- 1Faculty of Economic Sciences, Petroleum-Gas University of Ploiesti, Ploiesti, Romania
- 2Romanian Academy, National Institute for Economic Research “Costin C. Kiriţescu”, Bucharest, Romania
- 3Bucharest University of Economic Studies, Bucharest, Romania
In this study, we leverage sentiment analysis to investigate public perception towards environmental issues as conveyed through global news articles and its potential implications on the transition to a low-carbon economy. Utilizing an extensive corpus of news articles sourced globally, we deploy Natural Language Processing (NLP) techniques to quantify sentiment in these articles, capturing public sentiment’s dynamism and complexity towards various environmental issues. Our methodology involves sentiment scoring of key aspects like “climate change”, “climate policy”, “renewable energy”, “solar energy”, “wind energy”, and “environmental impact” which facilitated a detailed sentiment trend analysis over time. We also incorporated a Latent Dirichlet Allocation (LDA) model to conduct topic modelling, identifying five major topics recurring in the discourse. Our correlation analysis uncovers interesting relationships such as a positive correlation between sentiment scores of “low carbon” and “electric cars”, and a negative correlation between “greenhouse gas emissions” and “electric cars". The findings indicate that public sentiment towards environmental issues is not only multifaceted but also evolving, with significant implications for policy-making and stakeholder engagement in the low-carbon transition. These results exemplify sentiment analysis as a powerful tool in understanding public perception, providing actionable insights for researchers, policymakers, and stakeholders involved in environmental issues and the low-carbon economy transition.
Introduction
Environmental issues, primarily encompassing climate change and the necessity of transitioning to a low-carbon economy, have steadily become central topics in the global narrative. Public perceptions and opinions on these issues significantly influence individual behaviors, as well as broader policy and decision-making processes related to environmental action (Capstick et al., 2015).
The media, as a primary information source for many, plays a substantial role in shaping these perceptions and opinions (Schmidt et al., 2013). The manner in which environmental issues are represented and interpreted through the media can guide public response towards them. However, fully understanding this influence, and the spectrum of sentiment it fosters within the public regarding various environmental topics, requires in-depth investigation.
To contribute to this exploration, our study conducts sentiment analysis on news articles pertaining to environmental issues and the shift towards a low-carbon economy. Our primary research question is: “How are sentiments within news articles on environmental issues and the low-carbon economy transition represented, and how have these sentiments evolved over time?"
Literature review
Sentiment analysis
In the evolving field of sentiment analysis, a variety of scholarly works have provided substantial insights and advancements. The comprehensive study by Liu et al. (2016) serves as a cornerstone in this domain, offering an extensive exploration of sentiment analysis from its fundamental concepts to advanced methodologies. Their work is notable for its application in diverse sectors, encompassing marketing and political science, demonstrating the versatility of sentiment analysis.
Medhat et al. (2014) contribute to the field by summarizing various sentiment analysis algorithms and their applications, particularly highlighting the distinction between machine learning and lexicon-based methods. This comparison is crucial for understanding the diverse approaches within sentiment analysis.
The realm of social media, specifically Twitter, presents unique challenges and opportunities in sentiment analysis. Giachanou and Crestani (2016) address this by focusing on methods tailored for analyzing sentiments in Twitter data. Their work underscores the distinctive aspects of sentiment analysis within social media contexts. Further refining the approach to Twitter sentiment analysis, Tang et al. (2014) introduce a groundbreaking method for learning sentiment-specific word embeddings. This technique proves effective in capturing the nuances of sentiment in the context of Twitter’s textual data, marking a significant advancement in the field.
While not exclusively focused on sentiment analysis, the research by Turney and Pantel (2010) is instrumental in understanding vector space models. These models are fundamental to many sentiment analysis techniques, providing a mathematical and conceptual foundation for the field.
Ligthart et al. (2021) offer a systematic review of sentiment analysis, synthesizing results from various secondary studies. Their comprehensive overview identifies key topics, approaches, features, algorithms, datasets, challenges, and open problems in sentiment analysis, providing a thorough understanding of the field’s current state and future directions. Karmaniolos and Skinner (2019) delve into the foundational technologies of sentiment analysis, tracing the development of modern frameworks and exploring the taxonomy of its parent fields, namely, natural language processing and machine learning. Their literature review offers a historical perspective, tracing related research back to the early 1990s. Nanli et al. (2012) focus on methods for extracting opinions from online reviews, classifying them into positive, negative, or neutral sentiments. Their work is pivotal in understanding the application of sentiment analysis in the context of online consumer feedback. Lastly, Nandwani and Verma (2021) contribute a comprehensive review focusing on sentiment analysis and emotion detection from text. They discuss the various levels of sentiment analysis, the different emotion models, and the intricate process of sentiment analysis and emotion detection from textual data. Their work also sheds light on the challenges encountered in sentiment and emotion analysis, marking an important contribution to the field.
Sentiment analysis and environmental issues
In the field of sentiment analysis, recent studies have increasingly focused on applying these techniques to environmental and climate change topics, demonstrating the versatility and societal relevance of sentiment analysis. Kirilenko and Stepchenkova (2014) made a significant contribution in this arena by applying sentiment analysis to Twitter data concerning climate change. Their work uncovered a range of public sentiments and topics of discussion related to climate change, providing valuable insights into public perception and discourse on this critical global issue.
Expanding the application of sentiment analysis to the realm of public governance, Rocca et al. (2020) explored how local governments utilize social media to disseminate information about their environmental actions, plans, and policies. Their study is unique in that it not only examines the use of social media for environmental communication but also applies sentiment analysis to gauge citizens’ judgments of these environmental disclosures on Facebook. This approach offers a novel perspective on enhancing governmental accountability and organizational legitimacy through the lens of social media engagement.
In a comprehensive systematic review, Okky Ibrohim et al. (2023) delved deep into the tasks, techniques, and benchmarks developed for sentiment analysis specifically in the context of natural environment-related topics. Their analysis spanned seven dimensions: contribution, topical focus, data source and query, annotation, language, details of the task, and the technology or algorithm used. This review stands out for its thorough examination of the application of sentiment analysis in environmental discourse, providing a roadmap for future research in this area. Sham and Mohamed (2022) proposed an innovative climate change sentiment analysis framework that leverages natural language processing techniques to analyze tweets related to climate change. Their research goes beyond mere analysis of sentiments; it also investigates the relationship between the sentiment polarity in tweets and socio-economic indicators of the countries where these tweets originated. This aspect of their study offers a nuanced understanding of how socio-economic factors might influence public sentiment about climate change, reflected through social media.
A groundbreaking study by (Bessec and Fouquau, 2020) utilized textual analysis of 71,785 articles from The Wall Street Journal, revealing a significant impact of environmental sentiment on the stock prices of S&P500 firms, with sector-specific responses. Their findings highlight the financial market’s sensitivity to environmental concerns, suggesting that firms with better environmental performance could potentially leverage this sentiment to enhance their market valuation.
The transition towards post-carbon cities necessitates comprehensive evaluation tools that encompass environmental, social, and economic impacts (Bottero et al., 2021). emphasize the importance of such tools in aiding decision-makers to select sustainable solutions, thus facilitating the low-carbon transition at the urban level.
The intersection of Big Data with environmental management has opened new avenues for research (Su et al., 2020). conducted a bibliometric analysis to explore the application of Big Data in climate change and environmental management, uncovering a burgeoning field of study that spans across various industries and geographical regions.
(El Barachi et al., 2021) introduced a novel sentiment analysis framework employing a Bi-directional LSTM classifier for real-time monitoring of public opinion on climate change. Their method showcases the potential of advanced machine learning techniques in capturing and analyzing public sentiment towards environmental issues efficiently.
Exploring the link between environmental news and the financial performance of green industries (Wang et al., 2021), discovered a negative correlation between media attention to environmental issues and the stock returns of companies in the green sector, mediated by investor sentiment. This indicates the critical role of media in shaping investor perceptions and decisions in the context of environmental sustainability.
(Luxon, 2019) examined how environmental non-governmental organizations (ENGOs) utilize media to mobilize public sentiment on climate change. The study found that positive emotional framing in ENGO press releases effectively influences media coverage, suggesting a strategic avenue for environmental advocacy.
