- 1Guangxi-ASEAN Finance and Economics Research Center, Guangxi University of Finance and Economics, Nanning, China
- 2Urban–rural Construction College, Guangxi Vocational University of Agriculture, Nanning, China
- 3Nanchang Institute of Science and Technology, Nanchang, China
- 4International College, Krirk University, Bangkok, Thailand
Digitization is becoming the key to achieving rural revitalization, and there is a complex inter-active relationship with farmer enrichment, and agriculture development, making it highly valued by governments around the world. Quantitatively evaluating the symbiotic relationship between rural digitization, farmer enrichment, and agricultural development, and reveal the driving mechanism behind them, which can provide evidence for the rural government, agricultural enterprises, and relevant stakeholders. This paper conducts an empirical study of 84 digital village national pilots in China based on coupling coordination model and geographically weighted regression, with focus on the spatial characteristics, symbiotic relationship and driving mechanisms of the pilots. The symbiotic relationship between rural digitization, farmer enrichment, and agriculture development has significant heterogeneity, with most of the pilots in coordination state. The driving mechanism of rural digitization, farmer enrichment, agriculture development, and symbiotic relationship were complicated, with significant heterogeneity and synergy in the driving forces of different factors, and special attention should be paid to spatial effects (the nature and intensity of regression coefficients in local regions) in the policy design and implementation. It is worth noting that population aging mainly plays a negative role, while industrialization, government support, resident capacity plays a positive role. The other factors play both positive and negative roles. Income gap between urban and rural residents, population aged 65 and above, financial self-sufficiency are the most critical factors. We suggest developing differentiated management policy, accurate management policy, and integrated management policy, which can provide a basis for digital village and smart village planning, construction and management in China. In view of the significant differences in rural digitalization and its driving mechanisms in different pilots as shown in the study, it is necessary to design differentiated spatial policies according to local conditions, to design accurate management policies based on the driving effects of key single factors, and to design integrated development policies by taking into account the interactive effects of multiple factors.
1 Introduction
Due to the rapid process of informatization, the digitization is gradually covering all areas of economic and social development, driving digitalization to change production, life, and ecology in urban and rural areas in an irreversible way (Maja et al., 2020; Malik et al., 2022). In order to cater to and apply the Internet, big data, cloud computing, artificial intelligence and other new generation of information technology, digital village and smart village, it has become a global consensus to build an intelligent and holographic society with the construction of digital & smart village and smart city as the core (Wang et al., 2022; Bokun and Nazarko, 2023). The United States, the European Union, China, Russia and other major countries in the world have made rural digital development a priority in their digital national strategies in recent years, proposing “Rural Broadband Reconnect Program” (Gao and Wang, 2021), “Smart Countryside Initiative” (Visvizi and Lytras, 2018), “Outline of Digital Rural Development Strategy” (Xing, 2021; Xing et al., 2023), “Russian countryside online projects” (Schmidt, 2011), and the like.
To effectively address the problems and challenges in rural construction and governance, Chinese government has proposed the strategy of “rural revitalization”, with the construction of digital villages as a key starting point (Yang et al., 2023). In 2018, the “Digital Rural Development Strategy” first appeared in the central government’s rural revitalization policies and the National Strategic Planning for Rural Revitalization (2018–2022), followed by the frequent introduction of special policies and plans for rural digital development, including the outline, strategy, planning of digital village and agriculture, highlights of digital village development, and rural digitalization guide. The aforementioned national policies clearly set out the need to promote the synergistic development of rural, agriculture, farmers, for example, “rural digitization in a comprehensive manner to help revitalize the countryside in an all-round way, and comprehensively achieve a strong agriculture, a beautiful countryside, and a rich countryside”. Identifying the core issues and key objectives facing rural areas, agriculture and farmers in the new era to encourage the coupling and interaction of the three and reveal the relationship chain between them is of far-reaching significance to their integrated development and the revitalization of the countryside.
The rapid progress of industrialization, urbanization, and informationization has put agriculture, rural areas, and farmers at risk of marginalization, giving rise to a series of problems such as the abandonment of arable land, the hollowing out of the countryside, the return of farmers to poverty after they have been lifted out of poverty, the aging and feminization of the working population, the decline of vernacular cultures, and the great digital divide between urban and rural areas, all posing serious threats to food security, social stability, and ecological health. Pushing sustainable agricultural development, farmers’ enrichment, and rural digitization will be an effective means to address most of the aforementioned issues, and thus they should be promoted as key drivers of China’s comprehensive rural revitalization (Haefner and Stemberg, 2020; Liu et al., 2021). The decline of agriculture, the prosperity of farmers and the lagging process of rural digitization are closely interlinked with wide-ranging implications. Therefore, in the context of increasing uncertainty about external shocks, it is necessary to apply systems theory to solve the problem in an integrated manner and realize the synergistic effect of “1 + 1>2” (Li H., 2022).
2 Literature review
2.1 Rural digitization
Rural digitization is accompanied by information and digital technology, automation and intelligence in agriculture and rural areas, as well as a profound revolution in the adaptation and application of a new generation of information technology for farmers, agriculture and rural areas (Budziewicz-Guzlecka and Drozdz, 2022; Satola and Milewska, 2022). Therefore, a large number of papers currently focus on the application scenarios and paths of relevant emerging technologies in the process of rural construction, including blockchain, Internet of Things, machine learning, artificial intelligence, and big data technologies, and have developed rural smart transportation, Smart Land, Smart Grid, Smart Metering, Smart Financial, and smart governance, and a variety of other thematic application modules or technical solutions for rural digital development (Irwansyah, 2021; Kasinathan et al., 2022). Next-generation information technology is the basis but not the whole story of rural digitalization, not even the current difficulty. What is more important for most regions is how to raise enough money and resources to support the development of a digital village, leading to two trends in the rural digital practices.
On the one hand, a mass of studies focus on case analysis of digital villages driven by market-based forces, including Smart Tourist Village (Ciolac et al., 2022), Taobao Village (Leong et al., 2016), and special town or industrial village (Li J., 2022). Relying on their tourism resources, transportation location conditions, and advantages of agricultural and sideline products, these regions have developed their commercial and industrial economies better and have formed a unique development pattern (Li W. et al., 2022; Li L. et al., 2022). To boost industrial upgrading, these villages with distinctive resources and products commonly use industry digitization and enterprise digitization as entry points to create smart tourism service systems, intelligent production and manufacturing engineering, and smart supply chain systems, and further drive the digitalization in the whole countryside (Hu, 2022; Rodrigues et al., 2023).
On the other hand, numerous papers focus on digital village case studies driven by administrative forces, such as the government’s increased investment in digital conservation pathways for historical and cultural villages, minority gathering villages, traditional villages, and ancient villages (Huang and Liang, 2020), and the completion of village digital archives and digital/meta-universe village history museums (Qi et al., 2022); and then the government’s increased investment in digital development of villages in areas of soil erosion, rock desertification, desertification, soil degradation, ecological damage, environmental pollution, and severe poverty. They have created ecological, soil and poverty unmanned monitoring and smart governance systems, driving the emergence of smart ecological villages and climate smart village development patterns (Galiwango et al., 2022).
In addition, government policymakers, humanities and sociologists are paying increasing attention to rural digital development policies and planning design, and by the distillation of experiences and policy analysis of typical digital rural and smart rural spatial design solutions in Australia (Randell-Moon and Hynes, 2022), Slovenia (Zavratnik et al., 2018), Poland (Adamowicz, 2021; Wojcik et al., 2021), China (Li and Zhong, 2022) and other countries and regions, they try to propose key technologies for rural digital development policy design and spatial planning (Zhang and Zhang, 2021). The sustainability and spatial imbalance of rural digital development have aroused the attention of scholars. For example, Agusta (2023) established a technical model for assessing the sustainability of rural digital development and carried out an empirical test based on the case study of Hunan Province in China. Ren et al. (2022) and Li et al. (2023) quantitatively measured the degree of differences in rural digital development between different regions in China and the Yellow River Basin using a spatial econometric model, and analyzed their influencing factors.
2.2 Rural, agricultural, farmer integration
Most of the current scholars have studied rural, agricultural, and farmer in isolation and fragmentation, and analyze them professionally in three dimensions. They regard rural, agricultural, and farmer as a complex adaptive dynamic system, and integrate the three into a holistic and comprehensive exploration, which is a new perspective and framework in the field of rural sustainable development research. Most of the papers in the rural, agricultural and farmer integration research area focus on the interactions of the three subsystems, and a small number of scholars have begun to discuss the synergistic relationship between the three subsystems.
In interaction analysis, scholars fix one of the dimensions of rural, agricultural and farmer as the context of the study and then analyze the interaction of the remaining two dimensions. First, they have analyzed the impact of new phenomena, plans and trends in rural construction on agricultural development and farmers’ behavior. Danso-Abbeam (Danso-Abbeam et al., 2020) analyzed the impact of rural economic diversification on farmers’ wellbeing and agricultural technology adoption in Ghana through Propensity Score Matching and Inverse-Probability-weighted Regression Adjustment. Zhang analyzed the characteristics of adaptive changes in agricultural systems in Sichuan, China, in response to regional economic and climate change shocks (Zhang et al., 2015). Wang found that farmers’ willingness to adopt and the condition of information infrastructure are key factors influencing the digitization of farmers and agriculture in rural revitalization (Wang and Dong, 2023). Lin and Landmann further studied that farmer are constrained by the effects of poorly matched knowledge structures, leading to low willingness to apply new media to sell agricultural products and use smartphones to access agricultural knowledge (Landmann et al., 2020; Lin et al., 2022). Second, they have analyzed the impact of agricultural policies on rural development and farmers’ wellbeing. Teka discussed the impact of Ethiopia’s Agricultural Package Programs on farmers’ welfare and concluded that it has boosted the consumption expenditure of the rural population, but produced no significant drive on income and asset growth (Teka and Lee, 2020). Antwi-Agyei analyzed farmers’ acceptance of the Climate smart agriculture action plan and its influencing factors in Ghana through interviews and hierarchical analysis (Antwi-Agyei et al., 2023). Balezentis et al. (2020) analyzed the impact of the EU’s Common Agricultural Policy (CAP) on the application of support systems for youth-oriented farmers and sustainable rural development based on a case study in Lithuania, and Bilewicz et al. (2022) further analyzed the problems that have arisen from the implementation of the CPA in Poland and the main reasons for farmers’ dissatisfaction with it. Aggelopoulos et al. (2012) and Teye and Quarshie (2021) analyzed the impact of the Rural Development Programme of Greece 2007–2013 and agricultural financing on the development of young farmers and the enhancement of farmers’ wellbeing. Third, they have analyzed the impact of new farmer behaviors and organizations on agricultural development and rural construction. Wilmsen et al. (2023) and Ma and Abdulai (2017) analyzed the role played by Farmer Cooperatives in the process of agricultural system reform, economic development, and village construction in China and its limitations. Wang et al. (2020) and Kuntz et al. (2018) analyzed the impact of farmers’ participation in non-agricultural activities on rural land transfer and agricultural land abandonment.
