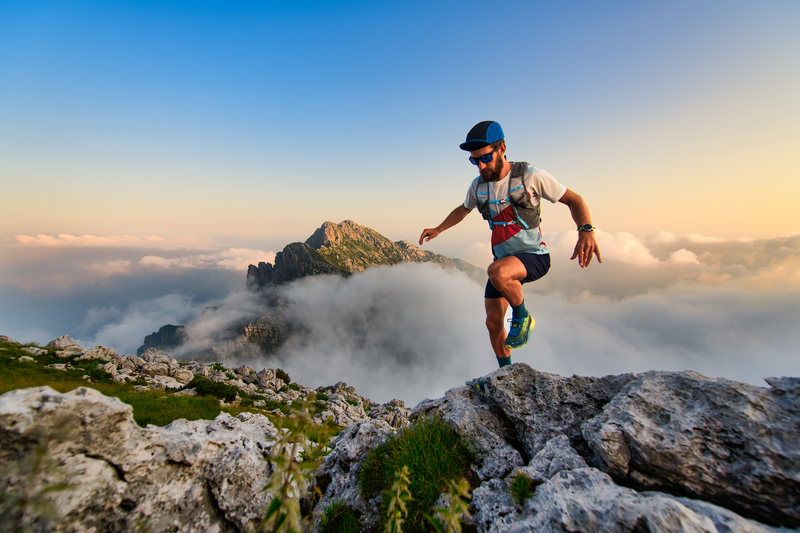
94% of researchers rate our articles as excellent or good
Learn more about the work of our research integrity team to safeguard the quality of each article we publish.
Find out more
ORIGINAL RESEARCH article
Front. Environ. Sci. , 22 October 2024
Sec. Environmental Economics and Management
Volume 12 - 2024 | https://doi.org/10.3389/fenvs.2024.1478649
This article is part of the Research Topic Sustainable and Mission-oriented Innovation in Economic Systems and Governance for Equitable Global Health and Wellbeing View all 25 articles
Rapid economic development has led to massive fossil energy consumption and emissions of air pollutants such as PM2.5, which have severely impacted human health and the environment. By uncovering the primary regions and pivotal sectors of PM2.5-related human health impacts (PM2.5-HHI) and evaluating the influence of economic structural factors on them, we can facilitate a more targeted strategy for managing PM2.5 pollution sources. This study employs a structural decomposition analysis method based on input–output analysis to evaluate the impact of China’s provincial economic structural transformation and changes in final demand on PM2.5-HHI in the years 2012, 2015, and 2017. Results indicated that PM2.5-HHI is primarily concentrated in economically developed provinces (e.g., Shandong and Guangdong), which is compared to Shanghai, Heilongjiang, Liaoning, and Hebei experienced negative growth in PM2.5-HHI during 2007–2017. The production-based PM2.5-HHI is primarily driven by energy-intensive sectors such as the production and distribution of electric power and heat power. By contrast, the building sector is key to driving consumption-based PM2.5-HHI. An increasing number of regions are reducing PM2.5-HHI by implementing production structure changes. Moreover, the driving effect of production structure changes on PM2.5-HHI growth is strengthening in Beijing and Tianjin. Changes in the final demand structure mainly led to the growth of PM2.5-HHI in areas with higher economic development levels, such as Beijing and Shandong, but this driving effect is weakening. The final demand–driven PM2.5-HHI shows an evolutionary trend of an increasing share driven by fixed capital formation and exports and a decreasing share driven by household consumption. Changes in emission intensity play a key role in decreasing PM2.5-HHI in each region. Alternatively, changes in the structure of emission sources have a relatively minor impact on PM2.5-HHI. To mitigate PM2.5-HHI, regional economic and resource endowment advantages should be used to promote regional coordinated development and strengthen green production-process innovation in energy-intensive industries. Meanwhcile, it is necessary to optimize urban construction planning and improve the energy efficiency of buildings.
China’s rapid economic growth and urbanization have boosted the demand for fossil energy such as coal and oil (Zhao et al., 2023; Zhao et al., 2024; Sun et al., 2024a) This demand has led to severe air pollution (Feng et al., 2022; Sun et al., 2024b), especially the emission of PM2.5, which is far beyond the limits of the environment (Li et al., 2021), resulting in more than a million premature deaths per year (Liu et al., 2020). Since China promulgated a series of clean air policies, including the Action Plan for the Prevention and Control of Air Pollution in 2013 (Geng et al., 2021), end-of-pipe measures have been effective and have significantly improved China’s atmospheric environmental quality (Zhang et al., 2020). However, with continuous innovation and optimization in the field of technology, the marginal benefits of reducing PM2.5 emissions through end-of-pipe treatment gradually weaken (Zhang et al., 2020). More importantly, the significant im-balance in regional economic distribution in China exacerbates disparities in environ-mental pollution and associated health risks (Yang et al., 2024). In addition, research based on environ-mental economic systems has begun to propose emission reduction strategies from the perspective of consumption (Liang et al., 2017; Wu et al., 2021), which suggests that relying on end-of-pipe management alone is no longer enough to address air quality issues and that considerable attention needs to be paid to overall adjustments at the industry and consumption ends.
China actively promotes economic structural reform, fully exploits the potential of energy and mineral resources in the central and western regions, and builds re-source-importing industrial bases in the coastal and border regions. Meanwhile, China promotes the relocation of manufacturing industries from economically developed regions in the east to the central and western provinces to meet the resource, environmental, and market demands of different regions and promote the coordinated development of regional industries (Li et al., 2019). China’s economic structure is gradually evolving from a manufacturing to a service industry (Wu et al., 2021). However, this economic transformation may prevent regional economies from catch-up and overtaking and is not positive for narrowing the gap between the central-western regions and the economically developed regions in the east (Liu et al., 2023b). Furthermore, China’s economy is developing toward another stage led by domestic circulation and complemented by international circulation, with the domestic market as the mainstay (Zhang et al., 2023b). This new development pattern emphasizes the restructuring of the regional economy to promote dual-circulation economic growth. The further deepening of the “du-al-circulation” development model will lead to a widening of the difference in economic factors and structural transformation of the economy across regions, with different im-pacts on pollutant emissions such as PM2.5 and related health burdens in different regions.
PM2.5 pollution is recognized as a major environmental health risk factor (Southerland et al., 2022), and the increasing burden of disease associated with air pollution has sparked widespread attention to the PM2.5-related human health impacts (PM2.5-HHI). PM2.5-HHI primarily mani-fests as increased emissions of PM2.5, which increases the risk of diseases such as cardio-vascular disease, chronic obstructive pulmonary disease, and lower respiratory infections (Forouzanfar et al., 2016). This further leads to premature deaths among exposed populations, making it the primary culprit endangering human health (Guan et al., 2023). Current research utilizes Disability-Adjusted Life Years (DALY) to quantify PM2.5-HHI from the perspectives of short-term and long-term impacts and predictions of future concentration exposures (Cony Renaud Salis et al., 2017; Mueller et al., 2021; Zou et al., 2023). In terms of socioeconomic influences, many scholars have explored the contribution to PM2.5 emissions and PM2.5 concentrations from technical and economic perspectives (Song et al., 2020), but research on influences on PM2.5-HHI is relatively limited.
The impact of spatial variations in economic structure and economic growth on PM2.5-HHI exhibits diverse characteristics (Li et al., 2023). China has a huge spatial difference in its geography and socioeconomic characteristics, which are more pronounced than in most developed countries (He et al., 2019). Such highly unbalanced resource endowments and economic development can lead to a mismatch between the health losses caused by trade and the gains from trade (Feng et al., 2022). Focusing on interprovincial differences may produce more valuable and significant findings than national-level studies, whereas treating China as a whole may belie internal differences and lead to misleading conclusions (Zhai et al., 2022). In addition, the drivers of PM2.5-HHI in China at the provincial level, especially in terms of economic structural factors, remain incompletely quantified and compared. Input–Output Structural Decomposition Analysis (SDA) is known for its ability to accurately quantify the contribution of each independent factor in an economic system to changes in a specific indicator (Liang et al., 2015). Therefore, SDA is widely used when assessing the socioeconomic drivers of re-source consumption (Sun et al., 2023) and pollutant emissions [e.g., PM2.5 (Liu et al., 2023a), NOx (Zhang et al., 2023a), SO2 (Wang et al., 2022a), CO2 (Wang S. et al., 2023b)]. When studying economic structural elements related to PM2.5-HHI, the SDA method similarly demonstrates its merits in an in-depth understanding of the contribution of eco-nomic structural factors to PM2.5-HHI.
