main analysis.
To address potential endogeneity issues arising from reverse causality or non-random selection bias, this study employs a multi-period difference-in-differences (DID) model combined with propensity score matching (PSM) as a robustness check. The primary PSM-DID regression uses 1:3 nearest neighbor matching with a caliper of 0.05. Robustness is further tested using alternative matching methods, including nearest neighbor matching, 1:3 nearest neighbor matching with a caliper of 0.01, radius caliper matching with a caliper of 0.01, kernel density matching, and local linear matching. The regression results in Table 4 show that all coefficient values are significantly negative across different matching methods. This confirms the robustness of the PSM-DID results, supporting the conclusion that the low-carbon city pilot policy significantly reduces urban carbon emission intensity. The consistency of negative coefficients across various matching techniques emphasizes the policy’s tangible impact on emission reductions. These results reinforce the validity of the baseline findings, confirming the success of the policy in achieving its environmental objectives. Moreover, the combination of PSM and DID models helps mitigate biases from unobservable factors and selection effects. The findings suggest that cities selected for the low-carbon city pilot program effectively utilized policy interventions to reduce carbon emissions. This highlights the importance of precise targeting and systematic implementation of environmental policies. Policymakers should continue identifying high-carbon cities and tailor pilot policies to local contexts to maximize their effectiveness. Furthermore, the robustness of the PSM-DID results suggests that expanding the program to more cities could yield similar emission reduction benefits while promoting sustainable urban development.
To address potential endogeneity issues, such as reverse causality and omitted variables, this study employs two methods. First, the lagged control variable method is used. Due to the difficulty of identifying a perfect instrumental variable, this study follows prior research by lagging all control variables by one period. This helps reduce bias caused by simultaneous causality between the policy and the outcomes. Second, the predetermined variable method is introduced. Recognizing that initial differences in carbon emission intensity across cities might affect the policy’s effectiveness, the study includes an interaction term of lagged carbon emission intensity and time trend as a predetermined variable, controlling for the impact of initial emission levels. The results in Table 5 show that the coefficient of the core explanatory variable (DID) remains significantly negative even after applying these methods. This confirms that the low-carbon city pilot policy continues to significantly reduce carbon emission intensity, and the results are robust to concerns about endogeneity. These findings suggest that the policy consistently reduces carbon emission intensity, even when accounting for issues like reverse causality and omitted variables. This strengthens the conclusion that the policy has long-term and sustainable impacts. These results provide strong evidence that well-implemented low-carbon policies can effectively reduce emissions. Policymakers should continue to prioritize low-carbon pilot zones and expand such initiatives while considering the impact of initial carbon emission levels in future policy design. Additionally, the findings highlight the importance of addressing endogeneity concerns in policy evaluation to ensure that observed effects are attributed to the policy itself, not confounding factors.
Since the selection of low-carbon pilot cities may not be random, this study investigates the impact of inherent differences in economic development and industrial bases on the selection process. Cities with lower initial carbon emission intensity are more likely to be chosen as pilot cities, as they are better aligned with the policy’s goals. Furthermore, city-specific characteristics may affect carbon emission intensity differently over time, potentially leading to estimation bias. To address these issues, city-specific dummy variables and their interaction terms with a polynomial time trend are incorporated into the baseline regression model. The model is specified as follows:
Where Kc represents city-specific attributes, such as whether a city is in a “two-control zone,” a pollution prevention pilot area, or a special economic zone, and f(t) is a polynomial time trend with linear and quadratic terms. The term Kc⋅f(t) accounts for how city attributes influence carbon emission intensity over time.
The regression results for the non-random selection model (Equation 7) in Table 5 show that even after controlling for city-specific attributes and their time-varying effects, the coefficient of the core explanatory variable (DID) remains significantly negative. This result is consistent with the baseline regression findings and provides robust evidence of the policy’s effectiveness in reducing carbon emission intensity. These results indicate that the selection of low-carbon pilot cities is influenced by initial factors such as economic and industrial characteristics. However, non-random selection does not undermine the policy’s overall effectiveness. Policymakers should be aware of potential biases in city selection to ensure a fair and comprehensive evaluation of the policy’s impact. Addressing these selection biases in future initiatives can help ensure a more equitable distribution of resources and policy benefits across cities. Furthermore, the findings suggest that policies targeting cities with distinct economic and industrial profiles may require tailored implementation strategies. Policymakers should consider designing complementary measures to address structural challenges in cities with higher initial carbon intensities, ensuring that low-carbon initiatives have a broader and more inclusive impact.
Since the implementation of the low-carbon city pilot policy may overlap with other policies in terms of timing and geographical scope, this study includes dummy variables for relevant policies to control for potential confounding effects. These dummy variables represent whether a city is a pilot city for “carbon emissions trading” (DID4), a key area for air pollution prevention and control (DID5), or a pilot city for pollutant discharge rights trading (DID6). By incorporating these variables, we can assess the impact of the low-carbon city pilot policy while accounting for the influence of concurrent policies. The regression results in Table 5 show that the coefficient of the core explanatory variable (DID) remains significantly negative across all models. This suggests that even with the presence of other concurrent policies, the low-carbon city pilot policy continues to effectively reduce urban carbon emission intensity. This finding strengthens the robustness of the baseline regression results, indicating that the policy is a key instrument for emission reduction, independent of other environmental and regulatory measures. These results confirm that the low-carbon city pilot policy is an effective and independent tool for reducing carbon emissions, even when other related policies are simultaneously implemented. Notably, the policy’s sustained effectiveness, despite the concurrent implementation of carbon emissions trading and air pollution control measures, suggests that the integration of multiple environmental policies can complement rather than undermine the overall emission reduction goal. This highlights the importance of policy coherence and synergy in addressing urban environmental challenges. The findings encourage further expansion of the low-carbon city pilot policy and emphasize the value of aligning it with other concurrent policies. Furthermore, these results underscore the need for a holistic approach to urban environmental governance, where various policies work in tandem to create a more sustainable future.
