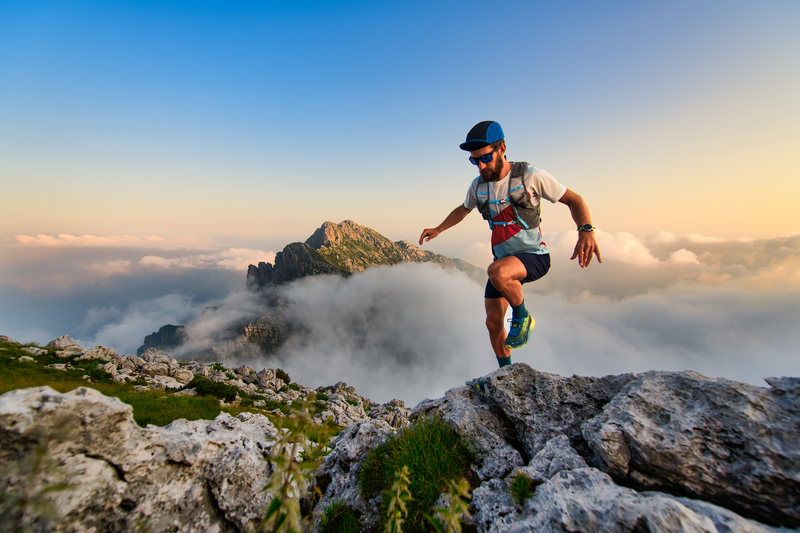
94% of researchers rate our articles as excellent or good
Learn more about the work of our research integrity team to safeguard the quality of each article we publish.
Find out more
ORIGINAL RESEARCH article
Front. Environ. Sci. , 09 December 2024
Sec. Interdisciplinary Climate Studies
Volume 12 - 2024 | https://doi.org/10.3389/fenvs.2024.1462700
Climate change has intensified urban heat risks through extreme heat and heat island effects. Using Fuzhou as a case study, we conducted assessments of heat risk and cool island quality to identify core heat risk sources (CHRSs) and core cold sources (CCSs). Based on the degree of resistance to surface heat transfer, we constructed a comprehensive resistance surface. This was followed by the construction of a composite cooling network using the minimal cumulative resistance and circuit theory models, along with the identification of key nodes to enhance the protection of cool island resources and ensure network stability. Our findings revealed that the central urban area had the highest heat risk, followed by the eastern coastal areas, showing a trend of further expansion towards the southeastern coast. Relatively high-quality cool island resources were distributed in the western mountainous area. We identified 21 CHRSs and 32 CCSs. The composite cooling network included 94 heat transport corridors and 96 cool island synergy corridors, with 148 cooling nodes and 78 barrier nodes. The average land surface temperature of transport and synergy corridors was 27.89°C and 25.34°C, respectively, significantly lower than the high-risk areas (31.14°C). Transport corridors enable heat transfer from CHRSs to CCSs, while synergy corridors can achieve further cooling by enhancing the synergy among cool islands.
Global climate change has resulted in an increase in the frequency of extreme heat events during summer (Sahoo et al., 2016), and often referred to as a “silent killer” owing to its imperceptible yet lethal effects, which lead to heat-related fatalities, extreme heat has become one of the most lethal natural disasters globally (Mora et al., 2017). As of 2023, the global average temperature has risen by 1.45°C ± 0.12°C relative to its pre-industrial level (the 1850–1900 baseline). This increase significantly exceeds the warming trends observed in previous years and is closer to the 1.5°C target outlined in the Paris Agreement. Projections also indicate that the average temperature in 2024 may exceed this level (Yao et al., 2022; Thanvisitthpon et al., 2023). Presently, approximately 30% of the global population is exposed to extreme heat, which results more than 38,000 deaths annually, primarily among children and older adults (Martin and Paneque, 2022). Additionally, it has been predicted that extreme heat will trigger a cascade of events globally, and if temperatures reach 4.0°C, half of the world’s regions will be at a greater risk of more severe natural disasters, such as droughts and wildfires, which can significantly impact the operation of various urban infrastructures and exacerbate heat-related risks in cities (Hu and Xiong, 2015). China is recognized as a sensitive and highly impacted region with respect to global climate change, and its overall climate risk index is steadily increasing (Chen Y. et al., 2020). According to The 2023 China Report of the Lancet Countdown on Health and Climate Change, in 2022, China experienced a record-breaking 21 days of heat waves per capita, leading to over 50,900 deaths (Zhang S. et al., 2023). Therefore, heat waves constitute a major threat to the urban ecological environment and human wellbeing, and to mitigate its effects, a precise and scientific evaluation to identify the spatiotemporal distribution patterns of urban heat risk and highlight tailored mitigation strategies is urgent. Such studies hold great significance for enhancing the quality of human habitation and fostering sustainable urban progress.
Heat risk assessment plays a crucial role in visualising the distribution of urban heat risk characteristics, and its research paradigm has experienced significant advancements in recent decades (Rowlinson et al., 2014). Heat risk depends on both hazardousness and vulnerability, with vulnerability including exposure to hazards and adaptive capacity; this hypothesis introduced a level of uncertainty and subjectivity to the concept of vulnerability, as it was determined not only by socio-ecological data reflecting urban coping capabilities but also hazard exposure estimates or projections (Sharma and Ravindranath, 2019; Estoque et al., 2020). In 2012, the Intergovernmental Panel on Climate Change (IPCC) formulated a climate risk-centred assessment framework in its “Special Report on Managing the Risks of Extreme Events and Disasters to Advance Climate Change Adaptation”. Within this updated framework, risk was articulated as a function comprising three primary components: hazard, exposure, and vulnerability (Murray and Ebi, 2012). Within this context, exposure was delineated as the presence of specific exposed elements or risk components, distinct from vulnerability, aligning with established frameworks commonly employed in disaster risk assessment. This recent framework by the IPCC has found practical application in recent research endeavours (Aerts et al., 2018; Wang et al., 2023) and has served as a fundamental guide in shaping our research methodology and design.
The development of cooling strategies based on urban heat risk assessment findings is critical for adaptive spatial planning, focusing on mitigating urban heat risk. Green infrastructure (GI) serves as a vital element of the urban ecosystem, demonstrating varying degrees of cooling effects based on its size, form, and spatial arrangement. Many GI components, including green spaces and water features, exhibit a “cool island effect” that counteracts the heat island phenomenon (Zhuang et al., 2017) and, hence, plays a significant role in addressing urban heat-related challenges during the summer months and reducing associated heat risks (Escobedo et al., 2015). Therefore, GI functions as urban cool islands as opposed to urban heat islands (Liu et al., 2022). In the pursuit of sustainable urban cooling, scholars have conducted quantitative research on the thermal environment benefits provided by GI, primarily through field observations and numerical simulations. Field measurement studies have predominantly discussed the significant factors influencing the GI cooling effect concerning area, shape, vegetation structure, and other relevant aspects (Fei et al., 2023; Zhao et al., 2023). The methodologies utilised primarily encompass mathematical and statistical techniques, including correlation analysis and regression modelling (Sun et al., 2020; Zhang et al., 2022). Regarding numerical simulation, investigations have predominantly utilised software tools such as CFD and ENVI-met to conduct scenario simulations, aiming to analyse the synergistic effects of vegetation, water bodies, and other key elements for optimal cooling in GI systems (Bouketta, 2023; Hao TP. et al., 2023).
While considerable progress has been made in clarifying the distribution of urban heat risk at micro- and meso-scales, studies in this regard at the urban macro level are limited. Further, the primary focus has been on statistical analyses at the regional or localised patch scale to identify the attributes of GI elements that are capable of mitigating land surface temperature (LST) (Shen et al., 2022; Xue et al., 2022). However, an important factor contributing to the escalation of urban heat risk, the alteration of urban thermal environment patterns owing to the combined impacts of human activities and the ecological environment, needs to be considered. Enhancing relevant mitigation strategies at a macro level is therefore important. Reportedly, the spatial layout and connectivity optimisation of urban cool islands can significantly enhance the thermal environment (Debbage and Shepherd, 2015). Moreover, the establishment of a comprehensive and well-organised natural cooling network can effectively mitigate the adverse effects of intensive urban development and human activities (Peng et al., 2022). However, the mere creation of a cool island network is insufficient to fully demonstrate the energy transfer mechanisms from high-temperature to low-temperature entities (Cheng and Wu, 2020). Thermal accumulation in urban patches with high temperatures can further exacerbate local overheating, thereby limiting the mitigation effects of cool islands on heat risk to some extent. Hence, the development of landscape networks necessitates a thorough analysis of the spatial structural attributes of cool islands and heat sources, along with their interactions. In urban settings, the heat absorbed by the land surface naturally transmits to distinct urban cold islands through ecological processes, following the second law of thermodynamics (Jamei et al., 2016). During this process, heat abides by the surface energy balance principle, transforming into alternate forms of energy to sustain system equilibrium. Research indicates that the construction of a landscape network can expedite this transfer and conversion process (Li and Chen, 2023a; Liu et al., 2024).
