- 1International Business School, Shaanxi Normal University, Xi’an, China
- 2School of Economics, Huazhong University of Science and Technology, Wuhan, China
This study uses the game theory combination weighting method to measure the level of coordinated development of green finance and digital technology coupling in China. An analysis was conducted using the Kernel density estimation method, traditional Markov chain model, and spatial Markov chain model to study the dynamic evolution characteristics and trends of the coupling and coordination level of green finance and digital technology in Chinese provinces. The results showed an upward trend in the coupling and coordination level of green finance and digital technology in the entire sample of Chinese, eastern, central, western, and northeastern provinces. Additionally, there is an observable club convergence phenomenon in the coordinated development of green finance and digital technology. The phenomenon of high-level convergence and low-level convergence is significant. The areas on the diagonal that are low-level and high-level have a higher probability of remaining stable. The Markov chain model and the spatial Markov chain model both show the “Matthew effect” in the level of coupling and coordinated development of green finance and digital technology. Empirical testing suggests that the coordinated horizontal development of green finance and digital technology can significantly promote pollution emission. Further analysis found that the coupling and coordination of green finance and digital technology achieves pollution emission by driving green innovation.
1 Introduction
Since the 1960s, the world has faced significant ecological and environmental challenges such as global warming and degradation (Hao et al., 2018; Chiu and Lee, 2020; Wang et al., 2022; Wen et al., 2022). The “Global Climate Status in 2023” report released by the World Meteorological Organization in March 2024 shows that records in greenhouse gas levels, surface temperature, ocean heat and acidification, sea level rise, Antarctic sea ice sheet and glacier retreat have been broken again. 2023 is the hottest year on record, with the average annual temperature 1.45°C ± 0.12°C higher than the pre-industrial level (1850–1900), far exceeding the warming range of the previous hottest year and further approaching the 1.5°C temperature control target set by the Paris Agreement. To solve the risks caused by environmental degradation and promote sustainable development, many countries have started to formulate relevant green development policies from the economic level to promote the coordinated development of the economy and the ecological environment. China, which has made remarkable progress in economic development since the reform and opening up in 1978 (Lee et al., 2021a), also faces environmental pollution and resource shortage problems due to rapid industrialization. On 22 September 2022, China announced its plan to achieve carbon neutrality by 2060, which will accelerate the reduction of carbon dioxide emissions. Exploring the path to achieve coordinated development of economy and environment provides ideas for solving the above-mentioned economic and environmental problems, and also provides solutions for other developing countries to achieve green development.
In the process of economic development, achieving green development by reducing pollution and emissions can not only promote sustainable economic development, but also achieve the ecological goal of harmonious coexistence between man and nature. Finance is an important force in supporting economic development. Green finance has the dual characteristics of financial resource allocation and environmental regulation (Tariq and Hassan, 2023). Its clear guiding characteristics provide new ideas for easing corporate financing constraints, reducing financial costs and increasing R&D investment. Most existing studies have studied green finance from a single dimension, exploring its pollution reduction effect (Simionescu and Gavurová, 2023; Zhou et al., 2023). However, information asymmetry makes it difficult for traditional financial institutions to penetrate the environmental benefit information of specific projects, which is not conducive to the development of enterprises to obtain green funds or avoid becoming the target of regulation. At the same time, there is a clear differentiation in the resource allocation decisions of financial institutions under the constraints of environmental goals (Liu and Xu, 2024). The increased risk of environmental regulation has caused banks and other financial institutions to guide credit resources away from polluting enterprises in order to avoid potential financial and reputational losses. This has ultimately led to a dilemma for polluting enterprises that have a strong desire to transform but cannot obtain effective financial support. The development of digital technology can help solve or alleviate such problems. Digital technology has efficient analytical capabilities, which not only breaks the isolation between banks and enterprises, but also provides effective preparation for the mutual sharing of enterprise resources, the absorption and diffusion of external knowledge, and the acceleration of the conception and formation of innovative projects. On the one hand, digital technology effectively reduces the cost of information collection between the supply and demand sides of funds, helps to solve the problem of “difficult and expensive financing” in the process of green transformation of enterprises, and enables financial institutions to better serve green development. On the other hand, through the establishment of a green finance monitoring and evaluation system through digital technology, the energy conservation and emission reduction, green transformation and pollution control effects of green finance can be comprehensively quantified, and the mismatch of green financial resources flowing to industries can be reversed, providing a policy basis for regulatory authorities to implement differentiated supervision and incentive measures. Therefore, promoting the coupled and coordinated development of green finance and digital technology can achieve green technology innovation in enterprises (Liu et al., 2023a), promote the realization of pollution reduction and emission reduction targets, and thus has important significance for achieving green economic transformation and sustainable development.
Existing studies have mainly examined the impact of green finance from two perspectives: environmental regulation and financial resource allocation. On the one hand, from the perspective of environmental regulation, the impact of environmental regulation on enterprises is analyzed based on the Porter hypothesis’s induced innovation effect. Some studies believe that environmental regulation has curbed illegal pollution discharge by enterprises, and the improvement of the ecological environment has attracted more investment in high-end service industries and promoted industrial upgrading and transfer (Xiong and Chen, 2022). Market incentive-based environmental regulation uses measures such as carbon emission trading and environmental protection taxes. Through the price mechanism, the cost of environmental pollution is internalized and the expected value of environmental investment is locked in, which encourages enterprises to carry out green activities and achieve green transformation of enterprises (Wu et al., 2024a; Yu and Zheng, 2024). On the other hand, from the perspective of financial resource allocation, the resource allocation effect of green finance on enterprise development is mainly examined from the perspective of green credit policy, green bonds, green financial innovation and green reform pilot (Liu and Xiong, 2022; Yin et al., 2023; Xiong et al., 2023).
Existing studies have confirmed that green finance has a significant impact on pollution reduction. However, the traditional financial model lacks the support of effective technical means and data-driven quantitative measurement, and cannot achieve effective supervision of post-loan funds, and cannot track and judge in a timely manner. The true use of funds and benefits of enterprises cannot accurately identify and screen high-quality green innovation projects, resulting in the insufficient role of green finance in improving the quality of green innovation of enterprises (Wang et al., 2023), which is not conducive to the long-term green development of the economy. In view of this, this study attempts to construct a green finance and digital technology coupling and coordinated development index based on China’s actual scenario, judge the degree of coupling and coordination between digital technology and green finance through measurement results, analyze the dynamic evolution characteristics of their coupled and coordinated development, and test their pollution emission effects.
This study has two possible contributions. First, it provides more reliable quantitative measurement evidence for the study of the coupling and coordination of green finance and digital technology. Second, it supplements the relevant research on the impact of the coupling and coordinated development of green finance and digital technology on pollution reduction, and opens up new ideas for achieving pollution reduction and emission reduction goals, promoting the green transformation of the economy, and promoting global sustainable development.
2 Literature review and hypothesis development
2.1 Literature review
To prevent credit risks, the “Opinions on Implementing Environmental Protection Policies and Regulations” were released in July 2007. Since then, China has experienced rapid development in green finance, leading to the creation of a green financial system. This system is primarily comprised of green credit, which is supported by green bonds, green funds, and green insurance. By providing green credit, high-energy-consuming and high-polluting enterprises are able to reduce credit rationing. As a result, air pollutants and carbon dioxide emissions can be suppressed (Tariq and Hassan, 2023). At the 2016 G20 Summit, “green finance” began to receive widespread attention from society. In 2016, the “Guiding Opinions on Building Green Financial System” was issued by the People’s Bank of China and other organizations, marking a significant milestone in the development of green finance. It signals a period of rapid growth in this field. The swift growth of green finance has transformed it into a significant instrument for promoting green economic development. As a result, scholars have started to focus more on the definition and assessment of green finance, as well as its influence on both the economy and the environment (He et al., 2019a; He et al., 2019b; Liu et al., 2019; Hu et al., 2021; Lee et al., 2021b).
At present, academic research on the macro-effects of green finance mainly focuses on the effects of green finance on high-quality economic development (Xu and Dong, 2023), low-carbon development (Wara, 2007), and green development (Liu et al., 2019). It also measures and evaluates the level of regional green finance development (Liu et al., 2023a; Jiang et al., 2020).
Based on the perspective of economics, some economists believe that green finance can provide capital support, rationalize economic structures, improve supply-side investment quality, and ultimately lead to more stable economic growth (Lee et al., 2022). Certain scholars conduct research from an environmental perspective and suggest that green finance can facilitate environmental progress, optimize resource utilization, and mitigate pollution and carbon emissions. This type of financing can provide sustainable, dependable financial backing for the advancement of ecological civilization and the attainment of carbon neutrality (Wu et al., 2023; Liu et al., 2020a; Xia and Xu, 2020; Yan et al., 2020; Wang and Ren, 2022). Although some articles touch upon the pollution control and emission reduction effects of green finance, most of them simply consider it as a part of sustainable development, green economic efficiency, and circular economy (Simionescu and Gavurová, 2023; Chin et al., 2024; Liu et al., 2023b), and do not study it as a separate subject.
Digital technology based on information data processing provides an effective way for sustainable development to achieve pollution reduction and emission reduction through the use of artificial intelligence, cloud computing, and big data. One key consensus in promoting sustainable development and achieving pollution reduction and emission reduction goals is through the vigorous development of green industries and the digital economy. In the field of low-carbon research, digital technology has attracted the attention of many scholars and has become an important research topic (Truong, 2022). Digital technology can alleviate information asymmetry in the field of factor allocation and break barriers to the flow of production factors by exerting value creation effects, network connection effects, resource allocation effects, and cost-saving effects (Xiang and Tian, 2022; Yang et al., 2022; Zhang et al., 2022; Qi and Ren, 2021; Xu et al., 2023; Guo and Qiu, 2020), providing a favorable digital facility platform for the realization of green economic development. Some scholars have analyzed the application of digital technology in the financial sector (Zhao et al., 2023) and its impact on carbon emission intensity (Zhang et al., 2023).
There are few studies on the coupling of green finance and digital technology, and only a few scholars have analyzed the impact of the coupling of green finance and digital technology on corporate green technology innovation (Xiong and Chen, 2022). Therefore, this study measures the level of green finance and digital technology coupling coordination based on the game theory combined weighting method, and analyzes its impact on pollution emission.
2.2 Theoretical analysis and hypothesis
Based on an in-depth analysis of green finance research and discussion of the impact of digital technology, we believe that the coupled and coordinated development of green finance and digital technology can achieve pollution emission reduction through green innovation mechanisms.
