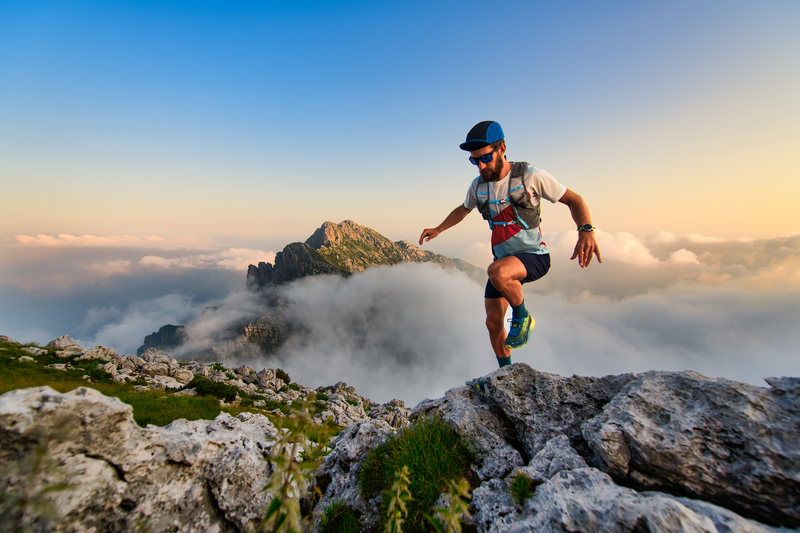
94% of researchers rate our articles as excellent or good
Learn more about the work of our research integrity team to safeguard the quality of each article we publish.
Find out more
ORIGINAL RESEARCH article
Front. Environ. Sci. , 14 November 2024
Sec. Freshwater Science
Volume 12 - 2024 | https://doi.org/10.3389/fenvs.2024.1476014
Water hyacinth (Pontederia crassipes) is an invasive weed that covers a significant portion of Lake Tana. The infestation has an impact on the lake’s ecological and socioeconomic systems. Early detection of the spread of water hyacinth using geospatial techniques is crucial for its effective management and control. The main objective of this study was to examine the spatiotemporal distribution of water hyacinth from 2016 to 2022 using a random forest machine learning model. The study used 16 variables obtained from Sentinel-2A, Sentinel-1 SAR, and SRTM DEM, and a random forest supervised classification model was applied. Seven spectral indices, five spectral bands, two Sentinel-1 SAR bands, and two topographic variables were used in combination to model the spatial distribution of water hyacinth. The model was evaluated using the overall accuracy and kappa coefficient. The findings demonstrated that the overall accuracy ranged from 0.91 to 0.94 and kappa coefficient from 0.88 to 0.92 in the wet season and 0.93 to 0.95 and 0.90 to 0.93 in the dry season, respectively. B11 and B5 (2022), VH, soil adjusted vegetation index (SAVI), and normalized difference water index (NDWI) (2020), B5 and B12 (2018), and VH and slope (2016) are the highly important variables in the classification. The study found that the spatial coverage of water hyacinth was 686.5 and 650.4 ha (2016), 1,851 and 1,259 ha (2018), 1,396.7 and 1,305.7 ha (2020), and 1,436.5 and 1,216.5 ha (2022) in the wet and dry seasons, respectively. The research findings indicate that variables derived from optical (Sentinel-2A and SRTM) and non-optical (Sentinel-1 SAR) satellite imagery effectively identify water hyacinth and display its spatiotemporal spread using the random forest machine learning algorithm.
Water hyacinth, scientifically known as Pontederia crassipes, is an aquatic plant that originates from South America. However, it has been introduced to numerous other regions globally, where it frequently becomes invasive and leads to ecological issues (Coetzee et al., 2009; Jones, 2009; Degaga, 2018; Ayanda et al., 2020; Simpson et al., 2022). It was brought into several countries of Europe, Africa, and North America over 100 years ago as an ornamental aquatic plant species to adorn the water bodies due to their appealing blue, lilac, or purplish flowers and round or oval leaves (Bhattacharya and Kumar, 2010; Zhang et al., 2010). Over time, in the place of introduction into new habitats, the plant’s status has evolved from being a beautiful ornamental plant to an invasive alien species (Laranjeira and Nadais, 2008; Wang and Yan, 2017). This is due to its rapid reproduction through both seeds and vegetative propagation (Barrett, 1980), formation of mats on the water’s surface (Zhang and Guo, 2017), and alteration of aquatic habitats by outcompeting native aquatic plants (Stiers et al., 2011), reducing biodiversity and disrupting the natural balance of the ecosystem (Ayyad, 2003; Williams and Hecky, 2005). Due to its high dispersal and growth capacity, the species is ranked on the 100 worst invasive plant species list, as reported by the International Union for Conservation of Nature (IUCN) (Patel, 2012; Cordeiro et al., 2020). Water hyacinth has become a danger to many aquatic habitats worldwide (Ayanda et al., 2020). Infestation has several detrimental ecological and social effects that endanger aquatic systems, prevent people from using surface waters, and impair hydraulic structures such as canals and pumping places (Gerardo and de Lima, 2022). It obstructs navigation and fishing (Hill and Coetzee, 2008), harms infrastructure, increases service costs, weakens riparian populations, and changes ecology (Laranjeira and Nadais, 2008; Julien, 2008). Water hyacinth, which displaces native aquatic plants and animals groups, is regarded as the most notorious weed in freshwater ecosystems throughout the world’s warm and tropical regions (Rezene, 2005).
Ethiopia is home to 35 terrestrial and aquatic alien plant species that have become invasive (Tasew and Wendimagegnehu, 2022). Among the aquatic alien species such as duckweeds and papyrus, water hyacinth is the most notorious and widely spreading in the lakes and freshwater resources of Ethiopia. It negatively affects slow-moving freshwater systems such as ponds, dams, lakes, rivers, and wetlands found in the rift valley regions of Ethiopia and the Lake Tana basin (Gedefaw and Gondar, 2018). The water quality is affected in a number of ways, following water hyacinth invasion. Dissolved oxygen levels are reduced beneath the floating mat by decreasing the natural transfer of oxygen from the air at the water surface (Gebremeskel, 2024). In addition, decomposing dead plant material can further reduce the amount of dissolved oxygen and increase sedimentation and silting in the water (Mironga et al., 2012). All of these factors lead to a decrease in the water quality. The decreased water oxygen levels result in a changed aquatic habitat, reducing fish diversity and impacting other aquatic flora and fauna communities (Abera, 2017).
Water hyacinth poses a significant threat to the livelihoods of people living around Lake Tana in Ethiopia. This invasive plant has harmful effects on the lake’s ecosystem, biodiversity, fisheries, and agriculture (Kibret, 2017; Enyew et al., 2020; Damtie et al., 2022; Cai et al., 2023). In Lake Tana, the coverage of water hyacinth has rapidly grown (Cai et al., 2023). It covered between 80 and 100 hectares (ha) when it first appeared in 2011 and was limited to one kebele located around the mouth of the Megech River (north of Lake Tana). It first appeared in some pocket grazing and wet farm areas, proliferating and covering shoreline habitats in the Ribb and Dirma river mouths (Wondie, 2012). Within a year, it had spread to approximately 20,000 ha (Tewabe, 2015). Despite significant efforts and investments by stakeholders each year, its coverage continues to increase over time (Enyew et al., 2020). Since 2014, the weed has increased in space and time and infested about 9 woredas (Takusa, West Dembia, East Dembia, Gondar Zuria, Libo Kemkem, Fogera, Dera, and Bahir Dar Zuria) and about 30 kebeles. The weed expands beyond its original area and moves toward the north, northeast, and eastern parts of the lake much faster than other types of coverage due to the wind direction and wind-induced wave direction that moves the weed toward the east (Dersseh et al., 2019), posing a threat to the lake’s sustainability and the riparian community, who rely on the lake for their livelihoods (Asmare et al., 2020). Moreover, water hyacinth has had a negative effect on fishing activities (Asmare, 2017) and biotic communities (Abera, 2017; Gezie et al., 2018). It has also affected pasture and agricultural land use (Dechassa and Abate, 2020; Damtie and Mengistu, 2022) and livestock and crop production (Enyew et al., 2020). The invasion has significant implications for pasture and agricultural land use, primarily affecting agricultural productivity and the accessibility of farming areas. The presence of water hyacinth can lead to the loss of pasture and agricultural land due to its invasive nature. As it spreads, it can outcompete native vegetation, leading to a decrease in indigenous grasses and other plants vital for both livestock grazing and crop production (Churko et al., 2023). The destruction of these natural habitats not only impacts the availability of forage for livestock but also reduces the overall resilience of agricultural systems to environmental changes (Ilo et al., 2020; Dersseh et al., 2019; Endgaw, 2021; Begum et al., 2021). It also affects agricultural land use by blocking irrigation channels and waterways essential for supplying water to crops. The dense mats impede water flow and reduce water availability for irrigation (Arp et al., 2017; Hammam et al., 2022; Churko et al., 2023). This situation is particularly detrimental in regions heavily dependent on irrigation for agriculture, resulting in decreased crop yields and financial losses for farmers (Enyew et al., 2020; Churko et al., 2023). Furthermore, the high water consumption of water hyacinth exacerbates water scarcity issues, further straining agricultural practices (Hammam et al., 2022). Despite years of effort and resources dedicated to reducing its rapid expansion and negative effects, water hyacinth remains one of the most harmful aquatic invasive weeds in Lake Tana and its surrounding areas (Enyew et al., 2020).
