- 1Natural Resources Survey Institute of Heilongjiang Province, Harbin, China
- 2Economics and Management College, China University of Geosciences, Wuhan, China
As a comprehensive and systematic approach to innovation, green innovation has become an important tool for reducing haze and carbon intensity. Based on balanced panel data at the city level in China, we use the two-way fixed-effects model to explore the impact of urban green innovation on haze pollution and carbon emission intensity. The conclusions are as follows: 1) The influence of green innovation on carbon emissions intensity and haze pollution exhibits a significant inverted U-shaped relationship. 2) We explore the mediating channels through which green innovation affects carbon emissions intensity and haze pollution. Specifically, the inverted U-shaped impacts of green innovation on energy intensity and resource dependence. 3) The inverted U-shaped relationship between green innovation and carbon emissions intensity holds for both resource-based and non-resource-based cities. 4) The U-shaped relationships, whether regarding carbon emissions intensity or haze pollution, are only applicable to non-environmental priority cities. This paper offers valuable insights for the formulation of more effective environmental management measures by governments.
1 Introduction
With the vigorous development of Chinese economy, the traditional extensive economic growth model has posed unprecedented challenges to sustainable economic development (Guang et al., 2023; Tang et al., 2023; Zor, 2023). Carbon emissions and haze pollution are two closely related and dual-threat environmental issues in China (Dong et al., 2023; Gong et al., 2024). As the largest carbon-emitting countries, China’s industrial production, energy consumption, and transportation activities contribute significantly to global climate change (Xia W. et al., 2022; Tian et al., 2023). Simultaneously, several major cities and industrial centers in China are grappling with severe haze pollution, negatively impacting public health and urban image (Sun et al., 2019). The introduction of the “Dual Carbon” target has propelled China’s environmental governance to new heights (Fan et al., 2022; Huang et al., 2022). Consequently, China faces immense pressure to reduce haze and carbon emissions while pursuing high-quality economic development. In this context, carbon-haze synergy governance has emerged as a key strategy for addressing air pollution and climate change in China (Yuan et al., 2022). Through such governance, improvements in residents' living environments and enhanced wellbeing can be achieved, while simultaneously contributing to global climate change mitigation efforts. With the introduction of the concept of ecological civilization construction, achieving a transformation towards green economic development has become a widespread consensus among various sectors and levels of government (Yang et al., 2022; Wu et al., 2024a). Green innovation provides a crucial entry point and avenue for addressing carbon-haze issues (Tang et al., 2024a; Zhang et al., 2024).
Green innovation, characterized by environmentally friendly and sustainable-oriented innovative activities, offers a novel approach to carbon-haze synergy governance (Feng et al., 2023; Liu et al., 2024). Emphasizing not only economic benefits but also environmental conservation and social sustainability, green innovation plays a pivotal role in mitigating haze and reducing carbon emissions (Liu and Dong, 2021). Firstly, green innovation fosters technological advancements and breakthroughs, thereby promoting the research and application of clean energy technologies (Peng et al., 2021; Xin et al., 2021). For example, the development and dissemination of renewable energy technologies has reduced society’s dependence on fossil fuels, thereby reducing carbon emissions and haze pollution. Secondly, green innovation drives the transformation of industrial structures and social production patterns, thereby advancing the development of green industries. The promotion of circular economy and intelligent manufacturing in green industries enables efficient resource utilization and minimizes waste generation, leading to reduced environmental pollution (Dogan and Ozturk, 2017; Usman et al., 2021). Additionally, green innovation facilitates the implementation of circular economy models, promoting waste recycling and reducing solid waste emissions, fundamentally alleviating the burden on environmental governance (Jin et al., 2019; Albitar et al., 2023a). As such, integrating green innovation into carbon-haze synergy governance strategies provides essential support and leadership for sustainable development and environmental protection (Ostadzad, 2022; Qureshi et al., 2022).
The pivotal role of technological progress in energy conservation and emission reduction has achieved widespread consensus. While green innovation plays multifaceted roles in carbon-haze synergy governance, uncertainties persist regarding its relationship with environmental performance (Sharif et al., 2022). Particularly, attention must be directed towards the “pollution rebound effect,” which has been known to arise from green innovation (Chang et al., 2018; Prest and Krupnick, 2021; Xia H. et al., 2022). In the short term, green innovation might not yield immediate improvements in environmental performance; rather, it may lead to temporary environmental deterioration. As exemplified by the “pollution rebound effect,” the introduction of green innovation often supplants high-pollution technologies. However, during the process of technology dissemination and application, new environmental challenges may emerge. For instance, certain green technologies may entail significant resource consumption during production, thereby increasing energy demands and potentially exacerbating environmental pollution. Consequently, from a short-term perspective, green innovation does not guarantee immediate enhancements in environmental performance and may even trigger adverse environmental consequences. Moreover, the environmental effects of green innovation may exhibit heterogeneity across different regions due to variations in policy support and market demands (Lei et al., 2023). Recent empirical research also suggests that green innovation might not promptly improve environmental performance (Mongo et al., 2021).
This paper may have the following contributions: Firstly, while previous research has primarily focused on exploring the driving factors of urban green innovation (e.g., Li et al., 2022; Feng et al., 2023; Qiu et al., 2023), limited attention has been paid to the consequences of green innovation activities. Our study emphasizes the investigation of the environmental performance of urban green innovation, thus enhancing empirical research on the effects of green innovation. Consequently, this paper unravels the puzzle of the environmental effects of urban green innovation. Secondly, we identify the nonlinear impact of green innovation on carbon emissions intensity and haze pollution. This not only contributes to the scholarly discussion on energy rebound effects but also provides important insights for the Chinese government in implementing policies for coordinated carbon and haze control. Lastly, environmental economics literature has often examined haze pollution or carbon emissions separately (e.g., Yi et al., 2020; Chen et al., 2023), thus neglecting the interconnectedness between the two. By integrating them into a unified analytical framework, this paper addresses the gap in the literature by bridging the disconnect between haze pollution and carbon emissions. Consequently, it enhances the comprehensive understanding of the coordinated governance of carbon and haze among researchers and provides a scientific basis for achieving sustainable development and improving environmental quality.
The remainder of the paper is organised as follows: Section 2 is institutional background and literature review; Section 3 outlines the research design; Section 4 provides the empirical results; Section 5 provides mechanism analysis and heterogeneity analysis; and Section 6 summarises the findings and implications.
2 Institutional background and literature review
2.1 Collaborative management of carbon and haze in China
Currently, China is confronted with the dual challenge of mitigating atmospheric pollution and addressing climate change. Given that fossil fuel consumption serves as the primary source of both air pollutants and greenhouse gas emissions, striking a balance between pollution reduction and carbon emission reduction is imperative (Zhang et al., 2023). While the Blue Sky Defense Campaign has yielded significant achievements, disparities persist with regard to the objectives of constructing a Beautiful China and meeting public expectations. Consequently, synergistically advancing atmospheric pollution control and climate change mitigation not only pertains to the sustained amelioration of environmental quality, but also stands as a pivotal driver for facilitating a comprehensive green transformation across economic and social domains (Zhang and Yang, 2024). Against this backdrop, the Chinese government must navigate efficacious pathways for the continual enhancement of air quality to foster harmonious coexistence among economic, social, and environmental dimensions.