Carbon dioxide and air pollutant emissions have the same roots, origins, and processes. Controlling carbon dioxide emissions is an important factor in reducing air pollutant emissions. It is feasible to reduce emissions of both at the same time (Dong et al., 2019; Cheng et al., 2021a).Green credit, as China’s most important green financial product, sets clear environmental access thresholds for loan recipients, raising financing costs for high-pollution and energy-consuming industries. Through the resource allocation effect, green credit reduces credit rationing, increases the risk of exiting the market, and releases market selection signals, thereby reducing atmospheric pollutants and carbon dioxide emissions (Bao and He, 2022). With environmental damage and climate warming, investors are beginning to worry about investment failures due to environmental risks and are more willing to buy green bonds, thereby promoting the development of green industries and achieving emission reduction effects (Gianfrate and Peri, 2019; Hu and Chen, 2023).
By building a green project information sharing platform, digital technology effectively reduces the information collection costs between the supply and demand sides of funds, enabling financial institutions to better serve green development, rationally allocate green financial resources, and reasonably avoid capital mismatch. The higher the level of coupling and coordination between green finance and digital technology, the lower the financing costs of enterprises, which can promote enterprises to carry out green transformation and achieve pollution reduction and emission reduction (Xiong and Chen, 2022). Therefore, this study proposes the following hypothesis.
Hypothesis 1. The coupled and coordinated development of green finance and digital technology can reduce pollution and emissions.
The coupling of green finance and digital technology can reduce pollution emissions by promoting green innovation. Green finance has the dual characteristics of environmental regulation and capital allocation. Based on Porter’s hypothesis theory, green finance cannot achieve ecological and economic benefits without technological innovation. Green finance can play a role in environmental regulation. By setting environmental access thresholds to restrict external financing, it can increase the pollution emission costs of enterprises. This will guide enterprises to increase their investment in environmental management and improve their production efficiency through effective innovation. While continuously improving environmental benefits and achieving pollution reduction and emission reduction, it will also bring about a continuous increase in the return on capital (Bao and He, 2022; Niu et al., 2022). At the same time, considering that green innovation requires a lot of time and sufficient resource support, problems such as resource mismatch may become the main factor hindering its development (Wu et al., 2024b). Digital technology-empowered green finance can conduct high-quality integration and reallocation of green innovation resources, achieve targeted allocation of green innovation resources, reconstruct the resource allocation pattern, and mobilize local enthusiasm for green innovation. Green innovation can assist the research and development process of green cleaning products, promote the efficient optimization of clean production technology, promote the improvement of carbon dioxide purification technology, rapidly reduce the emission intensity of pollutants, greenhouse gases, industrial wastewater, etc., and promote pollution reduction and emission reduction. In addition, green management innovation can effectively promote the improvement of management processes and infrastructure optimization such as production and research and development, so as to improve labor productivity, reduce carbon emission intensity, and achieve the goal of reducing pollution and emissions. Some studies believe that green innovation can significantly promote carbon emission reduction. Xu et al. (2021) found that green innovation has a significant inhibitory effect on carbon emissions, but the inhibitory effect of carbon sink technology and carbon storage technology is weak. Relevant scholars have found that green innovation in N-11 countries (Wang et al., 2020), G7 countries (Ding et al., 2021), Singapore (Meirun et al., 2021) and BRICS countries (Jiang et al., 2022)can significantly reduce carbon emissions. Therefore, this study proposes the following hypothesis.
Hypothesis 2. The coupled and coordinated development of green finance and digital technology can achieve pollution emission through green innovation.
3 Method and model
3.1 Sample and data
From 2011 to 2020, 30 provinces and municipalities in China were selected as research samples to evaluate their level of coupling and coordination of green finance and digital technology. The data is primarily sourced from various reliable sources including the “China Urban Statistical Yearbook,” WIND database, “China Insurance Yearbook,” “China Industrial Statistical Yearbook,” CSMAR database, and others. Some provinces with a large number of missing data were excluded.
3.2 Method
3.2.1 Construction of indicator system
Early domestic scholars used commercial banks’ green credit balances to reflect green finance and study the impact of green finance on commercial banks’ credit risks (Sun et al., 2017). To fully reflect the concept of green finance, it's not enough to focus solely on green credit. The development of financial products and the improvement of the green financial system are also important factors to consider. To promote sustainable development, Ren et al. created green financial indicators that cover four key areas: green credit, green securities, green insurance, and green investment (Ren et al., 2020). According to the Development Research Institute at the Southwestern University of Finance and Economics and the Environmental and Economic Policy Research Center of the Ministry of Environmental Protection, green finance has the potential to foster the integration of financial activities, including credit, insurance, securities, and industrial funds, in support of a balanced and sustainable development of the economy, resources and environment (The Study Group of Development Research, 2015). One way to categorize sustainable financing methods is through the five tools of green finance, which include green credit, green securities, green insurance, green investment, and carbon finance. In 2016, several Chinese ministries and commissions, including the People’s Bank of China and the Ministry of Finance, collaborated to release “Guiding Opinions on Building a Green Financial System.” This document outlines the meaning of green finance across five dimensions: green credit, green investment, green funds, green insurance, and carbon finance. Existing literature has not formed a consistent method for measuring the development level of regional digital technology and green finance, and there are few studies that measure the coordinated development of green finance and digital technology.
To evaluate the level of development in green finance, this study selected six indicators from five dimensions. These dimensions include green credit, green investment, green funds, green insurance, and carbon finance. The selected indicators were based on previous research and were used to construct an evaluation system. Refer to the practices of scholars such as Zhao (2022), Huang et al. (2019), Zhao et al. (2020), and Li (2021), the potential of digital technology can be evaluated through two dimensions: infrastructure and application. By examining these factors, we can gain insight into how to improve and shape a brighter future. Table 1 shows how digital technology and green finance are measured. It's useful for tracking progress and promoting sustainable practices.
3.2.2 Determination of indicator combination weight
3.2.2.1 Subjective weighting method-analytic hierarchy process
1) The weight of the indicator layer to the criterion layer
First, one of the commonly used methods for constructing the judgment matrix A is the 9-scale method. The specific calculation formula is shown in Equation 1. This method involves assigning numerical values from 1 to 9 to represent the degree of importance or preference of each criterion with the others.
Where:
M is the number of indicators in the criterion layer where the sth indicator and the rth indicator are located.
To find the index weight, the eigenvector method is used. The formula used for calculating the weight of the ith indicator under the kth criterion layer is shown in Equation 2.
Where:
Conduct a consistency test on the results. If the test passes, the weight obtained is reasonable. Otherwise, the judgment matrix needs to be reconstructed to find the weight.
3.2.2.2 The weight of the indicator layer to the overall target layer
According to the AHP weighting principle (Hoque et al., 2017), the weight of the ith indicator to the kth criterion is shown in Equation 3.
Where:
(2) Objective weighting method—entropy weight method
The objective weight of each dimension indicator is calculated using the entropy weight method. First, to ensure consistency, the indicators that are measured in different units are standardized. The specific calculation formula is shown in Equation 4.
Where:
Secondly, calculate the proportion of the ith region in the jth indicator and obtain the information entropy. The specific calculation formula is shown in Equations 5 and 6.
Then, the weight of each indicator has been determined. The specific calculation formula is shown in Equation 7.
Finally, the weights are multiplied by the normalized values. The specific calculation formula is shown in Equation 8.
3.2.3 Game theory combination weighting method
Game theory is the study of conflict and cooperation between decision-makers using mathematical models (Myerson, 1991). Compared to other methods of weighting combinations, the game theory method can minimize the deviation between subjective and objective weights, as well as the combination weighting, and maximize the value of subjective and objective weighting (Zhao et al., 2024a). To achieve the desired outcome, it is crucial to determine the appropriate weight for the combination of analytic hierarchy process and entropy weight method. In this article, the game theory combination weighting method is applied for determining the weight of the indicator system. The specific steps involved in the method are provided below.
Assume that from L perspectives, L methods are used to weight the two index weighting methods respectively and obtain L groups of index weight vectors. Assume that the index weight vector obtained by the ith weighting method is
where:
Using the equilibrium theory of game theory, the optimal solution to the weighted combination weight can be obtained by minimizing the deviation between the subjective and objective weights. This is achieved by minimizing the difference between the indicator weight vector
Where:
When i = 1,
Substitute the combined weights
3.2.4 Coupling coordination model
The concept of “coupling” was initially introduced into economic analysis from the field of physics. The degree of coupling is a measure of the level of interdependence and mutual constraints between multiple systems. This measurement can be used to evaluate the degree of dynamic correlation between two or more systems. Unlike causal relationship analysis based on econometric models, the coupling degree model does not focus on the causal relationship between two systems but instead concentrates on the dynamic correlation between them. Therefore, in this study, we have utilized the coupling coefficient model from physics to measure the coupling level between green finance and digital technology (Liu et al., 2023a). The specific form is shown in Equation 12.
Where:
In order to more accurately reflect the level of interactive development between green finance and digital technology, it is necessary to further build a coupling coordination model of green finance and digital technology. The specific formula is shown in Equations 13 and 14.
3.2.5 Kernel density estimation
This study uses non-parametric kernel density estimation to analyze the dynamic evolution trend of the coupling coordination level of green finance and digital technology in China. Kernel density estimation has weak dependence on the model and has good statistical properties, and has important applications in studying spatial distribution non-equilibrium. Assuming that the density function of the random variable X has the following form. The specific calculation formula is shown in Equation 15.
Where:
N represents the number of observations.
The kernel function is a smooth transformation or weighted function that must meet certain conditions as shown in Equation 16. Kernel functions include trigonometric kernel functions, quadrangular kernel functions, Gaussian kernel functions, etc. Empirical research shows that the less grouped data used, the greater the possibility of selecting the Gaussian kernel function (Sala-i-Martin, 2006). Therefore, this article has used the Gaussian kernel function to estimate the evolution trends and distribution dynamics of the coupling coordination level of green finance and digital technology.
3.2.6 Markov chain model
The traditional Markov Chain is a mathematical method that calculates the probability distribution and evolution trend of each type. It does so under the condition that time and state are both discrete. This method can help us explore the dynamic evolution characteristics of the distribution of the coupling and coordinated development levels of green finance and digital technology in different periods. Markov Chains are stochastic processes.
The set M of the random process grows exponentially with each period, while the finite state represents the number of states of the random variable. Equation 17 is valid for all periods t and all possible states j and i. Equation 18 states the characteristics of a first-order Markov chain, where the probability of the random variable X being in state j at period t depends solely on the state of X at period t-1.