The use of geospatial technology in identifying and mapping water hyacinth is crucial. These technologies provide valuable data on the geographic dispersal and extent of water hyacinth infestations. Multispectral satellite imagery helps discriminate reflectance values in different spectral bands, allowing for the accurate mapping of water hyacinth distribution, understanding of underlying factors, and development of effective management strategies across lakes (Thamaga and Dube, 2018; Begam et al., 2021; Chen et al., 2021). Effective and efficient monitoring largely depends on the accurate detection of weed spatial distribution. Since water hyacinth shows potential to impact biodiversity, ecosystem function, and services, it is imperative to address it in aquatic habitats (Robles et al., 2015; Pádua et al., 2022). Therefore, having up-to-date spatially explicit data and information about its dispersion and early identification is essential to comprehend its spatial arrangement and propagation rate (Thamaga and Dube, 2019). Asmare et al. (2020), Dersseh et al. (2020), and Bayable et al. (2023) investigated the spatiotemporal distribution of water hyacinth in Lake Tana using geospatial techniques. In particular, Bayable et al. (2023) utilized a machine learning-based classification method that relied on variables derived from spectral indices and bands. However, they used optical satellite imagery, which limited the range of variables to optical bands. In addition, the wavelength range and detection capability of the optical images for aquatic plant species, such as duckweed, papyrus, and hippo grass, which are prevalent in the coastal regions of Lake Tana, are limited. Therefore, optical imagery alone cannot detect the narrow reflectance differences between those plants. However, non-optical radar remote sensing offers significant advantages in detecting and monitoring aquatic vegetation, including both submerged and floating plants, in diverse water bodies. The key advantage lies in the ability of radar remote sensing to capture spectral reflectance within a longer wavelength, unaffected by cloud cover or other atmospheric conditions. The use of synthetic aperture radar (SAR) data, such as those from Sentinel-1, for monitoring aquatic weeds like water hyacinth, duckweed, and papyrus is advantageous due to several unique characteristics of radar technology. Unlike optical sensors, which can be hindered by cloud cover and limited visibility conditions, SAR can capture data day or night and under various weather conditions, making it particularly useful for the continuous monitoring of aquatic environments (Datta et al., 2021; Duc and Tong, 2021; Rowan and Kalacska, 2021). This capability is crucial for detecting the spatial distribution of invasive species, which often fluctuates with seasonal changes in water levels and vegetation growth. Sentinel-1 SAR is effective in distinguishing between different types of aquatic vegetation due to its sensitivity to the structural characteristics of the plants. Water hyacinth, for example, has a unique backscatter signature compared to other aquatic plants like duckweed and papyrus. The differences in reflectance can be attributed to the varying surface roughness and moisture content of these plants, which affect how radar waves interact with them (Simpson et al., 2022). Studies have shown that the dual-polarization capabilities of Sentinel-1 (VV and VH) enhance the detection accuracy of water hyacinth, yielding true positive detection rates of approximately 95% (Simpson et al., 2022). This high accuracy is essential for the effective management and control of invasive species.
In terms of spectral characteristics, water hyacinth typically exhibits higher reflectance in the infrared spectrum than duckweed, papyrus, and other aquatic vegetation, which can be attributed to its thicker leaves and denser structure. This difference allows for effective discrimination between these species using radar data (Simpson et al., 2022). The temporal detection capacity of Sentinel-1 is also noteworthy; it can monitor both submerged and floating water hyacinth across different seasons. For instance, during the rainy season, when water levels rise, submerged portions of water hyacinth may be detected, while in the dry season, floating mats become more prominent (Worqlul et al., 2020). This temporal flexibility is crucial for understanding the dynamics of aquatic weed populations and their ecological impacts.
Furthermore, the integration of SAR data with optical data from sensors like Sentinel-2 can enhance the overall monitoring capabilities. Although Sentinel-2A provides high-resolution optical imagery that can capture detailed vegetation indices, SAR complements this by providing consistent data, regardless of weather conditions, thus allowing for a more comprehensive analysis of aquatic vegetation dynamics (Elkhrachy et al., 2021; Singh et al., 2020). The combination of these data sources can improve the accuracy of mapping and monitoring efforts, ultimately aiding in the management of invasive aquatic species.
In addition, Bayable et al. (2023) did not consider topographic variables such as elevation and slope in their variable list. Valta-Hulkkonen et al. (2004) reported that the inclusion of topographic variables enables the identification of land use/land cover and helps improve classification accuracy for aquatic vegetation. As a result, this study examines the spatial coverage and temporal distribution of water hyacinth from 2016 to 2022 using a random forest (RF) machine learning model by considering optical, radar, and topographic variables. This study will provide a valuable contribution to the geographic extent of water hyacinth, assisting stakeholders in their management efforts. By accurately mapping and assessing the spatial coverage of the weed, this research will support informed decision-making and enable the development of effective strategies for controlling and mitigating this invasive species. The findings will empower stakeholders to prioritize areas for intervention, allocate resources efficiently, and implement targeted management actions to address the challenges posed by water hyacinth infestation.
The Lake Tana basin, which is the source of the Abay River, is situated in Ethiopia’s northwest highland at an elevation ranging from 1,674 to 4,104 meters (m) (Figure 1). In terms of geographical coordinates, it spans from 11° 00' 00'' to 12° 40' 00'' N and from 36° 40' 00'' to 38° 20' 00'' E. It is located in the Abay River basin (Figure 1). The total catchment area of the basin is approximately 15,000 km2 of which Lake Tana covers approximately 3,060 km2 (Kumlachew et al., 2023). The maximum depth of Lake Tana is 14 m, and the average depth is 8 m (Tibebe et al., 2019). The lake is drained by over 40 rivers, with Gilgel Abay being the largest from the south, followed by the Ribb and Gumara from the east and the Megech River from the north (Enyew et al., 2020). There are 347 kebeles and 21 woredas that make up the Lake Tana watershed.
The basin experiences a tropical highland monsoon climate. The primary period of rainfall is from June to September, which contributes to around 70%–90% of the overall annual precipitation. The basin experiences a mean annual rainfall of approximately 816–1,200 mm (Mequanent et al., 2021). The average air temperature throughout the year is approximately 19°C–23°C (Worqlul et al., 2020). Lake Tana serves as a multipurpose water resource that supports the livelihoods of millions of individuals in the region. The area surrounding the lake is known for its fertile soil, which has facilitated productive agricultural practices and vibrant fishing activities. Approximately 80% of the population relies on agriculture and engages in subsistence smallholder farming that encompasses a diverse variety of crops, such as sorghum, millet, rice, and maize (Abebe and Minale, 2017). Aside from agriculture, the Lake Tana region also benefits from other sectors, such as tourism, fishing, livestock breeding, and small-scale manufacturing and marketing enterprises (Birara et al., 2018).