In 2016, China revised the new “Law on the Prevention and Control of Atmospheric Pollution,” marking the first inclusion of “coordinated control of atmospheric pollutants and greenhouse gases” within its legal framework1. This initiative places the control of greenhouse gas emissions on a legal track, underscoring the government’s profound concern for atmospheric pollution and climate change. By incorporating greenhouse gas emissions into the legal framework, the government has established the crucial role of carbon reduction at the legal level, providing robust legal support for emissions reduction efforts across society. Meanwhile, the release of the “13th Five-Year Plan for Controlling Greenhouse Gas Emissions” also underscores the importance of strengthening coordinated control of carbon emissions and atmospheric pollutants2, further highlighting the government’s determination and actions in pollution reduction and carbon mitigation. The release of this plan provides clear guiding ideology and specific objectives for further enhancing the synergistic effects of pollution reduction and carbon mitigation. The enactment of these laws and policies signifies China’s adoption of more systematic and comprehensive governance measures for addressing both atmospheric pollution and climate change issues (Wang H. et al., 2023).
In China, the coordinated governance of carbon emissions and haze pollution has emerged as a crucial component of the national strategy. Although the government has strengthened regulation and enforcement, as well as increased penalties for environmental violations to ensure effective implementation of environmental policies (Jiang and Tang, 2023). However, China still faces several challenges in the coordinated governance of carbon and haze. Firstly, due to the rapid industrialization and urbanization processes, carbon emissions remain high, rendering carbon reduction targets difficult to achieve. Secondly, protectionist tendencies among local governments result in some regions displaying weak enforcement of environmental protection policies, with instances of superficial governance and a tendency towards one-size-fits-all approaches (Wang Z. et al., 2023). Moreover, certain enterprises exhibit a lack of environmental awareness, engaging in behaviors such as clandestine discharge and illegal pollution, thereby exacerbating the challenges of governance (Wu et al., 2024b). Hence, China necessitates further policy coordination and interdepartmental cooperation in the governance of carbon and haze, alongside strengthened environmental law enforcement, to realize the dual governance objectives of carbon emissions and haze pollution.
2.2 Literature review
Green innovation is an effective means to achieve the dual goals of economic growth and environmental protection, thereby garnering significant attention from the academic community in recent years. With regard to the consequences of green innovation, academic debate has centred on the economic and environmental effects of green innovation.
2.2.1 The economic effect of green innovation
The economic effect of green innovation has been the subject of extensive scholarly inquiry. However, no consistent conclusions have emerged from the existing literature. The “compliance cost hypothesis” posits that achieving simultaneous economic performance and environmental preservation is inherently challenging (Khattak et al., 2022; Albitar et al., 2023b). This perspective considers the low return rate, lengthy return cycle, and substantial investment associated with green innovation, as well as the common observation that local governments often respond passively to environmental regulations imposed by higher-level governmental entities (Puertas and Marti, 2021). In resource-constrained contexts, the implementation of green innovation necessitates the reallocation of existing resources by local governments, diverting a portion of them from traditional production toward environmental protection endeavors. This reallocation disrupts the original production deployment within a city, restricts industrial production activities, and consequently has a “crowding out effect” on economic performance. As a result, the allocation of resources by local governments toward environmental protection ultimately displaces productive investments in the city, thereby impeding improvements in economic performance (Chien et al., 2023).
In contrast, the “Porter Hypothesis” presents a divergent perspective from the “compliance cost hypothesis” (Porter and Van der Linde, 1995). According to the “Porter Hypothesis,” green innovation has the potential to optimize resource allocation efficiency, leading to a positive effect on economic performance. In response to mounting pressures on resources and the environment, governments can adopt proactive strategies to promote green innovation and enhance the overall competitiveness of cities. Green innovation serves as an effective solution to foster clean production and improve production efficiency, further enhancing the economic sustainability of cities (Yan et al., 2020). Moreover, the adoption of environmentally friendly technologies, process optimization, and product design improvements associated with green innovation significantly reduce production costs for society. Additionally, green industries represent the future direction of development, generating substantial employment opportunities and driving the economic advancement of cities. Cities that prioritize green innovation often enjoy a distinct advantage in attracting talent and investments, leading to considerable benefits for enhancing economic performance. In this context, engagement in green innovation activities does not entail a trade-off between economic performance and environmental preservation; instead, it can foster a win-win situation for both the environment and the economy (Huang et al., 2021; Zhao et al., 2022).
2.2.2 The environmental effect of green innovation
The academic community has conducted extensive research on the environmental performance of green innovation, demonstrating its significance in achieving sustainable development. Green innovation is recognized as a fundamental strategy for promoting high-quality development and is regarded as a key pathway towards realizing the concept of sustainable development in the global context (Yildirim et al., 2022). By adopting green innovation, societies can strive for a “win-win” scenario where economic growth is coupled with environmental protection. In particular, green innovation plays a critical role in addressing the dual challenges of carbon emissions and environmental degradation. It provides a transformative approach that enables economies to move away from traditional models of development that heavily rely on resource-intensive and polluting practices. Instead, green innovation promotes the adoption of sustainable and low-carbon technologies, processes, and practices. By doing so, green innovation not only mitigates environmental impacts but also fosters the efficient utilization of resources.
Green innovation has become an essential pathway for overcoming the limitations imposed by resource scarcity and environmental constraints. It offers innovative solutions to promote sustainable development by reducing ecological footprints, enhancing resource efficiency, and minimizing environmental pollution. Embracing green innovation is imperative for societies to transition towards more sustainable and resilient economies, as well as to meet global environmental targets and commitments. While green innovation has been shown to contribute to clean production, carbon emissions reduction, and the mitigation of global climate change (Xin et al., 2021; Sharif et al., 2022), it also plays a crucial role in promoting the efficient utilization of energy resources, thereby reducing resource consumption and environmental pollution (Ulucak and Baloch, 2023). Nevertheless, it is noteworthy that the environmental performance of green innovations is not always assured (Du and Li, 2019), and in some cases, low-quality or insufficient green innovations may even contribute to environmental pollution (Mongo et al., 2021).
Figure 1 represents the concise research framework. The primary focus of this research centers on examining the influence of green innovation on carbon emissions intensity and haze pollution. Building upon this foundation, we conduct an investigation from the perspectives of environmental performance and energy efficiency to elucidate the underlying mechanisms through which green innovation interacts with carbon intensity and haze pollution. Finally, we explore the heterogeneity of the baseline regression analysis based on urban categorizations, such as resource-based cities and key environmental cities. Our research aims to explore to the environmental-related outcomes green innovation, thus providing valuable insights for promoting sustainable development.
3 Research design
3.1 Sample selection and data source
In response to the increasingly severe phenomenon of haze-induced city lockdowns and the substantial emissions of greenhouse gases, the significance of ecological civilization construction was elevated to a prominent position in national governance during the 2012 18th National Congress of the Communist Party. Consequently, this study adopts 2012 as the starting year. The urban green patent application data are sourced from the CNRDS database. PM2.5 data are obtained from the Atmospheric Composition Group at Dalhousie University. The data for calculating carbon emissions are sourced from the “China Urban Statistical Yearbook,” the “China Energy Statistical Yearbook,” as well as provincial and municipal statistical yearbooks. As for the control variables, environmental regulations are derived from textual analysis of city government work reports. Other control variables are obtained from the EPS database. Ultimately, the authors compile a balanced panel dataset encompassing 280 prefecture-level and higher-level cities in China for the period 2012-2018.