The transition to a low-carbon economy
The transition to a low-carbon economy is a complex and multifaceted challenge that has attracted significant academic attention, resulting in a diverse array of theoretical frameworks, analytical approaches, and policy recommendations. Bergek et al. (2015) contribute a foundational theoretical framework for understanding the dynamics of technological innovation systems within the context of transitioning to a low-carbon economy. Their framework is instrumental in conceptualizing how technological advancements can facilitate this critical shift. Li and Pye (2018) delve into the uncertainties, political dynamics, and technological considerations inherent in the transition to a low-carbon energy future. Their work underscores the necessity of diversified transition pathways, highlighting the complexity and multifaceted nature of this global endeavor. McCauley and Heffron (2018) bring a unique perspective by advocating for the integration of climate, energy, and environmental justice in the transition process. They introduce the concept of “just transition,” emphasizing the importance of fairness and equity in the shift towards a low-carbon economy. Shove and Walker (2010) explore the impact of everyday practices on the transition to a sustainable, low-carbon society. They suggest a bottom-up approach, arguing that the governance of the low-carbon transition should be rooted in the daily behaviors and practices of individuals and communities.
Wang et al. (2022) provide a comprehensive systematic review and bibliometric analysis of research in the low-carbon economy field. Utilizing machine learning methods, they identify five major clusters of research topics, ranging from low-carbon energy policy to carbon emission drivers. Their analysis also sheds light on the influence and effects of low-carbon economic policies on energy futures. Dawson (2021) offers a practical guide for decision-makers on transitioning to a resilient, prosperous, and carbon-free economy. His work covers the necessary technologies, policies, legal structures, financing, and sectoral strategies. Furthermore, Dawson discusses the broader benefits and opportunities of this transition for economies, communities, and the natural environment. Finally, Krogstrup & Oman (2019) examine the macroeconomic and financial policies pertinent to climate change mitigation. They focus on the roles of fiscal, monetary, prudential, and financial market policies, providing a detailed menu of policy tools for mitigation and discussing their interactions and trade-offs.
Methodology
In this section, we outline the methodology employed in our study, serving to expose the methods of data collection, analysis, and processing that were pivotal in our quest to better understand public sentiment around environmental issues and the transition to a low-carbon economy.
Our study operates at the intersection of computational linguistics, media studies, and environmental policy, thereby necessitating a methodological framework that is capable of bridging these diverse fields. At the heart of this approach is the use of sentiment analysis—a field within Natural Language Processing (NLP) that aims to identify and quantify affective states and subjective information within source materials.
The primary sources of our data are news articles. We selected this data due to the significant role of media in shaping and reflecting public opinion. News media, in particular, has a widespread reach, can influence public perception, and plays a crucial role in shaping policy discussions and outcomes. The time period of these articles was carefully chosen to capture recent sentiment and relevant trends.
For the sentiment analysis, we employed a blend of existing tools and custom-developed algorithms to extract, process, and analyze sentiment from the text data in the articles. This process comprised several steps, including data cleaning, text preprocessing, sentiment scoring, and further statistical analysis. The combination of these methods allowed us to generate quantitative sentiment scores, detect trends, and draw insights about public sentiment over time.
Furthermore, we harnessed the power of machine learning for topic modelling to gain a deeper understanding of the recurrent themes within the articles. Specifically, we used the Latent Dirichlet Allocation (LDA) method, a generative statistical model that allows sets of observations to be explained by unobserved groups. Overall, the steps we followed enabled us to gain significant insights into the temporal dynamics of public sentiment concerning the environment and the transition towards a low-carbon economy. In the following subsections, we delve into a more detailed explanation of each step in our methodology (Figure 1).
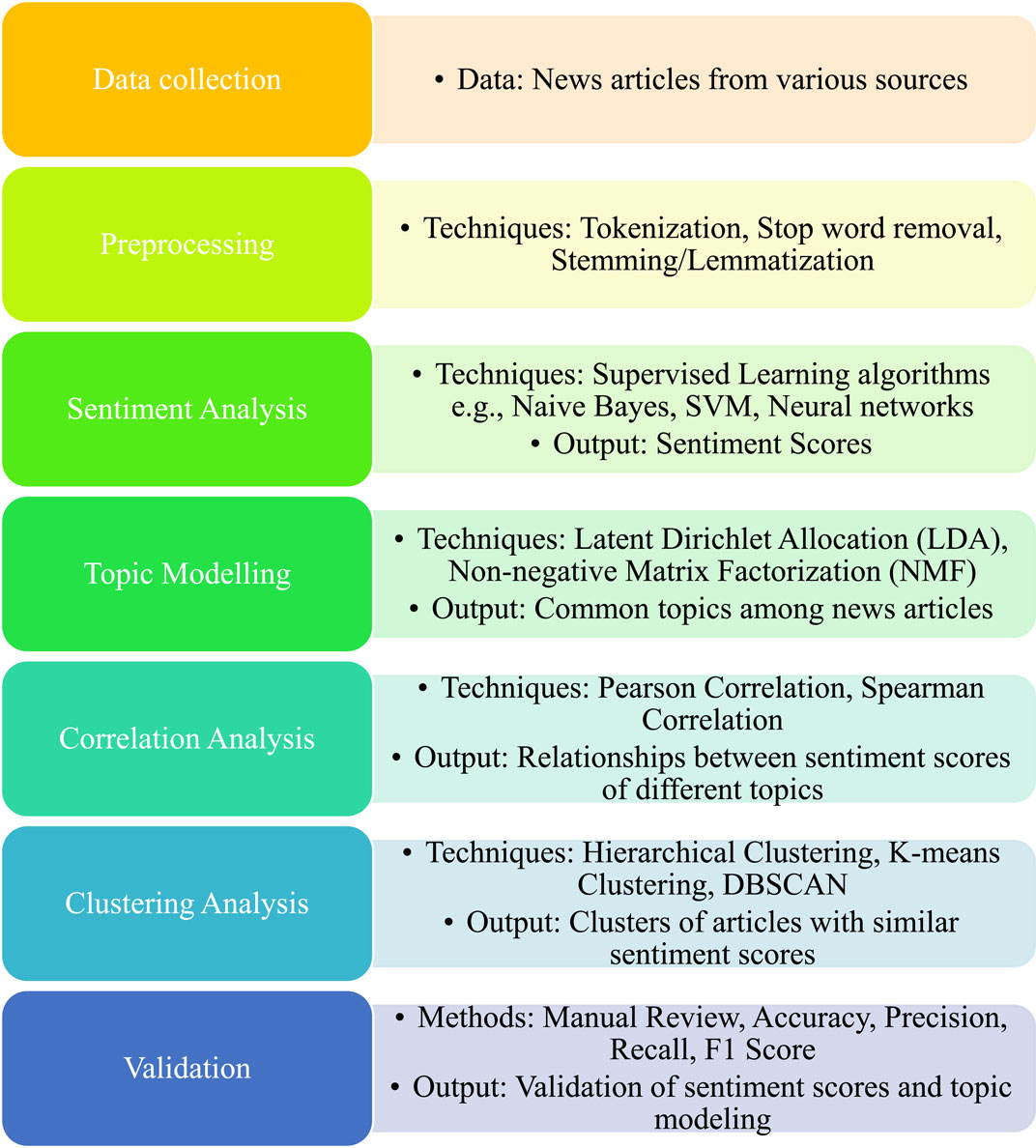
Figure 1. A schematic diagram of the methodology used for this research (figure built by the authors).
Data collection
The data we used for analysis originates from a comprehensive collection of Top 25 World News Headlines (2018–2023): https://www.kaggle.com/datasets/suruchiarora/top-25-world-news-2018-2023?select=WorldNewsData.csv.
This source presents a dataset containing a comprehensive collection of world news headlines from May 2018 to April 2023. The dataset was sourced from Reddit using the PMAW (Python Reddit API Wrapper) API. It covers a wide range of topics, including international news, politics, economics, science, technology, and entertainment. The dataset comprises seven columns, including the date and the top 25 news headlines ranked by importance. The dataset consists of seven columns that provide essential information for each news headline.
The columns include:
• Date: Represents the date of the news headline.
• Top1 to Top25: Includes the top 25 news headlines for each respective date. The headlines are ranked based on their importance, with Top1 being the most significant headline.
The dataset consists of 1,680 rows each with 25 news headlines making a total of 42.000 headlines.
We collected global news articles related to environmental issues.
Data preprocessing
Before conducting the sentiment analysis, we preprocessed the text data to improve the accuracy of the analysis. This involved several steps, including:
Handling noisy data. text cleaning
Our preprocessing begins with an extensive text cleaning process. This involves removing HTML tags, URLs, and non-alphanumeric characters, which are common sources of noise in text data. We also standardize the case by converting all text to lowercase to ensure uniformity.
Handling noisy data. normalization
Beyond basic cleaning, we employ text normalization techniques, including the removal of stop-words (common words that are unlikely to carry significant meaning, such as “and”, “the”, etc.), and the application of lemmatization to reduce words to their base or dictionary form. This step helps in focusing the analysis on meaningful words.
Addressing outliers. statistical analysis for anomaly detection
We conduct a statistical analysis to identify outliers in terms of word frequency and sentiment scores. Words that appear with an unusually high frequency and do not contribute to sentiment or thematic relevance are flagged for review and removed if deemed as outliers.