In the synergistic relationship research, scholars have analyzed the level of development of interaction between rural, agricultural, and farmer pairwise combinations by means of coupled coordination degree models, structural equations, and spatial analysis. Liu W. et al. (2022) found that coordination between rural economic development and agro-ecological environment in Guangxi, China, is at a low level, and that the two influence each other, but not in the synchronous progress; on the contrary, Zhu et al. (2021) found that Zhejiang has shifted from an uncoordinated to a coordinated state, suggesting some differences between the regions. Yang et al. (2019) discussed the matching between the supply of farmers and the demand for agricultural production during rural shrinkage, and found that a higher level of intensive management of agriculture further exacerbates the hollow villages and farmland abandonment. Kalantaryan et al. (2021) found that the growth of labor demand for agricultural development in the context of declining farmers in the EU did not help new immigrants living in rural areas to gain greater access to employment opportunities than locals.
2.3 Research gap
Digitization and intelligence, in general, have become the vital forces leading the transformation and innovative development of the countryside and shaping competitive advantages, and have received wide attention from the government, scholars, and the public, especially villagers, and relevant papers are emerging rapidly and in large numbers, creating possibilities for the construction of digital and smart village theories. And it is increasingly accepted by scholars to consider rural, agricultural, and farmer as complex adaptive systems for integrative research, and the analysis of the coupling and coordination relationship among the three is becoming an emerging hot spot. However, as emergencies (rural digitization and smart countryside) and new perspectives (rural, agricultural, farmer integration), they are still in the infancy of research (Rahoveanu et al., 2022; Zhao and Li, 2022) with evident gaps:
First, the current research does not integrate rural digitization with agricultural development, farmer enrichment, and moreover, fails to analyze the formation mechanism of the synergistic relationship among the three. There is a close relationship between the rural digitalization, agricultural development and farmer enrichment, and the synergistic development of the three is the key to the goal of rural revitalization. How to quantitatively measure the relationship between the three and reveal their influencing factors is still left in the basket.
Second, there are obvious defects in the selection of study objects. Scholars mainly focus on case analysis and developed countries, neglecting the overall analysis of all pilots and lacking attention to developing countries. It should be noted that developing countries, including China, Russia, India and other countries, are in a rapid stage of rural digitalization, and the different development environment and conditions make it impossible for them to directly apply the solutions of developed countries (Fennell et al., 2018; Cambra-Fierro and Perez, 2022).
At different stages of development and in different regions, rural, agricultural, and farmers have different goals and challenges. For different types of villages, development objectives are oriented differently. For example, the core of traditional villages lies in the preservation and transmission of history and culture, and the core of tourist villages lies in the development of scenic spots. For the national digital village pilots in China, the central goal of rural development is digitization. The greatest challenge facing agricultural development, as a result of the development of industrialization and urbanization, is to change its marginalized status and, in particular, to avoid recession. China is a developing country, and the increase of farmers’ incomes has been its development goal for a long time, and is also the key to common prosperity and better wellbeing. It is necessary to integrate rural digitization, agricultural development and farmer enrichment in China’s national digital village pilot construction, so as to truly build the countryside into a beautiful home where people can live and work in peace and contentment, forge agriculture into a promising industry, and make farming an attractive profession, to actually accomplish rural revitalization and sustainable development. This paper focuses on the following questions: What are the characteristics of the spatial pattern of the digital village national pilot in China? How to quantitatively evaluate the symbiotic relationship between rural digitization, farmer enrichment, and agricultural development? How to scientifically measure the driving mechanisms of influencing factors? What insights can they provide for policy design and spatial planning?
3 Materials and methods
3.1 Study area
The central government of China have picked out 117 county-level administrative regions in 31 provincial administrative region as national digital rural pilots. Each provincial administrative region is controlled to have 2-6 pilots whose digital village construction has leading advantages or regional characteristics in each province, autonomous region and municipality directly under the central government, and can represent the best level of digital development performance of the villages in their regions. Based on information review and data analysis, the study area of this paper covers 84 pilots, accounting for 71.79% of all. The pilots in Beijing, Tianjin, Shanghai, Shanxi Province, etc., Were excluded as their data and information were not available or incomplete (Figure 1).
National pilots, rather than all villages, were chosen as the study sample for the following reasons. First, the development of digital villages is currently still in its infancy, and national pilots are samples that have been jointly assessed by the local and central governments and found to be at the leading edge of digital development. Among China’s 2,844 county-level administrative regions, the 117 pilots generally have a leading edge in rural digital development, and they have also been uniformly recognized by the Chinese central government, making the analytical results of empirical studies conducted on them more credible and precise. For example, Deqing County, a state-level pilot in Zhejiang Province, recognized by the Central Internet Information Office and the Ministry of Agriculture and Rural Affairs as the nation’s only leading area for the development of digital villages, ranked first in terms of rural digitalization in China, both nationally and in Zhejiang Province. Deqing County started very early in the construction of digital countryside, and has established the overall structure of digital countryside of “1 + 1+N” (1 digital countryside standardization specification + 1 multi-cross synergistic countryside integrated intelligent platform + N agriculture-related scenarios). By leveraging digitalization to drive the transformation of traditional rural production, life, and governance models, Deqing County has iteratively upgraded more than 120 digital applications in rural scenes, with 100% coverage of agricultural informatization, 98.4% installation of broadband, and 100% express delivery to villages. Given the lagging and slow digitization process of non-pilots, mixing them with pilots in the analysis process would create more noise and reduce the accuracy of the results. Second, since the 117 pilots were initiated by the central government at the same time and have the same experimental time, it is more feasible to select them for empirical analysis when the second list of national pilots has not been announced so far. It is worth noting that the central government’s granting of early and distinctive development rights to the pilots has led to inconsistencies in the policies experimented with by the different pilots, but it has no effect on this study. This is because this study is an aggregate analysis of the pilots as a whole, rather than a segmented analysis of the performance of the different policies of each pilot. Segmented analyses require more data and longer periods of time, and the conditions are not yet perfect for such studies.
3.2 Theoretical framework and empirical testing technical route
Rural, as opposed to urban (in pairs), is a concept of community or settlement, representing the geographic space of farmers’ survival and life and the material carrier of agricultural development, and taking up an important position in the urban and rural system. Farmer is an occupational concept that represents those who are engaged in the production, processing, and trading of agricultural products in rural areas. Agricultural is a concept of industry, and as the earliest material production sector engaged in by human beings, it occupies an important fundamental status in the national economic system. Rural is the place of agricultural production and activities, as well as the space where farmers carry out their production and the home where they live. Agricultural is a prerequisite for the emergence, existence and development of farmers and rural areas, and its quantity and quality directly affect the wellbeing of farmers and the environmental quality of rural areas. Farmers are the mainstay of both rural construction and agricultural production activities, playing a leading role in the sustainable development of both.
Therefore, despite different concepts, the three have obvious differences in nature, structure, characteristics, status and roles; however, from the perspective of system theory, they are closely interlinked, mutually reinforcing and affect each other in a complex adaptive system of symbiotic development (Li et al., 2023; Landini et al., 2023). It has become a consensus among scholars that the digitization can significantly promote non-farm employment, and that the development of artificial intelligence and digital technology has increased opportunities for farmers’ entrepreneurship and agricultural innovation, contributing to the sustained growth of farmer income. For the government, enterprises, and relevant stakeholders, a deep understanding of the relationship of rural digitization with agricultural development and farmer enrichment as well as its driving mechanisms and the formulation of targeted integrated management policy are the key to the integrated of the three (Figure 2).
The symbiotic relationship among the three is manifested in the fact that digitization breaks down the information barriers in different areas of agricultural production, accelerates the flow and interaction of information between agriculture, industry, and commerce, bridges the digital divide between different industries, stimulates the development of smart agriculture and e-commerce of agricultural products, and continuously improves the efficiency as well as the degree of modernization and integration of agricultural production (Wang and Tang, 2023). Income from agricultural operations, wage income and property income are the main sources of farmers’ income. By bringing about enhanced rural information infrastructure construction, rural digitalization draws on digital technology to promote the development of smart agriculture, expand agricultural and rural big data application scenarios and improve the digital skills of farmers, promote the modernization of agriculture and rural development with digital technology services, and encourages full use of information, talent, capital, technology and other factors of production to provide an intrinsic driving force for farmers to increase their incomes. Rural digitalization will increase rural operational income by raising the quality and efficiency of agricultural production, increase wage income by enriching and broadening employment channels, and create more property income by optimizing farmers’ asset allocation (Komorowski and Stanny, 2020). Only a continuous increase in farmers’ incomes can ensure that they continue to update their information facilities and agricultural equipment, so that farmer enrichment has a reverse force in promoting rural digitization and agricultural development (Cao et al., 2023). As agriculture is the primary industry in rural areas and the core area of employment for farmers, the promotion of sustainable agricultural development has become an important strategy for the government to raise the income of farmers. Lagging rural information infrastructure and insufficient digital literacy and skills among farmers are major barriers to rural digitalization. In the information age, the government should take the development of smart agriculture as a key starting point for agricultural modernization and apply new-generation information technologies such as the Internet of Things, blockchain, cloud computing and artificial intelligence to the production, distribution and sales of agriculture. Smart agriculture, especially agricultural products e-commerce and online business, promotes the continuous improvement of farmers’ digital literacy and skills, while driving the continuous update of rural information technology facilities, significantly accelerating and expanding the application of new generation information technology in rural areas, and constantly actuating the high-quality development of rural digitalization (Ciruela-Lorenzo et al., 2020).