3.2 Variable definitions
3.2.1 Dependent variables
Carbon emissions intensity (Carbon). The carbon emissions in cities primarily originate from direct carbon emissions resulting from energy consumption, including liquefied petroleum gas, coal, and others, as well as indirect carbon emissions resulting from energy consumption, such as electricity and heat. Following the approach by Wu and Guo (2016), this study multiplies the energy consumption of various types in cities by their corresponding carbon emission coefficients and aggregates them to obtain the annual carbon emissions. Finally, we utilize the proportion of total urban carbon emissions to regional GDP as one of the dependent variables.
Haze pollution (Pm25). The primary source of Haze pollution is PM2.5, and similar to existing literature, we employ PM2.5 concentration as a measure of Haze pollution. PM2.5 concentration is sourced from Dalhousie University. This dataset consists of latitude and longitude grid data, which we further analyze using ArcGIS software to obtain annual average PM2.5 concentrations for each city.
3.2.2 Independent variable
Urban green innovation (Gi). Patents are widely recognized as a significant metric for assessing technological innovation. In the context of urban green innovation, it is common practice in academia to employ the count of green patents as an indicator. The number of green patents represents an objective measure that can provide insights into the overall level of a city’s green innovation. Green patents capture the innovative efforts and activities specifically focused on environmentally friendly technologies and solutions. By targeting patents related to green inventions and utility models, this approach directly reflects the degree of emphasis placed on environmentally sustainable practices within the urban context. Moreover, green patent applications consider not only the patents that have been granted but also those in the application stage, offering a more holistic perspective on the evolving landscape of green innovation. Referring to previous studies (e.g., Li et al., 2022), we use the natural logarithm of green patent applications to represent urban green innovation.
3.2.3 Control variables
Meanwhile, this study incorporates several control variables in the regression model, as suggested by previous research (e.g., Singh et al., 2020; Yi et al., 2020). The control variables include economic development (Pgdp), which is measured using per capita GDP as an indicator of regional economic development. As higher economic growth can stimulate the advancement of energy-saving and emission reduction technologies. Foreign trade (Fdir), measured as the proportion of actual foreign investment to GDP. Foreign trade often brings about technology spillover effects and economies of scale, which typically contribute to the reduction of environmental pollution. Education expenditure (Edur) is measured by the proportion of urban education investment to GDP. Population density (Pden) is accounted for by employing the proportion of total population to administrative area, serving as a proxy for population density. Science expenditure (Scir) is included as the ratio of urban science expenditure to GDP. Industrial structure (Second) is captured by the proportion of value added by the secondary sector to GDP, which provides insights into the composition of the regional economy. To account for the influence of environmental regulation, the ratio of environmental-related vocabulary in the urban government’s work report is employed as a proxy for environmental regulation (Er), following the approach of Chen et al. (2018).
3.3 Research model
To examine the influence of green innovation on carbon emissions intensity and haze pollution, the following two-way fixed effects model is constructed:
In Eq. 1, where Yit represents carbon emissions intensity and haze pollution of city i in year t; Giit indicates urban green innovation; Controlit denotes a set of urban characteristics; μi and νt represent the city and year fixed effects, respectively; εit is the error term. α is a constant term; β1 and β2 are the coefficients.
To test whether the energy efficiency channel and environmental performance channel hold, referring to Baron (2022), we design the following mediating effects model:
In Eq 2, Mechit refers to mechanisms, i.e., energy intensity and environmental performance.
4 Empirical results
4.1 Descriptive statistics
As exhibited in Table 1. The mean value of carbon emissions intensity (Carbon) is greater than its median, indicating that the data are generally right skewed. The mean value of haze pollution (Pm25) is slightly greater than the median value, and the extreme difference is relatively large, indicating significant variations in air pollution levels across different cities. The mean of green innovation (Gi) is very close to its median, with a small standard deviation, indicating that the city’s green innovation approximately obeys a positively distributed.
4.2 The spatial and temporal patterns of green innovation, carbon intensity and haze pollution
To visually display the spatiotemporal distribution of urban green innovation in Chinese cities, we utilize ArcGIS software to create spatiotemporal distribution maps (The foundational map utilized in this study originates from the Standard Map Service System administered by the Ministry of Natural Resources, with an assigned audit number of GS (2019)1822). As depicted in Figure 2, this study showcases the spatial distribution of urban green innovation for the years 2008, 2011, 2015, and 2018, respectively. Between 2012 and 2018, there was relatively little fluctuation in the green innovation levels across cities. Broadly speaking, cities exhibiting strong green innovation capabilities are predominantly concentrated in the eastern regions of China, while those with weaker green innovation capacities are mainly clustered in the northeastern and northwestern regions. It is not difficult to comprehend that the eastern provinces of China boast more advanced economic development, leading to increased allocation of resources and funds towards green innovation endeavors. Additionally, governments in the eastern provinces typically possess greater capacity and willingness to formulate and enforce policies conducive to promoting green innovation, thereby fostering an enabling environment for its development.
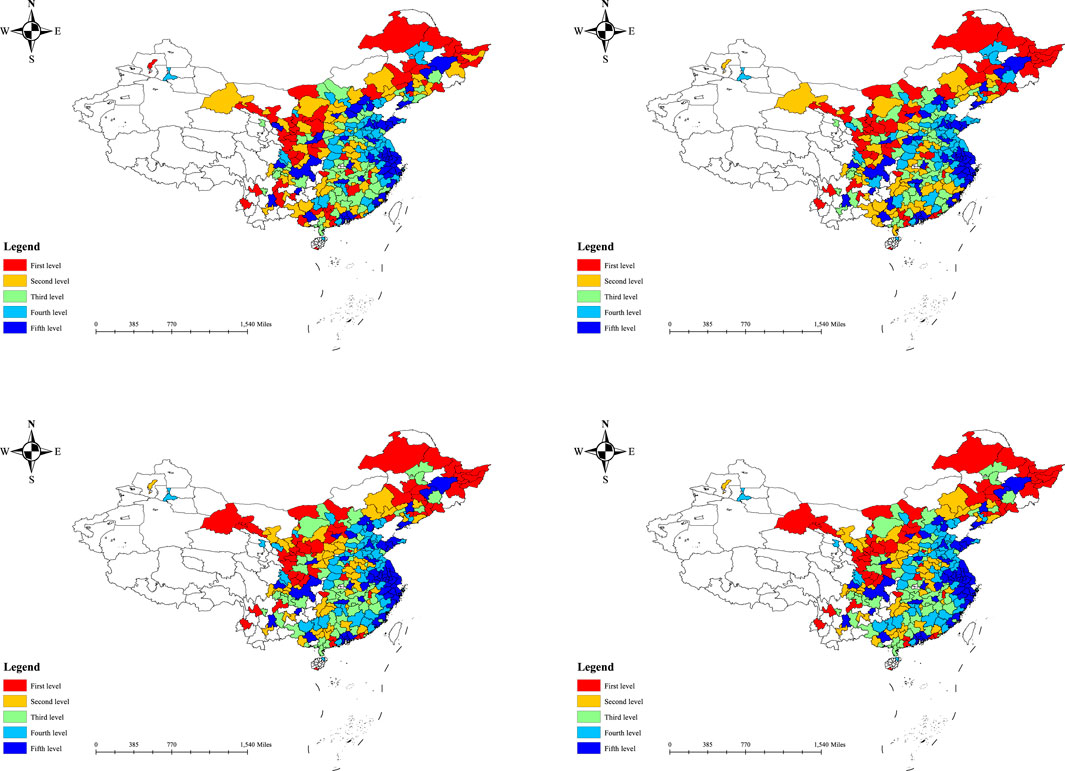
Figure 2. The spatial and temporal patterns of urban green innovation. Note: The representative years, from left to right and top to bottom, respectively, are 2012, 2014, 2016, and 2018.
