- 1Natural Resources Survey Institute of Heilongjiang Province, Harbin, China
- 2Economics and Management College, China University of Geosciences, Wuhan, China
Green innovation possesses dual externalities of “innovation” and “environmental protection”, and enhancing energy efficiency serves as a crucial means to promote high-quality economic development. Building upon the energy rebound effect, we use the balanced panel data of cities at prefecture level and above in China from 2008 to 2018 to explore the impact of urban green innovation on total factor energy efficiency (TFEE). The findings of this study indicate that, firstly, the impact of green innovation on TFEE exhibits a positive U-shaped pattern, characterized by initial suppression followed by subsequent promotion. This conclusion remains robust after undergoing a series of rigorous robustness tests. Second, high-quality green innovation is found to reach the turning point more quickly, implying that substantial green innovation can cross the turning point in smaller quantities. Thirdly, on the whole, in comparison to non-resource-based cities, resource-based cities are capable of reaching the turning point at an earlier stage. Finally, the new energy demonstration cities have not yet reached the turning point, while the non-new energy demonstration cities have crossed the turning point. This study contributes novel insights into the relationship between green innovation and TFEE, which holds significant implications for the formulation and implementation of sustainable development policies.
1 Introduction
In recent years, global climate change has garnered increasing attention, leading to a growing consensus on the importance of energy conservation and emissions reduction (Guo et al., 2023a; Guang et al., 2023). As China transitions into a phase of high-quality economic development, the conventional model characterized by “high energy consumption, high pollution, and low efficiency” has become unsustainable (Liu et al., 2023; Wu et al., 2024a). Notably, the Chinese government has committed to international objectives, targeting a carbon peak by 2030 and carbon neutrality by 2060, known as the “dual carbon” goals. Against this backdrop, improving energy efficiency has emerged as a key strategy for China to realize these goals (IEA, 2021; Zhao et al., 2022). Furthermore, the 14th Five-Year Plan explicitly sets forth the development goal of “more rational allocation of energy resources and substantial improvement in energy utilization efficiency”. However, given China’s continued prioritization of economic development and the persistent dominance of coal in its energy consumption landscape, achieving these goals presents significant challenges (Ren et al., 2022; Xu and Lin, 2022). Unlike developed nations, China’s process of industrialization and urbanization remains incomplete, further complicating efforts to meet the “dual carbon” goals within a short timeframe (Zor, 2023a; Cui et al., 2023). As the world’s largest energy consumer and carbon emitter, the Chinese government faces unprecedented pressure to implement measures for energy conservation and carbon reduction (Tang, et al., 2023; Wu et al., 2024b; Tang et al., 2024).
Addressing the urgent need to enhance energy efficiency for carbon emission reduction has become a pivotal concern for China to uphold its international competitiveness (Wang et al., 2019). The historical pursuit of GDP growth rate has shifted, with China’s economy progressively transitioning towards green and low-carbon development (Tang et al., 2022; Zor, 2023b; Jiang and Tang, 2023). The 20th National Congress further emphasizes the need to accelerate the green transformation of development patterns and promote the formation of green and low-carbon production modes. The pursuit of green development is increasingly gaining consensus across all societal sectors and governmental levels, constituting a vital driver for refining the energy mix and a focal point of academic inquiry (Guang et al., 2022). Despite the Chinese government’s significant emphasis on green development, the persistent challenge of reconciling the reduction of energy intensity with the rise in total energy consumption remains conspicuous. Navigating the transition away from traditional development models and propelling cities towards a green development trajectory has emerged as a critical agenda under the “dual carbon” goals (Bu et al., 2022; Gao et al., 2022). Presently, the emerging drivers of economic growth increasingly hinge on the efficient allocation of resources, necessitating the judicious utilization of energy resources. Green innovation, as a distinctive form of innovation, effectively harmonizes economic development with environmental conservation, serving as a pivotal mechanism for bolstering energy efficiency (Zhou and Qi, 2022).
The beneficial impacts of green innovation are multifaceted, with empirical evidence suggesting that it can contribute to heightened energy efficiency. Green innovation has the potential to foster the development of green and low-carbon production methods and lifestyles, curbing resource consumption, and fostering cleaner and more efficient production processes (Guo et al., 2023b). Additionally, it can bolster production technologies, diminish reliance on fossil fuels in production activities, thereby curbing energy consumption and augmenting energy efficiency. However, recent studies have indicated that green innovation may be a “double-edged sword” (Chen et al., 2023), and its impact on energy efficiency is not always positive. The energy rebound effect posits that in the short term, the cost reductions resulting from green innovation may spur an uptick in energy consumption, countering some of the intended energy-saving and emission-reducing benefits. Since green innovation activities are characterized by greater randomness (Noailly and Smeets, 2015; Xiang et al., 2022), large investments and low returns (Ren et al., 2021), there is a possibility that they may crowd out productive expenditures, ultimately undermining the improvement of energy efficiency. Moreover, considering that the positive externalities of green innovation are difficult to internalize in the short term, and environmental performance does not dominate the promotion process of local officials (Jiang et al., 2020; Tang et al., 2021). Local governments are often reluctant to invest excessive time and effort in green innovation, leading to a weakened supportive role of green innovation in energy efficiency.
Research on the relationship between green innovation and energy efficiency is one of the hot topics in the academic community. This paper measures total factor energy efficiency (TFEE) at the city level in China using the non-desired output super-efficiency Slack Based Measure model, and demonstrates the spatial and temporal distribution characteristics of TFEE using Arcgis software. Based on balanced panel data of 284 prefecture-level and above cities from 2008 to 2018, this paper empirically tests the impact of green innovation on urban TFEE using a two-way fixed effects model. The findings suggest that: (1) When the level of green innovation is low, it imposes a burden on TFEE. However, when urban green innovation reaches a certain level, green innovation is beneficial to improve TFEE. And this conclusion is quite robust. (2) Compared to strategic green innovation, substantive green innovation can reach the turning point faster and promote TFEE. (3) Overall, the observations of resource-based cities are evenly distributed on both sides of the symmetry axis. The level of green innovation in non-resource-based cities has not yet reached the turning point, but the turning point comes faster in resource-based cities. (4) The sample of new energy demonstration cities generally falls on the left side of the symmetry axis, while the opposite is observed for non-new energy demonstration cities. Furthermore, the turning point of non-new energy demonstration cities is significantly smaller than that of new energy demonstration cities.
Compared to existing studies, the potential contributions of this paper are as follows: First, this paper responds to the focus on energy rebound effects in the resource and environmental economics literature. Unlike previous studies that focus on measuring energy rebound effect (Belaid et al., 2020; Du et al., 2021; Chen et al., 2022), we validate the energy rebound effect in the Chinese context based on a city-level sample. We find a U-shaped relationship between green innovation and TFEE, thus revealing the “black box” of how green innovation affects TFEE. Second, this paper echoes the recent literature that focuses on the economic and environmental effects of urban green innovation (Singh et al., 2020; Chen et al., 2023; Bianchini et al., 2023), thus expanding on the non-economic performance of urban green innovation. By doing so, this research not only enriches our understanding of the multifaceted outcomes of urban green innovation but also broadens our perspective beyond its traditional economic dimensions. Therefore, our paper enriches the existing research on the consequences of green innovation activities. Lastly, our study extends the understanding of energy rebound effect from the resource dependence perspective, we examine the moderating role of resource dependence on the relationship between green innovation and TFEE. This study enriches the resource curse theory and, in turn, provide valuable insights for guiding both resource-dependent and non-resource-dependent cities in improving TFEE. By studying this urban character, the research sheds light on the role of resource dependency in shaping the relationship between green innovation and TFEE.
