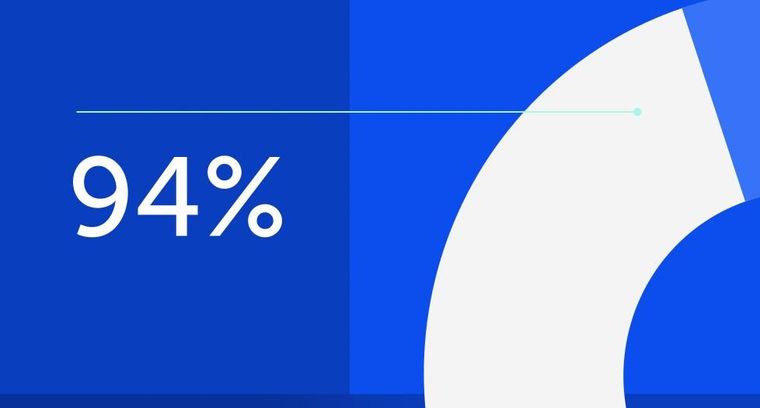
94% of researchers rate our articles as excellent or good
Learn more about the work of our research integrity team to safeguard the quality of each article we publish.
Find out more
ORIGINAL RESEARCH article
Front. Environ. Sci., 28 November 2024
Sec. Environmental Economics and Management
Volume 12 - 2024 | https://doi.org/10.3389/fenvs.2024.1422480
Introduction: The biased technological progress coordinated with factor endowment is an important support for achieving sustainable development in the forestry industry. This study focused on the coupled coordination relationship between forestry factor endowment and technological progress bias, analyzing their spatial impacts on the upgrading of forestry industry structure. The aim is to fully leverage the driving force of technological progress to promote sustainable development of the forestry economy.
Methods: Covering the years from 2005 to 2021, this study calculated the coupling coordination and constructed spatial econometric models to empirically analyze its spatial impacts on the rationalization, advancement, and ecologicalization of the forestry industry structure.
Results and Discussion: The results indicated that the overall level of coupling coordination was relatively low, with certain differences among provinces. The upgrading of the forestry industry structure showed significant spatial correlation, and after considering the cumulative effects of industrial structure upgrading, there existed a positive spatial spillover effect among regions. The improvement of coupling coordination promoted the rationalization and ecologicalization of industrial structure. However, in the short term, it had a negative impact on advancement. For the four major regions, the low level of coupling coordination and regional differences remained key obstacles hindering the promotion of industrial structure upgrading through technological progress. Therefore, there is a need to further optimize the coupling coordination between factor endowment and technological progress bias and place greater emphasis on regional coordinated development. This study may provide new insights into the development of the forestry industry from the perspective of the coupling of factors and technology, emphasizing the necessity of coupling forestry resource endowment with technological progress.
The forestry industry, as a crucial carrier for converting ecological benefits into economic gains, is an important component of the green economy. Developing the forestry industry not only promotes economic growth and employment but also drives environmental protection and sustainable development. China, as the world’s largest developing country and a major forestry industry player, ranks first globally in both forest product production and consumption. The report to the 20th National Congress of the Communist Party of China clearly stated that high-quality development is the primary task of comprehensively building a modern socialist country. As an integral part of ecological civilization construction, forestry plays an important role in ensuring national ecological security, improving the ecological environment, and promoting sustainable economic and social development. Technological progress as an important support for achieving sustainable development in forestry. However, China still faces challenges such as limited forestry resources and inadequate levels of technological progress in forestry, resulting in insufficient driving force for the upgrading of the forestry industry structure. Therefore, exploring how to scientifically and reasonably utilize limited forestry resources and promote rapid technological advancement in forestry is of great significance for achieving the development of China’s forestry industry and upgrading its industry structure. Moreover, it can serve as a valuable reference for forestry development in other developing countries and even globally.
Regarding technological progress, existing research generally indicated a positive correlation between technological progress and industrial structure upgrading (Wu and Liu, 2021; Su and Fan, 2022), suggesting a supportive role of technological progress in rationalizing and advancing industrial structures (Wang et al., 2021). However, due to China’s insufficient innovation capacity in key technologies, the efficacy of technological progress is not fully realized (Yang et al., 2018). Some scholars emphasized that transcending the middle-technology trap is a challenge that China must face, necessitating further enhancement of technological levels to promote industrial progress and achieve high-quality economic development (Zheng, 2023). Some scholars also argued that technological progress was only positively associated with industrial development in regions with moderate to high levels of economic development, and it cannot promote industrial development in underdeveloped areas in the short term (Abid et al., 2022). Overall, technological progress has a positive impact on industrial structure upgrading, but it is also influenced by regional development heterogeneity.
Moreover, technological progress exhibits a bias among different input factors, showing non-Hick neutrality. Hicks and Acemoglu defined technological bias as, under the influence of technological progress, the marginal output ratio of a certain factor increases relative to other factors, indicating that technological progress tends to favor this factor (Hicks, 1963; Acemoglu, 2002; 2007). When the level of technology is low, technological bias tends to drive factors from industries with lower technological progress rates to those with higher rates, thereby promoting industrial structural upgrading and increasing the growth rate of total factor productivity. However, due to the phenomenon of unbalanced development among various industries in China (Wu et al., 2021), there exists a mismatch between technological progress and factor endowments in some industries (Xue and Zhou, 2019; Wang et al., 2023), leading to ineffective inducement of technological change mechanisms, which hampers the high-quality development of industries. Optimizing the allocation of factors can enhance the efficiency of technological progress (Jianmin and Li, 2020). When biased technological progress matches factor endowments, it can improve total factor productivity in industry (Ren and Zeng, 2021; Li and Hu, 2023; Ye et al., 2024), thereby promoting industrial structural upgrading. Therefore, abandoning the neutral assumption of technological progress and promoting a favorable alignment between technological progress and factor endowments has a positive impact on industrial development.
In the forestry domain, most scholars believed that the upgrading of forestry industry structure is closely related to factors such as technological progress, factor endowment allocation, level of economic development, national policies, urbanization rate, and foreign investment (Tang and Li, 2017; Chen and Zhang, 2019; Jiang Y. and Jiang J., 2021; Hou et al., 2023; Ma et al., 2023). Moreover, the development of forestry industry in different regions is not independent of each other, there exists a certain spatial spillover effect. Therefore, many scholars adopted spatial econometric models to explore the influencing factors of forestry industry structure upgrading. However, research on biased technological progress in forestry remains limited, and the mechanism of the interaction between biased technological progress and factor endowment on forestry industry structure needs further investigation.
The above research suggests that achieving the coupling coordination between technological progress bias and factor endowments is of significant importance, and it can also have a positive impact on industrial structure upgrading. However, existing literature mainly focuses on macro-level studies at the national or regional level, or industrial sectors, with limited research on forestry. The interaction between factor endowments and technological progress bias ultimately reflects whether they can develop in a coupled manner. Currently, there are few literature on the coupling development of these two aspects, and no research has yet been found on how the combination of the two affects industrial structure.
Therefore, this study adopted a spatial perspective and constructed spatial econometric models to investigate the impact of the coupling coordination of forestry factor endowment and technological progress bias on industrial structure upgrading across 31 provinces and four major regions in China. The aim was to investigate how to fully leverage the driving force of technological progress in forestry to transform the traditional forestry development model and provide insights for promoting cross-regional coordinated development of the forestry industry. The main contributions were as follows: 1) From the novel perspective of the coupling development of forestry factor endowments and technological progress bias, this study has developed the coupling coordination degree indicator, which may help enhance the efficiency of forestry factor allocation and leverage the driving effect of technological progress. 2) By deeply exploring the impact of the coupling development of forestry factor endowments and technological progress bias on industrial structure upgrading, this study has revealed the significance of achieving their coupling development, which may provide valuable insights and decision-making support for transforming the traditional forestry development model and promoting cross-regional coordination in the forestry industry.