Empirical testing is divided into four steps: The first step is to collect data from the national digital village pilots, and use GIS software to analyze the spatial pattern characteristics of rural digitization, farmer enrichment, and agricultural development. The second step is to use a coupled coordination model to quantitatively measure the symbiotic relationship between the three, with special attention paid to identifying and warning of imbalanced relationships. The third step is to use a geographically weighted regression model to analyze the driving mechanism. Step four, Step four, apply the analysis results and focus on analyzing the implication of key conclusions and findings for policy design.
3.3 Research methods
3.3.1 Coupling coordination model
This paper uses the coupling coordination degree model to measure the relationship of rural digitization with agricultural development and farmer enrichment to provide theoretical references for the practical departments of digital rural and rural revitalization strategy. In this paper, the rural digitization subsystem is defined as RD (Rural Digitization), the agricultural development subsystem is defined as AD (Agricultural Development), and the farmer income subsystem is defined as FE (Farmer Enrichment). The degree of mutual reinforcement between the three subsystems DV, AD and FE is defined as the coupling degree index (C), and the degree of coordinated development between them is defined as the coupling coordination degree index (D).
3.3.2 Geographically weighted regression
The location-specific regression coefficients in the GWR model are no longer assumed constants obtained using global information, but are estimated from local regressions using information from a subsample of data from neighboring observations, and they (coefficients) vary with the spatial local geographic location. Assuming that
For a long time, the mainstream agricultural economics theories have assumed that objects in the space are uncorrelated and homogeneous, ignoring the existence of spatial utility. The traditional OLS is only an “average” or “full domain” estimation of the parameters, instead of a reflection of the spatial non-stationarity of the parameters in different spaces. When spatial data are used to build econometric models, the complexity, autocorrelation, and variability that rurally digitalization, agricultural development and farmer enrichment data exhibit spatially make it possible for the effects of explanatory variables on them (the explanatory variables) to vary considerably between different regions. Therefore, if it is assumed that regions are spatially heterogeneous and correlated, Geographically Weighted Regression (GWR) is an effective method to solve the problem of spatial non-stationarity, and it offers more accurate analysis than OLS. GWR, as a Spatial Varying - Coefficient Regression Model, is simple in concept and easy to implement.
3.4 Indicator selection and data source
The selection of independent variables should take into account the social needs and county-level economic support capabilities closely related to rural digitization, agricultural development, and farmer enrichment (Chinn and Fairlie, 2007; Zhang et al., 2022). County economic scale and stage effects are the underlying drivers of rural digital, agricultural development, and farmers’ income growth from the perspective of economic development support, while government intervention and residents’ capacity have important impacts on the pilots of digital village at this stage. Based on the findings of related scholars, it is known that
4 Results
4.1 Symbiotic evaluation
4.1.1 Spatial characteristics analysis
The changes in the statistical parameters of digital village index, added value of pri-mary industry and per capita income of farmers show that the maximum, minimum, mean and median values in 2020 were larger than those in 2018, indicating that they were all in positive growth. Besides, their range and standard deviation were progressively wider, indicating a gradual increase in data heterogeneity. For example, for the digital vil-lage index, the maximum value, from 2018 to 2020, increased from 87.32 to 122.08, the minimum value from 21.86 to 22.20, the mean value from 52.51 to 58.42, and the median value from 53.65 to 58.81, indicating that the rural digitization was in a steady progress. Notably, the range of the digital village index expanded from 65.46 to 99.88, and the standard deviation improved from 11.65 to 15.72, further suggesting a gradual increase in variability among the different pilots.
From the results of spatial clustering analysis, the development of digital village national pilot in China was characterized by gradient agglomeration in the east and weak in the west, with agricultural development having a gradient balanced distribution bounded by the Heihe- Tengchong Line (Hu Line), and farmer enrichment in a “π”-shaped band distribution. The high value areas (high and higher) of the digital village index in 2018 were clustered and distributed in the Beijing- Tianjin-Hebei, the Yangtze River and the middle and lower reaches of the Yellow River Basin, and most of the low value areas (low and lower) were clustered in the Northwest, Southwest, and Northeast regions of China. The spatial pattern of the digital village index in 2020 was generally similar to that of 2018, but the geographic coverage of the high value area was further reduced, and pilots in the high and higher category were further clustered in Beijing- Tianjin-Hebei and the Yangtze River Delta. The added value of primary industry featured generally the same spatial pattern in 2018 and 2020, with all high-value areas located on the east side of Hu Line and the low-value areas concentrated on the west side. The high value areas of per capita income of farmers in 2018 and 2020 were distributed in the east coast, along the roads and bridges in the north, and along the rivers in the south, similar to the pattern of China’s urban agglomerations and the network of arterial transportation routes, showing a “π” shape (Figure 3).
4.1.2 Coupling coordination analysis
For the coupling coordination index, the maximum value was 0.81 (Ningbo- Cixi), followed by high values of Lianyungang- Donghai, Maoming- Gaozhou, Huzhou- Deqing, Xuzhou- Fengxian, Hefei- Changfeng, which are greater than 0.7; the minimum value was 0.17 (Guoluo-Maduo), while Linfen- Xixian, Lasa- Qushui, Rikaze- Bailang, Linzhi- Milin, Hainan- Guinan, Hanzhong- Foping, Yanbian-Helong, Xinganmeng- Zhalaiteqi, Aletai- Jimunai, Shangluo- Zhashui, Xining- Huangyuan also had low values, less than 0.4. The highest number of pilots were in the proximity incoordination state at 41.67%; a similar number of pilots were in the coordination and proximity coordination states at around 20%; and the fewest pilots were in the incoordination state at less than less than 15%. Overall, about 65% of the country pilots have realized the coordinated interaction of rural digitalization with farmer enrichment, and agriculture development, with good results in common development of rural areas, agriculture and farmers; notably, Qiqihaer- Yi’an, Suihua- Wangkui, Rikaze- Bailang, Hainan- Guinan, Lingyuan, Siping- Lishu, Liaoyuan- Dongliao and other national pilots are in a state of dysfunction or early warning, which require more interventions to avoid affecting rural revitalization and sustainable development (Figure 3).
4.2 Driving mechanism
4.2.1 Overall analysis
For the digital village index, the AICs (Akaike information criterion) of OLS and GWR were-102.92 and −105.54, with a difference of 2.61, close to 3, indicating that the latter brought a better fit than the former, but the improvement was not significant. For the added value of primary industry, the AICs of OLS and GWR were −121.98 and −119.32, with a difference of 2.66, close to 3, indicating that the latter brought less fit than the former, but the reduction was small. For the per capita income of farmers, the AICs of OLS and GWR were −189.67 and −219.13, with a difference of 29.46, much larger than 3, indicating that the latter brought a better fit than the former and the improvement was significant. For the coupling coordination index, the AICs of OLS and GWR were −147.37 and −152.61, with a difference of 5.24, greater than 3, indicating that the latter brought a better fit than the former and the improvement was significant. In general, GWR regressions outperformed OLS for digital village index, per capita income of farmers and coupling coordination index.
Statistical analysis of the regression coefficients for each of the influencing factors made it possible to determine the nature and strength of their effects as a whole. The positive and negative maximum and minimum values helped to determine the action of each factor. Financial self-sufficiency, income gap between urban and rural residents, population urbanization rate, and household registration location within the province acted positively on the digital village index as their maximum and minimum values were both greater than zero. On the contrary, per capita GDP, the household registration location outside the province, and population aged 65 and above acted negatively. Notably, GDP had a complex influence on the digital village index by acting positively and negatively, as it had a minimum value less than zero and a maximum value greater than zero. The size of the mean and median enabled determination of the force of each factor. Financial self-sufficiency had the strongest influence on the digital village index as a key factor; income gap between urban and rural residents, population urbanization rate, household registration location within the province and population aged 65 and above also had a strong influence, and they were important factors; other factors had a weak influence and acted as auxiliary factors (Table 2; Figure 4).
Similarly, GDP, per capita GDP, the household registration location outside the province had a positive influence on the added value of primary industry; financial self-sufficiency, income gap between urban and rural residents, population urbanization rate, household registration location within the province, and population aged 65 and above played a negative role. Population aged 65 and above was a key factor for added value of primary industry, while per capita GDP, financial self-sufficiency, population urbanization rate, the household registration location outside the province were important factors. The other factors played an auxiliary role. Per capita GDP, financial self-sufficiency, and income gap between urban and rural residents acted positively on the per capita income of farmers, while the other factors had complex action mechanisms, all acting both positively and negatively. Income gap between urban and rural residents was the key factors of per capita income of farmers, while GDP, per capita GDP, financial self-sufficiency, and the household registration location outside the province, population aged 65 and above were important factors. The rest factors played an auxiliary role. Per capita GDP, financial self-sufficiency, income gap between urban and rural residents, and the household registration location outside the province acted positively on the coupling coordination index, while population aged 65 and above acted negatively. The rest factors acted both positively and negatively. Income gap between urban and rural residents, and population aged 65 and above were key factors for the coupling coordination index, while GDP, per capita GDP, financial self-sufficiency, and the household registration location outside the province were important factors. The rest factors played an auxiliary role.
4.2.2 Spatial effect
As for the spatial effect of the influence of GDP, first, it had a stronger influence on the digital village index in the east coast region of China, and the weakest influence on the west, especially the Loess Plateau region, generally showing the gradient characteristics of strong in the east and weak in the west, and it played a negative obstacle to all the pilots. Second, it had a stronger influence on the added value of primary industry in the western region and the weakest influence on the northeastern region, forming a west-east-northeast gradient weakening pattern in the whole, while playing a positive driving role for all pilots. Third, the mechanism of its impact on per capita income of farmers was very complex, and it acted negatively in the south and positively in the north with the Yellow River Basin as the boundary, In the south, with the Yunnan- Guizhou Plateau as the center, and Tibet, Sichuan, Qinghai, Chongqing, Hunan, Guangxi, Guangdong, Fujian and Hubei as the periphery, it formed a monocentric circle structure. In the north, it formed a large agglomeration in the Central Plains centered on Shanxi - Hebei - Henan, and a small agglomeration in the northeast centered on Xinganmeng - Zhalaiteqi. Fourth, it had a stronger influence on the western region of the coupling coordination index and the weakest influence on the eastern coast, generally characterized by a decreasing gradient of strong in the west and weak in the east. It is worth noting that it was positive in its effect on the west and negative on the east and northeast (Figure 5).