Similarly, as depicted in Figure 3, our paper showcases the spatial distribution of carbon emissions intensity for the years 2008, 2011, 2015, and 2018, respectively. In general, cities with higher carbon emission intensities are predominantly concentrated in China’s northeastern region, the Beijing-Tianjin-Hebei region, and the northwestern region. This phenomenon can be attributed to the fact that the northeastern and Beijing-Tianjin-Hebei regions of China are industrialized areas, dominated by traditional high-energy-consumption industries such as coal, steel, and chemicals. These regions possess a plethora of heavy industries and energy production facilities, which typically emit substantial amounts of carbon. Meanwhile, the northwestern region primarily relies on traditional fossil fuels such as coal, exhibiting a relatively irrational energy structure.
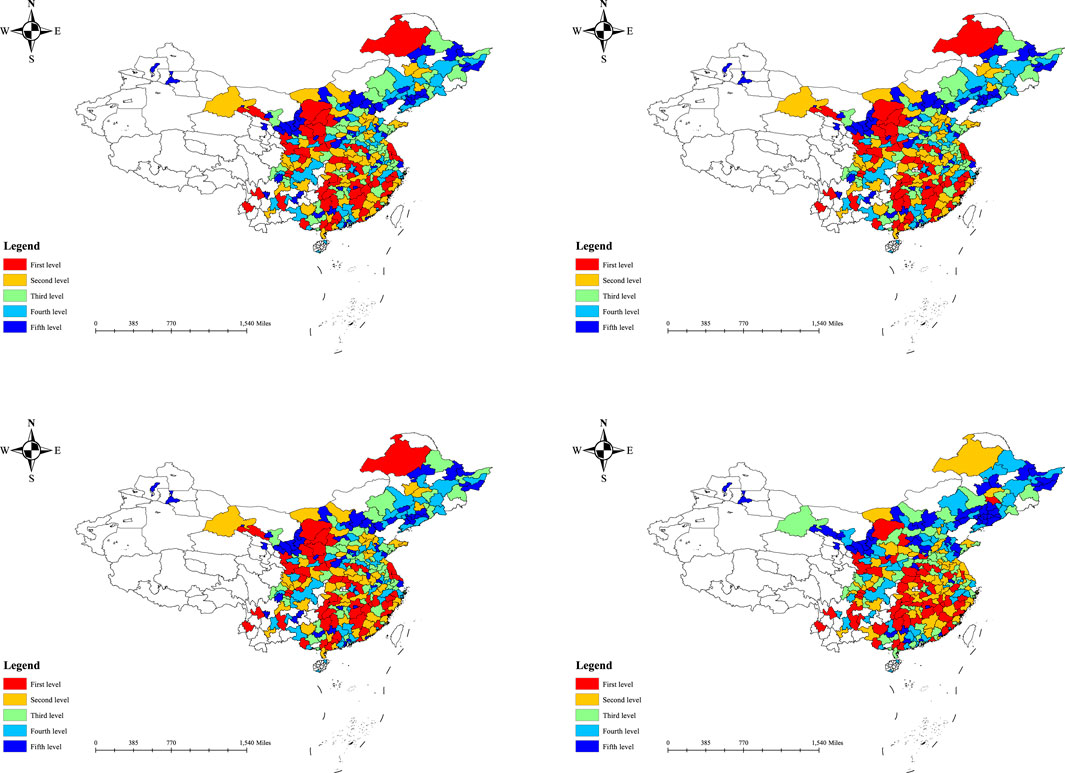
Figure 3. The spatial and temporal patterns of carbon emissions intensity. Note: The representative years, from left to right and top to bottom, respectively, are 2012, 2014, 2016, and 2018.
As depicted in Figure 4, our study showcases the spatial distribution of haze pollution for the years 2008, 2011, 2015, and 2018, respectively. It is evident that regions with severe smog pollution are highly concentrated, primarily in the northern provinces of China, particularly in the Beijing-Tianjin-Hebei region, Henan Province, Anhui Province, and the Shandong Peninsula. This outcome may be attributed to the urban traffic congestion and the presence of developed heavy industries in northern China.
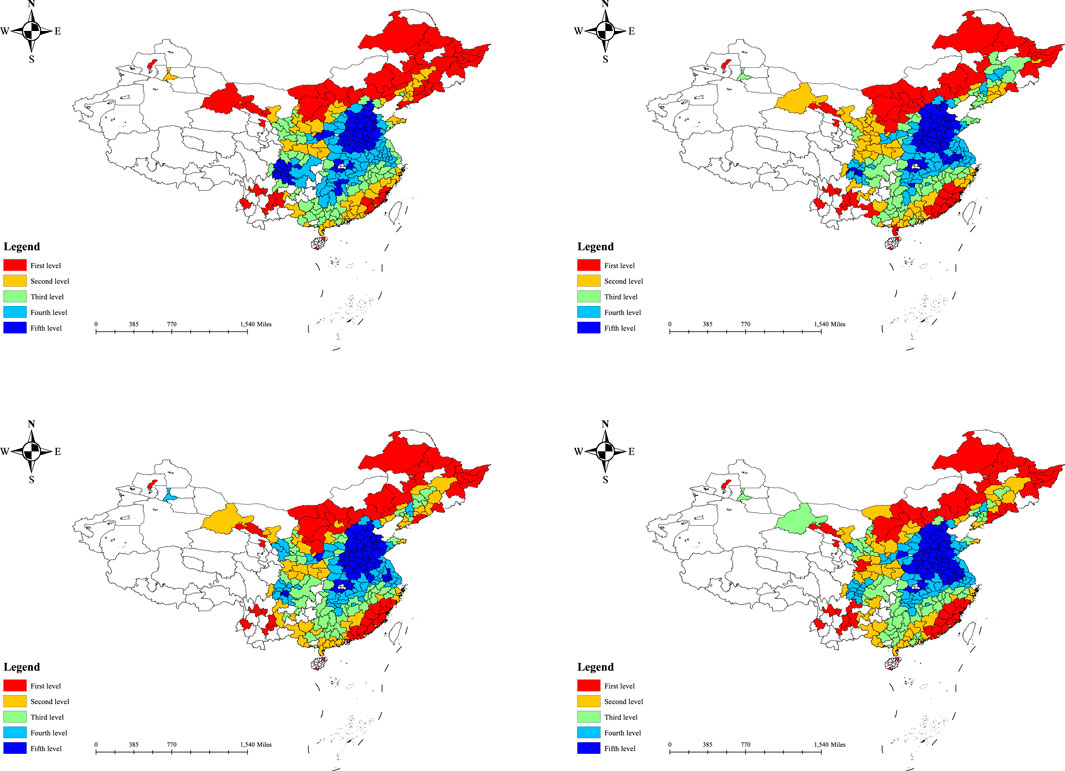
Figure 4. The spatial and temporal patterns of haze pollution. Note: The representative years, from left to right and top to bottom, respectively, are 2012, 2014, 2016, and 2018.
