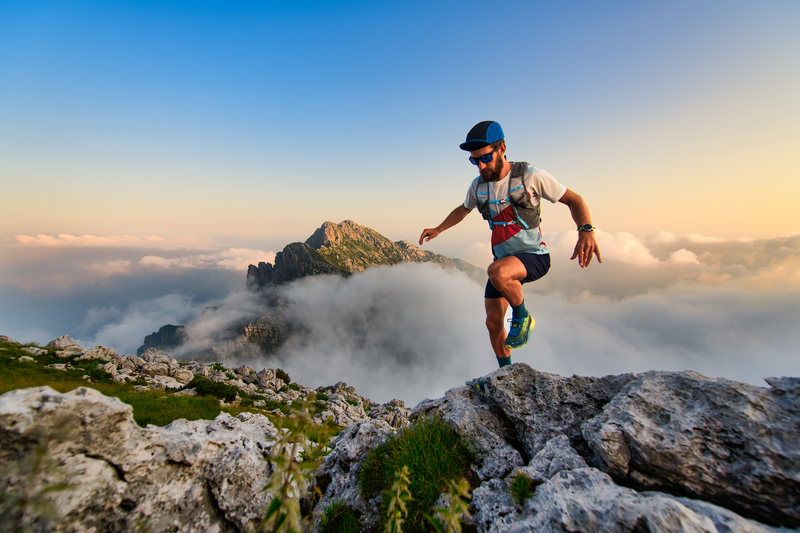
95% of researchers rate our articles as excellent or good
Learn more about the work of our research integrity team to safeguard the quality of each article we publish.
Find out more
ORIGINAL RESEARCH article
Front. Environ. Sci. , 16 April 2024
Sec. Toxicology, Pollution and the Environment
Volume 12 - 2024 | https://doi.org/10.3389/fenvs.2024.1381409
This article is part of the Research Topic Remediation and Health Risks of Heavy Metal Contaminated Soils View all 14 articles
Introduction: Soil pollution directly impacts food quality and the lives of both humans and animals. The concentration of heavy metals in Egypt’s drain-side soils is rising, which is detrimental to the quality of the soil and crops. The key to reducing the detrimental effects on the ecosystem is having accurate maps of the spatial distribution of heavy metals and the subsequent use of environmentally sustainable remediation approaches. The objective of this work is to assess soil contamination utilizing spatial mapping of heavy metals, determine contamination levels using Principal Component Analysis (PCA), and calculate both the contamination severity and the potential for bioremediation in the soils surrounding the main drain of Bahr El-Baqar. Furthermore, evaluating the capacity of microorganisms (bacteria, fungi, and “Actinomycetes) to degrade heavy elements in the soil.
Methodology: 146 soil sample locations were randomly selected near the Bahr El-Baqar drain to examine the degree of soil pollution Ordinary Kriging (OK), method was used to map and analyze the spatial distribution of soil contamination by seven heavy metals (Cr, Fe, Zn, Cd, Pb, As, and Ni). Modified contamination degree (mCd) and PCA were used to assess the research area’s soil pollution levels. The process involved isolating, identifying, and classifying the microorganisms present in the soil of the study area. The study findings showed that variography suggested the Stable model effectively matched pH, SOM, and Cd values. Furthermore, the exponential model proved suitable for predicting Fe, Pb and Ni, while the spherical model was appropriate for Ni, Cr, and Zn.
Results: The study revealed three levels of contamination, with an extremely high degree (EHDC) affecting approximately 97.49% of the area. The EHDC exhibited average concentrations of heavy metals: 79.23 ± 17.81 for Cr, 20,014.08 ± 4545.91 for Fe, 201.31 ± 112.97 for Zn, 1.33 ± 1.37 for Cd, 40.96 ± 26.36 for Pb, 211.47 ± 13.96 for As, and 46.15 ± 9.72 for Ni. Isolation and identification of microorganisms showed a significant influence on the breakdown of both organic and inorganic pollutants in the environment. The study demonstrated exceptionally high removal efficiency for As and Cr, with a removal efficiency reached 100%, achieved by Rhizopus oryzae, Pseudomonas aeruginosa, and Bacillus thuringiensis.
Conclusion: This study has designated management zones for soil contamination by mapping soil pollutants, geo-identified them, and found potential microorganisms that could significantly reduce soil pollution levels.
Soil plays a crucial role in supporting life on our planet. Approximately 95% of the world’s food supply is generated from soil, which also offers additional benefits such as biomass production, safeguarding natural resources, and conserving biodiversity (Ferrira et al., 2022). The processes of soil degradation, because of industrial and agricultural activities, along with increasing urbanization, lead to a decline in soil functions and ecosystem services (Dubey et al., 2020; Hendawy et al., 2019). The heavy metals, such as Zn, Fe, Cu, Mo, and Mn, play essential roles in trace amounts for plants, animals, and microbes. However, an excessive concentration of heavy metals beyond the necessary minimum levels can be considered toxic and harmful to life, even though some are not required by plants. For instance, mercury (Hg), cadmium (Cd), and lead (Pb) are heavy metals that are not essential for living organisms. Therefore, the presence of these heavy metals can disrupt the life cycles of organisms (Alsabhan et al., 2022). Recent research indicates that toxic metal contamination is a significant issue that affects both rural and urban areas, having detrimental effects on ecosystem and environmental sustainability (El-Behairt et al., 2022). Soil contamination is a major global problem that endangers both human health and ecological health (Singh and Singh, 2020). Over the past two decades, Egypt’s soils have been subjected to rising levels of soil contamination, which has reduced soil productivity and quality (Abdelrahman et al., 2019; Baroudy et al., 2020). Potentially toxic metals (PTMs) are harmful pollutants because they can cause death, and bio-accumulation, and are not easily degradable (Kumar et al., 2022). Pollution has an adverse effect on plants and soils, which makes it extremely dangerous for food security. This immediately eliminates the beneficial bacteria in the soil, lowers the amount of organic matter in the soil, makes the soil unusable for agriculture, and causes an imbalance in soil nutrients impacts plant growth and the way plants interact with microorganisms, which in turn impacts the soil’s quality and crop yield (Ayilara et al., 2023). Inhibiting enzyme activity, competing with necessary cations, and creating oxidative stress are only a few of the ways that an excess of PTMs stresses the soil biota (Nowicka, 2022). Consequently, the entire life cycle of the plant, From the initial stage of seed germination to reaching maturity, the process is negatively impacted, resulting in decreased crop yields and compromised quality (Yang et al., 2021). Numerous food, feed, and forage plants, often referred to as hyperaccumulators, exhibit strong resistance to metal stress and can absorb excessive potentially toxic metals (PTMs), subsequently transporting them to their above-ground parts (Chen et al., 2022; Abuzaid et al., 2019). The main source of soil pollutants is anthropogenic activity. According to (Peña, 2022; Gautam et al., 2023), Both organic and inorganic contaminants can move to the soil components through various pathways, such as the application of fertilizers and pesticides, inadequate wastewater disposal, the usage of plastics, as well as the combustion of fossil fuels. Remediation is very difficult and expensive after these pollutants have gotten into the soil matrix. Additionally, through the food chain, they can offer serious health hazards. Analyzing the spatial distribution of PTM concentration is the first step in improving soil pollution assessment (Hammam et al., 2022). This approach identifies contaminated regions and is a crucial step in risk management (Wang et al., 2021). This has prompted the utilization of Geographic Information Systems (GIS) for the spatial distribution of potentially toxic metals (PTMs) in numerous soils all over the world (Zhen et al., 2019; Ahmed and Pandey, 2020; Gozukara, 2021). Kriging is a potent geo-statistical technique during the examination of soil property spatial distribution and the integration of data into raster maps through spatial interpolation. To determine the spatial structure of soil variables, variograms and related metrics (nugget, sill, and range) are essentially used (Shi and Wang, 2020; Abuzaid et al., 2022). This technique’s fundamental application, ordinary kriging (OK), offers an accurate and optimal forecast (Golden et al., 2019). By using a variety of variograms, OK replicates spatial variability and provides a range of map outputs while reducing the variation of prediction errors (Dad and Shafiq, 2021). Numerous studies have been done so far to address the issue of soil contamination by creating workable risk assessment and remediation techniques. To solve this issue, researchers have investigated both biological and non-biological strategies. Various methods, including physical, chemical, and biological approaches, are available According to (Liu et al., 2018), physical approaches include physically removing, washing, encapsulating, and electro-kinetically extracting contaminants from soil. To immobilize and lower the bioavailability of contaminants, chemical techniques such as soil precipitation and solidification are used (Jiang et al., 2023). To eliminate or change pollutants into less hazardous species, biological techniques use plants and microorganisms. Because they may reduce pollutants without creating