This study used Sentinel-2A imageries, which were acquired free of charge from Sentinel Hub (https://scihub.copernicus.eu/) with a cloud cover less than 10%. The image was downloaded for the 2016–2022 wet (June, July, August, and September) and dry seasons (January, February, March, April, and May). This is due to the variation in geographic infestation coverage across seasons. The water hyacinth coverage in Lake Tana is larger during the wet season than that in the dry season (Thamaga and Dube, 2019; Bayable et al., 2023). From the Sentinel-2A satellite, seven indices were derived as covariates for modeling (Table 1). In addition, a total of five bands (B4, B5, B8, B11, and B12) were used to model the spatiotemporal spread of water hyacinth (Table 2).
Table 1. Remote sensing indices and predictor variables derived from Sentinel-2A satellite imagery for modeling water hyacinth using the random forest algorithm.
Table 2. List of variables for spatiotemporal change detection from Sentinel-2A, Sentinel-1 SAR, and SRTM imagery.
The study also used Sentinel-1 SAR image data. SAR data are excellent in areas with a regular cloud cover as it can pass through clouds and function under all weather conditions. Consequently, two bands (VV and VH) were used as predictive variables (Table 2). Furthermore, the slope and elevation data were downloaded from SRTM (https://www.earthdata.nasa.gov) with a 30-m spatial resolution and were used as a topographic variable. Topographic variables are valuable in land use/land cover mapping. They provide insights into the physical characteristics of the land that influence the distribution and patterns of land cover.
The main target of this study was to detect the spatial and temporal dynamics of water hyacinth in Lake Tana. Therefore, the study classified the land use/land cover of Lake Tana and the surrounding area into three groups, namely, water hyacinth, water body, and mixed (Table 3). The mixed class is used to train the model and accurately distinguish water hyacinth from riparian green vegetations that have similar spectral reflectance.
Distortions in remotely sensed images are caused by changes in illumination, weather, and sensor noise (Mukarugwiro et al., 2019). As a result, preprocessing assists in enhancing image quality and data. Hence, all variables were projected to WGS 1984 UTM Zone 37. In addition, digital numbers were converted into reflectance (top of the atmosphere). On the other hand, the Sentinel-2A image bands with different spatial resolutions of 10, 20, and 60 m were resampled to 10 m using the nearest neighbor resampling method. The remote sensing image was clipped to the extent of the study area. After clipping, the image was mosaicked to create multilayer raster images.
The land use/land cover classification was based on the pixel-based image classification method. The study applied a supervised classification method. Due to its robustness and accuracy among classification algorithms (Mukarugwiro et al., 2019; Bayable et al., 2023), an RF machine learning-based classification was applied. RF is an algorithm for machine learning commonly applied for land use and land cover classification. It provides accurate classification results with high overall accuracy and robustness in training (Gislason et al., 2006; Kulkarni and Lowe, 2016; Nguyen et al., 2018). RF is an ensemble method that makes predictions by combining several decision trees. Every decision tree within the RF is trained using randomly selected features and a random subset of data. At the end, all the individual predictions were summed up to form a final prediction (Rodriguez-Galiano et al., 2012; Adam et al., 2014; Svoboda et al., 2022). To train the RF model, the training data were labeled with ground control points (GCPs) and their corresponding land use/land cover classes. The model can then classify new data points based on their input features.
To determine the accuracy of the classified map using machine learning RF classifiers, a separate test dataset was used, and its results were presented using a confusion matrix. The RF machine learning model was trained with 70% of the GCP, and the remaining 30% was used to test the accuracy level. Modeling was executed using the Google Earth Engine platform. The classification accuracy was assessed by evaluating both the overall accuracy and the kappa coefficient. Asmare et al. (2020) reported that for supervised classification, a minimum of 30 points are needed. Therefore, more than 150 GCPs were used in the post-classification accuracy assessment. According to Bharatkar and Patel (2013), the classification accuracy of an image is calculated through the following formula:
where Po represents the proportion of units that exhibit agreement, while Pc represents the proportion of units expected to have chance agreement. If the kappa value is <0.4, the classification is poor; if it is between 0.4 and 0.75, it is a good kappa value; and if K > 0.75, it is an excellent kappa value.
The study utilized a total of 16 variables, including Sentinel-2A bands, indices, Sentinel-1 SAR bands, and topography (Table 2 and Figure 2). These variables demonstrated varying levels of performance in different years and seasons. The importance of each variable is influenced by the response variable (GCPs) used to train the model.
The most crucial variables during the dry season of the study period were the normalized difference aquatic vegetation index (NDAVI), soil adjusted vegetation index (SAVI), B5, B8, B4, and normalized difference vegetation index (NDVI) (Figure 3). However, topographic variables like slope and elevation, as well as SAR bands, also made significant contributions to the classification. For the years 2022 and 2020, NDAVI, SAVI, and B5 were the most relevant variables (Figures 3A, B, respectively). In 2018, B8, elevation, and B4 were highly important, while NDVI and B5 played a crucial role in 2016 (Figures 3C, D, respectively). This indicates that the bands and indices of Sentinel-2A imagery in near-infrared (NIR) and shortwave infrared (SWIR) wavelength regions were useful for the detection of water hyacinth and its surrounding land use/land cover. Pádua et al. (2022) identified that water hyacinth contains chlorophyll, which has a high degree of absorption of light in the red, shortwave infrared, and near-infrared regions.
Figure 3. Feature importance of Sentinel-2A bands, indices, Sentinel-1 SAR bands, and topography variables using the random forest algorithm during the dry seasons of (A) 2022, (B) 2020, (C) 2018, and (D) 2016 in Lake Tana, Ethiopia. Feature importance of Sentinel-2A bands, indices, Sentinel-1 SAR bands, and topography variables using the random forest algorithm during the wet seasons of (E) 2022, (F) 2020, (G) 2018, and (H) 2016 in Lake Tana, Ethiopia.
B5, B11, B12, VH, NDAVI, and normalized difference water index (NDWI) were among the most important covariates for classifying the wet season (Figure 3). Additionally, slope, SAVI, and VV variables also contributed significantly. In the classification of the RF model, B11 and B5 (2022), VH, SAVI, and NDWI (2020), B5 and B12 (2018), and VH and slope (2016) were highly important variables (Figures 3E–H, respectively). The results suggest that water hyacinth can be distinguished from other vegetation types or land use/land cover by analyzing its spectral reflectance patterns in these bands and indices. The most important variable, NDAVI, combines the reflectance values in the red and NIR bands to identify the presence and distribution of water hyacinth. Datta et al. (2021) discovered that the NIR region’s absolute reflectance allows for the clear differentiation of submerged macrophytes. Bayable et al. (2023) found that the classification, which utilized an RF classifier, identified the spectral indices and bands of Sentinel-2 as the most crucial variables. Specifically, band 12 played a significant role in classifying autumn and spring images. During autumn, winter, and spring, the weed displayed the highest reflectance values in the bands of red edge 2 (B6), red edge 3 (B7), near-infrared (B8), and red edge 4 (B8A). However, water hyacinth displayed a comparatively low spectral reflectance during the wet season. The primary reason for this phenomenon is the increased biomass and leaf area index (LAI) of the plant during the wet season, which leads to greater absorption of light rather than reflection. As water hyacinth grows rapidly in response to the abundant water and nutrients available during this period, its leaf structure becomes denser, effectively trapping more light for photosynthesis and reducing the amount of light that is reflected back into the environment (Gichuki et al., 2012; Mucheye et al., 2022). Moreover, the wet season often coincides with higher levels of chlorophyll production within the plant, which enhances its ability to absorb light, particularly in the blue and red wavelengths. This increase in chlorophyll concentration contributes to lower reflectance values as chlorophyll is known for its strong absorption characteristics in these regions of the spectrum (Dersseh et al., 2020). Additionally, VH polarization proves particularly useful in distinguishing water hyacinth from other land use/land cover types due to its specific backscatter response at a central frequency of 5.404 GHz. The excellent discrimination ability of VH is linked to water hyacinth’s sensitivity to the scattering characteristics and structural information on this aquatic macrophyte (Simpson et al., 2020). Simpson et al. (2022) indicated that the Sentinel-1 SAR image of VV and VH bands can effectively distinguish between clean water and water hyacinth-infested water. The value range for water hyacinth-infested areas is between −16 dB and −6 dB, with a peak value of −12 dB.