To further enhance the credibility and robustness of the empirical findings and minimize the influence of confounding factors, this study conducts additional robustness tests. These include excluding municipalities from the sample, shortening the sample period to 2008–2019, adjusting the second batch policy implementation year to 2012 (DID7), replacing the dependent variable with per capita carbon dioxide emissions, and performing two-tailed trimming and censoring at the 1% and 99% quantiles. The results in Table 6 show that the coefficients of the core explanatory variables remain significantly negative across all models, indicating that after applying these robustness procedures, the policy’s effects remain stable. This further confirms the robustness of the baseline regression results. These robustness tests strengthen the conclusion that the low-carbon city pilot policy significantly reduces urban carbon emission intensity. The consistent negative coefficients across various robustness checks reinforce the validity of the original findings, suggesting that the policy’s effectiveness is not sensitive to model specifications or sample variations. This consistency enhances the policy’s credibility and provides a solid foundation for its continued implementation. The findings have several important policy implications. First, the policy’s strong effectiveness across various tests suggests its potential for long-term success in achieving carbon reduction goals. Second, the consistency of results across different robustness checks indicates that the policy can be applied to cities with varying characteristics. This supports the case for scaling up the policy to other cities or regions that have not yet implemented low-carbon initiatives. Furthermore, the robustness tests using different outcome measures, such as per capita carbon emissions, further support the policy’s effectiveness in reducing urban carbon intensity. These findings suggest that expanding low-carbon initiatives to more cities while refining implementation could enhance national and regional efforts to combat climate change.
To explore the indirect effects of the low-carbon city pilot policy through three channels—enhancing carbon sink levels, fostering technological investment, and upgrading industrial structures—this study employs the bootstrap method. The results are presented in Table 7. According to the bootstrap method, if the indirect effect is not significant, there is no mediation effect; if both the indirect and direct effects are significant and have the same sign, this indicates a partial mediation effect, with the mediation proportion calculated as the ratio of the indirect effect to the sum of the direct and indirect effects.
In column (1) of Table 7, we observe that the indirect effect of carbon sink levels on reducing carbon emission intensity is statistically significant at the 1% level (−0.72%), with the direct effect also significant at the 1% level (−15.52%). This indicates a partial mediation effect, with the mediation proportion standing at 4.43%. In other words, 4.43% of the reduction in carbon emission intensity resulting from the pilot policy can be attributed to improvements in carbon sink levels. This reflects the policy’s success in strengthening investments and management in carbon sink projects, such as promoting “green cities” and expanding carbon sequestration areas, which enhance the ecosystem’s ability to absorb CO2. Similarly, column (3) reveals that industrial structure upgrades also contribute to a partial mediation effect on carbon emission intensity reduction. The indirect effect is 1.22%, and the direct effect is 15.64%, with the mediation proportion being 7.24%. This suggests that the policy’s success in reducing carbon emission intensity is, in part, due to its role in upgrading industrial structures, shifting energy consumption patterns, and reducing the share of high-pollution industries. However, as shown in column (2), technological investment does not exhibit a significant indirect effect. While the direct effect of technological investment on carbon emission intensity is significant at the 1% level (−17.03%), the lack of a mediation effect suggests that technological investment does not play a mediating role. Therefore, the reduction in carbon emission intensity resulting from the policy cannot be attributed to increased technological investment. The absence of a mediation effect may be due to factors such as bottlenecks in the development of critical technologies or the failure to capture green innovation aspects of technological investments in the model. In conclusion, these findings suggest that the low-carbon city pilot policy reduces carbon emission intensity primarily through enhanced carbon sink levels and industrial structure upgrades, rather than through technological investment. These results provide empirical support for the hypothesized mediation mechanisms of the policy.
Table 8 presents the results of the moderation effect analysis, which examines how environmental regulations, fiscal freedom, and population growth rate influence the effectiveness of the low-carbon city pilot policy. Columns (1), (3), and (5) present the regression results for the independent variable (DID), the moderating variables, and their interaction terms, while columns (2), (4), and (6) show the results after centering the variables.
From column (2), we infer that both the main effect of DID and the coefficient of the interaction term for environmental regulations (c_DID_c_ER) are significant but have opposite signs. This indicates that environmental regulations do not significantly enhance the inhibitory effect of the pilot policy on carbon emission intensity. In fact, they exhibit a significant negative moderation effect, meaning that stronger environmental regulations in a city do not necessarily align with the low-carbon pilot policy’s objectives and may even reduce its effectiveness. This could be due to a mismatch between the existing regulatory framework and the policy’s goals. To address this, it is recommended to strengthen the enforcement of environmental regulations and improve their alignment with low-carbon policies. Additionally, fine-tuning implementation details and increasing penalties for non-compliance could ensure better coordination between environmental, fiscal, and economic policies. In contrast, column (4) reveals that both the main effect of DID and the interaction term for fiscal freedom degree (c_DID_c_FFD) are significant and have the same sign, indicating a positive moderation effect. This shows that cities with greater fiscal freedom are more effective in implementing green low-carbon policies. Higher fiscal freedom enables local governments to better allocate resources, provide more financial support for environmental protection, and ultimately achieve the dual goals of sustainable development and environmental conservation. The positive moderation effect of fiscal freedom supports existing theories suggesting that fiscal autonomy enables more flexible and effective policy implementation. Similarly, column (6) demonstrates that population growth rate also exerts a significant positive moderation effect. Cities with higher population growth rates exhibit more significant reductions in carbon emission intensity due to the low-carbon city pilot policy. Rapid population growth increases resource demand, which in turn encourages governments and businesses to prioritize environmental protection and low-carbon technologies. This creates a more conducive environment for the successful implementation of the pilot policy. In conclusion, the findings suggest that the effectiveness of the low-carbon city pilot policy is enhanced when cities have weaker environmental regulations, greater fiscal freedom, and faster population growth. These results provide strong support for the conjecture that the moderation effects of these factors play a significant role in influencing the success of the policy.