To address the aforementioned research gaps, this study focuses on examining the spatial structural characteristics and interactions between cold islands and heat sources. By integrating relevant thermodynamic theories, we aimed to establish a new network connection between cool islands and areas with significant heat risk and ultimately propose a construction model for an urban composite cooling network. Our study follows the method of “source identification–resistance surface construction–corridor extraction” as a whole. Initially, following the climate risk assessment framework outlined by the IPCC, a thorough evaluation of urban heat risks was conducted using a variety of data sources. Core heat risk sources (CHRSs) were considered as areas of highest heat risk that are prioritised for cooling efforts. Furthermore, an assessment of the quality of urban cold islands, represented by GI, was conducted from two perspectives: the intensity and sustainability of the cold island effect. Exceptional cold island patches were identified as core cold sources (CCSs). Subsequently, spatial principal component analysis (SPCA) was utilised to create a comprehensive resistance surface, enabling the quantification of heat transfer resistance within the urban thermal environment pattern. Finally, the minimal cumulative resistance (MCR) model was utilised to establish heat transport corridors (TCs) connecting CHRSs and adjacent CCSs. Concurrently, cool island synergy corridors (SCs) linking all CCSs were developed based on circuit theory (CT). Furthermore, we implemented proactive measures at pivotal nodes to bolster the safeguarding of cold island resources, thereby mitigating and pre-empting the escalation of heat-related risks. Therefore, in this study, we aimed to construct a composite cooling network construction model to reveal the energy flow process in the urban thermal environment and thus, enable the transfer of heat from risk sources, improve cooling efficiency via the synergistic effect of cool island networks, reduce urban thermal risk, and provide useful guidance for cities to effectively adapt to and mitigate heat risk.
Fuzhou is the capital city of Fujian province in China characterised by a subtropical monsoon climate. According to data released by the Fuzhou Municipal Bureau of Statistics in 2020, Fuzhou covers a total area of 11,968 km2 and has a permanent population of 8.29 million, with an urbanisation rate of 72.5%.
Fuzhou exemplifies a typical estuary basin, with its central urban area situated at the basin’s core. The progression of urban expansion and societal advancement has brought about significant alterations in the surface landscape. The industrial, commercial, and transportation activities of the populace have contributed to substantial heat emissions. These factors, combined with Fuzhou’s distinctive geographical position and topographical attributes, have posed challenges in effectively dissipating LST; the prolonged heat saturation has resulted in a pronounced heat island effect (Pan et al., 2020). Since 2007, Fuzhou has experienced an average peak LST of 35°C–40°C during midsummer, with temperature differentials of up to 4°C between urban structures and green areas (Chen YH. et al., 2020), leading to severe heatwave calamities. Records from the Fuzhou Meteorological Bureau indicate that in 2020, the city encountered 61–76°days with temperatures exceeding 35°C, surpassing historical highs in some regions during the same timeframe.
To maintain the representation and universality of our study, we chose the urban area of Fuzhou (excluding Pingtan Island) for our empirical research (Figure 1).
The sources of the basic data used in this study and their usage are shown in Table 1. The datasets were converted into raster data and resampled to a resolution of 30 m × 30 m in ArcGIS 10.8. They included: (1) Landsat8 OLI satellite remote sensing imagery data downloaded directly from the website of the United States Geological Survey. (2) Level 1 and level 2 type land-use classification data, which met the accuracy requirements for our study (accuracies >93% and 90% respectively) were obtained from the Data Center for Resources and Environmental Sciences of the Chinese Academy of Science (Li et al., 2024). (3) Digital elevation model (DEM) data were obtained from the Geospatial Data Cloud platform and subsequently used to obtain slope data. (4) Nighttime light data (2020) was obtained from Harvard Dataverse) (Chen JD. et al., 2020). (5) Road data, including data on railways, expressways, national highways, provincial highways, primary roads, and other roads were obtained from Open Street Map. (6) Population data, including unrestricted population density data for 2020 and the ratio of men to women aged 0–80 in individual counties was obtained from World Pop). This population data was further stratified to obtain data for children (individuals aged <5) and older adults (individuals aged >65). (7) Data on buildings and (8) Medical point were obtained from Amap (Gaode) Maps API, and (9) Socio-economic data were obtained from the 2020 Fuzhou Statistical Yearbook.
Our research framework comprises three primary components. By conducting a thorough heat risk assessment and cold island quality evaluation in the study area, we identified the CHRSs and CCSs, which were subjected to additional screening incorporating landscape connectivity metrics. Eight surface spatial structure indicators were chosen to assess the level of heat transfer resistance in the thermal environment pattern, forming a comprehensive resistance surface. Subsequently, a composite cooling network representing the energy flow dynamics of the thermal environment pattern was meticulously developed utilising the MCR and CT models. This process included pinpointing key nodes for precise management and control. The complete research framework is illustrated in Figure 2.
The conceptual framework for climate risk assessment proposed by the IPCC delineates climate change risk as a consequence of the interaction between climate-related disasters and both human and natural systems. This risk is defined by the inherent peril of the disasters, as well as the exposure and vulnerability of at-risk elements. Heat hazard pertains to the probability of heat-related disasters occurring in the geographical area where the affected element is situated and signifies the degree to which the populace’s health is impacted by heat-induced stress. Both natural fluctuations and human intervention in the global climate can influence the heat hazard of a specific region, ultimately materialising in the temperature characteristics of that locale (Wilhelmi and Hayden, 2010; Heaton et al., 2014). Heat exposure refers to the quantity of exposed elements within a designated space where heat stress could potentially arise. This encompasses metrics concerning human undertakings and the direct exposure of land surfaces (Yin et al., 2013). Heat vulnerability denotes the level to which exposed elements are susceptible to heat impacts and their resilience. This encompasses two facets: sensitivity, evidenced in the attributes of individuals and environments exposed to heat, and adaptability, indicative of a city’s capacity, both socioeconomically and ecologically, to react to disasters.
Considering Fuzhou’s construction, data quality, and availability, we developed a Fuzhou heat risk assessment system (Figure 3). Supplementary Figure S1 displays data for the different indicators.
Based on previously reported methodologies (Morabito et al., 2015; Zhang et al., 2019), we utilised the inverted LST technique on remote-sensing imagery data to analyse the heat threat within the Fuzhou Region. The use of this imagery data as opposed to temperature data collected at meteorological stations, enabled a more accurate delineation of the spatial variations in heat stress at the municipal level (Yang et al., 2022). The remote-sensing imagery utilised in this study pertained to the dates of July 15 and 22 July 2020, a period marked by heightened summer heat risks in Fuzhou. The radiative transfer equation algorithm (atmospheric correction method) involves using real-time atmospheric detection and profile data to assess the atmospheric influence on land surface thermal radiation. In the radiative transfer process from the ground to the atmosphere, the thermal infrared radiation energy,
where
After calculating the blackbody radiance, the true ground temperature can be estimated using the inverse function of Planck’s law, as shown in Equation 3:
where
Prior to the calculations, we obtained the surface emissivity,
where
where
Heat exposure assessment essentially entails determining the spatial distribution characteristics of exposed elements in environments where heat stress may occur. A substantial body of evidence currently supports the pivotal role of human activities in the global modifications of diverse surface environmental elements, including soil, climate, geology, and hydrology. The stress condition of an ecosystem and its susceptibility to stress within a specific region can be elucidated by assessing the magnitude and intensity of human activities within that locale (Shen et al., 2020). Therefore, through the evaluation of human activities’ intensity, the exposure level of the urban populace and surface ecological patterns to high-temperature settings can be ascertained. This methodology offers a more in-depth analysis than previous research (Liu et al., 2022; Wang et al., 2023), which solely relied on population density to gauge heat exposure.
Currently, the Human Footprint index introduced by Sanderson et al. (2002) is widely utilised to measure the extent of human activities. This index is assessed across five dimensions: population density, land cover alteration, transportation accessibility, power infrastructure, and ecological communities. These metrics are then amalgamated to derive the global Human Footprint assessment (Sanderson et al., 2002; Liu S. et al., 2018). Considering the urbanisation patterns of Fuzhou and data accessibility and following the computation approach in the Human Footprint model, we opted for evaluating population density, land cover alteration, road network density, and nighttime luminosity to gauge human activity intensity for the purpose of heat exposure characterisation.