The overall accuracy for the wet season ranges from 0.91 to 0.94 (Table 4). These values indicate a high level of accuracy for the classification model. The kappa coefficients range from 0.88 to 0.92, with values closer to 1 suggesting a higher level of accuracy. Therefore, the kappa coefficients given in the table suggest a strong agreement between the observed land use/land cover class and the expected class.
Table 4. Accuracy assessment of Sentinel-2A, Sentinel-1 SAR, and SRTM image classification of water hyacinth invasion using the random forest algorithm during the dry and wet seasons in Lake Tana, Ethiopia.
Both the overall accuracy and kappa coefficient were high for the dry season (Table 4). The highest values were recorded in 2020, with an overall accuracy of 0.95 and a kappa coefficient of 0.93 (Table 4). This means that 95% of the pixels were correctly classified. The lowest values were recorded in 2016, with an overall accuracy of 0.93 and a kappa coefficient of 0.9. Overall, the accuracy assessment results indicated that the classification method is reliable and accurate in identifying water hyacinth and water bodies in Lake Tana.
In 2016, water hyacinth covered 686.5 ha, which accounted for 2.2% of the entire lake area (Table 5). By 2018, the coverage had significantly increased to 1,851 ha, representing 6% of the lake area. In 2020, the areal coverage slightly decreased to 1,396.7 ha, accounting for 4.6% of the total lake area. Finally, in 2022, the coverage increased once again to 1,436.5 ha, representing 4.8% of the lake area (Table 5). The coverage of water hyacinth in Lake Tana has exhibited notable fluctuations from 2016 to 2022, with significant decreases observed in 2020 and 2022 compared to 2018. From 2016 to 2018, water hyacinth coverage in Lake Tana increased, with estimates indicating an increase from approximately 5 km2 in 2015 to 25 km2 by 2019 (Dersseh et al., 2020). This period of expansion can be attributed to favorable conditions for growth, such as nutrient influx from agricultural runoff and the lake’s shallow, oligotrophic–mesotrophic nature, which supports the proliferation of aquatic weeds (Dejen et al., 2017; Moges et al., 2017). The increase in coverage during this time was also linked to the lack of effective management strategies to control the invasive species (Damtie et al., 2022).
Table 5. Spatial coverage of water hyacinth (2016–2022) derived from Sentinel-2A, Sentinel-1 SAR, and SRTM imagery classification using the random forest algorithm during the wet seasons in Lake Tana, Ethiopia.
However, in 2020, a marked decrease in water hyacinth coverage was reported, with estimates suggesting a reduction to approximately 2,279.4 ha (Dersseh et al., 2020). This decrease can be attributed to several factors, including the implementation of control measures such as manual and mechanical removal. Additionally, environmental conditions, such as changes in water levels and seasonal variations, may have influenced the spatial distribution of water hyacinth, leading to a temporary reduction in coverage (Worqlul et al., 2020). By 2022, the coverage of water hyacinth continued to show a downward trend, with studies indicating that the area affected by the plant was significantly less than in previous years (Mucheye et al., 2022). This sustained decrease can be linked to ongoing management efforts and, possibly, the natural ecological dynamics of the lake, where periods of decrease in invasive species populations are common due to factors such as competition with native species, changes in water quality, and climatic influences.
The coverage increased from 2.1% (650 ha) in 2016 to 4.3% (1,305.7 ha) in 2020, which indicates a significant change over a short period of time (Table 6). However, there was a slight decrease in the infested area from 2020 to 2022, with a value of 4%. A total of 89.2 ha of land was cleared of water hyacinth between 2020 and 2022 (Table 6). In both wet and dry seasons, the RF model incorrectly classified the encircled portion of the lake (5a, 5b, 6a, and 6b) as infested with water hyacinth. It means that water hyacinth and the land surrounding it have similar spectral reflectance. The wrong classification is associated with environmental factors such as seasonal changes that influence the spectral characteristics of water hyacinth and surrounding vegetation. For example, variations in water quality, turbidity, and nutrient levels can alter the reflectance properties of aquatic plants, complicating the classification process. The performance of random forest classifiers can vary with changes in environmental conditions, which may lead to inconsistent classification results across different times of the year (Chabot et al., 2018). The dense mat of water hyacinth is predominantly observed in the northern and northeastern regions of the lake (Figures 4, 5). This area exhibits a higher concentration of the weed, necessitating focused attention and management strategies to address the significant infestation in these specific zones.
Table 6. Spatial coverage of water hyacinth (2016–2022) derived from Sentinel-2A, Sentinel-1 SAR, and SRTM imagery classification using the random forest algorithm during the dry seasons in Lake Tana, Ethiopia.
Figure 4. Distribution map of water hyacinth in Lake Tana, Ethiopia, using Sentinel-2A, Sentinel-1 SAR, and SRTM imagery in the wet season of (A) 2016, (B) 2018, (C) 2020, and (D) 2022.
Figure 5. Distribution map of water hyacinth in Lake Tana, Ethiopia, using Sentinel-2A, Sentinel-1 SAR, and SRTM imagery in the dry season of (A) 2016, (B) 2018, (C) 2020, and (D) 2022.
The water hyacinth infestation increased from 2016 to 2018 but slightly decreased from 2020 to 2022 in both seasons (Tables 5, 6). Furthermore, compared to the dry season, the wet season had a much higher coverage. For instance, in 2018, it covered 1,259 ha (4.2%) of the lake in the dry season but 1,851 ha (6.0%) in the wet season. This indicates a difference of 592 ha (1.8%) between the two seasons. Bayable et al. (2023) confirmed that from 2021 to 2022, geographic infestation significantly reduced by 62.5% from winter to spring and increased by 81.7% from wet to autumn. The tables clearly show that water hyacinth coverage reached its peak in the 2018 wet season and then decreased considerably in 2020 and 2022. These findings align with those obtained by Dersseh et al. (2020), who used Sentinel-2 images and found that the maximum water hyacinth coverage in 2015, 2016, 2017, 2018, and 2019 was 278.3, 613.6, 1,108.7, 2,036.5, and 2,504.5 ha, respectively.
Study results revealed that water hyacinth spatiotemporal dynamics are influenced by seasonal climate variability. Dersseh et al. (2020) reported that fluctuations in lake levels and seasonal climate variability, including rainfall patterns, affect the extent of water hyacinth coverage. An increase in water levels enables the weed to spread, expanding its range of habitats from the shallows to the flooded region. Conversely, low water levels reduce the available area for the weed, subjecting it to higher temperatures and solar radiation, potentially impacting its survival and reproduction.
Worqlul et al. (2020) and Abebe et al. (2023) reported that seasonal climate variations, particularly those related to the lake level and water temperature, have an impact on water hyacinth infestation. This study found that the infestation of Lake Tana by water hyacinth in the wet season is higher than that in the dry season. The rapid spread in the rainy season is associated with the rise in the lake level and nutrient concentration. Dersseh et al. (2019) reported that the most triggering factors and sources of water hyacinth prevalence in Lake Tana are sedimentation, extensive fertilizer application and runoff from agricultural fields, and pollutants (nutrients) from the surrounding industrial zones and residential areas of cities, mainly Bahir Dar and Gondar. Among these factors, the application of fertilizer, particularly those with high levels of nitrogen and phosphorus, into farmlands surrounding Lake Tana and runoff from agricultural fields are most prevalent. These nutrients act as fertilizers for water hyacinth, promoting their growth and reproduction. In addition, during the dry season, as the water hyacinth coverage decreases, farmers plow and produce crops such as rice and maize on the residue area with the application of fertilizer. As a result of this practice, the fertilizer is heavily drained into the lake during the wet season, leading to the high prevalence of water hyacinth.