In summary, how to reduce anthropogenic emissions of PM2.5 and the resulting PM2.5-HHI is a focal issue of concern for the academic community as well as society. Systematic tracking of PM2.5-HHI drivers at the overall level, at the final demand level, and at the sectoral level is still lacking, especially at the provincial level in China, where resource endowments and economic structures vary significantly. To address the gap in this area, this study employs an environmentally extended input–output model (EEIO) and SDA methodology based on utilizing the most recent data available for China to reveal the drivers and heterogeneity of PM2.5-HHI at the provincial level in China, as well as to assess the contribution of structural transformation of the economy and the evolution of final demand. This study helps delve deeper into the potential for reducing PM2.5-HHI through economic structural transformation in different regions, providing valuable insights for formulating effective policy strategies. Due to the scope of our study being limited to the economic system, this study focuses only on PM2.5-HHI in the context of primary PM2.5 emissions associated with industrial processes (i.e., PM2.5 emissions directly from production activities).
The innovations and contributions of this study, compared with previous studies, consist of two main aspects. First, based on the latest data, we measured PM2.5-HHI in China’s provinces from the production and consumption sides using the EEIO model and analyzed the spatial and temporal distribution of PM2.5-HHI from regional and industrial perspectives, which provided strong policy implications for the formulation of industry-based and region-based policies to reduce the PM2.5-HHI. Second, we employed the SDA model to systematically decompose PM2.5-HHI, investigating the contributions of economic structural transformation and changes in final demand. This analysis provides policy-making support for provinces to adjust economic structures and optimize industrial layouts to reduce PM2.5-HHI.
The content is structured as follow: Section 2 describes the research methodology and data. Section 3 presents the results. The results of the study are discussed and a sensitivity analysis is presented in Section 4. Section 5 concludes the study and provides the policy recommendations, as well as discussing research limitations.
Production-based PM2.5-HHI signifies the health effects of PM2.5 emitted directly during production processes. Without considering the impacts of changes in PM2.5-related elements such as population exposure and meteorology, we mainly focus on analyzing the effects of final demand variations and economic structural changes on PM2.5-HHI. The study utilizes the research by Liang et al. (2017) and Wu et al. (2021) to compute PM2.5-HHI by employing transformation parameters obtained from PM2.5 emission data specific to different industries. Production-based PM2.5 emissions are determined by multiplying PM10 emissions by a coefficient reflecting the proportion of China’s PM2.5 emissions as a percentage of PM10 emissions, as shown in Equation 1.
The 1
Input–output models can be used to characterize the structure of economic systems, reflecting the interdependence between industries in economic activities (Munday and Beynon, 2011). Equation 3 reflects the balance relationship of the input–output table.
Vector
The EEIO model is built, treating production-based PM2.5-HHI as a satellite account of the IO model and evaluating each sector’s contribution to PM2.5-HHI from final demand (Equation 4). This approach can provide a more comprehensive view of the health im-pacts of PM2.5 pollution from a consumption perspective.
Vector
Studies have shown that coal combustion, diesel vehicles and industrial processes are the main sources of PM2.5 emissions (Guan et al., 2014). Promoting energy transition in various industrial sectors can effectively improve the source structure of PM2.5 emissions and thus reduce PM2.5-HHI (Wu et al., 2021). Notably, the effect of population growth on PM2.5-HHI is significantly higher than its direct effect on PM2.5 concentration (Zhang et al., 2019). In addition, the transformation of economic structure, such as changes in the production structure and changes in final demand (including the composition and total amount of demand), will also have a profound impact on socioeconomic development and PM2.5-HHI (Wen et al., 2024). Combining the SDA and EEIO models enables the quantification of the extent to which changes in economic structural factors contribute to PM2.5-HHI. Therefore, to deeply explore the drivers of PM2.5-HHI in each province of China, we decompose the PM2.5-HHI changes into emission source structure, emission intensity, production structure, final demand structure, per capita final demand, and population changes. The following is the specific process of decomposition:
In Equation 6,
To explore the relative contribution of changes in emission source structure and PM2.5 emission intensity to changes in PM2.5-HHI, we split
where the
We denote the full consumption coefficient matrix
The main sources of uncertainty in PM2.5-HHI stem from the Chinese Monetary In-put–Output Table (MIOT) data and PM10 emission data from the Eora database. Deter-mining the extent of PM2.5-HHI uncertainty is challenging since there is limited information on uncertainty in the input–output tables and certain parameters. The calculation of sensitivity coefficients is an effective method to quantify the extent to which marginal changes in each parameter affect PM2.5-HHI. Hence, we utilized a matrix-based method to analyze the model’s sensitivity to all parameters (Wu et al., 2021).
Specifically, regarding PM2.5-HHI, sensitivity coefficients for various factors, including population
To further mitigate the potential impact of the statistical units of the parameter units on the results, we used Equations 18–26 to compute the dimensionless elasticities for parameters. These elasticities express the relationship between the rate of change of PM2.5-HHI and the rate of change of the parameter. This helps diminish the effect of inconsistency in units on the analysis. The
This study utilized single-region input–output tables for each province, producer price indices (PPIs), population data for each province in China, and sectoral PM10 emissions for specific processes in China. Data from 30 provinces in China (excluding Taiwan, Macau, Hong Kong, and Tibet) were utilized for this study. Regional MIOT and population data for 2007, 2012, and 2017 were sourced from the National Bureau of Statistics of China. To eliminate the effects of price volatility, we use the double deflator method advocated by the United Nations to adjust the input–output tables for 2017 and 2007 to constant 2012 prices (United, 1999), with PPIs for each sector obtained from the China Statistical Yearbook. To eliminate the effect of imports on the competitive input–output tables (Wang et al., 2022), the competitive input–output tables of Chinese provinces were transformed into noncompetitive in-put–output tables (Wang et al., 2022b). For consistency with MIOT, process-specific PM10 emission data are utilized for various industries in China from the Eora database (Lenzen et al., 2013). In addition, this study measured the emission intensity of PM10 in China based on the PM10 emissions from various industry-specific processes at the national level in China, published by Eora. Assuming that the emission intensity of PM10 in each province of China is the same at the national level, we focus on the relative contributions of the final demand evolution and three structural factors (production structure, emission source structure, and final demand structure) to PM2.5-HHI between 2007 and 2017. After data adjustment and refinement, the input–output tables and PM10 emission datasets for the years 2007, 2012, and 2017 were finally aggregated into 28 industrial sectors (see Table 1) (Feng et al., 2023). Referring to Wen et al.’s study (Wen et al., 2024), we selected 50 types of emission sources from 55 types of processes that directly emit PM10 in the Eora database for this study. Notably, we did not include five natural sources of PM10 emissions in our study, including grassland fires, wetland/marsh decomposition, forest fires followed by decomposition, forest fires, and other vegetation fires, as they are not directly related to economic activities. In computing PM2.5-HHI, we applied the fraction factor of PM2.5 emissions to total PM10 emissions, sourced from the World Health Organization (Ostro, 2004), and consulted Wu et al.’s study for the dose–severity factor and PM2.5 intake fraction across 50 emission processes (Wu et al., 2021; Gronlund et al., 2015).