Details of the calculation method is as shown Equation 7:
where E is the heat exposure index, PD is the population density, and NTL is the nighttime light intensity. SLULC is the human influence index on land cover, which was calculated based on the scores assigned by Sanderson et al. (2002) for each land cover type (built-up land: 10; agricultural land: 8; other land cover types: 0). Road is the score for road network accessibility calculated based on the weighted sum of the Euclidean distance from a given 500 × 500 m grid cell to various road types. The weights of the different road types were determined based on previous studies (Shen et al., 2020) (primary roads: 0.87; provincial highways: 0.8; national highways: 0.53; railways: 0.37; expressways: 0.2; other roads: 1). The four indicators were normalised separately, and equal-weighted overlay was performed in ArcGIS 10.8 to obtain the heat exposure index of the study area.
Following the IPCC climate risk assessment framework, vulnerability was defined as a function of sensitivity and adaptive capacity. Sensitivity refers to the extent to which human populations and urban landscapes are predisposed to heat. Traditionally, children and the elderly are deemed more vulnerable to heat compared to other demographic groups (Åström et al., 2011). The urban expansion has led to land use conflicts, exacerbating the fragmentation of surface landscape patterns, leading to elevated LST and habitat deterioration (Zhou et al., 2017). This scenario highlights the vulnerability of surface ecological patterns to high-temperature conditions. Adaptive capacity denotes the ability of a city’s socio-economic and ecological systems to efficiently respond to disasters.
By integrating Fuzhou’s urban development with pertinent indicator data relating to population demographics, landscape configuration, municipal economy, healthcare infrastructure, ecological surroundings, and more, we formulated a comprehensive heat vulnerability assessment indicator framework (Table 2). Notably, the calculation of patch density and cohesion was conducted utilising the moving window technique in Fragstats 4.2.1, leveraging land use data.
The wetness index, NDVI, and modified normalised difference water index, MNDWI, are calculated by Equations 8–10:
where ρBlue, ρGreen, ρRed, ρNIR, ρMIR1, and ρMIR2 are the blue band, green band, red band, near-infrared band, mid-infrared band 1, and mid-infrared band 2, respectively, of Landsat8 images.
Two methodologies, namely, the Analytic Hierarchy Process (AHP) and Entropy Weight Method (EWM) (Liang et al., 2019; Wu et al., 2022), were utilised to determine the holistic weight through the integration of objective and subjective approaches founded on the principle of minimum information entropy and the Lagrangian function. The corresponding Equations 11–16 are as follows:
where ek is the information entropy for the kth indicator. If
Urban heat risk is determined by the weighted aggregation of three indices: heat hazard, heat exposure, and heat vulnerability. There is currently no consensus on the weighting of these three indicators (Johnson et al., 2012). Following previous studies (Aerts et al., 2018), we utilised an equal-weighted overlay model to calculate heat risk, as shown in the Equation 17 below:
where HRI is the comprehensive heat risk index; H, E, and V are the heat hazard, heat exposure, and heat vulnerability, respectively. By performing spatial overlay operations in ArcGIS 10.8, the comprehensive HRI of the study area was obtained to visualise the spatial distribution of HRI within this area.
GI is an efficient method to mitigate high-temperature urban climates and regulate the local energy balance (Su et al., 2021). Consequently, it holds a pivotal position in enhancing urban climate resilience and mitigating the effects of urban heat risks. To guarantee the efficacy of cooling network development, we specifically chose three types of green infrastructure land use (namely, forest lands, grasslands, and water bodies) in the research area to evaluate the quality of cool islands for precise identification of CCSs.
A cold source quality assessment system was devised based on two key dimensions: the intensity and sustainability of the cool island effect (Table 3). The intensity of the cool island effect signifies the cool island’s capacity to regulate urban heat, influenced by both the landscape features of the “cool island” itself and the surrounding climate. A cool island with a higher cooling contribution index, a more intricate shape, and a greater concentration of low-low clustering pixels will exert a more significant cool island effect (Feng et al., 2014; Li and Chen, 2023b). Sustainability denotes the ability of the “cool island” to consistently and durably exert the cool island effect in a high-temperature setting. A cold source with superior habitat quality, reduced nighttime light brightness, and increased landscape diversity is less susceptible to human disturbance (Shen, 2021), thus demonstrating enhanced sustainability in urban heat regulation.
The cooling contribution index, CCI, is given by the Equation 18 below:
where
The resistance index, RI, to heat stress involves dividing the specified study area into grid cells measuring 250 × 250 m. Utilising the regional analysis tool in ArcGIS 10.8, the average LST of each grid cell is obtained and further analysed through cluster and outlier assessments employing Anselin Local Moran’s I method. Values of 9, 7, 5, 3, and 1 are respectively assigned to pixels located within low-low clusters, low-high clusters, non-significant clusters, high-low clusters, and high-high clusters. RI is calculated by Equation 19:
where pi is the value assigned to the ith pixel, and n is the total number of pixels in the cool island.
According to the weight determination method described above for the urban heat vulnerability indicator system, we conducted the assignment of weights and normalisation of the different indicators. Subsequently, we integrated them to finalise the assessment of cool island quality for Fuzhou.
Based on the assessment results concerning urban heat risk and cool island quality, we identified patches with high heat risk and the top two tiers of cold island patches. Utilising morphological spatial pattern analysis with the GUIDOS software, we delineated core areas exhibiting robust stability. Subsequent screening was conducted based on the patch connectivity index, which assesses the relative significance of individual patches within the study area’s landscape. This index quantifies ecological structure connectivity and the strength of landscape links between patches, widely applied in landscape ecology (Liang et al., 2023). Enhancing heat transfer between CHRSs and CCSs, and quantifying patch connectivity substantially fosters cool island effects synergy among CCSs. Hence, we employed the integral index of connectivity (IIC), probability of connectivity (PC), and patch importance (dI) to assess patch connectivity levels.
The Equations 20–22 for which are shown below:
where n is the total number of patches; xa and xb are the areas of patch a and patch b, respectively; nIab is the number of linkages between patch a and patch b; Pab is the maximum probability of linkages between patch a and patch b; I is the value of the connectivity index; and Iremove is the connectivity index after a given patch is removed.
Considering the patch distribution in the research area and existing literature (Xiang et al., 2023), we selected two specific types of patches, namely, those with dIIC > 0.2 and dPC > 0.3, as the ultimate CHRSs and CCSs. Particularly, CCSs with high connectivity adjacent to CHRSs were individually identified as core transition cold sources (CTCSs) and were subsequently designated as target patches for TC linkage.
The resistance surface represents the distribution of resistance levels to heat diffusion across various thermal environment landscape patterns, and is reflective of accessibility and cooling effect trends. The spatial structure of the resistance surface directly impacts the conversion and redistribution of incident surface net solar radiation, with net solar radiation primarily distributed in near-surface sensible heat flux (exchange between the surface and the atmosphere), near-surface latent heat flux (evaporation or condensation), and soil heat flow (Li and Huang, 1996; Li and Chen, 2023a). Hence, we employed eight indicators, including land-use type, NDVI, normalised difference impervious surface index (NDISI), MNDWI, building height, elevation, slope, and floor area ratio (FAR), to preliminarily assess the level of heat transfer resistance within the surface thermal environment pattern.
The NDISI (Sun et al., 2017) and FAR, are calculated as shown in Equations 23, 24:
where ρNIR, ρMIR1, and ρTIR are the near-infrared band, mid-infrared band 1, and thermal infrared band of Landsat8 images, respectively and MNDWI is the modified normalised difference water index.
where C is the number of floors, F is the building land area, and A is the grid cell land use area.
The SPCA method was utilised to create the comprehensive resistance surface. SPCA, a multivariate statistical technique, transforms correlated variable data into linearly uncorrelated data, facilitating the mapping of three-dimensional spatial data to two-dimensional space with clarity (Zou and Yoshino, 2017). The analysis was conducted using the Principal Components tool in ArcGIS 10.8, as indicated by the Equation 25:
where R is the comprehensive result of the resistance surface, An is the contribution rate of the nth principal component, and PCn is the nth principal component.
The urban composite cooling network comprises TCs and SCs. TCs facilitate the acceleration of heat transport from CHRSs to CCSs, whereas SCs enhance the synergy of the cool island effect among CCSs, not only receiving and converting the transported heat but also providing compensation of the cool island effect to the surrounding environment.
