- 1Department of Architecture, Hangzhou City University, Hangzhou, Zhejiang, China
- 2Zhejiang Engineering Research Center of Building’s Digital Carbon Neutral Technology, Hangzhou, Zhejiang, China
Reducing carbon emissions in the building sector is crucial to achieving carbon peak and carbon neutrality goals. Identifying the influencing factors of carbon emissions from building operations at the city level and predicting carbon peaks is of great significance for developing locally adapted policies. This article examines the spatial and temporal trends of carbon emissions at the building operation stage in the Yangtze River Delta (YRD) region over the last 15 years. This article analyzes the driving factors at a city level using the GDIM method and evaluates the decoupling status of income growth and carbon emissions with the GDIM-based decoupling model. The peak time and peak value of operational carbon emissions in the YRD region are predicted through a combination of scenario analysis and the Monte Carlo method. The research shows that: (1) the total carbon emissions from the building operational stage in the YRD region increased by 163.63%, of residents’ disposable income and energy consumption contributed the most. (2) In terms of decoupling relationship between carbon emissions and income growth, rural residential buildings (RR) outperform urban residential buildings (UR) and public buildings (PB). (3) According to the dynamic simulation, the operational carbon emission in the YRD region is expected to peak at 498.18–504.83 Mt MtCO2 between 2030 and 2035. (4) The peak of PBs and URs in Nanjing, Suzhou and some third- and fourth-tier cities, and RRs in eastern and central cities in the YRD will occur at the latest, necessitating close monitoring. These findings can serve as a basis for developing scientific and reasonable building emission reduction policies in a multilevel governance context.
1 Introduction
A new era in the governance of climate change was ushered in with the signing of the Paris Agreement. Due to its high primary energy consumption, which accounted for 26.13% of global CO2 emissions in 2020, China is the world’s greatest energy user and emitter (Chen et al., 2023). The Chinese government has pledged to peak carbon emissions before 2030 and attain carbon neutrality before 2060 in order to combat global climate change and reduce emissions (Shi et al., 2023; You, 2023). China’s building carbon emissions and energy consumption have continued to rise in recent years due to the country’s increasing urbanization and ongoing improvements in people’s living conditions. Carbon emissions in the building sector account for 50.9% of the national total carbon emissions, with a total of 5.08 billion tCO2 emitted throughout the entire process. Specifically, the building operation stage contributes 2.16 billion tCO2, which is 21.7% of the national total carbon emissions (CABEE, 2022). The conflict between the pressing requirement for national energy conservation and emission reduction and the building operating stage’s increasing energy consumption is becoming more and more apparent (Li et al., 2023). In 2021, the State Council of China issued the Action Plan for Carbon Dioxide Peaking before 2030, which explicitly states that"promoting the green and low-carbon transformation of urban and rural construction, and accelerating the upgrading of energy efficiency in buildings.” Therfore, the achievement of China’s “dual carbon” goals is closely linked to the reduction of emissions during the operational phase of buildings.
Cities are the basic units for implementing energy policies (Wang Y. et al., 2020). Significant variations in climate, energy structure, and economic status among different cities create significant variations in the characteristics, influencing factors, and peak periods of carbon emissions from building operations among cities. Therefore, to establish building emission reduction pathways and scientifically formulate relevant policies, the total amount of carbon emissions at the building operation stage need to be accounted for and the factors influencing carbon emissions need to be identified to predict future carbon emissions at the city level.
The Yangtze River Delta (YRD) region is the largest urban agglomeration in China and the sixth largest in the world. The YRD is one of China’s most economically vibrant areas and the building carbon emission in this area is very high, thus takes more responsibility for emission reduction (Liu, et al., 2022). The study of carbon emissions at the building operation stage in the YRD region can provide data support and policy suggestions for low-carbon and green development in the YRD region and has great reference value for other provinces and cities.
2 Literature review
The decomposition and prediction of carbon emissions in the building sector is currently a hot research topic, but this research has primarily been on a global or national scale, with little investigations conducted at the province level and even fewer at the city level. This is because data on carbon emissions from building operations are less complete at the city level. Two approaches commonly used to measure carbon emissions from buildings at the city level are top-down and bottom-up methods. The top-down approach initially calculates the carbon emissions resulting from building operations at the provincial level based on the energy balance table (EBT) (Huo et al., 2018) and then downscales the carbon emissions to the city level by using population or economic indicators (Wang Y. et al., 2020; Wang Y. et al., 2023). However, this approach fails to account for variations in carbon emissions per capita among several cities within the identical province, potentially resulting in inaccuracies. The Professional Committee of Building Energy and Emissions (CABEE, 2022) improve the top-down method and calculated the building carbon emission at city level with energy consumption from the China City Statistical Yearbook, and China Urban Construction Statistical Yearbook, but this method neglect the gas and heating energy consumption in the town areas and rural areas (Geng et al., 2022). The bottom-up approach initially identifies the energy consumption of typical buildings within a city and subsequently estimates the energy demand and carbon emissions on a city level (Zhang et al., 2023). However, this method is applicable to a small number of cities with comprehensive basic data.
Regarding carbon emission decomposition, population, urbanization rate, per capita floor area, economic growth, and energy consumption are the main increasing factors (Chen H. et al., 2023; Chen and Bi, 2022; Zhang J., 2023; Huo et al., 2021; Ma, 2017), while improving energy intensity, emission factors, energy structure, etc., are the main decreasing factors (Yang et al., 2017; Wu P. et al., 2019; Wu S. et al., 2023; Zou et al., 2023). Commonly used decomposition methods include the structural decomposition method (SDA) and index decomposition analysis (IDA). Although the Logarithmic Mean Divisia method (LMDI) is the most commonly used IDA method, the LMDI solely considers absolute factors, and there exists a significant dependency among these factors, resulting in inaccuracies. Therefore, Vaninsky (2014) proposed the Generalized Divisia Index method (GDIM) to improve it, thereby enabling a more comprehensive and precise quantification of the contributions of various factors to carbon emissions. This method will also be employed in the present study.
Decoupling is an important tool to examine the correlation between economic development and environmental consequences (Wang Q. et al., 2018) and has been extensively utilized in various fields (Miao, Liu, and Chen, 2020; Yu and Fang, 2021; Zhang et al., 2022; Dong et al., 2021; Wang et al., 2019). Carbon emissions resulting from the operational phase of buildings are intricately linked to economic progress. However, research on the decoupling impact of building carbon emissions started late and is relatively few, mainly focus on the comparison of decoupling results between countries (Zhong et al., 2021), provinces (Huo et al., 2021) and megacities (Liang et al., 2019; Ma et al., 2022).
Regarding carbon emissions predictions, the majority of research employ scenario analysis, in combination with commonly used models including Long-range Energy Alternatives Planning (LEAP) models (Kim et al., 2020; Zhang and Luo, 2023), market allocation models (Chiodi et al., 2013; Sarıca et al., 2023), Stochastic Impacts by Regression on Population, Affluence, and Technology (STIRPAT) models (Cong et al., 2015; Xu and Wang, 2020; Zhu et al., 2022), system dynamics models (Huo et al., 2022; Huo et al., 2022), BP neural networks (Tang et al., 2024) and shared socioeconomic pathways (SSPs) (Zhang et al., 2021; Wang S. et al., 2022; Zhang et al., 2022; Huang et al., 2023). Among these, the representative concentration pathway (RCP) and SSPs proposed by the Intergovernmental Panel on Climate Change (IPCC) are recognized climate prediction models due to their consideration of uncertainties in the implementation of climate policies and the resulting socioeconomic changes (Gao and O’Neill, 2020; Wu et al., 2023). The relationship between carbon emissions during the operation of buildings and socioeconomic factors is significant. Therefore, the SSP framework is a suitable method for predicting future carbon emissions in the building sector (Gan et al., 2023). Therefore, how to adapt the SSPs framework to the carbon emission characteristics of buildings and apply it at different local levels, such as provinces and cities (Nilsson et al., 2017), has become of interest for guiding specific local policies.
In sum, while existing researches have provide a rich discussion on building carbon emission, there are still some deficiencies. First, most study on the building focus on the national or provincial level, but data at these levels obscure regional heterogeneity at the city level, makes it difficult to put forward specific suggestions. Second, the methodology of the calculation of building operational carbon emissions at the city-level needs to improved, as the town and rural areas are often neglected. Third, there are relatively few studies centered on the decoupling status between the building operating carbon emissions and income growth, thus the decoupling relationship is still unclear. Fourth, in the building carbon emission prediction, there is a tendency to place greater emphasis on technical details, with less attention paid to economic and social considerations, and a lack of sufficient consideration of uncertainty.
To fill these research gaps, this paper selects YRD region as the study area and explores the driving factors and decoupling status of carbon emissions from building operations at the city scale, and predicts the carbon peak time and value. Compared to the existing studies, the contributions of this paper include that:
• In terms of research scale, this study focus on the decomposition, decoupling and prediction of building carbon emission at the city level, addressing the gap in previous studies regarding this scale. The city-level analysis helps to formulate locally adapted energy conservation and emission reduction policies.
• In terms of carbon emission measurement at the city level, this study improve the methodology based on CABEE (2022)’s method by combing the energy consumption data from the China County Seat Construction Statistical Yearbook to cover the town and rural areas. This approach enhances the accuracy of carbon emission accounting.
• In terms of research method, this study use the GDIM methods to identify the drivers of building operation emissions, then reveals the decoupling effect by GDIM-based decoupling methods. This study reveals regional and urban-rural heterogeneity in the drivers and decoupling statues of building carbon emission, which helps to gain a comprehensive understanding of the changes in carbon emissions from different building types in different cities.
• For carbon emissions prediction, the SSPs framework is introduced to better consider the impacts of demographic and economic factors. By comparing the results of the projections of peak carbon emissions between cities and the region, it is possible to identify key cities for emission reduction and identify carbon emission reduction pathways in different cities.
3 Methods and data
3.1 Study area
The YRD region covers Shanghai, Jiangsu (JS), Zhejiang (ZJ), and Anhui (AH) Provinces; comprises 41 cities and spans 358,000 km2 (Figure 1). The YRD region’s GDP in 2021 amounted to 27.60 trillion yuan, representing around 25% of the nation’s overall economic output. The population of the YRD region was 236.47 million in 2021, with an urbanization rate of 71.44%, which is 6.72% higher than the national average. In the face of increasing energy and environmental issues, the 14th Five-Year Plan proposes to “build demonstration zone of green and integrated ecological development of the YRD at a high level.” The region has implemented policies such as the Overall Program for the Demonstration Zone of Green and Integrated Ecological Development of the YRD and has taken the lead in exploring an eco-friendly development model.
Classifying cities helps to identify the characteristics and trends of carbon emissions at the building operations in different types of cities. In this study, the classification of YRD cities is based on the research findings of Institute, (Institute, 2022). The classification is based on five dimensions: concentration of business resources, urban hub status, urban population activity, diversity of lifestyle, and future malleability. This classification is commonly used in city-level studies (Zhang et al., 2023; Dai and Yang, 2024; Wu et al., 2024). According to this criterion, the cities in YRD region are classified into five categories: first, new first, second, third, and fourth-tier cities, as shown in Table 1.
3.2 Methods for the calculation of building operating carbon emissions at the city level
Building operation carbon emissions refer to the carbon emissions during the operation phase of a building; these emissions in China mainly cover three building types: UR, RR, and PB (Huo et al., 2022). The carbon emission coefficient approach, recommended by the IPCC, is widely adopted for calculating carbon emissions due to its straightforward data collecting and uncomplicated calculation methodology. The calculating formula is expressed in Eq. 1.
where C is the carbon emissions at the building operation stage; j is the different building types; j = 1,2,3 represents PB, UR and RR, respectively; k is the different energy sources; E is the building energy consumption; and f is the carbon emission factor.