Addressing outliers. sentiment score distribution analysis
We analyze the distribution of sentiment scores across the dataset to identify and assess potential outliers. Articles with extreme sentiment scores that deviate significantly from the mean are subjected to a manual review to determine if they result from anomalies in data collection or preprocessing.
Tokenization
We broke down the text into individual words or “tokens”. This is a standard step in text analysis and allows us to analyze the frequency and distribution of words in the text.
Stop word removal
We removed common words that do not carry significant meaning, such as “the”, “is”, and “and”. These words are often removed in text analysis to focus on the words that carry more information.
Lemmatization
We reduced words to their base or root form (e.g., “running” to “run”). This helps to consolidate different forms of the same word and reduce the complexity of the analysis. We performed preprocessing and analysis on the “WorldNewsData.csv” dataset related to the low carbon transition. By converting the “Date” column to datetime, setting it as the index, and combining the top news into a single column, you have created a more manageable dataset. Furthermore, we filtered the dataset to focus on environmental issues by selecting rows where the “combined_news” column contains the term ‘environment’ (Figure 2). This allows us to narrow down the dataset specifically to news related to the environment and the low carbon transition.
Sentiment analysis
Sentiment analysis, also known as opinion mining, is a subfield of Natural Language Processing (NLP) that involves determining the sentiment or emotion expressed in a piece of text. The goal is to classify the text into one or more categories: positive, negative, neutral, and sometimes more specific emotions like happy, sad, angry, etc.
There are several approaches to sentiment analysis, including machine learning methods, lexicon-based methods, and hybrid methods.
We used a sentiment analysis model to assign a sentiment score to each news article. The sentiment score is a numerical value that represents the overall sentiment of the text, with higher values indicating more positive sentiment and lower values indicating more negative sentiment. We used the VADER (Hutto et al., 2014) (Valence Aware Dictionary and sEntiment Reasoner) model, which is a lexicon and rule-based sentiment analysis tool specifically attuned to sentiments expressed in social media.
Sentiment analysis model selection and validation
Model Selection: We utilize the VADER sentiment analysis model, chosen for its robustness in handling social media and news text. VADER is particularly adept at understanding the nuances of sentiment expressed in various forms, including slang and emoticons.
Validation Process: To ensure the accuracy and reliability of our sentiment analysis, we validate the model’s performance on a benchmark dataset with manually annotated sentiment labels. This allows us to adjust parameters and refine our approach based on precision, recall, and F1-score metrics.
Ensuring analysis reliability
Contextual Sentiment Analysis: Recognizing the importance of context in sentiment analysis, we implement additional NLP techniques that consider the surrounding text to determine the sentiment of specific words or phrases. This contextual analysis helps in accurately capturing the intended sentiment, especially in complex sentences.
Cross-validation with Human Annotation: For a subset of the dataset, we conduct a cross-validation process involving human annotators. The annotators review the sentiment scores assigned by our model to ensure they align with human judgment. Discrepancies are analyzed and used to fine-tune the model.
Handling noisy data in sentiment analysis
Filtering and Weighting: In the sentiment analysis phase, noisy data that may skew sentiment scores, such as sarcasm or hyperbole, are addressed through filtering and weighting mechanisms. Words and phrases identified as potential sources of noise are weighted less in the final sentiment score calculation.
To gain insights into the sentiment of the news articles, we employed the VADER (Valence Aware Dictionary and sEntiment Reasoner) sentiment analysis tool from the NLTK library. By applying the sentiment analysis to the “combined_news” column, we obtained sentiment scores for each news article.
VADER uses a combination of a sentiment lexicon, which is a list of lexical features (e.g., words, emoticons) weighted by their sentiment intensity, and a set of grammatical and syntactical rules to determine the sentiment of a piece of text. It uses a combination of qualitative and quantitative methods to analyze text data (C.J. Hutto, 2023).
Lexicon: VADER has a lexicon (a dictionary of words) that contains over 7,500 words, emoticons, and other lexical features. Each entry in the lexicon has been manually annotated by human raters with a sentiment intensity, which ranges from −4 (extremely negative) to +4 (extremely positive). For example, the word “good” has a positive sentiment intensity of 1.9, while the word “excellent” has a higher positive sentiment intensity of 2.7.
Sentiment Intensity Scoring: VADER analyzes a piece of text by looking at each word in the text, finding the word in its lexicon, and summing up the sentiment intensity of each word to calculate the total sentiment score of the text. The sentiment score of a text can range from −1 (extremely negative) to +1 (extremely positive).
Incorporating Contextual Information: VADER also takes into account the context in which a word is used. It uses grammatical and syntactical rules to handle things like negations (e.g., “not good” is negative), intensifiers (e.g., “very good” is more positive than “good”), and diminishers (e.g., “kind of good” is less positive than “good”).
The sentiment score assigned by VADER to a text document is computed as follows:
1. Each word in the text is matched with the sentiment lexicon to obtain its sentiment score. The sentiment score of a word is a real number in the range [-4, 4], with negative numbers indicating negative sentiment and positive numbers indicating positive sentiment.
2. The sentiment scores of the words are then summed up to obtain the raw sentiment score of the text.
3. The raw sentiment score is normalized to be in the range [-1, 1] to obtain the final sentiment score of the text. This is the ‘compound’ score in VADER.
The final sentiment score of a text is calculated using the following formula:
where normalize is a function that scales the sum of sentiment scores to be between −1 and +1. This compound score represents the overall sentiment of the text. The normalization is done using the following formula:
where α is a normalization constant (usually set to 15 by default in VADER) to ensure that the compound score is between −1 and 1.
The graph below (Figure 3) illustrates the normalization process. It demonstrates that as the value of x increases, it tends to approach −1 or 1. Similarly, when applying VADER sentiment analysis to documents with a significant number of words, the resulting score tends to be closer to −1 or 1. Consequently, VADER sentiment analysis is most effective for shorter documents such as tweets and sentences, rather than larger documents.
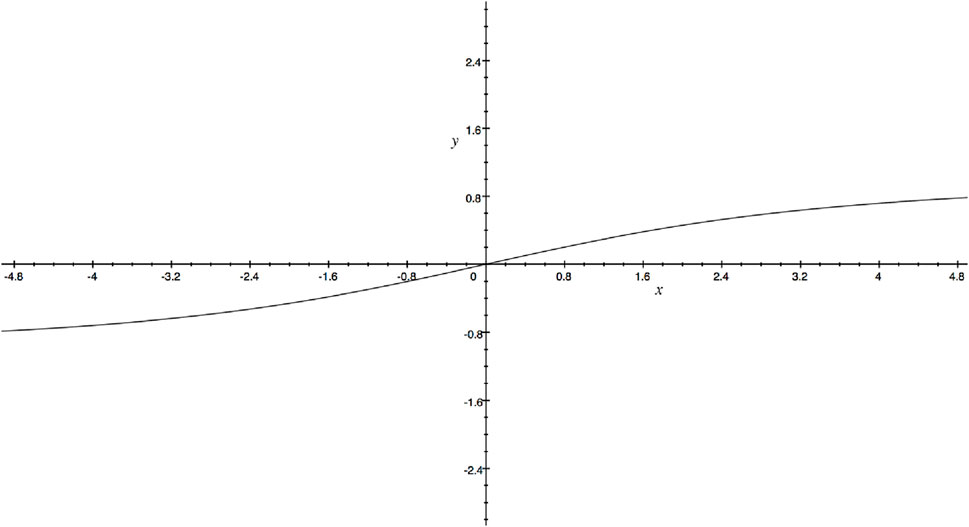
Figure 3. The normalization graph for VANDER (figure acquired from https://medium.com/@piocalderon/vader-sentiment-analysis-explained-f1c4f9101cd9).
VADER also takes into account intensifiers (e.g., ‘very’), diminishers (e.g., ‘kind of’), and negations (e.g., ‘not good’) by adjusting the sentiment scores of the words following these modifiers.
VADER is effective especially for social media text, as it may not capture all nuances and complexities of human sentiment, especially for more complex or specialized texts.
In selecting the VADER (Valence Aware Dictionary and sEntiment Reasoner) lexicon for sentiment analysis within our research, we made a deliberate choice guided by several key factors that align with the objectives and design of our study. This decision is rooted in the specific attributes of VADER that render it particularly suited to our research context, as outlined below, with justification grounded in the relevant literature (Hutto and Gilbert, 2014):
1. Designed for Social Media Text: VADER is explicitly designed to handle the nuances of social media text, including the use of slang, emoticons, capitalization, and punctuation to convey sentiment. Given the diversity of sources in our dataset, including news headlines that often adopt a concise and impactful style akin to social media discourse, VADER’s sensitivity to such linguistic features ensures a more accurate sentiment analysis.