We identified highly interconnected CCSs with substantial areas positioned adjacent to CHRSs. Leveraging the precision of the MCR model in delineating corridor initiation and termination points, we utilised the cost path, cost distance, and cost backlink functionalities within the ArcGIS platform to extract the optimal path between two different patches based on the comprehensive resistance surface raster data. The centre of CHRSs served as the point of origin, with the centre of CCSs designated as the terminus. These pathways were designated as TCs.
The extraction of SC was conducted based on the CT model utilising the Linkage Mapper tool, as compared with the MCR model, the CT model excels at eliminating corridor redundancies by pinpointing the most efficient path for establishing ecological connections between single-type sources. Additionally, the CT model can accurately identify pivotal nodes for enhancing or disrupting linkages through the analysis of simulated current flow and resistance. The calculation of current flow centrality for SCs was conducted using the Centrality Mapper module to assess their significance. To enhance the administration and conservation of premium cool islands within the research area, the Pinchpoint Mapper and Barrier Mapper tools were applied to pinpoint critical areas within SCs as cooling nodes and essential restoration efforts. Subsequently, a composite cooling network was developed by superimposing TCs and SCs in ArcGIS, with a focus on optimising duplicate corridors.
The natural breakpoint method was utilised to categorise the three assessment indices and HRI into five levels. Elevated levels of heat hazard and heat exposure were observed in the central urban area and southeastern coastal region according to each specific assessment criterion (Figures 4A, B). Additionally, urban expansion often coincides with intense human activities and alterations in the land cover, leading to a heightened exposure within these areas to the thermal environment and consequently increasing the level of heat exposure. In the western mountainous regions, there was a discernible decrease in heat hazard and exposure. Nevertheless, human activities and land development were observed in the upper regions of the Min River in the west and the southwestern forests, which exhibited elevated LST values compared with their surroundings. Fuzhou, being a mountainous city, has historically ensured the preservation of its forest resources. Consequently, the mountainous ecological forest zones and remote mountainous areas demonstrate reduced susceptibility to heat stress owing to their exceptional ecological assets. In contrast, the central urban area, despite its advanced socioeconomic status, experiences a diminishing resilience in the face of heat stress due to the fragmentation of green and blue spaces and a suite of ecological challenges stemming from intensive urban expansion (Figure 4C).
Figure 4. Result of heat risk assessment in the study area (A) Heat hazard, (B) Heat exposure, (C) Heat vulnerability, (D) Standard deviation ellipse, (E) Comprehensive heat risk in study area.
The results of the comprehensive HRI assessment are depicted in Figure 4E. Low and low-medium risk areas accounted for more than 60% of the total study area (40.03% and 22.26%, respectively), medium and high-medium risk areas accounted for more than 10% of the total area (15.98% and 11.94% respectively), and high-risk areas accounted for the least proportion of the study area (8.79%). It is evident that the central urban area and eastern coastal region of Fuzhou exhibited considerably elevated HRI levels. Between the central urban area and the southeastern coastal region, substantial medium to high-risk zones were discernible. Areas with lower risk were primarily concentrated in the western mountainous regions. The Min River, a pivotal water resource in Fuzhou, displayed varying degrees of heat risk, with localised high-risk zones noted in its upper reaches and the southwestern mountainous areas. Utilising the Directional Distribution tool in ArcGIS 10.8, standard deviational ellipses for HRI were computed (Figure 4D). The semi-major axis of the ellipse signifies the distribution and trajectory of heat risk, while the semi-minor axis indicates the range and dispersion of heat risk. The result of analysis reveals that the epicentre of heat risk in Fuzhou was concentrated in the central urban area, with a discernible tendency towards expansion towards the southeastern coast. Consequently, it is imperative to implement effective mitigation strategies for heat reduction to impede the further propagation and exacerbation of heat risk.
The intensity and sustainability indicator values of the cool island effect are shown in Figure 5A and B respectively. The comprehensive cold island quality assessment is shown in Figure 5C and is divided into four levels by natural breakpoint method. Cool islands rated as “excellent” and “poor” accounted for only 11.31% and 7.45% of the total cool island area, respectively, while the remaining 44.49% and 36.75% were “average” and “good”, respectively.
Figure 5. Result of cool islands quality assessment. (A) Cool islands effect intensity, (B) Cool islands effect sustainability, (C) Cool islands quality in study area.
“Good” cool islands were primarily dispersed as blocks in the western mountainous regions and northeastern areas, predominantly consisting of forest lands. “Excellent” cool islands were sporadically located within the “good” cool island patches. “Poor” cool islands were predominantly concentrated in proximity to urban developments, while relatively expansive segments of “average” cool islands were interspersed among the various cool island levels. The Min River traversing the central urban zone has suffered adverse effects due to urban expansion, with cool island quality predominantly falling into the categories of “average” and “good”. A limited number of “poor” cool islands were identified in select southwestern areas, attributed to landscape fragmentation and land use conflicts arising from human activities, significantly impacting the quality of adjacent cool islands. In conclusion, the “excellent” and “good” cool islands displayed notable patch and cluster characteristics, enabling them to consistently and effectively contribute to enhancing the local thermal environment.
Next, patches with high heat risk and cool islands rated “excellent” and “good” were extracted. Following a patch connectivity analysis (Supplementary Table S1), patches with small areas and poor connectivity were eliminated, yielding 21 CHRSs and 32 CCs (including 14 CTCSs) (Figure 6). The total area covered by these two types of sources was 11,41.62 km2 and 3,198.42 km2, respectively, while that of individual patches was >0.5 km2, with dIIC >0.2 and dPC >0.3. CHRSs were concentrated in the central urban area and southeastern coastal area. The largest CHRS was patch #21, whereas patch #9 was the smallest. CCSs were mainly concentrated in the northwestern mountainous area and northeastern area, the largest of which, patch #30, was in the southern part of Yongtai County. There were also relatively large CCS patches in the northern part of Minhou County and the eastern and northern parts of Luoyuan County and Lianjiang County. The smallest was patch #12 near the central urban area. CCSs #18, #21 and #22 were relatively complete linear rivers that, together with CCS #24 and #27, were located within the CHRS pattern and encountered heightened ecological pressures. Overall, CHRS and CCS exhibited a spatial pattern featuring the differentiation between the northwest and southeast, with a high degree of separation. Numerous large CCSs were relatively far from CHRSs, resulting in the lack of heat transport and long-term saturation of CHRSs.
The process and results relating to the establishment of the comprehensive resistance surface are outlined in Figure 7. Detailed data pertaining to the various resistance factors and the outcomes of SPCA analysis are available in Supplementary Tables S2, S3. The top five principal components, showcasing cumulative variance contribution rates exceeding 90%, were utilised for the development of the resistance surface. This surface serves as a broad representation of the heat transfer resistance levels within the surface thermal environment framework. Regions with high resistance levels were primarily concentrated in the central urban area and developed zones along the southeastern coast, aligning closely with the distribution of high heat-risk regions in the specified area. Conversely, low-resistance zones tended to coincide with cool islands within the study area, predominantly comprising green infrastructure such as forested areas, water bodies, and grasslands, which contribute significantly to ecological enhancements.
A total of 94 least-cost paths from CHRS to CTCS were extracted based on the MCR model (Figure 8A). Initially, all CHRSs were connected before linking to CTCSs, thus forming TCs spanning a total length of 2,178.73 km. After buffering by 200 m, the TCs were overlaid with the LST in 2020 (Figure 8C), showing the high-to-low changes in LST from the starting to the ending point of the corridors and reflecting the gradual heat transfer process from CHRSs to CCSs. Using the Linkage Mapper, a total of 96 SCs were extracted, spanning a total length of 2,615.64 km (Figure 8B). The current flow centrality and rank of the SCs were calculated using the Centrality Mapper. A total of 27 key SCs were extracted, spanning a total length of 524.01 km, most of which were links between large CCS patches and distributed within the rich forest resources of the northwestern mountainous areas. A small number was also distributed in the transition zones between the central urban area and the northwest. The remaining 69 were important SCs, spanning a total length of 2,091.63 km, primarily connecting CCS patches of uneven sizes in the northwestern region. Furthermore, there exists a relatively dense distribution of important SCs between the two major river systems, Min River and Wulong River, and the northwestern cool islands. This was due to the special, geographical, and environmental conditions of the central urban area within this study area; therefore, the cool island patches dominated by the Min River and Wulong River are key areas to strengthen the synergy between the northern and southern cool islands. The average LST of SCs (25.34°C) was lower than that of TCs (27.89°C) and significantly lower than that of high heat-risk areas (31.14°C). This explains, the hierarchical relationship between SCs and TCs, and the gradual regulation of the thermal environment climate. The composite cooling network constructed by overlaying the two types of corridors is shown in Figure 8D.