The calculation of carbon emissions from building operations at the city level is optimized using the methodology published by the CABEE (2022).
3.2.1 Indirect carbon emissions
• Electricity accounts for almost 70% of the total energy consumption. The electricity consumption of URs and RRs is shown as electricity consumption for urban and rural residents, respectively, in the city statistical yearbooks. The electricity consumption of PB originates from tertiary industry, which mainly includes wholesale and retail trade, accommodation and catering, leasing and business services, public services and management organizations. In addition, a portion of the energy consumption of buildings in the transportation, storage and postal sectors should be added.
• Centralized heating. There are a few centralized heating cities in the YRD region, including Hefei, Huainan, Chizhou and Chuzhou in Anhui Province. The heating energy consumption data were obtained from the China Urban Construction Statistical Yearbook.
3.2.2 Direct carbon emission
• Natural gas and LPG directly create carbon emissions. CABEE’s method for calculating carbon emissions indicates that the amount of natural gas and LPG consumption of households can be obtained from the China Urban Construction Statistical Yearbook. Importantly, this statistical scope includes only the urban area and excludes towns and villages, which may result in inaccuracies, particularly in cities with low levels of urbanization. This study takes the household consumption of natural gas and LPG in counties into account, as provided by the China County Seat Construction Statistical Yearbook. The calculation formula is shown in Eqs 2–4.
where i is the ith city in YRD region, j is the jth province in YRD region, k is the fossil fuel, including natural gas and LPG;
• Raw coal, briquettes, gasoline, and diesel oil have relatively low shares of energy. Based on the corresponding energy data from the provincial energy balance sheets, the allocation is based on the city’s energy consumption weights, as shown in Eq. 5.
where p is the city’s energy consumption weight and
3.3 GDIM method
The GDIM method is a multidimensional factor decomposition model based on the KAYA identity. The method takes into account several quantitative and relative indicators, so addressing the limitations associated with the interdependence of driving elements in conventional decomposition approaches (Yu and Fang, 2021; Gan et al., 2023). According to the GDIM proposed by Vaninsky (2014), the expression of building operation carbon emissions and related factors is shown in Eqs 6–9.
where i is the ith city in YRD region, j is different building types, j = 1,2,3 represents PB, UR and RR;
Thus, Eqs 6–9 can be transformed into Eqs 10–16 as
The contribution of factor to the change in carbon emissions is the function C (
According to the GDIM principle, the change in carbon emissions ∆C is decomposed into the sum of the contributions of the individual influencing factors as Eq. 18:
where L is the time span;
Based on the above factor decomposition, the changes in building operation carbon emissions are decomposed into the contributions of eleven driving factors. Among the four absolute factors,
3.4 The GDIM-based decoupling method
Based on the decomposition results from the GDIM, changes in building operation carbon emissions resulting from
where
where
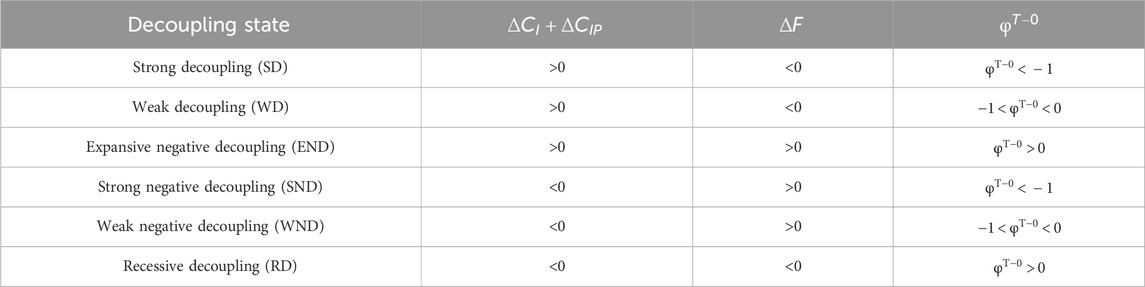
Table 3. Definition of decoupling state (Yu and Fang, 2021; Ma et al., 2022).
3.5 Carbon emission prediction simulation method
3.5.1 Building operation carbon emission prediction model
The static building operational carbon emissions model is based mainly on the KAYA identity and the results of the previous GDIM with reference to the studies of Yang et al. (2017). The building operation carbon emissions in the YRD region are the sum of the building operation emissions from 41 cities and each building type.
where C is the total building operation carbon emission;
where
3.5.2 Scenario and parameter settings
Scenario analysis is a systematic method for forecasting and assessing the future state of an object with high uncertainty. The IPCC created SSP and RCP climate change forecasts from various standpoints; their precise definitions are provided in Table 4. In order to objectively model the trajectory of carbon emissions during the operational phase of buildings in the YRD region, three carbon emission scenarios were created. These scenarios were based on the societal development trend, emission reduction policies, and specific actual situation by combining the RCP-SSP scenarios:
• Business as usual (BUA): Based on RCP6-SSP2. In order to reach the 2030 peak carbon goal, there will be ongoing policies focused on energy-efficient buildings in the near and medium future. Additionally, the majority of the occupants’ requirements for comfort in the built environment will be met. Carbon emissions in the building sector are projected to reach their maximum level and then decline.
• High carbon (HC): Based on the RCP8.5-SSP5 scenario. The government maintains high economic growth rates by relying on fossil fuels and not implementing low-carbon energy efficiency policies, resulting in an increase in carbon emissions.
• Low carbon (LC): Based on the RCP2.6-SSP1 scenario. The government is vigorously implementing measures pertaining to energy saving and emission reduction. The widespread adoption of low-carbon technology will lead to a gradual reduction in the annual growth of energy consumption and carbon emissions in the building sector.
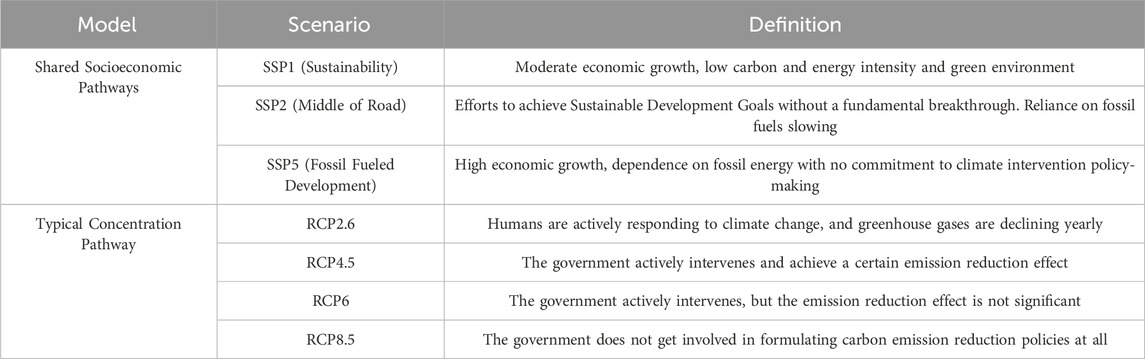
Table 4. Definition of the RCP-SSP scenario (Wang et al., 2022; Gan et al., 2023).
Regarding parameter setting, the population, GDP growth rate, and urbanization rate are obtained from the SSP database. In the BAU scenario, each city’s energy intensity per floor space and carbon emission factor will first continue to follow historical trends. Subsequently, with the implementation of relevant low-carbon policies, the growth rate of energy intensity per floor space and the carbon emission factor will slow and eventually decline. Table 5 presents the rules for determining the growth rate of energy intensity per floor space and the carbon emission factor in the BAU scenario. According to the BAU scenario, the energy intensity per floor space and the carbon emission factor of the HC and LC scenarios are projected to increase by 0.5% and decrease by 0.5%, respectively.
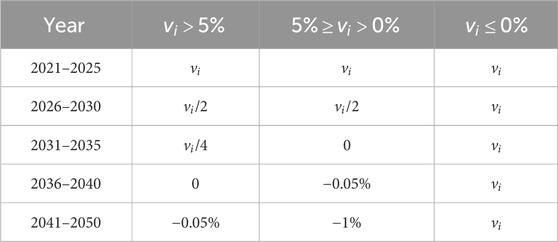
Table 5. The rate of increase in energy intensity per floor space and carbon emission factor in the BAU scenario.
3.5.3 Monte Carlo method
The Monte Carlo approach is employed to anticipate the dynamic trend of carbon emissions during the building operation stage, in order to address the constraints of static carbon emission scenario analysis. This method can randomly sample values and combine multiple variables according to a given probability distribution. It has the advantage of modeling multiple scenarios and conducting uncertainty analysis (Zhang Q. and Li, 2023). Therefore, the Monte Carlo method was used in this study to simulate multiple scenario prediction results. The following three steps were included. First, the distributional assumptions of the variables were established. As previously stated, the BAU scenario has the highest likelihood of happening, whereas the HC and LC scenarios reflect the most negative and positive possibilities, respectively. Due to the level of certainty associated with the highest expected value and potential range of each explanatory variable, we have designated all variables as triangular distributions. The Oracle Crystal Ball program was employed to conduct 100,000 Monte Carlo simulations based on the anticipated probability distribution, in order to guarantee the precision of the sampling outcomes. Finally, using Eqs 21–24, we calculated the building operation carbon emissions in the YRD region from 2022 to 2050 and analyzed the carbon peak year and peak value.
3.6 Data sources
The detailed data sources are as follows. Energy consumption at the provincial level was obtained from the EBTs of the China Energy Statistical Yearbook. The electricity consumption at the city level was obtained from the Statistical Yearbooks of the cities in the YRD regions. The heating, natural gas and LPG consumption data at the city level were obtained from the China Urban Construction Statistical Yearbook and the China County Seat Construction Statistical Yearbook. The carbon emission factors are shown in Supplementary Table S1.
Historical data related to population, urbanization rate, added value of tertiary industry, per capita disposable income, residential floor area per capita and other relevant factors were obtained from the Statistical Yearbooks of the cities and provinces in the YRD region. The floor space per employee in the tertiary sector of industry was assumed to follow a logistic function of per capita income (McNeil et al., 2012). This approach is widely used in the estimation of building floor space (Hong et al., 2016).
The predicted population and GDP were from the SSP database. This study applied the latest findings of Zhang et al. (2023)’s city-level SSPs population projections. These projections consider various factors, such as a city’s political status, development expectations, migration policies, and recent fertility rates. The GDP projection came from the study of Jiang et al. (2022). The database offers GDP raster data for China on a yearly basis, with a spatial resolution of 0.1°. The data were extracted by city via ArcGIS and served as a reference for GDP growth in different cities.
4 Results
4.1 Spatial-temporal changes in building operating carbon emissions in the YRD region
4.1.1 Temporal change in building operating carbon emissions
Carbon emissions at the building operation stage in the YRD region have continued to increase, from 142.86 Mt CO2 in 2006 to 376.57 Mt CO2 in 2021, as shown in Figure 2A. Total building operation carbon emissions decreased slightly in 2020 due to the COVID-19 pandemic but increased again in 2021. In terms of building types, PBs account for the highest proportion (approximately 50%), and the carbon emissions of URs slightly exceed those of RRs.