secondary contaminants and are more cost-effective than physical measures, biological solutions are regarded as the most promising of these strategies (Gustave et al., 2021). Bioaugmentation is a well-established method for remediating heavy metal contamination in the environment. It involves introducing native and external microorganisms capable of with-standing and mitigating the toxic effects of heavy metals (Hassan et al., 2019; Purwanti et al., 2020). Micro-organisms native to the contaminated soil are isolated and then re-introduced into the same contaminated soil (Purwanti et al., 2020). Fungi have emerged as a highly effective option for remedying heavy metal contamination due to their substantial biomass and adsorption capabilities. Additionally, they produce various extracellular metabolites, such as proteins and enzymes, and their biomass can serve as a valuable natural resource for treating industrial effluents (Dell’Anno et al., 2022). Fungi contribute to the cleanup of heavy metal-contaminated environments through both metabolically active mechanisms like biomineralization, biotransformation, bioprecipitation, and bioaccumulation, as well as metabolically passive mechanisms such as biosorption (Goutam et al., 2021; Mohamadhasani and Rahimi, 2022). Abu-Elsaoud et al. (2017) observed that inoculation with an indigenous mycorrhizal fungus (Funneliformis geosporum) enhances soil quality and increases wheat yields, even in the presence of high concentrations of Zn, by reducing zinc accumulation in wheat plants. On the other hand, exogenous microorganisms are collected from locations external to the contaminated areas and introduced into the targeted contaminated area (Huang and Ye, 2020). Various processes used for remediating heavy metals with the help of microbes include biosorption, bioaccumulation, bio-chelation, bio-digestion, biomineralization, and biotransformation as described by (Girma, 2015). The selection of microorganisms depends on two main criteria: their ability to degrade the targeted pollutants and their capacity to endure and flourish in various environments. Microorganisms such as bacteria, fungi, yeast, actinomycetes, and algae exhibit resilience and adaptability across a wide range of conditions, allowing them to effectively eliminate heavy metals from polluted areas. This capability primarily arises from the composition of microbial cell walls, which consist of polysaccharides, lipids, and proteins. These components play a crucial role in binding metal ions through carboxylate, hydroxyl, amino, and phosphate groups, leading to the creation of non-toxic complex compounds according to (Girma, 2015; Huang and Ye, 2020; Nwaehiri et al., 2020; Purwanti et al., 2020). According to (Chabukdhara et al., 2017), Candida spherica has been identified as a producer of biosurfactants that exhibit high removal efficiencies, with removal rates of 95%, 90%, and 79% for iron (Fe), zinc (Zn), and lead (Pb), respectively. Furthermore, it has been discovered that C (Luna et al., 2016). Vulgaris biomass serves as an exceptionally effective biosorbent for the removal of cadmium (Cd2+), copper (Cu2+), and lead (Pb2+) from a mixed solution containing 50 mg dm−3 of each metal ion. The removal efficiencies for these metals are notably high, reaching 95.5%, 97.7%, and 99.4%, respectively (Goher et al., 2016).
This research aims to generate a spatial distribution of soil heavy metals and determine contamination levels, in the soils surrounding Bahr El-Baqar drain. Additionally, discover novel enzymatic biodegrading agents produced by various microorganisms, which have the potential to extract heavy metals from the soil.
The investigated area is located south Al-shrkia Governorate adjacent Bahr El-Baqar’s drain. The drain collects the wastewater from Sharqia Governorate’s Belbeis and Qalubiya minor drains. El-Baqar’s main drain. Figure 1, showed latitudes 30° 18′47.05″ N to 30° 20′0.64″ N, and longitudes 31° 22′25.03″ E to 31° 24′35.84″ E. The area covers 294.67 ha. Two primary sources of irrigation are employed: water from the Bahr al-Baqar drain and groundwater. As for the soil in this region, it is primarily characterized by clay to sandy texture. The crop patterns in the region varied between wheat and clover, as well as tomatoes, strawberries, and peas. Sentinel-2A satellite data from October 2022 included a high-resolution image used to show the location of the study area.
Several 146 soil sample locations were randomly selected near the Bahr El-Baqar drain (Figure 1) to examine the degree of soil pollution. GPS has been used to keep track of each location’s coordinates. This involved grinding the samples, air-drying them, and sieving them through a 2 mm. Subsequently, the samples were subjected to analysis for electrical conductivity (EC) in soil paste extracts, pH levels, and soil organic matter (SOM). To assess the concentrations of Cr, Fe, Zn, Cd, Pb, As, and Ni, the samples, the entire quantities of these metals were extracted according to the guidelines provided by the United States Environmental Protection Agency (USEPA). This extraction process, known as Method 3052, entails acid digestion with microwave assistance, utilizing concentrated HNO3, HF, and HCl. The metal concentrations were determined using Inductively Coupled Plasma Optical Emission Spectroscopy (Thermo ICP-MS type iCAP-RQ), and each measurement was conducted three times.
The application of PCA aids in the identification of pollution sources. The statistical software package SPSS 28.0 is utilized to determine the existence of variance explained by each significant component and the number of significant factors by extracting their eigenvalues from the correlation matrix. Before conducting Principal Component Analysis (PCA), the Kaiser-Meyer-Olkin (KMO) test was employed to assess the sample’s suitability for each variable (Kaiser, 1960). The resulting z-scores were subjected to Pearson’s correlation to investigate metal correlations in soils. The Kaiser-Meyer-Olkin measure of sample adequacy, Bartlett’s test of sphericity, Varimax rotation, and principal component (PC) approach were used in the factor analysis of the correlation matrix to find possible metal sources. Absolute loading values greater than 0.75 were considered highly significant, values between 0.75 and 0.5 indicated a moderate association, and values falling in the range of 0.49 to 0.30 suggested a weak association with the principal component and the PCs with eigenvalues >1.0 were the only ones taken into consideration (Fan et al., 2019).
Utilizing the Kriging interpolation method, geostatistical studies were completed in ArcGIS 10.4 (36). The following semi-variogram models were employed with ordinary kriging (OK) (Eq. 1):
Where, (h) (h) represents the semivariance at a specified lag distance “h,” “N(h)” signifies the count of sample pairs at that particular lag distance, “Z (X_α)” denotes the value at a specific sample site, and “Z (X_α+h)” signifies the value at a sample site located at a distance “h” from the original location.
To establish spatial variation parameters, including the nugget (C0), partial sill (C), sill (C0 + C), and range (a), experimental semi-variograms are fitted using various models through the least square method. The nugget, which characterizes short-range variability, corresponds to the semi-variogram at a lag distance of zero. The sill, indicative of the overall sample variability, is the point at which the model flattens out. Range refers to the lag distance at which the sill is encountered (Goenster-Jordan et al., 2018). To evaluate the accuracy and effectiveness of OK models, the study utilized the cross-validation technique, considering predictive errors such as mean error (ME), root mean square error (RMSE), mean standardized error (MSE), root mean square standardized error (RMSSE), and average standard error (ASE). Model selection was determined based on the following criteria: the lowest ME and MSE (approaching zero), identical RMSE and ASE values, and an RMSSE value close to one, indicating the most suitable model (Mallik et al., 2020; Sebei et al., 2020). These errors’ mathematical expressions are as follows (Eqs 2–4):
In this context, “n” signifies the count of predicted values within a cluster of nearby soil samples, while “x_i” and “y_ denote the actual and predicted values, respectively. Additionally, “σM” stands for the standardized error of the predicted values.