Figure 8. Composite cooling network introduction (A) Transport corridors (TCs), (B) synergy corridors (SCs), (C) heat transfer paths for TCs, (D) composite cooling corridors.
Based on the construction of SCs, the Pinchpoint Mapper tool was utilised to calculate the current density in the all-to-one and pairwise modes (Figures 9A, B). A total of 148 cooling nodes were identified. The Barrier Mapper tool, with a search radius of 400 m, was employed to detect barriers in relation to the least-cost paths using the moving window search method in both improved and unimproved modes (Figures 9C, D). A total of 78 barrier nodes were determined. It is noteworthy that the cooling nodes exhibited dense distribution within the highly interconnected large cool island patches in the northwest, as well as in the transitional areas between the central urban region and northwestern cool islands. This distribution arose from the intricate structure of the cool island networks linking CTCSs in the transition zones. As these networks traversed the central urban area, their cooling impact extended effectively into this urban section. Furthermore, these cool island networks can be well-connected to TCs to further receive and convert the heat from CHRSs. The barrier points were distributed over a large area in the transition zone from the central urban area to the southeastern coastal area. CCS #24 and #27 within this area bear relatively high ecological pressure. Furthermore, some barrier nodes existed in areas with severe landscape fragmentation, such as the denuded mountains and certain sandbars in the west. The removal of these barrier nodes is critical to alleviating landscape fragmentation and ecological protection pressures, consequently strengthening the synergy of cool island effects among CCSs. The composite cooling network was overlaid with the two types of nodes to obtain the final overall layout of the composite cooling network for the study area (Figure 10).
Figure 9. Cooling nodes and barrier nodes identification (A) All-to-one model, (B) Pairwise model, (C) Unimproved model, (D) Improved model.
The mitigation of heat risk through sustainable urban cooling is a systematic and multi-objective process. Previous studies have primarily focused on the establishment of natural cooling networks known as “source-source” networks, utilising urban blue and green patches. Nonetheless, this approach fails to comprehensively depict the energy transfer dynamics of surface thermal environments in their natural state. Consequently, the cooling advantages of cool island patches located outside urban areas are not efficiently transmitted to high-risk zones, and heat accumulation in these areas remains inadequately managed or converted promptly. Drawing from the surface energy balance theory and the principles of energy conservation in thermodynamics, incoming surface energy can be converted into diverse forms of energy to uphold system equilibrium.
This process can be expressed by the surface energy balance theory, as shown in Equation 26 (Fernández et al., 2021):
where Q* is the surface net radiation; QF is anthropogenic heat; QH is the sensible heat flux; QE is the latent heat flux; and QS is the storage heat flux.
Numerous studies (Li and Huang, 1996; Liu J. et al., 2018; Fernández et al., 2021) have highlighted the close correlation between the spatial distribution of surface energy and land cover types. Specifically, water bodies and vegetation exhibit higher levels of net radiation and latent heat flux. In contrast, impervious surfaces display elevated levels of sensible heat flux and storage heat flux, while urban areas demonstrate increased anthropogenic heat (Liu J. et al., 2018). Fuzhou’s high heat-risk areas were found to be primarily distributed in the central urban region and developed areas along the southeastern coast. Relative to non-built-up-areas, these high heat-risk areas absorb solar radiation during the day owing to the physical properties of the impervious surface and building materials (Hao L. et al., 2023). When the absorbed incoming solar longwave and shortwave radiations exceed the outgoing radiation, heat exchange occurs between the artificial surface and the atmosphere, resulting in the conversion of energy into sensible heat flux and storage heat flux, hence increases in temperature (Gago et al., 2013). Additionally, this phenomenon greatly impedes vegetation transpiration and soil evaporation processes and also significantly reduces latent heat flux, weakening its cooling effect and leading to local surface energy imbalances (Hou et al., 2022). The implementation of a composite cooling network, as detailed in this study, effectively addressed this surface energy imbalance. TCs play a vital role in decreasing heat storage flux, sensible heat flux, and anthropogenic heat in the environment by promoting heat transfer from high-risk zones to adjacent cool islands. Further, compared with fragmented cold islands, interconnected cold islands have a more efficient and stable cooling effect (Qiu et al., 2023). Therefore, SCs can enhance latent heat flux in cool island patches to some extent, thereby mitigating potential high-risk sources in the western developed areas by reinforcing the sustainability and resilience of the cool island effect.
Consequently, the variables of the surface energy balance equation can be altered, thereby strengthening the overall energy flow and conversion process of the system and persistently maintaining its balance. This, in turn, can mitigate heat risk and curb its spread along the southeastern coast.
Owing to the low number of high-quality cool island patches distributed in the central urban area and southeastern coastal built-up area, the southeastern CHRSs were positioned at a considerable distance from these patches. As a result, a direct linkage to a corridor for southeast-northwest heat exchange was unattainable.
Therefore, based on the results of constructing the composite cooling network, we propose the following urban intervention strategies to mitigate urban heat risk: (1) high-quality urban cool islands in the west should be protected. The landscape features, and spatial layout of important GI, such as mountains and rivers, outside the city should be optimised. The abundant western mountain resources in Yongtai, Minqing, Luoyua, and Lianjiang Counties should be utilised to construct an ecological barrier for the western cool islands and accelerate the transformation of low-quality cool islands into high-quality cool islands (Li and Chen, 2023b). A cool island compensation system should be developed, radiating from the central urban area to the southeastern coast based on the landscape pattern of the main urban area dominated by the Min River, Qi Mountain and Gu Mountain. (2) Cooling nodes, which have a better cooling effect and are key locations for cold network connectivity, should be protected and optimised (Zhang Y. et al., 2023). The improvement measures mainly include adding green spaces at nodes to increase the greening rate, promoting the formation of new CCSs, and exploring potential corridors while also encouraging the transformation of important SCs to key SCs. In addition, cooling nodes can be added within built-up areas by building green paths and belts in park systems to improve the local climate (Xiang et al., 2023). (3) Barrier nodes should be removed and transformed. Barrier nodes are areas of the cold network that may impede its connectivity and may lead to breaks in the network (Liu et al., 2024). By incorporating the city master plan, adjustments should made to Fuzhou’s current land use status, barrier nodes should be removed, and future construction and development intensity in high-risk areas should be appropriately controlled. In addition, rooftop greening, vertical greening, and other greening strategies can be employed to transform barrier points into new cooling nodes to minimise the reflection of solar radiation by impervious surfaces and building materials (Xiang et al., 2024).
Unlike the direct patch approach, it has been previously conformed that connecting large cool island patches along a city’s periphery can effectively enhance their cooling effect and serve adjacent built-up areas (Mokhtari et al., 2022; Liu et al., 2024). However, built-up areas distant from the cool island network receive minimal compensation. The possibility of creating network connections between low-temperature and high-temperature patches at the regional scale has been investigated in previous studies (Li and Chen, 2023a; Xiang et al., 2023), and it has been observed that such network connections can have positive effects on the enhancement of local urban thermal environments. Thus, we approached the problem from the perspective of regulating surface energy balance. While constructing the urban peripheral cool island network, we followed relevant thermodynamic theories and attempted to build heat reduction corridors to neighbouring cool islands in high-risk areas. It is expected that the application of this approach will address the issue of insufficient compensation resulting from relying solely on the urban peripheral cool island. The composite cooling network forms a structure spanning the entire city, accelerates the energy flow process of the surface thermal environment pattern and also elucidates its complex and systematic nature. Therefore, this proactive measure can be implemented to mitigate urban heat risk.
Initially, an evaluation and ranking of urban heat risk and cool island quality was conducted based on data from multiple sources. This methodology diverges from previous studies (Liu et al., 2024) where only LST, in conjunction with land use data, were employed to identify sources. The current approach ensures a more precise selection of sources, enhancing the efficacy of network establishment in mitigating heat risk. Additionally, a blend of subjective and objective techniques (AHP and EWM) was utilised to ascertain the weightage of different indicators. This strategy circumvents excessive subjectivity and arbitrariness often associated with a singular method (Qian et al., 2023), thus augmenting the precision of the results derived from the comprehensive evaluation. Subsequently, in the construction of the resistance surface, consideration was given to both two-dimensional and three-dimensional spatial data, employing the SPCA method for weight determination, thereby refining the accuracy of the overall resistance surface details. Finally, while selecting the methodologies for developing the composite cooling network, it is crucial to note that TC primarily links two distinct sources (CHRS and CCS), whereas CT can solely establish network connections amongst sources of the same type. Therefore, the latter option was more suitable. SCs, on the other hand, serve as vital ecological network connections among CCSs. CT demonstrates a higher level of advancement in ecological network development than the MCR. The utilisation of the Centrality Mapper tool enables the classification of corridors and accentuates the energy flow distinctions between them, highlighting their pivotal role within the network structure (Li et al., 2023). CT can ascertain the spatial positions of cooling nodes and barrier points by analysing the formation of SCs, leading to the proposition of targeted enhancement strategies (Xiang et al., 2023). In conclusion, the establishment of the composite cooling network establishes a closed loop from the sources to the network and further to the nodes, thereby enhancing the thoroughness and practical applicability of this research. It also integrates pertinent thermodynamic principles to offer valuable insights for mitigating urban heat risks amidst rapid urbanisation, as well as proposing strategies for sustainable urban development and urban climate adaptation planning.