Let’s assume that the state space of the Markov process is represented by the set I, where I = {1, 2, 3}. The matrix P =
Where:
The Markov chain model overcomes the limitation of kernel density estimation in describing the distribution dynamics of the coordinated development levels of green finance and digital technology coupling. However, it assumes that regions are isolated and does not consider spatial factors. The level of coupled and coordinated development of green finance and digital technology is not isolated in geographical space and is closely related to the development level of surrounding areas. Therefore, the spatial Markov chain model uses the concept of “spatial lag” to examine the impact of spatial factors on the transition probability of the region, thereby determining the spatial status of the region. Based on the traditional Markov chain, k types of spatial lags are used as regional transfer conditions, which will produce k types of k×k conditional transition probability matrices (Table 4). The spatial transition probability of a region transitioning from state i in year t to state j in the next lag year, conditional on spatial lag type k, is denoted by
To calculate the level of adjacent areas in a certain area, the spatial weight matrix is used. That is,
3.3 Empirical research design
3.3.1 Variable
3.3.1.1 Explained variables
Referring to previous studies (Hu and Chen, 2023), this study measures pollution emission from the perspectives of air pollution and carbon emissions. This study uses per capita SO2 to measure air pollution and per capita CO2 to measure carbon emissions. The total SO2 emissions and total CO2 emissions are used as replacement variables for robustness testing. The data are logarithmized to avoid the influence of heteroscedasticity.
Based on the estimation method of the 2011 Provincial Greenhouse Gas Inventory Compilation Guidelines (Trial), and taking into account data availability and the actual carbon emission contribution of each energy source, this study selected 15 major energy sources to estimate carbon dioxide emissions. These 15 energy sources are raw coal, washed coal, other washed coal, coke, coke oven gas, crude oil, gasoline, kerosene, diesel, fuel oil, liquefied petroleum gas, refinery dry gas, natural gas, heat, and electricity. The consumption of electricity and heat does not directly produce carbon dioxide, so an indirect calculation method is used to estimate the carbon dioxide emissions of electricity and heat in each year (Hu and Chen, 2023).
3.3.1.2 Explanatory variables
The game theory combined weighting method and the coupling coordination degree model are used to measure the coupling coordination level (GDDF) of green finance and digital technology. The specific measurement method is as described above.
3.3.1.3 Control variables
Based on previous studies (Zhou et al., 2023; Hu and Chen, 2023), this study further controls for other potential factors that may affect Air Pollution and Carbon Emissions. This study selects economic development level (perGDP), population size (pop), industrial structure (IS), green tax (Gret) and green fiscal expenditure (Gref) as control variables.
The economic development level is measured by the logarithm of GDP per capita, the population size is measured by the logarithm of the total population at the end of the year, and the industrial structure is measured by the ratio of the tertiary industry to the secondary industry.
This study uses the ratio of environmental protection expenditure to local fiscal expenditure to represent green fiscal expenditure, and uses the ratio of the sum of environmental protection tax and resource tax to local tax revenue to represent green tax. The environmental protection taxes used in this study mainly include urban construction and maintenance tax, urban land use tax, and cultivated land occupation tax (Hu and Chen, 2023; Zhu and Lu, 2017; Jiang, 2018).
3.3.1.4 Instrumental variables
Referring to previous studies (Li et al., 2023; Zhang et al., 2020), this study uses the distance of each province to Zhejiang Province as an instrumental variable. Zhejiang Province not only has a typical case of coordinated development of digital technology and finance represented by Alipay, but is also the birthplace of the “Two Mountains Theory” and the location of two major green financial reform and innovation pilot zones. Its level of coordinated development of green finance and digital technology is at the forefront of the country. Therefore, it can be expected that the closer the province is to Zhejiang Province geographically, the higher the degree of coordinated development of green finance and digital technology will be.
3.3.1.5 Mechanism variable
Based on previous studies (Xiong and Chen, 2022), green innovation (GI) was selected as a mechanism variable for further analysis. Green innovation is measured by the number of green patent authorizations.
3.3.2 Model
This study examines the impact of the joint use of green finance and digital technology on reducing pollution. To achieve this, a double fixed-effect econometric model is constructed, and a fixed-effect model is selected through the Hausman test.
where:
In order to explore the transmission mechanism of the air pollution and carbon emission reduction effects of the coupling of green finance and digital technology, this study introduces green innovation (GI) to test the mechanism and constructs the following model based on existing research methods (Jiang and Luo, 2022).
where:
Other variables remain the same as in Formula 20.
4 Results
4.1 Indicator weighting results
This study utilized three different methods, subjective weighting, objective weighting, and game theory combined weighting, to calculate specific index weights for the evaluation index system. The results can be seen in Table 5.
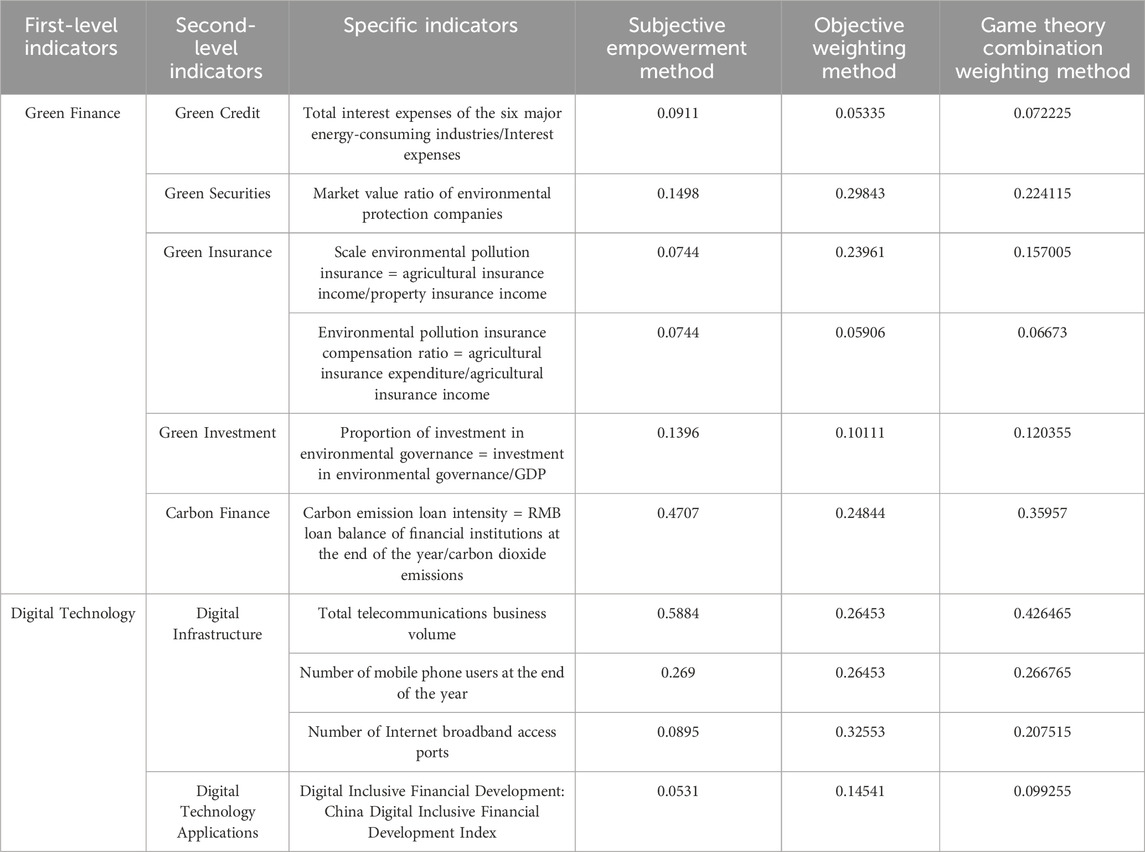
Table 5. Evaluation system for the coordinated development level of green finance and digital technology.
The measurement results of the coordinated development level of green finance and digital technology in different provinces in China are shown in Table 6.
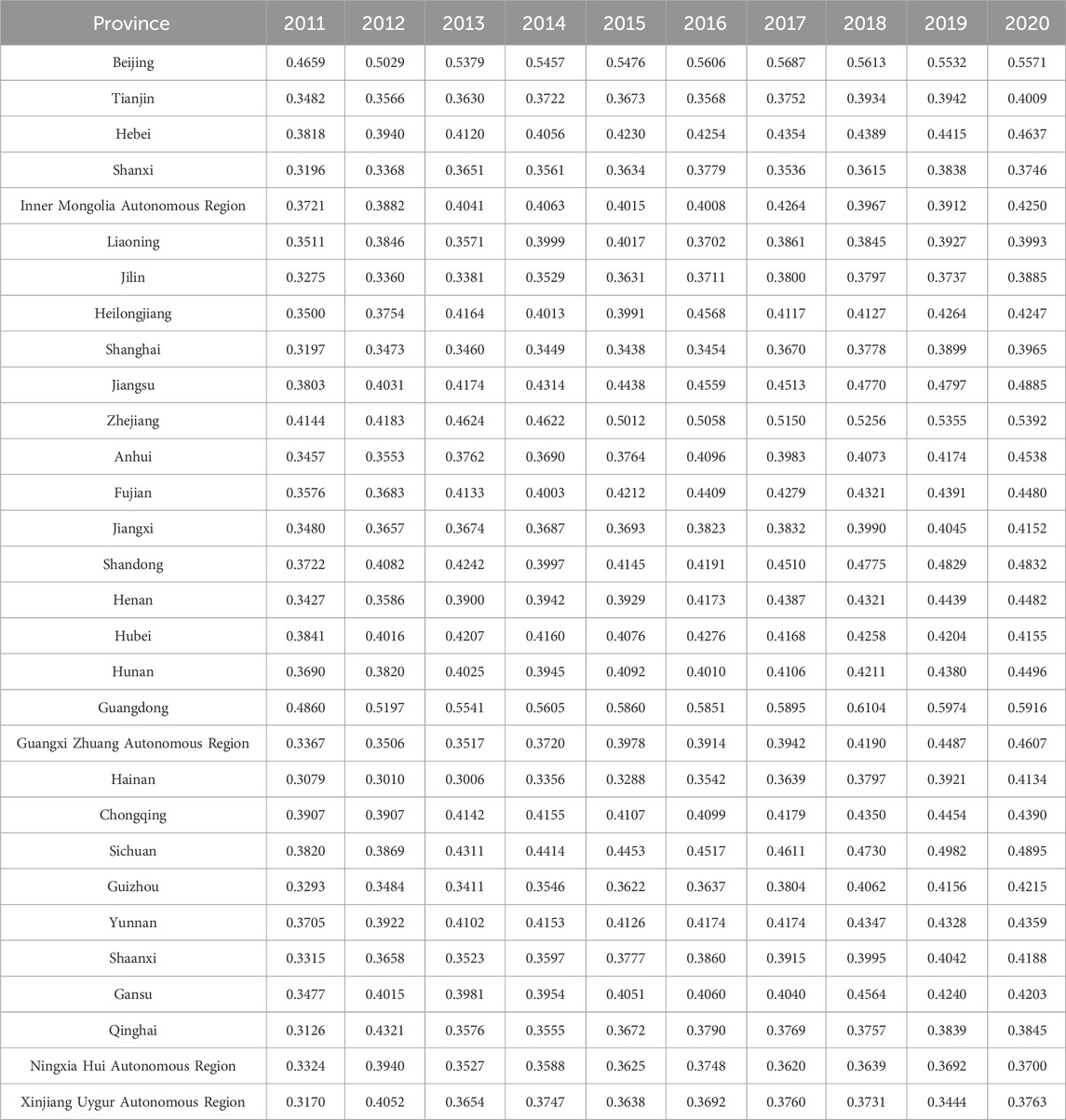
Table 6. Coupling and coordination degree of green finance and digital technology in different provinces in China.