The remainder of the paper is organised as follows: Section 2 provides the literature review; Section 3 outlines the research design, including sample selection, data collection, model building and variable definition; Section 4 presents the empirical results; Section 5 provides further analysis; and Section 6 summarises the findings and implications of the paper.
2 Literature review
Green innovation has important practical significance for improving energy efficiency and achieving green economic development. The literature closely related to the research topic of this article mainly includes the following two branches:
2.1 The economic and environmental effects of green innovation
In recent years, there has been considerable academic interest in green innovation as an effective strategy for simultaneously pursuing economic growth and environmental protection. Scholarly discourse has predominantly focused on analyzing the outcomes of green innovation, particularly in terms of its economic and environmental impacts.
Numerous prior literature reviews have explored the correlation between green innovation and economic performance, yet definitive conclusions remain elusive. The “compliance cost hypothesis” theoretically argues that achieving concurrent economic performance and environmental preservation is arduous. This challenge arises due to the low return rate, extended return cycle, and substantial investment associated with green innovation. Additionally, green innovation frequently involves local governments responding passively to environmental regulatory pressures imposed by higher-level governmental (Ren et al., 2021). In scenarios marked by resource constraints, the engagement in green innovation activities necessitates local governments to redirect a portion of their existing resources away from conventional production towards initiatives aimed at environmental preservation. This redirection disrupts the initial deployment of resources within the city’s production framework, curbing industrial production activities and thereby instigating a “crowding out effect” on economic performance (Pan et al., 2019). Therefore, the allocation of resources by local governments towards environmental protection inevitably crowds out productive investments in the city, ultimately impeding the improvement in economic performance.
The “Porter Hypothesis” proposes a completely different viewpoint from “compliance cost hypothesis” (Porter and Van der Linde, 1995). According to the “Porter Hypothesis”, green innovation can optimize resource allocation efficiency, thereby exerting a positive impact on economic performance. To address the ever-increasing pressure on resources and the environment, governments can implement proactive green innovation development strategies to enhance the overall competitiveness of cities. Green innovation serves as an effective solution to promote clean production and enhance production efficiency, which can further enhance the economic sustainability of cities (Yan et al., 2020). Furthermore, green innovation substantially diminishes societal production costs through the adoption of environmentally sustainable technologies, optimization of production processes, and enhancement of product designs. Additionally, green industries represent a pivotal trajectory for future development, offering the potential to generate a plethora of employment opportunities and catalyze economic advancement within urban areas. Cities that prioritize green innovation often enjoy a pronounced advantage in attracting skilled personnel and investments, thereby yielding significant benefits for bolstering economic performance. Within this framework, involvement in green innovation endeavors does not signify a trade-off between economic performance and environmental preservation. Rather, it has the potential to foster a mutually beneficial outcome for both ecological integrity and economic prosperity (Huang et al., 2021; Zhao et al., 2022b).
Indeed, the academic community has produced numerous studies examining the environmental performance of green innovation. Positioned as a pivotal approach toward sustainable development, green innovation is widely acknowledged as a foundational strategy for fostering high-caliber development. With the global promotion of the concept of sustainable development, green innovation has become an inevitable choice for achieving a “win-win” situation of economic growth and environmental protection (Yildirim et al., 2022). As a critical means to achieve the dual carbon goals, green innovation can help break away from traditional economic development model and steer the economy towards a green and low-carbon direction. It goes without saying that green innovation has become an important way to overcome resource and environmental constraints and achieve sustainable development. On the one hand, green innovation contributes to promoting clean production, reducing carbon emissions, and mitigating global climate change (Sun et al., 2021; Xin et al., 2021; Sharif et al., 2022). On the other hand, green innovation facilitates the efficient utilization of energy resources, reducing resource consumption and environmental pollution (Ulucak and Baloch, 2023). However, green innovations do not always help to improve environmental performance (Du and Li, 2019), and low quality and low quantity green innovations even exacerbates to environmental pollution (Mongo et al., 2021).
2.2 Energy efficiency related research
Energy efficiency plays an indispensable role in the cost-benefit analysis of energy conversion. Researchers have extensively delved into the exploration of energy efficiency, aiming to comprehend and optimize energy utilization. The research at hand encompasses two primary dimensions: firstly, what methods are used to accurately measure energy efficiency; the second is to explore various influencing factors of energy efficiency. By delving into these issues through profound investigation, valuable theoretical support and practical guidance can be provided to enhance energy utilization efficiency and promote sustainable development.
There are two main approaches to assessing energy efficiency in academia, namely, single-factor energy efficiency and total factor energy efficiency. The benefits of single-factor energy efficiency are simple methods, specific results, and the ability to intuitively reflect the impact of energy consumption on economic output. In earlier academic research, single-factor energy efficiency emerged as a widely employed indicator (Petrovic et al., 2018; Shao et al., 2019). However, the indicator only treats energy consumption as the only input to economic output, ignoring the substitution effects between different input factors. Therefore, the energy efficiency measured by this indicator is not comprehensive and accurate enough to fully reflect the changes in energy efficiency as well as energy consumption (Duro, 2015). From this, it can be seen that the single-factor energy efficiency indicator has obvious shortcomings, and cannot be served as the sole standard for comprehensively measuring energy efficiency.
As research on energy efficiency continues to grow, more scholars are focusing on and introducing TFEE. TFEE can better evaluate the combined impact of energy consumption and other input factors on economic output, and largely compensates for the inadequacy of single-factor energy efficiency (Gerarden et al., 2017; Li et al., 2021). Although TFEE is more realistic and accurate, however, the steps and procedures for measuring TFEE are more complex than for single-factor energy efficiency. Currently, the measurement methods for TFEE primarily involve two approaches: stochastic frontier analysis (SFA) and data envelopment analysis (DEA) (Guo et al., 2023). The larger the measurement result obtained, the higher the utilization efficiency of energy and other input factors. Compared to the traditional single-factor energy efficiency, TFEE incorporates factors such as capital and labor into the evaluation framework, thus taking into account the substitution relationships among various input factors comprehensively (Proskuryakova and Kovalev, 2015). In recent years, with the intensification of resource and environmental constraints, and improvements in measurement methods, some scholars have pointed out that energy efficiency that ignores environmental pollution is inaccurate and incomplete. As a result, an increasing number of scholars have started to consider incorporating environmental constraint indicators as undesirable outputs into the evaluation framework, aiming to avoid overestimating energy efficiency.