This study explores economic level heterogeneity in two ways. First, cities are grouped by their administrative hierarchy, with municipalities directly under the central government and vice-provincial cities categorized as non-prefecture-level cities. Columns (1) and (2) of Table 9 show significant differences in the effects of the pilot policy across cities with different administrative levels. Specifically, non-prefecture-level cities show better performance compared to prefecture-level cities. Second, based on the “2022 City Business Attractiveness Rankings,” cities are divided into high, medium, and low economic level groups, using third-tier and fourth-tier cities as thresholds. Columns (3) to (5) of Table 9 indicate that the higher the economic development level of the city, the better the effect of the pilot policy. The results from both approaches are consistent, suggesting that cities with higher administrative ranks and better business attractiveness tend to achieve better outcomes with the pilot policy. This can be attributed to several factors. First, higher-ranking cities generally have better access to resources, superior infrastructure, and more favorable policies, all of which facilitate low-carbon initiatives. Additionally, these cities attract more foreign investment and high-tech enterprises, benefiting from technological innovation and spillover effects that reduce carbon emissions. Furthermore, they often have more advanced industrial structures, particularly in the service sector, where industries such as finance are cleaner and more environmentally friendly. In contrast, cities with lower economic development levels are often more industrialized, with high-pollution, high-energy-consuming, and high-emission industries, which hinder reductions in carbon emission intensity. These findings have important policy implications. They suggest that low-carbon pilot policies are likely to be more effective in cities with higher administrative levels and stronger economic foundations, especially those with a more diversified and environmentally friendly industrial structure. To maximize the policy’s effectiveness, it would be beneficial to direct resources and interventions toward cities with lower economic levels, helping them improve their industrial structures and attract cleaner technologies.
This study explores geographical location heterogeneity by dividing China into four regions: Eastern, Central, Western, and Northeastern, based on the classification of the National Bureau of Statistics. As shown in Table 10, columns (1) to (4), the pilot policy yields the best results in the Western region, followed by the Central region, with the weakest results observed in the Eastern region. The effects in the Northeastern region are not significant. This pattern suggests that the effectiveness of the pilot policy declines from West to East. One reason for this is that the Western and Central regions are key areas for energy development, utilization, and industrial upgrading, offering more potential for emission reduction. In contrast, the Eastern region already has a relatively well-structured industrial layout and more advanced government policies, which may limit the improvement potential between pilot and non-pilot cities. Furthermore, the study divides regions into North and South based on the Qinling-Huaihe Line. As shown in Table 10, columns (5) and (6), the policy effects are significantly better in the Southern region compared to the Northern region. This suggests a gradual decline in the effectiveness of the pilot policy from South to North. The Northern region is characterized by higher carbon emissions from heavy industries, making significant reductions more challenging. This highlights the regional disparities in industrial structure and emission profiles, which influence the policy’s success. In summary, the pilot policy shows decreasing effectiveness from the Western to the Eastern regions and from the Southern to the Northern regions. These regional differences emphasize the need to tailor policies to local contexts. In regions with higher emission intensities or more room for industrial upgrading (such as the West and South), the policy is more effective. In contrast, regions with more balanced industrial structures (such as the East and North) may require more targeted interventions to achieve significant reductions in carbon emission intensity.
This study further examines the impact of resource endowments on the effectiveness of the pilot policy by dividing the sample into resource-based and non-resource-based cities. Resource-based cities are further categorized into growth, mature, fading, and rejuvenation types, according to the “National Sustainable Development Plan for Resource-based Cities (2013–2020).” The results presented in Table 11, columns (1) and (2), show that resource endowments significantly influence the policy’s effectiveness, with non-resource-based cities generally performing better than resource-based cities. In particular, columns (3) to (6) reveal that only rejuvenation-type resource-based cities experience a significant reduction in carbon intensity after the implementation of the pilot policy. This suggests that the success of the pilot policy is closely tied to the developmental stage of resource-based cities. Cities in the “growth” or “mature” stages, with their dependence on resource extraction and heavy industries, face limitations in reducing carbon emission intensity. In contrast, “rejuvenation-type” resource-based cities, undergoing industrial transformation and restructuring, create a more favorable environment for the success of low-carbon policies. These findings highlight the need for tailored policy approaches, considering the specific resource endowments and industrial structures of cities. Rejuvenation-type cities, focusing on industrial upgrading and environmental improvement, are more likely to benefit from low-carbon policies, while growth and mature resource-based cities may require additional measures to diversify their economies and reduce reliance on high-emission industries.
This study investigates the heterogeneity of the pilot policy’s effects across cities with different industrial foundations. The sample of 120 cities is divided into “old industrial base cities” based on the “State Council’s Plan for Adjusting and Transforming Old Industrial Bases (2013–2022).” Among these, 95 are prefecture-level cities, and 25 are non-prefecture-level cities. The results presented in Table 12, columns (1) and (2), show that the policy’s effects are significantly stronger in old industrial base cities compared to non-old industrial base cities. Further analysis in columns (3) and (4) shows that the policy effects are even more pronounced when old industrial base cities are classified as non-prefecture-level cities. This finding aligns with the previous analysis on administrative levels, suggesting that higher administrative levels contribute to more effective policy implementation. The better performance of old industrial base cities can be attributed to several factors. These cities tend to have more mature industrial systems and well-established production processes, which facilitate the rapid implementation of necessary upgrades and technological innovations to reduce emissions. In contrast, non-old industrial base cities may lack the same industrial infrastructure and experience in rapid transformation, making the implementation of low-carbon policies more challenging. Additionally, old industrial base cities, particularly those with higher administrative levels, are more likely to have earlier access to pilot policy programs, resulting in more noticeable and significant reductions in carbon emission intensity.