4.3 Baseline results
Table 2 presents the baseline regression results, the dependent variables in columns (1)–(2) represent carbon emissions intensity (Carbon), while columns (3)–(4) represent haze pollution (PM25). When we do not consider any control variables, the coefficients of Gi*Gi for both carbon emissions intensity and haze pollution are significantly negative. This indicates an inverted U-shaped relationship between green innovation and both carbon intensity and haze pollution. Similarly, columns (2) and (4) demonstrate the results when we consider all control variables. The U-shaped relationship still holds. Thus, our study confirms the pollution rebound effect in the Chinese context, which not only contributes to promoting coordinated governance of carbon and haze, but also provides valuable insights for addressing other types of pollutants.
4.4 Robustness checks
Additionally, this study conducts a battery of robustness checks. Firstly, the authors substitute the independent variable with the number of urban green patent grants, as shown in column (1) of Tables 3, 4. Despite controlling for various regional-level control variables, there is still a possibility of other regional factors biasing our findings. To address this concern, following the methodology of Yuan and Zhang (2015), province-year interaction fixed effects are incorporated into the model to account for time-varying and non-time-varying province characteristics. The outcomes are presented in column (2) of Tables 3, 4.
Moreover, it is important to highlight the unique administrative status of Beijing, Shanghai, Tianjin, and Chongqing within China. These cities enjoy a special designation as they are under direct governance by the central government. With their elevated administrative level, they receive heightened attention and priority in policy implementation. Given these distinctive characteristics, it is necessary to account for their potential influence on the research findings. Therefore, we exclude these four municipalities to ensure a more focused analysis that represents the majority of cities. The regression analysis is rerun based on the established baseline regression model, the results are exhibited in column (3) of Table 3 and Table 4.
5 Further analysis
5.1 Nonlinear mediating effect
This study further investigates the potential channels through which green innovation influences carbon emissions intensity and haze pollution.
5.1.1 Energy efficiency channel
Similar to Guang et al. (2019), we use single factor energy efficiency, i.e., urban energy intensity, as a mediating variable3. Based on the results in column (1) of Table 5, we observe that the coefficient of Gi*Gi is significantly negative. This indicates that when the green innovation level is low, it contributes to increased energy consumption. However, once the green innovation level reaches a turning point, it becomes conducive to reducing energy consumption. Therefore, energy intensity plays a mediating role in the relationship between green innovation and both carbon emissions intensity and haze pollution. This finding provides crucial insights for a deeper understanding of the effect of green innovation on energy consumption, and offers valuable implications for formulating environmental policies and promoting sustainable development.
5.1.2 Environmental performance channel
To verify whether environmental performance mediates the carbon haze synergy management process of green innovation. Similar to Tang et al. (2024b), this paper synthesizes urban environmental performance based on their three waste emissions using the entropy value method4. We examine environmental performance as the dependent variable, and the empirical results, as shown in column (2) of Table 5, reveal the following observations. The coefficient of Gi is 0.009 and is significantly positive. Additionally, the coefficient of Gi*Gi is −0.001 and is significantly negative. These findings indicate that when the green innovation level is low, green innovation exacerbates environmental issues. However, as the green innovation level reaches a certain threshold, it becomes beneficial in reducing environmental pollution and improving environmental performance. Hence, environmental performance plays a mediating effect in the carbon-haze synergistic governance effects of green innovation.
5.2 Heterogeneity analysis
5.2.1 Resource-based vs non-resource-based cities
The presence of favorable natural resource endowment confers a significant advantage on resource-based cities for achieving sustainable development, serving as the fundamental characteristic that distinguishes them from non-resource-based cities. The availability of abundant energy resources, such as oil, coal, and metal minerals, provides resource-based cities with a solid foundation for economic growth. These cities capitalize on their natural resource endowments by heavily engaging in the development and processing industries associated with these resources. In contrast, non-resource-based cities prioritize the development of alternative industries, such as manufacturing and services, as opposed to natural resource extraction. They seek to diversify their economic activities and reduce their dependence on resource-centric sectors. Consequently, the industrial structure and economic development paths of non-resource-based cities significantly differ from those that rely on natural resource exploitation. This differentiation stems from the varying reliance on natural resource exploitation versus alternative industries, shaping the overall economic landscape and trajectory of each city category. Consequently, it is pertinent to inquire whether the impact of green innovation on carbon emissions intensity and haze pollution differs substantially between resource-based and non-resource-based cities.
Our sample population is classified into two distinct categories: resource-based and non-resource-based cities5. The empirical findings, as presented in Table 6, reveal interesting patterns across different city types. Columns (1) and (3) represent resource-based cities, while columns (2) and (4) represent non-resource-based cities. The results indicate that the inverted U-shaped relationship between green innovation and carbon emissions intensity holds in non-resource-based cities, while it does not hold in resource-based cities. Conversely, the inverted U-shaped relationship between green innovation and haze pollution holds in resource-based cities, while it does not hold in non-resource-based cities. These research findings provide valuable insights for the differentiated governance strategies in carbon and haze synergistic governance between resource-based and non-resource-based cities.
5.2.2 Key environmental vs non-key environmental cities
China currently faces an increasingly acute contradiction between its economic development goals and the constraints imposed by resource availability and environmental concerns. The protection of the environment, in particular, confronts significant challenges. In response to this pressing issue, the State Council officially released the “11th Five-Year Plan for National Environmental Protection” in 2 November0076. This plan provides clear guidance for 113 key environmental protection cities, with comprehensive air pollution control being a critical task for these cities. The objective is to improve the quality of urban and regional air environments. Designated by the central government as priority areas for governance, these key cities face severe environmental problems that require targeted environmental management measures. Typically located in economically developed regions or environmentally sensitive areas, these key cities exhibit relatively higher levels of environmental pollution compared to non-designated cities. Moreover, many of these key cities are medium to large-sized urban areas with strong capabilities for green innovation.
The specific results are presented in Table 7, where columns (1) and (3) represent key environmental protection cities, while columns (2) and (4) represent non-key environmental protection cities. We observe that, when carbon emissions intensity is the dependent variable, the impact of green innovation in non-key environmental protection cities exhibits an inverted U-shaped relationship. Similarly, the inverted U-shaped relationship between green innovation and haze pollution is only evident in non-key environmental protection cities. These findings indicate significant variations in the influence of green innovation across different types of cities. These disparities may stem from differences between key environmental protection and non-key environmental protection cities in terms of environmental governance measures, industrial structure, and policy support, among other factors. Consequently, our study provides crucial insights for further investigating and elucidating the influence of city typology on the effectiveness of green innovation.