The factors influencing energy efficiency have been one of the hot topics in academia, encompassing multiple aspects such as economic, technological, and policy. In recent years, scholars have revealed the drivers of energy efficiency from different perspectives, such as technological progress (Paramati et al., 2022), environmental regulation (Wu et al., 2020), industrial agglomeration (Tanaka and Managi, 2021), internet development (Wu et al., 2021), economic globalization (Liu et al., 2023), and energy prices (Jacobsen, 2015). Undoubtedly, technological progress is one of the most critical factors affecting energy efficiency. Generally, technological progress tends to lower energy utilization costs, the constant updating of technologies provides additional means and possibilities for improving energy efficiency. However, while focusing on the significant role of technological progress in enhancing energy efficiency, the energy rebound effect caused by technological progress should not be ignored as well (Sun et al., 2019). Meanwhile, considering that the market mechanism often fails to achieve the expected effect, the environmental regulation measures issued by the government can correct market failure to a certain extent (Liu et al., 2023). Environmental regulations not only help promote technological innovation, but also guide enterprises to adopt energy-saving measures by providing incentives (Wang et al., 2022). In addition, the rise in energy prices will also guide enterprises and society to pay more attention to energy conservation and utilization efficiency, thereby improving TFEE (Guo and Liu, 2022).
Figure 1 briefly depicts the framework of this paper. The relationship between green innovation and TFEE is the focus of our attention. On this basis, we explore the heterogeneity of the impact of green innovation on TFEE from the perspectives of innovation and cities.
3 Research design
3.1 Sample selection and data source
The data on urban green patent applications is sourced from the CNRDS database. The PM2.5 data for calculating TFEE comes from the Atmospheric Composition Group at Columbia University. Other data are obtained from the China Urban Statistical Yearbook, China Urban Construction Statistical Yearbook, China Energy Statistical Yearbook, EPS database and provincial and municipal statistical yearbooks. Ultimately, the authors compile a balanced panel data for 284 prefecture-level and above cities in China from 2008 to 2018.
3.2 Variable definitions
3.2.1 Dependent variable
Traditional data envelopment analysis (DEA) is not sufficiently flexible when dealing with inputs and outputs, i.e., it does not take into account “slack variables”, which leads to a certain degree of bias in the measured results compared to the actual situation. To overcome the shortcomings of traditional DEA methods, Tone (2001) proposes the super-efficiency Slack Based Measure model that takes slack variables into account. By adding slack variables, it effectively solves the problem that the traditional DEA model cannot deal with non-desired output and the error caused by radial and angular, and breaks through the limitation that the optimal value of efficiency is 1. In recent years, the environmental pollution caused by human activities has received increasing attention, and it is necessary to consider non-desired outputs when evaluating TFEE. Hence, this study adopts the super-efficiency Slack-Based Measure model that incorporates non-desired outputs to calculate TFEE. Referring to Zhao et al. (2022a), this article sets up the following evaluation model:
In the above equation, assuming there are n decision-making units, any decision-making unit has m inputs, s kinds of desired outputs, and q kinds of non-desired outputs. x, yg and yb are the input, desired output and non-desired output matrices, respectively. pk is the measured efficiency value.
The input-output indicators selected in this paper are shown in Table 1. Input indicators include labor input, capital input and energy input. The desired output indicator is the urban GDP. As for the non-desired output, in addition to taking into account the three industrial wastes, we also include PM2.5 in the evaluation system. This is mainly because haze pollution has had profound and far-reaching impacts on various aspects, including human health, ecological environment, and economic development in recent years (Chen et al., 2023).
3.2.2 Independent variable
Patents are considered as one of the important indicators for measuring technological innovation, it is common academic practice to use the number of green patents to indicate urban green innovation. The number of green patents serves as an objective measure that can reflect the overall level of a city’s green innovation. Compared to the number of granted patents, patent application data is considered to be more stable, reliable, and timely. Like most literature (e.g., Xu et al., 2021; Fang, 2023), this study utilizes the number of green patent applications at the city level (the sum of green invention patents and green utility model patents) to measure green innovation. Ultimately, Gi is expressed as the natural logarithm of the number of green patent applications.
3.2.3 Control variables
To eliminate the influence of other important factors on the estimation results. Referring to Wang et al. (2022) and Hao et al. (2022), this paper also controls for the following factors in the regression model. Economic development level (Gdpr), this paper uses urban GDP growth rate to measure the regional economic development level, faster economic growth can promote the development of energy saving and emission reduction technologies. Foreign trade (Fdir), measured by the ratio of the actual amount of foreign investment used to GDP, and the technology spillover effects and economies of scale from foreign trade are usually beneficial in reducing energy consumption. Population density (Pden), Population gathering increases energy demand, this article uses the ratio of the total population at the end of the year to the administrative area to represent population density. The size of science expenditure (Scir), measured as the ratio of urban science expenditure to GDP. Industrial structure (Second), measured by the proportion of value added by the secondary sector to GDP, a higher proportion indicates that there may be higher energy consumption. Environmental regulation (Er), similar to Chen et al. (2018), is represented by the proportion of environmental-related vocabulary in the urban government’s work report.
3.3 Research model
To examine the impact of green innovation on TFEE, the following two-way fixed effects model is constructed:
Among them, where TFEEit represents the energy efficiency of city i in year t; i, t represent the city and the year, respectively; Giit indicates urban green innovation; Controlit denotes a set of urban characteristics; μi and νt represent the city and time fixed effects; εit is the error term. α is a constant term; β1 and β2 are the coefficients.
4 Empirical results
4.1 Descriptive statistics
Table 2 shows the variable definitions and descriptive statistics. For both the independent and dependent variables, their means are slightly greater than the medians, and they exhibit smaller standard deviations. This indicates that the data distribution is relatively even and approximately follows a normal distribution.
4.2 The spatial and temporal patterns of TFEE in Chinese cities
To visually display the spatiotemporal distribution of TFEE in Chinese cities, we utilized ArcGIS software to create spatiotemporal distribution maps. As depicted in Figure 2, this study showcases the spatial distribution of urban TFEE for the years 2008, 2011, 2015, and 2018, respectively. It is evident that there are significant differences in TFEE among various cities in China, and the distribution is not uniform. Overall, there are more cities with higher TFEE in the eastern region than in the western and central regions. Compared to cities in northern China, cities in southern China generally exhibit higher levels of TFEE. In 2008, the regions with lower TFEE are mainly located in Gansu, Guangxi, Guangdong and Northeast China, while the cities with higher TFEE are scattered. In 2018, cities with higher levels of TFEE are more concentrated in regions such as the Beijing-Tianjin-Hebei area, the Shandong Peninsula, and the Yangtze River Delta.