PM2.5-HHI shows significant spatial and temporal differences (Figure 1), which are mainly concentrated in the eastern provinces, such as Shandong, Guangdong, and Jiangsu. These three provinces accounted for approximately 30% of the national PM2.5-HHI during 2007–2017. Hainan, Qinghai, and Ningxia had the smallest PM2.5-HHI, less than 2% of the national total. On one hand, between 2007 and 2012, all 30 provinces in China experienced an increase in PM2.5-HHI. On the other hand, during 2012–2017, there were significant differences in PM2.5-HHI changes among provinces, with half of the provinces still experiencing an increasing trend. Large energy–consuming provinces such as Jiangsu, Shanxi, Hebei, and Guangdong have achieved negative PM2.5-HHI growth and decoupled energy consumption from PM2.5-HHI through green technology innovations and optimization of energy structures, despite growing energy consumption (Guan et al., 2021).
The difference in PM2.5-HHI between the production and consumption sides is shown in Figures 2A–C. Most of PM2.5-HHI at the production end is concentrated in energy-intensive heavy industries, mainly Power (S22) (32.53%), ManNonmet. (S13) (9.92%), and Petro. (S11) (9.15%). These three industries are energy-intensive, and they emit the most pollutants (including PM2.5) as well as the most harm to human health, together contributing 56% of China’s PM2.5-HHI. As can be seen from Figures 2D, E, among these sectors, the PM2.5-HHI share of ManNonmet. (S13) showed a gradual decrease from 2007 to 2017. By contrast, the PM2.5-HHI share of the Petro. (S11) sector gradually increased. Among the provinces, Guangdong, Jiangsu, and Zhejiang had the highest proportion of PM2.5-HHI in the Power (S22) sector. Meanwhile, Henan, Shandong, and Guangdong provinces had the highest share of PM2.5-HHI in the ManNonmet. (S13) sector, and Shandong, Liaoning, and Guangdong had the highest proportion of PM2.5-HHI in the Petro. (S11) sector. Notably, MinNonmet. (S5) (0.16%) and Extra. (S3) (0.18%) were the least damaging to human health, with a combined contribution of less than 0.4% of PM2.5-HHI in China. This suggests that the damage to human health from resources and energy is mainly in the combustion use chain.
Figure 2. (A) Distribution of PM2.5-HHI in different sectors at production and consumption ends by province in 2007. (B) Distribution of PM2.5-HHI in different sectors at production and consumption ends by province in 2012. (C) Distribution of PM2.5-HHI in different sectors at production and consumption ends by province in 2017. (D) Changes in PM2.5-HHI at the production and consumption ends for different industries in each province from 2007 to 2012. (E) Changes in PM2.5-HHI at the production and consumption ends for different industries in each province from 2012 to 2017.
The sectors of Constr. (S25) and Others (S28) are the largest contributors to consumption-based PM2.5-HHI, collectively accounting for 30% of China’s PM2.5-HHI. Among the sectors, in Beijing, the proportion of PM2.5-HHI driven by the Others (S28) sector shows a decreasing trend. By contrast, in Shandong and Jiangsu, the proportion of PM2.5-HHI driven by the Others (S28) sector continues to grow. With the acceleration of urbanization, Guangdong, Shandong, and Jiangsu provinces became the largest contributors of PM2.5-HHI in the Constr. (S25) sector, with the most significant growth in human health damage in the Constr. (S25) sector in Shandong, Hubei, and Sichuan. Furthermore, the consumption-based PM2.5-HHI attributed to the Power (S22) sector has approached 9% of the total, and the share of PM2.5-HHI driven by Power (S22) has shown an increasing trend as China’s demand for electricity and heat continues to grow. In particular, Beijing, Sichuan, and Guangdong have the most apparent increasing trend of Power (S22)-driven PM2.5-HHI. MinNonmet. (S5) and Extra. (S3) also contribute the least to the consumption-based PM2.5-HHI, together contributing less than 0.5% of China’s PM2.5-HHI. Although the consumption-based PM2.5-HHI driven by the Power (S22), ManNonmet. (S13), and Petro. (S11) industries should not be ignored, PM2.5-HHI driven by these three industries on the consumption side combined has been less than 18% of China’s PM2.5-HHI compared with the production side. In summary, there are significant spatial and temporal differences in PM2.5-HHI among different provinces and industries, which are not only affected by the natural environment and geographic location, but are also closely related to the intrinsic transformation of economic structure. In order to boost the understanding behind these differences, the impact of economic structural transformation on PM2.5-HHI needs to be further explored.
Changes in emission intensity are the primary driver of PM2.5-HHI reduction across all provinces, particularly noticeable during 2012–2017 (Figure 3). Notably, changes in emission intensity in Beijing were the sole factor driving the PM2.5-HHI reduction during this period. Notably, during the period 2007–2017, more regions have reduced PM2.5-HHI through structural changes in production. However, the driving effect of production structure changes on PM2.5-HHI growth is strengthening in Beijing, Tianjin, Inner Mongolia, Anhui, and Qinghai provinces. In addition, changes in the production structure in Fujian, Shandong, Yunnan, and Gansu have been positively affecting the enhancement of PM2.5-HHI, but this positive effect is weakening.
Figure 3. Relative contribution of socioeconomic factors to PM2.5-HHI changes. The top half of the figure is for 2007–2012, and the bottom half of the figure is for 2012–2017.
During the period 2007–2017, the impact of changes in the structure of final demand on PM2.5-HHI growth was mainly concentrated in provinces with more developed and larger provinces, such as Beijing, Henan, Shandong, Guangdong, and Sichuan. In Beijing, the changes in the final demand structure had a particularly notable impact on the in-crease in PM2.5-HHI, reaching 134,352.73 DALY. However, the changes in final demand structure in provinces such as Tianjin, Hebei, Shanxi, Shanghai, Anhui, Hubei, Hainan, Chongqing, Guizhou, and Yunnan have contributed to a decrease in PM2.5-HHI. Specifically, between 2007 and 2012, only Tianjin, Hebei, Shanxi, Shanghai, Inner Mongolia, Liaoning, Hubei, Guizhou, and Xinjiang had structural changes in final demand that drove reductions in PM2.5-HHI. By contrast, final demand in other provinces drove in-creases in PM2.5-HHI to varying degrees. With the continuous adjustment and optimization of the final demand structure of each province, more provinces have a green and healthy development of their final demand structure. During the 2012–2017 period, changes in the structure of final demand only drove the growth of PM2.5-HHI in Beijing, Jilin, Inner Mongolia, Liaoning, Shandong, Heilongjiang, Zhejiang, Shaanxi, Henan, Hubei, Sichuan, Xinjiang, and Ningxia, and the strength of this driving force weakened markedly compared with the 2007–2012 period.
Changes in per capita demand had a significant impact on PM2.5-HHI during the 2007–2017 periods, becoming the main driver of its growth. This growth was mainly concentrated in the central and eastern provinces of Jiangsu, Henan, and Shandong, which have better economic performance. Only the provinces of Inner Mongolia, Liaoning, Shandong, and Gansu saw changes in per capita demand drive reductions in PM2.5-HHI over the 2012–2017 period. Population change-driven PM2.5-HHI growth is mainly concentrated in the eastern coastal provinces. During 2007–2017, PM2.5-HHI, driven by population growth in the eastern provinces, accounted for 86.22% of the population change-driven growth in China. Henan is a large population province, and although its population change drove a marginal rise in PM2.5-HHI, the increase was not severe com-pared with the more developed provinces on the eastern coast. In contrast, population changes in Jilin, Heilongjiang, Anhui, and Gansu drove a decrease in PM2.5-HHI.
Changes in the structure of emission sources had a relatively small impact on PM2.5-HHI. During the period 2007–2012, although changes in the structure of emission sources reduced PM2.5-HHI to some extent in most provinces, none of the contributions exceeded 100 DALY. While the emission source structure of Tianjin, Shanghai, Jiangsu, Zhejiang, Shandong, and Guangdong has a positive contribution to PM2.5-HHI, it is also within 100 DALY. During 2012–2017, the contribution of changes in emission source structure to PM2.5-HHI in each region was nearly negligible.