As for the spatial effect of the influence of per capita GDP, first, its impact on the digital village index was characterized by a monocentric circle structure, with the center lying in the Loess Plateau and the middle reaches of the Yellow River Basin (Gansu, Inner Mongolia, Shanxi and Shaanxi), then the immediate area, and the weakest influence in the periphery, the border, and the northeast, and it exerted a negative blocking effect on all the pilots. Second, it had a stronger influence on the added value of primary industry in the eastern region and the weakest influence on the western region, forming an east-west gradient weakening pattern in the whole, and playing a positive driving role for all pilots. Third, its impact on per capita income of farmers was characterized by a southwest-northeast decreasing gradient pattern, with the center of high impact in the Yunnan- Guizhou Plateau. Fourth, its impact on the coupling coordination index was characterized by a northeast-southwest “V”-shaped pattern, decreasing from northeast to north and central China, and from southwest to south-central China (Figure 6).
As for the spatial effect of the influence of financial self-sufficiency, first, it had a strong influence on the digital village index in northeast, north, and northwest China, but a weak influence on the Beibu Gulf and the Yunnan- Guizhou Plateau, while exerting a positive driving force on all pilots. Second, its impact on the added value of primary industry was characterized by a gradient decreasing from south to north, with a stronger influence on the Pan-Pearl River Delta and the southeast coast, and the weakest influence on the northwest region, while exerting a negative obstacle effect on all the pilots. Third, it had the strongest impact on per capita income of farmers in the northeast and north regions, then in the Pan-Pearl River Delta and the Southeast Coast, and the weakest influence on the western region, while exerting a positive driving force on all pilots. Fourth, its impact on the coupling coordination index was characterized by high in the north and low in the south, high in the northwest and low in the southeast, and it played a positive driving role for all pilots (Figure 7).
As for the spatial effect of the influence of income gap between urban and rural residents, first, its impact on the digital village index was characterized by a decreasing gradient of high in the southwest and low in the northeast, with the center of high influence in the Yunnan- Guizhou Plateau, and the center of low influence covering the entire northeast, while exerting a positive driving force on all the pilots. Second, its impact on the added value of primary industry was exactly the opposite of that on the digital village index, presenting a gradient increasing characteristic of low in the southwest and high in the northeast, with the high influence centered in the Beijing- Tianjin- Hebei and Northeast regions, and it exerted a negative blocking effect on all pilots. Second, its impact on per capita income of farmers was characterized by high in the south and low in the north, lower in the northeast and north China than in the northwest, and it played a positive driving role for all pilots. Fourth, its impact on the coupling coordination index was characterized by a northeast-southwest “inverted V” shaped pattern, with the high-influence area concentrated in the Yellow River Basin, and gradually decreasing to the southwest and northeast, with the latter decaying faster than the former (Figure 8).
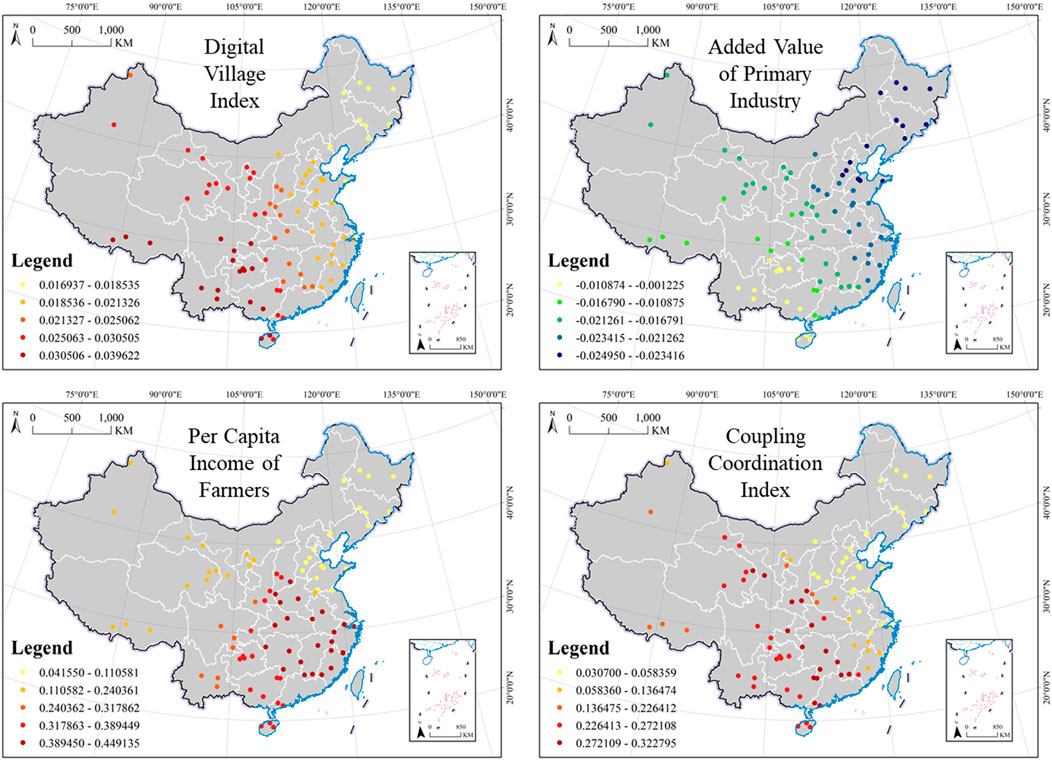
Figure 8. The impact of income gap between urban and rural residents on China’s digital village pilots.
As for the spatial effect of the influence of population urbanization rate, first, its impact on digital village index was characterized by high in the east and low in the west, with the high influence centers in the Beijing- Tianjin- Hebei and Northeast regions, and it played a positive driving effect on all pilots. Second, its impact on the added value of primary industry was largely the same as that on the digital village index in spatial pattern, but with a shift in the nature to a negative barrier. Third, its impact on per capita income of farmers was complex, both positive and negative, and presented the clustering characteristic. Positive high-impact pilots were clustered in the middle reaches of the Yangtze River (Hunan, Hubei, Jiangxi and Fujian), while negative high-impact pilots were in the northeast and northwest. Positive low-impact pilots formed two clusters in Southwest China (Yunnan, Guizhou, Guangxi) and North China (Shaanxi, Shanxi, Hebei), and negative low-impact pilots formed two clusters in the Qinghai- Tibet Plateau and Beibu Gulf. Fourth, its impact on the coupling coordination index was complex, both positive and negative, and characterized by a southwest-northeast “V” shaped pattern. Positive high-impact pilots were clustered in the southwest and northwest, negative high-impact pilots were clustered in the northeast, and negative low-impact pilots were clustered in the Yellow River and middle and lower Yangtze River regions. Of note is that Shaanxi, Hubei, Hunan, and Jiangxi were the transition zones at the bottom of the valley, with the lowest influence, both positive and negative (Figure 9).
As for the spatial effect of the influence of household registration location within the province, first, its impact on the digital village index was characterized by a decreasing northeast-southwest gradient and it played a positive driving role for all pilots. Second, its impact on the added value of primary industry was characterized by significant clustering and it exerted a negative blocking effect on all pilots. It had the strongest influence on the northeast, then in the eastern coast areas; it had the weakest influence on the northwest region, then in the southwest. Third, its impact on per capita income of farmers was very complex, both positive and negative, and presented cluster characteristics. Negative high-impact pilots formed four clusters in the Yunnan- Guizhou Plateau, the lower reaches of the Yangtze River, the Beijing- Tianjin- Hebei regions, and east China, while positive-impact pilots were clustered in the middle reaches of the Yellow River Basin. The upper reaches of the Yellow River Basin and the Pearl River Delta were transitional regions, where the influence of the factor on the pilot shifted from negative to positive (Figure 10).
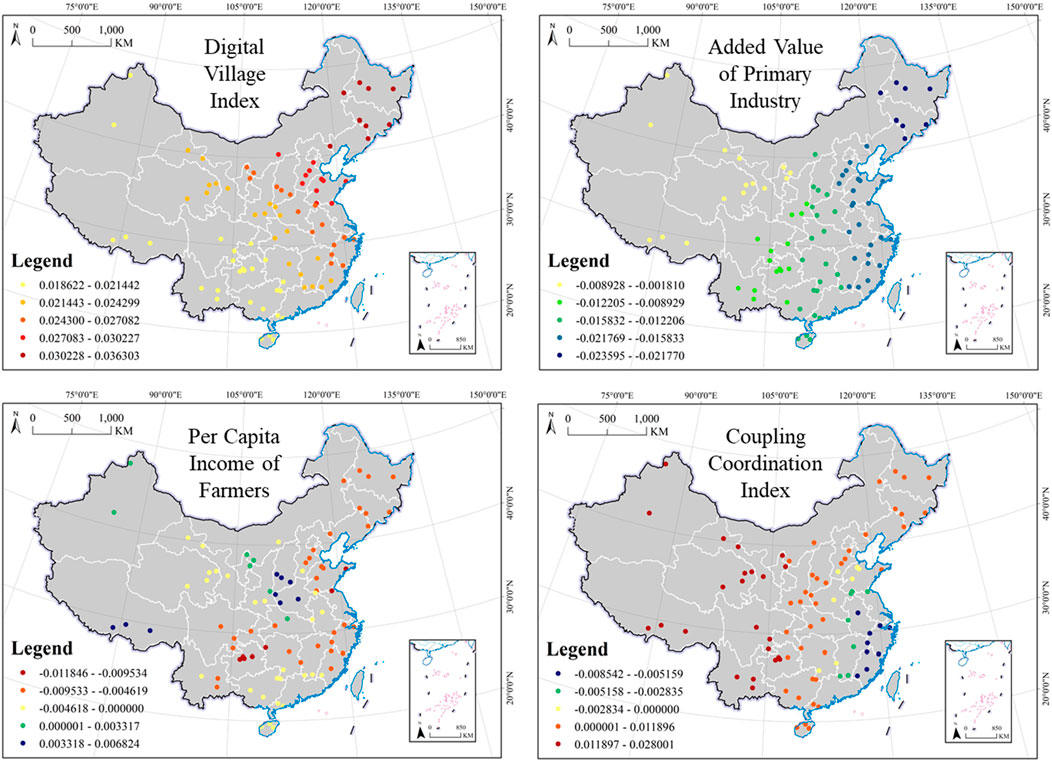
Figure 10. The Impact of Household Registration Location is Within the Province on China’s Digital Village Pilots.