In existing research, industrial structure upgrading is generally divided into three aspects: rationalization, advancement, and ecologicalization (Si and Yao, 2022). Rationalization refers to optimizing factor allocation and improving production efficiency to achieve coordinated development among industries, thereby better meeting market demands (Chen et al., 2024). Advancement emphasizes the transformation of industries toward higher value-added and more technologically advanced directions, promoting the development of emerging industries to enhance the overall competitiveness of the industry (Murakami, 2015; Chovancová et al., 2018). Ecologicalization focuses on the coordination between industrial development and environmental protection, emphasizing sustainability, with the goal of reducing resource waste and environmental pollution, and promoting the development of eco-friendly industries (Arabi et al., 2014; Smith et al., 2015; Gao and Zhang, 2021).
According to the theory of factor endowments and the theory of induced technological progress, the coupling coordination between forestry factor endowments and technological progress bias aims to change the relative marginal efficiency of production factors, promoting the flow of forestry production factors among the three major industries (Alvarez-Cuadrado et al., 2018). The process of factor redistribution inevitably leads to changes in the input proportions of factors and the production structure between sectors, altering the efficiency of factor allocation and ensuring continuous coordination in the factor configuration among the three major forestry industries (Agyeman and Ochuodho, 2021), thus achieving the rationalization of the industrial structure.
Referring to Maslow’s hierarchy of needs theory, as a country’s overall strength increases and the forestry industry develops, the demand for forestry products among residents also evolves. The demand shifts from basic products such as timber and forest materials to high-value-added products like ornamental forestry products and forest foods. The coupling coordination between forestry factor endowments and technological progress bias will effectively transform the supply of forestry industries, achieving the optimal allocation of forestry factors (Jung et al., 2017). This is conducive to fostering emerging industries, which in turn plays a positive role in promoting the advancement of the forestry industry.
Forestry itself has ecological benefits, and industry sectors that contribute to environmental development are likely to receive policy support, while sectors that generate excessive waste during production may face restrictions. If the coupling of forestry factor endowments and technological progress drives the development of environmentally beneficial sectors, it will promote the transformation of leading industries and foster the ecologicalization of the forestry industry structure (Gao and Zhang, 2021).
Based on this, this study proposed the following hypothesis:
H1. The coupling coordination between forestry factor endowments and technological progress bias directly promotes the upgrading of the forestry industry structure.
With the advancement of regional economic integration, the coupling coordination between forestry factor endowments and technological progress bias not only affects a single region but also plays a role in the upgrading of the forestry industry structure in neighboring regions through spatial spillover effects. According to regional economic theory, spatial spillover effects refer to the influence of economic activities in one region on the economic development of neighboring regions. This effect is realized through various channels such as the flow of factors, technology diffusion, and industrial linkages (Jiang Y. and Jiang J., 2021).
In the forestry industry, technological innovation and optimal factor allocation often achieve success in one region before gradually spreading to neighboring areas. For example, advanced forestry management technologies and equipment are disseminated to nearby regions through corporate cooperation, industry exchanges, and other channels, thereby improving overall forestry production efficiency (Hou et al., 2023). At the same time, the flow of factors such as labor and capital between regions promotes the reallocation of advantageous resources, driving the upgrading of the forestry industry structure in surrounding areas.
The synergistic effect of regional policies also provides favorable conditions for spatial spillover effects (Ye et al., 2024). When governments design relevant policies, if they can take into account the development needs of different regions and form a diversified support system, it will drive inter-regional cooperation and interaction. This enhances industrial agglomeration effects and creates a virtuous cycle along the upstream and downstream of the industrial chain (Chen and Zhang, 2019), thereby accelerating the rational flow of technology and resources, and further promoting the rationalization, advancement, and ecologicalization of the forestry industry structure.
Based on this, this study proposed the following hypothesis:
H2. The coupling coordination between forestry factor endowment and technological progress bias promotes the upgrading of the forestry industrial structure through spatial spillover effects.
Spatial autocorrelation testing is fundamental to spatial econometrics and typically involves using Moran’s I to study the spatial correlation and distribution characteristics of spatial units. In this study, we integrated economic attributes and geographical distance features by combining economic distance weights with geographical distance weights to construct a spatial econometric-geographic weight matrix. The formula for calculating the weight matrix is as follows:
In Formula 1, dij represents the geographical distance between regions i and j, Yi and Yj represent the per capita forestry total output value of regions i and j respectively. The α took the value of 0.5. Then, the global Moran’s I is calculated to describe the spatial characteristics of forestry industry structural upgrading, with the Formula 2 as follows:
If the global Moran’s I is positive, it indicates that the distribution of indicator values in each region shows spatial positive correlation; conversely, if it is negative, it indicates spatial negative correlation. If the global Moran’s I value is 0, it indicates that the distribution of indicator values in each region shows randomness.
This study used panel data to construct spatial econometric models, aiming to better observe the heterogeneity of individual behaviors across provinces in both time and space dimensions, thereby preventing estimation errors that may arise from omitted variables. Spatial Error Model (SEM) reflects unobserved spatial correlation by introducing spatial error terms. Spatial Lag Model (SAR) reflects the possibility that the value of the dependent variable in a certain area may be influenced by the values of explanatory variables in neighboring areas by introducing spatial lag effects. Spatial Durbin Model (SDM) integrates both spatial lag effects and spatial error terms, providing a more comprehensive reflection of spatial correlation and spatial heterogeneity. In this study, we constructed a spatial econometric model and determine the optimal model for analysis through testing. The model expression is as follows:
In Formula 2, Yit represents the dependent variable, where Y1it represents rationalization of forestry industry structure, Y2it represents advancement, and Y3it represents ecologicalization. ρ is the spatial autocorrelation coefficient, Wij is the spatial weight matrix, β is the general regression coefficient, γ is the spatial regression coefficient, μi and λi represent spatial and time fixed effects respectively, εit represents the error term. When γ = 0, the model degenerates into a Spatial Lag Model (SAR); when γ + ρβ = 0, the model degenerates into a Spatial Error Model (SEM); and when γ = 0 and ρ = 0, the model degenerates into a simple Linear Regression Model (OLS).
Considering the actual situation where the upgrading of forestry industry structure may be influenced by the previous period’s development level, this study introduced the spatial lag term lagged by one period of the dependent variable and constructs a dynamic SDM.
In Formula 3, τ represents the elasticity coefficient of spatial lag effect.
Lesage and Pace proposed that compared to traditional Linear Regression Models, the SAR and the SDM expand the information set by introducing spatial lag terms, resulting in regression coefficients that cannot effectively explain the impact of each explanatory variable on the dependent variable (Lesage and Pace, 2009). Therefore, following Elhorst’s approach, the total effect of the coupling coordination between forestry factor endowment and technological progress bias on the upgrading of forestry industry structure was decomposed into direct and indirect effects (Elhorst, 2012). The total effect refers to the average impact on the upgrading of the forestry industry structure, the direct effect refers to the impact on the upgrading of the forestry industry structure in the local area, and the indirect effect refers to the impact on the upgrading of the forestry industry structure in neighboring areas.