This study examines the impact of population differences on the effectiveness of the low-carbon city pilot policy from two perspectives. First, cities are classified based on their location relative to the Hu Huanyong Line, which divides China into regions with varying population densities. According to the results in Table 12, columns (5) and (6), cities east of the Hu Huanyong Line (i.e., those with higher population density) show significantly better policy effects compared to cities to the west. This suggests that higher population density contributes to better policy outcomes, likely due to the greater opportunities for energy efficiency improvements and public transportation use, both of which are key drivers of carbon reduction. Second, cities are categorized by population size, with large cities defined as those exceeding a specific population threshold, as outlined in the “Notice on Adjusting City Size Classification Standards.” The results in columns (7) and (8) of Table 12 show that large cities exhibit significantly better policy effects compared to medium and small cities. This reflects the pioneering role of large cities in implementing low-carbon policies, often serving as leaders and models for others. These findings are likely driven by the higher carbon emissions associated with larger populations, especially in urban areas where population and economic activity are concentrated, leading to higher energy consumption. In large cities, policy interventions, such as energy-efficient housing, public transportation, and green infrastructure, can be more effectively implemented due to their scale and available resources. Additionally, the population density and urbanization in large cities enable more impactful reductions in both residential and transportation-related emissions.
The impact of low-carbon city pilot policies may vary significantly across different regions of China. Due to substantial regional disparities in economic development, industrial composition, and population density, the effects observed in cities with high economic activity, such as those in the eastern and coastal regions, may not be directly applicable to cities in the western or less-developed areas. Cities in the eastern regions generally benefit from advanced infrastructure, higher population densities, and greater financial resources to implement low-carbon measures, which may result in more substantial reductions in carbon emission intensity. In contrast, cities in the western regions face challenges such as limited resources, slower industrial upgrading, and lower urbanization rates, which could impede the effectiveness of low-carbon policies. However, the core mechanisms identified in this study—such as enhancing carbon sink capacity, fostering technological innovation, and upgrading industrial structures—are likely to be applicable across different regions. Although the relative impacts of these mechanisms may vary depending on local contexts, the underlying principles of the policies remain relevant. Therefore, while the core components of the policy may be applicable nationwide, their effectiveness will depend on regional characteristics and may require tailored approaches to address the specific challenges of each region.
The findings of this study are also relevant to other developing countries facing similar challenges, such as rapid urbanization and increasing carbon emissions. Many countries in Asia, Africa, and Latin America are experiencing urban growth and industrial expansion, both of which contribute to higher carbon emissions. The policy mechanisms examined in this study—such as strengthening environmental regulations, enhancing fiscal autonomy, managing population growth, and promoting a shift toward low-carbon industries—are applicable in similar contexts within these regions. However, the effectiveness of these measures may be influenced by local factors, such as governance structures, economic conditions, and levels of public awareness. For instance, public participation and environmental awareness may play a more significant role in some countries than in others, thereby influencing the success of these policies. Additionally, the capacity of local governments to implement low-carbon initiatives and the level of technological advancement in these countries may differ, which could impact the effectiveness of the policies.
Despite the potential relevance of the findings to other regions or countries, there are certain limitations in the generalizability of the results. The institutional, political, and economic context in China is unique and may not be directly comparable to other countries. For example, the strong central government control and coordinated policy-making in China have played a crucial role in the success of the low-carbon city pilot policies. In countries with weaker institutional frameworks or different governance structures, the implementation and outcomes of similar policies may differ significantly. Moreover, variations in the availability of resources, technological capabilities, and public awareness in other countries could affect the implementation of these policies. For instance, countries with underdeveloped infrastructure or lower levels of public awareness may face challenges in achieving the same success in reducing carbon emissions. Therefore, while the mechanisms explored in this study offer valuable insights, their applicability to other regions and countries requires careful consideration of the specific local conditions and challenges. Future research should examine how these mechanisms apply in different contexts and assess the factors that may influence the effectiveness of such policies outside of China.
This study emphasizes the importance of evaluating low-carbon city pilot policies, particularly in the context of China’s ambitious “carbon peaking” and “carbon neutrality” goals. Using data from 283 cities spanning from 2005 to 2021, we employed a multi-period difference-in-differences (DID) approach to assess the impact of the low-carbon city pilot policies on carbon emission intensity. Multiple robustness checks, including dynamic effects, placebo tests, and matching techniques, further validate the results. Additionally, we explored the underlying mechanisms and heterogeneity of the policy’s effects across various urban contexts. The main findings of this study are as follows.
(1) Significant Reduction in Carbon Emission Intensity: The low-carbon city pilot policy has led to a significant reduction in carbon emission intensity, with the policy’s effects strengthening and stabilizing over time. Although some cities show “free-rider” behavior, robustness checks confirm the validity of the results.
(2) Mediation Mechanisms: The policy reduces carbon intensity primarily through two channels: enhancing carbon sinks (contributing 4.3% to the reduction) and upgrading industrial structures (contributing 7.1%). However, the impact of technological investment remains minimal, suggesting that greater efforts are needed to scale up green technological innovations.
(3) Policy Moderation Effects: Local environmental regulations negatively moderate the policy’s effectiveness, while fiscal autonomy and population growth rates positively influence the outcomes. This underscores the importance of balanced local governance in maximizing the policy’s impact.
(4) Heterogeneity in Policy Effects: The policy’s effectiveness varies across cities depending on economic development, geographical location, industrial foundation, resource endowment, and population size. Cities with higher economic development, more favorable locations (west and south), stronger industrial bases, and larger populations tend to experience greater reductions in carbon intensity.
Based on the above conclusions, we provide the following policy recommendations.
(1) Strengthen Policy Evaluation Systems: The findings highlight the importance of continuous and comprehensive evaluation of low-carbon city pilot policies. Given the success of the pilot policies in reducing carbon emission intensity, a gradual and expanding approach should be adopted. This involves scaling successful practices from pilot cities to broader regions, while establishing a feedback mechanism for ongoing policy improvement. Local governments should implement robust post-policy performance evaluation systems to monitor long-term effects and ensure sustained carbon reduction. Additionally, measures should be taken to prevent free-rider behavior in cities that benefit from the policy without contributing to its success. The focus should be on stabilizing and sustaining the policy’s dynamic effects to achieve long-term carbon reduction goals.