This study had several limitations that need to be acknowledged. First, TCs were constructed aiming to rectify disparities in surface energy balance without taking the real-world conditions of the study area into account. Therefore, to ensure the feasibility of such corridors in practical settings, it is imperative to integrate the real-world conditions of the study area and conduct a comprehensive assessment based on third-party reports and relevant theoretical foundations. Additionally, given that SCs function as conduits to the urban fringe cool island network, further field studies are necessary to validate the credibility of all sources and nodes and assess the networking potential. Second, we omitted three-dimensional variables, such as the sky view factor and wind direction. Therefore, to improve the resistance surface through in-depth analysis of multi-dimensional environmental factors in future, it is necessary to take into account these variables. Third, in this study, we used fixed date surface temperature data, which may not fully capture seasonal changes in extreme heat, to characterize thermal heat. Fourth, to assess urban thermal risk and cold island quality, the selection of some indicators was subjective due to the constraints of data accuracy and availability. In the future, it will be necessary to comprehensively employ multi-source data and research methods to accurately quantify the periodicity of extreme heat, and more research results will be consulted to ensure the accuracy and objectivity of the screening of evaluation indicators. Moreover, we focused on how to construct a composite cooling network and identify key nodes, without fully discussing the cooling effect of network construction. In future studies, higher-resolution data can be integrated to quantify and analyse the cooling effect of the network and the extent of heat risk mitigation in greater detail.
The exacerbation of urban heat risk presents a significant challenge to contemporary urban development. In this study, a dual evaluation of heat risk and cool island quality was conducted in the research location to precisely pinpoint CHRSs and CCS. Subsequently, MCR and CT models were employed to establish a comprehensive cooling network for alleviating heat risk. Our findings were as follows: (1) the central urban area of Fuzhou had the most severe heat risk, followed by the eastern coastal areas, showing a trend of further expansion towards the southeastern coast. (2) The cool islands in the western mountainous areas of Fuzhou were relatively high quality, whereas cool islands located in the periphery of the central urban area and the transition zone from the central urban area to the southeastern coastal area were subjected to a higher level of ecological pressure. (3) A total of 21 CHRSs and 32 CCSs were extracted, and 94 TCs and 96 SCs were constructed, which constituted the urban composite cooling network; 148 cooling nodes and 78 cooling barrier points were also identified. Based on the relevant theories of thermodynamics and landscape ecology, we explained the feasibility of constructing a network to achieve sustainable urban cooling in the urban thermal environment of the study area. (4) Cooling nodes mostly occurred in the key SCs between western mountainous areas and within high-quality CCSs. Barrier points were mostly located near the relatively scattered cool islands in the outskirts of the central urban area. Implementing specific strategies to safeguard cooling nodes and remove obstacles hindering the cooling process can enhance the safeguarding of cool island assets within the research area. This action also improves the overall reliability of the integrated cooling system, enhancing its practical applicability in construction projects.
In conclusion, ecological network identification techniques were applied to analyse the urban thermal environment. Heat risk assessment and cool island quality assessment were integrated into the preliminary phase of network construction, emphasising the importance of proactively adapting to and mitigating urban heat risk changes. The methodological framework presented in this study offers valuable insights for future research on optimising the thermal environment pattern and reducing heat risk.
The original contributions presented in the study are included in the article/Supplementary Material, further inquiries can be directed to the corresponding author.
HX: Conceptualization, Formal Analysis, Methodology, Software, Writing–original draft, Writing–review and editing. JY: Funding acquisition, Methodology, Writing–review and editing. YL: Formal Analysis, Validation, Writing–review and editing. NX: Investigation, Visualization, Writing–review and editing. ML: Conceptualization, Data curation, Writing–review and editing. YX: Methodology, Writing–review and editing. XL: Software, Writing–review and editing. FL: Funding acquisition, Project administration, Supervision, Writing–review and editing.
The author(s) declare that financial support was received for the research, authorship, and/or publication of this article. This research was funded by the Fujian Provincial Department of Science and Technology (No. 2021Y4009).
The authors declare that the research was conducted in the absence of any commercial or financial relationships that could be construed as a potential conflict of interest.
All claims expressed in this article are solely those of the authors and do not necessarily represent those of their affiliated organizations, or those of the publisher, the editors and the reviewers. Any product that may be evaluated in this article, or claim that may be made by its manufacturer, is not guaranteed or endorsed by the publisher.
The Supplementary Material for this article can be found online at: https://www.frontiersin.org/articles/10.3389/fenvs.2024.1462700/full#supplementary-material
GI, green infrastructure; CHRSs, core heat risk sources; CCSs, core cold sources; MCR, minimal cumulative resistance; CT, circuit theory; TCs, transport corridors; SCs, synergy corridors; IPCC, Intergovernmental Panel on Climate Change; GI, Green infrastructure; LST, land surface temperature; SPCA, spatial principal component analysis; AHP, analytic hierarchy process, EWM, entropy weight method; IIC, integral index of connectivity; PC, probability of connectivity; CTCSs, core transition cold sources; HRI, heat risk index.
Aerts, J., Botzen, W. J., Clarke, K. C., Cutter, S. L., Hall, J. W., Merz, B., et al. (2018). Integrating human behaviour dynamics into flood disaster risk assessment. Nat. Clim. Chang. 8 (3), 193–199. doi:10.1038/s41558-018-0085-1
Åström, D. O., Forsberg, B., and Rocklöv, J. (2011). Heat wave impact on morbidity and mortality in the elderly population: a review of recent studies. Maturitas 69 (2), 99–105. doi:10.1016/j.maturitas.2011.03.008
Bouketta, S. (2023). Urban Cool Island as a sustainable passive cooling strategy of urban spaces under summer conditions in Mediterranean climate. Sust. Cities Soc. 99, 104956. doi:10.1016/j.scs.2023.104956
Chen, J. D., Gao, M., Cheng, S. L., Hou, W. X., Song, M. L., Liu, X., et al. (2020a). County-level CO2 emissions and sequestration in China during 1997-2017. Sci. Data. 7 (1), 391. doi:10.1038/s41597-020-00736-3
Chen, Y., Zhang, N., and Zhu, Y. (2020b). Simulation of summer high temperature regulation mechanism in urban areas. J. Nat. Disasters 29 (01), 193–202. doi:10.13577/j.jnd.2020.0120
Chen, Y. H., Cai, Y., and Tong, C. (2020c). Temperature effect under the green space evolution based on remote sensing: a case study of Fuzhou, China. Acta Ecol. Sin. 40 (07), 2439–2449. doi:10.5846/stxb201901030023
Cheng, Y., and Wu, C. (2020). Planning approach of urban blue-green space based on local climate optimization: a review. Chin. J. Appl. Ecol. 31 (11), 3935–3945. doi:10.13287/j.1001-9332.202011.014