During the period 2007–2017, the largest final demand driver for PM2.5-HHI stemmed from exports (47.77% of all), followed by gross fixed capital formation (investment) and household consumption (Figure 4A). China’s provincial household consumption, investment, and export-driven PM2.5-HHI dominate the final demand–driven PM2.5-HHI, and more than half of the provinces have experienced a shift in final demand from household consumption to investment and exports. During the period 2007–2017, the average share of PM2.5-HHI driven by investment and exports increased by 2.71% and 2.54%, respectively. By contrast, PM2.5-HHI, driven by household consumption, average decreased by 1.88% in each province. Notably, the share of total output accounted for by fixed investment demand grew in Jilin, Heilongjiang, Jiangxi, and Henan. By contrast, the share of PM2.5 driven by fixed investment declined in these provinces. In contrast, the proportion of total output attributed to consumption demand has decreased in provinces such as Inner Mongolia, Shanxi, Shanghai, and Guangdong, whereas the share of PM2.5-HHI driven by household consumption demand has increased. Overall, export-driven factors dominate in driving PM2.5-HHI. By contrast, the influence of household consumption is weakening, and investment is becoming increasingly significant in driving PM2.5-HHI. Furthermore, from the perspective of final demand driving PM2.5-HHI, eastern provinces such as Tianjin, Guangdong, Jiangsu, Shanghai, and Hebei show a trend where the proportion of PM2.5-HHI driven by final demand has an increasing share attributed to household consumption and a decreasing share attributed to exports. In contrast, resource-rich provinces in central and western China, such as Inner Mongolia, Shanxi, Jilin, Heilongjiang, Jiangxi, Henan, Chongqing, Shaanxi, and Ningxia, show a trend where the proportion of PM2.5-HHI driven by final demand attributed to exports is continuously increasing, with the share of PM2.5-HHI driven by exports approaching or exceeding half of the total.
Figure 4. (A) PM2.5-HHI driven by different types of final demand by region in China during 2007–2017. Note: Figures 4A–D are abbreviated as follows: BJ-Beijing, TJ-Tianjin, HeB-Hebei, SX-Shanxi, IM- Mongolia, LN-Liaoning, JL-Jilin, HLJ-Heilongjiang, SH-Shanghai, JS -Jiangsu, ZJ-Zhejiang, AH-Anhui, FJ-Fujian, JX-Jiangxi, SD-Shandong, HeN-Henan, HuB-Hubei, HuN-Hunan, GD-Guangdong, GX-Guangxi, HaN-Hainan, CQ -Chongqing, SC-Sichuan, GZ-Guizhou, YN-Yunnan, SaX-Shaanxi, GS-Gansu, QH-Qinghai, NX-Ningxia, XJ-Xinjiang. (B) Changes in household consumption-driven PM2.5-HHI across different regions and sectors from 2007 to 2017. (C) Changes in PM2.5-HHI Driven by Investment in Different Sectors Across Various Re-gions from 2007 to 2017. (Note: only sectors with nonzero values of investment-driven PM2.5-HHI are shown in the figure; the rest of the sectors have zero-value investment changes and are therefore not shown in the figure). (D) Changes in PM2.5-HHI driven by exports in different regions and sectors during 2007–2017.
Influenced by the level of regional economic development and consumption level, there are significant differences in the dominant industry in household consumption driving PM2.5-HHI in different regions (Figure 4B). Overall, the Power (S22), Petro. (S11), and Others (S28) sectors are the key industries driving household consumption-driven PM2.5-HHI growth. This driving effect is particularly pronounced in Shandong, Guangdong, and Jiangsu provinces, which are economically developed. The Petro. (S11) and Power (S22) sectors are key sectors driving household consumption-driven PM2.5-HHI growth in Liaoning, Jilin, and Heilongjiang, but the Others (S28) sectors emerge as key sectors driving household consumption-driven PM2.5 reductions in these provinces. Furthermore, the Petro. (S11) and Power (S22) sectors are key sectors driving household consumption-driven PM2.5-HHI growth in Liaoning, Jilin, and Heilongjiang, but the Others (S28) sectors emerge as key sectors driving household consumption-driven PM2.5 reductions in these provinces. Furthermore, the ManNonmet. (S13), Agr. (S1), and Gas (S23) sectors are the core sectors for reducing PM2.5-HHI. Notably, ManNonmet. (S13) is the dominant sector driving the reduction of PM2.5-HHI in large light manufacturing provinces such as Inner Mongolia, Hebei, and Shanxi. Meanwhile, the Agr. (S1) sector is the primary sector driving the reduction of PM2.5-HHI in Henan, Liaoning, Jilin, Heilongjiang, and other agricultural provinces, as well as in economically developed provinces such as Beijing, Shanghai, and Guangdong.
The investment structures of different regions have a significant impact on PM2.5-HHI formed by investment-driven factors (Figure 4C). Overall, with the advancement of region-al urbanization, Constr. (S25), Others (S28), and Mach. (S16) have become the leading sec-tors driving the growth of investment-driven PM2.5-HHI. Conversely, ElecMach. (S18) and Computer (S19) are the leading sectors driving the reduction of PM2.5-HHI, driven by fixed capital formation. However, the impact of these sectors on the fixed capital–driven formation of PM2.5-HHI in different regions has substantially different results. The construction sector in provinces with rapid urbanization, such as Shandong, Jiangsu, Sichuan, and Hubei, contributes to the main fixed capital–driven formation of PM2.5-HHI. In regions where urbanization has stabilized, such as Beijing, Shanghai, and Hainan, and in areas experiencing significant population outflows, such as Liaoning, Jilin, and Heilongjiang, Constr. (S25) is the leading sector driving the reduction of PM2.5-HHI driven by fixed capital formation. During 2007–2017, investment in Others (S28) sectors drove the growth of fixed asset formation–driven PM2.5-HHI in most regions. By contrast, driving down fixed capital formation–driven PM2.5-HHI in Qinghai, Ningxia, and Xinjiang. Investment in the Mach. (S16) sector has driven a reduction in fixed capital formation–driven PM2.5-HHI in developed regions that focus on environmental protection and the adoption of cleaner technologies, such as Beijing, Shanxi, Shanghai, Zhejiang, and Chongqing. By contrast, some large manufacturing provinces (e.g., Tianjin, Guangdong, and Anhui) may have been more focused on industrial growth in the course of their rapid development, thereby increasing the fixed capital formation–driven PM2.5-HHI. Most provinces, such as Hebei, Liaoning, Jilin, Jiangxi, Shandong, and Henan, showed an increase during 2007–2012 and a decrease during 2012–2017, suggesting that investment in the machinery industry in most provinces tends to be cleaner and greener.
Overall, Power (S22), Others (S28), and Who.&Cat. (S27) were the dominant sectors driving the export-driven increase in PM2.5-HHI. By contrast, Metal (S14), Petro. (S12), and MinNonmet. (S7) were the crucial sectors primarily contributing to the export-driven de-crease in PM2.5-HHI (Figure 4D). Specifically, Power (S22) plays a key role in export-driven PM2.5-HHI reductions in provinces that are relatively backward in terms of economic and industrial development, such as Guizhou, Yunnan, Guangxi, and Hainan. On the contrary, in energy resource-rich provinces such as Inner Mongolia, Shaanxi, and Xinjiang, their export-driven PM2.5-HHI growth stems mainly from the extraction and processing of energy as a major export product, which leads to more emissions of pollutants such as PM2.5 and pushes up the export-driven PM2.5-HHI growth. Notably, Power (S22) emerged as the dominant sector driving PM2.5-HHI growth in Beijing’s exports. In addition, the Who.&Cat. (S27) and Others (S28) sectors primarily drove the rise in export-driven PM2.5-HHI in the economically developed provinces of Beijing, Henan, Jiangsu, and Zhejiang. By contrast, the Who.&Cat. (S27) and Others (S28) sectors contributed to the decrease in export-driven PM2.5-HHI in the developed and industrialized provinces such as Shandong and Hebei.