4.2 Analysis of dynamic evolution characteristics
In this study, we have utilized the Kernel Density Estimation method to investigate the dynamic evolution patterns of the coordinated development levels of green finance and digital technology coupling across various provinces in China during the given period. Furthermore, we have analyzed the dynamic evolution characteristics of the coordinated development level of green finance and digital technology coupling in eastern, central, western, and northeastern China, considering the regional heterogeneity. The figure below shows the results obtained from the Kernel Density Estimation.
The trends in the coupling and coordinated development levels of green finance and digital technology in different provinces in China are shown in Figure 1. The kernel density curve shows that from the distribution position, the center position of the curve moves to the right, indicating that the level of coordinated development of green finance and digital technology in China is on the rise. From the perspective of distribution pattern, the core density curve generally shows that the main peak height decreases and the amplitude width generally increases, indicating that the absolute gap in the coordinated development level of green finance and digital technology coupling in different provinces in China increased during the sample period. The kernel density curves of the coordinated development levels of green finance and digital technology in different provinces in China have an obvious right tail, indicating that the level of coupled and coordinated development of green finance and digital technology in one province is much greater than that of another province. The kernel density curves of the coordinated development levels of green finance and digital technology coupling in different provinces in China do not show an obvious bimodal distribution, indicating that there are no two poles in the coupling and coordinated development levels of green finance and digital technology in different provinces in China.
To analyze the evolving trend of the coupling and coordinated development of green finance and digital technology in different regions, Figure 2 displays the estimated results for the eastern region. The kernel density curve results are shown, and from the distribution position, it can be seen that the center position of the curve generally moves towards the right, indicating an increase in the level of coordinated development of green finance and digital technology in the eastern region. The kernel density curve generally shows that the main peak height is decreasing, and the amplitude width is slightly widening. This suggests that the absolute gap in the coordinated development level of green finance and digital technology coupling in the eastern region has slightly increased during the sample period.
From the perspective of distribution extensibility, the kernel density curve of the coordinated development level of green finance and digital technology coupling in the eastern region has a noticeable right tail. This means that the level of coupled coordinated development of green finance and digital technology is much higher in one province than in another within the eastern region. Furthermore, there is no obvious bimodal distribution in the coordinated development level of green finance and digital technology coupling in the eastern region, which indicates that there is no polarization phenomenon.
The results of the estimation for the central region are displayed in Figure 3. The kernel density curve results are depicted in the figure. The position of the curve’s center generally moves to the right, indicating that the level of coordinated development between green finance and digital technology in the central region is on the rise. The kernel density curve generally shows that the main peak height is decreasing, and the amplitude width is slightly widening, indicating that the absolute disparity in the level of coordinated development between green finance and digital technology coupling in the central region has slightly increased during the sample period. The kernel density curve of the coordinated development level of green finance and digital technology coupling in the central region has a significant right tail, suggesting that the level of coupling and coordinated development of green finance and digital technology in one province is much higher than that in another province. The kernel density curve of the level of coupled and coordinated development of green finance and digital technology in the central region does not exhibit an obvious bimodal distribution, indicating that there is no significant polarization phenomenon in the level of coupled and coordinated development of green finance and digital technology in the central region.
The results of the estimation for the western region are presented in Figure 4. The results are represented in the form of a kernel density curve. Looking at the distribution position, we can observe that the center position of the curve generally moves to the right, indicating a rise in the level of coordinated development of green finance and digital technology in the western region. Considering the distribution shape, we can see that the height of the main peak shows a downward trend, and the amplitude width widens slightly. This suggests that the absolute gap in the coordinated development level of green finance and digital technology coupling in the western region has slightly increased during the sample period. From the perspective of distribution extensibility, the kernel density curve of the coordinated development level of green finance and digital technology coupling in the western region does not show a significant right tailing phenomenon. This indicates that no province in the western region has a much better level of coordinated development of green finance and digital technology than others. Lastly, the kernel density curve of the coordinated development level of green finance and digital technology in the western region does not show a bimodal distribution, which suggests that there is no significant polarization phenomenon in the coordinated development level of green finance and digital technology in the western region.
The results of the estimation for the Northeast region are presented in Figure 5, which displays the kernel density curve outcomes. From the perspective of the distribution position, the center position of the curve generally moves to the right, indicating that the level of coordinated development of green finance and digital technology coupling in Northeast China is generally increasing. From the distribution pattern viewpoint, the kernel density curve generally shows a downward trend in the main peak height, and the amplitude width generally widens slightly, indicating that the absolute gap in the level of coordinated development of green finance and digital technology coupling in Northeast China has slightly increased during the sample period. Regarding distributed ductility, the kernel density curve of the coordinated development level of green finance and digital technology in the Northeast doesn't have any apparent right-tail phenomenon, indicating a relatively uniform distribution between the provinces in the Northeast region. Similarly, there isn't any obvious double-peak distribution in the kernel density curve of the coordinated development level of green finance and digital technology in the Northeast, which demonstrates that there is no visible phenomenon of bipolar differentiation in the coordinated development level of green finance and digital technology coupling in the Northeast region.
To summarize, the coordination between green finance and digital technology has increased in the national average, east, central, west, and northeast regions of the country. However, there is a significant gap between these regions. One province stands out as having a much higher level of coordination compared to the national average, as well as the eastern and central regions. This phenomenon is not observed in the western and northeast regions. There is no clear evidence of bipolar differentiation in the coordinated development level of green finance and digital technology coupling across different regions.
4.3 Forecast of long-term evolution trend based on traditional Markov chain model
The Kernel density curve shows the overall form and dynamic evolution of the level distribution of this coupling, but does not reflect its long-term evolution trend. To predict this trend, the study uses a traditional Markov chain model. Firstly, the coordinated development level of green finance and digital technology is classified into four levels using the quadrilateral bias method. Then, the Markov chain transfer probability matrix of Chinese green finance and digital technology coupling coordination is calculated. The results are presented in Table 7.
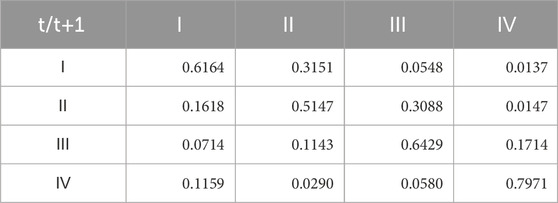
Table 7. Coupling coordination level Traditional Markov chain the model transfer probability matrix.
The results shown in Table 7 indicate that 61.64% of provinces have remained at a low-level year after year. 31.51% of provinces have moved from low to low-medium levels annually. The probability of a province moving to the medium-high level and high levels of coordinated development between green finance and digital technology is 5.48% and 1.37%, respectively. One year later, 51.47% of provinces maintain their unchanged low-medium level of coordinated development between green finance and digital technology. The probability of a province moving to the medium-high level of coordinated development is 30.88%. The probability of transitioning to a high level of coordinated development between green finance and digital technology is 1.47%, while 16.18% of provinces move to a low level. One year later, 64.29% of provinces still maintain their medium-high level of coordinated development between green finance and digital technology. 17.14% of provinces will move from their current level to a higher level of coordinated development. The probability of moving from the current level to the low-medium level of coordinated development between green finance and digital technology is 11.43%, and the probability of moving to a low level is 7.14%. After a year, 79.71% of provinces will still maintain their high level of coordinated development between green finance and digital technology. The probability of moving to the medium-high level of coordinated development between green finance and digital technology is 5.8%. The probability of moving down to a low-medium level and low-level of coordinated development between green finance and digital technology is 2.9% and 11.59%, respectively.
Based on the analysis results, it can be observed that in the Markov metastasis probability matrix, the probability of metastasis on the diagonal line is higher than the probability on the non-diagonal line. This indicates that when the probability of maintaining the original level is higher than the probability of transfer, the club with this level will converge. Thus, there is a clear phenomenon of “club convergence” in the coordinated development level of green finance and digital technology coupling. The convergence is more significant in medium-high and high levels, with probabilities of 64.29% and 79.71%, respectively. On the other hand, the probability of low-medium and low levels is less, with probabilities of 51.47% and 61.64%, respectively. This means that the probability of maintaining a stable development level of Chinese green finance and digital technology coupling is at least 51.47%. Additionally, it indicates that there is a “Matthew effect” of the rich and poor in the coordinated development level of Chinese green finance and digital technology coupling.
4.4 Long-term evolution trend forecast based on space Markov chain model
In order to keep up with the evolving trends in the coordinated development of Chinese green finance and digital technology, the Space Markov Chain has been adopted to analyze the impact of spatial factors on this development. The traditional Markov chain alone is not sufficient in reflecting the spatial overflow. To overcome this limitation, a spatial Markov transfer probability matrix has been built by adding space lag conditions to the traditional transfer probability matrix. By comparing the probability of coordinated development levels in different neighborhoods, we can analyze the impact of neighborhood backgrounds on the development of green finance and digital technology.
Table 8 reflects the spatial Markov chain transition probability of the coupling and coordinated development level of green finance and digital technology. The data shows that when the neighboring provinces are at a low level (k = 1), the probability of low-level provinces in the region remaining at the original level is 62.26%, and the probability of moving upward to the medium-low level is 30.19%. The probability of positive jump transfer to medium-high and high levels is 5.66% and 1.89% respectively. The probability that provinces with low-to-medium levels in this region will remain at the original level is 54.84%. The probability of upward transfer to medium-high levels is 19.35%, the probability of forward jump transfer to a high level is 3.23%, and the probability of downward transfer to a low level is 22.58%. The probability that provinces in this region are medium-high levels will remain at the original level is 73.08%, the probability of moving upward to a high level is 15.38%, the probability of moving downward to a medium-low level is 7.69%, and the probability of a negative jump to a low level is 3.85%. The probability that high-level provinces will remain at their original levels is 88.24%. The probability of downward transfer to medium-high levels is 5.88%, and the probability of negative jump transfer to medium-low and low levels is 0% and 5.89% respectively.