As for the spatial effect of the influence of the household registration location outside the province, first, its impact on the digital village index was characterized by a decreasing southeast-northwest gradient and it played a negative blocking role for all pilots the Hu line as the transition area. Second, it had a strong impact on the added value of primary industry in the vast majority of the pilots, except for the northwest corner, and played a positive driving role in all the pilots. Third, its impact on per capita income of farmers was very complex, both positive and negative, and presented cluster characteristics. Most of the positive high-impact pilots were clustered in the Yunnan- Guizhou Plateau, while most of the negative high-impact pilots were in the southeastern coastal region. The negative low-impact pilots were mostly clustered in the downstream and northeastern regions of the Yellow River, and the areas from the middle and upper reaches of the Yellow River to the west were the transition zone, where the influence of the factor shifted from negative to positive. Fourth, it had the strongest impact on the coupling coordination index in the western region, the weakest impact on the east coast, and the middle impact on the Bohai Bay and northeastern regions, and exerted a positive driving effect on all pilots (Figure 11).
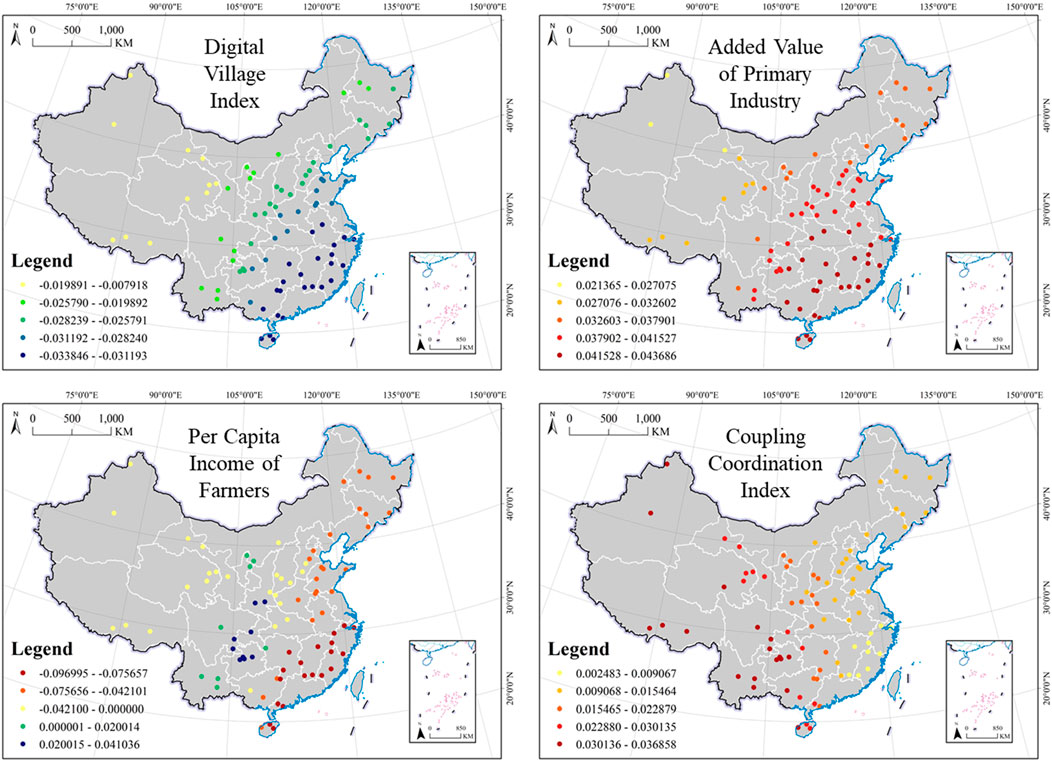
Figure 11. The Impact of The Household Registration Location is Outside the Province on China’s Digital Village Pilots.
As for the spatial effect of the influence of population aged 65 and above, it exerted a negative blocking effect on all dependent variables and formed spatially agglomerated clusters of varying shapes. First, it had the strongest influence on the digital village index in the northwest, and diminished along the Loess Plateau and the Yunnan- Guizhou Plateau to the west and north. Second, it had a stronger impact on the added value of primary industry in all coastal regions from the northeast to the southwest, which decreased to the west and north along the Tibetan and Loess Plateaus, reaching its weakest point in the northwest region. Third, it had the strongest influence on per capita income of farmers in the Yunnan- Guizhou Plateau, a weak influence on the Loess Plateau, Bohai Bay and Northeast China, and the weakest influence on the middle reaches of the Yellow River Basin (Shanxi and Henan). Fourth, its impact on the coupling coordination index was characterized by a “V” shaped pattern in the northeast and southwest, with the strongest influence in the northeast, then in the middle and lower reaches of the Yellow River Basin and the Yangtze River Delta, and the weakest in the Pearl River Delta and the middle reaches of the Yangtze River basin, while elevated again in the Yunnan- Guizhou Plateau and the west (Figure 12).
4.3 Policy implication
4.3.1 Differentiated spatial planning policy
Due to the diverse types of Chinese villages, the differences in resource conditions and capabilities, construction environment of each place determine that the level of rural digitization should be tailored to local conditions, and that the involved villages should adopt development strategies of zoning planning and classification management to actively explore diversified development models and paths. The analysis results in Section 4.1 show that there are significant differences in the pilot’s rural digitization, farmer enrichment, agricultural development, and their symbiotic relationships. Therefore, they should choose a rural revitalization and village construction mode that is compatible with the local development base and matches the local comparative advantages according to the status and needs of pilots in different planning zonings. For the pilots as higher and coordination areas, efforts should be made to maintain their leading edge and give full play to their role of regional leadership and demonstration. They should also be encouraged to actively establish cooperation, exchange and interaction mechanisms, such as “peer-to-peer” partnership/sisterhood support mechanisms, with the pilots in other adjacent zonings to promote the enhancement of rural digitization, farmer enrichment, agriculture development, and their symbiotic relationships in neighboring areas. The pilots as high, medium, proximity incoordination, proximity coordination areas should insist on highlighting the key points and making up for the shortcomings, and actively seek support and investment from the central, provincial and other higher governments to promote rural revitalization and village construction to a higher level. For the pilots as low, lower and Incoordination rural areas, the local governments should stimulate their own positive development capacity with the help of external assistance, especially the transfer payment from the higher-level government and the spillover effect from the neighboring areas.
4.3.2 Accurate development management policy
In response to the driving mechanism, targeted and practical support policies should be designed at two levels: direct impact and spatial effect, to enhance the precision of management policy in pilots (Li and Yang, 2023). On the one hand, the government should highlight the direct driving force of talents and technological innovation, industrial structure, especially commercial and consumer economy, and optimize the talent and industrial policies related to rural digitization and agriculture development; on the other hand, it should pay attention to the interactive effects of new urbanization, population mobility, agricultural modernization and common wealth strategy, and give full play to their roles as the “gas pedal” and “multiplier” for rural digitization development and coupling coordination relationship, so as to activate the endogenous power of digital village construction and smart agriculture development. It is noteworthy that the lost pilots in the western and northeastern regions will need to accelerate the reversal of the unfavorable development situation with the help of exogenous forces and external assistance. The central or higher-level government departments should increase their transfer payments and investment efforts, and establish sister city relationships as soon as possible to achieve the driving and assistance of the strong to the weak. During this process, the superior government pushing forward their digital development through strong government intervention and external assistance, while deciding whether to exclude them from the pilot list based on regular monitoring and evaluation, assessment and supervision. The application and demonstration of digital technology cannot be separated from the guidance and drive of digital talents (Zhao et al., 2022; Zhao and Li, 2022). Therefore, all pilots should invest more energy in the future to explore the establishment of a rural digital talent system, focusing on cultivating local digital talent and guiding urban digital talent to the countryside with the support of higher-level governments.
4.3.3 Integrated construction interaction policy
In the design of policies for coupling digital village construction and agricultural development, it is important to promote the upgrading of the agricultural structure depending on rural digitalization. According to the internal structure of agriculture, the rural digitalization helps to overcome the fragmentation between traditional planting, farming, tourism, and rural cultural industries. It is necessary to mine and analyze the characteristics and interconnections of different industries and products with the help of big data information technology, and to create a new agricultural industry chain through integration and innovation. From the viewpoint of the external structure of agriculture, digital villages and smart villages can enhance the connection and interaction between agriculture, industry and services for the integration of production, supply and marketing of agricultural products. For example, Policies should be introduced to accelerate the application of next-generation information and internet technology in the agricultural production and Management, promote the digital transformation of the whole industrial chain, build a database of agricultural market information, increase data forecasting and analysis, and guide the transformation of rural production from production-oriented to demand-oriented (Salemink et al., 2017). With regard to rural digitalization and the growth of farmer income, digitalization has led to a large number of information technologies “going to the countryside”, which will help farmer income, consumption and entrepreneurship break through technological and spatial constraints. The new infrastructure should be relied on to drive the comprehensive upgrading of rural infrastructure to effectively narrow the urban-rural digital divide (Barbier, 2022). The database should be leveraged to precisely match the quality and diversified consumption demands of towns and cities, so as to reduce the production risks and enhance the premium capacity of agricultural products. Industrial policies, fiscal policies, tax policies, financial policies, and talent policies should be developed to attract all types of “capable” workers to return to their hometowns and efforts should be made to promote the transformation of the digital divide into a digital dividend by carrying out innovative and entrepreneurial activities around the digital development of villages (Tang et al., 2022).