2. Pre-calibrated Lexicon: Unlike many sentiment analysis tools that require extensive calibration to specific datasets or domains, VADER comes pre-calibrated with a lexicon that is optimized for general sentiment analysis across various contexts. This aspect of VADER allows for immediate application to our diverse dataset without the need for time-consuming manual calibration.
3. Rule-based Model with Quantitative Scoring: VADER combines both qualitative and quantitative approaches, utilizing a rule-based model that interprets the intensity of sentiment expressed, thereby providing nuanced sentiment scores ranging from strongly negative to strongly positive. This capability is particularly valuable for our research, as it allows us to capture the subtleties and degrees of sentiment within environmental discourse.
4. Efficiency and Scalability: The efficiency and scalability of VADER make it highly suitable for analyzing large datasets. Its implementation in the Python Natural Language Toolkit (NLTK) allows for seamless integration into our analytical pipeline, enabling the processing of our extensive collection of news articles with minimal computational overhead.
5. Proven Effectiveness in Similar Research Contexts:
Several studies have demonstrated VADER’s effectiveness in sentiment analysis within contexts similar to ours. For instance (Ribeiro et al., 2016), successfully applied VADER in their comparative analysis of sentiments. While other lexicons and sentiment analysis tools exist, such as LIWC (Linguistic Inquiry and Word Count) or SentiWordNet, these alternatives either require extensive customization to the specific dataset or lack the nuanced understanding of internet-specific language forms that VADER offers. Given our dataset’s diversity and the importance of accurately capturing sentiment expressed in modern, concise news language, VADER’s design and capabilities offer a compelling fit for our research objectives.
A comprehensive study by Das et al. (2021) compared seven sentiment analysis tools, including VADER, across four datasets from Twitter, Facebook, Economic Times Headlines, and stock market news articles. The study found VADER to perform exceptionally well, particularly on Facebook data, demonstrating its robustness across different sources of text data (Das et al., 2021). Another research by Dhanalakshmi et al. (2023) compared VADER and logistic regression techniques on a dataset of airline tweets. Despite logistic regression achieving higher accuracy, VADER’s lexicon-based method was recognized for its simplicity and efficiency, particularly when quick, rule-based sentiment analysis is required (Dhanalakshmi et al., 2023).
The adaptability of VADER was further illustrated in studies that modified the tool for languages other than English. A study by Amin et al. (2019) developed Bengali VADER, showcasing the tool’s versatility and its application in sentiment analysis for low-resource languages, providing a significant improvement over existing models (Amin et al., 2019). Additionally, Stanley et al. (2023) explored the use of VADER in analyzing sentiments expressed in tweets about the COVID-19 vaccine, demonstrating its capability in quickly processing and extracting sentiments from social media texts. This study highlighted VADER’s potential in public health research, emphasizing its utility in capturing public sentiment during health crises (Stanley et al., 2023).
After the sentiment analysis has been successfully performed on the cleaned text, we obtain results as in preview table below (Table 1/Figure 4):
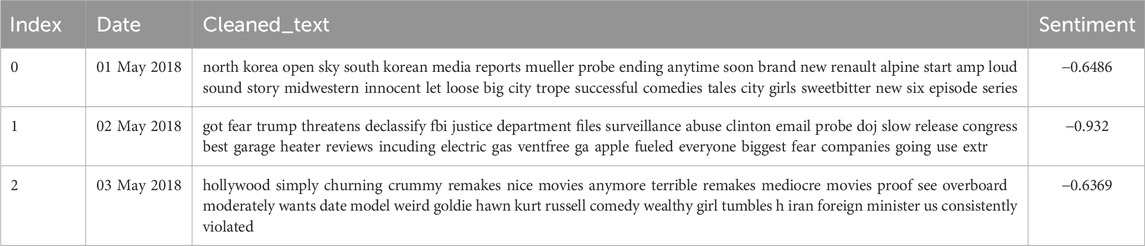
Table 1. Excerpt from the results obtained from the sentiment analysis stage (table built by the authors).
Topic modelling
To identify common themes or topics among the news articles, we used a topic modelling technique called Latent Dirichlet Allocation (LDA). LDA is a probabilistic model that assumes each document is a mixture of a certain number of topics, and each word in the document is attributable to one of the document’s topics.
Topic modelling is a type of statistical modelling for discovering the abstract “topics” that occur in a collection of documents (e.g., in Figure 5). One popular method for topic modelling is Latent Dirichlet Allocation (LDA). LDA is a generative probabilistic model that assumes each document is a mixture of a certain number of topics, and each word in the document is attributable to one of the document’s topics. Here’s a more detailed explanation:
1. Topics: In LDA, a topic is defined as a distribution over a fixed vocabulary. For example, the topic “environment” might consist of words like “climate”, “pollution”, “sustainability”, with each word having a certain probability.
2. Documents: Each document is modelled as a multinomial distribution over topics, and each word in the document is modelled as a multinomial distribution over the words for the chosen topic.
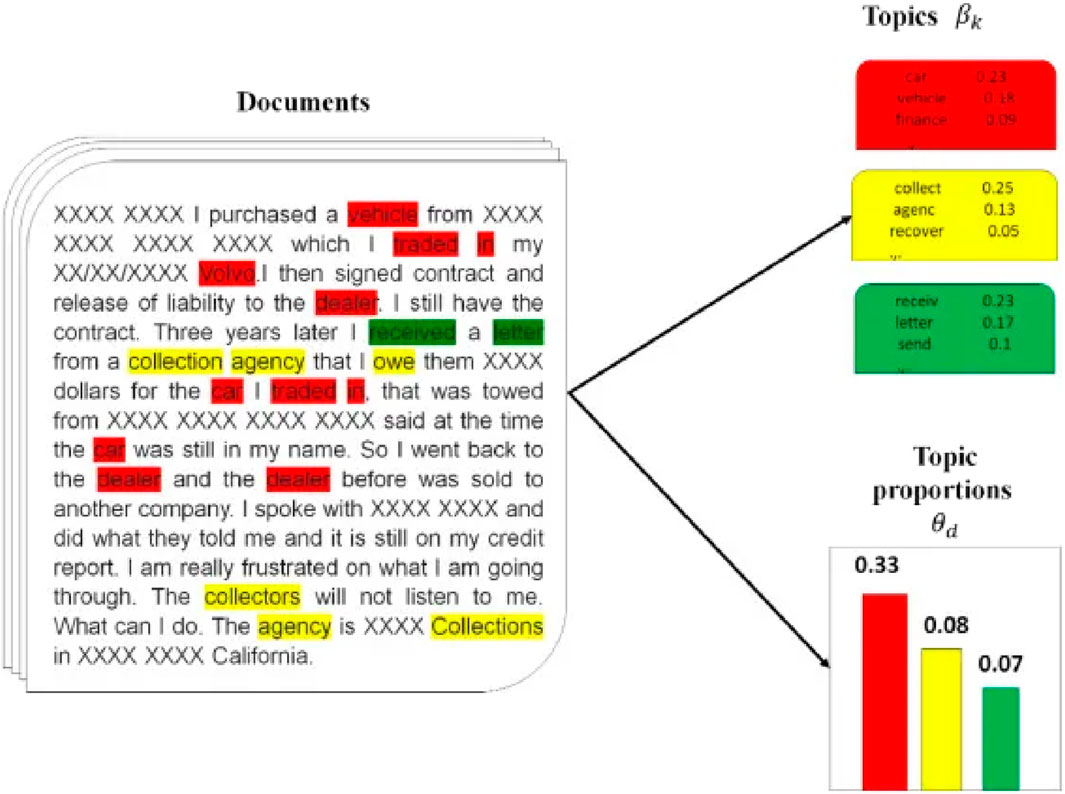
Figure 5. LDA used to detect the topic representation for a document (figure acquired from https://medium.com/analytics-vidhya/latent-dirichelt-allocation-1ec8729589d4).
The goal of LDA is to find the topic distribution that best explains the set of documents in the corpus. This is done by maximizing the following joint probability (Figure 6):
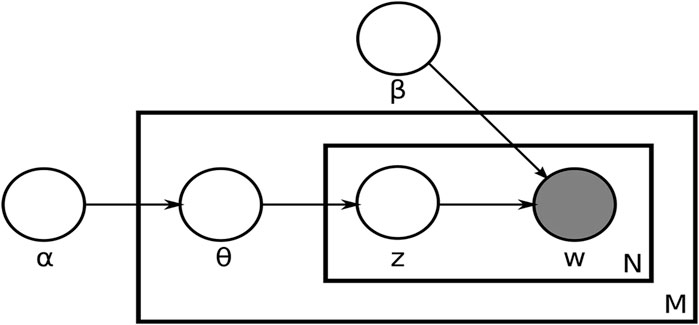
Figure 6. The LDA model (figure acquired from https://medium.com/analytics-vidhya/latent-dirichelt-allocation-1ec8729589d4).