When the neighboring provinces are at medium-low level (k = 2), the probability of low-level provinces in the region remaining at the original level is 50%. The probability of upward transfer to medium-low levels is 50%, and the probability of forward jump transfer to medium-high levels is 0%. The probability that provinces with low-medium levels in this region will remain at the original level is 53.33%. The probability of upward transfer to medium-high levels is 40%, the probability of forward jump transfer to a high level is 6.67%, and the probability of downward transfer to a low level is 0%. Provinces with medium-high levels in this region have an 80% probability of remaining at the original level. The probability of upward transfer to a high level is 20%, the probability of downward transfer to a medium-low level is 0%, and the probability of negative jump transfer to a low level is also 0%. The probability of a high-level province remaining at the original level is 100%, the probability of moving downward to a medium-high level is 0%, and the probability of a negative jump to a medium-low level or low level is also 0%.
When the neighboring provinces are at medium and high levels (k = 3), the probability of low-level provinces in the region maintaining their original levels is 80%, the probability of moving upward to medium-low levels is 20%, and the probability of forward jumps to medium-high level and high level are both 0%. The probability of low-medium level provinces in this region maintaining the original level is 50%, the probability of moving upward to a medium-high level is 50%, and the probability of moving downward to a low level and jumping to a high level is 0%. The probability that provinces with medium-high levels in this region will maintain their original level is 60%, the probability of moving upward to a high level is 40%, and the probability of moving downward to a medium-low level and making a negative jump to a low level are both 0%. The probability of high-level provinces in this region maintaining the original level is 66.67%, the probability of moving downward to a medium-high level is 33.33%, and the probability of negative jump transfer is 0%.
When the neighboring provinces are at a high level (k = 4), the probability that the low-level provinces in the region remain at the original level is 53.85%. The probability of upward transfer to the middle-low level is 38.46%, the probability of forward jump transfer to the middle-high level is 7.69%, and the probability of high level is 0% respectively. The probability of provinces with medium-low levels in this region remaining at the original level is 43.48%, and the probability of moving upward to a medium-high level is 47.83%. The probability of a positive jump to a high level is 4.35%, and the probability of a downward jump to a low level is also 4.35%. The probability of provinces with medium-high levels in this region remaining at the original level is 66.67%. The probability of upward transfer to a high level is 20%, the probability of downward transfer to a medium-low level is 13.33%, and the probability of negative jump transfer to a low level is 0%. The probability of a high-level province remaining at the original level is 97.22%, the probability of moving downward to a medium-high level is 2.78%, and the probability of a negative jump to a medium-low level and low level is both 0%.
By comparing Tables 7, 8, the following conclusions can be drawn. First, the four transition probability matrices under the four spatial lag conditions are all different. It shows that when neighboring provinces have different levels of coupled and coordinated development of green finance and digital technology, the probabilities of being affected and transferred vary, and there is a certain degree of spatial and geographical dependence. Second, spatial factors have a significant impact on the dynamic transfer of the coordinated development level of green finance and digital technology. Third, the evolution of development types is unstable. In the four transition probability matrices in Table 8, the lower triangle area is not all 0, indicating that even if it is in a better development type in year t, it may still shift downward to a lower development type in year t + 1. In particular, the medium-low level and the medium-high level are most susceptible to transformation, indicating that the level of coordinated and coordinated development of green finance and digital technology is still unstable. Fourth, the level of coupled and coordinated development of green finance and digital technology in a province has an important impact on the future coupled and coordinated development of green finance and digital technology. Being adjacent to medium-high-level provinces, the probability of low-level development upward is only 20%, but the probability of medium-low level turning to high-level is 50%, which is a significant change. This shows that areas with higher levels of their own are more likely to move to higher levels. Fifth, there is an obvious club convergence phenomenon in the coupling and coordinated development of green finance and digital technology. The phenomenon of high-level convergence and low-level convergence is more significant, that is, the probability of maintaining stability in low-level and high-level areas on the diagonal are all relatively high, which also verifies the “Matthew Effect” existing in the coordinated development level of green finance and digital technology in the previous analysis.
5 Analysis of pollution reduction and emission reduction effects
5.1 Descriptive statistics
This study focuses on 30 provinces, autonomous regions, and municipalities in China, from 2011 to 2020. Tibet, Hong Kong, Macao, and Taiwan are not included due to missing data. To ensure accuracy, the data has been winsorized at the 5% level. Descriptive statistics for each variable can be found in Table 9.
5.2 Empirical test
5.2.1 Baseline regression
Table 10 reports the baseline regression results of the pollution emission effects of the coupling and coordination level of green finance and digital technology. The results of regression (1) show that, based on controlling for time and province, the coefficient of GDDF is significant at the 1% level, indicating that the coupled and coordinated development of green finance and digital technology has a significant air pollution reduction effect. The results of regression (2) show that the coefficient of GDDF is significant at the 5% level, indicating that the coupled and coordinated development of green finance and digital technology has a significant carbon emission reduction effect. Hypothesis 1 is verified. This shows that the coupling of green finance and digital technology has a significant emission reduction effect. Since the development of green finance has certain environmental restrictions, digital technology can provide a paperless platform for the development of green finance, realize the dissemination and sharing of lending information, break the barriers of information dissemination, achieve green economic development, and then promote global sustainable development.
An analysis of the control variables found that the level of economic development (perGDP) has a certain inhibitory effect on pollution reduction and emission reduction, and has a significant impact on reducing air pollution, but has an insignificant impact on carbon dioxide emission reduction. The impact of industrial structure (IS) on pollution reduction and emission reduction is not significant. Green tax policy (Gret) has a certain inhibitory effect on pollution reduction and emission reduction, and has a significant impact on reducing air pollution, but has an insignificant effect on carbon dioxide emission reduction. Green fiscal expenditure (Gref) has a significant promoting effect on reducing air pollution, but has an insignificant inhibitory effect on carbon dioxide emission reduction. Population will increase air pollution and carbon dioxide emissions, and will have a significant impact on increasing carbon dioxide.
5.2.2 Robustness analysis
First, the original data before winnowing are used for robustness testing. The results are shown in regressions (1) and (2) of Table 11. Secondly, the explained variables are replaced for robustness testing. The logarithm of total SO2 emissions and total CO2 emissions was used to conduct robustness testing, and the results are shown in regressions (3) and (4) in Table 11.
Table 11 shows that after using the original data and replacing the explained variables, the regression results of the pollution emission reduction effects of the coupled and coordinated development of green finance and digital technology are still significant. The estimation results are consistent with the previous baseline regression results. It further proves that the coupled and coordinated development of green finance and digital technology has significant pollution emission reduction effects.
5.2.3 Endogeneity analysis
Endogeneity problems may still exist when conducting robustness tests by replacing the explained variables. Therefore, this study draws on the practices of previous scholars (Li et al., 2023; Zhang et al., 2020) and selects the product of the distance from each province to Zhejiang Province and the average value of the coordinated development of green finance and digital technology in other regions except this province as the instrumental variable to conduct endogeneity testing. Because Zhejiang Province not only has typical cases of coordinated development of digital technology and finance represented by Alipay, but is also the birthplace of the “Two Mountains Theory” and the location of two major green financial reform and innovation pilot zones. Its level of coordinated development of green finance and digital technology is at the forefront of the country. Therefore, it can be expected that the closer the geographical distance to Zhejiang Province, the higher the level of coordinated development of green finance and digital technology will be.
The results are displayed in Table 12, where Regression (1) and (2) present the regression outcomes of the second stage. The coefficients before GDDF are all positive, indicating that the coordinated development of green finance and digital technology still has a significant pollution emission reduction effect after taking potential endogeneity issues into account. This result is consistent with the baseline regression conclusion. It shows that the coupled and coordinated development of green finance and digital technology can achieve the goal of pollution emission reduction.
5.3 Further analysis: mechanism testing
The above-mentioned research shows that the coupled development of green finance and digital technology can produce significant pollution emission reduction effects, which is of great significance to the realization of China’s “double carbon” goal and global sustainable development under the new development pattern. Then, what needs to be further explored is how the coupling of green finance and digital technology can help achieve pollution emission reduction goals.
Based on the theoretical mechanism analysis, this study introduces green innovation (GI) to explore the transmission mechanism of green finance and digital technology coupling coordination to reduce air pollution and carbon emissions. Drawing on the existing research methods (Jiang and Luo, 2022), the relationship between each mediating variable and the dependent variable is first determined through theoretical analysis, and then the impact of the coupling coordination level of green finance and digital technology on the mediating variable is empirically tested. Table 13 reports the mechanism test based on green innovation. The regression results show that the coefficient of coupling and coordination level of green finance and digital technology is significantly positive, indicating that the coordinated development of green finance and digital technology has a significant promotion effect on green innovation. The coupling and coordination of green finance and digital technology achieve pollution emission reduction by driving green innovation. Hypothesis 2 is verified. This is similar to the conclusion drawn by Li et al. (2023).
The control variable results show that the coefficient of economic development level (perGDP) is 0.321, which is significantly positive at the 1% level, indicating that the level of economic development has a significant promoting effect on green innovation. The coefficients of industrial structure and green tax policy are both positive and insignificant, indicating that their impact on green innovation is not significant. The coefficient of green tax policy is −2.388, which is significant at the 1% level, indicating that green tax policy has a significant promotion effect on green innovation. The coefficient of population size is 1.139, which is significant at the 1% level, indicating that population size has a significant promotion effect on green innovation.
6 Discussion
First, this study found that the level of green finance and digital technology coupling coordination in the entire sample of Chinese provinces, provinces in the eastern region, provinces in the central region, provinces in the western region, and provinces in the northeast region showed an upward trend, and there were differences in different regions, which is similar to the trend of studying the single status of green finance (Xu and Jiang, 2021)and digital finance (Sui and Xu, 2024). Compared with other studies, this study newly found that there is an obvious club convergence phenomenon in the level of green finance and digital technology coupling coordination development, and there is a “Matthew effect.” This is due to the imbalance of regional development in China (Xing and He, 2024) and resource mismatch (Lu and Lu, 2024).