Previous studies have focused on the human health impacts of PM2.5 emissions in China (Wu et al., 2021; Wang et al., 2021; Hu et al., 2024), but relatively few studies have estimated the differences in human health impacts due to PM2.5 emissions at the disaggregated industry level and the regional level. In our study, based on the latest data, we used the EEIO model to measure China’s provincial PM2.5-HHI from two perspectives, the production side and the consumption side, by industry. Based on the research findings, the total PM2.5-HHI of the 30 provinces closely aligns with Wu et al.’s national-level study (Wu et al., 2021), suggesting good comparability, and shows a consistent increasing trend from 2007 to 2012. During 2012–2017, more than half of the provinces in China experienced a significant PM2.5-HHI decreasing trend, consistent with the findings of Dong et al. (2022). The observed trend can be largely attributed to the implementation of China’s Air Pollution Prevention and Control Action Plan in 2013 (Wang et al., 2023a), which has effectively curbed PM2.5 emissions. To provide a more nuanced analysis of PM2.5-HHI heterogeneity, we conducted a comprehensive test of its spatial and temporal distribution across both regional and industrial dimensions. Our findings reveal significant spatial disparities in PM2.5-HHI (Liu et al., 2023c), with prominent concentrations observed in eastern provinces such as Jiangsu, Guangdong, and Shandong. This difference mainly stems from the fact that these provinces are large manufacturing and industrial provinces, and the development of their light industry, energy and chemical industry, automobile industry, and other secondary industries has increased resource and energy consumption, leading to an in-crease in the emission of pollutants, which has serious negative impacts on the quality of the atmosphere and human health. Specifically, like Shandong provinces has characteristics of energy and raw material industry basement, the development of its coal chemical industry, iron and steel industry, chemical industry, and other high-pollution and high-consumption industries has increased the consumption of resources and energy, resulting it the most energy-consuming province in China (Guan et al., 2021). Concurrently, petroleum processing, coking, and nuclear fuel processing belong to the heavy industry sector, whose production processes are usually more complex, the cost of updating equipment and environmental protection technologies is relatively high, the implementation of environmental protection technologies and measures may be more difficult, and the lag in the development of green and clean technologies may also lead to an increase in the emission of air pollutants, which will maintain the growth of PM2.5-HHI over the period 2007–2017. In addition, analyzing from both production- and consumption-side perspectives, most of the production-based PM2.5-HHI is concentrated in energy-intensive heavy industries, with Power (S22), ManNonmet. (S13), and Petro. (S11) alone collectively contributing 56% of China’s PM2.5-HHI. The Constr. (S25) and Others (S28) sectors are the largest contributors to consumption-based PM2.5-HHI, together contributing 30% of China’s PM2.5-HHI. Therefore, the potential for reducing PM2.5 emissions and lowering PM2.5-HHI lies mainly in the industrial and residential sectors, especially in the economically developed provinces in eastern China.
From the perspective of the influence of socioeconomic factors on PM2.5-HHI, changes in the structure of emission sources have a relatively small impact on PM2.5-HHI. By contrast, emission intensity plays a dominant role in reducing PM2.5-HHI in all regions. This is mainly due to the implementation of air pollution control policies, with governments adopting stricter environmental regulations and policies to limit and regulate the emission of pollutants from different industries. With the implementation of air pollution control policies, provinces have actively promoted the optimization and reform of the production structure, which has facilitated the development of a cleaner and healthier production structure. In particular, Guangdong, Henan, Zhejiang, Hebei, and other major industrial provinces have achieved notable success in optimizing their production structures to reduce PM2.5-HHI, especially during the period from 2012 to 2017. However, in some regions, such as Beijing, Tianjin, Inner Mongolia, and Anhui, the total input coefficients of Transport (S26), Who.&Cat. (S27), and Others (S28) industries such as services increased over the 2012–2017 period, driving more PM2.5-HHI growth. This may be attributed to rapid urbanization and population growth in these areas, leading to increased demand for transportation, logistics, commerce, and services. The expansion of these industries is accompanied by increased emissions from energy-intensive equipment such as internal combustion engines, diesel trucks, and diesel generators. Additionally, the rapid growth in traffic congestion reduces the efficiency of vehicle emissions, resulting in increased energy waste and emissions. Therefore, improving urban planning and promoting the use of cleaner transportation are key steps toward sustainable urban development and the reduction of PM2.5-HHI.
Changes in the final demand structure are driving the growth of PM2.5-HHI mainly in regions with higher economic levels, and this driving effect is diminishing. To avoid an increase in PM2.5-HHI, the key to the future lies in reducing waste, especially in affluent areas, and by optimizing the structure of final demand. Rising per capita demand levels are the main driver of PM2.5-HHI growth, especially in areas with higher levels of economic development. This indicates that PM2.5-HHI is significantly correlated with urbanization, the industrialization process, and economic performance (Lu et al., 2019). Analyzed from the perspective of final demand classifications, PM2.5-HHI, driven by household consumption, investment, and exports, dominated the final demand in all provinces of China. In addition, the final demand–driven PM2.5-HHI shows an evolutionary trend of an increase in the share of fixed capital formation and exports and a decrease in the share of household consumption. Energy-intensive industries and other sectors are key drivers of the growth of household consumption-driven PM2.5-HHI, especially in economically developed regions. Notably, the Power (S22) sector has become the leading driver of export-driven PM2.5-HHI growth in Beijing. This phenomenon may be attributed to Beijing’s exports being primarily focused on high-tech industries such as electronics and financial services, which rely heavily on energy sources such as electricity and heat to meet their production needs. Thus, the Power (S22) sectors expand the demand for energy consumption, especially for fuel-burning-based electricity and heat production processes, leading to more emissions of pollutants such as PM2.5 and further stimulating export-driven PM2.5-HHI growth.
In 2013, China enacted the Action Plan for Prevention and Control of Air Pollution, identifying Beijing, Shanghai, and Jiangsu as pivotal regions for combating air pollution (Yue et al., 2020). The plan aims to mitigate the environmental and health impacts of PM2.5 emissions by optimizing industrial layout, eliminating outdated technologies, promoting cleaner production, and using other strict control strategies. However, during the period 2007–2017, PM2.5-HHI in Beijing continued to increase, despite the fact that the city is one of the key regions for air pollution control. In contrast, Shanghai and Jiangsu, also key regions, both showed decreases in PM2.5-HHI between 2012 and 2017, with Shanghai in particular showing negative growth between 2007 and 2017. This trend validates, to some extent, Whang et al.’s view that existing air pollution control measures are mainly concentrated in developed coastal areas, whereas environmental regulations in inland provinces are more lenient (Wang et al., 2023a). Notably, the Action Plan for Continuous Improvement of Air Quality, which will be enacted and implemented in 2023, includes inland provinces such as Henan and Shanxi as key prevention and control areas, demonstrating the elevated attention to and treatment of inland areas. Although a series of stringent air pollution control measures implemented in the past decade have achieved remarkable results, the east-ern region will remain a priority area for future PM2.5 emission control. The prioritization of future emission treatment directions can be determined based on emission sources and regions. The shift in the contribution of pollution sources calls for adjusting policy attention to the emitting sectors (Liu et al., 2020). Industrial sectors such as Power (S22), ManNonmet. (S13), and Petro. (S11) are the largest sources of emissions. Although the Power (S22) sector has achieved significant emission reductions (Zheng et al., 2018), it still uses fossil fuels as its main energy source, and the damage to human health is still preeminent. Therefore, relevant laws and regulations should be implemented to accelerate the shift to a renewable energy-based system, especially in the industrial sector, where a cleaner energy shift is essential. In addition, population growth and rising levels of consumer demand are relevant factors contributing to the growth in PM2.5-HHI. SDAs show that de-spite the health benefits of reducing PM2.5 emission intensity through the implementation of mitigation measures and policies, in 80% of the regions, these health benefits are offset by changes in population demand and size.
The uncertainty in the results originates from the uncertainty in the quality of the PM10 emissions data in the provincial MIOT tables and the Eora database. The ideal assumption for estimating PM10 emissions across different regions is that the PM10 intensity of emissions from the same sector’s production processes remains constant within each region. This means that the PM10 emission intensity of each region adopts the PM10 emission intensity of the same industry at the overall national level in China. The focus of the study primarily lies in the differences in economic structural transformation and changes in final demand across various provinces. However, this has also led to some degree of uncertainty in the relative contributions of regional PM2.5-HHI and socioeconomic factors. In the future, based on more advanced measurement tools and methods, the accuracy of PM10 emission inventories and MIOT tables for each region may be improved, and the un-certainty of the study results can be reduced.