Conversely, when the lake level decreases and the water temperature increases, water hyacinth coverage decreases. In the dry season, water levels in Lake Tana typically decrease due to reduced rainfall and increased evaporation. Lower water levels expose the roots and base of water hyacinths, making them more vulnerable to desiccation and limiting their ability to access nutrients and water (Cai et al., 2023). The results show that the decrease in water hyacinth coverage is linked to human interventions, such as mechanical removal using harvesting machinery and physical removal of the weed by humans. The local communities, along with the government, have been attempting to manage the rapid infestation by using mechanical removal methods, which involve the use of machinery and equipment to physically remove the weed from water bodies. The harvesting machine has been operating in places where dense mats of water hyacinth are commonly found, such as the Fogera area. Manual removal, on the other hand, involves the manual pulling of the plants out from the water to reduce their spatial coverage. Physical control or manual removal is the most important and highly practiced water hyacinth management practice in Lake Tana. Since 2012, a significant number of individuals have been actively participating in the campaign to remove weeds. This finding is consistent with that of the study conducted by Dersseh et al. (2020). In their study, they utilized satellite images to estimate the coverage in Lake Tana from 2011 to 2019. They discovered that the areal coverage increased from 20 km2 in 2011 to 40 km2 in 2019, reaching a peak of 50 km2 in 2018. They also observed that water hyacinth was primarily distributed along the northeastern shore of the lake. Worqlul et al. (2020) utilized high-resolution PlanetScope satellite images to analyze the dynamics of spatial coverage from August 2017 to July 2018. They found that the geographic coverage increased from 4.3 km2 in August 2017 to 23.4 km2 in April 2018 and then decreased to 14.5 km2 in July 2018. Abebe et al. (2023) discovered that the annual water hyacinth area significantly expanded between 2011 and 2019. For example, from 2011 to 2019, the lake’s surface area increased by approximately 1,603 ha due to the quick spread of water hyacinth. Bayable et al. (2023) reported that the seasonal coverage of water hyacinth, derived from Sentinel-2 images, was as follows: 2,240 ha in autumn (2021), 1,120 ha in winter (2022), 420 ha in spring (2022), and 220 ha in summer (2022).
Regarding the comparison of optical and non-optical satellite imagery, Mucheye et al. (2022) used Sentinel-2A/B imagery to monitor the seasonal variation in water hyacinth in Lake Tana. Their study reported an overall accuracy of 90% and a kappa coefficient of 0.85, indicating a strong agreement between the classified data and ground-truth observations. Damtie et al. (2021) used a maximum likelihood classifier on Sentinel-2 imagery to assess the spatial coverage of water hyacinth. They reported an overall accuracy of 92% with a kappa coefficient of 0.88, demonstrating the effectiveness of the classification method in detecting water hyacinth infestations. Janssens et al. (2022), who introduced a more advanced classification approach that utilized all spectral characteristics of Sentinel-2 bands, rather than relying solely on NDVI masks, showed an overall accuracy of 93% and a kappa coefficient of 0.90, reflecting the enhanced capability of their method to detect water hyacinth infestation across varying conditions. This approach underscores the significance of utilizing the full spectral range of satellite data for improved classification outcomes. Ade et al. (2022) contributed to the body of research by using random forest models on Sentinel-2 data, achieving an average overall accuracy of 90% for water hyacinth classification. The class accuracies ranged from 79% to 91%, indicating a robust performance in distinguishing water hyacinth from other aquatic vegetation. Overall, the studies indicate that Sentinel-2 imagery is highly effective in classifying water hyacinth in Lake Tana, with reported overall accuracies ranging from 90% to 93% and kappa coefficients between 0.85 and 0.90. However, studies have demonstrated that Sentinel-1 SAR can achieve high detection rates for water hyacinth, with true positive detection rates around 95% (Simpson et al., 2022). More importantly, the integration of Sentinel-1 and Sentinel-2 data significantly enhances the classification accuracy beyond what either sensor can achieve alone. The synergistic use of both datasets allows for the exploitation of the strengths of each modality, leading to improved feature extraction and classification outcomes. For example, the combination of SAR and optical data yields overall accuracies of up to 95.10% in agricultural contexts, highlighting the effectiveness of this approach (Yang et al., 2022; Chakhar et al., 2021). The fusion of these datasets leverages the temporal resolution of SAR with the spectral richness of optical data, thereby improving the classification of complex land cover types, including water hyacinth (Ibrahim et al., 2022).
The study used 16 variables obtained from Sentinel-2A, Sentinel-1 SAR, and ASTER DEM. A supervised random forest classification model was applied. Seven spectral indices, five spectral bands, two Sentinel-1 SAR bands, and two topographic variables were used in combination to model the spatial extent and coverage of water hyacinth. The results from the land use/land cover classification showed that the overall accuracy for 2016–2022 ranged from 0.91 to 0.94 in the dry season and 0.93 to 0.95 in the wet season. B3, B5, B11, B12, VH, elevation, NDAVI, and NDWI were the most relevant features in the land use/land cover classification of the study area. The findings demonstrated that the spatial coverage increased from 2016 to 2018 and decreased from 2020 to 2022. The highest infestation was recorded during 2018, with 6% (1,851 ha) and 4.2% (1,259 ha), and the lowest infestation was recorded in 2016, with 2.2% (686.5 ha) and 2.1% (650.4 ha) of the lake in the wet and dry seasons, respectively. The decrease in spatial coverage was associated with the manual and mechanical harvesting of the weed from the infested areas. Additionally, the highest biomass and distribution of weeds were detected around the northern, eastern, and northeastern lake shores.
The raw data supporting the conclusion of this article will be made available by the authors, without undue reservation.
MB: conceptualization, formal analysis, investigation, methodology, writing–original draft, and writing–review and editing. ZC: software and writing–review and editing. NA: conceptualization, software, and writing–review and editing. YN: methodology, software, and writing–review and editing. AA: methodology and writing–review and editing.
The author(s) declare that no financial support was received for the research, authorship, and/or publication of this article.
The authors declare that the research was conducted in the absence of any commercial or financial relationships that could be construed as a potential conflict of interest.
All claims expressed in this article are solely those of the authors and do not necessarily represent those of their affiliated organizations, or those of the publisher, the editors, and the reviewers. Any product that may be evaluated in this article, or claim that may be made by its manufacturer, is not guaranteed or endorsed by the publisher.
Abebe, T., Awoke, B. G., and Nega, W. (2023). Spatiotemporal patterns of water hyacinth dynamics as a response to seasonal climate variability in Lake Tana, Ethiopia. Appl. Water Sci. 13 (9), 170. doi:10.1007/s13201-023-01975-6
Abebe, W. B., and Minale, A. S. (2017). “Land use and watershed management practices in Lake Tana Basin,” in Social and ecological system dynamics: characteristics, trends, and integration in the Lake Tana Basin, Ethiopia, 479–521. doi:10.1007/978-3-319-45755-0_28
Abera, M. W. (2017). Impact of water hyacinth, Eichhornia crassipes (Martius) (Pontederiaceae) in Lake tana Ethiopia: a review. J. Aquac. Res. Dev. 9, 520.
Adam, E., Mutanga, O., Odindi, J., and Abdel-Rahman, E. M. (2014). Land-use/cover classification in a heterogeneous coastal landscape using RapidEye imagery: evaluating the performance of random forest and support vector machines classifiers. Int. J. Remote Sens. 35 (10), 3440–3458. doi:10.1080/01431161.2014.903435
Ade, C., Khanna, S., Lay, M., Ustin, S. L., and Hestir, E. L. (2022). Genus-level mapping of invasive floating aquatic vegetation using Sentinel-2 satellite remote sensing. Remote Sens. 14 (13), 3013. doi:10.3390/rs14133013
Arp, R. S., Fraser, G. C. G., and Hill, M. P. (2017). Quantifying the economic water savings benefit of water hyacinth (Eichhornia crassipes) control in the Vaalharts Irrigation Scheme. Water sa. 43 (1), 58–66. doi:10.4314/wsa.v43i1.09
Asmare, E. (2017). Current trend of water hyacinth expansion and its consequence on the fisheries around north eastern part of Lake Tana. Ethiop. J. Biodivers. Endanger. Species 5, 189.