(2) Enhance Mediation Mechanisms for Carbon Reduction: In order to deepen carbon emission reductions, policies should prioritize enhancing carbon sinks and promoting industrial transformation. To this end, carbon sink enhancement, such as accelerating reforestation and land restoration, should become a core strategy. These efforts can significantly improve the natural carbon storage capacity of ecosystems, thus contributing to long-term emission reductions. Furthermore, industrial upgrading is essential for achieving low-carbon development. Policies should support the transition to low-carbon industries, especially through technological innovation in carbon capture, utilization, and storage (CCUS) technologies. Given the minimal impact of technological investments observed in this study, greater efforts are needed to scale up green technologies and foster innovation in low-carbon solutions. Additionally, local governments should provide targeted incentives for industries to adopt low-carbon production methods, encouraging them to reduce their carbon footprint and shift towards more sustainable practices.
(3) Focus on Modulatory Mechanisms: The study indicates that fiscal autonomy, population growth, and environmental regulations can either amplify or undermine the policy’s effectiveness. To maximize the impact of low-carbon policies, local governments should strengthen environmental regulations to align them better with low-carbon objectives. This may involve introducing higher taxes on carbon-intensive activities and offering subsidies for green technologies and energy-efficient practices, which can incentivize businesses to transition to low-carbon models. At the same time, fiscal autonomy should be carefully managed to prevent the crowding-out effect, where excessive fiscal pressures inadvertently lead to increased carbon emissions. Setting appropriate thresholds for fiscal freedom will help ensure that financial resources are allocated efficiently and sustainably. Lastly, encouraging population growth in cities through migration policies can leverage economies of scale and human capital benefits. Larger cities tend to have more resources and better infrastructure to implement green initiatives, and higher population density can make public transport, waste management, and energy use more efficient. Therefore, policies that facilitate migration to pilot cities can enhance the overall success of low-carbon initiatives.
(4) Tailored Approaches for Diverse Urban Contexts: The study demonstrates that low-carbon city policies are more effective in cities with higher economic development, stronger industrial bases, and larger populations. Therefore, tailored approaches are necessary to ensure the policy’s success across various urban contexts. The central government should prioritize cities with strong industrial bases, higher economic development, and favorable geographic locations (e.g., southwestern regions) for the initial phase of policy implementation. These cities can serve as models for successful policy deployment. For cities facing specific challenges, such as resource-based cities or those with lower economic development, a phased implementation approach should be adopted. Policies should be customized to suit the local conditions and gradually expand to other regions as cities build their capacity for low-carbon transformation. Moreover, policy assessments should be comprehensive, considering factors like industrial structure, resource endowment, and population demographics. This will help avoid unintended negative consequences in specific contexts and ensure that policies are effective in promoting sustainable urban development.
While this study provides important insights into the effectiveness of China’s low-carbon city pilot policies, several limitations remain.
(1) Limited Scope of Mediating Factors: This study focuses on a few key mediation channels, but future research could examine additional factors such as local innovation systems, public-private partnerships, and social capital. Understanding the role of these factors could provide a more comprehensive view of how low-carbon transitions unfold in various urban contexts.
(2) Barriers to Technological Investment: The limited impact of technological investments suggests that future studies should explore the barriers to scaling low-carbon technologies, particularly in urban environments. Research could focus on how innovation contributes to emission reductions and identify strategies to accelerate technological development and investment, especially in renewable energy and carbon capture technologies.
(3) Regional Disparities: Future research should investigate how low-carbon city policies can be tailored to regions facing specific challenges. For example, northern cities may be more reliant on coal, while resource-based cities could experience “carbon lock-in” effects. Longitudinal studies could assess the long-term sustainability of low-carbon city policies and how these policies evolve in different regions over time.
(4) Behavioral and Social Factors: Further studies could investigate the behavioral and socio-political factors influencing the success or failure of low-carbon city policies. This could include examining the role of public awareness, citizen engagement, and local leadership in driving successful transitions to a low-carbon economy.
(5) Generalizability of Findings: The findings of this study are based on data from 283 Chinese cities and may not fully apply to other regions or countries with different institutional, economic, or environmental conditions. Future research should explore the generalizability of these findings to other regions, particularly developing countries or cities with varying levels of industrialization and resource endowment. Understanding the context-specific factors that influence the success of low-carbon policies is critical for scaling up similar initiatives globally.
In conclusion, while this study highlights the effectiveness of low-carbon city pilot policies in reducing carbon emission intensity, achieving China’s long-term carbon neutrality goals will require policies that consider regional and urban diversity. Future research will be crucial in refining these policies, overcoming implementation barriers, and ensuring that low-carbon transitions are inclusive and sustainable across different urban contexts.
The original contributions presented in the study are included in the article/supplementary material, further inquiries can be directed to the corresponding author.
J-XS: Conceptualization, Funding acquisition, Project administration, Supervision, Writing–review and editing. J-JP: Data curation, Formal Analysis, Investigation, Methodology, Resources, Software, Writing–original draft, Writing–review and editing. JZ: Conceptualization, Methodology, Validation, Visualization, Writing–review and editing.
The author(s) declare that financial support was received for the research, authorship, and/or publication of this article. This work was supported in part by a grant from the Major Scientific Research Project of Anhui Provincial Colleges and Universities, titled “Research on Fiscal and Financial Coordination Policies to Support the Development of Carbon Finance” (Approval No. 2022AH040082), and the Postgraduate Research Innovation Fund of Anhui University of Finance and Economics, titled “Impact Assessment and Effect Study of Carbon Subsidy Policies on Carbon Emissions” (Approval No. ACYC2022387), and the Key research projects of Anhui University of Finance and Economics (Approval No. ACKYB23012).
We sincerely thank the handling editor and other reviewers of this journal for their insightful comments. During the preparation of this work, the authors used OpenAI’s ChatGPT (version: GPT-4 model) to improve the language. After using this tool, the authors reviewed and edited the content as needed and take full responsibility for the content of the publication.
The authors declare that the research was conducted in the absence of any commercial or financial relationships that could be construed as a potential conflict of interest.
All claims expressed in this article are solely those of the authors and do not necessarily represent those of their affiliated organizations, or those of the publisher, the editors and the reviewers. Any product that may be evaluated in this article, or claim that may be made by its manufacturer, is not guaranteed or endorsed by the publisher.