Where:
• M represents the total number of documents.
• N denotes the number of words in a particular document (document “i" has “Ni” words).
• α is a parameter that determines the prior belief about the distribution of topics within each document.
• β is a parameter that determines the prior belief about the distribution of words within each topic.
• θ represents the distribution of topics for a specific document “i."
• φ represents the distribution of words for a particular topic “k."
• “z" represents the topic assignment for the jth word in document “i."
• “w" represents a specific word.
For each word in each document, we calculate the likelihood of it belonging to a specific topic. This likelihood is determined by the proportion of words in the document that are currently assigned to that topic (“P (Topic T | Document D)"). We also calculate the likelihood of a word being generated by a specific topic. This is determined by the proportion of topic assignments across all documents that originate from that word (“P(Word W | Topic T)").
We then reassigned the word to a new topic based on probabilities. We choose a new topic based on the combined probability of “P (Topic T | Document D)" and “P(Word W | Topic T)." This process essentially means that the new topic for word “w" is selected based on how likely that topic generated the word.
Natural language processing (NLP) techniques: suitability
For our study, NLP techniques are particularly suitable due to their ability to process and analyze large volumes of unstructured text data from global news articles. The diverse and dynamic nature of language used in news media to describe environmental issues demands the robust and flexible analytical capabilities provided by NLP. By employing these techniques, we can systematically categorize sentiments expressed in the news, thereby unveiling public perceptions and narratives surrounding environmental issues and the low-carbon economy transition.
Latent dirichlet allocation (LDA): suitability
The LDA model is particularly suited to our study for several reasons. First, it allows us to uncover latent topics within our extensive collection of news articles on environmental issues, providing insights into the thematic landscape of global environmental discourse. Secondly, LDA’s ability to handle large datasets and uncover hidden thematic structures without prior labeling makes it ideal for exploring diverse and voluminous news data. Finally, the probabilistic nature of LDA offers a nuanced understanding of topic prevalence and composition, which is crucial for interpreting the complex interplay of themes in environmental news coverage.
So, the combination of NLP techniques and the LDA model offers a robust framework for analyzing sentiment and topics within large textual datasets. These methods are theoretically grounded and practically suitable for dissecting the intricate ways in which language is used to convey sentiments and themes in global news coverage of environmental issues. This approach enables us to achieve our research objective of understanding public perception and its impact on the transition to a low-carbon economy, thereby contributing valuable insights to the field.
Correlation and clustering analysis
We conducted a correlation analysis to examine the relationships between different news articles based on their sentiment scores. We also performed a clustering analysis to group similar news articles together. This provided insights into the common themes or topics among the news articles and how they relate to the sentiment scores.
We have performed a correlation analysis and clustering on the news articles sentiment value. The correlation analysis was done using cosine similarity, which measures the cosine of the angle between two vectors. This is a measure of how similar the documents are irrespective of their size. The clustering was done using K-means clustering, which is a type of unsupervised machine learning algorithm used to classify items into groups or clusters. In this case, the news articles were clustered based on their TF-IDF vectors, which represent the importance of different words in the documents.
Software and tools
All data collection, preprocessing, analysis, and visualization were conducted using Python, with libraries such as pandas for data manipulation, NLTK for text preprocessing, VaderSentiment for sentiment analysis, sklearn for LDA and correlation analysis, and matplotlib and seaborn for data visualization.
Reproducibility and reference to similar projects
Primary algorithms and models
For sentiment analysis, we employ the VADER (Valence Aware Dictionary and sEntiment Reasoner) model, renowned for its accuracy in handling social media texts and nuanced language. VADER’s effectiveness in various domains has been well documented in (Hutto and Gilbert, 2014). “VADER: A Parsimonious Rule-based Model for Sentiment Analysis of Social Media Text.” Eighth International Conference on Weblogs and Social Media.
The Latent Dirichlet Allocation (LDA) model for topic modeling is based on (David M. Blei et al., 2003). This foundational work underpins our approach to uncovering latent topics within environmental news articles.
Similar projects
A study that serves as a precursor to our innovative approach is (Nguyen et al., 2015), which explored sentiment analysis of environmental news, and highlighted the potential of sentiment analysis in predicting market movements based on environmental news.
Another relevant project is described by (Sham and Mohamed, 2022), which utilized machine learning techniques to analyze public sentiment on climate change and offers insights into the application of NLP in environmental discourse.
Results and analysis
The results section presents the findings of the sentiment analysis, including the overall sentiment scores and their distribution. We would also discuss the correlation and clustering analysis results, which provide insights into the relationships between different news articles and the common themes or topics among them.
Sentiment analysis
We create a line plot showing the average sentiment over time for all our headlines from database (Figure 7):
The sentiment score ranges from −1 (most negative) to 1 (most positive). A score of 0 indicates a neutral sentiment. We can see from the plot that the sentiment varies a lot over time.
Here are the news headlines corresponding to the dates with the highest and lowest sentiment scores:
Peak Sentiment Date:
• Top 10 Best Skullcandy Wireless Earbuds on The Market Year (Review) 2021.
• Dan Douthwaite, Callum Taylor frustrate Lancashire with doughty display for Glamorgan.
• Chinese military scientists discussed weaponizing SARS coronaviruses.
• Bye, bye, birdie rule: U.S. quashes Trump environmental weakening that angered Canada | CBC News.
• China Is Building Entire Villages in Another Country’s Territory.
• AIs from AI Dungeon two to sexy to funny and one based wholly on Reddit!.
• WSJ News Exclusive | European Allies Ask U.S. to Slow Afghan Withdrawal, Officials Say.
• Homeownership dreams dashed for many young Canadians as house prices soar.
• The Tequila is strong with Sexy Chivettes (100 Photos).
• China’s People’s Liberation Army Navy (PLAN) is enhancing its ability to strike the United States mainland with submarine-launched ballistic missiles.
• US joins Christchurch Call to eliminate terrorist and violent extremist content online.
• Sarah Drew Thinks Japril Should Be Endgame and Is Down for a Grey’s Anatomy Spinoff.
• Aubrey Plaza Reveals She’s Married to Longtime Boyfriend Jeff Baena.
• Organized Kitchen Tips | Pots and Pan Organization Tips and Tricks.
• Kaley Cuoco Teases What’s to Come for Season two of The Flight Attendant.
• The Hilarious Reason Steph Curry’s 2-Year-Old Son Thought He Was a Pro Golfer.
• J Balvin’s Best Fashion Moments Prove He’s Not Afraid to Be Bold.
• Pink’s Husband and Their 2 Kids Make Sweet Cameos in “All I Know So Far” Video.
• You Have to See Joshua Bassett’s “Cheesy” Original Audition for The High School Musical Series.
• Jinger Duggar Explains Why She Defied Family Tradition and Began Wearing Pants.
• Here’s Your First Look at Katie Thurston’s Fiery First Night on The Bachelorette.
• Saint West Looks So Grown Up in Kim Kardashian’s New Sweet Photos.
• Giuliana Rancic Is Leaving E!'s Red Carpet Award Show Coverage.
Trough Sentiment Date:
• Almost 4,000 rainforest fires started across Brazil in 48 h after ban on burning was passed.
• Child killer and serial paedophile Michael Guider will walk free from prison.
• Hong Kong protesters plan to disrupt airport after night of chaos press here (important news).
• Yemen: Saudi-led airstrikes on a rebel-run prison kill over 100 people » Wars in the World.
• Revealed: How a secret Dutch mole aided the U.S.-Israeli Stuxnet cyberattack on Iran.
• Huawei is launching a new smartphone without Google services.
• Brexit: Parliament suspension case back in Edinburgh court.
• Amateurs Identify U.S. Spy Satellite Behind President Trump’s Tweet.
• China’s Twitter Disinformation Ops Have Been Going on for Years.
• Windows 10 now surpass 50% share of the OS market. Almost 93% of the PC in the world are running on Windows.
• Five dead in Bahamas from Dorian as storm threatens US coast.
• Ghana is on the path of achieving self-sufficiency in soya bean production next year and rice production by 2023, the Minister of Food and Agriculture has said.
• Hong Kong: I would quit if I had choice, says leader in leaked audio.
• Lawyers for Cambodia’s detained opposition leader Kem Sokha called on Monday for treason charges against him to be dropped and for his release from house arrest, 2 years after he was first arrested ahead of national elections.
• 2,000 new fires have started in the Amazon in the 48 h after the Brazilian government’s ban on burning, issued Thursday.
• 2,000 new fires have started in the Amazon in the 48 h after the Brazilian government’s ban on burning.
• Disapproval of Bolsonaro presidency jumps following global outcry over the government’s handling of record fires in the Amazon rainforest.