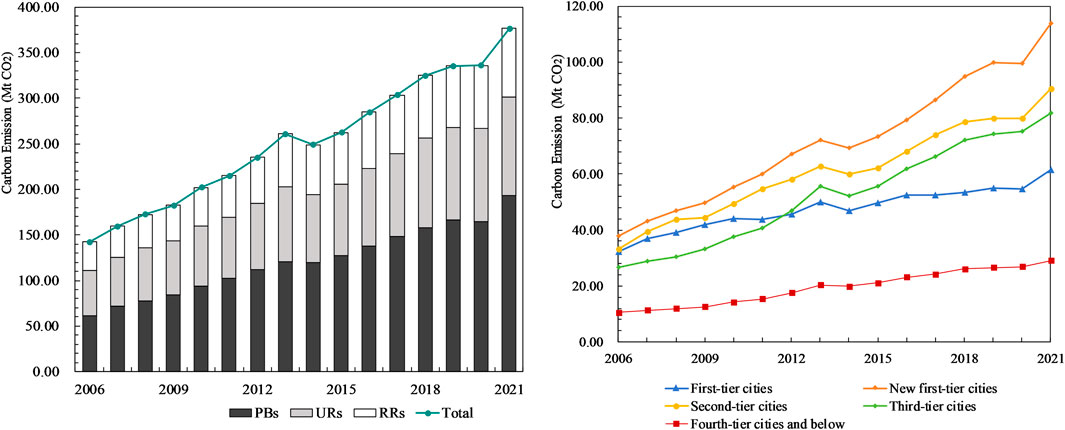
Figure 2. Building operation carbon emissions of different (A) building types and (B) city groups in YRD region.
Figure 2B shows the changes in carbon emissions at building operation stage in different types of cities in the YRD region from 2006 to 2021. In the YRD region, the first-tier city is has the highest building operational carbon emissions and the lowest emission growth rate, thus its proportion of carbon emissions in the YRD region is gradually decreasing, from 22.96% in 2006 to 16.34% in 2021. There are only five new first-tier cities but they account for the highest share of building operation carbon emissions, which reached 30.21% in 2021. These cities’ carbon emissions have grown rapidly, from 37.89 Mt CO2in 2006 to 113.78 Mt CO2 in 2021, representing a 200.27% increase. The carbon emission shares of the second-tier cities in the YRD region have remained relatively stable, fluctuating between 23.66% and 25.40% from 2006 to 2021. Among all city types, third-tier cities have experienced the highest growth rate in building operation carbon emissions, increasing from 26.71 Mt CO2 in 2006 to 81.66 Mt CO2 in 2021, an increase of 205.70%. The proportion of building operation carbon emissions in fourth-tier and below cities has remained stable, fluctuating between 6.89% and 8.13%.
4.1.2 Changes in the spatial distribution of carbon emissions
Figure 3 illustrates the spatial distribution of carbon emissions from building operations in various cities in the YRD region from 2006 to 2021. The building operation carbon emissions are greater in the eastern coastal region than in the western and central regions. Each year, first-tier cities have the highest building operation carbon emissions, while new first-tier, second-tier, third-tier, and fourth-tier cities show a gradual decrease. For instance, in 2021, Shanghai had the highest contribution to carbon emissions, with 61.53 Mt CO2, followed by Hangzhou, a second-tier city, with 27.34 Mt CO2. The lowest contribution was from Chizhou, a fifth-tier city, with 1.47 Mt CO2.
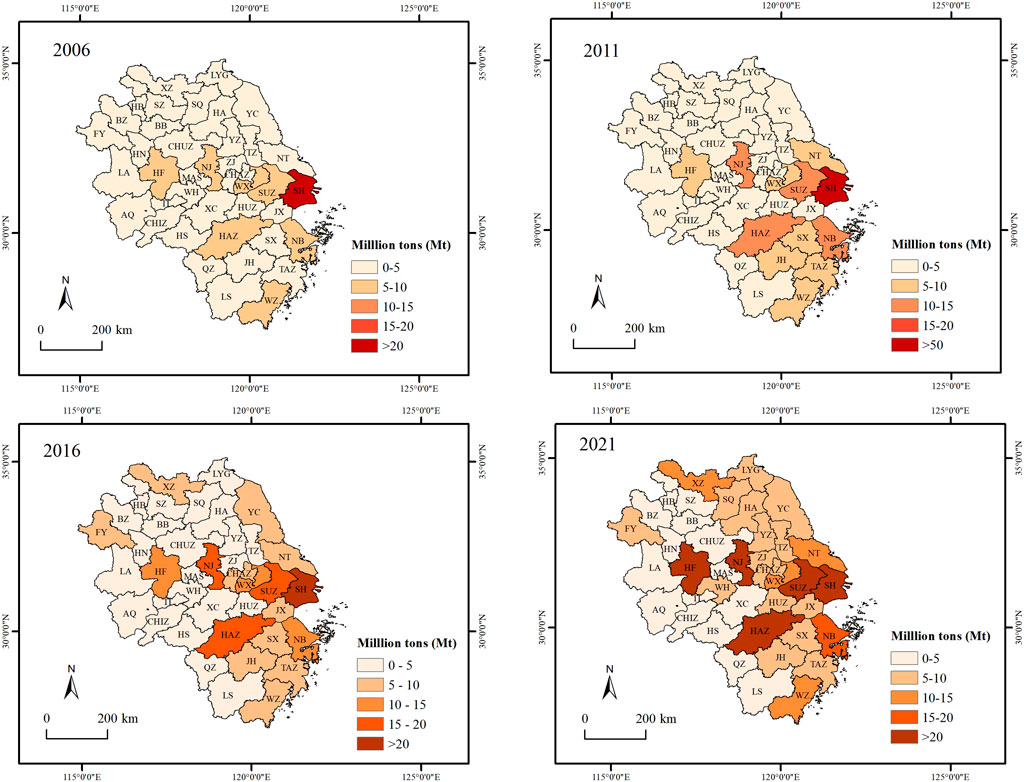
Figure 3. The spatial-temporal characteristics and evolution of building operating carbon emissions in the YRD region.
In terms of building types, the carbon emissions in PBs and URs exhibit spatial distributions similar to those of all building types. As shown in Figures 4A, B, PBs and URs exhibit a spatial pattern of high carbon emissions in the east and low in the west, as well as a gradual decrease in carbon emissions in first-tier, new first-tier, second-, third- and fourth-tier cities. The spatial pattern of building operation carbon emissions in the RR is relatively different: high in the east and northwest, low in the central and southwest.
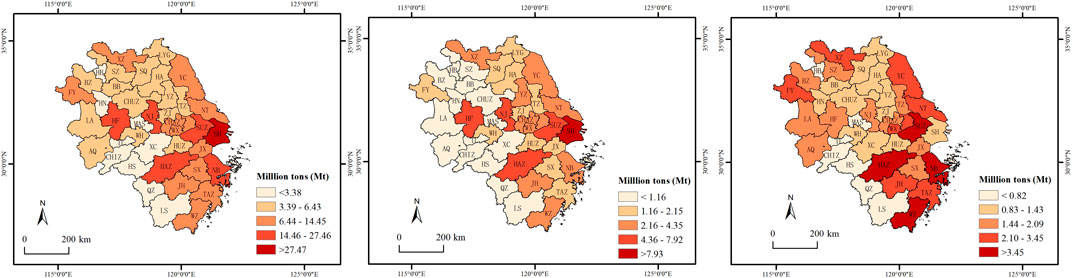
Figure 4. Building operating carbon emission values of (A) PBs, (B) URs and (C) RRs in the YRD region in 2021.
4.2 Decomposition results of building operating carbon emissions
4.2.1 Decomposition results at the regional level
Based on the GDIM decomposition method, the total carbon emissions from PBs, URs and RRs in the YRD region were attributed to changes in 11 factors. As shown in Figure 5, the result reveal the following key findings:
(1) From 2006 to 2021, building operating carbon emission in the YRD region has increased by 233.78 Mt CO2, which was mainly attributed to the PBs (131.45 Mt, 56.23%) and the URs (57.89 Mt, 24.76%). RRs accounted to a smaller proportion (44.43 Mt, 19.01%).
(2) The main driving factors for PBs and URs are similar. Residents’ disposable income (
(3) The driving factors of carbon emissions in RRs exhibit different characteristics. Carbon intensity per capita (
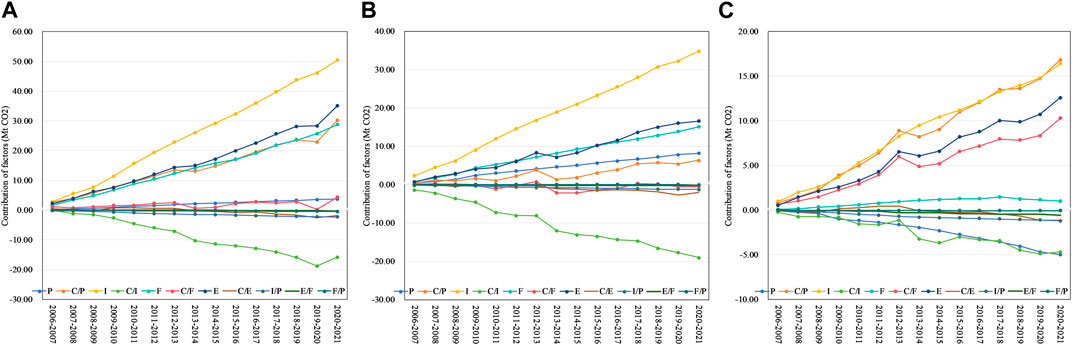
Figure 5. Contribution of driving factors of (A) PB, (B) UR and (C) RR carbon emissions changes in YRD region from 2006 to 2021.
4.2.2 Decomposition results at the city level
The results of carbon emission decomposition for the cities in the YRD region by building type are shown in Figure 6. For most cities, residents’ disposable income (I), energy consumption (E) and floor space (I) are the major positive drivers for the carbon emission of PBs, URs and RRs while carbon intensity of income growth (C/I), per capita disposable income (I/P) and carbon emission factor (C/E) are the major negative drives. This is consistent with the decomposition analysis at the regional level. The impact of carbon intensity of floor space (C/F) and carbon emission per capita (C/P) and population (P) varies widely between cities. The heterogeneity of drivers at the city level will be discussed further in Section 5.1.
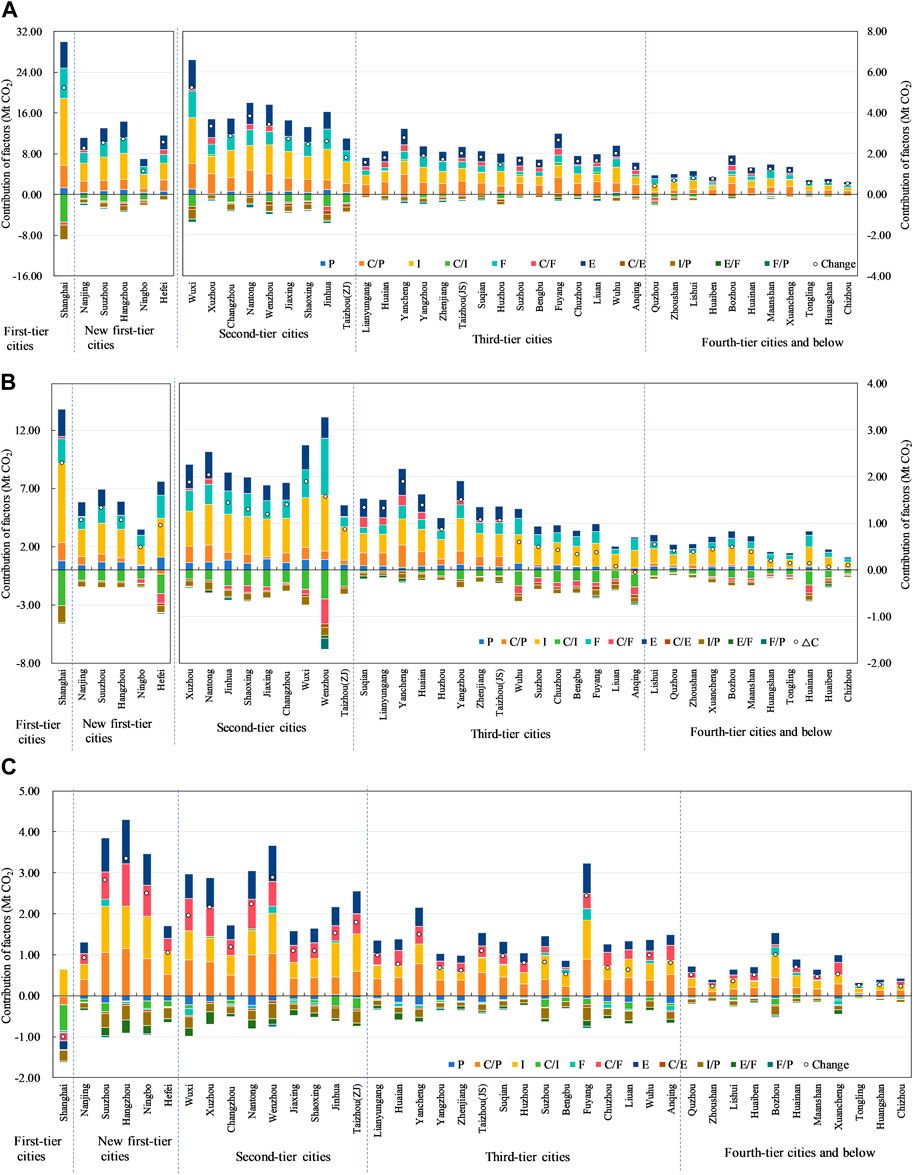
Figure 6. Contribution of driving factors of (A) PB, (B) UR and (C) RR carbon emissions changes at the city level in YRD region from 2006 to 2021.