According to (Håkanson, 1980), the contamination factor (CF) serves as a measure to express the degree of contamination. This CF ratio is computed by dividing the concentration of each metal in the sediment by its corresponding background value. To assess the overall contamination of various heavy metals in each soil sample, a modified degree of contamination (mCd) was employed, as developed by (Abrahim and Parker, 2008; Cheng et al., 2015). The calculation of mCd involves the use of the following formulas (Eq. 5):
n is number of studied elements.
The terms used to categorize the mCd are as follows:
mCd <1.5: Indicates negligible to very minimal contamination.
1.5 ≤ mCd <2: Implies a low level of contamination.
2 ≤ mCd <4: Signifies a moderate level of contamination.
4 ≤ mCd <8: Represents a substantial degree of contamination.
8 ≤ mCd <16: Reflects an exceptionally high degree of contamination.
16 ≤ mCd <32: Points to an extraordinarily high degree of contamination.
mCd ≥32: Corresponds to an extremely high level of contamination.
To extract microorganisms from soil samples, we initiated the process by combining precisely 10 g of soil with 90 mL of deionized water. For isolating bacteria, actinomycetes and fungi from effluent samples, we diluted 10 mL of the effluent with 90 mL of deionized water. Next, the mixture underwent vigorous shaking using an automated orbital shaker (Searchtech Nig Ltd.) at 180 rpm for 3 h. Subsequently, the resulting suspension underwent ten-fold serial dilution. Subsequently, we aseptically dispensed 0.1 mL aliquots from each of these dilutions (10−2, 10−3, 10−4, or 10−5) onto nutrient agar, starch nitrate agar and potato dextrose agar plates (for bacteria, actinomycetes and fungi, respectively) using the spread plate technique. These plates were incubated at 38°C for 24 h (for bacteria) and incubated at 28°C for 3–7 days (for actinomycetes and fungi) in an incubator. After the incubation period, the colonies that developed were sub-cultured to obtain pure samples for subsequent identification and grouping.
Bacterial and actinomyctes isolates underwent meticulous identification through the assessment of their biochemical, physiological, and morphological traits, including detailed examination of colony morphology and the use of staining techniques. Following Bargey’s manual, this comprehensive approach facilitated precise characterization of bacterial species and actinomycetes. In contrast, fungal isolates were primarily identified using morphological methods, which involved observations of colony and microscopic morphology. Through careful scrutiny of these characteristics, fungal species were accurately distinguished and classified.
The evaluation of microbial remediation of heavy metals in soil employed a precise microbial culture technique. Initially, a targeted microorganism was introduced into heavily polluted soil. Subsequently, the soil underwent incubation under controlled conditions: 38°C for 48 h to facilitate bacterial activity and 28°C for 96 h to support fungal and actinomycetes growth. Following the incubation period, the concentration of remaining heavy metals was quantified using a spectrophotometer and compared against pre-injection levels. To ascertain the concentration of unabsorbed metal ions and gauge the effectiveness of microbial restoration, an atomic absorption spectrophotometer was employed. This methodological approach allowed for a thorough assessment of how microbial activity influenced the remediation of heavy metal contamination in soil, shedding light on the efficacy of bacterial and fungal processes in metal ion absorption and retention.
The determination of heavy metal loss percentage and bioaccumulation factor adhered to the methodology outlined by Akhtar et al. (2013). Calculating the reduction in heavy metal content involved subtracting the initial concentration of heavy metals (measured at 0 h) from the final concentration (measured after 96 h). To assess the impact of microorganisms on heavy metal reduction, the amount of metal lost in a medium with microorganisms was compared to that in a medium lacking microorganisms. The bioaccumulation factor was calculated as the ratio of heavy metal content within the microorganism to the heavy metal content in the medium after 96 h. This comprehensive analysis provided insight into the efficacy of microbial activity in mitigating heavy metal contamination and elucidated the role of microorganisms in metal accumulation processes.
Wastewater from urban areas, agricultural fields, and industrial facilities flows into the Bahr El-Baqar drain, resulting in soil contamination since this irrigation water source is low in the area. The total volume of drainage water in the Bahr El-Baqar drain is mixed by 40%, industrial sources contribute 2%, and agricultural sources contribute 58%, according to data from (Saad, 1997; El-Bady, 2014; Nawar et al., 2023). In total, the wastewater discharged into the Bahr El-Baqar drain amounts to 2,049,030 m3 day−1 (El-Bady, 2014). Table 1 provides descriptive analysis data for the soil heavy metals: Cr, Fe, Zn, Cd, Pb, As, and Ni that were studied. The total content of chromium varied from 28.61 to 146.45, with an average of 77.29 ± 18.55 mg kg−1. The average content of iron was 19565.15 ± 4751.74 mg kg−1, varying from 6468.17 to 38526.67. The average total zinc concentration was 191.56 ± 112.231 mg kg−1, with a range varying from 51.31 to 931.22. The average total cadmium content is 1.48 ± 2.52 mg kg−1. Pb, As, and Ni total concentrations were 11.47–203.87, 164.933–267.544, and 21.296–82.143 mg kg-1, respectively. Except for Fe, all means of the heavy metals under study were greater than background values (Wedepohl, 1995) (Table 1). All environmental media contain chromium, which is an element Referring to elements and minerals inherently occurring in the Earth’s crust. Although human activity releases significantly bigger amounts of chromium Releasing it into the atmosphere, the flow of continental dust. Is the main natural source (Mohamed et al., 2023). The research area’s typical Fe content is lower than that of the upper earth crust on average (Wedepohl, 1995) (Table 1). Although humans and plants both need zinc, excessive amounts of the metal can be dangerous (Swartjes, 2011). Hence, it is vital to regulate the correct quantity in agricultural soils. It could cause problems with the immune system and digestive tract, among other immediate and detrimental effects. Furthermore, high zinc levels might hinder the absorption of copper, resulting in symptoms of copper deficiency (Swartjes, 2011).
Table 1. Statistics of studies variables and recommended values of heavy metal concentrations for 146 samples.
The widespread use of herbicides, phosphate fertilizers, and sewage sludge has been linked to soil contamination with cadmium (Cd), as discussed in (Khan et al., 2021). Considering that a substantial portion of this cadmium enters the human body via food sources that accumulate it from the soil, it becomes crucial to establish soil protection measures to preserve or enhance the existing conditions. This may include measures like restricting the use of phosphorus fertilizers with high cadmium content to prevent further contamination. While ingestion of dust and soil can also result in Pb exposure, the food chain is the main cause of exposure (Abuzaid et al., 2019). Even at relatively low lead levels, lead (Pb) can cause damage to the brain and nervous system, particularly in youngsters. As a result, a comprehensive assessment of the risk posed by lead in topsoil is required. Clayey soils are expected to contain higher concentrations of arsenic, which is generally believed to have a geological origin. But there’s a lot of anthropogenic arsenic pollution because there are more man-made than natural sources of arsenic released into the environment (ATSDR, 2000). In addition, the primary sources of soil nickel (Ni) contamination stem from industries involved in metal plating, fossil fuel utilization, nickel mining, and electroplating. Besides, these human-induced origins, phosphate-based agricultural fertilizers, atmospheric deposition, inorganic fertilizers, sewage sludge, and various waste materials are used as soil amendments, adding to the potential sources of soil contamination (Wuana and Okieimen, 2011; Mcgrath, 1995; Parth et al., 2011; Senesi et al., 1999; Luo et al., 2009). On the other hand, soil pH plays a crucial role in influencing nutrient availability to plant roots, biological activity under varying soil conditions, and the activity of enzymes (Nicholson et al., 2003; Neina, 2019). The pH contents varied between 6.20 and 8.40. The EC values across the study area exhibited significant variability, with an average of 1.33 ± 2.32 dS m−1, ranging from 0.14 to 8.05 dS m−1. The elevated EC levels observed in certain parts of the study region could be attributed to the high salinity of the groundwater. Arid regions, in particular, are known for typical phenomena such as soil salinization and alteration (Stavi et al., 2021). The long-term process of land salinization and sodification jeopardizes both agricultural crop productivity and environmental sustainability (Singh, 2015; Ivushkin et al., 2019; Jamil et al., 2021; Shrivastava and Kumar 2021).