5 Discussion
Most governments around the world are now committed to promoting the planning, construction, and management of digital and smart villages as an important way to reverse rural decline (Adamowicz and Zwolinska-Ligaj, 2020). Therefore, it is an important fundamental task to analyze the dynamics of rural digital development and its driving mechanisms in different regions using scientific methods and econometric models (Zhang et al., 2020). This study found significant spatial heterogeneity and correlation in rural digital development, which gives spatial econometric modeling an advantage over traditional statistical methods to provide more accurate analytical results and conclusions. Past studies often resorted to qualitative or qualitative analysis and tried to propose the characteristics and paths of rural digital development through comprehensive analysis of single or multiple cases, providing technical guidance and experience for other regions. With the rise of the awareness of “evidence-based decision-making” among governments, enterprises and villagers in the era of big data, as well as the establishment of the data collection and statistical system for digital village development, the data and information on rural digital development are becoming more and more abundant, laying a solid foundation for quantitative research. Due to the lack of complexity of single-sample and small-sample empirical or case studies, the results of the analysis are not sufficiently representative and comprehensive. Quantitative analysis based on large samples or even full samples has been a new trend. That is, extracting the common characteristics, development power and evolution law of rural digitalization through the introduction of econometric models and technical methods will provide more accurate technical guidance for the planning, construction and management of digital villages and smart villages (Wang PP. et al., 2023).
The “policy pilots” characterized by “crossing the river by groping the stones” as a governance feature is a highly distinctive Chinese signature of knowledge condensation and discourse expression, and also the key to explaining the “miracle of China”. Pilot units, as explorers of early and pilot implementation, spread advanced experiences to the whole country after knowledge production to demonstrate the achievement of “driving plane by point” and “expanding from point to plane”. Although the current pilot work on rural digitalization is still in its advancement stage, a generalized construction path and practical experience have been explored to provide an option and policy inspiration for other regions. For example, the synergy of the three-dimensional conditions of rural digitization, increased income for farmers and agricultural development shows that the construction of digital villages is a complex task, and that it is difficult to achieve the expected results by sticking to a single dimension. Therefore, importance should be attached to the adaptability and coupling of multiple dimensions from a “holistic perspective”. As the high-quality development of rural digitalization is not driven by a single factor, but rather by the superposition of multiple factors, revealing the “complex causality” behind the construction of digital villages is the basis for policy design. Looking at the overall, the construction of rural digitalization in the pilot areas has made great success, as evidenced by the fact that the foundation for the development of rural informatization has become more solid; the urban-rural “digital divide” is being rapidly bridged; data resources related to agriculture have been shared and interoperated; rural digital application scenarios continue to emerge; the digital economy is playing an increasingly prominent role in promoting common prosperity, and the internal impetus for rural revitalization is growing. The Chinese central government is now planning the second batch of national pilot work on digital villages, and local governments are required to explore models and practices with regional characteristics based on local realities.
To promote the construction of a digital country, rural digitalization is indispensable, which aims to enable smarter industrial development and more scientific rural governance to facilitate rural production and life. The value of national pilots is shown in minimizing the risks associated with the uncertainty of rural digital development in the context of limited investment and resources, and in exploring efficient pathways for digital application and expansion in villages. Specifically, national pilots will provide non-pilot villages with successful experiences that can be built upon for digital development, such as prioritizing the promotion of digital infrastructure and government-led digital transformation of agriculture and rural areas by e-commerce platforms and farmers. In addition, the predicament encountered by national pilots will provide early warning to non-pilot villages. For example, in many pilots, it is believed that the construction of a platform is to be digitized, and the installation of a large screen is to be intelligent. As a result, the mistaken view leads to the blind construction of a digital village map or a rural digital brain platform that is divorced from the local development reality. It should be noted that the rural digitization in some of the national pilots is a very slow process and lags behind, requiring the central government to establish a mechanism for regular evaluation and dynamic management of national pilot projects in digital villages in coming days. Therefore, strengthened summarization is required for distinctive highlights and experience by evaluating the effectiveness of the pilot construction. Local governments should establish provincial, municipal and county-level digital village pilot assessment and recognition mechanisms, set up a national-provincial-municipal-county multi-scale integrated rural digital development pilot system, and include pilot planning, construction and management work in the list of key indicators for government performance assessment.
The study shows a wide variation in coupled coordination between rural digitization, agricultural development and farmer enrichment, with only about 65% of the pilots being in an ideal state to achieve a symbiosis of digital village construction with agricultural development and farmer income. And the symbiosis between the three is affected by the complexity of many factors, with the nature and intensity of the role of the factor varying greatly, and there is a significant spatial effect. Besides, Since rural digitalization is a long-term and difficult systematic project that cannot be accomplished in a short period of time, it is necessary to establish and practice a correct concept of performance, correctly address the chronic problems in the construction, and establish a long-term mechanism to ensure the progression of construction and full-cycle management early. Therefore, in the new round of pilot selection and in the future pilot management process, a differentiated spatial planning policy, an accurate development management policy, and an integrated construction interaction policy should be designed to combine the degree pilot symbiosis and its driving mechanisms for more accurate and practical policy interventions. Besides, for more than 30% of the pilots in a dysfunctional or early warning status, it is necessary to elevate the priority of intervention and policy adjustments by higher levels of government, and to strengthen the investigation and analysis of each pilot to push the update of the test direction and program.
Quantitative measurement of the construction performance of rural digitization and its symbiotic relationship with the farmer enrichment and agricultural development are the basis of relevant policy design, which has received extensive attention from scholars and governments, and is becoming a hot area of development and research on digital villages and smart agriculture. Compared with the available papers, the creative contribution is to analyze the coupling coordination relationship of rural digitalization with farmer enrichment and agricultural development, reveal its driving mechanisms behind them, and further put forward the design direction of differentiated, accurate and integrated policies, which will help the scholars and the government to deepen the understanding of the relationship between the construction of smart villages and the agriculture and the farmers, while providing the basis of the preparation of the related planning for the rural revitalization and village construction. The planning, construction, and management of digital villages and smart villages are a systematic project involving many elements such as rural production, life, ecology, and politics, economy, and culture. Through an empirical study of the development dynamics, coupling relationship, and driving mechanisms of national digital village pilots, this paper provides the important insights for the design of rural digital development policies in China and other similar countries (e.g., developing countries such as India, Egypt, and Iran that are undergoing rapid urbanization and digitization (Venkatesh and Sykes, 2013). As for the research methodology, this paper introduces a geographically weighted regression model and takes into full account the spatial effects of rural digitalization, yielding a more accurate analysis of the driving mechanism. Most of the studies available have explored the success or failure of the pilot construction of digital villages from typical cases, with a focus on the in-depth experience and theoretical generalization of local cases from a single perspective at the “point” level. However, they did not discuss the different paths and regional comparisons in the pilot construction of digital countryside at the “plane” level nationally. This paper bridges this gap with a quantitative analysis based on multiple cases (numbers of samples).
6 Conclusion
With a sample of 84 national digital villages in China, we conducted an empirical study around the symbiotic evaluation, driving mechanism and policy implication, using the coupling coordination model, Geographically Weighted Regression. The findings are as follows. First, there are significant differences between different digital village national pilots in China, making it is necessary to strengthen zoning planning and overall coordination in the management. Second, the coupling coordination relationship of rural digitization with farmer enrichment and agricultural development is characterized by spatial differentiation, with most of the pilots in the coordination state and a small number of pilots in the proximity coordination and incoordination states. Thirdly, the driving mechanism of rural digitization, farmer enrichment, agricultural development, and their symbiotic relationships were complicated, and the driving forces of different factors were heterogeneous, requiring special attention to the spatial effect of multiple measures in policy design and implementation. It is worth noting that population aging (population aged 65 and above) mainly play a negative impact, industrialization (per capita GDP), government support (financial self-sufficiency), resident capacity (income gap between urban and rural residents) mainly plays a positive influence, other factors have a two-sided role, and income gap between urban and rural residents, population aged 65 and above, financial self-sufficiency is the most critical factor. Finally, by applying the analysis results, we suggest developing differentiated spatial planning policy, accurate development management policy, and integrated construction interaction policy, which can provide a basis for rural revitalization and digital/smart village planning, construction and management in China.
There are also some limitations in this study, mainly due to the difficulty of data acquisition. For example, the construction of digital villages often focuses on certain administrative or natural villages and does not cover the whole area of a county, however, since no official data of the relevant villages were available, the analysis was only performed on the county scale in this paper. This study puts forward a symbiotic theoretical framework and empirical techniques for rural digitization, agricultural development and farmer enrichment, quantitatively measures the coupled cooperation between the three and their driving mechanisms, and analyzes their policy implications. But unfortunately, it fails to analyze their interactions in detail and empirically, which will be the direction of future efforts. It should be noted that in addition to China, the methods and findings of this study also have reference for rural digital development in similar regions, including India, America, Iran, Egypt, Europe and others.
Data availability statement
The original contributions presented in the study are included in the article/supplementary material, further inquiries can be directed to the corresponding authors.
Author contributions
ML: Data curation, Investigation, Methodology, Project administration, Resources, Software, Writing–original draft. WL: Data curation, Formal Analysis, Investigation, Methodology, Resources, Software, Visualization, Writing–original draft. ZG: Conceptualization, Formal Analysis, Funding acquisition, Investigation, Resources, Supervision, Validation, Writing–original draft, Writing–review and editing. ZX: Conceptualization, Formal Analysis, Funding acquisition, Project administration, Resources, Supervision, Validation, Writing–original draft, Writing–review and editing.
Funding
The author(s) declare that financial support was received for the research, authorship, and/or publication of this article. This research was funded by Scientific Research Project of Hunan Provincial Department of Edu-cation (21C1087) and this work was funded by a new round first-class disciplines (statistics) of Guangxi.
Acknowledgments
All the authors are grateful to the reviewers and editors.
Conflict of interest
The authors declare that the research was conducted in the absence of any commercial or financial relationships that could be construed as a potential conflict of interest.
The reviewer PZ declared a past co-authorship with the author WL to the handling editor.
Publisher’s note
All claims expressed in this article are solely those of the authors and do not necessarily represent those of their affiliated organizations, or those of the publisher, the editors and the reviewers. Any product that may be evaluated in this article, or claim that may be made by its manufacturer, is not guaranteed or endorsed by the publisher.