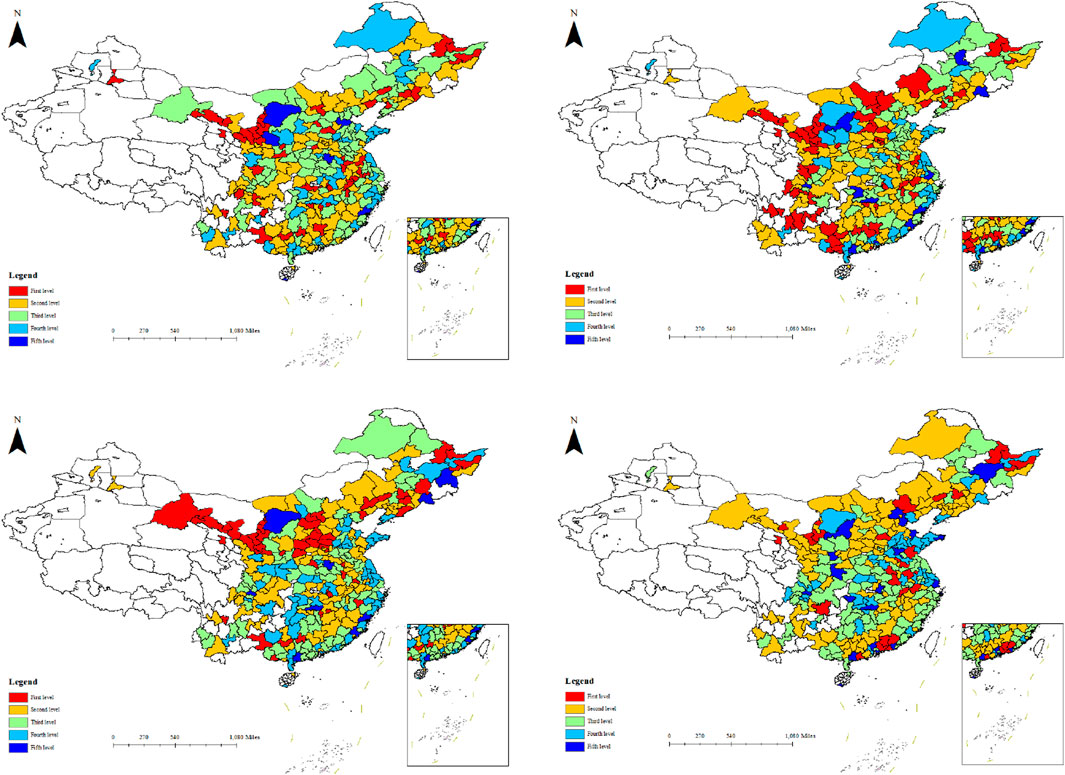
Figure 2. The spatial and temporal distribution of TFEE in Chinese cities. Note: The representative years, from left to right and top to bottom, respectively, are 2008, 2011, 2015, and 2018. The base map is sourced from the Standard Map Service System of the Ministry of Natural Resources, and the base map review number is GS (2019) 1822.
4.3 Baseline results
The benchmark regression results are presented in Table 3. Column (1) shows the effect of green innovation when no regional characteristics are included. In this case, the coefficient of Gi is significantly negative at the 1% level, while the coefficient of Gi2 is significantly positive at the 1% level. When we include all control variables in the regression model, the result is presented in column (2) of Table 3. The results demonstrate a positive U-shaped relationship between green innovation and TFEE, characterized by an initial inhibition followed by subsequent promotion. In other words, the energy rebound effect of green innovation has been preliminarily tested. Specifically, the turning point of the U-shaped relationship is 4.6279, corresponding to a green patent number of 102. The economic implication is that when the number of urban green patent applications is below 102, green innovation reduces TFEE. When the number of urban green patent applications exceeds 102, green innovation can significantly improve TFEE. This result suggests that for cities with lower green innovation capacity, it is necessary to further expand investment in innovation, especially in terms of expenditure on green innovation.
However, it is not appropriate to solely rely on the significance of Gi2 to conclude the presence of a U-shaped relationship between green innovation and TFEE. Lind and Mehlum (2010) argue that such a criterion is weak or even erroneous. Lind and Mehlum (2010), Haans et al. (2016) have provided a set of steps for testing U-shaped relationships. Step 1, the coefficients of the quadratic terms must be significant, and in the same direction as expected by the theory. Step 2, at the left and right endpoints of the U-shaped relationship, the slope must be significant and the sign must be opposite. Step 3, the turning point of the U-shaped relationship falls within the range of the independent variable. According to Lind and Mehlum (2010), the U-shaped relationship between green innovation and TFEE is only valid when all three conditions mentioned above are simultaneously met.
The empirical result in column (2) of Table 3 shows that the first step holds. Subsequently, we employ the “utest” command developed by Lind and Mehlum (2010) to examine the second step. We find slopes of −0.0398 and 0.0477 for the left and right endpoints of the independent variable, respectively, and corresponding t-values of −3.6563 and 2.4644, respectively. This shows that the second step also holds. Finally, according to the descriptive statistics in Table 2. It is apparent that the inflection point (4.6279) of the U-shaped relationship is located between the minimum and maximum values of the independent variable. Thus, the third step also holds. The U-shaped relationship between green innovation and TFEE can be verified.
4.4 Robustness checks
In addition, this paper also performs other robustness tests to demonstrate the robustness of the baseline regression results. In column (1) of Table 4, the authors employ the number of urban green patent grants as a robustness check for substituting explanatory variables. In the baseline regression, we utilize the TFEE computed from the non-desirable output super-efficiency Slack Based Measure model as the dependent variable. In column (2) of Table 4, the authors employ single-factor energy efficiency (the ratio of GDP to energy consumption) as a robustness check to substitute the dependent variable. In this case, the coefficient of Gi2 remains significantly positive. Although we control for numerous regional-level control variables, there may still be other regional factors bias our results. Therefore, following Yuan and Zhang (2015), we include province-year interaction fixed effects in the model to control for time-varying and non-time-varying province characteristics. The results is presented in column (3) of Table 4.
Finally, unlike other cities in China, Beijing, Shanghai, Tianjin, and Chongqing hold a “special status” as they are directly administered by the central government. The administrative level of municipalities is higher than that of ordinary cities, and the municipalities are able to receive more attention and importance from the central government. Compared to general cities, municipalities directly under the central government consume more energy. Therefore, the four municipalities were removed from the sample in this paper and the regression test was re-run according to the baseline regression model. The result is shown in column (4) of Table 4.
4.5 Endogenous analysis
In this paper, the endogeneity is a fatal issue when discussing the impact of green innovation on TFEE. That is, there may be an endogenous problem between green innovation and TFEE, which can seriously interfere with the estimation results. Although this paper controls a range of control variables that may affect TFEE, many other macroeconomic factors may also influence TFEE. Therefore, there may be endogenous problems caused by omitted variables in the econometric model. In view of this, our paper employs the instrumental variable approach, which is widely used in academia, to alleviate the authors’ concerns regarding the endogeneity.
To be specific, the authors try to alleviate endogenous problems by using two-stage least squares (2SLS) regression. In fact, a perfect instrumental variable is the “God’s gift”. It is common practice in empirical studies to use lagged term of independent variable as instrumental variable to mitigate endogeneity (e.g., Zhao and Wang, 2022; Chen et al., 2023). Therefore, we employ lagged one-period (Gi_1) and lagged two-period green innovation (Gi_2) as instrumental variables. On the one hand, Gi_1 and Gi_2 are highly correlated with Gi. On the other hand, the TFEE of the current period does not affect Gi_1 and Gi_2. The estimation results of 2SLS are shown in Table 5. The first stage F-value is greater than 10, and the weak instrumental variable problem can be excluded. In the second stage, TFEE is used as the dependent variable. The U-shaped relationship still holds. Thus, the benchmark regression results remain robust after mitigating the endogeneity.
5 Further analysis
5.1 Substantive vs. strategic green innovation
The baseline regression result indicates a positive U-shaped relationship between green innovation and TFEE. Considering the differences in the motivation of green innovation agents to undertake green innovation activities, do different types of green innovation show significant differences in their impact on TFEE? Based on this consideration, following Zhang et al. (2022), we categorize green invention patents as substantive green innovation and green utility model patents as strategic green innovation. Strategic green innovation refers mainly to strategic actions taken to meet government policies on environmental protection. These strategies may be innovations that appear green on the surface but are actually just marketing tools. In contrast to strategic green innovation, substantive green innovation is high-quality green innovation behaviour aimed at gaining competitive advantage and environmental performance. The focus of substantive green innovation is on environmental protection and economic efficiency through technical and managerial means.