This study took the indices of rationalization, advancement, and ecologicalization of the forestry industry structure, denoted as RFIS, AFIS, and EFIS, respectively, as the dependent variables. The RFIS represents the degree of coordination in the development of different industries, reflecting the quality of aggregation and efficiency of resource allocation of capital, labor, and land among different industrial sectors. The rationalization of industrial structure can be measured using either the degree of deviation in industrial structure or the Theil index. Considering that the Theil index does not have an absolute value calculation, while also incorporating the theoretical foundation and economic significance of the structural deviation. This study measured the RFIS using an improved Theil index (Yao and Ma, 2022; Hu J. et al., 2023; Hu L. et al., 2023), calculated by the following formula:
In Formula 4, Y represents the total output value of the forestry industry, Yi represents the output value of the ith sector of the forestry industry. L represents the total number of employees in the forestry industry, and Li represents the number of employees in the ith sector of the forestry industry. When RFIS = 0, it indicates a rational industrial structure; conversely, the larger the RFIS value, the less rational the industrial structure.
The AFIS reflects the transition of industries from a lower-level structure to a higher-level structure. The advancement of industrial structure is currently measured mainly through three methods: the non-agricultural output ratio, the industrial structure adjustment coefficient, and the industrial structure hierarchy coefficient. Both the non-agricultural output ratio and the industrial structure adjustment coefficient fail to reflect the overall changes in the industrial structure and do not provide a comprehensive assessment of the three sectors. On the other hand, the industrial structure hierarchy coefficient can simultaneously reflect the structure of the three sectors as well as the relative structure between them, offering a more comprehensive interpretation. This study measured the AFIS using the coefficient of industrial structure hierarchy (Yao and Ma, 2022; Hu J. et al., 2023), calculated by the following formula:
In Formula 5, Ci and Si represent the weight and output proportion of the ith industry, respectively. Following common practice, weights for the primary, secondary, and tertiary industries were assigned values of 1, 2, and 3, respectively. The higher the proportion of output for the secondary and tertiary industries, the greater the degree of industrial structure advancement.
The EFIS reflects the coordinated development between industry and the environment. It is manifested in maximizing the ecological benefits of forestry while ensuring that pollution and environmental burdens caused during the production process are minimized through efficient resource recycling. This study, following common practices, constructed a comprehensive index system (Lyu et al., 2018; Si and Yao, 2022; Zhou et al., 2024), with the calculation formula as follows:
In Formula 6, wi represents the weight of the index determined by the entropy weight method, xi represents the evaluation index, including afforestation area, total energy consumption of forestry production, emissions of sulfur dioxide, particulate matter, and solid waste from forestry industry. All indices had been standardized and treated in the same direction. A higher value of EFIS indicates a higher degree of ecologicalization of the industrial structure.
In this study, the core explanatory variable selected was the coupling coordination degree (D) of forestry factor endowment and technological progress bias. Which reflects the matching degree of forestry factor endowment and technological progress bias, serving as a necessary condition for fully utilizing forestry resources, enhancing the value of forestry technology, and promoting industrial structure upgrading.
First, the relative abundance of forestry factor in different regions was measured by the relative scarcity between forestry capital, labor, and land factors. Specifically, the relative endowment coefficients of forestry factors were used to evaluate the relative endowment level of forestry factors in each region. The calculation formulas for the relative endowment coefficients of labor and capital factors are as follows (Xue and Zhou, 2019):
In Formula 7, Ki, Li and Ai represent the forestry capital, labor, and land inputs in region i respectively. If λLKi > 1, it means that in region i, the labor input is relatively abundant compared to the capital input; if λLKi < 1, it means that the labor input in region i is relatively scarce compared to the capital input. The calculations and interpretations of λKai and λAli are similar.
Forestry technological progress bias was measured by constructing bias indices BLK, BKA and BAL. The methods for measuring technological progress bias mainly include the CES production function, data envelopment analysis (DEA), and transcendental logarithmic production function (Translog). However, the CES production function and DEA are generally used to analyze technological progress bias under the conditions of two production factors, capital and labor. These methods cannot calculate the technological progress bias between multiple factors. In contrast, the transcendental logarithmic production function assumes variable substitution elasticity, considers the interactions between input factors, and decomposes the total error into random error and inefficiency-related errors. This method is more rational and can accurately calculate the technological progress bias for the three production factors. First, we established a transcendental logarithmic production function that includes capital, labor, and land, as follows (Heathfield and Wibe, 1987):
In Formula 8, Yit represents the total output value of the forestry industry in each province, β0 is the mean cross-section effect, Kit, Lit and Ait denote the stock of forestry capital, the quantity of labor input, and the area of land used in forestry production, respectively. T represents the time trend; βK, etc. denote the accumulation effects of each factor; βKK, etc. represents the scale effect; and βKL, etc. represents the synergistic effect. In this study, the forestry capital stock was calculated using the perpetual inventory method with 2005 as the base year. Labor input was represented by the number of forestry workers at the end of each year. Forestland input was derived from the data of the sixth, seventh, eighth, and ninth National Forest Resources Inventories.
Using the results from the transcendental logarithmic production function, the forestry output elasticity α was calculated to reflect the extent of changes in forestry economic output influenced by the input of production factors over a certain period. Subsequently, this can be used to calculate the technological progress bias in forestry. For example, the formulas for the technological progress bias of capital and labor are as follows:
In Formula 9, MP represents the marginal product of the factor, and ∂MP/∂T represents the increment of the marginal product of the factor. If BLKi > 0, it indicates that technological bias in forestry is inclined towards labor; otherwise, it is biased towards capital. The calculation formulas and meanings for BKA and BAL are similar (Diamond, 1965; Jiang et al., 2024).
Finally, this study adopted the coupling coordination model to calculate the coupling coordination of two subsystems: forestry factor endowment and technological progress bias. The coupling degree is calculated using the following formula:
In Formula 10, Ui represents the comprehensive value of each subsystem, and the distribution range of C values is [0,1]. A larger C value indicates less dispersion among subsystems and a higher degree of coupling. To avoid the phenomenon of false coupling, a coordination degree is introduced to reflect whether the system coupling is benign, constructing a coupling coordination model (Yang et al., 2023; Yuan et al., 2024). The calculation formula is as follows:
In Formulas 11, 12, represents the coordination degree between the two, where D represents the coupling coordination degree of forestry factor endowment and technological progress bias. α and β represent the degree to which each subsystem affects the entire system. This study assumed that the importance of the two subsystems was equal, so α = β = 0.5.
Control variables encompassed two main aspects: forestry industry factors and macroeconomic factors (Xiong et al., 2018). Regarding forestry industry factors, forestry inputs comprised capital input, labor input, and land input, while forestry output was measured by the total forestry output value (Chen and Jiang, 2014). The essence of upgrading the forestry industry structure lies in the advancement of both vertical integration and horizontal connectivity among forestry sectors under the influence of technological innovation. The focus of technological innovation ultimately manifests in the improvement of efficiency in utilizing forestry resources. Therefore, forestry capital productivity, forestry labor productivity, and forestry land productivity were chosen to comprehensively measure the input-output efficiency of forestry factors. When these ratios increase, the efficiency of factor production improves, thereby promoting the upgrading of the industry structure. Additionally, factors reflecting the forestry industry’s situation were selected, including the control rate of forestry pests and diseases (Xiang et al., 2021), the average income of forestry personnel, and national forestry investment. In terms of macroeconomic factors, this included the degree of openness (Lu and Zhang, 2022), measured by the ratio of the total value of imports and exports to regional GDP after adjustment; the level of economic development, measured by per capita GDP after adjustment; and the urbanization rate (Tang and Li, 2017).