Asmare, T., Demissie, B., Nigusse, A. G., and GebreKidan, A. (2020). Detecting spatiotemporal expansion of water hyacinth (Eichhornia crassipes) in Lake Tana, Northern Ethiopia. J. Indian Soc. Remote Sens. 48, 751–764. doi:10.1007/s12524-020-01107-6
Ayanda, O. I., Ajayi, T., and Asuwaju, F. P. (2020). Eichhornia crassipes (Mart.) Solms: uses, challenges, threats, and prospects. Sci. World J. 2020, 1–12. doi:10.1155/2020/3452172
Ayyad, M. A. (2003). Case studies in the conservation of biodiversity: degradation and threats. J. Arid Environ. 54 (1), 165–182. doi:10.1006/jare.2001.0881
Barrett, S. C. H. (1980). Sexual reproduction in Eichhornia crassipes (water hyacinth). I. Fertility of clones from diverse regions. J. Appl. Ecol. 17, 101–112. doi:10.2307/2402966
Bayable, G., Cai, J., Mekonnen, M., Legesse, S. A., Ishikawa, K., Imamura, H., et al. (2023). Detection of water hyacinth (Eichhornia crassipes) in Lake tana, Ethiopia, using machine learning algorithms. Water 15 (5), 880. doi:10.3390/w15050880
Begam, M., Pal, S., Mitra, N., Chatterjee, A., Mukhopadhyay, A., and Mukhopadhyay, S. K. (2021). GIS based approach to determine the changes of water hyacinth (Eichhornia crassipes) cover and relation with lesser whistling teal (Dendrocygna javanica) assemblage at santragachi wetland, West Bengal. Res. Ecol. 3 (1), 52–58. doi:10.30564/re.v3i1.2905
Begum, S. R., Himaya, S., and Afreen, S. (2021). Potential of water hyacinth (Eichhornia crassipes) as compost and its effect on soil and plant properties: a review. Agric. Rev. Of. doi:10.18805/ag.r-184
Bharatkar, P. S., and Patel, R. (2013). Approach to accuracy assessment tor RS image classification techniques. Int. J. Sci. and Eng. Res. 4 (12), 79–86.
Bhattacharya, A., and Kumar, P. (2010). Water hyacinth as a potential biofuel crop. Electron. J. Environ. Agric. Food Chem. 9 (1), 112–122.
Birara, H., Pandey, R. P., and Mishra, S. K. (2018). Trend and variability analysis of rainfall and temperature in the Tana basin region, Ethiopia. J. Water Clim. Change 9 (3), 555–569. doi:10.2166/wcc.2018.080
Cai, J., Jiao, C., Mekonnen, M., Legesse, S. A., Ishikawa, K., Wondie, A., et al. (2023). Water hyacinth infestation in Lake Tana, Ethiopia: a review of population dynamics. Limnology 24 (1), 51–60. doi:10.1007/s10201-022-00706-1
Chabot, D., Dillon, C., Shemrock, A., Weissflog, N., and Sager, E. P. S. (2018). An object-based image analysis workflow for monitoring shallow-water aquatic vegetation in multispectral drone imagery. ISPRS Int. J. Geo-Information 7 (8), 294. doi:10.3390/ijgi7080294
Chakhar, A., Hernández-López, D., Ballesteros, R., and Moreno, M. (2021). Improving the accuracy of multiple algorithms for crop classification by integrating sentinel-1 observations with Sentinel-2 data. Remote Sens. 13 (2), 243. doi:10.3390/rs13020243
Chen, J., Chen, S., Fu, R., Wang, C., Li, D., Jiang, H., et al. (2021). Simulation of water hyacinth growth area based on multi-source geographic information data: an integrated method of WOE and AHP. Ecol. Indic. 125, 107574. doi:10.1016/j.ecolind.2021.107574
Churko, E. E., Nhamo, L., and Chitakira, M. (2023). Phytoremediation capacity of water hyacinth (Eichhornia crassipes) as a nature-based solution for contaminants and physicochemical characterization of lake water. Water 15 (14), 2540. doi:10.3390/w15142540
Coetzee, J. A., Hill, M. P., Julien, M. H., Center, T. D., and Cordo, H. A. (2009). Eichhornia crassipes (mart.) solms-laub. (pontederiaceae). biological control of tropical weeds using arthropods. (New York, NY: Cambridge University Press), 183–210.
Cordeiro, P. F., Goulart, F. F., Macedo, D. R., Campos, M. D. C. S., and Castro, S. R. (2020). Modeling of the potential distribution of Eichhornia crassipes on a global scale: risks and threats to water ecosystems. Revista. Ambiente. Água 15 (2), e2421. doi:10.4136/ambi-agua.2421
Damtie, Y. A., Berlie, A. B., and Gessese, G. M. (2022). Impact of water hyacinth on rural livelihoods: the case of Lake Tana, Amhara region, Ethiopia. Heliyon 8 (3), e09132. doi:10.1016/j.heliyon.2022.e09132
Damtie, Y. A., Mengistu, D., and Meshesha, D. T. (2021). Spatial coverage of water hyacinth (eichhornia crassipes (mart.) solms) on lake tana and associated water loss. Heliyon 7 (10), e08196. doi:10.1016/j.heliyon.2021.e08196
Damtie, Y. A., and Mengistu, D. A. (2022). Water hyacinth (Eichhornia crassipes (Mart.) Solms) impacts on land-use land-cover change across northeastern Lake Tana. J. Indian Soc. Remote Sens. 50 (6), 975–986. doi:10.1007/s12524-022-01504-z
Datta, A., Maharaj, S., Prabhu, G. N., Bhowmik, D., Marino, A., Akbari, V., et al. (2021). Monitoring the spread of water hyacinth (Pontederia crassipes): challenges and future developments. Front. Ecol. Evol. 9, 631338. doi:10.3389/fevo.2021.631338
Dechassa, N., and Abate, B. (2020). Current status of water hyacinth (Eichhornia crassipes) in Ethiopia: achievements, challenges and prospects: a review. J. Environ. Earth Sci. 10 (12), 1–13.
Degaga, A. H. (2018). Water hyacinth (Eichhornia crassipes) biology and its impacts on ecosystem, biodiversity, economy and human well-being. J. Life Sci. Biomed. 8 (6), 94–100.
Dejen, E., Anteneh, W., and Vijverberg, J. (2017). The decline of the lake tana (Ethiopia) fisheries: causes and possible solutions. Land Degrad. and Dev. 28 (6), 1842–1851. doi:10.1002/ldr.2730
Dersseh, M. G., Kibret, A. A., Tilahun, S. A., Worqlul, A. W., Moges, M. A., Dagnew, D. C., et al. (2019). Potential of water hyacinth infestation on Lake Tana, Ethiopia: a prediction using a GIS-based multi-criteria technique. Water 11 (9), 1921. doi:10.3390/w11091921
Dersseh, M. G., Tilahun, S. A., Worqlul, A. W., Moges, M. A., Abebe, W. B., Mhiret, D. A., et al. (2020). Spatial and temporal dynamics of water hyacinth and its linkage with lake-level fluctuation: lake Tana, a sub-humid region of the Ethiopian highlands. Water 12 (5), 1435. doi:10.3390/w12051435
Duc, B. P., and Tong, S. (2021). Monitoring spatial-temporal dynamics of small lakes based on SAR Sentinel-1 observations: a case study over Nui Coc lake (Vietnam). Vietnam J. Earth Sci. doi:10.15625/2615-9783/16315
Elkhrachy, I., Pham, Q. B., Costache, R., Mohajane, M., Rahman, K. U., Shahabi, H., et al. (2021). Sentinel-1 remote sensing data and hydrologic engineering centres river analysis system two-dimensional integration for flash flood detection and modelling in new Cairo city, Egypt. J. Flood Risk Manag. 14 (2). doi:10.1111/jfr3.12692
Endgaw, F. (2021). Socio-ecological impacts of water hyacinth, Eichhornia crassipes (mart.) in lake tana, gulf of Gorgora, Ethiopia. J. Appl. Sci. Environ. Manag. 24 (12), 2017–2025. doi:10.4314/jasem.v24i12.2
Enyew, B. G., Assefa, W. W., and Gezie, A. (2020). Socioeconomic effects of water hyacinth (echhornia crassipes) in Lake tana, north western Ethiopia. PloS one 15 (9), e0237668. doi:10.1371/journal.pone.0237668
Gao, B. C. (1995). Normalized difference water index for remote sensing of vegetation liquid water from space. Imaging Spectrom. 2480, 225–236. doi:10.1117/12.210877
Gebremeskel, D. (2024). The effect of water hyacinth on water ecosystem: control mechanisms and utilization for different purposes.