Columns (1)–(2) of Table 6 present the regression results for substantive green innovation and strategic green innovation, respectively. Regardless of whether it is substantive green innovation or strategic green innovation, the impact on TFEE shows a U-shaped relationship. However, the difference is that the extreme value point for substantive green innovation (23) is much smaller than the extreme value point for strategic green innovation (104). This suggests that substantial green innovation with high innovation capacity can reach the turning point earlier, and achieve the effect of promoting TFEE. Unsurprisingly, substantive green innovation is more concerned with transformative innovation in products or technologies. The success of substantive green innovation can generate greater benefits for the environment, businesses, and society as a whole. In practice, companies and governments can focus on raising the level of substantive green innovation, and adopting more environmentally friendly and efficient technologies to achieve the goals of sustainable development and energy saving and emission reduction.
5.2 Resource-based cities vs. non-resource-based cities
China is endowed with abundant energy resources, and there are significant differences in the energy resource endowments among different regions. A good natural resource endowment is a tremendous advantage for the sustainable development of resource-based cities, and the most basic feature that distinguishes resource-based cities from non-resource-based cities. Typically, the resource-based cities mainly rely on natural resource development and processing industries, such as oil, coal and metal minerals, to develop their economies. In contrast, the non-resource-based cities rely on other industries such as manufacturing and services. As a result, the industrial structure and economic development paths of the two types of cities are distinctly different. Naturally, then, we want to ask whether the impact of green innovation on TFEE differs significantly between resource-based and non-resource-based cities?
Following the “National Sustainable Development Plan for Resource-Based Cities (2013–2020)”, we categorize the samples into two groups: resource-based cities and non-resource-based cities. Columns (3)–(4) of Table 6 present the regression results for resource-based cities and non-resource-based cities, respectively. There is a significant U-shaped relationship between green innovation and TFEE for both resource-based and non-resource-based cities. However, the extreme value point for resource-based cities (51) is much smaller than that for non-resource-based cities (612). This indicates that, in comparison to non-resource-based cities, resource-based cities have a significantly lower level of green innovation capability. For resource cities, the median (51) is exactly equal to the extreme value point, indicating that the sample is evenly distributed on both sides of the symmetry axis. For non-resource-based cities, the median value of green innovation (145) is smaller than the turning point, indicating that the majority of the observations are located on the left side of the symmetry axis. It is not difficult to explain that resource-based cities are mostly small and medium-sized cities, with a single economic structure and insufficient economic development potential. In addition, the development of resource-based cities has long relied on the extraction and processing of resources, and the demand for green technology innovation is relatively low, resulting in a lack of urban innovation capacity and competitiveness.
5.3 New energy demonstration cities vs. non-new energy demonstration cities
To promote the research and development, application, and promotion of new energy technologies, and to play the key role of renewable energy in adjusting energy structure and building ecological civilization. In January 2014, the National Energy Administration of China announced the first batch of new energy demonstration cities (industrial parks). This measure aims to reduce the reliance of cities on fossil fuels, increase the proportion of new energy in urban energy consumption, and enhance the sustainable development capacity of cities. Meanwhile, recent empirical studies have shown that new energy demonstration cities significantly improve the energy efficiency of pilot cities (Cheng et al., 2023). Based on this, it is natural to question whether the impact of green innovation on TFEE differs significantly between pilot cities and non-pilot cities.
The columns (5)–(6) of Table 6 show the results for pilot and non-pilot cities. Similarly, whether for new energy demonstration cities or non-demonstration cities, the U-shaped relationship between green innovation and TFEE holds. The difference is that the extreme points in the pilot cities (565) are much larger than those in the non-pilot cities (68). Compared to non-demonstration cities, the turning point in demonstration cities occurs earlier, indicating that the level of green innovation is higher in the pilot cities. For the pilot cities, the median green innovation (118) is smaller than the extreme value point, indicating that most of the observations are located on left of the symmetry axis. For non-pilot cities, the median green innovation (83) is greater than the extreme value point, indicating that the majority of observations are located on the right of the symmetry axis. The possible reason for this is that the new energy demonstration cities are at the early stage of policy implementation and the pilot cities have failed to effectively integrate various energy resources in the promotion and application of new energy technologies, thus affecting energy efficiency (Guo et al., 2024). Most of the pilot cities belong to large and medium-sized cities, and their green innovation capabilities are also stronger than general cities. In addition, new energy technologies such as solar and wind, although widely used and tested in demonstration cities, may still present challenges in terms of their technological maturity, cost-effectiveness and marketing. This may lead to slow progress in reaching the tipping point in new energy demonstration cities. The findings can yield valuable insights for enhancing green innovation and promoting sustainable urban development.
6 Conclusion and implications
How to use green innovation tools to improve energy efficiency is strategically important for achieving the vision of “dual carbon” goals. Based on balanced panel data at the city level in China from 2008 to 2018, this paper analyses the impact of green innovation on TFEE using a two-way fixed effects model. The main findings are as follows: (1) Green innovation inhibits TFEE when the urban green innovation capacity is low, and helps promote TFEE when green innovation reaches a certain level. This conclusion still holds after several robustness tests. (2) Compared to strategic green innovation, the impact of substantive green innovation on TFEE reached the turning point earlier. (3) Cities with higher resource dependency can reach the turning point earlier than cities with lower resource dependency. This is mainly due to the lack of green innovation capacity of resource-based cities. (4) For new energy demonstration cities, overall, they are at the stage where green innovation inhibiting TFEE. For non-new energy demonstration cities, on the whole, they are at the stage where green innovation promotes TFEE.
This U-shaped relationship provides valuable insights for us to understand the interactive mechanism between green innovation and TFEE, offering important implications for policy-making and sustainable development strategies. Our research has clear policy implications: Firstly, in order to achieve the double carbon target on schedule, the central government can adopt a multi-pronged approach to improve energy efficiency. The government needs to strengthen energy management, promote the green and low-carbon transformation of the energy consumption structure, and set more stringent energy consumption standards. The central government can consider providing more financial support or preferential policies to cities with high energy efficiency, promoting local governments to attach importance to improving energy efficiency. Moreover, incorporating energy performance into the officials’ assessment system could be put on the agenda to motivate local government officials to save energy and reduce emissions.
Secondly, considering the dual impact of green innovation on TFEE, the government can take multiple measures to guard against energy rebound effect. For example, the impact of a single policy on the energy market should be avoided, and measures such as taxation, government regulation, and market mechanisms should be adopted to adjust the energy consumption structure from multiple aspects. Simultaneously, vigorously promote the synergistic low-carbon development of the energy supply side and consumption side, aiming to establish a clean, low-carbon, secure, and efficient energy system. On the supply side, invest more in renewable energy, and promote clean energy technologies to strengthen the supporting role of non-fossil energy supply for carbon reduction. On the consumption side, promote green and low-carbon production and lifestyle, especially to guide high-energy-consuming enterprises to establish a scientific energy management system.