The variography as represented in Supplementary Table S1 showed that, pH, SOM, and Cd values were all fitted by the Stable model. Six properties were properly mapped by both the exponential and spherical models: Cr, Zn, and As for the spherical, and Fe, Pb, and Ni for the exponential. The nugget-to-sill ratio was employed to evaluate the spatial relationships. Dependency (SDC). If this ratio is less than 0.25, between 0.25 and 0.75, or more than 0.75, the SDC is considered strong, moderate, or weak (Cambardella et al., 1994). The nugget-to-sill ratio of all the models’ parameters ranged from 0 to 0.70. Furthermore, all models exhibited a substantial spatial dependence (SDC) ranging from moderate to strong predictions, according to the data. In Figure 2, it is evident that the northern regions of the study area displayed the most elevated levels of heavy metal concentrations. According to the geographical distribution maps of those metals that were analyzed. These sites are near urban areas, where various forms of pollution affect drainage systems and irrigation channels (Mohamed et al., 2023). For every parameter that was chosen, the MSE values were nearly equal to zero, but the RMSSE values were nearly equal to one. Nevertheless, ASE levels were low in every model that was produced.
Figure 2. (Continued). Ordinary kriging maps of soil properties and heavy metals total concentrations; (A) is spatial distribution of Cr (mg kg-1), (B) is spatial distribution of Fe (mg kg-1), (C) is spatial distribution of Zn (mg kg-1), (D) is spatial distribution of Cd (mg kg-1), (E) is spatial distribution of Pb (mg kg-1), (F) is spatial distribution of As (mg kg-1), (G) is spatial distribution of Ni (mg kg-1), (H) is spatial distribution of pH, (I) is spatial distribution of SOM (%), and (J) is spatial distribution of EC (dS m-1).
There is positive correlation between studied metals. A shared origin could be suggested by the metals’ extremely positive connection (Nazzal et al., 2015). SOM had a negative association with each of the variables, regardless of the strength of the connection. The overall concentrations of Cr, Fe, Cd, and Pb showed strong positive relationships with soil EC. Soil pH had a negative connection with every element (except from Fe and Cd), regardless of the strength of the correlation (Supplementary Table S2).
The soil sample was considered suitable based on a KMO value of 0.59. which was more than 0.5 (Said et al., 2020; Shokr et al., 2022). With 146 observations and 10 variables, a PCA may be produced. Due to their eigenvalues being greater than 1, the first three Principal Components (PCs) were employed by Kaiser’s (Kaiser, 1960) technique, whereas the remaining PCs were disregarded (Supplementary Table S3; Figure 3). According to the findings, 56.45% of the variance can be explained by the first three PCs. The first PC1, responsible for 29.02% of the overall variance, demonstrates more pronounced positive correlations with Cr, Fe, Zn, Pb, As, and Ni, as indicated by the factor loadings. In contrast, the second PC2, which accounts for 14.41% of the total variance, is notably linked with pH and EC. Additionally, the PC3, correlated with SOM, contributes to 12.74% of the overall variance. The PCA displays the PC scores of the samples and the variable loadings.
Figure 4 showed, the principal component analysis biplot of F1 (29.02%) and F2 (14.41%), rescaling the loadings plot and score plot to present them together on a single plot. The variables are depicted as arrows in the biplot arrows in the biplot, and the cosine of the angle formed by the arrows between each pair of variables determines the correlation between them. The correlation between variables is stronger when the angle between each pair of arrows is narrower (Smith et al., 2002). A variable like SOM, though, was correlated negatively with other variables and was connected to them at a nearly 180° angle (Suárez et al., 2016).
When compared to other clusters, the observations in this cluster are similar to one another (Jain and Dubes, 1988; Penkova, 2017). In this study, Agglomerative Hierarchical Clustering (AHC) was employed to categorize the data into three distinct clusters (Supplementary Figure S1). The dendrogram shown in Supplementary Figure S1 illustrates the distinctions among the three clusters., with each cluster possessing unique attributes. According to descriptive data presented in Table 2, there are 79 observations in the first cluster, 63 in the second, and 4 in the third. Except for As, the acquired data demonstrated significant differences on all heavy metals between the three clusters. However, there were no appreciable variations in pH, EC, or SOM between the three clusters. Data from soil types affected by heavy metals in El-Minia Governorate, Egypt, were successfully subjected to clustering analysis (Hammam et al., 2022).
Table 3 and Figure 5 illustrate, there were three classifications of pollution in the research region. Most of the investigated region (97.49%) was EHDC, with average concentrations of heavy metals to this degree being 79.23 ± 17.81, 20014.08 ± 4545.91, 201.31 ± 112.97, 1.33 ± 1.37, 40.96 ± 26.36, 211.47 ± 13.96, and46.15 ± 9.72 for Cr, Fe, Zn, Cd, Pb, As, and Ni, respectively. Numerous forms of pollution are brought about by human activity in this field of study, including contamination from irrigation water sources and agricultural management (Mohmed et al., 2023). Moreover, the extremely high degree only occupies 0.46 percent of the whole area.
The results showed that a total of 13 bacterial isolates were successfully obtained from contaminated soil samples as follows: 4 isolates from the soil irrigated with fresh water, 3 from soil irrigated with sewage water, 4 from soil irrigated with artesian water, and 2 from the sediment in-depth sewage (refer to Supplementary Table S4). These isolated bacteria were categorized into nine phenotypes based on their characteristics, such as colony shape, Gram stain, spore formation, presence of capsules, oxygen requirements, and motility. Besides their morphological, biochemical, and physiological traits. The results showed a nine bacterial species were identified as: Enterobacter cloacae, Bacillus cereus, Bacillus thuringeinsis, Pseudomonas aeurginosa, Pseudomonas veronii, Klebsiella pneumonia, E. coli, Staphylococcus aureaus, Staphylococcus saprophyticus.
The findings indicated that a total of 7 fungal isolates were successfully acquired from the contaminated soil samples. These isolates were distributed as follows: 3 isolates from soil irrigated with fresh water, 2 isolates from soil irrigated with sewage water, and 2 isolates from soil irrigated with artesian water (refer to Supplementary Table S4). Based on their morphological and cultural characteristics, the isolated fungi were categorized into three fungal species: Aspergillus fumigatus, Aspergillus versicolor, and Rhizopus oryzae (as shown in Supplementary Figure S2). The results obtained regarding the impact of fungi on soil pollutants align with prior research, confirming a negative correlation between cadmium and the abundance of both bacteria and fungi. Conversely, a positive correlation was observed between fungi and zinc. The influence of soil properties on fungal diversity was also evident. Therefore, it can be concluded that significant variations exist in the soil characteristics within this region. (Alsabhan et al., 2022; Sahu et al., 2023).
The results of actinomycete isolates revealed that a total of 9 isolates were successfully collected from the contaminated soil. These isolates were distributed as follows: 5 isolates from soil irrigated with sewage water, 1 isolate from soil irrigated with artesian water, and 3 isolates from sediments in deep sewage (refer to Supplementary Table S4). These isolates were categorized into four phenotypes based on characteristics such as colony shape, Gram stain, spore formation, presence of capsules, oxygen requirements, and motility. Additionally, the morphological, biochemical, and physiological characteristics as follow: Streptomyces sp., Actioplanes sp., Sporichthya sp. And Actinomyces sp. The findings indicated that Actioplanes sp. Is efficacious in reducing the levels of various heavy metals, including Cr, Zn, Fe, As, Cd, and Ni. Moreover, incorporating actinomycetes into the system enhances the complexity of the microbial network structure and strengthens the stability of the microecology around the plant’s roots. Additionally, the combined application of soil amendments and actinomycetes can promote plant growth (Hamid et al., 2021; Xu et al., 2023).