6 Conclusion and implications
With the intensification of global climate change and environmental issues, reducing carbon emissions and controlling haze pollution have become urgent tasks worldwide. In this context, green innovation, as a new approach to innovation, has emerged as a crucial means of synergistically addressing carbon and haze governance. Our research discusses the impact of green innovation on carbon emissions intensity and haze pollution using city-level data from China for the period 2012-2018. The main findings are as follows: (1) When the green innovation level in cities has not yet reached a critical threshold, it promotes both carbon emissions intensity and haze pollution. However, once the green innovation level surpasses the critical threshold, it inhibits carbon emissions intensity and haze pollution. (2) Energy intensity and environmental performance serve as mediating channels through which green innovation influences carbon emissions intensity and haze pollution. (3) The inverted U-shaped relationship between green innovation and carbon emissions intensity holds for both resource-based and non-resource-based cities. (4) The U-shaped relationship between green innovation and carbon emissions intensity and haze pollution is only observed in non-environmental priority cities, while it does not hold in environmental priority cities. The research findings of this study carry important implications for the formulation of targeted environmental policies and strategies for sustainable development.
Based on the aforementioned findings, we propose: First, it is recommended that the government strengthen the formulation and implementation of policies and regulations related to green innovation, providing clear policy guidance and market standards for businesses. Additionally, establishing robust market mechanisms such as carbon emission trading markets and green finance systems can incentivize companies to increase their investment and implementation of green innovation, thereby achieving the desired outcomes of reducing carbon emissions and haze pollution. Second, the government should develop and promote energy transition policies that encourage the use of clean energy and high-efficiency energy technologies to reduce energy consumption and lower carbon emissions intensity. Concurrently, enhancing environmental management and supervision while establishing a sound environmental management system can ensure that polluting enterprises adhere to environmental regulations and standards in their production and operations. Third, local governments need to adopt differentiated environmental governance measures for different types of cities. For example, resource-based cities require measures that strengthen environmental management and supervision while promoting the development of low-carbon industries. As for key environmental cities, the influence of green innovation on carbon emissions intensity and haze pollution is not significant. Therefore, while focusing on enhancing the level of green innovation, environmental priority cities should also pay attention to other types of mandatory environmental governance measures to directly improve environmental quality.
Despite the efforts made by the authors, this paper still has some limitations. Firstly, the spatial effects of green innovation have not been considered, providing a new research avenue for future studies in environmental economics. Building on a solid theoretical foundation, future research could utilize spatial econometric models to investigate the environmental performance of green innovation. Secondly, this study focuses only on the city-level analysis. As data availability improves, future research could utilize finer-grained county-level data to conduct more detailed analyses. County-level data can provide more precise and detailed information, thus revealing the variations and characteristics among cities to a greater extent. Lastly, this paper does not explicitly differentiate the environmental effects of different types of green innovation. Future research could further categorize green innovation into multiple types (e.g., Xu et al., 2021) and explore the environmental effects and underlying mechanisms of different types of green innovation. This would provide policymakers and businesses with more targeted guidance to achieve more effective environmental protection and sustainable development goals.
Data availability statement
The raw data supporting the conclusions of this article will be made available by the authors, without undue reservation.
Author contributions
WL: Conceptualization, Funding acquisition, Investigation, Resources, Writing–original draft, Writing–review and editing. CW: Investigation, Methodology, Software, Writing–original draft, Writing–review and editing. DD: Conceptualization, Investigation, Software, Writing–original draft. QL: Investigation, Writing–original draft, Writing–review and editing. RT: Investigation, Supervision, Writing–review and editing. TM: Investigation, Supervision, Writing–review and editing.
Funding
The author(s) declare that no financial support was received for the research, authorship, and/or publication of this article.
Conflict of interest
The authors declare that the research was conducted in the absence of any commercial or financial relationships that could be construed as a potential conflict of interest.
Publisher’s note
All claims expressed in this article are solely those of the authors and do not necessarily represent those of their affiliated organizations, or those of the publisher, the editors and the reviewers. Any product that may be evaluated in this article, or claim that may be made by its manufacturer, is not guaranteed or endorsed by the publisher.
Footnotes
1More details can be found at the following link: https://www.mee.gov.cn/ywgz/fgbz/fl/201811/t20181113_673567.shtml
2More details can be found at the following link: https://www.gov.cn/zhengce/content/2016-11/04/content_5128619.htm
3The total energy consumption is calculated by converting the annual electricity consumption (in ten thousand kilowatt-hours), liquefied petroleum gas consumption (in tons), and natural gas consumption (in ten thousand cubic meters) into the unit of “ten thousand metric tons of standard coal,” and then summing them up. In this study, the energy intensity is denoted as “energy consumption/GDP.”
4In this study, to assess the environmental performance of cities, the authors selected industrial wastewater discharge, industrial sulfur dioxide emissions, and industrial particulate matter emissions as the fundamental indicators at the city level. These indicators were then aggregated using the entropy method to calculate the City Environmental Performance Index. A higher index value indicates a more severe environmental pollution, reflecting lower environmental performance. Conversely, a lower index value indicates lower environmental pollution and higher environmental performance.