We performed a sensitivity assessment of the findings by calculating the elasticity of each parameter. The research results indicate that, except for population, per capita final demand level, the elasticity of the dose–response coefficient, and the proportion coefficient of PM2.5 emissions to PM10 emissions, which are all elasticities of 1, the elasticities of other parameters are extremely small. This suggests that the sensitivity of the model results is low. The highest PM10 emission intensity elasticity is found for the Power (S22) sector in Qinghai province, which is 0.6253. This means that a 10% change in PM10 emission intensity in this sector would result in a 6.25% change in PM2.5-HHI. Apart from the elasticity of PM10 emission intensity in the production sector of electricity and heat, the elasticity of final demand structure in the Constr. (S25) and Others (S28) sectors is also noteworthy (Figures 5A, B).
Figure 5. (A) PM10 emission intensity elasticity. Note: The numbers “07,” “12,” and “17” above the horizontal axis in Figures 5A, B represent the years 2007, 2012, and 2017, respectively. (B) Elasticity of final demand structure.
This study utilized EEIO and SDA models to assess the impacts of China’s regional economic structural transformation and final demand evolution on PM2.5-HHI from 2007 to 2017. The results of the study revealed significant differences in the regional and sec-toral sources of PM2.5-HHI in various regions of China. During the study period, PM2.5-HHI in 30 regions across the country generally increased to varying degrees between 2007 and 2012, but nearly half of the provinces experienced negative PM2.5-HHI growth between 2012 and 2017, and Shanghai, Heilongjiang, Liaoning, and Hebei had negative PM2.5-HHI growth between 2007 and 2017. PM2.5-HHI is mainly concentrated in the eastern provinces with manufacturing and industry, which is related to the demand for large amounts of fossil energy. The leading sectors driving production-based PM2.5-HHI include energy-intensive Power (S22), ManNonmet. (S13), and Petro. (S11), which collectively contribute to 56% of China’s PM2.5-HHI. The notable contribution stems from the fact that these sectors serve as the backbone of energy and basic materials that extensively support the operation and development of other industries. These energy-intensive sectors not only provide the necessary energy and material base for the economy and society, but are also major contributors to PM2.5-HHI due to the emission characteristics of their production processes. On the consumption-based PM2.5-HHI, Constr. (S25) and Others (S28) sectors emerge as the strongest driving forces for the growth of PM2.5-HHI, which is closely linked to their rapid expansion and vibrant economic activity. The rapid growth of the construction sector has not only exacerbated the demand for materials and energy but has also led directly to a significant increase in construction dust and combustion emissions. At the same time, diversified consumption and production patterns in other sectors also contribute to PM2.5 emissions, which together raise overall pollution levels. The wide range of activities in these sectors, the high level of emissions and the difficulty of controlling them are key factors in the growth of PM2.5-HHI on the consumption side. Overall, the potential for reducing PM2.5 emissions and lowering PM2.5-HHI exists mainly in the industrial and residential sectors, especially in the economically developed provinces in eastern China.
In the trend of PM2.5-HHI changes, changes in the structure of emission sources have a relatively small impact on PM2.5-HHI. By contrast, emission intensity plays a dominant role in reducing PM2.5-HHI in all regions. This suggests that adjusting and optimizing the structure of emission sources is essential for long-term environmental quality improvement, but direct reduction of the pollutant emission intensity of each source is a more direct and effective means at the current stage. Due to differences in resource endowment and level of economic development in different provinces, the effect of changes in production structure on driving PM2.5-HHI varies in different regions. Among these, the optimization of production structures in major industrial provinces such as Guangdong, Henan, Zhejiang, and Hebei notably decreased PM2.5-HHI. Changes in the final demand structure primarily drove PM2.5-HHI growth in regions with higher economic levels, although this driving effect is diminishing. This change stems mainly from the upgrading of the industrial structure and the transformation of consumption patterns in these regions. Although changes in the demand structure still have a certain impact on PM2.5 emissions, with the increasing awareness of environmental protection, the strict implementation of environmental protection policies, the widespread use of clean energy, and the upgrading of pollution control technologies, the intensity of emissions in these regions has gradually been reduced, thus weakening the role of the final demand structure as a driving force for PM2.5-HHI. This trend reflects the efforts and effectiveness of economically developed regions in striking a balance between economic development and environmental protection. Rising per capita demand levels are a major driver of PM2.5-HHI growth, especially in regions with higher levels of economic development. As the population migrates from the less economically developed central and western provinces to the economically developed eastern coastal provinces, this trend of population migration leads to differences in population size changes between regions, which in turn have opposite effects on PM2.5-HHI. To curb the growth of PM2.5-HHI, it is essential to formulate and improve air pollution and energy policies, promote industrial production optimization, and reduce PM2.5 emission intensity. Additionally, optimizing the structure of final demand is crucial to lowering PM2.5-HHI, such as by encouraging consumption and export of products with low PM2.5-HHI and minimizing waste, particularly in affluent areas.
Coordinating regional pollution control, strengthening the level of pollution control in key industries, reducing particulate matter emissions and effectively improving the health and wellbeing of the population. With regard to key regions and industries with high PM2.5-HHI, there is an urgent need to strengthen synergistic management of regional pollution control and precise control of key pollution sources. The pollution monitoring system in key regions should be strengthened, and management efforts should be intensified to curb the surge in particulate matter emissions brought about by blind investment expansion and disorderly growth in production capacity. At the same time, we are actively promoting the transformation and upgrading of enterprises in key industries such as Power (S22), ManNonmet. (S13), and Petro. (S11), and the wide application of cleaner production technologies, energy-saving and consumption-reducing technologies, and environmentally friendly equipment, so as to not only strengthen the emission reduction measures at the end of the production process, but also to promote the green transformation of the entire production process, so as to achieve comprehensive PM2.5-HHI control from the source to the end. In addition, the regulation of structural emission reduction measures in the eastern region should be strengthened, especially the implementation of special regulation of highly pollution-intensive industries to prevent the transfer of pollution to the central and western regions.
Promote clean energy and efficient production technologies, strengthen emission standards, change consumption patterns and encourage green and low-carbon living. Reducing emissions intensity has had a significant effect in reducing PM2.5-HHI, but changes in final demand continue to drive PM2.5-HHI growth. Therefore, the PM2.5 emission intensity of enterprises should be reduced through the promotion of clean energy and efficient production technologies, as well as the strengthening of emission standards. It also promotes energy-saving and green consumption patterns and encourages consumers to shift to low PM2.5-HHI products to meet the challenges posed by growing consumer demand, especially in regions with higher levels of economic development.
The study is limited by the lack of PM10 emission data at the regional level. We assume that the PM10 emission intensity in each region remains consistent with the national level throughout the same period. We measure changes in PM2.5-HHI with total economic output over the study period and focus on exploring the impact of economic structural transformation and changes in final demand on PM2.5-HHI. As the data are updated, we will continue to further refine the study. Additionally, addressing the insufficient research on air exposure and climate environmental impacts, we plan to conduct a systematic assessment of the effects of PM2.5 on human health based on atmospheric chemistry transport models and exposure–response models.
The original contributions presented in the study are included in the article/supplementary material, further inquiries can be directed to the corresponding author.
YW: Methodology, Supervision, Writing–review and editing. XaZ: Conceptualization, Data curation, Formal Analysis, Methodology, Software, Visualization, Writing–original draft, Writing–review and editing. YH: Data curation, Writing–review and editing. XD: Data curation, Formal Analysis, Software, Writing–review and editing. XnZ: Formal Analysis, Visualization, Writing–review and editing. YS: Data curation, Visualization, Writing–review and editing.
The author(s) declare that financial support was received for the research, authorship, and/or publication of this article. This study was supported by the Fundamental Research Funds for the Central Universities (Ph.D. Top Innovative Talents Fund of CUMTB) (NO.BBJ2024075).