4.3 Decoupling results
Based on the GDIM-Tapio decoupling model in Section 3.4, decoupling indicator of building operation carbon emissions from income growth in the YRD region was calculated (Table 6). As the disposable income of residents in the YRD region has been growing since 2006 to 2021, there are only three decoupling states, namely, expansive negative decoupling (END), weak decoupling (WD) and strong decoupling (SD). For PBs and RRs, SD occurred twice, and END status occurred for the remaining years. For URs, SD occurred once, WD occurred twice, and END occurred during the remaining years. This finding indicates that the carbon emissions at the building operation stage and disposable income are both increasing rapidly and have not yet been decoupled.
At the city level, the decoupling indicator for each building type was calculated according to the Five-year Plan (FYP) periods. To ensure the continuity of the analysis and avoid inaccuracies caused by COVID-19, 2021 was included in the 13th FYP period. The results are shown in Supplementary Table S2–S4; Figure 7. For PBs, most cities in the YRD region experienced END during the three periods, indicating that the growth rate of carbon emissions from PBs is comparable to the growth rate of income growth. Regarding URs, the majority of cities exhibited END status, with only one city exhibiting SD status and 6, 4, and 7 cities exhibiting WD status during the 11th, 12th, and 13th FYP periods, respectively. For the RRs, the numbers of cities with WD and SD statuses in the 13th FYP period were 4 and 9, respectively, which is significantly greater than that in the 11th and 12th FYP periods. The cities with SD or WD statuses were clustered in the northwestern part of the YRD region, indicating that the Anhui Province’s RRs made significant progress in terms of energy savings and carbon reduction in the 13th FYP period.
4.4 Prediction of carbon emissions
4.4.1 Prediction of carbon emission at the regional level
Based on the scenarios outlined in Section 3.5, projections are made for carbon emissions at the building operation stage in the YRD region from 2022 to 2050. The forecast results are shown in Figure 8A. In the BAU scenario, carbon emissions at the building operation stage in the YRD region will increase gradually from 376.60 Mt CO2 in 2021 and reach a plateau between 2030 and 2035, maintaining a level of 499.51–500.29 Mt CO2, before gradually decreasing to 370.88 Mt CO2 in 2050. In the LC scenario, carbon emissions are projected to peak at 449.69 Mt CO2 in 2030, which is 50.62 Mt CO2 lower than that in the BAU scenario. In the HC scenario, carbon emissions are expected to peak in 2035, with a peak of 569.34 Mt CO2. This suggests that maintaining the current carbon reduction policies alone may not be enough to achieve the 2030 carbon peaking target for the YRD region.
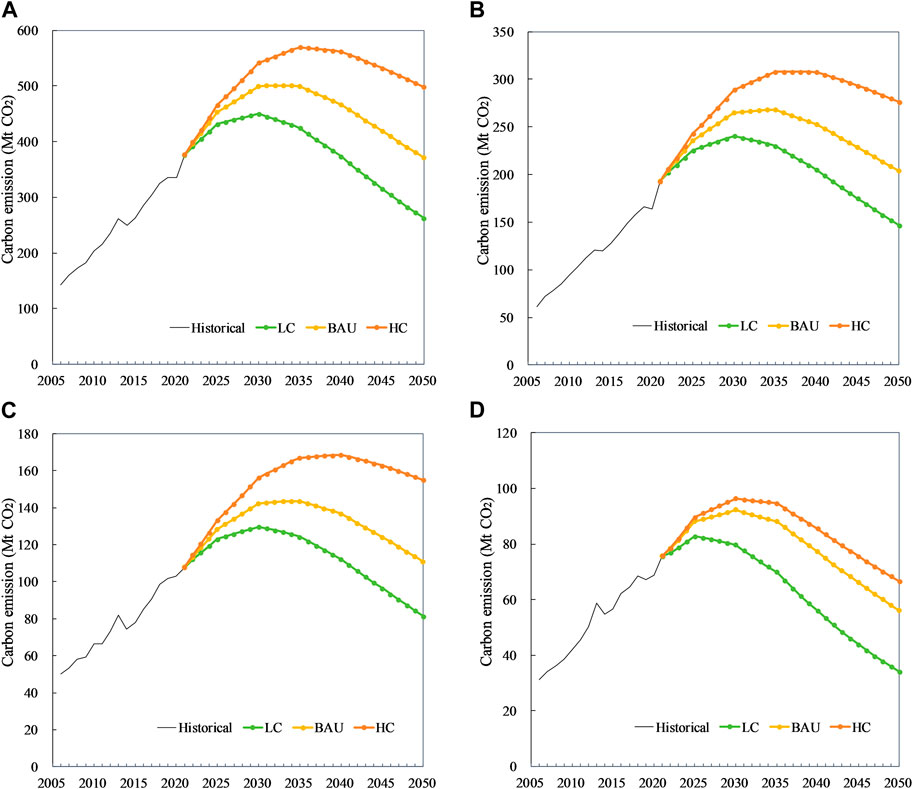
Figure 8. Prediction of building operating carbon emissions of (A) all types, (B) PB, (C) UR and (D) RR in the YRD region under static simulation.
Figure 8B–D shows the projected carbon emissions at the building operation stage for PBs, URs, and RRs, respectively. In the BAU scenario, carbon emission peaks for different building types will occur first for RR in 2030 and then for the URs and PBs in 2034. The corresponding peak emissions will be 92.22 Mt CO2, 143.59, Mt CO2, and 267.76 Mt CO2. In the LC scenario, PBs and URs are projected to reach their peak in 2030 at 240.22 Mt CO2 and 129.72 Mt CO2, respectively; additionally, RRs are expected to peak in 2025 at 82.75 Mt CO2. In the HC scenario, PBs and URs are projected to reach their peak at 308.08 Mt CO2 in 2037 and 168.48 Mt CO2 in 2040, respectively. Moreover, the RRs are expected to peak at 96.39 Mt CO2 in 2030.
In order to comprehensively consider the uncertainties associated with different elements, the Monte Carlo simulation technique was employed to dynamically simulate building carbon emissions 100,000 times to determine the potential emission peak value and peak times. As shown in Figure 9, the carbon emissions at the building operation stage in the YRD region are most likely to reach a carbon peak during the period 2030–2035. The estimated peak value of total carbon emissions falls within the range of 498.18–504.83 Mt CO2, with an average of 501.17 Mt CO2. The uncertainty of the carbon emissions is high, possibly because of the expectation that they will plateau between 2030 and 2035, and the decreasing trend is not evident.
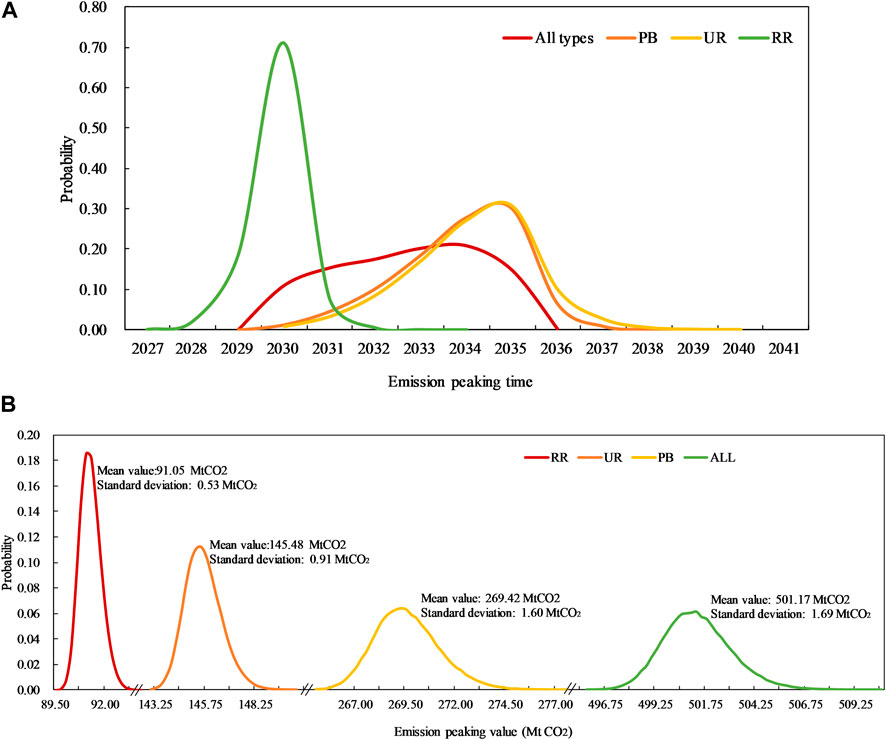
Figure 9. Possible emission (A) peak time and (B) peaking value of building operating carbon emissions in The YRD region under dynamic simulation.
For each building type, PBs are projected to reach the carbon peak between 2031 and 2036, with the most likely years being 2035 and 2034, with probabilities of 29.96% and 27.93%, respectively. The most likely range of peak emissions is 266.62–272.88 Mt CO2. URs are projected to peak in carbon emissions between 2031 and 2036, with the most likely years being 2035 and 2034, with probabilities of 30.49% and 27.17%, respectively. The range of possible peak emissions is 143.89–147.46 Mt CO2. RRs are most likely to achieve peak carbon emissions in 2030, with a probability of 71.15%, followed by 2031, with a probability of 18.56%. The most likely range of peak carbon emissions from RRs is 90.16–92.22 Mt CO2.
4.4.2 Prediction of carbon emission at the city level
As shown in Figure 10A, for PBs, the number of cities that can achieve peak carbon emissions by 2030 and before is 21, 31 and 3 in the BAU, LC and HC scenarios, respectively. As shown in Figure 10B for URs, 24, 35, and 2 cities can achieve peak carbon by 2030 in the BAU, LC, and HC scenarios, respectively. Figure 11 further reveals the relationships between the disposable income, the total value of carbon emissions and the emissions peak time. Cities with the lowest per capita disposable income generally experience a later peak in carbon emissions, such as Lu’an, and Bozhou. Cities with high disposable income and low total carbon emissions are possible to peak early, such as Xuzhou and Nantong. For first-and new-tier cities, which have the highest per capita disposable income and hugest amount of carbon emission, are most likely to peak by 2035, indicating the high pressure for carbon reduction in these cities.