Gedefaw, E., and Gondar, E. (2018). College of agriculture and rural transformation department of agricultural economics senior seminar on the socio economic impacts of water hyacinth invasion in Ethiopia.
Gerardo, R., and de Lima, I. P. (2022). Assessing the potential of Sentinel-2 data for tracking invasive water hyacinth in a river branch. J. Appl. Remote Sens. 16 (1), 014511. doi:10.1117/1.JRS.16.014511
Gezie, A., Assefa, W. W., Getnet, B., Anteneh, W., Dejen, E., and Mereta, S. T. (2018). Potential impacts of water hyacinth invasion and management on water quality and human health in Lake Tana watershed, Northwest Ethiopia. Biol. Invasions 20, 2517–2534. doi:10.1007/s10530-018-1717-0
Gichuki, J., Omondi, R., Boera, P., Okorut, T., Matano, A. S., Jembe, T., et al. (2012). Water hyacinth Eichhornia crassipes (mart.) solms-laubach dynamics and succession in the Nyanza gulf of Lake Victoria (East Africa): implications for water quality and biodiversity conservation. Sci. World J. 2012, 1–10. doi:10.1100/2012/106429
Gislason, P. O., Benediktsson, J. A., and Sveinsson, J. R. (2006). Random forests for land cover classification. Pattern Recognit. Lett. 27 (4), 294–300. doi:10.1016/j.patrec.2005.08.011
Hammam, A. A., Mohamed, E. S., El-Namas, A. E., Abd-Elmabod, S. K., and Eldin, R. M. B. (2022). Impacted application of water-hyacinth-derived biochar and organic manures on soil properties and barley growth. Sustainability 14 (20), 13096. doi:10.3390/su142013096
HemaLatha, M., and Varadarajan, S. (2019). “Feature enhancement of multispectral images using vegetation, water, and soil indices image fusion,” in Proceedings of the international conference on ISMAC in computational vision and bio-engineering 2018 (ISMAC-CVB) (Springer International Publishing), 329–337. doi:10.1007/978-3-030-00665-5_34
Hill, M. P., and Coetzee, J. A. (2008). Integrated control of water hyacinth in Africa 1. EPPO Bull. 38 (3), 452–457. doi:10.1111/j.1365-2338.2008.01263.x
Huete, A., Didan, K., Miura, T., Rodriguez, E. P., Gao, X., and Ferreira, L. G. (2002). Overview of the radiometric and biophysical performance of the MODIS vegetation indices. Remote Sens. Environ. 83 (1-2), 195–213. doi:10.1016/S0034-4257(02)00096-2
Huete, A. R. (1988). A soil-adjusted vegetation index (SAVI). Remote Sens. Environ. 25 (3), 295–309. doi:10.1016/0034-4257(88)90106-X
Ibrahim, G. R. F., Rasul, A., and Abdullah, H. Y. (2022). Integration of sentinel 1 and Sentinel 2 data for crop classification improvement: barley and wheat as an example. doi:10.20944/preprints202209.0169.v1
Ilo, O. P., Simatele, M. D., Nkomo, S. L., Mkhize, N. M., and Prabhu, G. N. (2020). The benefits of water hyacinth (Eichhornia crassipes) for southern Africa: a review. Sustainability 12 (21), 9222. doi:10.3390/su12219222
Janssens, N., Schreyers, L., Biermann, L., Ploeg, M. v. d., Bui, T. L., and Emmerik, T. v. (2022). Rivers running green: water hyacinth invasion monitored from space. Environ. Res. Lett. 17 (4), 044069. doi:10.1088/1748-9326/ac52ca
Jones, R. W. (2009). The impact on biodiversity, and integrated control, of water hyacinth, Eichhornia crassipes (martius) solms-laubach (pontederiaceae) on the Lake nsezi-nseleni river system doctoral dissertation. South Africa: Rhodes University.
Julien, M. (2008). Plant biology and other issues that relate to the management of water hyacinth: a global perspective with focus on Europe 1. EPPO Bull. 38 (3), 477–486. doi:10.1111/j.1365-2338.2008.01267.x
Karanam, H. K., and Neela, V. B. (2017). Study of normalized difference built-up (NDBI) index in automatically mapping urban areas from Landsat TN imagery. Int. J. Eng. Sci. Math. 8, 239–248.
Kulkarni, A. D., and Lowe, B. (2016). Random forest algorithm for land cover classification. Available at: http://hdl.handle.net/10950/341.
Kumlachew, Y. Z., Tilahun, S. A., Cherie, F. F., Akale, A. T., Kibret, E. A., Alemie, N. A., et al. (2023). Quantifying flow rate using stage-discharge rating curve and Scs runoff equation on upland watershed of Lake Tana Sub Basin, Ethiopia. Sustain. Water Resour. Manag. 9 (2), 47. doi:10.1007/s40899-022-00793-z
Laranjeira, C. M., and Nadais, G. (2008). Eichhornia crassipes control in the largest Portuguese natural freshwater lagoon 1. EPPO Bull. 38 (3), 487–495. doi:10.1111/j.1365-2338.2008.01268.x
Mequanent, D., Mingist, M., Getahun, A., and Anteneh, W. (2021). Impact of irrigation practices on Gilgel Abay, Ribb and Gumara fisheries, tana sub-basin, Ethiopia. Heliyon 7 (3), e06523. doi:10.1016/j.heliyon.2021.e06523
Mironga, J. M., Mathooko, J. M., and Onywere, S. M. (2012). Effect of water hyacinth infestation on the physicochemical characteristics of Lake Naivasha. Int. J. Humanit. Soc. Sci. 2 (7), 103–113.
Moges, M. A., Schmitter, P., Tilahun, S. A., Ayana, E. K., Ketema, A. A., Nigussie, T. E., et al. (2017). Water quality assessment by measuring and using Landsat 7 ETM+ images for the current and previous trend perspective: lake tana Ethiopia. J. Water Resour. Prot. 09 (12), 1564–1585. doi:10.4236/jwarp.2017.912099
Mucheye, T., Haro, S., Papaspyrou, S., and Caballero, I. (2022). Water quality and water hyacinth monitoring with the Sentinel-2a/b satellites in lake tana (Ethiopia). Remote Sens. 14 (19), 4921. doi:10.3390/rs14194921
Mukarugwiro, J. A., Newete, S. W., Adam, E., Nsanganwimana, F., Abutaleb, K. A., and Byrne, M. J. (2019). Mapping distribution of water hyacinth (Eichhornia crassipes) in Rwanda using multispectral remote sensing imagery. Afr. J. Aquatic Sci. 44 (4), 339–348. doi:10.2989/16085914.2019.1650712
Nguyen, H. T. T., Doan, T. M., and Radeloff, V. (2018). Applying random forest classification to map land use/land cover using Landsat 8 OLI. Int. Archives Photogrammetry, Remote Sens. Spatial Inf. Sci. 42, 363–367. doi:10.5194/isprs-archives-XLII-3-W4-363-2018
Pádua, L., Antão-Geraldes, A. M., Sousa, J. J., Rodrigues, M. Â., Oliveira, V., Santos, D., et al. (2022). Water hyacinth (Eichhornia crassipes) detection using coarse and high-resolution multispectral data. Drones 6 (2), 47. doi:10.3390/drones6020047
Patel, S. (2012). Threats, management and envisaged utilizations of aquatic weed Eichhornia crassipes: an overview. Rev. Environ. Sci. Bio/Technology 11, 249–259. doi:10.1007/s11157-012-9289-4
Rezene, F. (2005). Water hyacinth (Eichhornia crassipes): a review of its weed status in Ethiopia. Arem 6, 105–111.