Böhringer, C., and Welsch, H. (2003). Contraction and Convergence of carbon emissions: an intertemporal multi-region CGE analysis. J. Policy Model. 26, 21–39. doi:10.1016/j.jpolmod.2003.11.004
Cai, B. F., Bo, X., Zhang, L. X., Boyce, J. K., Zhang, Y. S., and Lei, Y. (2016). Gearing carbon trading towards environmental co-benefits in China: measurement model and policy implications. Glob. Environ. Change 39, 275–284. doi:10.1016/j.gloenvcha.2016.05.013
Cai, D. L., Yan, Y., and Cheng, S. L. (2019). Research on the impact of carbon emission subsidies and carbon taxes on environmental quality. China Popul. Resour. Environ. 29 (11), 59–70. doi:10.12062/cpre.20190615
Cao, X. Y., and Zhang, J. F. (2018). Supply chain carbon reduction decision optimization and coordination under carbon reduction subsidies and carbon taxes. Operations Res. Manag. 27 (04), 57–61. doi:10.12005/orms.2018.0085
Chen, H., Guo, W., Feng, X., Wei, W. D., Liu, H. B., Feng, Y., et al. (2021). The impact of low-carbon city pilot policy on the total factor productivity of listed enterprises in China. Resour. Conservation and Recycl. 169, 105457–105457. doi:10.1016/J.RESCONREC.2021.105457
Cheng, F. X., Shao, S. L., Xu, L. F., and Sun, L. C. (2015). Research on the optimal strategy for proactive carbon reduction by enterprises based on government subsidies. China Popul. Resour. Environ. 25 (07), 32–39. doi:10.3969/j.issn.1002-2104.2015.07.005
Fan, L., Peng, B. B., Lin, Z. G., Zou, H. Y., and Du, H. B. (2024). The effects of green finance on pollution and carbon reduction: evidence from China’s industrial firms. Int. Rev. Econ. Finance 95, 103490. doi:10.1016/J.IREF.2024.103490
Ganda, F. (2021). The non-linear influence of trade, foreign direct investment, financial development, energy supply and human capital on carbon emissions in the BRICS. Environ. Sci. Pollut. Res. Int. 28 (41), 57825–57841. doi:10.1007/S11356-021-14704-W
Guan, H., and Liu, K. (2022). Research on the evaluation and heterogeneity of low-carbon city policies under the “dual carbon” background: based on a quasi-natural experiment with multi-point DID analysis. J. Shandong Univ. Finance Econ. 34 (04), 15–27+38. doi:10.3969/j.issn.1008-2670.2022.04.003
He, Q. C. (2015). Fiscal decentralization and environmental pollution: evidence from Chinese panel data. China Econ. Rev. 36, 86–100. doi:10.1016/j.chieco.2015.08.010
Huang, J. C., Zhao, J., and Cao, J. E. (2021). Environmental regulation and corporate R&D investment—evidence from a quasi-natural experiment. Int. Rev. Econ. Finance 72, 154–174. doi:10.1016/J.IREF.2020.11.018
Huang, S., Du, C., Jin, X., Zhang, D. N., Wen, S. Y., and Jia, Z. J. (2023). The impact of carbon emission trading on renewable energy: a comparative analysis based on the CGE model. Sustainability 15 (16), 12649. doi:10.3390/SU151612649
Jiang, J. J., Xie, D. J., Ye, B., Shen, B., and Chen, Z. M. (2016). Research on China’s cap-and-trade carbon emission trading scheme: overview and outlook. Appl. Energy 178, 902–917. doi:10.1016/j.apenergy.2016.06.100
Jiang, X. G., Xu, W. W., and Du, L. X. (2024). Empirical analysis of the impact of China’s carbon emissions trading policy using provincial-level data. Energy Inf. 7 (1), 40. doi:10.1186/S42162-024-00346-Y
Li, T. X., Xiao, Q., Jia, H. J., Mu, Y. F., Wang, X. Y., Lu, W. B., et al. (2022). Multi-agent schedule optimization method for regional energy internet considering the improved tiered reward and punishment carbon trading model. Front. Energy Res. 10, 10. doi:10.3389/FENRG.2022.916996
Li, W., and Jia, Z. J. (2016). The impact of emission trading scheme and the ratio of free quota: a dynamic recursive CGE model in China. Appl. Energy 174, 1–14. doi:10.1016/j.apenergy.2016.04.086
Li, X. Y. (2022). Local government decision-making competition and regional carbon emissions: experience evidence and emission reduction measures. Sustain. Energy Technol. Assessments 50, 101800. doi:10.1016/J.SETA.2021.101800
Li, Y. L., and Zhang, J. J. (2024). Research on the carbon emission reduction effect of the dual pilot policy of low-carbon cities and new energy demonstration cities. Sustainability 16 (21), 9572. doi:10.3390/SU16219572
Lin, A. H., and Fu, Y. Z. (2023). Did carbon emission trading scheme improve energy use in China? An integrated approach based on SCM and threshold regression. Environ. Sci. Pollut. Res. Int. 31, 6200–6212. doi:10.1007/S11356-023-31425-4
Liu, J. B., Peng, X. B., and Zhao, J. (2023). Analyzing the spatial association of household consumption carbon emission structure based on social network. J. Comb. Optim. 45, 79. doi:10.1007/S10878-023-01004-X
Liu, J. B., and Yuan, X. (2023). Prediction of the air quality index of Hefei based on an improved ARIMA model. Aims Math. 8, 18717–18733. doi:10.3934/math.2023953
Liu, J. B., and Zhao, B. Y. (2023). Study on environmental efficiency of Anhui province based on SBM-DEA model and fractal theory. Fractals 31, 2340072. doi:10.1142/S0218348X23400728
Lu, C. H., and Li, H. (2023). Have China’s regional carbon emissions trading schemes promoted industrial resource allocation efficiency? The evidence from heavily polluted industries at the provincial level. Sustainability 15 (3), 2657. doi:10.3390/SU15032657
Luo, L. W., Ma, Y. Q., and Zhou, Q. (2023). The impact and transmission mechanism of green credit policy on energy efficiency: new evidence from China. Environ. Sci. Pollut. Res. Int. 30 (19), 56879–56892. doi:10.1007/S11356-023-26376-9
Ma, J. T., Hu, Q. G., Shen, W. T., and Wei, X. Y. (2021). Does the low-carbon city pilot policy promote green technology innovation? Based on green patent data of Chinese A-share listed companies. Int. J. Environ. Res. Public Health 18 (7), 3695. doi:10.3390/IJERPH18073695
Miao, S., Tuo, Y. D., Zhang, X., and Hou, X. (2023). Green fiscal policy and ESG performance: evidence from the energy-saving and emission-reduction policy in China. Energies 16 (9), 3667. doi:10.3390/EN16093667
Pan, A., Zhang, W. N., Shi, X. P., and Dai, L. (2022). Climate policy and low-carbon innovation: evidence from low-carbon city pilots in China. Energy Econ. 112, 106129. doi:10.1016/J.ENECO.2022.106129
Qiu, S. L., Wang, Z. L., and Liu, S. (2021). The policy outcomes of low-carbon city construction on urban green development: evidence from a quasi-natural experiment conducted in China. Sustain. Cities Soc. 66, 102699–102699. doi:10.1016/J.SCS.2020.102699
Sadorsky, P.Renewable energy consumption (2009). CO2 emissions and oil prices in the G7 countries. Energy Econ. 31, 456–462.