The study focused on 31 provinces across China, categorizing them into four regions: Eastern, Central, Western, and Northeastern China based on the classification provided by the National Bureau of Statistics. For the reason of data availability and timeliness, the sample data for each variable covered the period from 2005 to 2021, spanning a total of 17 years. The data provided a relatively complete time span while ensuring consistent statistical standards. This ensured a comprehensive and accurate reflection of the development status of China’s forestry industry. The original data for all variables were sourced from authoritative publications, such as the China Statistical Yearbook and the China Forestry Statistics Yearbook (or China Forestry and Grassland Statistics Yearbook), which underwent systematic statistical processing and verification. Some of the forestry data were sourced from the Sixth, Seventh, Eighth, and Ninth National Forest Resources Inventory of China, ensuring a certain level of reliability and accuracy. In order to mitigate the impact of differences in data ranges on model fitting results and to alleviate heteroscedasticity effects, logarithmic transformations were applied to LR, AR, AI, NI, and GDP during the testing and fitting of the spatial econometric models in this study. The descriptive statistics of variables were shown in supplementary materials (Supplementary Table A1).
Between 2005 and 2021, the average annual values of the coupling coordination between forestry factor endowment and technological progress bias nationwide ranged between 0.3 and 0.5, indicating a state of mild to imminent imbalance. The relatively low degrees of coupling coordination had hindered the effective operation of inducement mechanisms for technological transitions, a trend similar to findings in agricultural research by other scholars (Xue and Zhou, 2019).
Figure 1 intuitively illustrates the spatial distribution of coupling coordination between forestry factor endowment and technological progress bias in the years 2005, 2011, 2016, and 2021. Overall, there was an upward trend in coupling coordination from 2005 to 2016, followed by a downward trend from 2017 to 2021. Specifically, Shanghai and Xizang exhibited relatively favorable and stable degrees of coupling coordination. Beijing showed a rapid upward trend, transitioning from being on the verge of disorder to basic coupling coordination during the observation period. Heilongjiang exhibited a certain downward trend, while southern provinces such as Jiangxi, Jiangsu, Zhejiang, and Guangxi showed relatively more fluctuations. However, their overall levels remained higher than those of other provinces.
Figure 1. The spatial distribution of the coupling development level between forestry factor endowment and technological progress bias.
Supplementary Table A2 shows that the Moran indices were mostly positive, with a few exceptions, and significant at the 10% level. The results indicate a significant positive spatial correlation in the rationalization, advancement, and ecologicalization of the forestry industry structure, suggesting the presence of spatial clustering phenomena.
This study used LM test, Hausman test, LR test, and Wald test to select the appropriate spatial econometric model for rationalizing the forestry industry structure. The LM test was used to determine whether spatial lag effects and spatial error effects were present. If neither was significant, the OLS model should have been used. The Hausman test was employed to distinguish between fixed effects and random effects, while the LR test further identified whether the fixed effects pertained to spatial fixed effects, time fixed effects, or both. Additionally, the LR test and Wald test together assessed whether the SDM model degenerated into a SAR model or SEM model. Table 1 shows that at the national level, the SDM with dual fixed effects was adopted. For the central China, the SEM with dual fixed effects was adopted. However, the spatial lag and spatial error models for the eastern, western, and northeastern China did not pass the test at the significance level of 10%. Therefore, the OLS was adopted for these three regions. Simultaneously, the variance inflation factor (VIF) values for all variables at both the national and regional levels were less than 5, indicating that there was no multicollinearity.
Table 1. Test results of the spatial econometric model for the rationalization of the forestry industry structure.
From the results at the national level (Table 2), the spatial autocorrelation coefficient (rho) of the static SDM model was significantly negative (1% level), while that of the dynamic SDM model was significantly positive (1% level). As the rationalization level of the forestry industry structure improves within a local area, the degree of optimal allocation among various sectors of the forestry industry increases. In the short term, there may be competitive effects between neighboring regions. However, in the long term, positive spatial spillover effects emerge between neighboring regions. This phenomenon stimulates various sectors of the forestry industry to fully exploit their comparative advantages. Through geographical and economic associations, it promotes the optimal allocation of resources and industries across various regions.
From the results of the effect decomposition in the dynamic SDM model (Table 3), it can be observed that the improvement in the degree of coupling coordination between forestry factor endowment and technological progress bias (D) was conducive to fully exploiting the advantages of factor endowment and the value of forestry technology in the region (at a significance level of 5%). This enhancement increases the efficiency of resource utilization and allocation, thereby creating a better environment for the rational development and optimization of the forestry industry structure in the region. Moreover, it attracts more technology and capital into the forestry industry sectors of the region, facilitating the optimal allocation of resources among the three sectors of the forestry industry and advancing the rationalization process of the forestry industry structure. This also confirmed the positive effect on the rationalization of the forestry industrial structure, as proposed in hypothesis H1. However, due to the overall low degree of coupling coordination in China, coupled with the geographic constraints on the mobility of forest land resources and significant spatial disparities in both forest land resources and forestry capital investment, the impact of coupling coordination on the rationalization of the forestry industry structure in neighboring regions is not prominent. The positive effect of spatial spillover, as proposed in hypothesis H2, on the rationalization of the forestry industrial structure was not yet evident.
Table 3. Decomposition results of the effect of dynamic SDM on the rationalization of the forestry industry structure.
As for the control variables, the indirect effect coefficients of the control rate of forestry pests and diseases (PCR) was consistently negative and significant (1% level), indicating a positive spatial spillover effect. This suggests that through technological spillovers, neighboring regions’ industrial structures are facilitated towards rationalization. The coefficients of openness (OPEN) and economic development level (GDP) were both negative and significant (1% level), promoting the rationalization of the forestry industry structure. This openness brings about a richer market space and demand for the forestry industry, providing more financial and technological support, thus offering new impetus and support for the coordinated development of the three sectors of the forestry industry. The coefficients of urbanization rate (UR) were consistently positive and significant (1% level). The increase in the urbanization rate may lead to population concentration in urban areas, potentially imposing constraints on the development of some labor-intensive forestry industries.
From the regional analysis results (Table 4), there was a certain negative impact of coupling coordination on the rationalization of the forestry industry structure in the eastern China (at a significance level of 1%). This region has relatively limited forest land resources and a relatively homogeneous industrial structure, with a stronger focus on the development of the forestry tertiary industry. Some coastal provinces rely on timber imports from southern forestry areas and external sources to develop the forestry secondary industry, such as wood product manufacturing (Song and Yang, 2020). The reliance on a single development model hampers the effectiveness of coupling coordination and may impede the rationalization of the industrial structure. The insignificant impact of coupling coordination on the western, central, and northeastern China may be attributed to the prevailing imbalance in most provinces, resulting in evident misalignment phenomena and insufficient driving force towards the rationalization of the forestry industrial structure. The western China is influenced by its diverse natural environment, leading to significant differences in the demand for and applicability of forestry technologies across different areas. There are considerable variations in forestry production technology efficiency, with overall efficiency being relatively low, and the region’s capacity to introduce and absorb forestry technologies is weak (Zang et al., 2014). In the northeastern China, the forestry industry has been affected by the comprehensive cessation of natural forest logging. As a result, the industry has gradually transitioned from traditional timber harvesting to a more diversified structure. The rationalization process of the forestry industrial structure is further driven by policy and institutional reforms.
Table 4. Estimated results of the rationalization of the forestry industry structure in the four regions.