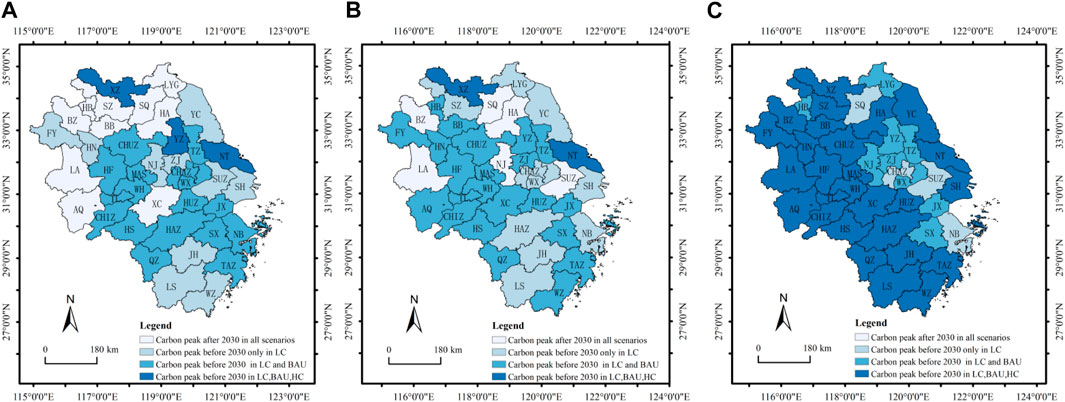
Figure 10. The spatial distribution of carbon peak target achievement of (A) PB, (B) UR and (C) RR in 41 cities in YRD region.
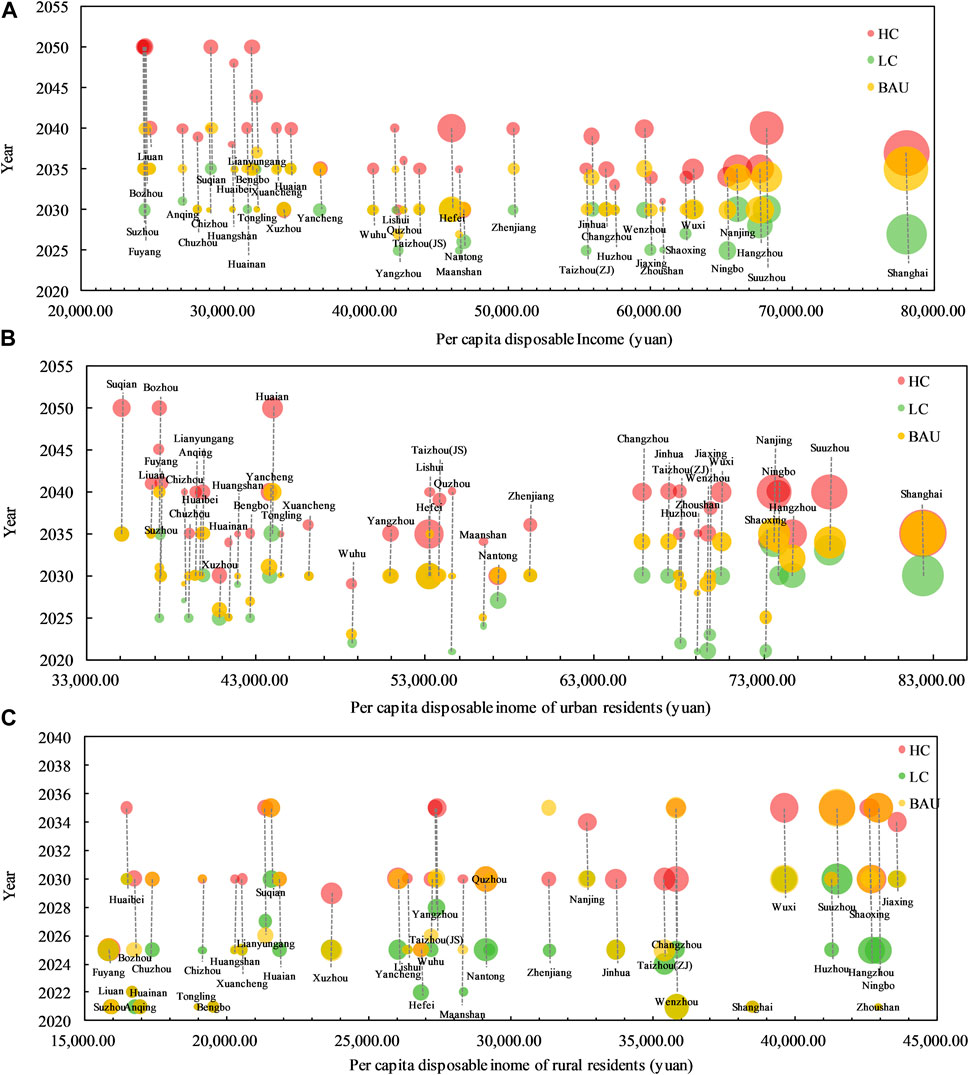
Figure 11. Building operation carbon peak prediction of (A)PB, (B) UR and (C) RR at the city level. Note: The vertical axis represents the year of the carbon emission peak, while the horizontal axis represents the per capita disposable income of each city. The size of the bubble denotes the value of the carbon emission peak.
For RRs, in the LC scenario, all cities in the YRD region can achieve peak carbon emissions by 2030 or earlier. In the BAU and HC scenarios, 36 and 29 cities, respectively, can achieve peak carbon emissions by 2030 or earlier. In terms of spatial distribution, the cities that can achieve peak carbon emissions under all three scenarios are concentrated in the western part of the YRD region (Figure 10C).
5 Discussion
5.1 Drivers of the building carbon emission at city level
As the economic, demographic and technological profiles of different cities vary greatly, the drivers of carbon emissions at building operation stage also vary considerably. This section will further analyse the key drivers at the city level based on the decomposition results in Section 4.2. Drivers with a small contribution, less than 10% in most cities, such as energy intensity (E/F) and per capita floor space (F/P) are not discussed in this section.
5.1.1 Analysis of residents’ disposable income factors
Residents’ disposable income (I) is the major increasing drivers for all the cites. This is in line with the existing studies such as Boqiang Lin and Liu (2015) and Li et al. (2023), which get similar results at national and provincial level. With increasing incomes, residents demand better working and living conditions, thus use more energy-intensive household appliances, such as air purifiers and intelligent appliances, and more heating appliances for thermal comfort in the winter, which leads to an increase in carbon emissions (Wang et al., 2021; Hao et al., 2023). Among city groups, residents’ disposable income plays the least important role in first-tier city’s URs and PBs, which has the highest urban residents income. This can be explained by the previous findings. Shaobin Zhang et al. (2023) reveals that the increasing living standards reduces the extent to which disposable income drives household carbon emissions in urban. Ke and Cai (2023) further reveals that the driving effect of increased income on household carbon emission lessens when income is higher, as more energy-saving and efficient electrical appliances will be used to pursue a higher quality of life.
Carbon intensity of income growth (C/I) is the major increasing drivers for most cities. According to the definition, C/I represents the carbon emission caused by per unit income under different income levels of residents. When C/I is negatively correlated with carbon emission, it means that with the increase in income, there are fewer and fewer parts used for increasing carbon emission. Therefore, C/I can be used to reflect the residential willingness to save carbon emission (Hao et al., 2023). Among city groups, C/I has greater impact on urban areas than on rural areas, and on first-, new first-, second tier cities than on third- and fourth-tier cities, indicating the better carbon reduction awareness in the urban area and developed areas. Li et al. (2019) revealed that the subjective factors have significant impacts on household carbon emissions and found substantial differences across rural/urban areas and regional in China
5.1.2 Analysis of population factors
Population is a positive driver to the carbon emission for all cities’ URs, most cities’ PBs while a negative driver for most cities’ RRs. In terms of city group, population (P) has stronger impact on the new first- and second-tier cities on emission of URs and PBs. This is close relate to the population migration, not only between urban and rural areas, but also in developed and undeveloped areas (Lin and Liu, 2015; Wang et al., 2022; You, 2023). According to the seventh national population census, cities such as Hangzhou, Ningbo, Suzhou, Hefei and Nanjing have the highest population growth rates from 2010 to 2020, indicating that the population in the YRD region is continuing to concentrate in the new first- and second-tier economically developed cities, which has also led to an increase in carbon emissions in these cities.
Carbon emission per capita (C/P) is a major positive drivers for the PBs in all cities and for RRs in all cities except Shanghai. For URs, C/P’s contribution is small and becomes an emission decreasing factors in one-fourth of the cities in YRD region, which of most are third-and fourth cities, such as Wuhu and Chuzhou. This is consistent with previous research. Wu et al. (2023) reveals that the per capita urban residential carbon emissions followed a variable trajectory of decrease-increase-decrease while per capita rural residential carbon emissions maintained rapid growth, and attributed to transformation of energy structure and the ability to use energy. Fan et al. (2015) explained it from the perspective of end-use activities. As a result, attention should be paid on the carbon emission intensity control in the rural areas (Yao et al., 2012; Zhao et al., 2021).
5.1.3 Analysis of floor space factors
Floor space has a small contribution on the carbon emissions of RRs but is an important carbon emissions increasing factors for the URs and PBs. This is consistent with the findings of Rundong Xu et al. (2024) and (Zhao et al., 2023). In terms of city differences, the impact of floor space is higher in the URs in new first- and second-tier cities than in other cities. This is because the rapid development of urbanisation leads to a large demand for urban housing, and the financial characteristics of housing further increase people’s willingness to invest in housing (Li et al., 2023), which is especially evident in large cities, such as first, new first and second tier cities. For first-tier cities, due to the strict property purchase restriction policy, the size of housing is better controlled (Song et al., 2023), resulting the less important effect of floor space size.
For RRs in most cities, carbon intensity of floor space (C/F) is an emission increasing factor. This is consistent with the findings of Huo et al. (2024), which found that the C/F of RRs is increasing in most of the regions in China and can be attributed to the improvement of the living standard of rural residents. For PBs in one-third of the cities and UR in most cities, C/F is carbon reduction factor, indicating these cities have better building energy efficiency and emission reduction. This also occurred in other economically developed regions, for example, Geng et al. (2022) reveals that the growth rate of C/F has slowed down in Greater Bay Area cities in China, which is mainly due to the promotion of the application of building energy efficiency technologies.
5.1.4 Analysis of energy consumption factors
Energy consumption is a positive driver for building carbon emission in all the cities while carbon emission factor (C/E) is a negative driver for most cities. The contribution of carbon emission factors is larger on URs and PBs than that on RRs, which is mainly due to the high levels of electrification of URs and PBs (Gan et al., 2022), and the electricity carbon emission factors have been falling in recent years. For RRs, the contribution of carbon emission factor is mainly related to the change in building energy structure, especially for cities in the north of YRD region with heating demand. As a economically developed region, the YRD region is one of the first regions to implement coal-to-gas and gas-to-electricity policies. In addition, the increased incomes provide additional clean energy consumpn options and reduce the use of coal and PLG, thus adding the proportion of clean energy in households (Zhang et al., 2023; Huang et al., 2024).
Although the effect of carbon emission factor is limited by now, which in line with the findings of Lin et al. (2024) and Zhang et al. (2024), its potential is large. The YRD are promoting the optimisation of the energy structure of the power grids. According to the Chinese Academy of Environmental Planning (CAEP), the carbon emission factors of the provincial power grids in Shanghai, Zhejiang, Jiangsu, and Anhui Province will drop by 48.8%, 42.3%, 40.9%, and 21.9%, respectively, by 2035.
5.2 Comparison of the decoupling results with existing studies
The decoupling results of this paper have both similarities and differences with previous studies. Due to the differences in the spatial scale, carbon accounting scope, and building types of the existing studies, there are differences in the decoupling results. Huo et al. (2021) analysed the decoupling status of carbon emissions from residential buildings and per capita income in 30 provinces and found that the decoupling status was more desirable in the central regions, and the province in the YRG region have shifted from an expanding decoupling to a weak decoupling statue. This paper finds that decoupling statues at the city level is less optimistic than at the provincial level, possibly due to the fact that first-tier cities have a high share of the province’s carbon emissions and tend to have a more favourable decoupling statues (Liang et al., 2019), which leads to an overestimation of decoupling situations in other less economically developed cities.