Sandoff, A., and Schaad, G. (2009). Does EU ETS lead to emission reductions through trade? The case of the Swedish emissions trading sector participants. Energy Policy 37, 3967–3977. doi:10.1016/j.enpol.2009.04.043
Shahbaz, M., Hye, A. M. Q., Tiwari, K. A., and Leitão, C. N. (2013). Economic growth, energy consumption, financial development, international trade and CO2 emissions in Indonesia. Renew. Sustain. Energy Rev. 25, 109–121. doi:10.1016/j.rser.2013.04.009
Shi, B. G., Xiang, W. J., Bai, X. D., Wang, Y. J., Geng, G. H., and Zheng, J. (2022). District level decoupling analysis of energy-related carbon dioxide emissions from economic growth in Beijing, China. Energy Rep. 8 (8), 2045–2051. doi:10.1016/J.EGYR.2022.01.124
Song, M. L., Zheng, H. Y., and Shen, Z. Y. (2023). Whether the carbon emissions trading system improves energy efficiency – empirical testing based on China's provincial panel data. Energy 275, 127465. doi:10.1016/J.ENERGY.2023.127465
Suopajärvi, H., Pongrácz, E., and Fabritius, T. (2014). Bioreducer use in Finnish blast furnace ironmaking – analysis of CO2 emission reduction potential and mitigation cost. Appl. Energy 124, 82–93. doi:10.1016/j.apenergy.2014.03.008
Tan, J. R., and Chen, L. (2023). Green finance, energy consumption, and carbon dioxide emissions: a GMM panel data analysis from China. IOP Conf. Ser. Earth Environ. Sci. 1152 (1), 012001. doi:10.1088/1755-1315/1152/1/012001
Tan, X. J., Liu, Y. S., Dong, H. M., and Zhang, Z. (2022). The effect of carbon emission trading scheme on energy efficiency: evidence from China. Econ. Analysis Policy 75, 506–517. doi:10.1016/J.EAP.2022.06.012
Wang, C. A., Liu, X. Q., Li, H., and Yang, C. Y. (2023b). Analyzing the impact of low-carbon city pilot policy on enterprises' labor demand: evidence from China. Energy Econ. 124, 106676. doi:10.1016/J.ENECO.2023.106676
Wang, C. Z., Yan, J. P., and Li, J. B. (2024a). Does China’s carbon emission trading scheme reduce CO2 emissions? Comprehensive evaluation from synthetic control method using lasso. Energy Rep. 12, 2429–2440. doi:10.1016/J.EGYR.2024.08.057
Wang, K. L., Li, J., Xu, R. Y., Pang, S. Q., Miao, Z., and Sun, H. P. (2022b). The impact of low-carbon city pilot policy on green total-factor productivity in China's cities. Environ. Sci. Pollut. Res. Int. 30 (9), 24299–24318. doi:10.1007/S11356-022-23934-5
Wang, Q., and Xiao, Y. H. (2022). Has urban construction land achieved low-carbon sustainable development? A case study of North China plain, China. Sustainability 14 (15), 9434. doi:10.3390/SU14159434
Wang, S. G., Zhang, Z. Q., Zhou, Z. C., and Zhong, S. (2024b). The carbon emission reduction effect of green fiscal policy: a quasi-natural experiment. Sci. Rep. 14 (1), 20317. doi:10.1038/S41598-024-71728-1
Wang, X. F., and Li, Y. N. (2023). Impact of low-carbon policies on carbon emissions—empirical evidence from low-carbon city pilot policy. Front. Soc. Sci. Technol. 5 (7). doi:10.25236/FSST.2023.050711
Wang, Z. S., Xie, W. C., and Zhang, C. Y. (2023a). Towards COP26 targets: characteristics and influencing factors of spatial correlation network structure on U.S. carbon emission. Resour. Policy 81, 103285. doi:10.1016/J.RESOURPOL.2022.103285
Wang, Z. S., Zhou, Y. X., Zhao, N., Wang, T., and Zhang, Z. S. (2022a). Spatial correlation network and driving effect of carbon emission intensity in China’s construction industry. Buildings 12 (2), 201. doi:10.3390/BUILDINGS12020201
Wen, S. Y., Jia, Z. J., and Chen, X. Q. (2022). Can low-carbon city pilot policies significantly improve carbon emission efficiency? Empirical evidence from China. J. Clean. Prod. 346, 131131. doi:10.1016/J.JCLEPRO.2022.131131
Wen, Z. L., Zhang, L., Hou, J. T., and Liu, H. Y. (2004). Mediation effect test procedures and their applications. Acta Psychol. Sin. (05), 614–620.