5The official document is derived from: https://www.gov.cn/zwgk/2013-12/03/content_2540070.htm
6The official document is derived from: https://www.mee.gov.cn/zcwj/gwywj/201811/t20181129_676435.shtml
References
Albitar, K., Al-Shaer, H., and Liu, Y. S. (2023a). Corporate commitment to climate change: the effect of eco-innovation and climate governance. Res. Policy 52 (2), 104697. doi:10.1016/j.respol.2022.104697
Albitar, K., Borgi, H., Khan, M., and Zahra, A. (2023b). Business environmental innovation and CO2 emissions: the moderating role of environmental governance. Bus. Strategy Environ. 32 (4), 1996–2007. doi:10.1002/bse.3232
Baron, E. J. (2022). School spending and student outcomes: evidence from revenue limit elections in Wisconsin. Am. Econ. Journal-Economic Policy 14 (1), 1–39. doi:10.1257/pol.20200226
Chang, J. J., Wang, W. N., and Shieh, J. Y. (2018). Environmental rebounds/backfires: macroeconomic implications for the promotion of environmentally-friendly products. J. Environ. Econ. Manag. 88, 35–68. doi:10.1016/j.jeem.2017.09.004
Chen, H., Yi, J., Chen, A., Peng, D., and Yang, J. (2023). Green technology innovation and CO2 emission in China: evidence from a spatial-temporal analysis and a nonlinear spatial durbin model. Energy Policy 172, 113338. doi:10.1016/j.enpol.2022.113338
Chen, Z., Kahn, M. E., Liu, Y., and Wang, Z. (2018). The consequences of spatially differentiated water pollution regulation in China. J. Environ. Econ. Manag. 88, 468–485. doi:10.1016/j.jeem.2018.01.010
Chien, F., Paramaiah, C., Pham, H. C., Phan, T. T. H., and Ngo, T. Q. (2023). The impact of eco-innovation, trade openness, financial development, green energy and government governance on sustainable development in ASEAN countries. Renew. Energy 211, 259–268. doi:10.1016/j.renene.2023.04.109
Dogan, E., and Ozturk, I. (2017). The influence of renewable and non-renewable energy consumption and real income on CO2 emissions in the USA: evidence from structural break tests. Environ. Sci. Pollut. Res. 24, 10846–10854. doi:10.1007/s11356-017-8786-y
Dong, F., Li, J., Li, Z., Chen, Y., Zheng, L., Lu, B., et al. (2023). Exploring synergistic decoupling of haze pollution and carbon emissions in emerging economies: fresh evidence from China. Environ. Dev. Sustain., 1–38. doi:10.1007/s10668-023-03338-2
Du, K. R., and Li, J. L. (2019). Towards a green world: how do green technology innovations affect total-factor carbon productivity. Energy Policy 131, 240–250. doi:10.1016/j.enpol.2019.04.033
Fan, J., Wang, J., Liu, M., Sun, W., and Lan, Z. (2022). Scenario simulations of China's natural gas consumption under the dual-carbon target. Energy 252, 124106. doi:10.1016/j.energy.2022.124106
Feng, Y., Chen, Z., and Nie, C. (2023). The effect of broadband infrastructure construction on urban green innovation: evidence from a quasi-natural experiment in China. Econ. Analysis Policy 77, 581–598. doi:10.1016/j.eap.2022.12.020
Gong, Y., Cao, H., and Yuan, L. (2024). Does patent pledge reduce pollution and carbon emissions? Evidence from China. Environ. Res. 247, 118274. doi:10.1016/j.envres.2024.118274
Guang, F., Deng, Y., Wen, L., Sharp, B., and Hong, S. (2023). Impact of regional energy allocation distortion on carbon emission efficiency: evidence from China. J. Environ. Manag. 342, 118241. doi:10.1016/j.jenvman.2023.118241
Guang, F., He, Y., Wen, L., and Sharp, B. (2019). Energy intensity and its differences across China’s regions: combining econometric and decomposition analysis. Energy 180, 989–1000. doi:10.1016/j.energy.2019.05.150
Huang, H. Y., Wang, F. R., Song, M. L., Balezentis, T., and Streimikiene, D. (2021). Green innovations for sustainable development of China: analysis based on the nested spatial panel models. Technol. Soc. 65, 101593. doi:10.1016/j.techsoc.2021.101593
Huang, R., Zhang, S., and Wang, P. (2022). Key areas and pathways for carbon emissions reduction in Beijing for the “Dual Carbon” targets. Energy Policy 164, 112873. doi:10.1016/j.enpol.2022.112873
Jiang, Q., and Tang, P. (2023). All roads lead to Rome? Carbon emissions, pollutant emissions and local officials’ political promotion in China. Energy Policy 181, 113700. doi:10.1016/j.enpol.2023.113700
Jin, P., Peng, C., and Song, M. (2019). Macroeconomic uncertainty, high-level innovation, and urban green development performance in China. China Econ. Rev. 55, 1–18. doi:10.1016/j.chieco.2019.02.008
Khattak, S. I., Ahmad, M., ul Haq, Z., Shaofu, G., and Hang, J. (2022). On the goals of sustainable production and the conditions of environmental sustainability: does cyclical innovation in green and sustainable technologies determine carbon dioxide emissions in G-7 economies. Sustain. Prod. Consum. 29, 406–420. doi:10.1016/j.spc.2021.10.022
Lei, L., Ozturk, I., Murshed, M., Abrorov, S., Alvarado, R., and Mahmood, H. (2023). Environmental innovations, energy innovations, governance, and environmental sustainability: evidence from South and Southeast Asian countries. Resour. Policy 82, 103556. doi:10.1016/j.resourpol.2023.103556
Li, L., Li, M., Ma, S., Zheng, Y., and Pan, C. (2022). Does the construction of innovative cities promote urban green innovation? J. Environ. Manag. 318, 115605. doi:10.1016/j.jenvman.2022.115605
Liu, C., Wang, C., Yang, S., Wang, W., Zhao, L., and Li, Q. (2024). Catalyst or Obstacle? Green innovation and total factor energy efficiency. Front. Environ. Sci. 12, 1397462. doi:10.3389/fenvs.2024.1397462
Liu, Y., and Dong, F. (2021). How technological innovation impacts urban green economy efficiency in emerging economies: a case study of 278 Chinese cities. Resour. Conservation Recycl. 169, 105534. doi:10.1016/j.resconrec.2021.105534
Mongo, M., Belaid, F., and Ramdani, B. (2021). The effects of environmental innovations on CO2 emissions: empirical evidence from Europe. Environ. Sci. Policy 118, 1–9. doi:10.1016/j.envsci.2020.12.004
Ostadzad, A. H. (2022). Innovation and carbon emissions: fixed-effects panel threshold model estimation for renewable energy. Renew. Energy 198, 602–617. doi:10.1016/j.renene.2022.08.073
Peng, W., Yin, Y., Kuang, C., Wen, Z., and Kuang, J. (2021). Spatial spillover effect of green innovation on economic development quality in China: evidence from a panel data of 270 prefecture-level and above cities. Sustain. Cities Soc. 69, 102863. doi:10.1016/j.scs.2021.102863
Porter, M. E., and Van der Linde, C. (1995). Toward a new conception of the environment-competitiveness relationship. J. Econ. Perspect. 9 (4), 97–118. doi:10.1257/jep.9.4.97
Prest, B. C., and Krupnick, A. (2021). How clean is “refined coal”? An empirical assessment of a billion-dollar tax credit. Energy Econ. 97, 105023. doi:10.1016/j.eneco.2020.105023
Puertas, R., and Marti, L. (2021). Eco-innovation and determinants of GHG emissions in OECD countries. J. Clean. Prod. 319, 128739. doi:10.1016/j.jclepro.2021.128739
Qiu, Y., Wang, H., and Wu, J. (2023). Impact of industrial structure upgrading on green innovation: evidence from Chinese cities. Environ. Sci. Pollut. Res. 30 (2), 3887–3900. doi:10.1007/s11356-022-22162-1
Qureshi, M. A., Ahsan, T., and Gull, A. A. (2022). Does country-level eco-innovation help reduce corporate CO2 emissions? Evidence from Europe. J. Clean. Prod. 379, 134732. doi:10.1016/j.jclepro.2022.134732
Sharif, A., Saqib, N., Dong, K., and Khan, S. A. R. (2022). Nexus between green technology innovation, green financing, and CO2 emissions in the G7 countries: the moderating role of social globalisation. Sustain. Dev. 30 (6), 1934–1946. doi:10.1002/sd.2360
Singh, S. K., Del Giudice, M., Chierici, R., and Graziano, D. (2020). Green innovation and environmental performance: the role of green transformational leadership and green human resource management. Technol. Forecast. Soc. Change 150, 119762. doi:10.1016/j.techfore.2019.119762
Sun, H. P., Edziah, B. K., Sun, C. W., and Kporsu, A. K. (2019). Institutional quality, green innovation and energy efficiency. Energy Policy 135, 111002. doi:10.1016/j.enpol.2019.111002
Tang, P., Jiang, Q., and Wang, C. (2024b). Beyond environmental actions: how environmental regulations stimulate strategic-political CSR engagement in China? Energy Econ. 129, 107171. doi:10.1016/j.eneco.2023.107171
Tang, P., Wang, C., Jiang, Q., Liu, X., and Wang, J. (2023). Symbol or substance? Environmental regulations and corporate environmental actions decoupling. J. Environ. Manag. 346, 118950. doi:10.1016/j.jenvman.2023.118950
Tang, P., Wang, G., Liu, X., and Wang, J. (2024a). When income inequality reduction meets green transition: pay gap and green innovation in China. J. Environ. Plan. Manag., 1–22. doi:10.1080/09640568.2024.2323608
Tian, M., Wang, Y., and Wang, Y. (2023). High-speed rail network and urban agglomeration economies: research from the perspective of urban network externalities. Socio-Economic Plan. Sci. 85, 101442. doi:10.1016/j.seps.2022.101442
Ulucak, R., and Baloch, M. A. (2023). An empirical approach to the nexus between natural resources and environmental pollution: do economic policy and environmental-related technologies make any difference? Resour. Policy 81, 103361. doi:10.1016/j.resourpol.2023.103361
Usman, A., Ozturk, I., Ullah, S., and Hassan, A. (2021). Does ICT have symmetric or asymmetric effects on CO2 emissions? Evidence from selected Asian economies. Technol. Soc. 67, 101692. doi:10.1016/j.techsoc.2021.101692
Wang, H., Gu, K., Sun, H., and Xiao, H. (2023b). Reconfirmation of the symbiosis on carbon emissions and air pollution: a spatial spillover perspective. Sci. Total Environ. 858, 159906. doi:10.1016/j.scitotenv.2022.159906
Wang, Z., Li, L., and Ge, J. (2023a). Modernizing one-size-fits-all policy for haze pollution from enterprise registration big data: spatial effects of industry activities. J. Clean. Prod. 423, 138829. doi:10.1016/j.jclepro.2023.138829
Wu, J., and Guo, Z. (2016). Research on the convergence of carbon dioxide emissions in China: a continuous dynamic distribution approach. Stat. Res. 33 (1), 54–60. (in Chinese).