Thirdly, when green innovation reaches a higher level, it can improve TFEE. Therefore, the central government should further improve the market-oriented green technology innovation system, increase green innovation investment, and especially encourage innovative entities to carry out substantive green innovation research. The government can establish public green innovation platforms to guide more social capital to invest in the field of green innovation. More meticulously, the government can consider establishing a scientific green innovation evaluation system and strictly controlling green patent certification, especially for green invention patents. Of course, considering the significant differences in green innovation levels among different cities, the government needs to develop differentiated incentive measures. For example, for resource-based cities and other small and medium-sized cities, the government can implement tax and fee reduction policies for innovative entities that carry out green innovation activities, and provide more resource incentives for such cities.
Although this paper can attract the attention of several disciplines, frankly speaking, there are some limitations to our study. Firstly, this study uses panel data at the city level in China for analysis, and the generalizability of the findings needs further verification. Future studies could expand the sample to different countries and regions to improve the generalizability and reliability of the findings. Second, due to the availability of data, we do not consider the impact of other types of green innovations on TFEE. Subsequent research can consider categorizing green innovation (e.g., Xu et al., 2021), and delve into the differentiated impact of heterogeneous green innovations on TFEE. Finally, this paper does not address the spatial effects of green innovation. On the basis of more solid theoretical studies, follow-up studies can use spatial econometric models to explore the spatial effects of urban green innovation on TFEE to understand their relationships more comprehensively.
Data availability statement
The raw data supporting the conclusion of this article will be made available by the authors, without undue reservation.
Author contributions
CL: Data curation, Investigation, Writing–review and editing. CW: Investigation, Methodology, Validation, Writing–original draft, Writing–review and editing. SY: Conceptualization, Supervision, Writing–review and editing. WW: Conceptualization, Supervision, Writing–review and editing. LZ: Conceptualization, Investigation, Writing–review and editing. QL: Formal Analysis, Investigation, Supervision, Visualization, Writing–review and editing.
Funding
The author(s) declare that no financial support was received for the research, authorship, and/or publication of this article.
Conflict of interest
The authors declare that the research was conducted in the absence of any commercial or financial relationships that could be construed as a potential conflict of interest.
Publisher’s note
All claims expressed in this article are solely those of the authors and do not necessarily represent those of their affiliated organizations, or those of the publisher, the editors and the reviewers. Any product that may be evaluated in this article, or claim that may be made by its manufacturer, is not guaranteed or endorsed by the publisher.
References
Belaid, F., Ben Youssef, A., and Lazaric, N. (2020). Scrutinizing the direct rebound effect for French households using quantile regression and data from an original survey. Ecol. Econ. 176, 106755. doi:10.1016/j.ecolecon.2020.106755
Bianchini, S., Damioli, G., and Ghisetti, C. (2023). The environmental effects of the "twin" green and digital transition in European regions. Environ. Resour. Econ. 84 (4), 877–918. doi:10.1007/s10640-022-00741-7
Bu, C. Q., Zhang, K. X., Shi, D. Q., and Wang, S. Y. (2022). Does environmental information disclosure improve energy efficiency? Energy Policy 164, 112919. doi:10.1016/j.enpol.2022.112919
Chen, H. Y., Yi, J. Z., Chen, A. B., Peng, D. X., and Yang, J. Q. (2023a). Green technology innovation and CO2 emission in China: evidence from a spatial-temporal analysis and a nonlinear spatial durbin model. Energy Policy 172, 113338. doi:10.1016/j.enpol.2022.113338
Chen, Q., Zha, D. L., Wang, L. J., and Yang, G. L. (2022). The direct CO2 rebound effect in households: evidence from China's provinces. Renew. Sustain. Energy Rev. 155, 111888. doi:10.1016/j.rser.2021.111888
Chen, X. G., Chen, L. Y., Xie, W., Mueller, N. D., and Davis, S. J. (2023b). Flight delays due to air pollution in China. J. Environ. Econ. Manag. 119, 102810. doi:10.1016/j.jeem.2023.102810
Chen, Z., Kahn, M. E., Liu, Y., and Wang, Z. (2018). The consequences of spatially differentiated water pollution regulation in China. J. Environ. Econ. Manag. 88, 468–485. doi:10.1016/j.jeem.2018.01.010
Cheng, Z. H., Yu, X. J., and Zhang, Y. (2023). Is the construction of new energy demonstration cities conducive to improvements in energy efficiency? Energy 263, 125517. doi:10.1016/j.energy.2022.125517
Cui, D., Ding, M. F., Han, Y. K., and Suardi, S. (2023). Regulation-induced financial constraints, carbon emission and corporate innovation: evidence from China. Energy Econ. 127, 107081. doi:10.1016/j.eneco.2023.107081
Du, K. R., and Li, J. L. (2019). Towards a green world: how do green technology innovations affect total-factor carbon productivity. Energy Policy 131, 240–250. doi:10.1016/j.enpol.2019.04.033
Du, K. R., Shao, S., and Yan, Z. M. (2021). Urban residential energy demand and rebound effect in China: a stochastic energy demand frontier approach. Energy J. 42 (4), 175–194. doi:10.5547/01956574.42.4.kdu
Duro, J. A. (2015). The international distribution of energy intensities: some synthetic results. Energy Policy 83, 257–266. doi:10.1016/j.enpol.2015.03.022
Fang, Z. (2023). Assessing the impact of renewable energy investment, green technology innovation, and industrialization on sustainable development: a case study of China. Renew. Energy 205, 772–782. doi:10.1016/j.renene.2023.01.014
Gao, D., Li, G., and Yu, J. Y. (2022). Does digitization improve green total factor energy efficiency? Evidence from Chinese 213 cities. Energy 247, 123395. doi:10.1016/j.energy.2022.123395
Gerarden, T. D., Newell, R. G., and Stavins, R. N. (2017). Assessing the energy-efficiency gap. J. Econ. Literature 55 (4), 1486–1525. doi:10.1257/jel.20161360
Guang, F. T., Deng, Y. T., Wen, L., Sharp, B., and Hong, S. F. (2023). Impact of regional energy allocation distortion on carbon emission efficiency: evidence from China. J. Environ. Manag. 342, 118241. doi:10.1016/j.jenvman.2023.118241
Guang, F. T., Wen, L., and Sharp, B. (2022). Energy efficiency improvements and industry transition: an analysis of China's electricity consumption. Energy 244, 122625. doi:10.1016/j.energy.2021.122625
Guo, B. N., Feng, Y., Lin, J., and Wang, X. (2024). New energy demonstration city and urban pollutant emissions: an analysis based on a spatial difference-in-differences model. Int. Rev. Econ. Finance 91, 287–298. doi:10.1016/j.iref.2024.01.048
Guo, B. N., Hu, P. J., Zhang, H., Hu, F., and Wang, J. (2023b). Can the energy-consuming right trading policy improve urban green technology innovation capacity? Evidence from China. Pol. J. Environ. Stud. 32 (6), 5583–5597. doi:10.15244/pjoes/169899
Guo, B. N., Hu, P. J., Zhang, H., Weng, X. Y., and Hu, F. (2023a). The improvement of energy-consuming right trading policy on the efficiency of urban green development. Front. Environ. Sci. 11, 1123608. doi:10.3389/fenvs.2023.1123608
Guo, Q. T., Dong, Y., Feng, B., and Zhang, H. (2023c). Can green finance development promote total-factor energy efficiency? Empirical evidence from China based on a spatial Durbin model. Energy Policy 177, 113523. doi:10.1016/j.enpol.2023.113523
Guo, W., and Liu, X. R. (2022). Market fragmentation of energy resource prices and green total factor energy efficiency in China. Resour. Policy 76, 102580. doi:10.1016/j.resourpol.2022.102580
Haans, R. F. J., Pieters, C., and He, Z. L. (2016). Thinking about U: theorizing and testing U-and inverted U-shaped relationships in strategy research. Strategic Manag. J. 37 (7), 1177–1195. doi:10.1002/smj.2399
Hao, Y., Guo, Y. X., and Wu, H. T. (2022). The role of information and communication technology on green total factor energy efficiency: does environmental regulation work? Bus. Strategy Environ. 31 (1), 403–424. doi:10.1002/bse.2901
Huang, H. Y., Wang, F. R., Song, M. L., Balezentis, T., and Streimikiene, D. (2021). Green innovations for sustainable development of China: analysis based on the nested spatial panel models. Technol. Soc. 65, 101593. doi:10.1016/j.techsoc.2021.101593
IEA. (2021). Global energy review. Avilable at: https://iea.blob.core.windows.net/assets/d0031107-401d-4a2f-a48b-9eed19457335/GlobalEnergyReview2021.pdf.