Wolff, H. (2014). Keep your clunker in the suburb: low-emission zones and adoption of green vehicles. Econ. J. 124, F481–F512. doi:10.1111/ecoj.12091
Xiao, D., Yu, F., and Guo, C. H. (2023). The impact of China's pilot carbon ETS on the labor income share: based on an empirical method of combining PSM with staggered DID. Energy Econ. 124, 106770. doi:10.1016/J.ENECO.2023.106770
Xu, B., and Lin, B. Q. (2025). Green credit and green technology innovation: impact mechanism and nonlinear effect test. Environ. Impact Assess. Rev. 110, 107652. doi:10.1016/J.EIAR.2024.107652
Xu, W. L., Jiang, C. J., Jia, K. W., and Yu, X. Y. (2023). Did the “double carbon” policy improve the green total factor productivity of iron and steel enterprises? a quasi-natural experiment based on carbon emission trading pilot. Front. Energy Res. 11, 11. doi:10.3389/FENRG.2023.1307347
Yang, X. Y., Yang, X. H., Zhu, J. G., Jiang, P., Lin, H. X., Cai, Z. Y., et al. (2023). Achieving co-benefits by implementing the low-carbon city pilot policy in China: effectiveness and efficiency. Environ. Technol. and Innovation 30, 103137. doi:10.1016/J.ETI.2023.103137
Yang, Y., and Peng, C. L. (2022). Low-carbon city pilot policy, fiscal pressure, and carbon productivity: evidence from China. Front. Environ. Sci. 10, 10. doi:10.3389/FENVS.2022.978076
Zhang, A. L., Wang, S. Y., and Liu, B. (2022a). How to control air pollution with economic means? Exploration of China's green finance policy. J. Clean. Prod. 353, 131664. doi:10.1016/J.JCLEPRO.2022.131664
Zhang, D., Sebastian, R., Valerie, K. J., and Zhang, X. L. (2013). Quantifying regional economic impacts of CO2 intensity targets in China. Energy Econ. 40, 687–701. doi:10.1016/j.eneco.2013.08.018
Zhang, H., Feng, C., and Zhou, X. X. (2022c). Going carbon-neutral in China: does the low-carbon city pilot policy improve carbon emission efficiency? Sustain. Prod. Consum. 33, 312–329. doi:10.1016/J.SPC.2022.07.002
Zhang, J. G., Gao, L., Wang, W. K., Deng, Z. Z., and Zhang, X. (2022b). The impact of low-carbon city pilot policies on air quality: quasi-natural experimental evidence from China. Atmosphere 13 (9), 1355. doi:10.3390/ATMOS13091355
Zhang, K., Zhang, Z. Y., and Liang, Q. M. (2017b). An empirical analysis of the green paradox in China: from the perspective of fiscal decentralization. Energy Policy 103, 203–211. doi:10.1016/j.enpol.2017.01.023
Zhang, R. N., Ai, X. N., and Li, H. (2023). How to design subsidy policies for clean energy projects? A study on “coal-to-gas” project in China. Resour. Policy 85 (PB), 103928. doi:10.1016/J.RESOURPOL.2023.103928
Zhang, Y. J., Peng, Y. L., Ma, C. Q., and Shen, B. (2017a). Can environmental innovation facilitate carbon emissions reduction? Evidence from China. Energy Policy 100, 18–28. doi:10.1016/j.enpol.2016.10.005
Zhao, G. G., Zhang, Y. N., and Wu, Y. G. (2024). Implementation effect, long-term mechanisms, and industrial upgrading of the low-carbon city pilot policy: an empirical study based on city-level panel data from China. Sustainability 16 (19), 8316. doi:10.3390/SU16198316
Zhao, S., Zhang, L. Q., An, H. Y., Peng, L., Zhou, H. Y., and Hu, F. (2023). Has China's low-carbon strategy pushed forward the digital transformation of manufacturing enterprises? Evidence from the low-carbon city pilot policy. Environ. Impact Assess. Rev. 102, 107184. doi:10.1016/J.EIAR.2023.107184
Zheng, Y. X., Zhang, M., Wang, S., and Wang, L. (2023). The impacts of low-carbon city pilot policies on natural population growth: empirical evidence from China's prefecture-level cities. Front. public health 11, 1214070. doi:10.3389/FPUBH.2023.1214070
Zhu, Q. S., Gao, K., and Liu, J. B. (2023a). Cloud model for new energy vehicle supply chain management based on growth expectation. J. Comb. Optim. 45, 125. doi:10.1007/S10878-023-01052-3
Keywords: China’s dual carbon goals, low carbon city pilot policy, “carbon emission reduction” effect, multi-period double difference, heterogeneity analysis
Citation: Shu J-X, Peng J-J and Zhang J (2025) The impact of low-carbon city pilot policy on carbon emission intensity: evidence from China using a multi-period difference-in-differences model. Front. Environ. Sci. 12:1488526. doi: 10.3389/fenvs.2024.1488526
Received: 30 August 2024; Accepted: 23 December 2024;
Published: 10 January 2025.
Edited by:
Jiachao Peng, Wuhan Institute of Technology, ChinaReviewed by:
Cüneyt Dumrul, Erciyes University, TürkiyeCopyright © 2025 Shu, Peng and Zhang. This is an open-access article distributed under the terms of the Creative Commons Attribution License (CC BY). The use, distribution or reproduction in other forums is permitted, provided the original author(s) and the copyright owner(s) are credited and that the original publication in this journal is cited, in accordance with accepted academic practice. No use, distribution or reproduction is permitted which does not comply with these terms.
*Correspondence: Jia-Jia Peng, cGpqMTc4NTY5OTA4NTVAMTYzLmNvbQ==
Disclaimer: All claims expressed in this article are solely those of the authors and do not necessarily represent those of their affiliated organizations, or those of the publisher, the editors and the reviewers. Any product that may be evaluated in this article or claim that may be made by its manufacturer is not guaranteed or endorsed by the publisher.
Research integrity at Frontiers
Learn more about the work of our research integrity team to safeguard the quality of each article we publish.