Robles, W., Madsen, J. D., and Wersal, R. M. (2015). Estimating the biomass of waterhyacinth (Eichhornia crassipes) using the normalized difference vegetation index derived from simulated landsat 5 TM. Invasive Plant Sci. Manag. 8 (2), 203–211. doi:10.1614/IPSM-D-14-00033.1
Rodriguez-Galiano, V. F., Ghimire, B., Rogan, J., Chica-Olmo, M., and Rigol-Sanchez, J. P. (2012). An assessment of the effectiveness of a random forest classifier for land-cover classification. ISPRS J. photogrammetry remote Sens. 67, 93–104. doi:10.1016/j.isprsjprs.2011.11.002
Rouse, J. W., Haas, R. H., Schell, J. A., and Deering, D. W. (1974). Monitoring vegetation systems in the great plains with ERTS. NASA Spec. Publ. 351 (1), 309.
Rowan, G. S., and Kalacska, M. (2021). A review of remote sensing of submerged aquatic vegetation for non-specialists. Remote Sens. 13 (4), 623. doi:10.3390/rs13040623
Simpson, M., Marino, A., Prabhu, G. N., Bhowmik, D., Rupavatharam, S., Datta, A., et al. (2020). “Monitoring water hyacinth in Kuttanad, India using Sentinel-1 SAR data,” in 2020 IEEE India geoscience and remote sensing symposium (InGARSS) (IEEE), 13–16.
Simpson, M. D., Akbari, V., Marino, A., Prabhu, G. N., Bhowmik, D., Rupavatharam, S., et al. (2022). Detecting water hyacinth infestation in kuttanad, India, using dual-pol Sentinel-1 SAR imagery. Remote Sens. 14 (12), 2845. doi:10.3390/rs14122845
Singh, G., Reynolds, C., Byrne, M., and Rosman, B. (2020). A remote sensing method to monitor water, aquatic vegetation, and invasive water hyacinth at national extents. Remote Sens. 12 (24), 4021. doi:10.3390/rs12244021
Stiers, I., Crohain, N., Josens, G., and Triest, L. (2011). Impact of three aquatic invasive species on native plants and macroinvertebrates in temperate ponds. Biol. Invasions 13, 2715–2726. doi:10.1007/s10530-011-9942-9
Svoboda, J., Štych, P., Laštovička, J., Paluba, D., and Kobliuk, N. (2022). Random forest classification of land use, land-use change and forestry (LULUCF) using sentinel-2 data-A case study of Czechia. Remote Sens. 14 (5), 1189. doi:10.3390/rs14051189
Tasew, A. Y., and Wendimagegnehu, W. W. (2022). Occurrence, diversity and potential of fungal pathogens associated with water hyacinth [Eichhornia crassipes (mart.) solms] for sustainable biological management in Ethiopia: a review. Adv. Environ. Stud. 6 (1), 497–503. doi:10.36959/742/249
Tewabe, D. (2015). Preliminary survey of water hyacinth in Lake. Glob. J. Allergy 1 (1), 013–018. doi:10.17352/2455-8141.000003
Thamaga, K. H., and Dube, T. (2018). Remote sensing of invasive water hyacinth (Eichhornia crassipes): a review on applications and challenges. Remote Sens. Appl. Soc. Environ. 10, 36–46. doi:10.1016/j.rsase.2018.02.005
Thamaga, K. H., and Dube, T. (2019). Understanding seasonal dynamics of invasive water hyacinth (Eichhornia crassipes) in the Greater Letaba river system using Sentinel-2 satellite data. GIScience and Remote Sens. 56 (8), 1355–1377. doi:10.1080/15481603.2019.1646988
Tibebe, D., Kassa, Y., Melaku, A., and Lakew, S. (2019). Investigation of spatio-temporal variations of selected water quality parameters and trophic status of Lake Tana for sustainable management, Ethiopia. Microchem. J. 148, 374–384. doi:10.1016/j.microc.2019.04.085
Valta-Hulkkonen, K., Kanninen, A., and Pellikka, P. (2004). Remote sensing and GIS for detecting changes in the aquatic vegetation of a rehabilitated lake. Int. J. Remote Sens. 25 (24), 5745–5758. doi:10.1080/01431160412331291170
Villa, P., Bresciani, M., Braga, F., and Bolpagni, R. (2013). “Mapping aquatic vegetation through remote sensing data: a comparison of vegetation indices performances,” in Proceedings of the 6th EARSeL workshop on remote sensing of the coastal zone, Matera, Italy 10–15.
Wang, Z., and Yan, S. H. (2017). Impact of water hyacinth on aquatic environment in phytoremediation of eutrophic lakes. Water Hyacinth Environ. Challenges. Manag. Util., 204–249.
Williams, A. E., and Hecky, R. E. (2005). “Invasive aquatic weeds and eutrophication: the case of water hyacinth in Lake victoria: 1 and robert E. Hecky2,” in In Restoration and management of tropical eutrophic lakes (USA: CRC Press), 211–250.
Wondie, A. (2012). Biodiversity and ecosystem services of lake Tana Wetlands, Ethiopia. Biodivers. Conservation Ecosyst. Serv. Clim. Change Mitig. Sustain. Dev. 20, 91.
Worqlul, A. W., Ayana, E. K., Dile, Y. T., Moges, M. A., Dersseh, M. G., Tegegne, G., et al. (2020). Spatiotemporal dynamics and environmental controlling factors of the Lake Tana water hyacinth in Ethiopia. Remote Sens. 12 (17), 2706. doi:10.3390/rs12172706
Yang, Z., Zhang, H., Lyu, X., and Du, W. (2022). Improving typical urban land-use classification with active-passive remote sensing and multi-attention modules hybrid network: a case study of Qibin district, Henan, China. Sustainability 14 (22), 14723. doi:10.3390/su142214723
Zhang, Y. Y., Zhang, D. Y., and Barrett, S. C. (2010). Genetic uniformity characterizes the invasive spread of water hyacinth (Eichhornia crassipes), a clonal aquatic plant. Mol. Ecol. 19 (9), 1774–1786. doi:10.1111/j.1365-294x.2010.04609.x
Keywords: aquatic invasive plant, Lake Tana, machine learning model, remote sensing indices, Sentinel image, water hyacinth
Citation: Belayhun M, Chere Z, Abay NG, Nicola Y and Asmamaw A (2024) Spatiotemporal pattern of water hyacinth (Pontederia crassipes) distribution in Lake Tana, Ethiopia, using a random forest machine learning model. Front. Environ. Sci. 12:1476014. doi: 10.3389/fenvs.2024.1476014
Received: 05 August 2024; Accepted: 24 October 2024;
Published: 14 November 2024.
Edited by:
YoonKyung Cha, University of Seoul, Republic of KoreaReviewed by:
Doru Stelian Banaduc, Lucian Blaga University of Sibiu, RomaniaCopyright © 2024 Belayhun, Chere, Abay, Nicola and Asmamaw. This is an open-access article distributed under the terms of the Creative Commons Attribution License (CC BY). The use, distribution or reproduction in other forums is permitted, provided the original author(s) and the copyright owner(s) are credited and that the original publication in this journal is cited, in accordance with accepted academic practice. No use, distribution or reproduction is permitted which does not comply with these terms.
*Correspondence: Matiwos Belayhun, bWF0aWJlbGF5aHVuQGdtYWlsLmNvbQ==
Disclaimer: All claims expressed in this article are solely those of the authors and do not necessarily represent those of their affiliated organizations, or those of the publisher, the editors and the reviewers. Any product that may be evaluated in this article or claim that may be made by its manufacturer is not guaranteed or endorsed by the publisher.
Research integrity at Frontiers
Learn more about the work of our research integrity team to safeguard the quality of each article we publish.