Many studies confirm that biological methods are effective in treating pollution. They have demonstrated that microorganisms, including bacteria, fungi, and algae, can remediate environments contaminated with heavy metals (Neboht et al., 2013; Kim et al., 2015). Among all microorganisms isolated in our study, Rhizopus oryzae and Actioplanes sp. showed highest removal for 5 different heavy metal, followed by Enterobacter cloacae and P. veronii showed removal for 4 different heavy metal, while E. coli, Staphylococcus aureaus and Actinomyces sp. Do not have any effect on one of heavy metal bioremediation as illustrated in Supplementary Table S4. Conversely, numerous microbial species, such as bacteria and fungi found in Bacillus, Pseudomonas, Streptomyces, Aspergillus, and Penicillium, possess substantial removal capabilities (Tastan et al., 2010; Dasola et al., 2014; Wierzba- 2015). Escherichia coli K-12 exhibits the broadest capacity for absorbing various metal ions, with its outer membrane capable of absorbing over 30 different types of metal ions (Dermont et al., 2008). Rhizopus can uptake heavy metal ions such as Zn, Cu, Cd, and Pb, among others. This finding is consistent with (Abd-Alla et al., 2012) Thiobacillus can assimilate heavy metal ions, along with inorganic ions like sulfur (S), resulting in the formation of a precipitate when combined with metal ions, which can be subsequently separated from the soil (Leusch et al., 1995).
As presented in Table 4, the highest removal efficiency for heavy metals, such as and Cr, reaching 100%, was achieved by R. oryzae, Pseudomonas aeruginosa, and Bacillus thuringiensis. On the other hand, the lowest removal efficiency, 56%, was observed for the heavy metal Ni, and this was recorded for Aspergillus versicolor. These results demonstrate the remarkable efficacy of Rhizopus oryzae, P. aeruginosa, and Bacillus thuringiensis in removing heavy metals, particularly As and Cr, while highlighting the need for further investigation and optimization when dealing with Ni removal by Aspergillus versicolor (Luna et al., 2016).
Table 4. Determination of the highest heavy metal removal efficiency (%) related to the most potent microorganism.
This study proved that variography Analysis models are a useful tool for forecasting the heavy metals’ spatial distribution maps in the investigated area. Additionally, the fusion of AHC and PCA produced unique classification of the study region into three zones, each with distinct characteristics from one another in terms of heavy metal content and pattern. In addition, we looked at the potential of actinomycetes, fungi, and bacteria as microorganisms to lessen the consequences of different types of soil pollution. To carry out the process, the microorganisms found in the core of the study soil had to be isolated, identified, and classified. The study area was divided into three pollutant categories. The majority of the examined region (97.49%) was extremely high degree of contamination (EHDC). It raises a serious concern for the local ecosystems, every pollution level was higher than the threshold of its average concentration in the surface crust of the earth except for Fe. The separation and identification of microorganisms (fungi, bacteria, and actinomycetes) has been found to have a major impact on the decomposition of environmental contaminants, both organic and inorganic. The soil in the study area contained four phenotypes of actinomycetes, three fungal species, and nine bacterial species, according to the findings. Additionally, the investigation showed that R. oryzae, P. aeruginosa, and Bacillus thuringiensis were able to attain 100% removal efficiency for As and Cr, which is an extraordinarily high level of removal efficiency. In conclusion, defining management zones for soil contamination through the process of mapping soil pollutants, geographical identification, and then identifying the kinds of microorganisms capable of lowering soil pollution levels is a crucial and efficient aspect of precise management.
The original contributions presented in the study are included in the article/Supplementary Material, further inquiries can be directed to the corresponding authors.
EM: Conceptualization, Methodology, Supervision, Visualization, Writing–original draft, Writing–review and editing. MJ: Data curation, Methodology, Software, Writing–original draft, Writing–review and editing. EH: Writing–original draft, Writing–review and editing. AE-A: Writing–original draft, Writing–review and editing. SN: Conceptualization, Writing–original draft, Writing–review and editing. NR: Resources, Validation, Writing–original draft, Writing–review and editing. AS: Writing–original draft, Writing–review and editing. MS: Writing–original draft, Writing–review and editing.
The author(s) declare that financial support was received for the research, authorship, and/or publication of this article. This paper was supported by the RUDN University Strategic Academic Leadership Program.
The authors would like to thank the National Authority for Remote Sensing and Space Science (NARSS) for funding the field survey laboratory analysis and remote sensing work.
The authors declare that the research was conducted in the absence of any commercial or financial relationships that could be construed as a potential conflict of interest.
All claims expressed in this article are solely those of the authors and do not necessarily represent those of their affiliated organizations, or those of the publisher, the editors and the reviewers. Any product that may be evaluated in this article, or claim that may be made by its manufacturer, is not guaranteed or endorsed by the publisher.
The statements, opinions and data contained in all publications are solely those of the individual author(s) and contributor(s) and not of MDPI and/or the editor(s). MDPI and/or the editor(s) disclaim responsibility for any injury to people or property resulting from any ideas, methods, instructions or products referred to in the content.
The Supplementary Material for this article can be found online at: https://www.frontiersin.org/articles/10.3389/fenvs.2024.1381409/full#supplementary-material
Abd-Alla, M. H., Morsy, F. M., El-Enany, A. W. E., and Ohyama, T. (2012). Isolation and characterization of a heavy-metal-resistant isolate of Rhizobium leguminosarum bv. viciae potentially applicable for biosorption of Cd2+ and Co2+. Int. Biodeter. Biodegr. 67, 48–55. doi:10.1016/j.ibiod.2011.10.008
Abdelrahman, M. A. E., Shalaby, A., and Mohamed, E. S. (2019). Comparison of two soil quality indices using two methods based on geographic information system. J. Remote Sens. Space Sci. 22, 127–136. doi:10.1016/j.ejrs.2018.03.001
Abrahim, G., and Parker, R. (2008). Assessment of heavy metal enrichment factors and the degree of contamination in marine sediments from Tamaki Estuary, Auckland, New Zealand. Environ. Monit. Assess. 136, 227–238. doi:10.1007/s10661-007-9678-2
Abu-Elsaoud, A. M., Nafady, N. A., and Abdel-Azeem, A. M. (2017). Arbuscular mycorrhizal strategy for zinc mycoremediation and diminished translocation to shoots and grains in wheat. Plos One 12 (11), e0188220–e0188221. doi:10.1371/journal.pone.0188220
Abuzaid, A. S., Bassouny, M., Jahin, H., and Abdelhafez, A. (2019). Stabilization of lead and copper in a contaminated Typic Torripsament soil using humic substances. Clean. Soil Air Water 47, 1800309. doi:10.1002/clen.201800309
Abuzaid, A. S., Mazrou, Y. S. A., El Baroudy, A. A., Ding, Z., and Shokr, M. S. (2022). Multi-indicator and geospatial based approaches for assessing variation of land quality in arid agroecosystems. Sustainability 14, 5840. doi:10.3390/su14105840
Ahmad, N., and Pandey, P. (2020). Spatio-temporal distribution, ecological risk assessment, and multivariate analysis of heavy metals in bathing district, Punjab, India. Water Air Soil Pollut. 231, 431. doi:10.1007/s11270-020-04767-9
Akhtar, M. S., Chali, B., and Azam, T. (2013). Bioremediation of arsenic and lead by plants and microbes from contaminated soil. Res. Plant Sci. 1 (3), 68–73. doi:10.12691/plant-1-3-4
Alsabhan, A. H., Perveen, K., and Alwadi, A. S. (2022). Heavy metal content and microbial population in the soil of Riyadh Region, Saudi Arabia. J. King Saud University-Science 34 (1), 101671. doi:10.1016/j.jksus.2021.101671
ATSDR (United States Agency for Toxic Substances and Disease Registry) (2000). Toxicological profile for arsenic. Washington, DC, USA: US Department of Health and Human Services, Public Health Service, Agency for Toxic Substances and Disease Registry, 428.