Similarly, Table 5 shows that at the national level, the SDM with dual fixed effects was adopted. The eastern, central, and northeastern China should adopt the SAR with double fixed effects, while the western China should adopt the OLS.
Table 5. Test results of the spatial econometric model for the advancement of the forestry industry structure.
From the results at the national level (Table 6), the spatial autocorrelation coefficient (rho) of the static SDM model was significantly negative (1% level), while that of the dynamic SDM model was significantly positive (5% level). As the advancement level of the forestry industry structure improves within a local area, the development mode increasingly relies on technological progress. Simultaneously, the forestry industry changes towards deep processing and high value-added products. In the short term, there is a noticeable competitive effect among different regions. However, in the long term, the advancement level of industrial structuring between neighboring regions mutually promotes each other. Knowledge and technology exhibit spillover effects, enabling neighboring regions to mutually propel the forestry industry towards higher levels of transformation.
From the results of the effect decomposition in the dynamic SDM model (Table 7), the degree of coupling coordination between forestry factor endowment and technological progress bias (D) may have a short-term negative impact on the advancement of the local forestry industry structure (at a significance level of 5%). Simultaneously, there exists a certain level of competition among different regions (at a significance level of 1%). Due to the overall low degree of coupling coordination in China, the introduction of new technologies into the forestry industry is constrained by limited forestry factor endowments. As a result, forestry resources face obstacles in entering emerging forestry industries with high value-added and deep processing. This hinders the transition of development modes and is unfavorable for the advancement of the local forestry industry structure. Additionally, differences exist in the factor endowment structures and technological progress biases among regions, leading to potential contradictions between the spatial spillover effects experienced by neighboring areas and their own resource structures. However, with the continuous improvement of coupling coordination, the adverse effects are expected to gradually diminish over the long term. As China’s forestry industry continues to develop, the aspects related to the advancement of the forestry industry structure in both Hypothesis H1 and Hypothesis H2 are expected to be gradually validated in the future.
Table 7. Decomposition results of the effect of dynamic SDM on the advancement of the forestry industry structure.
As for the control variables, the coefficients for forestry labor productivity (LR) and degree of openness (OPEN) were negative. In this context, the short-term significance level of LR direct effect was 1%, the long-term was 5%, and the short-term significance level of indirect effect was 10%. The short-term significance level of OPEN direct effect was 5%, and the short-term and long-term indirect effects were both 1%. This observation may be attributed to issues such as slow advancement in the quality of forestry labor and an insufficient quantity of skilled forestry workforce in China. The lack of highly skilled forestry personnel could impede innovation within the production process. Additionally, the overall quality of forest product exports in China tends to be low. With an increase in the degree of openness, the rise in exports of primary forest products may accelerate forest resource depletion, exacerbating resource constraints, and adversely affecting the advancement of the forestry industry structure. The short-term direct effect coefficient of forestry land productivity (AR) was positive and significant (10% level), indicating a promoting effect in the short term. Limited forest land resources can be combined with forestry capital and labor to form more efficient production combinations, which is conducive to further developing high value-added forestry industries such as deep processing of forest products, thereby promoting the advancement of the forestry industry structure towards a higher level. The coefficients of economic development level (GDP) were all significantly positive (short term and long-term levels were 1% and 5% respectively), providing a better economic foundation, development environment, and innovation impetus for the development of the forestry industry. This stimulates deeper demand for forest products among consumers, and this deeper demand structure can guide the forestry industry towards higher value-added and higher-quality directions. The coefficients of urbanization rate (UR) were all significantly positive (except for short-term indirect effects at the 5% level, all were significant at the 1% level), which can promote the gathering of more capital and talent in urban areas. Simultaneously, it also drives the clustering of industries and the improvement of industrial chains in the surrounding areas of cities. Leveraging the advantages of urban areas facilitates the synergistic development and positive interaction of upstream and downstream industries, thereby promoting the transformation of the forestry industry towards deep processing and higher value-added.
From the regional analysis results (Tables 8, 9), it was observed that the degree of coupling coordination in the eastern China has a certain negative impact on the advancement of the local forestry industry structure (5% level). However, it exhibits significant spatial spillover effects on neighboring regions (10% level). This phenomenon may be attributed to the relatively higher level of economic development in the eastern China, along with the abundance of forestry technology and talent compared to other regions. Nonetheless, it faces constraints such as low resource allocation efficiency and increasing environmental pressures, resulting in some negative impacts. Moreover, the presence of technological spillovers and non-exclusivity implies that the eastern region has a more pronounced spatial spillover effect on neighboring regions. Due to factors such as the remote geographical locations, poor transportation, and uneven resource distribution in some provinces, the degree of spatial correlation in the western region is relatively low. Xizang, Qinghai, and Xinjiang are relatively isolated within the western region, with weaker connections to other provinces, resulting in no significant spatial spillover effects. Additionally, some provinces in the western region experience notable land desertification and soil erosion, making it an ecologically fragile area. Therefore, the development of forestry in this region should still prioritize ecological construction. The central China stands out for its distinctive characteristics in the development of the forestry industry, focusing mainly on industries such as woody oil crops, medicinal herbs from forests, and tea. It exhibits a higher reliance on the natural geographical environment, with relatively weaker effects from advancements in forestry technology. The Northeast China may be influenced by policies such as the comprehensive logging ban and the transformation of state-owned forest areas. As a result, the output of commercial timber continues to decline, leading the forestry industry to gradually shift towards activities such as forest carbon sink, undergrowth economy, forest tourism, and advanced wood processing. However, influenced by the management system of state-owned forestry enterprises, the development mindset remains conservative (Zhu et al., 2024a), and the driving effect of coupling coordination is not yet evident.
Table 8. Estimated results of the advancement of the forestry industry structure in the four regions.
Table 9. Decomposition results of the advancement effect of forestry industry structure in four regions.
Table 10 shows that at the national level, the SDM with dual fixed effects was adopted. Similarly, The SAR with double fixed effects was adopted for eastern and central China, and the OLS was adopted for the western China. The spatial lag test results for the northeastern China were superior to the spatial error. Therefore, we adopted the SAR with random effects for this region.
Table 10. Test results of the spatial econometric model for the ecologicalization of the forestry industry structure.
From the results at the national level (Table 11), the spatial autocorrelation coefficient (rho) of the static SDM was significantly negative (10% level), while that of the dynamic SDM was positive but not significant. With the improvement of the ecological level of the local forestry industry structure, the development of the forestry industry pays more attention to environmental protection and sustainable development. In the short term, there exists a certain competitive effect among neighboring regions. However, in the long term, the ecological level between neighboring regions mutually promotes each other, albeit not significantly. This may be due to differences in factors and policies between regions, resulting in the lack of obvious promotion of spatial correlation.
Table 11. Estimated results of the SDM for the ecologicalization of the forestry industry structure.
As the spatial autocorrelation coefficient of the dynamic SDM was not significant, the static SDM model was chosen for effect decomposition. The results (Table 12) indicate that the direct effect coefficient of the coupling coordination between forestry factor endowment and technological progress bias (D) on the ecologicalization of the forestry industry structure was positive but not significant, while the indirect effect coefficient was negative and significant (1% level). This may be attributed to the overall low degree of coupling coordination in China, and the emphasis of the ecologicalization of the forestry industry structure on transitioning towards a greener, low-carbon, and circular economy, as well as restricting the development of highly polluting and energy-intensive forestry industries. This process requires joint promotion from technological progress and policy regulation. However, mismatches in policy regulation may affect the effectiveness of these efforts. Although theoretically, the coupling coordination between forestry factor endowments and technological progress bias should promote the ecological transformation of the industrial structure, the deviation in actual results may be related to the effectiveness and specificity of policy regulation. Effective policy regulation needs to be integrated with technological progress to jointly drive the sustainable development of the forestry industry. However, the current mismatch in policy regulation may result in the failure to achieve the expected outcomes, thus affecting the overall process of ecological transformation.