References
Adamowicz, M. (2021). The potential for innovative and smart rural development in the peripheral regions of eastern Poland. Ag-riculture-Basel 11 (3), 188. doi:10.3390/agriculture11030188
Adamowicz, M., and Zwolinska-Ligaj, M. (2020). The "smart village" as a way to achieve sustainable development in rural areas of Poland. Sustainability 12 (16), 6503. doi:10.3390/su12166503
Aggelopoulos, S., Pavloudi, A., Tselembis, D., and Soutsas, K. (2012). Financing agricultural holdings: evaluation of the measure 'setting up of young farmers', based on the rural development Programme of Greece 2007-2013. J. Environ. Prot. Ecol. 12 (4), 1985–1995.
Agusta, Y. (2023). Managing the development of a sustainable digital village. Sustainability 15 (9), 7575. doi:10.3390/su15097575
Antwi-Agyei, P., Atta-Aidoo, J., Asare-Nuamah, P., Stringer, L. C., and Antwi, K. (2023). Trade-offs, synergies and acceptability of climate smart agricultural practices by smallholder farmers in rural Ghana. Int. J. Agric. Sustain. 21 (1), 2193439. doi:10.1080/14735903.2023.2193439
Balezentis, T., Ribasauskiene, E., Morkunas, M., Volkov, A., Streimikiene, D., and Toma, P. (2020). Young farmers' support under the common agricultural policy and sustainability of rural regions: evidence from Lithuania. Land Use Policy 94, 104542. doi:10.1016/j.landusepol.2020.104542
Barbier, E. B. (2022). Overcoming digital poverty traps in rural Asia. Rev. Dev. Econ. 27, 1403–1420. doi:10.1111/rode.12962
Bilewicz, A., Mamonova, N., and Burdyka, K. (2022). Paradoxical" dissatisfaction among post-socialist farmers with the EU's common agricultural policy: a study of farmers' subjectivities in rural Poland. East Eur. Polit. Soc. 36 (3), 892–912. doi:10.1177/08883254211036717
Bokun, K., and Nazarko, J. (2023). Smart villages concept - a bibliometric analysis and state-of-the-art literature review. Prog. Plan. 175, 100765. doi:10.1016/j.progress.2023.100765
Budziewicz-Guzlecka, A., and Drozdz, W. (2022). Development and implementation of the smart village concept as a challenge for the modern power industry on the example of Poland. Energies 15 (2), 603. doi:10.3390/en15020603
Cambra-Fierro, J. J., and Perez, L. (2022). (Re)thinking smart in rural contexts: a multi-country study. Growth Change 53 (2), 868–889. doi:10.1111/grow.12612
Cao, X. Z., Yan, M. Y., and Wen, J. (2023). Exploring the level and influencing factors of digital village development in China: insights and recommendations. Sustainability 15 (13), 10423. doi:10.3390/su151310423
Chinn, M. D., and Fairlie, R. W. (2007). The determinants of the global digital divide: a cross-country analysis of computer and internet pen-etration. Oxf. Econ. Papers-New Ser. 59 (1), 16–44. doi:10.1093/oep/gpl024
Ciolac, R., Iancu, T., Popescu, G., Adamov, T., Feher, A., and Stanciu, S. (2022). Smart tourist village-an entrepreneurial necessity for maramures rural area. Sustainability 14 (14), 8914. doi:10.3390/su14148914
Ciruela-Lorenzo, A. M., Del Aguila-Obra, A. R., Padilla-Melendez, A., and Plaza-Angulo, J. J. (2020). Digitalization of agri-cooperatives in the smart agriculture context. Proposal of a digital diagnosis tool. Sustainability 12 (4), 1325. doi:10.3390/su12041325
Danso-Abbeam, G., Dagunga, G., and Ehiakpor, D. S. (2020). Rural non-farm income diversification: implications on smallholder farmers' welfare and agricultural technology adoption in Ghana. Heliyon 6 (11), e05393. doi:10.1016/j.heliyon.2020.e05393
Fennell, S., Kaur, P., Jhunjhunwala, A., Narayanan, D., Loyola, C., Bedi, J., et al. (2018). Examining linkages between smart villages and smart cities: learning from rural youth accessing the internet in India. Telecommun. Policy 42 (10), 810–823. doi:10.1016/j.telpol.2018.06.002
Galiwango, H., Miiro, R. F., Turyahabwe, N., and Egeru, A. (2022). Does the climate-smart village extension model enhance farmer empow-erment? Outlook Agric. 51 (3), 281–292. doi:10.1177/00307270221113600
Gao, F., and Wang, J. (2021). International experience and enlightenment of digital rural construction. Jiangsu Agric. Sci. 49 (23), 1–8. doi:10.15889/j.issn.1002-1302.2021.23.001
Haefner, L., and Sternberg, R. (2020). Spatial implications of digitization: state of the field and research agenda. Geogr. Compass 14 (12), e12544. doi:10.1111/gec3.12544
Hu, X. Q. (2022). Optimization of rural smart tourism service model with internet of things. Secur. Commun. Netw. 2022, 1–10. doi:10.1155/2022/6004728
Huang, Z. M., and Liang, Y. M. (2020). Digital protection and inheritance of ancient villages in southwest minority areas under the strategy of rural revitalization. Technol. Forecast. Soc. Change 160, 120238. doi:10.1016/j.techfore.2020.120238
Irwansyah, I. (2021). The social contractual utilitarianism of a digital village in rural Indonesia. Technol. Soc. 63, 101354. doi:10.1016/j.techsoc.2020.101354
Kalantaryan, S., Scipioni, M., Natale, F., and Alessandrini, A. (2021). Immigration and integration in rural areas and the agricultural sector: an EU perspective. J. Rural Stud. 88, 462–472. doi:10.1016/j.jrurstud.2021.04.017
Kasinathan, P., Pugazhendhi, R., Elavarasan, R. M., Ramachandaramurthy, V. K., Ramanathan, V., Subramanian, S., et al. (2022). Realization of sustainable development goals with disruptive technologies by integrating industry 5.0, society 5.0, smart cities and villages. Sustainability 14 (22), 15258. doi:10.3390/su142215258
Komorowski, L., and Stanny, M. (2020). Smart villages: where can they happen? Land 9 (5), 151. doi:10.3390/land9050151
Kuntz, K. A., Beaudry, F., and Porter, K. L. (2018). Farmers' perceptions of agricultural land abandonment in rural western New York state. Land 7 (4), 128. doi:10.3390/land7040128
Landini, F., Conti, S., and Villalba, A. (2023). Problems in the linkage among agricultural research, rural extension and farmers: an inter-national literature review. Outlook Agric. 52, 382–390. doi:10.1177/00307270231180900
Landmann, D., Lagerkvist, C. J., and Otter, V. (2020). Determinants of small-scale farmers' intention to use smartphones for generating agricultural knowledge in developing countries: evidence from rural India. Eur. J. Dev. Res. 33 (6), 1435–1454. doi:10.1057/s41287-020-00284-x
Leong, C., Pan, S. L., Newell, S., and Cui, L. L. (2016). The emergence of self-organizing E-commerce ecosystems in remote villages of China: a tale of digital empowerment for rural development. MIS Q. 40 (2), 475–484. doi:10.25300/MISQ/2016/40.2.11
Li, H. (2022a). Study on the development model of rural smart tourism based on the background of internet of things. Wirel. Commun. Mob. Comput. 2022, 1–6. doi:10.1155/2022/9688023
Li, H., and Yang, S. G. (2023). The road to common prosperity: can the digital countryside construction increase household income? Sustainability 15 (5), 4020. doi:10.3390/su15054020
Li, J. (2022b). Application of spatial-temporal behavioral trajectory analysis in the space design of digital villages. Wirel. Com-munications Mob. Comput. 2022, 1–10. doi:10.1155/2022/2304820
Li, L., Zhao, K., Wang, X., Zhao, S., Liu, X., and Li, W. (2022b). Spatio-temporal evolution and driving mechanism of urbanization in small cities: case study from Guangxi. Land 11, 415. doi:10.3390/land11030415
Li, W., Zhang, P., Zhao, K., Chen, H., and Zhao, S. (2023). The evolution model of and factors influencing digital villages: evidence from Guangxi, China. Agriculture 13, 659. doi:10.3390/agriculture13030659
Li, W., Zhang, P., Zhao, K., and Zhao, S. (2022a). The geographical distribution and influencing factors of COVID-19 in China. Trop. Med. Infect. Dis. 7, 45. doi:10.3390/tropicalmed7030045
Li, W. Z., and Zhong, H. (2022). Development of a smart tourism integration model to preserve the cultural heritage of ancient villages in Northern Guangxi. Herit. Sci. 10 (1), 91. doi:10.1186/s40494-022-00724-3
Li, Y. L., and Wen, X. (2023). Regional unevenness in the construction of digital villages: a case study of China. Plos One 18 (7), e0287672. doi:10.1371/journal.pone.0287672
Lin, L., Zhou, X., Zhang, S. H., and Fan, J. W. (2022). On the willingness of fujian farmers to sell agricultural products by means of new media from the perspective of rural revitalization: analysis model design based on programmed grounded theory. Sci. Pro-gramming 2022, 1–10. doi:10.1155/2022/3644525
Liu, L. H., Zhang, Y. X., and Bi, Y. M. (2022b). Digital village construction boosts rural revitalization: internal mechanism and empirical test. World Agric. 2022 (08), 51–65. doi:10.13856/j.cn11-1097/s.2022.08.005
Liu, W., Zhou, W., and Lu, L. X. (2022a). An innovative digitization evaluation scheme for spatio-temporal coordination relationship Be-tween multiple knowledge driven rural economic development and agricultural ecological environment- coupling coordi-nation model analysis based on Guangxi. J. Innovation Knowl. 7 (3), 100208. doi:10.1016/j.jik.2022.100208
Liu, Y. S., Zang, Y. Z., and Yang, Y. Y. (2021). China's rural revitalization and development: theory, technology and management. J. Geogr. Sci. 30 (12), 1923–1942. doi:10.1007/s11442-020-1819-3
Ma, W. L., and Abdulai, A. (2017). The economic impacts of agricultural cooperatives on smallholder farmers in rural China. Agribusiness 33 (4), 537–551. doi:10.1002/agr.21522
Maja, P. W., Meyer, J., and Von Solms, S. (2020). Development of smart rural village indicators in line with industry 4.0. IEEE Access 8, 152017–152033. doi:10.1109/ACCESS.2020.3017441