Jacobsen, G. D. (2015). Do energy prices influence investment in energy efficiency? Evidence from energy star appliances. J. Environ. Econ. Manag. 74, 94–106. doi:10.1016/j.jeem.2015.09.004
Jiang, Q., Yang, S., Tang, P., and Bao, L. (2020). Promoting the polluters? The competing objectives of energy efficiency, pollutant emissions, and economic performance in Chinese municipalities. Energy Res. Soc. Sci. 61, 101365. doi:10.1016/j.erss.2019.101365
Jiang, Q. S., and Tang, P. C. (2023). All roads lead to Rome? Carbon emissions, pollutant emissions and local officials' political promotion in China. Energy Policy 181, 113700. doi:10.1016/j.enpol.2023.113700
Li, W. C., Xu, J., Ostic, D., Yang, J. L., Guan, R. D., and Zhu, L. (2021). Why low-carbon technological innovation hardly promote energy efficiency of China? Based on spatial econometric method and machine learning. Comput. Industrial Eng. 160, 107566. doi:10.1016/j.cie.2021.107566
Lind, J. T., and Mehlum, H. (2010). With or without U? The appropriate test for a U-shaped relationship. Oxf. Bull. Econ. Statistics 72 (1), 109–118. doi:10.1111/j.1468-0084.2009.00569.x
Liu, F. Q., Sim, J. Y., Sun, H. P., Edziah, B. K., Adom, P. K., and Song, S. F. (2023a). Assessing the role of economic globalization on energy efficiency: evidence from a global perspective. China Econ. Rev. 77, 101897. doi:10.1016/j.chieco.2022.101897
Liu, X., Li, H., and Yang, C. (2023b). Analyzing the impact of low-carbon city pilot policy on enterprises' labor demand: evidence from China. Energy Econ. 124, 106676. doi:10.1016/j.eneco.2023.106676
Liu, X. Y., Wen, H. X., and Ding, D. D. (2023c). The impact of new ambient air quality standards on green total factor energy efficiency: evidence from an environmental information disclosure policy in China. Front. Environ. Sci. 11, 1120893. doi:10.3389/fenvs.2023.1120893
Mongo, M., Belaid, F., and Ramdani, B. (2021). The effects of environmental innovations on CO2 emissions: empirical evidence from Europe. Environ. Sci. Policy 118, 1–9. doi:10.1016/j.envsci.2020.12.004
Noailly, J., and Smeets, R. (2015). Directing technical change from fossil-fuel to renewable energy innovation: an application using firm-level patent data. J. Environ. Econ. Manag. 72, 15–37. doi:10.1016/j.jeem.2015.03.004
Pan, X. F., Ai, B. W., Li, C. Y., Pan, X. Y., and Yan, Y. B. (2019). Dynamic relationship among environmental regulation, technological innovation and energy efficiency based on large scale provincial panel data in China. Technol. Forecast. Soc. Change 144, 428–435. doi:10.1016/j.techfore.2017.12.012
Paramati, S. R., Shahzad, U., and Dogan, B. (2022). The role of environmental technology for energy demand and energy efficiency: evidence from OECD countries. Renew. Sustain. Energy Rev. 153, 111735. doi:10.1016/j.rser.2021.111735
Petrovic, P., Filipovic, S., and Radovanovic, M. (2018). Underlying causal factors of the European Union energy intensity: econometric evidence. Renew. Sustain. Energy Rev. 89, 216–227. doi:10.1016/j.rser.2018.03.061
Porter, M. E., and Van der Linde, C. (1995). Toward a new conception of the environment-competitiveness relationship. J. Econ. Perspect. 9 (4), 97–118. doi:10.1257/jep.9.4.97
Proskuryakova, L., and Kovalev, A. (2015). Measuring energy efficiency: is energy intensity a good evidence base? Appl. Energy 138, 450–459. doi:10.1016/j.apenergy.2014.10.060
Ren, S. G., Wang, Y., Hu, Y. C., and Yan, J. (2021). CEO hometown identity and firm green innovation. Bus. Strategy Environ. 30 (2), 756–774. doi:10.1002/bse.2652
Ren, S. Y., Hao, Y., and Wu, H. T. (2022). The role of outward foreign direct investment (OFDI) on green total factor energy efficiency: does institutional quality matters? Evidence from China. Resour. Policy 76, 102587. doi:10.1016/j.resourpol.2022.102587
Shao, S., Yang, Z. B., Yang, L., and Ma, S. (2019). Can China's energy intensity constraint policy promote total factor energy efficiency? Evidence from the industrial sector. Energy J. 40 (4), 101–128. doi:10.5547/01956574.40.4.ssha
Sharif, A., Saqib, N., Dong, K. Y., and Khan, S. A. R. (2022). Nexus between green technology innovation, green financing, and CO2 emissions in the G7 countries: the moderating role of social globalisation. Sustain. Dev. 30 (6), 1934–1946. doi:10.1002/sd.2360
Singh, S. K., Del Giudice, M., Chierici, R., and Graziano, D. (2020). Green innovation and environmental performance: the role of green transformational leadership and green human resource management. Technol. Forecast. Soc. Change 150, 119762. doi:10.1016/j.techfore.2019.119762
Sun, H. P., Edziah, B. K., Sun, C. W., and Kporsu, A. K. (2019). Institutional quality, green innovation and energy efficiency. Energy Policy 135, 111002. doi:10.1016/j.enpol.2019.111002
Sun, Y. P., Yesilada, F., Andlib, Z., and Ajaz, T. (2021). The role of eco-innovation and globalization towards carbon neutrality in the USA. J. Environ. Manag. 299, 113568. doi:10.1016/j.jenvman.2021.113568
Tanaka, K., and Managi, S. (2021). Industrial agglomeration effect for energy efficiency in Japanese production plants. Energy Policy 156, 112442. doi:10.1016/j.enpol.2021.112442
Tang, P. C., Jiang, Q. S., and Mi, L. L. (2021). One-vote veto: the threshold effect of environmental pollution in China's economic promotion tournament. Ecol. Econ. 185, 107069. doi:10.1016/j.ecolecon.2021.107069
Tang, P. C., Jiang, Q. S., and Wang, C. (2024). Beyond environmental actions: how environmental regulations stimulate strategic-political CSR engagement in China? Energy Econ. 129, 107171. doi:10.1016/j.eneco.2023.107171
Tang, P. C., Liu, X., Hong, Y., and Yang, S. W. (2022). Moving beyond economic criteria: exploring the social impact of green innovation from the stakeholder management perspective. Corp. Soc. Responsib. Environ. Manag. 30, 1042–1052. doi:10.1002/csr.2401
Tang, P. C., Wang, C., Jiang, Q. S., Liu, X., and Wang, J. Y. (2023). Symbol or substance? Environmental regulations and corporate environmental actions decoupling. J. Environ. Manag. 346, 118950. doi:10.1016/j.jenvman.2023.118950