Ayilara, M. S., Adeleke, B. S., Adebajo, M. T., Akinola, S. A., Fayose, C. A., Adeyemi, U. T., et al. (2023). Remediation by enhanced natural attenuation; an environment-friendly remediation approach. Front. Environ. Sci. 11, 1182586. doi:10.3389/fenvs.2023.1182586
Baroudy, A. A. E., Ali, A. M., Mohamed, E. S., Moghanm, F. S., Shokr, M. S., Savin, I., et al. (2020). Modeling land suitability for rice crop using remote sensing and soil quality indicators: the case study of the nile delta. Sustainability 12, 9653. doi:10.3390/su12229653
Cambardella, C. A., Moorman, T. B., Novak, J. M., Parkin, T. B., Karlen, D. L., Turco, R. F., et al. (1994). Field-scale variability of soil properties in central Iowa soils. Soil Sci. Soc. Am. J. 58, 1501–1511. doi:10.2136/sssaj1994.03615995005800050033x
Chabukdhara, M., Gupta, S. K., and Gogoi, M. (2017). “Phytoremediation of heavy metals coupled with generation of bioenergy,” in Algal biofuels. Editors S. Gupta, A. Malik, and F. Bux (Cham.: Springer), 163–188. doi:10.1007/978-3-319-51010-1_9
Chen, L., Beiyuan, J., Hu, W., Zhang, Z., Duan, C., Cui, Q., et al. (2022). Phytoremediation of potentially toxic elements (PTEs) contaminated soils using alfalfa (Medicago sativa L.): a comprehensive review. Chemosphere 293, 133577. doi:10.1016/j.chemosphere.2022.133577
Cheng, Q., Wang, R., Huang, W., Wang, W., and Li, X. (2015). Assessment of heavy metal contamination in the sediments from the yellow river wetland national nature reserve (the sanmenxia section), China. Environ. Sci. Pollut. Res. 22, 8586–8593. doi:10.1007/s11356-014-4041-y
Dad, J. M., and Shafiq, M. U. (2021). Spatial variability and delineation of management zones based on soil micronutrient status in apple orchard soils of Kashmir valley, India. Environ. Monit. Assess. 193, 797. doi:10.1007/s10661-021-09588-9
Dasola, A. M., Adeyemi, A. L., Tunbosun, L. A., Abidemi, O. O., and Razaq, S. O. (2014). Kinetic and equilibrium studies of the heavy metal remediation potential of Helix pomentia. Afr. J. Pure Appl. Chem. 8, 123–133. doi:10.5897/AJPAC2014.0544
Dell'anno, F., Rastelli, E., Buschi, E., Barone, G., Beolchini, F., and Dell'anno, A. (2022). Fungi can be more effective than bacteria for the bioremediation of marine sediments highly contaminated with heavy metals. Microorganisms 10 (5), 993. doi:10.3390/microorganisms10050993
Dermont, G., Bergeron, M., Mercier, G., and Richerlaflèche, M. (2008). Soil washing for metal removal: a review of physical/chemical technologies and field applications. J. Hazard. Mater. 152, 1–31. doi:10.1016/j.jhazmat.2007.10.043
Dubey, P. K., Singh, A., Raghubanshi, A., and Abhilash, P. (2020). Steering the restoration of degraded agroecosystems during the united nations decade on ecosystem restoration. J. Environ. Manag. 280, 111798. doi:10.1016/j.jenvman.2020.111798
El-Bady, M. S. (2014). Spatial distribution of some important heavy metals in the soils south of Manzala Lake in Bahr El-Baqar region, Egypt. Nova J. Eng. Appl. Sci. 3, 1–12. doi:10.4236/gep.2015.36017
El Behairy, R. A., El Baroudy, A. A., Ibrahim, M. M., Mohamed, E. S., Rebouh, N. Y., and Shokr, M. S. (2022). Combination of GIS and multivariate analysis to assess the soil heavy metal contamination in some arid zones. Agronomy 12, 2871. doi:10.3390/agronomy12112871
Fan, S., Wang, X., Lei, J., Ran, Q., Ren, Y., and Zhou, J. (2019). Spatial distribution and source identification of heavy metals in a typical Pb/Zn smelter in an arid area of northwest China. Hum. Ecol. Risk Assess. 25, 1661–1687. doi:10.1080/10807039.2018.1539640
Ferreira, C. S., Seifollahi-Aghmiuni, S., Destouni, G., Ghajarnia, N., and Kalantari, Z. (2022). Soil degradation in the European Mediterranean region: processes, status and consequences. Sci. Total Environ. 805, 150106. doi:10.1016/j.scitotenv.2021.150106
Gautam, K., Sharma, P., Dwivedi, S., Singh, A., Gaur, V. K., Varjani, S., et al. (2023). A review on control and abatement of soil pollution by heavy metals: emphasis on artificial intelligence in recovery of contaminated soil. Environ. Res. 115592, 115592. doi:10.1016/j.envres.2023.115592
Girma, G. (2015). Microbial bioremediation of some heavy metals in soils. Egypt. Acad. J. Biol. Sci. G Microbiol. 6 (1), 147–161. doi:10.21608/eajbsg.2015.16483
Goenster-Jordan, S., Jannoura, R., Jordan, G., Buerkert, A., and Joergensen, R. G. (2018). Spatial variability of soil properties in the floodplain of a river oasis in the Mongolian Altay Mountains. Geoderma 330, 99–106. doi:10.1016/j.geoderma.2018.05.028
Goher, M. E., El-Monem, A. M. A., Abdel-Satar, A. M., Ali, M. H., Hussian, A. E. M., and Napiórkowska-Krzebietke, A. (2016). Biosorption of some toxic metals from aqueous solution using non-living algal cells of Chlorella vulgaris.
Golden, N., Zhang, C., Potito, A., Gibson, P. J., Bargary, N., and Morrison, L. (2019). Use of ordinary cokriging with magnetic susceptibility for mapping lead concentrations in soils of an urban contaminated site. J. Soils Sediments 20, 1357–1370. doi:10.1007/s11368-019-02537-7
Goutam, J., Sharma, J., Singh, R., and Sharma, D. (2021). “Fungal-mediated bioremediation of heavy metal–polluted environment,” in Microbial rejuvenation of polluted environment. Microorganisms for sustainability. Editors D. G. Panpatte, and Y. K. Jhala (Singapore: Springer), 26. doi:10.1007/978-981-15-74559_3
Gozukara, G. (2021). Rapid land use prediction via portable X-ray fluorescence (pXRF) data on the dried lakebed of Avlan Lake in Turkey. Geoderma Reg. 28, e00464. doi:10.1016/j.geodrs.2021.e00464
Gozukara, G., Acar, M., Ozlu, E., Dengiz, O., Hartemink, A. E., and Zhang, Y. (2022). A soil quality index using Vis-NIR and pXRF spectra of a soil profile. Catena 211, 105954. doi:10.1016/j.catena.2021.105954
Gustave, W., Yuan, Z., Liu, F., and Chen, Z. (2021). Mechanisms and challenges of microbial fuel cells for soil heavy metal (loid) s remediation. Sci. Total Environ. 756, 143865. doi:10.1016/j.scitotenv(2020).143865
Håkanson, L. (1980). An ecological risk index for aquatic pollution control: a sedimentological approach. Water Res. 14, 975–1001. doi:10.1016/0043-1354(80)90143-8
Hamid, Y., Tang, L., Hussain, B., Usman, M., Liu, L., Ulhassan, Z., et al. (2021). Sepiolite clay: a review of its applications to immobilize toxic metals in contaminated soils and its implications in soil–plant system. Environ. Technol. Innovation 23, 101598. doi:10.1016/j.eti.2021.101598
Hammam, A. A., Mohamed, W. S., Sayed, S.E.-E., Kucher, D. E., and Mohamed, E. S. (2022). Assessment of soil contamination using GIS and multi-variate analysis: a case study in el-minia governorate, Egypt. Agronomy 12, 1197. doi:10.3390/agronomy12051197
Hassan, A., Pariatamby, A., Ahmed, A., Auta, H. S., and Hamid, F. S. (2019). Enhanced bioremediation of heavy metal contaminated landfill soil using filamentous fungi consortia: a demonstration of bioaugmentation potential. Water Air Soil Pollut. 230, 215. doi:10.1007/s11270-019-4227-5
Hendawy, E., Belal, A. A., Mohamed, E. S., Elfadaly, A., Murgante, B., Aldosari, A. A., et al. (2019). The prediction and assessment of the impacts of soil sealing on agricultural land in the North Nile Delta (Egypt) using satellite data and GIS modeling. Sustainability 11 (17), 4662. doi:10.3390/su11174662
Huang, H., Ye, L., and Zhou, Z. (2020). Sharing earth with all life. High-Risk Pollut. Wastewater 42, 209–210. doi:10.1016/j.pld.2020.08.002
Ivushkin, K., Bartholomeus, H., Bregt, A. K., Pulatov, A., Kempen, B., and De Sousa, L. (2019). Global mapping of soil salinity change. Remote Sens. Environ. 231, 111260. doi:10.1016/j.rse.2019.111260
Jain, A. K., and Dubes, R. C. (1988). Algorithms for clustering data. Hoboken, NJ: Prentice-Hall, Inc.