Malik, P. K., Singh, R., Gehlot, A., Akram, S. V., and Das, P. K. (2022). Village 4.0: digitalization of village with smart internet of things tech-nologies. Comput. Industrial Eng. 165, 107938. doi:10.1016/j.cie.2022.107938
Qi, T. J., Ma, L. Q., Zhou, W. H., and Dai, L. X. (2022). Traditional village digital archival conservation: a case study from Gaoqian, China. Archives Records-the J. Archives Rec. Assoc. 44, 202–220. doi:10.1080/23257962.2022.2104236
Rahoveanu, M. M. T., Serban, V., Zugravu, A. G., Rahoveanu, A. T., Cristea, D. S., Nechita, P., et al. (2022). Perspectives on smart villages from a bibliometric approach. Sustainability 14 (17), 10723. doi:10.3390/su141710723
Randell-Moon, H. E. K., and Hynes, D. (2022). Too smart': infrastructuring the Internet through regional and rural smart policy in Australia. Policy Internet 14 (1), 151–169. doi:10.1002/poi3.286
Ren, J. M., Zheng, C. R. Y., Guo, F. Y., Zhao, H. B., Ma, S., and Cheng, Y. (2022). Spatial differentiation of digital rural development and influencing factors in the Yellow River Basin, China. Int. J. Environ. Res. Public Health 19 (23), 16111. doi:10.3390/ijerph192316111
Rodrigues, S., Correia, R., Goncalves, R., Branco, F., and Martins, J. (2023). Digital marketing's impact on rural destinations' image, intention to visit, and destination sustainability. Sustainability 15 (3), 2683. doi:10.3390/su15032683
Salemink, K., Strijker, D., and Bosworth, G. (2017). Rural development in the digital age: a systematic literature review on unequal ICT availability, adoption, and use in rural areas. J. Rural Stud. 54, 360–371. doi:10.1016/j.jrurstud.2015.09.001
Satola, L., and Milewska, A. (2022). The concept of a smart village as an innovative way of implementing public tasks in the era of in-stability on the energy market-examples from Poland. Energies 15 (14), 5175. doi:10.3390/en15145175
Schmidt, H. (2011). Digital’nye derevenščiki/digital villagers: Russian online projects from the countryside. Stud. East Eur. Thought 63 (2), 95–109. doi:10.1007/s11212-011-9137-z
Shrestha, A., and Luo, W. (2017). Analysis of groundwater nitrate contamination in the central valley: comparison of the geodetector method, principal component analysis and geographically weighted regression. ISPRS Int. J. Geo-Information 6 (10), 297. doi:10.3390/ijgi6100297
Tang, G. N., Ren, F., and Zhou, J. (2022). Does the digital economy promote "innovation and entrepreneurship" in rural tourism in China? Front. Psychol. 13, 979027. doi:10.3389/fpsyg.2022.979027
Teka, A., and Lee, S. K. (2020). Do agricultural package programs improve the welfare of rural people? Evidence from smallholder farmers in Ethiopia. Agriculture-Basel 10 (5), 190. doi:10.3390/agriculture10050190
Teye, E. S., and Quarshie, P. T. (2022). Impact of agricultural finance on technology adoption, agricultural productivity and rural household economic wellbeing in Ghana: a case study of rice farmers in shai-osudoku district. South Afr. Geogr. J. 104 (2), 231–250. doi:10.1080/03736245.2021.1962395
Venkatesh, V., and Sykes, T. A. (2013). Digital divide initiative success in developing countries: a longitudinal field study in a village in India. Inf. Syst. Res. 24 (2), 239–260. doi:10.1287/isre.1110.0409
Visvizi, A., and Lytras, M. D. (2018). It's not a fad: smart cities and smart villages research in European and global contexts. Sustainability 10 (8), 2727. doi:10.3390/su10082727
Wang, B., and Dong, H. (2023). Research on the farmers' agricultural digital service use behavior under the rural revitalization strat-egy-based on the extended technology acceptance model. Front. Environ. Sci. 11, 1180072. doi:10.3389/fenvs.2023.1180072
Wang, H., and Tang, Y. T. (2023). Spatiotemporal distribution and influencing factors of coupling coordination between digital village and green and high-quality agricultural development-evidence from China. Sustainability 15 (10), 8079. doi:10.3390/su15108079
Wang, J. Y., Xin, L. J., and Wang, Y. H. (2020). How farmers' non-agricultural employment affects rural land circulation in China? J. Geogr. Sci. 30 (3), 378–400. doi:10.1007/s11442-020-1733-8
Wang, P. P., Li, C. Z., and Huang, C. H. (2023b). The impact of digital village construction on county-level economic growth and its driving mechanisms: evidence from China. Agriculture-Basel 13 (10), 1917. doi:10.3390/agriculture13101917
Wang, Q., Luo, S. X., Zhang, J., and Furuya, K. (2022). Increased attention to smart development in rural areas: a scientometric analysis of smart village research. Land 11 (8), 1362. doi:10.3390/land11081362
Wang, Y., Huang, Y. F., and Zhang, Y. H. (2023a). Coupling and coordinated development of digital economy and rural revitalisation and analysis of influencing factors. Sustainability 15 (4), 3779. doi:10.3390/su15043779
Wilmsen, B., Rogers, S., Duan, Y. F., and Wang, J. H. Z. (2023). Farmer cooperatives and the limits of agricultural reform in rural Hubei. China J. 89, 1–23. doi:10.1086/722258
Wojcik, M., Dmochowska-Dudek, K., and Tobiasz-Lis, P. (2021). Boosting the potential for GeoDesign: digitalisation of the system of spatial planning as a trigger for smart rural development. Energies 14 (13), 3895. doi:10.3390/en14133895
Xing, Z. J. (2021). The national logic of digital rural construction. J. Jishou Univ. Soc. Sci. 42 (06), 58–68. doi:10.13438/j.cnki.jdxb.2021.06.006
Xing, Z. P., Zhao, S. D., and Wang, D. (2023). Performance and sustainability evaluation of rural digitalization and its driving mechanism: evidence from Hunan province of China. Front. Environ. Sci. 11, 1326592. doi:10.3389/fenvs.2023.1326592
Yang, X., Li, W., Zhang, P., Chen, H., Lai, M., and Zhao, S. (2023). The dynamics and driving mechanisms of rural revitalization in western China. Agriculture 13, 1448. doi:10.3390/agriculture13071448
Yang, Y. M. N., Zhang, Y., Yang, Q. S., Liu, J., and Huang, F. (2019). Coupling relationship between agricultural labor and agricultural pro-duction against the background of rural shrinkage: a case study of songnen plain, China. Sustainability 11 (20), 5804. doi:10.3390/su11205804
Zavratnik, V., Kos, A., and Duh, E. S. (2018). Smart villages: comprehensive review of initiatives and practices. Sustainability 10 (7), 2559. doi:10.3390/su10072559
Zhang, H., Du, K. W., and Jin, B. Y. (2020). Research on evaluation of digital rural development readiness under rural revitalization strategy. J. Xi'an Univ. Finance Econ. 33 (01), 51–60.
Zhang, L. J., Tang, Y., and Liu, B. H. (2015). Changes in agricultural system as farmers adapt to economic-social and climatic changes in the min upriver rural areas in western sichuan, southwestern China. J. Mt. Sci. 12 (3), 747–758. doi:10.1007/s11629-014-3386-5
Zhang, P., Chen, H., Zhao, K., Zhao, S., and Li, W. (2022). Dynamics, risk and management performance of urban real estate inventory in Yangtze River Delta. Buildings 12, 2140. doi:10.3390/buildings12122140
Zhang, P., Li, W., Zhao, K., and Zhao, S. (2021). Spatial pattern and driving mechanism of urban–rural income gap in gansu province of China. Land 10, 1002. doi:10.3390/land10101002
Zhang, P., Li, W., Zhao, K., Zhao, Y., Chen, H., and Zhao, S. (2023). The impact factors and management policy of digital village devel-opment: a case study of gansu province, China. Land 12, 616. doi:10.3390/land12030616
Zhang, X. J., and Zhang, Z. G. (2021). How do smart villages become a way to achieve sustainable development in rural areas? Smart village planning and practices in China. Sustainability 12 (24), 10510. doi:10.3390/su122410510
Zhao, S., Yan, Y., and Han, J. (2021). Industrial land change in Chinese silk road cities and its influence on environments. Land 10, 806. doi:10.3390/land10080806
Zhao, W., Liang, Z. Y., and Li, B. R. (2022). Realizing a rural sustainable development through a digital village construction: experiences from China. Sustainability 14 (21), 14199. doi:10.3390/su142114199
Zhao, Y. X., and Li, R. Y. (2022). Coupling and coordination analysis of digital rural construction from the perspective of rural revitali-zation: a case study from Zhejiang province of China. Sustainability 14 (6), 3638. doi:10.3390/su14063638
Keywords: digital village, smart village, influence factor, policy design, China
Citation: Lai M, Li W, Gao Z and Xing Z (2024) Evaluation, mechanism and policy implications of the symbiotic relationship among rural digitization, agricultural development and farmer enrichment: evidence from digital village pilots in China. Front. Environ. Sci. 12:1361633. doi: 10.3389/fenvs.2024.1361633
Received: 26 December 2023; Accepted: 29 April 2024;
Published: 24 May 2024.
Edited by:
Yantuan Yu, Guangdong University of Foreign Studies, ChinaReviewed by:
Ping Zhang, Jiaxing University, ChinaAhmad Sururi, Sultan Ageng Tirtayasa University, Indonesia
Jiamin Ren, Shandong Normal University, China
Huasheng Zeng, Yangzhou University, China
Copyright © 2024 Lai, Li, Gao and Xing. This is an open-access article distributed under the terms of the Creative Commons Attribution License (CC BY). The use, distribution or reproduction in other forums is permitted, provided the original author(s) and the copyright owner(s) are credited and that the original publication in this journal is cited, in accordance with accepted academic practice. No use, distribution or reproduction is permitted which does not comply with these terms.
*Correspondence: Zhenyu Gao, MTM2OTk1Njg2NTFAMTYzLmNvbQ==; Zhipeng Xing, emhpcGVuZzUyMUBidS5hYy5rcg==