Nevertheless, the main conclusions were consistent. Most studies concluded the regional and urban-rural decoupling difference at the provincial level (Liang et al., 2019; Zhao et al., 2021; Huo et al., 2024). This study reveals similar differences at the city level. In YRD region, this study found the decoupling statues of RRs are better than that of URs and the causes of the decoupling statues are different (Figure 12). For URs, the decoupling statues is mainly driven by technical factors, such as the decline in C/P,C/F and E/F. This is in line with the previous findings. Based on the CKC theory, Ma, et al. (2019) confirms the inverted-U-shaped relationship between residential building carbon emission and income growth, and finds that the inflection point of C/F and C/P occur earlier than that of the total carbon emission. Liang et al. (2019) get similar conclusion in megacites. For RRs in cities such as Hefei, Huaian and Chuzhou, the decoupling statues is mainly driven by the non-technical effect, such as the decrease in population and building floor space, indicating the passive carbon decline in these cities. For RRs in cities such as Shanghai, Suzhu and Huainan, the decoupling statues is mainly driven by the technical effect, indicating these cities reach their carbon peak actively. This is consistent of with Huo et al. (2024)'s finding at the provincial level, which reveals that RRs in Shanghai are in a strong decoupling state while Beijing, Shanxi, Heilongjiang and Qinghai belong to the stage of passive decline caused by serious population loss.
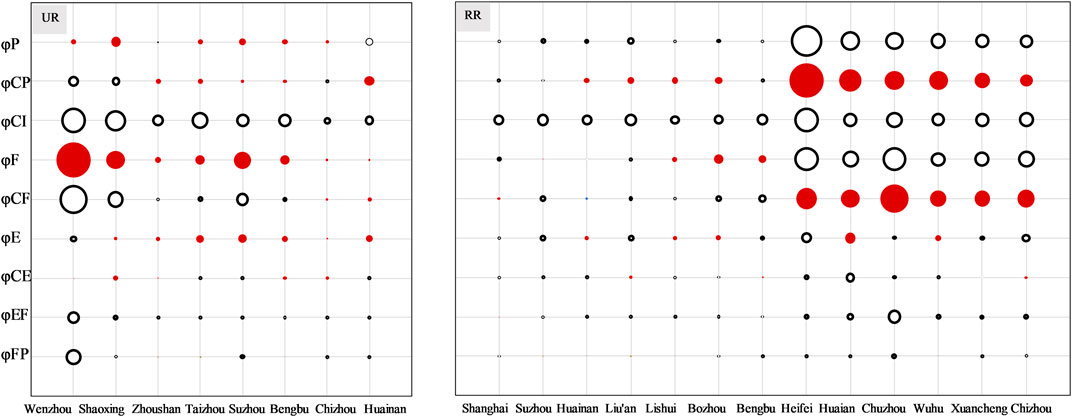
Figure 12. Contribution of different factors for the change in decoupling indicators in different cities. The black circles indicate negative values of the decoupling factor and the red circles indicate positive ones.
5.3 Key cities for reducing emissions identification based on the prediction results
In contrast to previous studies that projected carbon emissions at the national and provincial levels (Li et al., 2023), this study makes building carbon emissions prediction at the city level and found that the carbon peak value and time varied considerably from city to city. This is supported by Lei et al. (2023)’s study of the Central China in the building sector and Tian et al. (2022) ’s peak prediction of urban differentiated carbon emissions in Shandong Province. By comparing the results of the projections of peak carbon emissions in cities and the YRD region, it is possible to identify those cities with late peak times that require particular attention. URs and PBs, with a high proportion of the total carbon emissions and late peaking, are the focus of emission reductions. Based on the city-level carbon emission prediction of PBs and URs, the cities can be divided into three categories:
(1) Cities which can achieve carbon peak before 2030 in all scenario, including PBs in Xuzhou, Nantong, and Yangzhou, and URs in Xuzhou and Nantong. These cities share the common characteristics of developed economy, high disposable income and low total carbon emissions. These cities are easy to achieve the 2030 target if they can persist the current pace to control the increase of building carbon emission.
(2) Cities which are feasible to achieve carbon peak before 2030. For cities in this category, peaking before 2030 can be achieved in one or two scenarios, and the number of these cites is the largest: 28 cities for PBs and 33 cities for URs. This indicates that for the majority of cities in the YRD region, more rigorous energy saving and emission reduction measures are required.
(3) Cities which are hard to achieve carbon peak before 2030. For PBs and URs, 10 and 6 cities are identified that may not achieve carbon peak by 2030 in all scenarios (Figure 10), indicating these cities require particular attention. Further analysis of these cities reveals that they are mainly third- and fourth-tier cities except for Suzhou and Najing of URs. In third- and fourth-tier cities with less developed economies, formal environmental regulation is not implemented as effectively as in the developed cities, which reduces the carbon reduction effect of environmental regulations (Wang and Guo, 2024). In addition, the cities in this category are concentrated in the northern part of the YRD region, where average winter temperatures are lower, air humidity is higher, and the demand for winter heating increases as incomes rise (Chen et al., 2023), making it difficult to achieve carbon peak.
For RRs, although most cities are able to achieve carbon peak by 2030, combined with decoupled analyses, it can be seen that a portion of cities are passively peaking due to population and floor space shrinkage, and their per capita carbon emissions are still high. Therefore, with the exception of a few cities such as Shanghai, Lu’an and Huainan, which are actively peaking, other cities still need to reduce energy intensity and optimise energy structure in order to facilitate the reduction of rural carbon emissions (Zhang and Li, 2022).
6 Conclusion and policy implications
6.1 Main findings
This study calculates carbon emissions at the building operation stage in the YRD region at the city level and analyses the driving factors with the GDIM decomposition model. Furthermore, by utilizing the GDIM-TAPIO method, this study analyses the decoupling status between carbon emissions from each building type and the residents’ incomes. Additionally, the scenario analysis method is applied to forecast peak carbon emissions at the building operation stage in the YRD region. The findings are as follows.
• The total carbon emissions at the building operation stage in the YRD region increased from 142.86 Mt CO2 in 2006 to 376.57 Mt CO2 in 2021. The spatiotemporal characteristics of carbon emissions are “high in the east and low in the west.” GDIM decomposition reveals that residents’ disposable income (I) and energy consumption (E) are the main driving factors of carbon emission increases, and the carbon intensity of income growth (C/I) and the carbon emission factor (C/E) inhibit the growth of carbon emissions for all building types. Floor area (F) is an important driving factor for PBs and URs but has little impact on RRs.
• In terms of the decoupling relationship between carbon emissions from the building operation stage and residents’ disposable income growth, the RRs performed better than did the URs and PBs. For PBs, the decoupling status in almost all cities was END. For URs, 6 and 1 out of 41 cities in the YRD region showed weak and strong decoupling, respectively, in the 13th FYP period. For the RRs, 4 and 9 cities exhibited weak and strong decoupling, respectively, in the 13th FYP period.
• The dynamic scenario simulation result shows the building sector in the YRD region is projected to peak between 2030 and 2035, and the estimated peak value is in the range of 498.18–504.83 Mt CO2. For each building type, PBs and URs are likely to peak between 2031 and 2036 at 266.62–272.88 Mt CO2 and 143.89–147.46 Mt CO2, respectively; RRs are most likely to peak in 2030, at 90.16–92.22 Mt CO2.
• Predictions of carbon emissions from building operations at the city level show that the key cities for reducing emissions from PBs and URs are Nanjing, Suzhou and some third- and fourth-tier cities such as Lu’an, Bozhou. The key cities for reducing emissions from RRs are eastern and central cities in the YRD region, such as Ningbo, Suzhou, Suqian and Changzhou.
6.2 Policy recommendations
To achieve the objective of “dual carbon,” the findings of this study suggest the following policy recommendations:
(1) Formulating scientific carbon reduction targets and strategies in the building sector for different cities. For example, for cities, which are hard to achieve carbon peak before 2030 of PBs and URs, stricter building energy efficiency regulations is needed and energy-saving renovations can be implemented to improve the energy performance of existing buildings (Zhu et al., 2022). For first-, new first-and second-tier cities, the total amount of carbon emissions at the building operation stage is high; and further control of the scale of building floor space and promoting low-carbon technologies are essential. For RRs in most cities, with high per capita carbon emission, it is important to optimise the structure of building energy use, such as promoting renewable energy sources and improving the ratio of electricity (Chen et al., 2024).
(2) Promoting green and low-carbon lifestyles among urban and rural residents. In YRD regions, carbon emissions from building operations have not yet been decoupled from residents’ disposable income. Therefore, it is important to promote low-carbon concepts among the residents. This can be achieved by popularising the knowledge of low-carbon building operation through news media, online platforms, and other means, and guiding people towards a green and low-carbon lifestyle. Furthermore, the advanced digital technology in the YRD region allows for the development of carbon emission management platforms to monitor building energy consumption. This will aid in promoting green and low-carbon energy usage habits.
(3) Improving the policy system for building conserving energy and reducing emissions. The carbon emission market trading system can be beneficial for reducing carbon emissions and urban-rural disparities (Tang and Li, 2022; Tang et al., 2023). However, due to the challenges of emission accounting and the decentralized of building ownership, only PBs are currently included in the market. Carbon Inclusion Methodology of Occupancy Energy Conservation in Residential Building in Heibei Province was released in 2023 to investigate the potential of including residential buildings in carbon emissions trading. For YRD region, a carbon trading system could be developed in the building sector, taking into account the differing carbon emission characteristics among PBs, URs and RRs.
6.3 Limitations and further perspectives
This study has certain limitations. Firstly, zoning adjustments in some cities in Anhui Province in 2011 and 2015 caused sudden changes in the building operations carbon emissions, and economic, social indicators, leading to the inaccurate results in the related cities of the driving factors and decoupling status. Secondly, for the carbon emission prediction, the energy intensity and carbon emission factors were determined based on the rate of change over the past 10 years. However, the COVID-19 pandemic has caused significant fluctuations in the energy intensity and carbon emission factors in 2020 and 2021, which may result in inaccurate forecast results. Furthermore, this study only examines the decoupling status of total building operating carbon emissions from disposable income. Further research could extend this analysis to encompass the decoupling of per capita carbon emission, per floor area carbon intensity and disposable income, as well as the drivers of decoupling statues.
Data availability statement
The original contributions presented in the study are included in the article/Supplementary Material, further inquiries can be directed to the corresponding author.
Author contributions
ZW: Writing–original draft, Writing–review and editing.
Funding
The author(s) declare that financial support was received for the research, authorship, and/or publication of this article. This research was funded by Scientific Research Fund of Zhejiang Provincial Education Department, grant number “Y202146094” and Scientific Research Foundation of Hangzhou City University, grant number “J-202217”, Hangzhou Philosophy and Social Science Project, grant number “M22JC053”, and Research Project on Campus Planning and Construction in Zhejiang Province, grant number “2023PCBG010”.
Conflict of interest
The author declares that the research was conducted in the absence of any commercial or financial relationships that could be construed as a potential conflict of interest.
Publisher’s note
All claims expressed in this article are solely those of the authors and do not necessarily represent those of their affiliated organizations, or those of the publisher, the editors and the reviewers. Any product that may be evaluated in this article, or claim that may be made by its manufacturer, is not guaranteed or endorsed by the publisher.