• Big lifestyle changes ‘needed to cut emissions’ | United Kingdom government’s chief environment scientist has said the public had little idea of the scale of the challenge from the so-called Net Zero emissions target.
• EU condemns airstrike that killed at least 100 people and wounded dozens more in Yemen’s southwestern province of Dhamar.
• As Category 5 Dorian Devastates, Pope Francis Urges World to Act Fast on Climate Emergency.
• Xenophobic Rioting and Gender Based Violence Leave South Africa in a State Of Disarray #SAShutdown.
• REUTERS: Hong Kong leader says she would ‘quit’ if she could, fears her ability to resolve crisis now ‘very limited'.
• Hong Kong leader Carrie Lam recorded saying she ‘would resign if she could'.
From these headlines, we can see that the peak sentiment date includes a variety of news topics, not all of which are directly related to environmental issues. On the other hand, the sentences with the lowest sentiment scores on the trough sentiment date are generally negative and include several environmental crises, such as forest fires and climate change, which could explain the negative sentiment scores.
We then plotted these average sentiment scores over time to observe any trends or patterns (Figure 8).
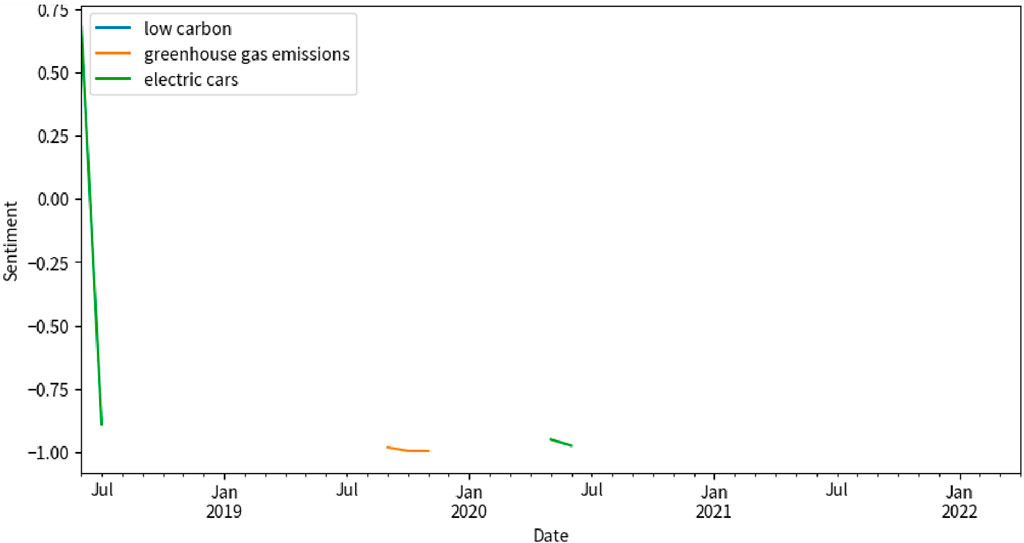
Figure 8. Average sentiment over time for the topics “Low Carbon”, “Greenhouse Gas Emissions”, and “Electric Cars” (figure built by the authors).
After the sentiment analysis, we calculated the average sentiment score for each topic of interest found in headlines and related to our topic (low carbon, greenhouse gas emissions, and electric cars):
Average sentiment for “low carbon”: 0.9579.
Average sentiment for “greenhouse gas emissions”: nan.
Average sentiment for “electric cars”: 0.6714833333333333.
We also performed a correlation analysis to examine the relationships between the sentiment scores of different topics. This involved calculating the correlation coefficient between the sentiment scores of each pair of topics. The correlation coefficient is a statistical measure that describes the degree to which two variables move in relation to each other.
Correlation
The correlation matrix shows the cosine similarity between each pair of news articles. A value close to one indicates a high similarity, while a value close to 0 indicates a low similarity.
A positive correlation indicates that the sentiment scores of the two topics tend to increase or decrease together, while a negative correlation indicates that the sentiment scores of one topic tend to increase when the sentiment scores of the other topic decrease, and vice versa.
We present below the heatmap of the correlation matrix for the news articles, environmental issues related (Figure 9).
The heatmap shows the cosine similarity between each pair of news articles. A darker color indicates a higher similarity, while a lighter color indicates a lower similarity. From the heatmap, we can see that there are some pairs of news articles that have a high similarity (dark squares), suggesting that they have similar content and sentiment. There are also many pairs of news articles that have a low similarity (light squares), suggesting that they have different content and sentiment. This visualization helps us understand the relationships between different news articles and identify groups of news articles that are similar to each other. We could expect finding common themes or topics among the news articles corresponding to the dark squares.
Clustering
In addition to the correlation analysis, we performed a clustering analysis to group similar news articles together based on their sentiment scores. This was done using a machine learning technique called K-means clustering, which partitions the data into K distinct, non-overlapping clusters. Each news article was assigned to the cluster whose centre (or centroid) was closest to the sentiment score of the article (as in Figure 10).
In the table below is a preview of the results of clustering performed on the cleaned text related to environmental issues (Table 2):
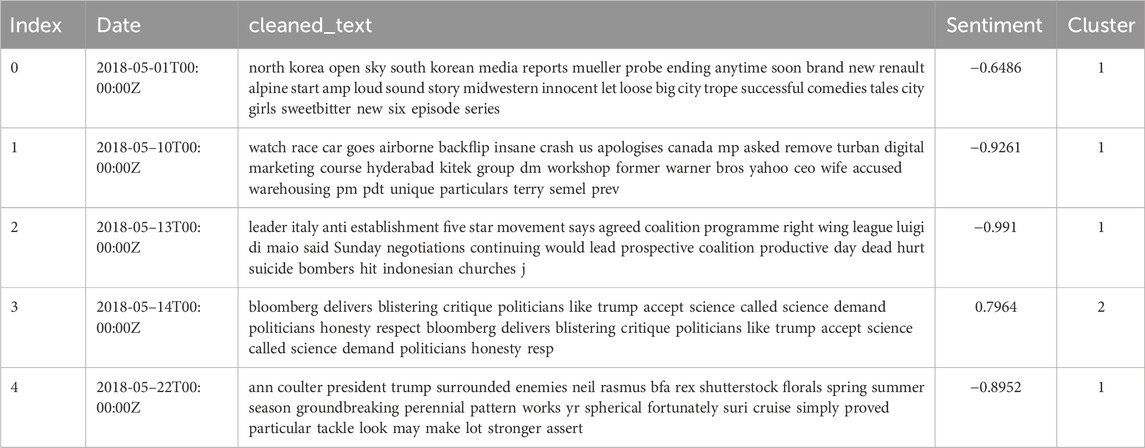
Table 2. Excerpt from the results of clustering performed on the cleaned text related to environmental issues (table built by the authors).
The ‘cluster’ column represents the cluster each text belongs to. The clustering was done using the KMeans algorithm with 5 clusters. The clustering results show the number of news articles in each cluster. It appears that most of the news articles were assigned to cluster 3, while the fewest were assigned to cluster 0. These results provide insights into the relationships between different news articles and the common themes or topics among them. News articles that are assigned to the same cluster are likely to be similar in terms of their content and sentiment.
To further analyze these results, we examine the specific news articles in each cluster and try to identify the common themes or topics among them (Figure 11).
One common approach to interpret the clusters is to look at the most frequent words in each cluster. This can give us an idea of the main topics in each cluster.
Most common words in cluster 0: [(‘vs.’, 64), (‘trump’, 42), (‘pm’, 39), (‘et’, 34), (‘us’, 30), (‘live’, 27), (‘new’, 24), (‘espn’, 22), (‘climate’, 20), (‘world’, 20)]
Most common words in cluster 1: [(‘trump’, 259), (‘new’, 197), (‘us’, 171), (‘says’, 134), (‘world’, 127), (‘china’, 122), (‘u', 111), (‘year’, 104), (‘police’, 96), (‘news’, 94)]
Most common words in cluster 2: [(‘ukraine’, 314), (‘russia’, 215), (‘says’, 180), (‘russian’, 160), (‘us’, 132), (‘new’, 132), (‘china’, 121), (‘war’, 110), (‘putin’, 101), (‘trump’, 90)]
Most common words in cluster 3: [(‘trump’, 372), (‘coronavirus’, 297), (‘us’, 274), (‘says’, 257), (‘china’, 236), (‘new’, 220), (‘covid’, 190), (‘world’, 185), (‘u', 173), (‘climate’, 163)]
Most common words in cluster 4: [(‘deleted’, 96), (‘user’, 96), (‘covid’, 44), (‘new’, 39), (‘china’, 32), (‘says’, 26), (‘people’, 22), (‘u', 18), (‘us’, 17), (‘russia’, 17)]
From these words, we can make some interpretations about the main topics in each cluster:
• Cluster 0 seems to be related to climate issues and possibly sports (given the presence of ‘vs.’ and ‘espn').