Debbage, N., and Shepherd, J. M. (2015). The urban heat island effect and city contiguity. Comput. Environ. Urban Syst. 54, 181–194. doi:10.1016/j.compenvurbsys.2015.08.002
Escobedo, F. J., Adams, D. C., and Timilsina, N. (2015). Urban forest structure effects on property value. Ecosyst. Serv. 12, 209–217. doi:10.1016/j.ecoser.2014.05.002
Estoque, R. C., Ooba, M., Seposo, X. T., Togawa, T., Hijioka, Y., Takahashi, K., et al. (2020). Heat health risk assessment in Philippine cities using remotely sensed data and social-ecological indicators. Nat. Commun. 11 (1), 1581. doi:10.1038/s41467-020-15218-8
Fei, F., Wang, L. Y., Wang, Y., Yao, W. X., Fukuda, H., Xiao, Y. L., et al. (2023). A new method for evaluating the synergistic effect of urban water body and vegetation in the summer outdoor thermal environment. J. Clean. Prod. 414, 137680. doi:10.1016/j.jclepro.2023.137680
Feng, Y., Hu, T., and Zhang, L. (2014). Impacts of structure characteristics on the thermal environment effect of city parks. Acta Ecol. Sin. 34 (12), 3179–3187. doi:10.5846/stxb201306101641
Fernández, M. E., Picone, N., Gentili, J. O., and Campo, A. M. (2021). Analysis of the urban energy balance in bahia blanca (Argentina). Urban Clim. 37, 100856. doi:10.1016/j.uclim.2021.100856
Gago, E. J., Roldan, J., Pacheco-Torres, R., and Ordonez, J. (2013). The city and urban heat islands: a review of strategies to mitigate adverse effects. Renew. Sust. Energ. Rev. 25, 749–758. doi:10.1016/j.rser.2013.05.057
Hao, L., Sun, G., Huang, X., Tang, R., Jin, K., Lai, Y., et al. (2023a). Urbanization alters atmospheric dryness through land evapotranspiration. Npj Clim. Atmos. Sci. 6 (1), 149. doi:10.1038/s41612-023-00479-z
Hao, T. P., Zhao, Q. S., and Huang, J. X. (2023b). Optimization of tree locations to reduce human heat stress in an urban park. Urban For. Urban Green. 86, 128017. doi:10.1016/j.ufug.2023.128017
Heaton, M. J., Sain, S. R., Greasby, T. A., Uejio, C. K., Hayden, M. H., Monaghan, A. J., et al. (2014). Characterizing urban vulnerability to heat stress using a spatially varying coefficient model. Spatial spatio-temporal Epidemiol. 8, 23–33. doi:10.1016/j.sste.2014.01.002
Hou, H., Su, H., Liu, K., Li, X., Chen, S., Wang, W., et al. (2022). Driving forces of UHI changes in China's major cities from the perspective of land surface energy balance. Sci. Total Environ. 829, 154710. doi:10.1016/j.scitotenv.2022.154710
Hu, H., and Xiong, Y. (2015). A research summary of extreme heat wave. Adv. Meteorological Sci.5 (01), 18–22. doi:10.3969/j.issn.2095-1973.2015.01.002
Jamei, E., Rajagopalan, P., Seyedmahmoudian, M., and Jamei, Y. (2016). Review on the impact of urban geometry and pedestrian level greening on outdoor thermal comfort. Renew. Sust. Energ. Rev. 54, 1002–1017. doi:10.1016/j.rser.2015.10.104
Johnson, D. P., Stanforth, A., Lulla, V., and Luber, G. (2012). Developing an applied extreme heat vulnerability index utilizing socioeconomic and environmental data. Appl. Geogr. 35 (1-2), 23–31. doi:10.1016/j.apgeog.2012.04.006
Li, C., and Chen, T. (2023a). Construction of multi-level source-sink landscape network to alleviate urban thermal environment. Acta Ecol. Sin. 43 (08), 3068–3078. doi:10.5846/stxb202201120112
Li, C., and Chen, T. (2023b). Evaluation and optimization strategy of cold source quality to alleviate urban thermal environment. Econ. Geogr. 43 (03), 100–108. doi:10.15957/j.cnki.jjdl.2023.03.011
Li, C., Wu, Y. M., Gao, B. P., Zheng, K. J., Wu, Y., and Wang, M. J. (2023). Construction of ecological security pattern of national ecological barriers for ecosystem health maintenance. Ecol. Indic. 146, 109801. doi:10.1016/j.ecolind.2022.109801
Li, J., Nie, W., Zhang, M., Wang, L., Dong, H., and Xu, B. (2024). Assessment and optimization of urban ecological network resilience based on disturbance scenario simulations: a case study of Nanjing city. J. Clean. Prod. 438, 140812. doi:10.1016/j.jclepro.2024.140812
Li, Y., and Huang, M. (1996). Analysis of Land surface evaporation and heat balance in the transational zone of oasis-desert. Arid. Land Geogr. (03), 80–87. doi:10.13826/j.cnki.cn65-1103/x.1996.03.012
Liang, G. F., Niu, H. B., and Li, Y. (2023). A multi-species approach for protected areas ecological network construction based on landscape connectivity. Glob. Ecol. Conserv. 46, e02569. doi:10.1016/j.gecco.2023.e02569
Liang, J., Li, Z. J., Yang, Q. C., Lei, X. H., Kang, A. Q., and Li, S. F. (2019). Specific vulnerability assessment of nitrate in shallow groundwater with an improved DRSTIC-LE model. Ecotox. Environ. Safe. 174, 649–657. doi:10.1016/j.ecoenv.2019.03.024
Liu, F., Liu, J., Zhang, Y., Hong, S., Fu, W., Wang, M., et al. (2024). Construction of a cold island network for the urban heat island effect mitigation. Sci. total Environ. 915, 169950. doi:10.1016/j.scitotenv.2024.169950
Liu, J., Chen, T., and Wang, L. (2022). Thermal health risk identification, assessment and urban design intervention in high density cities from the perspective of climate change: a case study of Macao. Urban Plan. Int., 1–14. doi:10.19830/j.upi.2022.135
Liu, J., Zhao, X., and Lin, J. (2018a). Urban expansion and its impact on surface energy balance: a case study of xiamen. Environ. Sci. and Technol. 41 (S1), 336–344. doi:10.19672/j.cnki.1003-6504.2018.S1.060
Liu, S., Liu, L., Wu, X., Hou, X., Zhao, S., and Liu, G. (2018b). Quantitative evaluation of human activity intensity on the regional ecological impact studies. Acta Ecol. Sin. 38 (19), 6797–6809. doi:10.5846/stxb201711172048
Martin, Y., and Paneque, P. (2022). Moving from adaptation capacities to implementing adaptation to extreme heat events in urban areas of the European Union: introducing the U-ADAPT! research approach. J. Environ. Manag. 310, 114773. doi:10.1016/j.jenvman.2022.114773
Mokhtari, Z., Barghjelveh, S., Sayahnia, R., Karami, P., Qureshi, S., and Russo, A. (2022). Spatial pattern of the green heat sink using patch- and network-based analysis: implication for urban temperature alleviation. Sust. Cities Soc. 83, 103964. doi:10.1016/j.scs.2022.103964
Mora, C., Dousset, B., Caldwell, I. R., Powell, F. E., Geronimo, R. C., Bielecki, C. R., et al. (2017). Global risk of deadly heat. Nat. Clim. Chang. 7 (7), 501–506. doi:10.1038/nclimate3322
Morabito, M., Crisci, A., Gioli, B., Gualtieri, G., Toscano, P., Di Stefano, V., et al. (2015). Urban-hazard risk analysis: mapping of heat-related risks in the elderly in major Italian cities. PLoS One 10 (5), e0127277. doi:10.1371/journal.pone.0127277
Murray, V., and Ebi, K. L. (2012). IPCC special report on managing the risks of extreme events and disasters to advance climate change adaptation (SREX). J. Epidemiol. Community Health. 66 (9), 759–760. doi:10.1136/jech-2012-201045
Pan, M., Lan, S., Zhu, L., Liu, S., Zhang, X., and Xiu, X. (2020). Influence of landscape pattern types on heat island effect over central Fuzhou City. China Environ. Sci. 40 (06), 2635–2646. doi:10.19674/j.cnki.issn1000-6923.20200323.001
Peng, J., Cheng, X. Y., Hu, Y. X., and Corcoran, J. (2022). A landscape connectivity approach to mitigating the urban heat island effect. Landsc. Ecol. 37 (6), 1707–1719. doi:10.1007/s10980-022-01439-3
Qian, W. Q., Zhao, Y., and Li, X. Y. (2023). Construction of ecological security pattern in coastal urban areas: a case study in Qingdao, China. Ecol. Indic. 154, 110754. doi:10.1016/j.ecolind.2023.110754
Qiu, J., Li, X., and Qian, W. (2023). Optimizing the spatial pattern of the cold island to mitigate the urban heat island effect. Ecol. Indic. 154, 110550. doi:10.1016/j.ecolind.2023.110550
Rowlinson, S., Yunyanjia, A., Li, B. Z., and Chuanjingju, C. (2014). Management of climatic heat stress risk in construction: a review of practices, methodologies, and future research. Accid. Anal. Prev. 66, 187–198. doi:10.1016/j.aap.2013.08.011