Supplementary material
The Supplementary Material for this article can be found online at: https://www.frontiersin.org/articles/10.3389/fenvs.2024.1388739/full#supplementary-material
References
Chen, C., and Bi, L. (2022). Study on spatio-temporal changes and driving factors of carbon emissions at the building operation stage- A case study of China. Build. Environ. 219, 109147. doi:10.1016/j.buildenv.2022.109147
Chen, H., Du, Q., Huo, T., Liu, P., Cai, W., and Liu, B. (2023). Spatiotemporal patterns and driving mechanism of carbon emissions in China’s urban residential building sector. Energy 263, 126102. doi:10.1016/j.energy.2022.126102
Chen, L., Liu, S., Cai, W., Chen, R., Zhang, J., and Yu, Y. (2024). Carbon inequality in residential buildings: evidence from 321 Chinese cities. Environ. Impact Assess. Rev. 105, 107402. doi:10.1016/j.eiar.2023.107402
Chen, L., Ma, M., and Xiang, X. (2023). Decarbonizing or illusion? How carbon emissions of commercial building operations change worldwide. Sustain. Cities Soc. 96, 104654. doi:10.1016/j.scs.2023.104654
Chen, L., You, K., Lv, G., Cai, W., Zhang, J., and Zhang, Y. (2024). The influence of demographic structure on residential buildings’ carbon emissions in China. J. Build. Eng. 87, 108951. doi:10.1016/j.jobe.2024.108951
Chen, R., Xu, P., Chen, L., and Yao, H. (2024). Did electrification of the building sector achieve carbon mitigation? A provincial retrospection in China. Build. Environ. 248, 111084. doi:10.1016/j.buildenv.2023.111084
China Association of Building Energy Efficiency (2022) 2022 research report of China building energy consumption and carbon emissions.
Chiodi, A., Gargiulo, M., Rogan, F., Deane, J. P., Lavigne, D., Rout, U. K., et al. (2013). Modelling the impacts of challenging 2050 European climate mitigation targets on Ireland’s energy system. Energy Policy 53, 169–189. doi:10.1016/j.enpol.2012.10.045
Cong, X., Zhao, M., and Li, L. (2015). Analysis of carbon Dioxide emissions of buildings in different regions of China based on STIRPAT model. Procedia Eng. 121, 645–652. doi:10.1016/j.proeng.2015.08.1057
Dai, G., and Yang, S. (2024). A comparative study of motivations driving EV purchases in different-tier Chinese cities. Transp. Res. Part D Transp. Environ. 126, 103993. doi:10.1016/j.trd.2023.103993
Dong, J., Li, C., and Wang, Q. (2021). Decomposition of carbon emission and its decoupling analysis and prediction with economic development: a case study of industrial sectors in henan province. J. Clean. Prod. 321, 129019. doi:10.1016/j.jclepro.2021.129019
Donglan, Z., Dequn, Z., and Peng, Z. (2010). Driving forces of residential CO2 emissions in urban and rural China: an index decomposition analysis. Energy Policy 38 (7), 3377–3383. doi:10.1016/j.enpol.2010.02.011
Fan, J.-L., Yu, H., and Wei, Y.-M. (2015). Residential energy-related carbon emissions in urban and rural China during 1996–2012: from the perspective of five end-use activities. Energy Build. 96, 201–209. doi:10.1016/j.enbuild.2015.03.026
Gan, L., Liu, Y., and Cai, W. (2023). Carbon neutral projections of public buildings in China under the shared socioeconomic pathways: a tertiary industry perspective. Environ. Impact Assess. Rev. 103, 107246. doi:10.1016/j.eiar.2023.107246
Gan, L., Liu, Y., Shi, Q., Cai, W., and Ren, H. (2022). Regional inequality in the carbon emission intensity of public buildings in China. Build. Environ. 225, 109657. doi:10.1016/j.buildenv.2022.109657
Gao, J., and O’Neill, B. C. (2020). Mapping global urban land for the 21st century with data-driven simulations and shared socioeconomic pathways. Nat. Commun. 11 (1), 2302. doi:10.1038/s41467-020-15788-7
Geng, J., Wang, J., Huang, J., Zhou, D., Bai, J., Wang, J., et al. (2022). Quantification of the carbon emission of urban residential buildings: the case of the greater Bay area cities in China. Environ. Impact Assess. Rev. 95, 106775. doi:10.1016/j.eiar.2022.106775
Hao, P., Yin, S., Wang, D., and Wang, J. (2023). Exploring the influencing factors of urban residential electricity consumption in China. Energy Sustain. Dev. 72, 278–289. doi:10.1016/j.esd.2022.12.015
Hong, L., Zhou, N., Feng, W., Khanna, N., Fridley, D., Zhao, Y., et al. (2016). Building stock dynamics and its impacts on materials and energy demand in China. Energy Policy 94, 47–55. doi:10.1016/j.enpol.2016.03.024
Huang, R., Zhang, X., and Liu, K. (2024). Assessment of operational carbon emissions for residential buildings comparing different machine learning approaches: a study of 34 cities in China. Build. Environ. 250, 111176. doi:10.1016/j.buildenv.2024.111176
Huang, Y., Wang, Y., Peng, J., Li, F., Zhu, L., Zhao, H., et al. (2023). Can China achieve its 2030 and 2060 CO2 commitments? Scenario analysis based on the integration of LEAP model with LMDI decomposition. Sci. Total Environ. 888, 164151. doi:10.1016/j.scitotenv.2023.164151
Huo, T., Cong, X., Cheng, C., Cai, W., and Zuo, J. (2023). What is the driving mechanism for the carbon emissions in the building sector? An integrated DEMATEL-ISM model. Energy 274, 127399. doi:10.1016/j.energy.2023.127399
Huo, T., Du, Q., Yuan, T., Cai, W., and Zhang, W. (2024). Has the provincial-level residential building sector reached the carbon peak? An integrated assessment model. Environ. Impact Assess. Rev. 105, 107374. doi:10.1016/j.eiar.2023.107374
Huo, T., Ma, Y., Cai, W., Liu, B., and Mu, L. (2021). Will the urbanization process influence the peak of carbon emissions in the building sector? A dynamic scenario simulation. Energy Build. 232, 110590. doi:10.1016/j.enbuild.2020.110590
Huo, T., Ma, Y., Xu, L., Feng, W., and Cai, W. (2022). Carbon emissions in China’s urban residential building sector through 2060: a dynamic scenario simulation. Energy 254, 124395. doi:10.1016/j.energy.2022.124395
Huo, T., Ma, Y., Yu, T., Cai, W., Liu, B., and Ren, H. (2021). Decoupling and decomposition analysis of residential building carbon emissions from residential income: evidence from the provincial level in China. Environ. Impact Assess. Rev. 86, 106487. doi:10.1016/j.eiar.2020.106487
Huo, T., Ren, H., Zhang, X., Cai, W., Feng, W., Zhou, N., et al. (2018). China’s energy consumption in the building sector: a statistical yearbook-energy balance sheet based splitting method. J. Clean. Prod. 185, 665–679. doi:10.1016/j.jclepro.2018.02.283
Huo, T., Xu, L., Liu, B., Cai, W., and Feng, W. (2022). China’s commercial building carbon emissions toward 2060: an integrated dynamic emission assessment model. Appl. Energy 325, 119828. doi:10.1016/j.apenergy.2022.119828
Jiang, T., Su, B., Wang, Y., Huang, J., Jing, C., Gao, M., et al. (2022). Data from: Gridded datasets for population and economy under shared socioeconomic pathways. Science Data Bank. doi:10.57760/sciencedb.01683
Jiang, T., Su, B., Wang, Y., Huang, J., Jing, C., Gao, M., et al. (n.d.) Gridded datasets for population and economy under shared socioeconomic pathways.
Ke, Y., and Cai, W. (2023). Breaking the “income-waiting dilemma” to decrease residential building carbon emissions. Energy Policy 175, 113463. doi:10.1016/j.enpol.2023.113463
Kim, S.-H., Lee, S., Han, S.-Y., and Kim, J.-H. (2020). Scenario analysis for GHG emission reduction potential of the building sector for new city in South Korea. Energies 13 (20), 5514. doi:10.3390/en13205514
Lei, F., Yan, Y., Yue, Y., Bi, W., and Ni, P. (2023). The pathway of regional carbon peak in building sector: a case study of Central China. J. Build. Eng. 80, 108031. doi:10.1016/j.jobe.2023.108031
Li, J., Zhang, D., and Su, B. (2019). The impact of social awareness and lifestyles on household carbon emissions in China. Ecol. Econ. 160, 145–155. doi:10.1016/j.ecolecon.2019.02.020
Li, R., Chen, L., Cai, W., You, K., Li, Z., and Ran, L. (2023). Historical peak situation of building carbon emissions in different climate regions in China: causes of differences and peak challenges. Sci. Total Environ. 903, 166621. doi:10.1016/j.scitotenv.2023.166621
Li, R., Liu, Q., Cai, W., Liu, Y., Yu, Y., and Zhang, Y. (2023). Echelon peaking path of China’s provincial building carbon emissions: considering peak and time constraints. Energy 271, 127003. doi:10.1016/j.energy.2023.127003
Li, R., You, K., Cai, W., Wang, J., Liu, Y., and Yu, Y. (2023). Will the southward center of gravity migration of population, floor area, and building energy consumption facilitate building carbon emission reduction in China? Build. Environ. 242, 110576. doi:10.1016/j.buildenv.2023.110576
Li, Y., Wang, J., Deng, B., Liu, B., Zhang, L., and Zhao, P. (2023). Emission reduction analysis of China’s building operations from provincial perspective: factor decomposition and peak prediction. Energy Build. 296, 113366. doi:10.1016/j.enbuild.2023.113366
Liang, Y., Cai, W., and Ma, M. (2019). Carbon Dioxide intensity and income level in the Chinese megacities’ residential building sector: decomposition and decoupling analyses. Sci. Total Environ. 677, 315–327. doi:10.1016/j.scitotenv.2019.04.289
Lin, B., and Liu, H. (2015). CO2 emissions of China’s commercial and residential buildings: evidence and reduction policy. Build. Environ. 92, 418–431. doi:10.1016/j.buildenv.2015.05.020
Lin, C., and Li, X. (2024). Carbon peak prediction and emission reduction pathways exploration for provincial residential buildings: evidence from fujian province. Sustain. Cities Soc. 102, 105239. doi:10.1016/j.scs.2024.105239
Liu, Y., Yang, M., Cheng, F., Tian, J., Du, Z., and Song, P. (2022). Analysis of regional differences and decomposition of carbon emissions in China based on generalized Divisia index method. Energy 256, 124666. doi:10.1016/j.energy.2022.124666
Ma, M., and Cai, W. (2019). Do commercial building sector-derived carbon emissions decouple from the economic growth in tertiary industry? A case study of four municipalities in China. Sci. Total Environ. 650, 822–834. doi:10.1016/j.scitotenv.2018.08.078
Ma, M., Feng, W., Huo, J., and Xiang, X. (2022). Operational carbon transition in the megalopolises’ commercial buildings. Build. Environ. 226, 109705. doi:10.1016/j.buildenv.2022.109705
Ma, M., Ma, X., Cai, W., and Cai, W. (2019). Carbon-dioxide mitigation in the residential building sector: a household scale-based assessment. Energy Convers. Manag. 198, 111915. doi:10.1016/j.enconman.2019.111915
Ma, M., Yan, R., and Cai, W. (2017). An extended STIRPAT model-based methodology for evaluating the driving forces affecting carbon emissions in existing public building sector: evidence from China in 2000–2015. Nat. Hazards 89, 741–756. doi:10.1007/s11069-017-2990-4
McNeil, M. A., Letschert, V. E., Stephane, de la R. du C., and Ke, J. (2012). Bottom-up energy analysis system - methodology and results (Berkeley, CA: Lawrence Berkeley National Laboratory).