The authors are grateful to reviewers and editors for helpful comments and suggestions.
The authors declare that the research was conducted in the absence of any commercial or financial relationships that could be construed as a potential conflict of interest.
All claims expressed in this article are solely those of the authors and do not necessarily represent those of their affiliated organizations, or those of the publisher, the editors and the reviewers. Any product that may be evaluated in this article, or claim that may be made by its manufacturer, is not guaranteed or endorsed by the publisher.
Cony Renaud Salis, L., Abadie, M., Wargocki, P., and Rode, C. (2017). Towards the definition of indicators for assessment of indoor air quality and energy performance in low-energy residential buildings. Energy Build. 152, 492–502. doi:10.1016/j.enbuild.2017.07.054
Dong, J., Li, S., Xing, J., Sun, Y., Yang, J., Ren, L., et al. (2022). Air pollution control benefits in reducing inter-provincial trade-associated environmental inequality on PM2.5-related premature deaths in China. J. Clean. Prod. 350, 131435. doi:10.1016/j.jclepro.2022.131435
Feng, C., Dong, L., Adbiat, M., Xu, L., and Yu, A. (2023). Critical transmission sectors in China’s energy supply chains. Energy 266, 126492. doi:10.1016/j.energy.2022.126492
Feng, T., Chen, H., and Liu, J. (2022). Air pollution-induced health impacts and health economic losses in China driven by US demand exports. J. Environ. Manag. 324, 116355. doi:10.1016/j.jenvman.2022.116355
Forouzanfar, M. H., Afshin, A., Alexander, L. T., Anderson, H. R., Bhutta, Z. A., Biryukov, S., et al. (2016). Global, regional, and national comparative risk assessment of 79 behavioural, environmental and occupational, and metabolic risks or clusters of risks, 1990–2015: a systematic analysis for the Global Burden of Disease Study 2015. Lancet 388 (10053), 1659–1724. doi:10.1016/S0140-6736(16)31679-8
Geng, G., Xiao, Q., Liu, S., Liu, X., Cheng, J., Zheng, Y., et al. (2021). Tracking air pollution in China: near real-time PM2.5 retrievals from multisource data fusion. Environ. Sci. and Technol. 55 (17), 12106–12115. doi:10.1021/acs.est.1c01863
Gronlund, C. J., Humbert, S., Shaked, S., O’Neill, M. S., and Jolliet, O. (2015). Characterizing the burden of disease of particulate matter for life cycle impact assessment. Air Qual. Atmos. and Health 8 (1), 29–46. doi:10.1007/s11869-014-0283-6
Guan, D., Su, X., Zhang, Q., Peters, G. P., Liu, Z., Lei, Y., et al. (2014). The socioeconomic drivers of China’s primary PM2.5 emissions. Environ. Res. Lett. 9 (2), 024010. doi:10.1088/1748-9326/9/2/024010
Guan, Y., Rong, B., Kang, L., Zhang, N., and Qin, C. (2023). Measuring the urban-rural and spatiotemporal heterogeneity of the drivers of PM2.5-attributed health burdens in China from 2008 to 2021 using high-resolution dataset. J. Environ. Manag. 346, 118940. doi:10.1016/j.jenvman.2023.118940
Guan, Y., Shan, Y., Huang, Q., Chen, H., Wang, D., and Hubacek, K. (2021). Assessment to China’s recent emission pattern shifts. Earth’s Future 9 (11), e2021EF002241. doi:10.1029/2021EF002241
He, S., Zhao, L., Ding, S., Liang, S., Dong, L., Wang, J., et al. (2019). Mapping economic drivers of China’s NOx emissions due to energy consumption. J. Clean. Prod. 241, 118130. doi:10.1016/j.jclepro.2019.118130
Hu, Y., Chao, K., Zhu, Z., Yue, J., Qie, X., and Wang, M. (2024). A study on a health impact assessment and healthcare cost calculation of beijing-tianjin-hebei residents under PM2.5 and O3 pollution. SUSTAINABILITY 16 (10), 4030. doi:10.3390/su16104030
Lenzen, M., Moran, D., Kanemoto, K., and Geschke, A. (2013). Building Eora: a global multi-region input–output database at high country and sector resolution. Econ. Syst. Res. 25 (1), 20–49. doi:10.1080/09535314.2013.769938
Li, C., Zhang, B., Lai, Y., Dong, M., and Li, D. (2019). Does the trans-regional transfer of resource-oriented enterprises generate a stress effect? Resour. Policy 64, 101524. doi:10.1016/j.resourpol.2019.101524
Li, R., Zhang, J., and Krebs, P. (2021). Consumption- and income-based sectoral emissions of polycyclic aromatic hydrocarbons in China from 2002 to 2017. Environ. Sci. and Technol. 55 (6), 3582–3592. doi:10.1021/acs.est.0c08119
Li, Y., Li, B., Liao, H., Zhou, B.-B., Wei, J., Wang, Y., et al. (2023). Changes in PM2.5-related health burden in China’s poverty and non-poverty areas during 2000–2020: a health inequality perspective. Sci. Total Environ. 861, 160517. doi:10.1016/j.scitotenv.2022.160517
Liang, S., Stylianou, K. S., Jolliet, O., Supekar, S., Qu, S., Skerlos, S. J., et al. (2017). Consumption-based human health impacts of primary PM2.5: the hidden burden of international trade. J. Clean. Prod. 167, 133–139. doi:10.1016/j.jclepro.2017.08.139
Liang, S., Wang, Y., Cinnirella, S., and Pirrone, N. (2015). Atmospheric mercury footprints of Nations. Environ. Sci. and Technol. 49 (6), 3566–3574. doi:10.1021/es503977y
Liu, J., Zheng, Y., Geng, G., Hong, C., Li, M., Li, X., et al. (2020). Decadal changes in anthropogenic source contribution of PM2.5 pollution and related health impacts in China, 1990–2015. Atmos. Chem. Phys. 20 (13), 7783–7799. doi:10.5194/acp-20-7783-2020
Liu, L. H., Wang, L., An, S. X., and Feng, T. T. (2023b). More is less: economic structural transformation and economic catch-up in developing countries. J. Finance Econ. 49, 94–108. (in Chinese). doi:10.16538/j.cnki.jfe.20221217.202
Liu, L.-J., Liang, Q.-M., and Shuai, Y.-X. (2023a). Common driving forces of provincial-level greenhouse Gas and air pollutant emissions in China. Environ. Sci. and Technol. 57 (14), 5806–5820. doi:10.1021/acs.est.2c09309
Liu, M., Lei, Y., Wang, X., Xue, W., Zhang, W., Jiang, H., et al. (2023c). Source contributions to pm2.5-related mortality and costs: evidence for emission allocation and compensation strategies in China. Environ. Sci. and Technol. 57 (12), 4720–4731. doi:10.1021/acs.est.2c08306
Lu, X., Lin, C., Li, W., Chen, Y., Huang, Y., Fung, J. C. H., et al. (2019). Analysis of the adverse health effects of PM2.5 from 2001 to 2017 in China and the role of urbanization in aggravating the health burden. Sci. Total Environ. 652, 683–695. doi:10.1016/j.scitotenv.2018.10.140
Mueller, W., Vardoulakis, S., Steinle, S., Loh, M., Johnston, H. J., Precha, N., et al. (2021). A health impact assessment of long-term exposure to particulate air pollution in Thailand. Environ. Res. Lett. 16 (5), 055018. doi:10.1088/1748-9326/abe3ba
Munday, M., and Beynon, M. J. (2011). “Input-output analysis: foundations and extensions, by ronald E. Miller and peter D. Blair,” J. Regional Sci. Editors R. E. Miller, and P. D. Blair, 51, 196–197. doi:10.1111/j.1467-9787.2010.00711_2.x
Ostro, B. (2004). Outdoor air pollution: assessing the environmental burden of disease at national and local levels. Environ. Burd. Dis. doi:10.3923/pjn.2009.691.694
Song, J., Wang, B., Yang, W., Duan, H., and Liu, X. (2020). Extracting critical supply chains driving air pollution in China. J. Clean. Prod. 276, 124282. doi:10.1016/j.jclepro.2020.124282
Southerland, V. A., Brauer, M., Mohegh, A., Hammer, M. S., van Donkelaar, A., Martin, R. V., et al. (2022). Global urban temporal trends in fine particulate matter (PM2·5) and attributable health burdens: estimates from global datasets. Lancet Planet. Health 6 (2), e139–e146. doi:10.1016/S2542-5196(21)00350-8
Sun, X., Zhang, R., Yu, Z., Zhu, S., Qie, X., Wu, J., et al. (2024a). Revisiting the porter hypothesis within the economy-environment-health framework: empirical analysis from a multidimensional perspective. J. Environ. Manag. 349, 119557. doi:10.1016/j.jenvman.2023.119557
Sun, X., Zhu, S., Guo, J., Peng, S., Qie, X., Yu, Z., et al. (2024b). Exploring ways to improve China’s ecological well-being amidst air pollution challenges using mixed methods. J. Environ. Manag. 364, 121457. doi:10.1016/j.jenvman.2024.121457
Sun, Y.-F., Yu, S., Zhang, Y.-J., and Su, B. (2023). How do imports change the energy consumption of China? An analysis of its role in intermediate inputs and final demands. Energy 270, 126947. doi:10.1016/j.energy.2023.126947
United, N. (1999). Handbook of input-output table compilation and analysis. Stud. Methods Ser. F (74). Available at: https://digitallibrary.un.org/record/370160.