Jamil, A., Riaz, S., Ashraf, M., and Foolad, M. R. (2021). Gene expression profiling of plants under salt stress. Crit. Rev. Plant Sci. 30, 435–458. doi:10.1080/07352689.2011.605739
Jiang, M., He, L., Niazi, N. K., Wang, H., Gustave, W., Vithanage, M., et al. (2023). Nanobiochar for the remediation of contaminated soil and water: challenges and opportunities. Biochar 5 (1), 2. doi:10.1007/s42773-022-00201-x
Kaiser, H. F. (1960). The application of electronic computers to factor analysis. Educ. Psychol. Meas. 20, 141–151. doi:10.1177/001316446002000116
Khan, S., Naushad, M., Lima, E. C., Zhang, S., Shaheen, S. M., and Rinklebe, J. (2021). Global soil pollution by toxic elements: current status and future perspectives on the risk assessment and remediation strategies—a review. J. Hazard. Mater. 417, 126039. doi:10.1016/j.jhazmat.2021.126039
Kim, I. H., Choi, J. H., Joo, J. O., Kim, Y. K., Choi, J. W., and Oh, B. K. (2015). Development of a microbe-zeolite carrier for the effective elimination of heavy metals from seawater. J. Microbiol. Biotechnol. 25 (9), 1542–1546. doi:10.4014/jmb.1504.04067
Kumar, V., Pandita, S., and Setia, R. (2022). A meta-analysis of potential ecological risk evaluation of heavy metals in sediments and soils. Gondwana Res. 103, 487–501. doi:10.1016/j.gr.2021.10.028
Leusch, A., Holan, Z. R., and Volesky, B. (1995). Biosorption of heavy metals (Cd, Cu, Ni, Pb, Zn) by chemically-reinforced biomass of marine algae. J. Chem. Technol. Biotechnol. 62, 279–288. doi:10.1002/jctb.280620311
Liu, L., Li, W., Song, W., and Guo, M. (2018). Remediation techniques for heavy metal-contaminated soils: principles and applicability. Sci. Total Environ. 633, 206–219. doi:10.1016/j.scitotenv.2018.03.161
Luna, J. M., Rufino, R. D., and Sarubbo, L. A. (2016). Biosurfactant from Candida sphaerica UCP0995 exhibiting heavy metal remediation properties. Process Saf. Environ. Prot. 102, 558–566. doi:10.1016/j.psep.2016.05.010
Luo, L., Ma, Y. B., Zhang, S. Z., Wei, D. P., and Zhu, Y. G. (2009). An inventory of trace element inputs to agricultural soils in China. J. Environ. Manag. 90, 2524–2530. doi:10.1016/j.jenvman.2009.01.011
Mallik, S., Mishra, U., and Paul, N. (2020). Groundwater suitability analysis for drinking using GIS-based fuzzy logic. Ecol. Indic. 121, 107179. doi:10.1016/j.ecolind.2020.107179
Mcgrath, S. P. (1995). in Nickel in heavy metals in soils. Editor B. J. Alloway 2nd ed. (London, UK: Blackie Academic and Professional), 371.
Mohamadhasani, F., and Rahimi, M. (2022). Growth response and mycoremediation of heavy by fungus Pleurotus sp. doi:10.1038/s41598-022-24349-5
Mohamed, E. S., Jalhoum, M. E., Belal, A. A., Hendawy, E., Azab, Y. F., Kucher, D. E., et al. (2023). A novel approach for predicting heavy metal contamination based on adaptive neuro-fuzzy inference system and GIS in an arid ecosystem. Agronomy 13 (7), 1873. doi:10.3390/agronomy13071873
Nawar, S., Mohamed, E. S., Essam-Eldeen Sayed, S., Mohamed, W. S., Rebouh, N. Y., and Hammam, A. A. (2023). Estimation of key potentially toxic elements in arid agricultural soils using Vis-NIR spectroscopy with variable selection and PLSR algorithms. Front. Environ. Sci. 11, 1222871. doi:10.3389/fenvs.2023.1222871
Nazzal, Y., Al-Arifi, N., Jafri, M. K., Kishawy, H. A., A Ghrefat, H., M El-Waheidi, M., et al. (2015). Study the urban soils contamination by heavy metals for selected industrial locations in the Greater Toronto area, Canada, using multivariate statistical analysis. Geol. Croat. 68 (2), 147–159. doi:10.4154/gc.2015.10
Neboht, H. A., Ilusanya, O. A., Ezekoye, C. C., and Orji, F. A. (2013). Assessment of IjebuIgbo Abattoir effluent and its impact on the ecology of the receiving soil and river. J. Environ. Sci. Toxicol. Food Technol. 7 (5), 61–67. doi:10.9790/2402-0756167
Neina, D. (2019). The role of soil pH in plant nutrition and soil remediation. Appl. Environ. soil Sci. 2019, 1–9. doi:10.1155/2019/5794869
Nicholson, F. A., Smith, S. R., Alloway, B. J., Carlton-Smith, C., and Chambers, B. J. (2003). An inventory of heavy metals inputs to agricultural soils in England and Wales. Sci. Total Environ. 311, 205–219. doi:10.1016/s0048-9697(03)00139-6
Nowicka, B. (2022). Heavy metal-induced stress in eukaryotic algae mechanisms of heavy metal toxicity and tolerance with particular emphasis on oxidative stress in exposed cells and the role of antioxidant response. Environ. Sci. Pollut. Res. 29, 16860–16911. doi:10.1007/s11356-021-18419-w
Nwaehiri, U. L., Akwukwaegbu, P. I., and Nwoke, B. E. B. (2020). Bacterial remediation of heavy metal polluted soil and effluent from paper mill industry. Environ. Health Toxicol. 35, 1–10. doi:10.5620/eaht.e2020009
Parth, V., Murthy, N. N., and Saxena, P. R. (2011). Assessment of heavy metal contamination in soil around hazardous waste disposal sites in Hyderabad City (India): natural and anthropogenic implications. J. Environ. Res. Manag. 2, 27–34.
Peña, A. (2022). A comprehensive review of recent research concerning the role of low molecular weight organic acids on the fate of organic pollutants in soil. J. Hazard. Mat. 434, 128875. doi:10.1016/j.jhazmat.2022.128875
Penkova, T. (2017). Principal component analysis and cluster analysis for evaluating the natural and anthropogenic territory safety. Procedia Comput. Sci. 112, 99–108. doi:10.1016/j.procs.2017.08.179
Purwanti, I. F., Kurniawan, S. B., Titah, H. S., and Tangahu, B. V. (2018). Identification of acid and aluminium resistant bacteria isolated from aluminum recycling area. Int. J. Civ. Eng. Technol. 9, 945–954.