Table 12. Decomposition results of the effect of static SDM on the ecologicalization of the forestry industry structure.
As for the control variables, the forestry labor productivity (LR) had a significant positive effect on the ecological transformation of both the local forestry industry and neighboring regions (10% level). It promotes the refinement of forestry production division, reducing waste and pollution generation during production processes, thereby enhancing ecological benefits. The average income of forestry personnel (AI) had a significant positive effect on the local forestry industry (10% level), attracting more talents to engage in forestry-related activities, thereby promoting technological innovation and industrial upgrading, leading to more efficient and environmentally friendly production methods. However, national forestry investment (NI) had certain negative effects (1% level), potentially due to mismatches between investment direction and environmental protection priorities, which may impact the effectiveness of forestry industry environmental protection measures. The control rate of forestry pests and diseases (PCR), degree of openness (OPEN), and economic development level (GDP) exhibited significant positive spatial spillover effects (1%, 5% and 1% levels respectively). Local areas achieve green transformation of the forestry industry through resource integration, introduction of green technologies, and deepening cooperation with neighboring regions, facilitating the sharing of resources, technology, and experience, thereby coordinating the green transformation of the forestry industry with neighboring regions. Forestry land productivity (AR) and urbanization rate (UR) had certain negative spatial spillover effects (5% and 1% levels respectively). With the advancement of urbanization, the development space of the forestry industry is constrained, leading to excessive intensive development of forestry land, such as excessive fertilization, pesticide abuse, and intensive planting, which may disrupt soil structure, affecting soil fertility and ecological balance.
From the regional analysis results (Tables 13, 14), The eastern China exhibited a positive effect of coupling coordination on the ecologicalization of the local forestry industry structure, albeit not significant. This region demonstrates relatively superior ecological and resource efficiency, which enables better utilization of resources and consequently enhances ecological benefits (Jiang et al., 2020). In the central China, the natural attributes of the forestry industry are more pronounced. However, there exists a certain degree of imbalance in coupling coordination. This hinders the effective application of technological progress toward enhancing the ecological benefits of the forestry industry. In the western China, there are significant regional disparities among provinces, with notable variations in the demand for and applicability of forestry technologies. Therefore, the central and western China exhibit limited driving force towards the ecologicalization of the forestry industry structure. The northeastern China demonstrates a significantly positive effect (10% level), as the forestry industry embarks on establishing mechanisms for realizing the value of forest ecological products, fostering the development of forest ecological products that yield both economic and ecological benefits (Zhu et al., 2024b). With the elevation of coupling coordination levels, this not only promotes the economic benefits of the forestry industry but also enhances ecological benefits.
Table 13. Estimated results of the ecologicalization of the forestry industry structure in the four regions.
Table 14. Decomposition results of the ecologicalization effect of forestry industry structure in four regions.
To ensure the reliability of the empirical analysis results, we conducted robustness test by changing the sample size and changing the core explanatory variables. First, we shortened the observation period by excluding the first and last few years of the sample data and re-running the regression. Then, we adjusted the calculation method of the core explanatory variables. In the baseline regression model, forestry factor endowment was measured by relative factor endowment (Equation 8). In the robustness test, it was adjusted to factor endowment coefficients, measuring the coupling development level of forestry factor endowment and technological progress bias in different ways, and used as a new core explanatory variable in the regression. For example, the formula for the capital factor endowment coefficient is:
Due to space limitations, the detailed results can be found in the supplementary materials (Supplementary Table A3–8). From the robustness test results, it is observed that while the values in the overall analysis model and regional heterogeneity analysis model fluctuate, the signs and significance levels remain generally stable. Additionally, after addressing the endogeneity issue, we introduced a one-period spatial lag term to construct the dynamic SDM model. Compared to the static SDM model, the regression coefficients’ signs and significance levels were consistent, further confirming the reliability of the empirical analysis conclusions.
This study adopted a spatial perspective and employed panel data from 31 provinces in China spanning from 2005 to 2021 to construct spatial models and investigate the impact of the coupling coordination between forestry factor endowment and technological progress bias on industrial structure upgrading, as well as regional disparities. This study can provide new perspectives and decision-making support for achieving the upgrading of the forestry industry structure and promoting high-quality development. The main conclusions drawn from the analysis are as follows. (1) The overall coupling coordination between forestry factor endowment and technological progress bias in China remained at a relatively low level. Provinces such as Heilongjiang and Xizang, as well as Jiangxi, Jiangsu, and Zhejiang, leveraged their superior forest resource conditions, while Beijing and Shanghai relied on robust economic strength, leading to a higher level of coupling coordination compared to other provinces. (2) There was a significant spatial correlation in the upgrading of the forestry industry structure. Although the competitive effect was more pronounced in the short term, the cumulative effects of structural upgrading drove more significant synergistic effects in the long run. This was conducive to spatial agglomeration of regional forestry industries and achieving coordinated development. (3) The coupled development of forestry factor endowment and technological progress promoted the rationalization and ecologicalization of the industrial structure. However, due to the relatively low level of coupling coordination, it exerted a certain negative impact on advancement. Regionally, the low level of coupling coordination and regional disparities remained key barriers to promoting industrial structural upgrading through technological progress. In the eastern China, coupling coordination played a facilitating role in the advancement and ecologicalization of the industrial structure, with a clear trend in industrial development. Conversely, the influence of coupling coordination on various aspects of the industrial structure in the central and western China was not significant. In the northeast China, there was a promotion effect on the ecologicalization of the industrial structure, but the effect on rationalization and advancement was not evident.
Promoting the coupling coordination between forestry factor endowment and technological progress bias, and driving the upgrading of forestry industry structure, was conducive to achieving high-quality development of China’s forestry economy and advancing the construction of ecological civilization in the new era. Based on the research results above, the following recommendations were proposed. 1) Tailoring the bias of forestry technological progress and the structure of factor allocation according to local conditions. Develop differentiated forestry industry development strategies based on regional disparities and forestry factor endowments, fully leveraging the comparative advantages of different regions, optimizing the forestry industry structure, and promoting high-quality development of the forestry economy. 2) Establishing a sound mechanism for regional forestry development collaboration. Strengthen cooperation and communication between regions, deepen technological exchanges and resource sharing, promote the coordinated development and positive interaction of upstream and downstream industries between regions, and realize the coordinated development of the forestry industry. 3) Promoting forestry scientific and technological innovation and talent development. Encourage forestry scientific and technological innovation to enhance innovation capabilities and technical efficiency. Establish a sound mechanism for talent cultivation to train high-quality forestry management and technical personnel adapted to the needs of the new era. 4) Improving policy frameworks and regulations. Promote cross-departmental policy coordination and integration to strengthen policy guidance and stimulate innovation vitality in forestry enterprises. Deepen supervision of forestry industry development and establish a sound and scientific evaluation system to ensure ecological benefits while enhancing economic benefits.