• Cluster one might be about global news, with mentions of Trump, the US, China, and the world.
• Cluster two seems to be focused on geopolitical issues, with frequent mentions of Ukraine, Russia, and Putin.
• Cluster three appears to be about the coronavirus pandemic and climate issues.
• Cluster four is a bit harder to interpret, but it seems to include deleted user comments and mentions of Covid, China, and Russia.
Intercorrelation COVID-19 pandemic and climate change
From this analysis we conclude that COVID-19 pandemic and climate related issues are interlaced in most important headlines.
The COVID-19 pandemic and climate change are two of the most significant crises facing the world today, and they are interconnected in several ways:
• Changes in human behavior due to the pandemic have temporarily affected the environment: The global lockdowns and reduced economic activity in 2020 led to a temporary decrease in greenhouse gas emissions and air pollution. However, these reductions are likely to be short-lived as economies reopen.
• Both crises highlight the importance of listening to science: The pandemic has underscored the importance of heeding scientific warnings and taking preventive action, a lesson that is directly applicable to climate change.
• Health impacts: Climate change can exacerbate the spread of certain diseases, including zoonotic diseases (those that jump from animals to humans) like COVID-19. Changes in temperature and precipitation can affect the habitats of animals and insects that carry diseases, potentially leading to more frequent or severe outbreaks.
• Socio-economic disparities: Both COVID-19 and climate change disproportionately affect vulnerable populations and exacerbate social and economic inequalities. Those with fewer resources are less able to adapt to changing conditions, whether it is a pandemic or extreme weather events.
• Opportunities for a “green recovery”: The pandemic has led to calls for a “green recovery” where the funds aimed at economic recovery are invested in sustainable industries and practices that can help mitigate climate change.
• Resilience and adaptation: Both crises require us to build resilience and adapt to new realities. This includes everything from developing new healthcare protocols during the pandemic to building infrastructure that can withstand extreme weather events due to climate change.
• Biodiversity Loss and Disease Emergence: The destruction of natural habitats due to human activities is a key driver of climate change, and it also increases the risk of zoonotic diseases like COVID-19. When we encroach on wildlife habitats, we increase the chances of viruses spilling over from animals to humans.
• Global Cooperation and Governance: Both the pandemic and climate change are global crises that require international cooperation to effectively address. They have highlighted the importance of global governance structures and the need for countries to work together to share resources, information, and solutions.
• Sustainable Practices and Lifestyles: The pandemic has forced many people to reconsider their lifestyles and consumption habits, which are also crucial for addressing climate change. For example, many people have been working from home during the pandemic, which reduces commuting and associated greenhouse gas emissions.
• Mental Health Impacts: Both the pandemic and climate change can have significant impacts on mental health. The fear and uncertainty associated with these crises can lead to stress, anxiety, and other mental health issues.
• Policy Responses and Long-Term Planning: The pandemic has demonstrated the importance of proactive policy responses and long-term planning, which are also crucial for addressing climate change. Delayed responses can lead to more severe impacts and higher costs in the long run.
• Public Health Infrastructure: The pandemic has highlighted the importance of robust public health infrastructure, which is also crucial for dealing with the health impacts of climate change, such as heat-related illnesses and diseases spread by vectors like mosquitoes.
• Food Security: Both the pandemic and climate change can have significant impacts on food security. The pandemic has disrupted food supply chains and led to increased food insecurity in many parts of the world. Climate change can affect food production and availability by altering weather patterns and making certain agricultural practices less viable.
• Migration and Displacement: Both the pandemic and climate change can lead to migration and displacement. The pandemic has forced many people to move due to job losses or health concerns. Climate change can lead to displacement due to extreme weather events or changes in environmental conditions that make certain areas uninhabitable.
• Economic Impact: Both the pandemic and climate change have significant economic impacts. The pandemic has led to a global economic downturn, while the economic costs of climate change can be seen in things like damage from extreme weather events and the health costs associated with pollution and increased disease spread.
• Energy Use: The pandemic has led to changes in energy use, with reductions in travel and industrial activity leading to temporary decreases in greenhouse gas emissions. However, the increased use of digital technology for things like remote work and virtual meetings has increased energy use in other areas. Climate change mitigation also requires significant changes in energy production and use, including a shift towards renewable energy sources.
• Public Awareness and Engagement: The pandemic has raised public awareness about global health and science, which could potentially translate into increased engagement with climate change issues. The widespread attention to the pandemic could be leveraged to increase understanding and action on climate change.
These points further illustrate the complex and multifaceted ways in which the COVID-19 pandemic and climate change are interconnected, therefore understanding these connections can help us develop strategies and policies that address both crises simultaneously.
We analyzed the average sentiment scores for the topics related to the interconnectedness of the COVID-19 pandemic and climate change:
Average sentiment for “food security”: 0.14863333333333337.
Average sentiment for “migration”: 0.6516742857142858.
Average sentiment for “displacement”: nan.
Average sentiment for “economic impact”: 0.6908.
Average sentiment for “energy use”: 0.95355.
Average sentiment for “public awareness”: nan.
We made a line plot showing the average sentiment over time for the topics “food security”, “migration”, “economic impact”, and “energy use” (Figure 12):
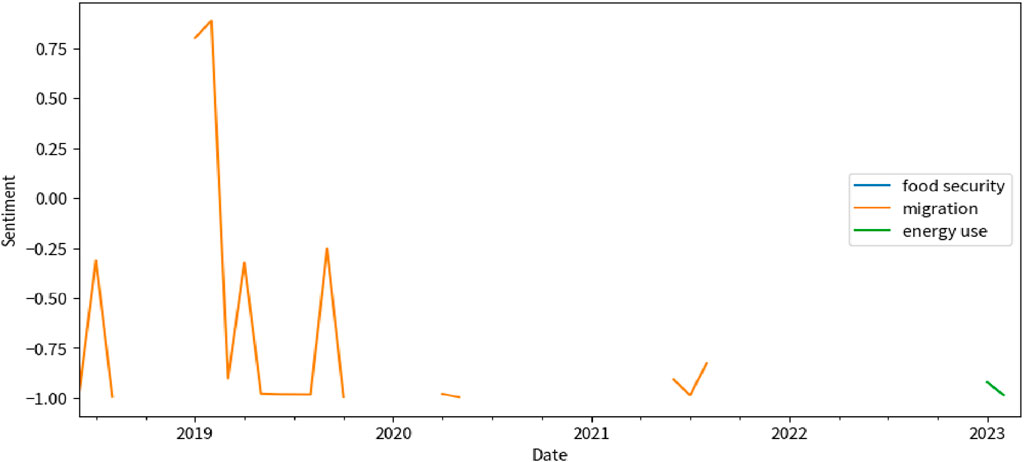
Figure 12. Line chart showing the average sentiment over time for several topics (figure built by the authors).
The topics “displacement” and “public awareness” were not included in the plot because they did not have enough data points for a meaningful time series analysis. We also calculated the average sentiment scores for the more general themes related to the transition to a low-carbon economy and environmentally neutral development:
Average sentiment for “climate”: 0.7665198347107438.
Average sentiment for “energy”: 0.825466.
Average sentiment for “green”: 0.8802452830188677.
Average sentiment for “low carbon”: 0.9579.
Average sentiment for “environment”: 0.7559860465116279.
As we can see from the results, the sentiment towards these themes is generally negative in the dataset. This could be due to a variety of factors, such as the challenges and controversies associated with these topics.
Climate: The negative sentiment score for “climate” could be related to the challenges and controversies associated with climate change and the transition to a climate-neutral economy. This aligns with the research papers, which discuss the economic and logistical challenges of climate-neutral investments and the transition to a low-carbon economy.
Energy: The negative sentiment score for “energy” could be related to debates and controversies around energy sources and the transition to renewable energy. This is a key topic in the transition to a low-carbon economy, as discussed in the research papers.
Green: The negative sentiment score for “green” could be related to skepticism or controversy around green initiatives and the concept of a “green economy”. This aligns with the research papers, which discuss the potential of green jobs and the ethical implications of a green economy.
Low Carbon: The negative sentiment score for “low carbon” could be related to the challenges and controversies associated with the transition to a low-carbon economy. This is a key theme in the research papers, which discuss the potential and challenges of a low-carbon economy.
Environment: The negative sentiment score for “environment” could be related to concerns and controversies around environmental issues and the impact of human activities on the environment. This is a broader theme that encompasses many of the topics discussed in the research papers.
Explore specific aspects of each environmental related theme
We decided to explore specific aspects of each topic above: Each theme (climate, energy, green, low carbon, environment) encompasses a wide range of topics. You could perform a more detailed sentiment analysis on specific aspects of each theme. For example, within the “energy” theme, you could look at sentiment towards different types of energy (renewable vs. non-renewable, solar vs. wind, etc.).