Purwanti, I. F., Obenu, A., Tangahu, B. V., Kurniawan, S. B., Imron, M. F., and Abdullah, S. R. S. (2020). Bioaugmentation of Vibrio alginolyticus in phytoremediation of aluminium-contaminated soil using Scirpus grossus and Thypa angustifolia. Heliyon 6, e05004. doi:10.1016/j.heliyon.2020.e05004
Saad, A. (1997). Environmental hydrogeologic impacts of ground water withdrawal in the eastern nile delta region with emphasis on ground-water pollution potential. Cairo, Egypt: Ain Shams University. Ph.D. Thesis.
Sahu, V., Mani, D., Singh, B., Mohasin, M., and Verma, J. (2023). Enrichment of Cd, Cr, Pb and biological detoxification strategies in sewage irrigated soils. Int. J. Plant and Soil Sci. 35 (18), 599–609. doi:10.9734/ijpss/2023/v35i183325
Said, M. E. S., Ali, A. M., Borin, M., Abd-Elmabod, S. K., Aldosari, A. A., Khalil, M. M. N., et al. (2020). On the use of multivariate analysis and land evaluation for potential agricultural development of the northwestern coast of Egypt. Agronomy 10, 1318. doi:10.3390/agronomy10091318
Sebei, A., Chaabani, A., Abdelmalek-Babbou, C., Helali, M. A., Dhahri, F., and Chaabani, F. (2020). Evaluation of pollution by heavy metals of an abandoned Pb-Zn mine in northern Tunisia using sequential fractionation and geostatistical mapping. Environ. Sci. Pollut. Res. 27, 43942–43957. doi:10.1007/s11356-020-10101-x
Senesi, G. S., Baldassarre, G., Senesi, N., and Radina, B. (1999). Trace element inputs into soils by anthropogenic activities and implications for human health. Chemosphere 39, 343–377. doi:10.1016/s0045-6535(99)00115-0
Shi, C., and Wang, Y. (2020). Non-parametric machine learning methods for interpolation of spatially varying non-stationary and non-Gaussian geotechnical properties. Geosci. Front. 12, 339–350. doi:10.1016/j.gsf.2020.01.011
Shokr, M. S., Abdellatif, M. A., El Behairy, R. A., Abdelhameed, H. H., El Baroudy, A. A., Mohamed, E. S., et al. (2022). Assessment of potential heavy metal contamination hazards based on GIS and multivariate analysis in some mediterranean zones. Agronomy 12, 3220. doi:10.3390/agronomy12123220
Shrivastava, P., and Kumar, R. (2021). Soil salinity: a serious environmental issue and plant growth promoting bacteria as one of the tools for its alleviation. Saudi J. Biol. Sci. 22, 123–131. doi:10.1016/j.sjbs.2014.12.001
Singh, A. (2015). Soil salinization and waterlogging: a threat to environment and agricultural sustainability. Ecol. Indic. 57, 128–130. doi:10.1016/j.ecolind.2015.04.027
Singh, S. P., and Singh, M. K. (2020). “Soil pollution and human health,” in Plant responses to soil pollution. Editors P. Singh, S. K. Singh, and S. M. Prasad (Singapore: Springer). doi:10.1007/978-981-15-4964-9_13
Smith, A., Cullis, B., and Thompson, R. (2002). “Exploring variety-environment data using random effects AMMI models with adjustments for spatial field trend: Part 1: theory,” in Quantitative genetics, genomics and plant breeding (Wallingford: CABI).
Stavi, I., Thevs, N., and Priori, S. (2021). Soil salinity and sodicity in drylands: a review of causes, effects, monitoring, and restoration measures. Front. Environ. Sci. 330. doi:10.3389/fenvs.2021.712831
Suárez, M. H., Pérez, D. M., Rodríguez-Rodríguez, E. M., Romero, C. D., Borreguero, F. E., and Galindo-Villardón, P. (2016). The compositional HJ-biplot—a new approach to identifying the links among bioactive compounds of tomatoes. Int. J. Mol. Sci. 17, 1828. doi:10.3390/ijms17111828
Swartjes, F. A. (2011). “Introduction to contaminated site management,” in Dealing with contaminated sites (Berlin/Heidelberg, Germany: Springer Science Business Media B.V.).
Tastan, B. E., Ertu˘grul, S., and Dönmez, G. (2010). Effective bioremoval of reactive dye and heavy metals by Aspergillus versicolor. Bioresour. Technol. 101, 870–876. doi:10.1016/j.biortech.2009.08.099
Wang, N., Guan, Q., Sun, Y., Wang, B., Ma, Y., Shao, W., et al. (2021). Predicting the spatial pollution of soil heavy metals by using the distance determination coefficient method. Sci. Total. Environ. 799, 149452. doi:10.1016/j.scitotenv.2021.149452
Wedepohl, K. H. (1995). The composition of the continental crust. Geochimica Cosmochimica Acta 59, 1,217–1,239. doi:10.1016/0016-7037(95)00038-2
Wierzba, S. (2015). Biosorption of lead (II), zinc (II) and nickel (II) from industrial wastewater by Stenotrophomonas maltophilia and Bacillus subtilis. Pol. J. Chem. Technol. 17, 79–87. doi:10.1515/pjct-2015-0012
Wuana, R. A., and Okieimen, F. E. (2011). Heavy metals in contaminated soils: a review of sources, chemistry, risks and best available strategies for remediation. Int. Sch. Res. Not., 402647. doi:10.5402/2011/402647
Xu, T., Xi, J., Ke, J., Wang, Y., Chen, X., Zhang, Z., et al. (2023). Deciphering soil amendments and actinomycetes for remediation of cadmium (Cd) contaminated farmland. Ecotoxicol. Environ. Saf. 249, 114388. doi:10.1016/j.ecoenv.2022.114388
Yang, Z., Yang, F., Liu, J.-L., Wu, H.-T., Yang, H., Shi, Y., et al. (2021). Heavy metal transporters: functional mechanisms, regulation, and application in phytoremediation. Sci. Total. Environ. 809, 151099. doi:10.1016/j.scitotenv.2021.151099
Keywords: wastewater, GIS, PCA, novel bioremediation, Nile Delta
Citation: Mohamed ES, Jalhoum MEM, Hendawy E, El-Adly AM, Nawar S, Rebouh NY, Saleh A and Shokr MS (2024) Geospatial evaluation and bio-remediation of heavy metal-contaminated soils in arid zones. Front. Environ. Sci. 12:1381409. doi: 10.3389/fenvs.2024.1381409
Received: 05 February 2024; Accepted: 02 April 2024;
Published: 16 April 2024.
Edited by:
Khandaker Rayhan Mahbub, Primary Industries and Resources South Australia, AustraliaReviewed by:
Richard K. Omole, Obafemi Awolowo University, NigeriaCopyright © 2024 Mohamed, Jalhoum, Hendawy, El-Adly, Nawar, Rebouh, Saleh and Shokr. This is an open-access article distributed under the terms of the Creative Commons Attribution License (CC BY). The use, distribution or reproduction in other forums is permitted, provided the original author(s) and the copyright owner(s) are credited and that the original publication in this journal is cited, in accordance with accepted academic practice. No use, distribution or reproduction is permitted which does not comply with these terms.
*Correspondence: Elsayed Said Mohamed, c2FsYW1hNTVAbWFpbC5ydQ==
Disclaimer: All claims expressed in this article are solely those of the authors and do not necessarily represent those of their affiliated organizations, or those of the publisher, the editors and the reviewers. Any product that may be evaluated in this article or claim that may be made by its manufacturer is not guaranteed or endorsed by the publisher.
Research integrity at Frontiers
Learn more about the work of our research integrity team to safeguard the quality of each article we publish.