Tone, K. (2001). A slacks-based measure of efficiency in data envelopment analysis. Eur. J. Operational Res. 130 (3), 498–509. doi:10.1016/s0377-2217(99)00407-5
Ulucak, R., and Baloch, M. A. (2023). An empirical approach to the nexus between natural resources and environmental pollution: do economic policy and environmental-related technologies make any difference? Resour. Policy 81, 103361. doi:10.1016/j.resourpol.2023.103361
Wang, L., Dykstra, J. E., and Lin, S. H. (2019). Energy efficiency of capacitive deionization. Environ. Sci. Technol. 53 (7), 3366–3378. doi:10.1021/acs.est.8b04858
Wang, Y., Deng, X. Z., Zhang, H. W., Liu, Y. J., Yue, T. X., and Liu, G. (2022). Energy endowment, environmental regulation, and energy efficiency: evidence from China. Technol. Forecast. Soc. Change 177, 121528. doi:10.1016/j.techfore.2022.121528
Wu, H. T., Hao, Y., and Ren, S. Y. (2020). How do environmental regulation and environmental decentralization affect green total factor energy efficiency: evidence from China. Energy Econ. 91, 104880. doi:10.1016/j.eneco.2020.104880
Wu, H. T., Hao, Y., Ren, S. Y., Yang, X. D., and Xie, G. (2021). Does internet development improve green total factor energy efficiency? Evidence from China. Energy Policy 153, 112247. doi:10.1016/j.enpol.2021.112247
Wu, T., Wen, L., and Yi, M. (2024a). Balancing growth targets and environmental regulations: an empirical analysis of dual policy impact on corporate environmental responsibility-insights from China. J. Environ. Manag. 355, 120500. doi:10.1016/j.jenvman.2024.120500
Wu, T., Yi, M., and Zhang, Y. (2024b). Towards cities' green growth: the combined influence of economic growth targets and environmental regulations. Cities 146, 104759. doi:10.1016/j.cities.2023.104759
Xiang, X. J., Liu, C. J., and Yang, M. (2022). Who is financing corporate green innovation? Int. Rev. Econ. Finance 78, 321–337. doi:10.1016/j.iref.2021.12.011
Xin, D. L., Ahmad, M., Lei, H., and Khattak, S. I. (2021). Do innovation in environmental-related technologies asymmetrically affect carbon dioxide emissions in the United States? Technol. Soc. 67, 101761. doi:10.1016/j.techsoc.2021.101761
Xu, L., Fan, M. T., Yang, L. L., and Shao, S. (2021). Heterogeneous green innovations and carbon emission performance: evidence at China's city level. Energy Econ. 99, 105269. doi:10.1016/j.eneco.2021.105269
Xu, M. M., and Lin, B. Q. (2022). Energy efficiency gains from distortion mitigation: a perspective on the metallurgical industry. Resour. Policy 77, 102758. doi:10.1016/j.resourpol.2022.102758
Yan, Z. M., Zou, B. L., Du, K. R., and Li, K. (2020). Do renewable energy technology innovations promote China's green productivity growth? Fresh evidence from partially linear functional-coefficient models. Energy Econ. 90, 104842. doi:10.1016/j.eneco.2020.104842
Yildirim, D. C., Esen, O., and Yildirim, S. (2022). The nonlinear effects of environmental innovation on energy sector-based carbon dioxide emissions in OECD countries. Technol. Forecast. Soc. Change 182, 121800. doi:10.1016/j.techfore.2022.121800
Yuan, C., and Zhang, L. (2015). Public education spending and private substitution in urban China. J. Dev. Econ. 115, 124–139. doi:10.1016/j.jdeveco.2015.02.006
Zhang, S. L., Wu, Z. H., He, Y. A., and Hao, Y. (2022). How does the green credit policy affect the technological innovation of enterprises? Evidence from China. Energy Econ. 113, 106236. doi:10.1016/j.eneco.2022.106236
Zhao, C., and Wang, B. (2022). How does new-type urbanization affect air pollution? Empirical evidence based on spatial spillover effect and spatial Durbin model. Environ. Int. 165, 107304. doi:10.1016/j.envint.2022.107304
Zhao, J. F., Yan, J. L., Yang, X. D., Nie, C. X., Sun, H., and Ran, Q. Y. (2022c). Government competition, corruption and green total factor energy efficiency: an empirical analysis from China. Front. Environ. Sci. 10, 976451. doi:10.3389/fenvs.2022.976451
Zhao, X., Mahendru, M., Ma, X. W., Rao, A. M., and Shang, Y. P. (2022a). Impacts of environmental regulations on green economic growth in China: new guidelines regarding renewable energy and energy efficiency. Renew. Energy 187, 728–742. doi:10.1016/j.renene.2022.01.076
Zhao, X., Nakonieczny, J., Jabeen, F., Shahzad, U., and Jia, W. X. (2022b). Does green innovation induce green total factor productivity? Novel findings from Chinese city level data. Technol. Forecast. Soc. Change 185, 122021. doi:10.1016/j.techfore.2022.122021
Zhou, C. B., and Qi, S. Z. (2022). Has the pilot carbon trading policy improved China's green total factor energy efficiency? Energy Econ. 114, 106268. doi:10.1016/j.eneco.2022.106268
Zor, S. (2023a). A neural network-based measurement of corporate environmental attention and its impact on green open innovation: evidence from heavily polluting listed companies in China. J. Clean. Prod. 432, 139815. doi:10.1016/j.jclepro.2023.139815
Keywords: green innovation, total factor energy efficiency, energy rebound effect, U-shaped relationship, fixed effects model
Citation: Liu C, Wang C, Yang S, Wang W, Zhao L and Li Q (2024) Catalyst or Obstacle? Green innovation and total factor energy efficiency. Front. Environ. Sci. 12:1397462. doi: 10.3389/fenvs.2024.1397462
Received: 07 March 2024; Accepted: 19 April 2024;
Published: 06 May 2024.
Edited by:
Le Wen, University of Auckland, New ZealandReviewed by:
Shutter Zor, Xiamen University, ChinaBingnan Guo, Jiangsu University of Science and Technology, China
Copyright © 2024 Liu, Wang, Yang, Wang, Zhao and Li. This is an open-access article distributed under the terms of the Creative Commons Attribution License (CC BY). The use, distribution or reproduction in other forums is permitted, provided the original author(s) and the copyright owner(s) are credited and that the original publication in this journal is cited, in accordance with accepted academic practice. No use, distribution or reproduction is permitted which does not comply with these terms.
*Correspondence: Chao Wang, 18790108839@163.com