Although this study examined the impact of the coupling coordination between forestry factor endowment and technological progress bias on industrial structure upgrading, there are still certain limitations. Firstly, due to data constraints, it is difficult to obtain complete micro-level statistical data, and our analysis at the regional level is not yet sufficiently in-depth, which may not fully capture the potential heterogeneity at the municipal or county level. Secondly, this study primarily focused on the impact on forestry industrial structure upgrading, but the coupling coordination between forestry factor endowment and technological progress bias may also influence forestry industry development from other perspectives. Therefore, in the future, we will further focus on specific regions, explore suitable data alternatives, and conduct more micro-level studies. Additionally, we plan to expand the research approach from more angles, incorporating more key variables to enrich research related to the coupling of factors and technology.
The raw data supporting the conclusions of this article will be made available by the authors, without undue reservation.
YJ: Conceptualization, Data curation, Funding acquisition, Methodology, Project administration, Resources, Supervision, Writing–review and editing. YL: Formal Analysis, Validation, Writing–original draft, Writing–review and editing, Methodology. CS: Data curation, Writing–original draft.
The author(s) declare that financial support was received for the research, authorship, and/or publication of this article. This research was funded by the general project of the National Social Science Fund of China, Function Mechanism and Effect Analysis of the Coupling Development between Forestry Factor Endowment and Technological Progress Bias on Industrial Structure Upgrading in China, grant number 20BJY077.
The authors declare that the research was conducted in the absence of any commercial or financial relationships that could be construed as a potential conflict of interest.
All claims expressed in this article are solely those of the authors and do not necessarily represent those of their affiliated organizations, or those of the publisher, the editors and the reviewers. Any product that may be evaluated in this article, or claim that may be made by its manufacturer, is not guaranteed or endorsed by the publisher.
The Supplementary Material for this article can be found online at: https://www.frontiersin.org/articles/10.3389/fenvs.2024.1422480/full#supplementary-material
Abid, N., Marchesani, F., Ceci, F., Masciarelli, F., and Ahmad, F. (2022). Cities trajectories in the digital era: exploring the impact of technological advancement and institutional quality on environmental and social sustainability. J. Clean. Prod. 377, 134378. doi:10.1016/j.jclepro.2022.134378
Acemoglu, D. (2002). Directed technical change. Rev. Econ. Stud. 69 (4), 781–809. doi:10.1111/1467-937X.00226
Acemoglu, D. (2007). Equilibrium bias of technology. Econometrica 75 (5), 1371–1409. doi:10.1111/j.1468-0262.2007.00797.x
Agyeman, D., and Ochuodho, T. (2021). Factor endowment and structural change in Kentucky forest industry. For. Policy Econ. 131, 102558. doi:10.1016/j.forpol.2021.102558
Alvarez-Cuadrado, F., Van Long, N., and Poschke, M. (2018). Capital-labor substitution, structural change and the labor income share. J. Econ. Dyn. Control 87, 206–231. doi:10.1016/j.jedc.2017.12.010
Arabi, B., Munisamy, S., Emrouznejad, A., and Shadman, F. (2014). Power industry restructuring and eco-efficiency changes: a new slacks-based model in malmquist–luenberger index measurement. Energy Policy 68 (1), 132–145. doi:10.1016/j.enpol.2014.01.016
Chen, S., and Zhang, X. (2019). Optimization of regional forestry industrial structure and economic benefit based on deviation share and multi-level fuzzy comprehensive evaluation. J. Intelligent and Fuzzy Syst. 37 (1), 145–157. doi:10.3233/JIFS-179073
Chen, W., Liu, Y., and Liu, Y. (2024). Exploring the spatial spillover effects of climate mitigation policies on the upgrading of industrial structure: evidence from 31 provinces of China. Environ. Challenges 16, 100963. doi:10.1016/j.envc.2024.100963
Chen, X., and Jiang, X. (2014). A study on total factor productivity of Chinese forestry. For. Econ. 36 (03), 75–82. doi:10.13843/j.cnki.lyjj.2014.03.015
Chovancová, B., Dorocáková, M., and Malacka, V. (2018). Changes in the industrial structure of GDP and stock indices also with regard to industry 4.0. Bus. Econ. Horizons 14 (2), 402–414. doi:10.15208/beh.2018.29
Diamond, P. A. (1965). Disembodied technical change in a two-sector model. Rev. Econ. Stud. 32 (2), 161–168. doi:10.2307/2296060
Elhorst, J. P. (2012). Dynamic spatial panels: models, methods, and inferences. J. Geogr. Syst. 14 (1), 5–28. doi:10.1007/s10109-011-0158-4
Gao, J., and Zhang, W. (2021). Research on the impact of green finance on the ecologicalization of China’s industrial structure——empirical test based on system GMM model. Econ. Rev. J. (02), 105–115. doi:10.16528/j.cnki.22-1054/f.202102105
Heathfield, D. F., and Wibe, S. (1987). “The translog function,” in An introduction to cost and production functions (London: Palgrave). doi:10.1007/978-1-349-18721-8_6
Hou, F., Li, X., Wu, C., Yin, Y., and Xiao, H. (2023). The impact of foreign direct investment on the forestry industry structure upgrading: the moderating effect on labor migration. Int. J. Environ. Res. Public Health 20, 2621. doi:10.3390/ijerph20032621
Hu, J., Wu, Y., Irfan, M., and Hu, M. (2023a). Has the ecological civilization pilot promoted the transformation of industrial structure in China? Ecol. Indic. 155, 111053. doi:10.1016/j.ecolind.2023.111053
Hu, L., Yuan, W., Jiang, J., Ma, T., and Zhu, S. (2023b). Asymmetric effects of industrial structure rationalization on carbon emissions: evidence from thirty Chinese provinces. J. Clean. Prod. 428, 139347. doi:10.1016/j.jclepro.2023.139347
Jiang, W., Tang, X., and Liu, J. (2020). Research on ecological efficiency of forestry industry in 13 provinces of south China: spatio-temporal and influence factor. Acta Ecol. Sin. 40 (16), 5663–5673. doi:10.5846/stxb201909031828
Jiang, Y., and Jiang, J. (2021). Spatial influence effect of forestry technology progress on the upgrading of forestry industrial structure. J. Northeast For. Univ. 49 (05), 148–152. doi:10.13759/j.cnki.dlxb.2021.05.026
Jiang, Y., Li, R., and Jiang, S. (2024). The impact of technological progress bias and factor allocation on total factor productivity in forestry in China. Sci. Technol. Manag. Res. 44, 70–80.