Second, this study mainly analyzes the pollution reduction and emission reduction effects brought about by the coupled and coordinated development of digital technology and green finance. Similar conclusions to previous studies show that digital finance or green finance has a significant effect on reducing pollution and emissions. Digital finance connects the business systems of financial institutions with the underlying data platform of the green information system, shortens the time for financial institutions to identify green projects, simplifies the approval and issuance process of green loans, and improves the efficiency of financial services. Secondly, digital finance is mainly based on online virtual outlets. With the help of business models such as Internet banking, it relies on massive customer resources and digital technology to form strong network externalities, conduct contactless transactions, reduce the energy consumption of cash, paper, and rides during transactions, and promote green development. Green development plays a key role in achieving pollution reduction and emission reduction goals. This approach can reduce the moral hazard caused by information asymmetry and enable enterprises to more smoothly use green finance to reduce pollution and control emissions (Zhao et al., 2024b; Wang et al., 2024b; Wang et al., 2024b; Magazzino et al., 2023). In addition, the coupled and coordinated development of green finance and digital technology can exacerbate financing constraints for the progress and development of ecological pollution-related enterprises. However, existing research only considers the carbon emission reduction effect of a single aspect of green finance or digital finance (Xie and Liu, 2019; Guo et al., 2022; Mao and Wang, 2023; Ran and Zhang, 2023). This study examines the pollution reduction effect of the coupled and coordinated development of the two. Empirical results show that after controlling for population, economic development level, industrial structure development level, green tax policy and green fiscal policy, the coupled and coordinated development of green finance and digital technology has a significant pollution reduction effect.
The main reason for this result is that the improvement of digital transformation construction enables the government to efficiently and accurately obtain carbon emission-related data and formulate timely response measures (Alam and Murad, 2020). Secondly, compared with green enterprises, the coordinated development of green finance and digital technology breaks the barriers to the penetration of green finance and green benefit information, improves the ability to process and identify green financial information, and effectively drives green technology innovation in polluting enterprises (Li et al., 2023). Finally, by utilizing the digital infrastructure effect to improve the coverage and efficiency of green financial services, and using infrastructure such as information and communication technology to improve energy efficiency through a shared resource network, urban carbon emissions can be reduced and green development can be promoted (Anser et al., 2021; Lange et al., 2020).
Third, Schumpeter’s innovation theory suggests that financial development has a strong supporting role in innovation (Aghion et al., 2012). Since green innovation has the characteristics of sunk investment, uncertainty of results and high adjustment costs, it is difficult to meet the funds required for innovation by relying solely on internal corporate funds and national R&D expenditures. Therefore, external financing is the key to promoting technological innovation. The development of digital finance makes up for the mismatch of attributes, fields and stages in traditional financial services, improves the efficiency of capital allocation, and can effectively support corporate green technology innovation and enable green development. On the one hand, digital finance has lowered the threshold and cost of financial services, increased the availability of financial resources, and can provide more targeted financial support for corporate R&D and innovation (Nie et al., 2021), thereby improving the utilization rate of financial resources, accelerating the flow of innovation capital, and increasing the number of green technology innovation results (Tang et al., 2020). On the other hand, digital finance uses digital technology to search, analyze and make decisions on consumer preference information for green products. It helps enterprises determine the direction and path of green innovation, promotes the transformation of enterprise innovation from experience-driven to data-driven (Liu et al., 2020b), realizes effective green technology R&D decision-making, and improves the quality of green technology innovation. Secondly, the improvement of green technology innovation capabilities has the effect of energy conservation and emission reduction, and promotes green development. On the one hand, enterprises with strong green technology innovation improve energy utilization and reduce energy consumption by developing new or improving existing technologies. On the other hand, enterprises penetrate green technology into all aspects of production, break the path dependence of the development of polluting industries, guide enterprises to save energy and reduce emissions, reduce pollutant emissions based on production output, and are conducive to green development (Mao and Wang, 2023). This study examines the mechanism of green innovation in the coordinated development of green finance and digital technology on pollution reduction and emission reduction. The results show that the coordinated development of green finance and digital technology achieves pollution reduction and emission reduction through green innovation. This is similar to the conclusion of the previous study.
This result is mainly due to the fact that digital technology can enhance the financing capacity of enterprises by breaking down the information barriers of green finance, providing digital platforms and innovative service support measures, thereby increasing investment in research and development. This in turn promotes technological innovation in enterprises and promotes the adoption of green technologies, thereby promoting innovation and directly achieving pollution reduction through the coordinated development of green finance and digital technology. In addition, technological innovation reduces carbon emissions by promoting the development of the renewable energy industry and reducing energy intensity (Han and Gu, 2021; Cheng et al., 2021b; Yao and Yang, 2022), and this phenomenon also exists in some countries (Miśkiewicz, 2021; Ullah et al., 2021).
Finally, this study provides a model and ideas for developing countries to analyze the current level of coordinated development of green finance and digital technology. It provides a new policy-making path for achieving the coordinated development of green finance and digital technology in China at this stage. It is of great significance to the green transformation of the economy and high-quality development, and also provides empirical evidence and reference for other developing countries to achieve the coordinated development of green finance and digital technology and promote pollution reduction and emission reduction.
7 Conclusion and outlook
7.1 Conclusion
This study employs the game theory combination weighting method to evaluate the degree of coordinated development between green finance and digital technology in China. It uses Kernel density estimation, traditional Markov chain model, and spatial Markov chain model to analyze the dynamic evolution characteristics and trends, and also examines the pollution reduction effect of the coordinated development of green finance and digital technology through empirical testing. The key findings of this study are as follows.
First, the level of coupling and coordination between green finance and digital technology is increasing in all Chinese provinces, including the eastern, central, western, and northeastern regions. However, there is a noticeable difference in the level of coordinated development between different provinces, particularly in the eastern and central regions. This difference is not as pronounced in the western and northeastern regions. Overall, there is no significant polarization in the level of coupling and coordinated development of green finance and digital technology across all regions.
Second, The traditional Markov transition probability matrix results of China’s green finance and digital technology coupling and coordinated development level show that there is an obvious “club convergence” phenomenon. This shows that there is a “Matthew effect” in which the rich get richer and the poor get poorer in China’s coupled and coordinated development of green finance and digital technology. Spatial factors are further introduced and the spatial Markov chain model is used for research. The results show that spatial factors have a significant impact on the dynamic transfer of the coordinated development level of green finance and digital technology, and the evolution of development types is unstable. The level of provincial green finance and digital technology coupling and coordinated development has an important impact on the future green finance and digital technology coupling and coordinated development. The results show that regions with higher levels are more likely to move to higher levels.
Third, through empirical testing, it is found that the coupled and coordinated development of green finance and digital technology has significant pollution emission reduction effects. Further mechanism testing of the pollution emission reduction effects of the coupling of green finance and digital technology showed that the coupling of green finance and digital technology achieves pollution emission reduction by driving green innovation.
7.2 Recommendations and outlook
First, we should continue to enhance and develop green finance, encourage the use of digital technology, and promote the optimization of industrial structures. Currently, the focus of domestic green finance is mainly on green credit, which makes it challenging to meet the financing requirements of different levels, terms, and natures. There is an urgent need for rich green financial products, convenient investment and financing processes, and scientific and reasonable financial supervision to support industrial transformation and structural optimization. In addition, none of the published green finance policy documents reflects attitudes towards greenhouse gases such as carbon dioxide, making governance less precise. If greenhouse gas emission indicators such as carbon dioxide can be included in the financing conditions of green financial products and paperless product transactions can be implemented, it will be more conducive to promoting pollution reduction and carbon reduction.
Second, we can adjust the green tax rate and scale. Resource tax and environmental protection tax rates are low, which is not conducive to environmental governance. Pilot work can be carried out in areas with a low proportion of green taxes and good economic development levels, appropriately increase the tax rates on taxable resources and pollutants, increase green tax revenue, and adjust the scale of green taxation to a reasonable range. At the same time, green fiscal policies such as tax incentives and financial subsidies for new energy, renewable energy, and industrial structure optimization will be adopted.
Third, we can optimize green fiscal spending. The scope of environmental protection expenditures is limited to pollution control, environmental management, and monitoring, and there is a lack of supervision and management of greenhouse gases such as carbon dioxide. Therefore, adjusting the scope of environmental protection expenditures, paying more attention to carbon emissions, and releasing policy signals and determination to intervene will promote green finance to better exert its effects on pollution reduction and carbon reduction.
Fourth, we can build and optimize a digital green financial product trading platform, provide a paperless business processing platform, and implement platform supervision to achieve pollution reduction and emission reduction effects.
This study examines the current characteristics and pollution reduction effects of the coordinated development of green finance and digital technology in China, in light of the progress of society and the rapid growth of the economy and the Internet. However, there are also some shortcomings in the writing process of this article, which need to be further studied in the future. The impact pathway of the coordinated development of green finance and digital technology has not been specifically analyzed, and there is still room for discussion. Due to limited time, this article did not delve deeper into this aspect, which needs to be further explored in subsequent research.
Data availability statement
The raw data supporting the conclusions of this article will be made available by the authors, without undue reservation.
Author contributions
KL: Funding acquisition, Methodology, Writing–original draft. RD: Software, Writing–original draft. BX: Software, Resources, Data curation, Writing–review and editing. JH: Resources, Conceptualization, Writing–review and editing. ZW: Conceptualization, Resources, Writing–review and editing.
Funding
The author(s) declare that financial support was received for the research, authorship, and/or publication of this article. Supported by Excellent Graduate Training Program of Shaanxi Normal University, Grant/Award Number: LHRCTS23012 (KL). General project of the National Social Science Fund of China, Grant/Award Number: 21BJY086 (ZW). The funders had no role in study design, data collection and analysis, decision to publish, or preparation of the manuscript.
Conflict of interest
The authors declare that the research was conducted in the absence of any commercial or financial relationships that could be construed as a potential conflict of interest.
Publisher’s note
All claims expressed in this article are solely those of the authors and do not necessarily represent those of their affiliated organizations, or those of the publisher, the editors and the reviewers. Any product that may be evaluated in this article, or claim that may be made by its manufacturer, is not guaranteed or endorsed by the publisher.