Wu, T., Wen, L., and Yi, M. (2024b). Balancing growth targets and environmental regulations: an empirical analysis of dual policy impact on corporate environmental responsibility-insights from China. J. Environ. Manag. 355, 120500. doi:10.1016/j.jenvman.2024.120500
Wu, T., Yi, M., and Zhang, Y. (2024a). Towards cities' green growth: the combined influence of economic growth targets and environmental regulations. Cities 146, 104759. doi:10.1016/j.cities.2023.104759
Xia, H., Ding, L., and Yang, S. (2022b). The impact of technological progress on China’s haze pollution-based on decomposition and rebound research. Environ. Sci. Pollut. Res. 29, 22306–22324. doi:10.1007/s11356-021-16895-8
Xia, W., Apergis, N., Bashir, M. F., Ghosh, S., Doğan, B., and Shahzad, U. (2022a). Investigating the role of globalization, and energy consumption for environmental externalities: empirical evidence from developed and developing economies. Renew. Energy 183, 219–228. doi:10.1016/j.renene.2021.10.084
Xin, D., Ahmad, M., Lei, H., and Khattak, S. I. (2021). Do innovation in environmental-related technologies asymmetrically affect carbon dioxide emissions in the United States? Technol. Soc. 67, 101761. doi:10.1016/j.techsoc.2021.101761
Xu, L., Fan, M., Yang, L., and Shao, S. (2021). Heterogeneous green innovations and carbon emission performance: evidence at China's city level. Energy Econ. 99, 105269. doi:10.1016/j.eneco.2021.105269
Yan, Z. M., Zou, B. L., Du, K. R., and Li, K. (2020). Do renewable energy technology innovations promote China's green productivity growth? Fresh evidence from partially linear functional-coefficient models. Energy Econ. 90, 104842. doi:10.1016/j.eneco.2020.104842
Yang, S., Wang, C., Zhang, H., Lu, T., and Yi, Y. (2022). Environmental regulation, firms’ bargaining power, and firms’ total factor productivity: evidence from China. Environ. Sci. Pollut. Res. 29, 9341–9353. doi:10.1007/s11356-021-16116-2
Yi, M., Wang, Y., Sheng, M., Sharp, B., and Zhang, Y. (2020). Effects of heterogeneous technological progress on haze pollution: evidence from China. Ecol. Econ. 169, 106533. s. doi:10.1016/j.ecolecon.2019.106533
Yildirim, D. C., Esen, O., and Yildirim, S. (2022). The nonlinear effects of environmental innovation on energy sector-based carbon dioxide emissions in OECD countries. Technol. Forecast. Soc. Change 182, 121800. doi:10.1016/j.techfore.2022.121800
Yuan, C., and Zhang, L. (2015). Public education spending and private substitution in urban China. J. Dev. Econ. 115, 124–139. doi:10.1016/j.jdeveco.2015.02.006
Yuan, S., Pan, X., and Li, M. (2022). The nonlinear influence of innovation efficiency on carbon and haze co-control: the threshold effect of environmental decentralization. Environ. Dev. Sustain. 25, 14283–14307. doi:10.1007/s10668-022-02664-1
Zhang, C., and Yang, S. (2024). The synergy effect of energy security and carbon-haze collaborative management: from the perspective of biased technological progress. Environ. Res. 252, 118741. doi:10.1016/j.envres.2024.118741
Zhang, L., Peng, J., Liu, J., and Huang, W. (2023). The impact of carbon-biased technological progress on carbon haze coordinated governance: insights from China. Environ. Sci. Pollut. Res. 31, 36329–36348. doi:10.1007/s11356-023-28614-6
Zhang, S., Cheng, L., Ren, Y., and Yao, Y. (2024). Effects of carbon emission trading system on corporate green total factor productivity: does environmental regulation play a role of green blessing? Environ. Res. 248, 118295. doi:10.1016/j.envres.2024.118295
Zhao, X., Nakonieczny, J., Jabeen, F., Shahzad, U., and Jia, W. X. (2022). Does green innovation induce green total factor productivity? Novel findings from Chinese city level data. Technol. Forecast. Soc. Change 185, 122021. doi:10.1016/j.techfore.2022.122021
Keywords: urban green innovation, collaborative governance, haze pollution, carbon emissions, energy intensity
Citation: Li W, Wang C, Duan D, Li Q, Tian R and Ma T (2024) Unlocking the nexus: exploring the effect of urban green innovation on haze pollution and carbon emissions intensity in Chinese cities. Front. Environ. Sci. 12:1440976. doi: 10.3389/fenvs.2024.1440976
Received: 30 May 2024; Accepted: 20 June 2024;
Published: 12 July 2024.
Edited by:
Le Wen, University of Auckland, New ZealandCopyright © 2024 Li, Wang, Duan, Li, Tian and Ma. This is an open-access article distributed under the terms of the Creative Commons Attribution License (CC BY). The use, distribution or reproduction in other forums is permitted, provided the original author(s) and the copyright owner(s) are credited and that the original publication in this journal is cited, in accordance with accepted academic practice. No use, distribution or reproduction is permitted which does not comply with these terms.
*Correspondence: Qiming Li, MTIwMTkxMDU4MUBjdWcuZWR1LmNu