Jianmin, W., and Li, Y. (2020). Does factor endowment allocation improve technological innovation performance? An empirical study on the Yangtze River Delta region. Sci. Total Environ. 716, 137107. doi:10.1016/j.scitotenv.2020.137107
Jung, S., Lee, J. D., Hwang, W. S., and Yeo, Y. (2017). Growth versus equity: a CGE analysis for effects of factor-biased technical progress on economic growth and employment. Econ. Model. 60, 424–438. doi:10.1016/j.econmod.2016.10.014
LeSage, J., and Pace, R. K. (2009). Introduction to spatial econometrics. Chapman and Hall/CRC. doi:10.1201/9781420064254
Li, X., and Hu, Q. (2023). A study of transnational convergence and influencing factors of TFP growth: from the perspective of biased technological change. Stat. Res. 40 (05), 37–50. doi:10.19343/j.cnki.11-1302/c.2023.05.003
Lu, Y., and Zhang, C. (2022). The lnteraction of green credit and technology lnnovation on the forestry industry structure in China. Issues For. Econ. 42 (05), 524–531. doi:10.16832/j.cnki.1005-9709.20220170
Lyu, M., Sun, X., and Lyu, Q. (2018). Empirical study of ecological industrial structure endogenously determined by the factor endowment structure-based on the data of 30 provinces in China. Soft Sci. 32 (10), 49–53. doi:10.13956/j.ss.1001-8409.2018.10.11
Ma, Z., Liu, T., Li, J., Liu, Z., and Chen, W. (2023). Spatial effect analysis of forestry technology innovation on forestry industry economic growth. Forests 14 (3), 557. doi:10.3390/f14030557
Murakami, N. (2015). Changes in Japanese industrial structure and urbanization: evidence from prefectural data. J. Asia Pac. Econ. 20 (3), 385–403. doi:10.1080/13547860.2015.1054166
Ren, W., and Zeng, Q. (2021). Is the green technological progress bias of mariculture suitable for its factor endowment? ——empirical results from 10 coastal provinces and cities in China. Mar. Policy 124, 104338. doi:10.1016/j.marpol.2020.104338
Si, L., and Yao, X. (2022). Green finance reform and innovation and the ecologicalization of regional industrial structure. Study and Explor. 2022 (04), 129–138+2. doi:10.3969/j.issn.1002-462X.2022.04.018
Smith, R., Sengupta, D., Takkellapati, S., and Lee, S. (2015). An industrial ecology approach to municipal solid waste management: I. Methodology. Resour. Conservation Recycl. 104, 311–316. doi:10.1016/j.resconrec.2015.04.005
Song, W., and Yang, C. (2020). Forestry industrial structure, spatial distribution and evolution mechanism since 1949. For. Econ. 42 (06), 3–17. doi:10.13843/j.cnki.lyjj.20200911.006
Su, Y., and Fan, Q. (2022). Renewable energy technology innovation, industrial structure upgrading and green development from the perspective of China’s provinces. Technol. Forecast. Soc. Change 180, 121727. doi:10.1016/j.techfore.2022.121727
Tang, Z., and Li, H. (2017). An empirical analysis of the impact of urbanization on the optimization of forestry industry structure. Res. Agric. Mod. 38, 226–233. doi:10.13872/j.1000-0275.2017.0007
Wang, D., Du, M., and Yu, Z. (2023). Technological progress orientation and total factor productivity growth of high-tech industry in China. J. Hebei Univ. Econ. Bus. 44 (01), 55–66. doi:10.14178/j.cnki.issn1007-2101.20221230.004
Wang, L., Su, M., Kong, H., and Ma, Y. (2021). The impact of marine technological innovation on the upgrade of China’s marine industrial structure. Ocean and Coast. Manag. 211, 105792. doi:10.1016/j.ocecoaman.2021.105792
Wu, H., Liu, X., and Su, Z. (2021). Structural change and productivity growth driven by biased technological progress. Shanghai J. Econ., 45–59. doi:10.19626/j.cnki.cn31-1163/f.2021.03.005
Wu, N., and Liu, Z. (2021). Higher education development, technological innovation and industrial structure upgrade. Technol. Forecast. Soc. Change 162, 120400. doi:10.1016/j.techfore.2020.120400
Xiang, H., Chen, Z., Liao, W., Ai, J., and Zhang, M. (2021). Analysis on driving mechanism of forestry development and influencing factors of technical efficiency in collective forest regions in south China. For. Econ. 43 (08), 5–21. doi:10.13843/j.cnki.lyjj.20211021.002
Xiong, L., Wang, F., and Cheng, B. (2018). The optimization of forestry industrial structure and its influencing factors in China. Res. Agric. Mod. 39 (3), 378–386. doi:10.13872/j.1000-0275.2018.0023
Xue, C., and Zhou, H. (2019). Regional disparities analysis of the alignment between agricultural technological progress direction and factor structure in China. Statistics and Decis. 35, 136–140. doi:10.13546/j.cnki.tjyjc.2019.05.030
Yang, L., Gong, S., Wang, B., and Chao, Z. (2018). Human capital, technology progress and manufacturing upgrading. China Soft Sci. 1, 138–148. doi:10.3969/j.issn.1002-9753.2018.01.013
Yang, T., Sun, Z., Du, M., Du, Q., Li, L., and Shuwaikh, F. (2023). Correction: the impact of the degree of coupling coordination between green finance and environmental regulations on firms’ innovation performance: evidence from China. Ann. Operations Res. 332, 1179–1180. doi:10.1007/s10479-023-05704-9
Yao, P., and Ma, Z. (2022). Non-tax autonomy and upgrading of department structure: unsustainable exploitation or improvement of long standing? J. Finance Econ. 48 (09), 124–138. doi:10.16538/j.cnki.jfe.20220518.401
Ye, J., Ai, W., and Shi, Q. (2024). The impacts of green policy on grain technology progress and factor endowment coordinated development. Chin. J. Agric. Resour. Regional Plan. 2024 (07), 39–53. doi:10.7621/cjarrp.1005-9121.20240704
Yuan, D., Du, M., Yan, C., Wang, J., Wang, C., Zhu, Y., et al. (2024). Coupling coordination degree analysis and spatiotemporal heterogeneity between water ecosystem service value and water system in Yellow River Basin cities. Ecol. Inf. 79, 102440. doi:10.1016/j.ecoinf.2023.102440
Zang, L., Zhi, L., and Guo, X. (2014). Research on calculation and dynamic evolution of forestry production technical efficiency in western China. J. Statistics Inf. 29 (01), 13–20. doi:10.3969/j.issn.1007-3116.2014.01.003
Zheng, Y. (2023). How can China avoid the middle-technology trap? Bull. Chin. Acad. Sci. Chin. Version 38 (11), 1579–1592. doi:10.16418/j.issn.1000-3045.20230804001
Zhou, X., Hu, Q., Luo, H., Hu, Z., and Wen, C. (2024). The impact of digital infrastructure on industrial ecology: evidence from broadband China strategy. J. Clean. Prod. 141589, 141589. doi:10.1016/j.jclepro.2024.141589
Zhu, H., Cao, B., and Zhang, X. (2024a). From forest dependence to value realization of forest ecological products: development path of northeast state-owned forest areas in China. World For. Res. 37 (01), 16–22. doi:10.13348/j.cnki.sjlyyj.2023.0093.y
Keywords: forestry factor endowment, technological progress bias, rationalization of the forestry industrial structure, advancement of the forestry industrial structure, ecologicalization of the forestry industrial structure
Citation: Jiang Y, Liao Y and Shen C (2024) Spatial impact effects of coupled coordination between forestry factor endowment and technological progress bias on forestry industry structural upgrading in China. Front. Environ. Sci. 12:1422480. doi: 10.3389/fenvs.2024.1422480
Received: 24 April 2024; Accepted: 18 November 2024;
Published: 28 November 2024.
Edited by:
Quande Qin, Shenzhen University, ChinaReviewed by:
Ailiang Xie, Linyi University, ChinaCopyright © 2024 Jiang, Liao and Shen. This is an open-access article distributed under the terms of the Creative Commons Attribution License (CC BY). The use, distribution or reproduction in other forums is permitted, provided the original author(s) and the copyright owner(s) are credited and that the original publication in this journal is cited, in accordance with accepted academic practice. No use, distribution or reproduction is permitted which does not comply with these terms.
*Correspondence: Yu Jiang, amlhbmd5X2xqQG5lZnUuZWR1LmNu
Disclaimer: All claims expressed in this article are solely those of the authors and do not necessarily represent those of their affiliated organizations, or those of the publisher, the editors and the reviewers. Any product that may be evaluated in this article or claim that may be made by its manufacturer is not guaranteed or endorsed by the publisher.
Research integrity at Frontiers
Learn more about the work of our research integrity team to safeguard the quality of each article we publish.