Miao, Z., Liu, S., and Chen, X. (2020). Driving factors and spatio-temporal features underlying industrial SO2 emissions in “2+26” in north China and extended cities. Chin. J. Popul. Resour. Environ. 18 (4), 296–318. doi:10.1016/j.cjpre.2021.04.006
Nilsson, A. E., Bay-Larsen, I., Carlsen, H., Van Oort, B., Bjørkan, M., Jylhä, K., et al. (2017). Towards extended shared socioeconomic pathways: a combined participatory bottom-up and top-down methodology with results from the barents region. Glob. Environ. Change 45, 124–132. doi:10.1016/j.gloenvcha.2017.06.001
Pan, Y., Wei, J., Tang, S., Wang, J., Jin, Y., and Wang, Y. (2022). Prediction and analysis of carbon neutrality in building sector in Shanghai. HV&Ac 52 (8), 18–28. doi:10.19991/j.hvac1971.2022.08.03
Sarıca, K., Harputlugil, G. U., İnaner, G., and Kollugil, E. T. (2023). Building sector emission reduction assessment from a developing European economy: a bottom-up modelling approach. Energy Policy 174, 113429. doi:10.1016/j.enpol.2023.113429
Shi, Q., Liang, Q., Wang, J., Huo, T., Gao, J., You, K., et al. (2023). Dynamic scenario simulations of phased carbon peaking in China’s building sector through 2030–2050. Sustain. Prod. Consum. 35, 724–734. doi:10.1016/j.spc.2022.12.003
Song, X., Zhang, X., Ding, X., Guo, L., and Huang, J. (2023). Building and carbon emissions: evidence from real estate purchase restriction policy. Urban Clim. 52, 101726. doi:10.1016/j.uclim.2023.101726
Tang, X., Hao, G., Wang, Y., Li, D., and Luo, Y. (2023). Research on an equilibrium development model between urban and rural areas of henan including carbon sink assets under the dual carbon goal. Front. Environ. Sci. 10 (January 4), 1037286. doi:10.3389/fenvs.2022.1037286
Tang, X., and Li, J. (2022). Study of the mechanism of digitalization boosting urban low-carbon transformation. Front. Environ. Sci. 10, 982864. doi:10.3389/fenvs.2022.982864
Tang, X., Liu, S., Wang, Y., and Wan, Y. (2024). Study on carbon emission reduction countermeasures based on carbon emission influencing factors and trends. Environ. Sci. Pollut. Res. 31 (9), 14003–14022. doi:10.1007/s11356-024-31962-6
Tian, S., Xu, Y., Wang, Q., Zhang, Y., Yuan, X., Ma, Q., et al. (2022). Research on peak prediction of urban differentiated carbon emissions -- a case study of Shandong province, China. J. Clean. Prod. 374, 134050. doi:10.1016/j.jclepro.2022.134050
Vaninsky, A. (2014). Factorial decomposition of CO2 emissions: a generalized Divisia index approach. Energy Econ. 45, 389–400. doi:10.1016/j.eneco.2014.07.008
Wang, H., and Guo, J. (2024). Research on the impact mechanism of multiple environmental regulations on carbon emissions under the perspective of carbon peaking pressure: a case study of China’s coastal regions. Ocean Coast. Manag. 249, 106985. doi:10.1016/j.ocecoaman.2023.106985
Wang, J., Li, N., Huang, M., Zhao, Y., and Qiao, Y. (2021). The challenges of rising income on urban household carbon emission: do savings matter? J. Clean. Prod. 326, 129295. doi:10.1016/j.jclepro.2021.129295
Wang, J., You, K., Qi, L., and Ren, H. (2022). Gravity center change of carbon emissions in Chinese residential building sector: differences between urban and rural area. Energy Rep. 8, 10644–10656. doi:10.1016/j.egyr.2022.08.208
Wang, Q., Zhao, M., Li, R., and Su, M. (2018). Decomposition and decoupling analysis of carbon emissions from economic growth: a comparative study of China and the United States. J. Clean. Prod. 197, 178–184. doi:10.1016/j.jclepro.2018.05.285
Wang, S., Mo, H., and Fang, C. (2022). Carbon emissions dynamic simulation and its peak of cities in the pearl River Delta urban agglomeration. Chin. Sci. Bull. 67 (7), 670–684. doi:10.1360/TB-2021-0681
Wang, Y., Guo, J., Yue, Q., Chen, W.-Q., Du, T., and Wang, H. (2023). Total CO2 emissions associated with buildings in 266 Chinese cities: characteristics and influencing factors. Resour. Conservation Recycl. 188, 106692. doi:10.1016/j.resconrec.2022.106692
Wang, Y., Su, X., Qi, L., Shang, P., and Xu, Y. (2019). Feasibility of peaking carbon emissions of the power sector in China’s eight regions: decomposition, decoupling, and prediction analysis. Environ. Sci. Pollut. Res. 26 (28), 29212–29233. doi:10.1007/s11356-019-05909-1
Wang, Y., Wu, T., Li, H., Skitmore, M., and Su, B. (2020). A statistics-based method to quantify residential energy consumption and stock at the city level in China: the case of the guangdong-Hong Kong-Macao greater Bay area cities. J. Clean. Prod. 251, 119637. doi:10.1016/j.jclepro.2019.119637
Wu, P., Song, Y., Zhu, J., and Chang, R. (2019). Analyzing the influence factors of the carbon emissions from China’s building and construction industry from 2000 to 2015. J. Clean. Prod. 221, 552–566. doi:10.1016/j.jclepro.2019.02.200
Wu, S., Hu, S., Frazier, A. E., and Hu, Z. (2023). China’s urban and rural residential carbon emissions: past and future scenarios. Resour. Conservation Recycl. 190, 106802. doi:10.1016/j.resconrec.2022.106802
Wu, X., Xu, X., Chen, G., Liao, W., and Liu, X. (2024). Exploring urban building space provision and inequality: a three-dimensional perspective on Chinese cities toward sustainable development goals. Sustain. Cities Soc. 102, 105202. doi:10.1016/j.scs.2024.105202
Xu, G., and Wang, W. (2020). China’s energy consumption in construction and building sectors: an outlook to 2100. Energy 195, 117045. doi:10.1016/j.energy.2020.117045
Yan, R., Xiang, X., Cai, W., and Ma, M. (2022). Decarbonizing residential buildings in the developing world: historical cases from China. Sci. Total Environ. 847, 157679. doi:10.1016/j.scitotenv.2022.157679
Yang, T., Pan, Y., Yang, Y., Lin, M., Qin, B., Xu, P., et al. (2017). CO 2 emissions in China’s building sector through 2050: a scenario analysis based on a bottom-up model. Energy 128, 208–223. doi:10.1016/j.energy.2017.03.098
Yao, C., Chen, C., and Li, M. (2012). Analysis of rural residential energy consumption and corresponding carbon emissions in China. Energy Policy 41, 445–450. doi:10.1016/j.enpol.2011.11.005
You, K., Ren, H., Cai, W., and Huang, R. (2023). Modeling carbon emission trend in China’s building sector to year 2060. Resour. Conservation Recycl. 188, 106679. doi:10.1016/j.resconrec.2022.106679
Yu, B., and Fang, D. (2021). Decoupling economic growth from energy-related PM2.5 emissions in China: a GDIM-based indicator decomposition. Ecol. Indic. 127, 107795. doi:10.1016/j.ecolind.2021.107795
Zhang, C., and Luo, H. (2023). Research on carbon emission peak prediction and path of China’s public buildings: scenario analysis based on LEAP model. Energy Build. 289, 113053. doi:10.1016/j.enbuild.2023.113053
Zhang, F., Deng, X., Xie, L., and Xu, N. (2021). China’s energy-related carbon emissions projections for the shared socioeconomic pathways. Resour. Conservation Recycl. 168, 105456. doi:10.1016/j.resconrec.2021.105456
Zhang, H., and Li, S. (2022). Carbon emissions’ spatial-temporal heterogeneity and identification from rural energy consumption in China. J. Environ. Manag. 304, 114286. doi:10.1016/j.jenvman.2021.114286
Zhang, J., Yan, Z., Bi, W., Ni, P., Lei, F., Yao, S., et al. (2023). Prediction and scenario simulation of the carbon emissions of public buildings in the operation stage based on an energy audit in xi’an, China. Energy Policy 173, 113396. doi:10.1016/j.enpol.2022.113396
Zhang, Q., and Li, J. (2023). Building carbon peak scenario prediction in China using system dynamics model. Environ. Sci. Pollut. Res. 30 (42), 96019–96039. doi:10.1007/s11356-023-29168-3
Zhang, S., Shi, B., and Ji, H. (2023). How to decouple income growth from household carbon emissions: a perspective based on urban-rural differences in China. Energy Econ. 125, 106816. doi:10.1016/j.eneco.2023.106816
Zhang, S., Wang, M., Zhu, H., Jiang, H., and Liu, J. (2024). Impact factors and peaking simulation of carbon emissions in the building sector in Shandong province. J. Build. Eng. 87, 109141. doi:10.1016/j.jobe.2024.109141
Zhang, S., Zhao, M., Liu, Z., Yang, F., Lu, B., Zhao, Z., et al. (2023). City-level population projection for China under different pathways from 2010 to 2100. Sci. Data 10 (1), 809. doi:10.1038/s41597-023-02735-6
Zhang, Y., Zhang, Y., Zhang, Y., Gong, C., and Kong, Y. (2022). Analysis of the carbon emission driving factors and prediction of a carbon peak scenario—a case study of xi’an city. Heliyon 8 (11), e11753. doi:10.1016/j.heliyon.2022.e11753
Zhao, L., Zhao, T., and Yuan, R. (2021). Drivers of household decarbonization: decoupling and decomposition analysis. J. Clean. Prod. 289, 125154. doi:10.1016/j.jclepro.2020.125154
Zhao, Q., Gao, W., Su, Y., and Wang, T. (2023). Carbon emissions trajectory and driving force from the construction industry with a city-scale: a case study of Hangzhou, China. Sustain. Cities Soc. 88, 104283. doi:10.1016/j.scs.2022.104283
Zhong, X., Hu, M., Deetman, S., Rodrigues, J. F. D., Lin, H.-X., Tukker, A., et al. (2021). The evolution and future perspectives of energy intensity in the global building sector 1971–2060. J. Clean. Prod. 305, 127098. doi:10.1016/j.jclepro.2021.127098
Zhu, C., Chang, Y., Li, X., and Shan, M. (2022). Factors influencing embodied carbon emissions of China’s building sector: an analysis based on extended STIRPAT modeling. Energy Build. 255, 111607. doi:10.1016/j.enbuild.2021.111607
Zhu, C., Li, X., Zhu, W., and Gong, W. (2022). Embodied carbon emissions and mitigation potential in China’s building sector: an outlook to 2060. Energy Policy 170, 113222. doi:10.1016/j.enpol.2022.113222
Keywords: carbon emission prediction, Yangtze River Delta (YRD) region, decoupling state, Monte Carlo simulation, dynamic scenario simulation, building operations, generalized divisia index method
Citation: Wang Z (2024) Decomposition, decoupling and dynamic prediction of carbon emissions from city-level building operations: a case study of the Yangtze River Delta. Front. Environ. Sci. 12:1388739. doi: 10.3389/fenvs.2024.1388739
Received: 20 February 2024; Accepted: 07 May 2024;
Published: 28 May 2024.
Edited by:
Kaifeng Duan, Fuzhou University, ChinaReviewed by:
Tang Xinfa, Jiangxi Science and Technology Normal University, ChinaWeijun Gao, University of Kitakyushu, Japan
Copyright © 2024 Wang. This is an open-access article distributed under the terms of the Creative Commons Attribution License (CC BY). The use, distribution or reproduction in other forums is permitted, provided the original author(s) and the copyright owner(s) are credited and that the original publication in this journal is cited, in accordance with accepted academic practice. No use, distribution or reproduction is permitted which does not comply with these terms.
*Correspondence: Zhuoyao Wang, d2FuZ3podW95YW9AaHpjdS5lZHUuY24=