References
Aghion, P., Askenazy, P., Berman, N., Cette, G., and Eymard, L. (2012). Credit constraints and the cyclicality of R&d investment: evidence from France. J. Eur. Econ. Assoc. 10, 1001–1024. doi:10.1111/j.1542-4774.2012.01093.x
Alam, M. M., and Murad, M. W. (2020). The impacts of economic growth, trade openness and technological progress on renewable energy use in organization for economic co-operation and development countries. Renew. Energy 145, 382–390. doi:10.1016/j.renene.2019.06.054
Anser, M. K., Ahmad, M., Khan, M. A., Zaman, K., Nassani, A. A., Askar, S. E., et al. (2021). The role of information and communication technologies in mitigating carbon emissions: evidence from panel quantile regression. Environ. Sci. Pollut. Res. 28, 21065–21084. doi:10.1007/s11356-020-12114-y
Bao, J., and He, M. (2022). Does green credit promote green sustainable development in regional economies? empirical evidence from 280 cities in China. PLOS ONE 17, e0277569. doi:10.1371/journal.pone.0277569
Cheng, C., Ren, X., Dong, K., Dong, X., and Wang, Z. (2021b). How does technological innovation mitigate CO2 emissions in OECD countries? Heterogeneous analysis using panel quantile regression. J. Environ. Manag. 280, 111818. doi:10.1016/j.jenvman.2020.111818
Cheng, J., Tong, D., Zhang, Q., Liu, Y., Lei, Y., Yan, G., et al. (2021a). Pathways of China’s PM2.5 air quality 2015-2060 in the context of carbon neutrality. Natl. Sci. Rev. 8, nwab078. doi:10.1093/nsr/nwab078
Chin, M.-Y., Ong, S.-L., Ooi, D. B.-Y., and Puah, C.-H. (2024). The impact of green finance on environmental degradation in BRI region. Environ. Dev. Sustain 26, 303–318. doi:10.1007/s10668-022-02709-5
Chiu, Y.-B., and Lee, C.-C. (2020). Effects of financial development on energy consumption: the role of country risks. Energy Econ. 90, 104833. doi:10.1016/j.eneco.2020.104833
Ding, Q., Khattak, S. I., and Ahmad, M. (2021). Towards sustainable production and consumption: assessing the impact of energy productivity and eco-innovation on consumption-based carbon dioxide emissions (CCO2) in G-7 nations. Sustain. Prod. Consum. 27, 254–268. doi:10.1016/j.spc.2020.11.004
Dong, F., Yu, B., and Pan, Y. (2019). Examining the synergistic effect of CO2 emissions on PM2.5 emissions reduction: evidence from China. J. Clean. Prod. 223, 759–771. doi:10.1016/j.jclepro.2019.03.152
Gianfrate, G., and Peri, M. (2019). The green advantage: exploring the convenience of issuing green bonds. J. Clean. Prod. 219, 127–135. doi:10.1016/j.jclepro.2019.02.022
Guo, C.-Q., Wang, X., Cao, D.-D., and Hou, Y.-G. (2022). The impact of green finance on carbon emission--analysis based on mediation effect and spatial effect. Front. Environ. Sci. 10. doi:10.3389/fenvs.2022.844988
Guo, Z., and Qiu, Y. (2020). The reconstruction of global value chains in the era of digital economy: typical features, theoretical mechanisms and China’s counter measures. Reform, 73–85.
Han, H., and Gu, X. (2021). Linkage between inclusive digital finance and high-tech enterprise innovation performance: role of debt and equity financing. Front. Psychol. 12, 814408. doi:10.3389/fpsyg.2021.814408
Hao, Y., Wang, L., Zhu, L., and Ye, M. (2018). The dynamic relationship between energy consumption, investment and economic growth in China’s rural area: new evidence based on provincial panel data. Energy 154, 374–382. doi:10.1016/j.energy.2018.04.142
He, L., Liu, R., Zhong, Z., Wang, D., and Xia, Y. (2019a). Can green financial development promote renewable energy investment efficiency? A consideration of bank credit. Renew. Energy 143, 974–984. doi:10.1016/j.renene.2019.05.059
He, L., Zhang, L., Zhong, Z., Wang, D., and Wang, F. (2019b). Green credit, renewable energy investment and green economy development: empirical analysis based on 150 listed companies of China. J. Clean. Prod. 208, 363–372. doi:10.1016/j.jclepro.2018.10.119
Hoque, M. A.-A., Phinn, S., Roelfsema, C., and Childs, I. (2017). Tropical cyclone disaster management using remote sensing and spatial analysis: a review. Int. J. Disaster Risk Reduct. 22, 345–354. doi:10.1016/j.ijdrr.2017.02.008
Hu, G., Wang, X., and Wang, Y. (2021). Can the green credit policy stimulate green innovation in heavily polluting enterprises? Evidence from a quasi-natural experiment in China. Energy Econ. 98, 105134. doi:10.1016/j.eneco.2021.105134
Hu, J., and Chen, X. (2023). Will green fiscal policy influence the effect of green finance? From the perspective of reducing pollution and carbon emission. Collect. Essays Finance Econ., 25–35. doi:10.13762/j.cnki.cjlc.2023.10.003
Huang, Q., Yu, Y., and Zhang, S. (2019). Internet development and productivity growth in manufacturing industry: internal mechanism and China experiences. China Ind. Econ., 5–23. doi:10.19581/j.cnki.ciejournal.2019.08.001
Jiang, L., Wang, H., Tong, A., Hu, Z., Duan, H., Zhang, X., et al. (2020). The measurement of green finance development index and its poverty reduction effect: dynamic panel analysis based on improved entropy method. Discrete Dyn. Nat. Soc. 2020, 1–13. doi:10.1155/2020/8851684
Jiang, N. (2018). Does environmental fiscal spending help achieve economic and environmental win-wins? J. Zhongnan Univ. Econ. Law., 95–103. doi:10.19639/j.cnki.issn1003-5230.2018.0010
Jiang, Q., Rahman, Z. U., Zhang, X., and Islam, M. S. (2022). An assessment of the effect of green innovation, income, and energy use on consumption-based CO2 emissions: empirical evidence from emerging nations BRICS. J. Clean. Prod. 365, 132636. doi:10.1016/j.jclepro.2022.132636
Jiang, T., and Luo, Z. B. (2022). LOC102724163 promotes breast cancer cell proliferation and invasion by stimulating MUC19 expression. China Ind. Econ. 23, 100–120. doi:10.3892/ol.2022.13220
Lange, S., Pohl, J., and Santarius, T. (2020). Digitalization and energy consumption. Does ICT reduce energy demand? Ecol. Econ. 176, 106760. doi:10.1016/j.ecolecon.2020.106760
Lee, C.-C., Lee, C.-C., and Li, Y.-Y. (2021b). Oil price shocks, geopolitical risks, and green bond market dynamics. North Am. J. Econ. Finance 55, 101309. doi:10.1016/j.najef.2020.101309
Lee, C.-C., Lee, C.-C., and Xiao, S. (2021a). Policy-related risk and corporate financing behavior: evidence from China’s listed companies. Econ. Model. 94, 539–547. doi:10.1016/j.econmod.2020.01.022
Lee, C.-C., Wang, C.-W., and Ho, S.-J. (2022). Financial aid and financial inclusion: does risk uncertainty matter? Pacific-Basin Finance J. 71, 101700. doi:10.1016/j.pacfin.2021.101700
Li, S. (2021). Digital technology empowers productivity of services industry: theoretical mechanisms and empirical evidence. Research on Economics and Management, 42, 51–67. doi:10.13502/j.cnki.issn1000-7636.2021.10.004
Li, X., Fang, X., Yang, Z., and Zhang, Y. (2023). The impact of collaborative development of green finance and digital technology on enterprise green technology innovation. J. East China Normal Univ. Soc. Sci. 55, 139–158+174. doi:10.16382/j.cnki.1000-5579.2023.06.012
Liu, C., and Xiong, M. (2022). Green finance reform and corporate innovation: evidence from China. Finance Res. Lett. 48, 102993. doi:10.1016/j.frl.2022.102993
Liu, D., Chang, Y., Yao, H., and Kang, Y. (2023b). The impact of green finance on green economy development efficiency: based on panel data of 30 provinces in China. Front. Environ. Sci. 11. doi:10.3389/fenvs.2023.1328914
Liu, G., Wang, B., Cheng, Z., and Zhang, N. (2020a). The drivers of China’s regional green productivity, 1999–2013. Resour. Conservation Recycl. 153, 104561. doi:10.1016/j.resconrec.2019.104561
Liu, R., Wang, D., Zhang, L., and Zhang, L. (2019). Can green financial development promote regional ecological efficiency? A case study of China. Nat. Hazards 95, 325–341. doi:10.1007/s11069-018-3502-x
Liu, Y., Xie, K., and Deng, H. (2020b). Data-driven R&D transformation of new product:A case study from the perspective of adaptive change in organizational practices. J. Manag. World 36, 164–183. doi:10.19744/j.cnki.11-1235/f.2020.0040
Liu, Y., and Xu, R. (2024). Money talks: how financial constraint influence corporate environmental behavior prioritization. Int. Rev. Econ. & Finance 91, 556–578. doi:10.1016/j.iref.2024.01.014
Liu, Z., Zhang, X., Wang, J., Shen, L., and Tang, E. (2023a). Evaluation of coupling coordination development between digital economy and green finance: evidence from 30 provinces in China. PLOS ONE 18, e0291936. doi:10.1371/journal.pone.0291936
Lu, W., and Lu, S. (2024). A study on loan interest rate liberalization, banking competition and resource misallocation in cities. Economist, 45–56. doi:10.16158/j.cnki.51-1312/f.2024.07.003
Magazzino, C., Pakrooh, P., and Abedin, M. Z. (2023). A decomposition and decoupling analysis for carbon dioxide emissions: evidence from OECD countries. Environ. Dev. Sustain 26, 28539–28566. doi:10.1007/s10668-023-03824-7
Mao, X., and Wang, R. (2023). Digital finance and green development ——empirical evidence from 286 cities in China. Finance Forum 28, 69–80. doi:10.16529/j.cnki.11-4613/f.2023.09.006
Meirun, T., Mihardjo, L. W., Haseeb, M., Khan, S. A. R., and Jermsittiparsert, K. (2021). The dynamics effect of green technology innovation on economic growth and CO2 emission in Singapore: new evidence from bootstrap ARDL approach. Environ. Sci. Pollut. Res. 28, 4184–4194. doi:10.1007/s11356-020-10760-w
Miśkiewicz, R. (2021). The impact of innovation and information technology on greenhouse gas emissions: a case of the visegrád countries. J. Risk Financial Manag. 14, 59. doi:10.3390/jrfm14020059
Myerson, R. B. (1991). Game theory: analysis of conflict. Harvard University Press. doi:10.2307/j.ctvjsf522
Nie, X., Jiang, P., Zheng, X., and Wu, Q. (2021). Research on digital finance and regional technology innovation. J. Financial Res., 132–150.
Niu, H., Zhao, X., Luo, Z., Gong, Y., and Zhang, X. (2022). Green credit and enterprise green operation: based on the perspective of enterprise green transformation. Front. Psychol. 13, 1041798. doi:10.3389/fpsyg.2022.1041798
Qi, J., and Ren, Y. (2021). The impact of digital economy penetration on global value chain position —transnational empirical research based on industrial heterogeneity. J. Int. Trade, 105–121. doi:10.13510/j.cnki.jit.2021.09.007
Ran, C., and Zhang, Y. (2023). The driving force of carbon emissions reduction in China: does green finance work. J. Clean. Prod. 421, 138502. doi:10.1016/j.jclepro.2023.138502
Ren, X., Shao, Q., and Zhong, R. (2020). Nexus between green finance, non-fossil energy use, and carbon intensity: empirical evidence from China based on a vector error correction model. J. Clean. Prod. 277, 122844. doi:10.1016/j.jclepro.2020.122844
Sala-i-Martin, X. (2006). The world distribution of income: falling poverty and convergence, period. Q. J. Econ. 121, 351–397. doi:10.1162/qjec.2006.121.2.351
Simionescu, M., and Gavurová, B. (2023). Pollution, income inequality and green finance in the new EU member states. Humanit Soc. Sci. Commun. 10, 677–711. doi:10.1057/s41599-023-02197-6
Sui, X., and Xu, N. (2024). Green finance’s supporting carbon neutrality:its current situations, mechanisms and paths. Scientific Decision Making, 153–167.