Wang, C., Wang, Y., Shi, Z., Sun, J., Gong, K., Li, J., et al. (2021). Effects of using different exposure data to estimate changes in premature mortality attributable to PM2.5 and O3 in China. Environ. Pollut. 285, 117242. doi:10.1016/j.envpol.2021.117242
Wang, E., Su, B., Zhong, S., and Guo, Q. (2022a). China’s embodied SO2 emissions and aggregate embodied SO2 intensities in interprovincial and international trade. Technol. Forecast. Soc. Change 177, 121546. doi:10.1016/j.techfore.2022.121546
Wang, H., Li, X., Tian, X., Ma, L., Wang, G., Wang, X., et al. (2022b). Socioeconomic drivers of China’s resource efficiency improvement: a structural decomposition analysis for 1997–2017. Resour. Conservation Recycl. 178, 106028. doi:10.1016/j.resconrec.2021.106028
Wang, J., Zhou, S., Huang, T., Ling, Z., Liu, Y., Song, S., et al. (2023a). Air pollution and associated health impact and economic loss embodied in inter-provincial electricity transfer in China. Sci. Total Environ. 883, 163653. doi:10.1016/j.scitotenv.2023.163653
Wang, S., Wang, J., Chen, X., Fang, C., Hubacek, K., Liu, X., et al. (2023b). Impact of international trade on the carbon intensity of human well-being. Environ. Sci. and Technol. 57 (17), 6898–6909. doi:10.1021/acs.est.2c07582
Wang, Y., Wang, X., Wang, H., Zhang, X., Zhong, Q., Yue, Q., et al. (2022). Human health and ecosystem impacts of China’s resource extraction. Sci. Total Environ. 847, 157465. doi:10.1016/j.scitotenv.2022.157465
Wen, W., Su, Y., Yang, X., Liang, Y., Guo, Y., and Liu, H. (2024). Global economic structure transition boosts PM2.5-related human health impact in Belt and Road Initiative. Sci. Total Environ. 916, 170071. doi:10.1016/j.scitotenv.2024.170071
Wu, X., Yang, X., Qi, J., Feng, C., and Liang, S. (2021). Effects of economic structural transition on pm2.5-related human health impacts in China. J. Clean. Prod. 298, 126793. doi:10.1016/j.jclepro.2021.126793
Yang, M., Liang, X., Chen, H., Ma, Y., and Gulibaiheremu, A. (2024). Analysis of the evolution and drivers of carbon inequality based on a human well-being equity perspective. J. Clean. Prod. 448, 141706. doi:10.1016/j.jclepro.2024.141706
Yue, H., He, C., Huang, Q., Yin, D., and Bryan, B. A. (2020). Stronger policy required to substantially reduce deaths from PM2.5 pollution in China. Nat. Commun. 11 (1), 1462. doi:10.1038/s41467-020-15319-4
Zhai, X.-Q., Xue, R., He, B., Yang, D., Pei, X.-Y., Li, X., et al. (2022). Dynamic changes and convergence of China’s regional green productivity: a dynamic spatial econometric analysis. Adv. Clim. Change Res. 13 (2), 266–278. doi:10.1016/j.accre.2022.01.004
Zhang, F., Xing, J., Zhou, Y., Wang, S., Zhao, B., Zheng, H., et al. (2020). Estimation of abatement potentials and costs of air pollution emissions in China. J. Environ. Manag. 260, 110069. doi:10.1016/j.jenvman.2020.110069
Zhang, G., Han, J., and Su, B. (2023a). Contributions of cleaner production and end-of-pipe treatment to NOx emissions and intensity reductions in China, 1997–2018. J. Environ. Manag. 326, 116822. doi:10.1016/j.jenvman.2022.116822
Zhang, L., Yang, D., and Guo, Y. (2023b). Dual circulation development model and credit growth. Finance Res. Lett. 55, 103873. doi:10.1016/j.frl.2023.103873
Zhang, Z., Shao, C., Guan, Y., and Xue, C. (2019). Socioeconomic factors and regional differences of PM2.5 health risks in China. J. Of Environ. Manag. 251, 109564. doi:10.1016/j.jenvman.2019.109564
Zhao, F., Hu, Z., Yi, P., and Zhao, X. (2024). Does environmental decentralization improve industrial ecology? Evidence from China’s Yangtze River Economic Belt. Econ. Analysis Policy 82, 1250–1270. doi:10.1016/j.eap.2024.05.013
Zhao, H., Wu, R., Liu, Y., Cheng, J., Geng, G., Zheng, Y., et al. (2023). Air pollution health burden embodied in China’s supply chains. Environ. Sci. Ecotechnology 16, 100264. doi:10.1016/j.ese.2023.100264
Zheng, B., Tong, D., Li, M., Liu, F., Hong, C., Geng, G., et al. (2018). Trends in China’s anthropogenic emissions since 2010 as the consequence of clean air actions. Atmos. Chem. Phys. 18 (19), 14095–14111. doi:10.5194/acp-18-14095-2018
Keywords: economic structural transformation, PM2.5-HHI, final demand evolution, structural decomposition analysis, input-output analysis, regional heterogeneity
Citation: Wang Y, Zhang X, Hu Y, Du X, Zhao X and Sun Y (2024) Quantitative assessment of PM2.5-related human health impacts at the provincial level in China and analysis of its heterogeneity affected by economic structural transformation. Front. Environ. Sci. 12:1478649. doi: 10.3389/fenvs.2024.1478649
Received: 10 August 2024; Accepted: 07 October 2024;
Published: 22 October 2024.
Edited by:
Sam Agatre Okuonzi, Ministry of Health, UgandaReviewed by:
Beidi Diao, China University of Mining and Technology, ChinaCopyright © 2024 Wang, Zhang, Hu, Du, Zhao and Sun. This is an open-access article distributed under the terms of the Creative Commons Attribution License (CC BY). The use, distribution or reproduction in other forums is permitted, provided the original author(s) and the copyright owner(s) are credited and that the original publication in this journal is cited, in accordance with accepted academic practice. No use, distribution or reproduction is permitted which does not comply with these terms.
*Correspondence: Xiaoyi Zhang, amF5ZGVuemhhbmdAYWxpeXVuLmNvbQ==
Disclaimer: All claims expressed in this article are solely those of the authors and do not necessarily represent those of their affiliated organizations, or those of the publisher, the editors and the reviewers. Any product that may be evaluated in this article or claim that may be made by its manufacturer is not guaranteed or endorsed by the publisher.
Research integrity at Frontiers
Learn more about the work of our research integrity team to safeguard the quality of each article we publish.