Sahoo, S., Dhar, A., and Kar, A. (2016). Environmental vulnerability assessment using Grey Analytic Hierarchy Process based model. Environ. Impact Assess. Rev. 56, 145–154. doi:10.1016/j.eiar.2015.10.002
Sanderson, E. W., Jaiteh, M., Levy, M. A., Redford, K. H., Wannebo, A. V., and Woolmer, G. (2002). The human footprint and the last of the wild. Bioscience 52 (10), 891–904. doi:10.1641/0006-3568(2002)052[0891:Thfatl]2.0.Co;2
Sharma, J., and Ravindranath, N. H. (2019). Applying IPCC 2014 framework for hazard-specific vulnerability assessment under climate change. Environ. Res. Commun. 1 (5), 051004. doi:10.1088/2515-7620/ab24ed
Shen, C. H., Hou, H., Zheng, Y. Y., Murayama, Y., Wang, R. C., and Hu, T. A. (2022). Prediction of the future urban heat island intensity and distribution based on landscape composition and configuration: a case study in Hangzhou. Sust. Cities Soc. 83, 103992. doi:10.1016/j.scs.2022.103992
Shen, F. X., Yang, L., He, X. L., Zhou, C. H., and Adams, J. M. (2020). Understanding the spatial-temporal variation of human footprint in Jiangsu Province, China, its anthropogenic and natural drivers and potential implications. Sci. Rep. 10 (1), 13316. doi:10.1038/s41598-020-70088-w
Shen, J. (2021). The measurement of urban concentration in China and the study of its spatial differentiation characteristics: based on DMSP-OLS nighttime light data. Econ. Geogr. 41 (05), 46–56. doi:10.15957/j.cnki.jjdl.2021.05.006
Sobrino, J. A., Jiménez-Muñoz, J. C., and Paolini, L. (2004). Land surface temperature retrieval from LANDSAT TM 5. Remote Sens. Environ. 90 (4), 434–440. doi:10.1016/j.rse.2004.02.003
Su, W., Chang, Q., Liu, X., and Zhang, L. (2021). Cooling effect of urban green and blue infrastructure: a systematic review of empirical evidence. Acta Ecol. Sin. 41 (07), 2902–2917. doi:10.5846/stxb201903290607
Sun, X., Tan, X. Y., Chen, K. L., Song, S., Zhu, X. D., and Hou, D. L. (2020). Quantifying landscape-metrics impacts on urban green-spaces and water-bodies cooling effect: the study of Nanjing, China. Urban For. Urban Green. 55, 126838. doi:10.1016/j.ufug.2020.126838
Sun, Z. C., Wang, C. Z., Guo, H. D., and Shang, R. R. (2017). A modified normalized difference impervious surface index (MNDISI) for automatic urban mapping from Landsat imagery. Remote Sens. 9 (9), 942. doi:10.3390/rs9090942
Thanvisitthpon, N., Nakburee, A., Khamchiangta, D., and Saguansap, V. (2023). Climate change-induced urban heat Island trend projection and land surface temperature: a case study of Thailand?s Bangkok metropolitan. Urban Clim. 49, 101484. doi:10.1016/j.uclim.2023.101484
Wang, S. Q., Sun, Q. C., Huang, X., Tao, Y. G., Dong, C. Y., Das, S., et al. (2023). Health-integrated heat risk assessment in Australian cities. Environ. Impact Assess. Rev. 102, 107176. doi:10.1016/j.eiar.2023.107176
Wilhelmi, O. V., and Hayden, M. H. (2010). Connecting people and place: a new framework for reducing urban vulnerability to extreme heat. Environ. Res. Lett. 5 (1), 014021. doi:10.1088/1748-9326/5/1/014021
Wu, J. R., Chen, X. L., and Lu, J. Z. (2022). Assessment of long and short-term flood risk using the multi-criteria analysis model with the AHP-Entropy method in Poyang Lake basin. Int. J. Disaster Risk Reduct. 75, 102968. doi:10.1016/j.ijdrr.2022.102968
Xiang, Y., Cen, Q. Y., Peng, C. C., Huang, C. B., Wu, C. G., Teng, M. J., et al. (2023). Surface urban heat island mitigation network construction utilizing source-sink theory and local climate zones. Build. Environ. 243, 110717. doi:10.1016/j.buildenv.2023.110717
Xiang, Y., Yuan, C., Cen, Q., Huang, C., Wu, C., Teng, M., et al. (2024). Heat risk assessment and response to green infrastructure based on local climate zones. Build. Environ. 248, 111040. doi:10.1016/j.buildenv.2023.111040
Xue, X. Y., He, T., Xu, L. C., Tong, C., Ye, Y., Liu, H. J., et al. (2022). Quantifying the spatial pattern of urban heat islands and the associated cooling effect of blue-green landscapes using multisource remote sensing data. Sci. Total Environ. 843, 156829. doi:10.1016/j.scitotenv.2022.156829
Yang, W., Shi, P., Zhang, G., and Yang, S. (2022). Study of population exposure to extreme heat and the prediction of disaster index in Xi'an. J. Nat. Disasters 31 (01), 29–39. doi:10.13577/j.jnd.2022.0103
Yao, L., Sun, S., Song, C., Wang, Y., and Xu, Y. (2022). Recognizing surface urban heat 'island' effect and its urbanization association in terms of intensity, footprint, and capacity: a case study with multi-dimensional analysis in Northern China. J. Clean. Prod. 372, 133720. doi:10.1016/j.jclepro.2022.133720
Yin, Z. E., Yin, J., and Zhang, X. W. (2013). Multi-scenario-based hazard analysis of high temperature extremes experienced in China during 1951-2010. J. Geogr. Sci. 23 (3), 436–446. doi:10.1007/s11442-013-1020-z
Zhang, Q., Zhou, D., Xu, D., Cheng, J. Y., and Rogora, A. (2022). Influencing factors of the thermal environment of urban green space. Heliyon 8 (11), e11559. doi:10.1016/j.heliyon.2022.e11559
Zhang, S., Zhang, C., Cai, W., Bai, Y., Callaghan, M., Chang, N., et al. (2023a). The 2023 China report of the Lancet Countdown on health and climate change: taking stock for athriving future. Lancet Public Health 8 (12), E978–E995. doi:10.1016/s2468-2667(23)00245-1
Zhang, W., Zheng, C. G., and Chen, F. (2019). Mapping heat-related health risks of elderly citizens in mountainous area: a case study of Chongqing, China. China. Sci. Total Environ. 663, 852–866. doi:10.1016/j.scitotenv.2019.01.240
Zhang, Y., Tian, N., Chen, A., Qiu, J., He, C., and Cao, Y. (2023b). Identification of a wetland ecological network for urban heat island effect mitigation in Changchun, China. Ecol. Indic. 150, 110248. doi:10.1016/j.ecolind.2023.110248
Zhao, L. Y., Li, T. T., Przybysz, A., Liu, H., Zhang, B. J., An, W. Y., et al. (2023). Effects of urban lakes and neighbouring green spaces on air temperature and humidity and seasonal variabilities. Sust. Cities Soc. 91, 104438. doi:10.1016/j.scs.2023.104438
Zhou, B., Rybski, D., and Kropp, J. P. (2017). The role of city size and urban form in the surface urban heat island. Sci. Rep. 7, 4791. doi:10.1038/s41598-017-04242-2
Zhuang, X., Duan, Y., and Jin, H. (2017). Research review on urban landscape micro-climate. Chin. Landsc. Archit. 33 (04), 23–28. doi:10.3969/j.issn.1000-6664.2017.04.005
Keywords: extreme heat and heat island effects, urban areas, risk assessment, energy flow, cooling network construction
Citation: Xu H, Yang J, Lin Y, Xu N, Li M, Xu Y, Liu X and Li F (2024) Construction of a composite cooling network for the mitigation of urban heat risk in Fuzhou. Front. Environ. Sci. 12:1462700. doi: 10.3389/fenvs.2024.1462700
Received: 10 July 2024; Accepted: 26 November 2024;
Published: 09 December 2024.
Edited by:
Farrukh Chishtie, University of British Columbia, CanadaReviewed by:
Amit Kumar, Purdue University, United StatesCopyright © 2024 Xu, Yang, Lin, Xu, Li, Xu, Liu and Li. This is an open-access article distributed under the terms of the Creative Commons Attribution License (CC BY). The use, distribution or reproduction in other forums is permitted, provided the original author(s) and the copyright owner(s) are credited and that the original publication in this journal is cited, in accordance with accepted academic practice. No use, distribution or reproduction is permitted which does not comply with these terms.
*Correspondence: Fangying Li, ZnlsaTIwMTFAMTI2LmNvbQ==
Disclaimer: All claims expressed in this article are solely those of the authors and do not necessarily represent those of their affiliated organizations, or those of the publisher, the editors and the reviewers. Any product that may be evaluated in this article or claim that may be made by its manufacturer is not guaranteed or endorsed by the publisher.
Research integrity at Frontiers
Learn more about the work of our research integrity team to safeguard the quality of each article we publish.