Sun, G., Wang, Y., and Li, Q. (2017). The influence of green credits on credit risks of commercial banks. Finance Forum 22, 31–40. doi:10.16529/j.cnki.11-4613/f.2017.10.006
Tang, S., Wu, X., and Zhu, J. (2020). Digital finance and enterprise technology innovation:structural Feature,Mechanism identification and effect difference under financial supervision. J. Manag. World 36, 52–66+9. doi:10.19744/j.cnki.11-1235/f.2020.0069
Tariq, A., and Hassan, A. (2023). Role of green finance, environmental regulations, and economic development in the transition towards a sustainable environment. J. Clean. Prod. 413, 137425. doi:10.1016/j.jclepro.2023.137425
The Study Group of Development Research (2015). Institute of southwestern university of finance, economics and research center for environment and economic policy of the MEP. Green finance and sustainable development. Finance Forum 20, 30–40. doi:10.16529/j.cnki.11-4613/f.2015.10.009
Truong, T. C. (2022). The impact of digital transformation on environmental sustainability. Adv. Multimedia 2022, 1–12. doi:10.1155/2022/6324325
Ullah, S., Ozturk, I., Majeed, M. T., and Ahmad, W. (2021). Do technological innovations have symmetric or asymmetric effects on environmental quality? Evidence from Pakistan. J. Clean. Prod. 316, 128239. doi:10.1016/j.jclepro.2021.128239
Wang, E.-Z., Lee, C.-C., and Li, Y. (2022). Assessing the impact of industrial robots on manufacturing energy intensity in 38 countries. Energy Econ. 105, 105748. doi:10.1016/j.eneco.2021.105748
Wang, L., Yang, X., and Cai, Q. (2024b). Influence mechanism of green finance on regional emission reduction. Heliyon 10, e23861. doi:10.1016/j.heliyon.2023.e23861
Wang, R., Mirza, N., Vasbieva, D. G., Abbas, Q., and Xiong, D. (2020). The nexus of carbon emissions, financial development, renewable energy consumption, and technological innovation: what should be the priorities in light of COP 21 Agreements? J. Environ. Manag. 271, 111027. doi:10.1016/j.jenvman.2020.111027
Wang, Y., Liu, J., Yang, X., Shi, M., Ran, R., Wang, Y., et al. (2023). The mechanism of green finance’s impact on enterprises’ sustainable green innovation. GF 5, 452–478. doi:10.3934/GF.2023018
Wang, Y., Liu, S., Abedin, M. Z., and Lucey, B. (2024a). Volatility spillover and hedging strategies among Chinese carbon, energy, and electricity markets. J. Int. Financial Mark. Institutions Money 91, 101938. doi:10.1016/j.intfin.2024.101938
Wang, Y., and Ren, Y. (2022). Construction of green financial system under the carbon peak and carbon neutrality goals. Mod. Econ. Sci. 44, 1–13+139.
Wen, H., Lee, C.-C., and Zhou, F. (2022). How does fiscal policy uncertainty affect corporate innovation investment? Evidence from China’s new energy industry. Energy Econ. 105, 105767. doi:10.1016/j.eneco.2021.105767
Wu, G., Sun, M., and Feng, Y. (2024a). How does the new environmental protection law affect the environmental social responsibility of enterprises in Chinese heavily polluting industries? Humanit Soc. Sci. Commun. 11, 168–214. doi:10.1057/s41599-024-02674-6
Wu, K., Liu, S., Zhu, M., and Qu, Y. (2024b). The impact of digital transformation on resource mismatch of Chinese listed companies. Sci. Rep. 14, 9011. doi:10.1038/s41598-024-59285-z
Wu, Z., Zeng, J., Liu, H., and Li, C. (2023). Mechanism and spatial effect of green finance promoting the pollution control and emission reduction. Econ. Geogr. 43, 128–138. doi:10.15957/j.cnki.jjdl.2023.10.013
Xia, F., and Xu, J. (2020). Green total factor productivity: a re-examination of quality of growth for provinces in China. China Econ. Rev. 62, 101454. doi:10.1016/j.chieco.2020.101454
Xiang, S., and Tian, R. (2022). Input digitization and climbing global value chain in manufacturing: a global test based on world input-output data. Int. Bus. Res. 43, 13–26. doi:10.13680/j.cnki.ibr.2022.06.009
Xie, T.-T., and Liu, J.-H. (2019). How does green credit affect China’s green economy growth? Chin. J. Of Popul. Resour. And Environ. 29, 83–90. doi:10.12062/cpre.20190501
Xing, Z., and He, C. (2024). Regional inequality: theoretical review, research progress, and prospects. Prog. Geogr. 43, 1839–1852. doi:10.18306/dlkxjz.2024.09.012
Xiong, J., and Chen, L. (2022). Does industrial up-gradation, environment regulations, and resource allocation impact on foreign direct investment: empirical evidence from China. Front. Psychol. 13, 999953. doi:10.3389/fpsyg.2022.999953
Xiong, X., Wang, Y., Liu, B., He, W., and Yu, X. (2023). The impact of green finance on the optimization of industrial structure: evidence from China. PLOS ONE 18, e0289844. doi:10.1371/journal.pone.0289844
Xu, L., Fan, M., Yang, L., and Shao, S. (2021). Heterogeneous green innovations and carbon emission performance: evidence at China’s city level. Energy Econ. 99, 105269. doi:10.1016/j.eneco.2021.105269
Xu, S., and Dong, H. (2023). Green finance, industrial structure upgrading, and high-quality economic development–intermediation model based on the regulatory role of environmental regulation. Int. J. Environ. Res. Public Health 20, 1420. doi:10.3390/ijerph20021420
Xu, Xi, Zhao, M., Li, T., and Li, S. (2023). Data factor and enterprise innovation: the perspective of R&D competition. Econ. Res. J. 58, 39–56.
Xu, Z., and Jiang, X. (2021). Green finance’s supporting carbon neutrality:its current situations, mechanisms and paths. Academic Exchange, 78–87.
Yan, Z., Zou, B., Du, K., and Li, K. (2020). Do renewable energy technology innovations promote China’s green productivity growth? Fresh evidence from partially linear functional-coefficient models. Energy Econ. 90, 104842. doi:10.1016/j.eneco.2020.104842
Yang, Z., Jin, C., and Jizhen, lI. (2022). Global value chain in the era of digital economy: trends, risks and countermeasures. Economist, 64–73. doi:10.16158/j.cnki.51-1312/f.2022.02.008
Yao, L., and Yang, X. (2022). Can digital finance boost SME innovation by easing financing constraints? evidence from Chinese GEM-listed companies. PLOS ONE 17, e0264647. doi:10.1371/journal.pone.0264647
Yin, X., Wang, D., Lu, J., and Liu, L. (2023). Does green credit policy promote corporate green innovation? Evidence from China. Econ. Change Restruct. 56, 3187–3215. doi:10.1007/s10644-023-09521-9
Yu, H., and Zheng, C. (2024). Environmental regulation, land use efficiency and industrial structure upgrading: test analysis based on spatial durbin model and threshold effect. Heliyon 10, e26508. doi:10.1016/j.heliyon.2024.e26508
Zhang, W., Fan, H., and Zhao, Q. (2023). Seeing green: how does digital infrastructure affect carbon emission intensity? Energy Econ. 127, 107085. doi:10.1016/j.eneco.2023.107085
Zhang, X., Yang, T., Wang, C., and Wan, G. (2020). Digital finance and household consumption: theory and evidence from China. J. Manag. World 36, 48–63. doi:10.19744/j.cnki.11-1235/f.2020.0168
Zhang, Y., Ling, D., and Liu, H. (2022). Does the digital economy promote the upgrading of global value vain in China’s manufacturing? Stud. Sci. Sci. 40, 57–68. doi:10.16192/j.cnki.1003-2053.20210326.003
Zhao, B., Shao, Y.-B., Yang, C., and Zhao, C. (2024a). The application of the game theory combination weighting-normal cloud model to the quality evaluation of surrounding rocks. Front. Earth Sci. 12. doi:10.3389/feart.2024.1346536
Zhao, H., Chen, S., and Zhang, W. (2023). Does digital inclusive finance affect urban carbon emission intensity: evidence from 285 cities in China. Cities 142, 104552. doi:10.1016/j.cities.2023.104552
Zhao, T., Zhang, Z., and Liang, S. (2020). Digital economy, entrepreneurship, and high-quality economic development: empirical evidence from urban China. J. Manag. World 36, 65–76. doi:10.19744/j.cnki.11-1235/f.2020.0154
Zhao, X. (2022). Research on the technology innovation effect of new digital infrastructure. Stat. Res. 39, 80–92. doi:10.19343/j.cnki.11-1302/c.2022.04.006
Zhao, X., Benkraiem, R., Abedin, M. Z., and Zhou, S. (2024b). The charm of green finance: can green finance reduce corporate carbon emissions? Energy Econ. 134, 107574. doi:10.1016/j.eneco.2024.107574
Zhou, W., Wu, X., and Zhou, D. (2023). Does green finance reduce environmental pollution? a study based on China’s provincial panel data. Environ. Sci. Pollut. Res. 30, 123862–123881. doi:10.1007/s11356-023-30738-8
Keywords: game theory combination empowerment, green finance, digital technology, coupling coordination, Markov chain model
Citation: Liu K, Du R, Xu B, He J and Wang Z (2024) Measurement, dynamic evolution and pollution emission effects of the coupling of green finance and digital technology-evidence from China. Front. Environ. Sci. 12:1395302. doi: 10.3389/fenvs.2024.1395302
Received: 03 March 2024; Accepted: 05 November 2024;
Published: 19 November 2024.
Edited by:
Mobeen Ur Rehman, Keele University, United KingdomCopyright © 2024 Liu, Du, Xu, He and Wang. This is an open-access article distributed under the terms of the Creative Commons Attribution License (CC BY). The use, distribution or reproduction in other forums is permitted, provided the original author(s) and the copyright owner(s) are credited and that the original publication in this journal is cited, in accordance with accepted academic practice. No use, distribution or reproduction is permitted which does not comply with these terms.
*Correspondence: Ran Du, ZDIwMjE4MTMwNUBodXN0LmVkdS5jbg==