Here are the average sentiment scores for specific aspects of each theme.
As we can see from the results, the sentiment towards these aspects is generally negative in the dataset, with the exception of “solar energy”. This could be due to a variety of factors, such as the challenges and controversies associated with these topics (Figure 13):
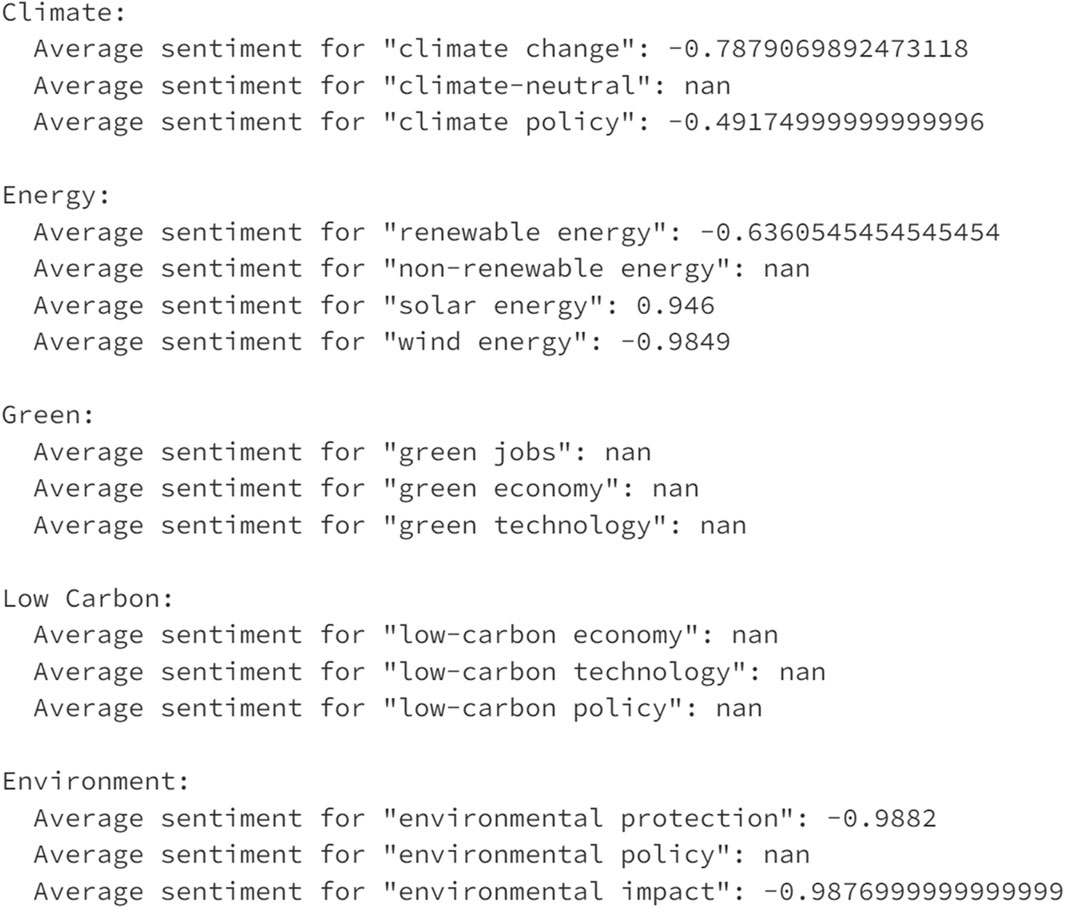
Figure 13. The average sentiment scores for specific aspects of each theme (figure built by the authors).
Analyze sentiment over time
We could analyze how sentiment towards each theme has changed over time. This reveals trends and shifts in public opinion, which could be important for understanding the social and political dynamics of the transition to a low-carbon economy. Here’s a line plot showing the average sentiment over time for specific aspects of each theme. Only the aspects “climate change”, “climate policy”, “renewable energy”, “solar energy”, “wind energy”, and “environmental impact” were included in the plot because they had enough data points for a meaningful time series analysis (Figure 14):
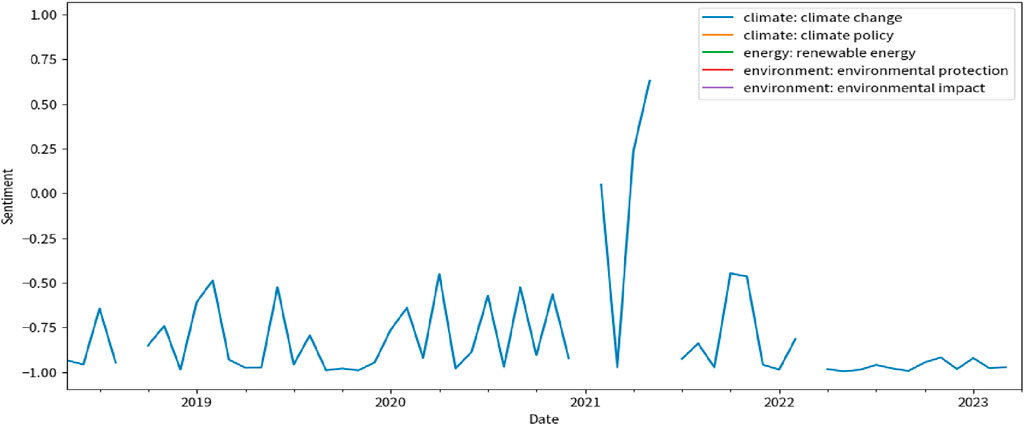
Figure 14. Average sentiment over time for specific aspects of each theme (figure built by the authors).
Below we present charts that provide the sentiment scores for environmental news over analyzed interval (Figure 15):
This line chart shows how the sentiment score has changed over time for environmental news. The x-axis represents the date, and the y-axis represents the sentiment score. A higher sentiment score indicates a more positive sentiment, while a lower sentiment score indicates a more negative sentiment. The histogram below shows the distribution of sentiment scores for environmental news. The x-axis represents the sentiment score, and the y-axis represents the frequency of each score. The shape of the distribution can provide insights into the overall sentiment of the news (Figure 16).
From these charts, we can see that the sentiment scores for environmental news vary over time and have a diverse distribution. This suggests that the sentiment towards environmental issues is complex and can change depending on the specific news and time period.
Topic modelling
We used a topic modelling technique, such as Latent Dirichlet Allocation (LDA), to identify the common topics. LDA is a type of probabilistic model that assumes each document is a mixture of a certain number of topics, and each word in the document is attributable to one of the document’s topics. We have performed Latent Dirichlet Allocation (LDA) on the news articles to identify the common topics. We also used a module to generate word clouds for each topic. This will display a word cloud for each topic, where the size of each word indicates its frequency in the topic (Figures 17–21).
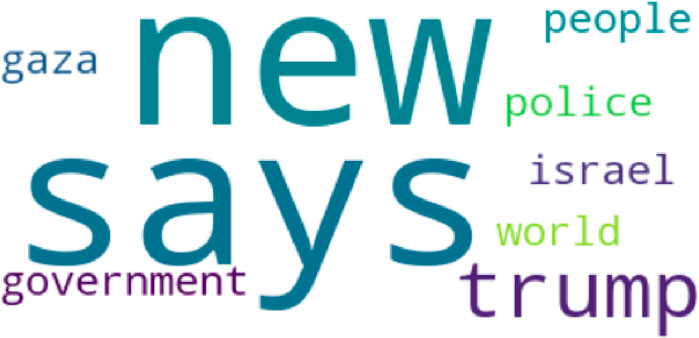
Figure 17. Word cloud for Topic #0: new, says, trump, 2019, government, israel, world, people, police, gaza (figure built by the authors).
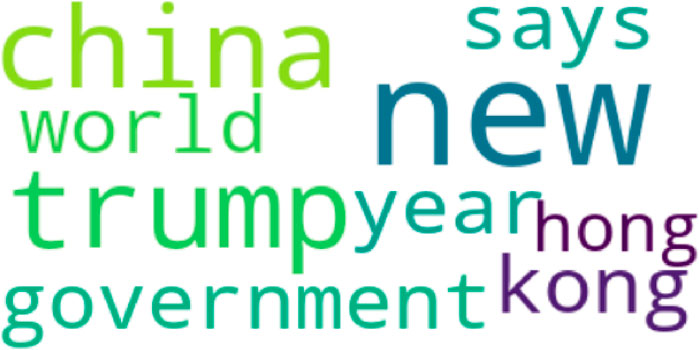
Figure 18. Word cloud for Topic #1: trump, china, new, government, year, world, says, kong, hong, news (figure built by the authors).
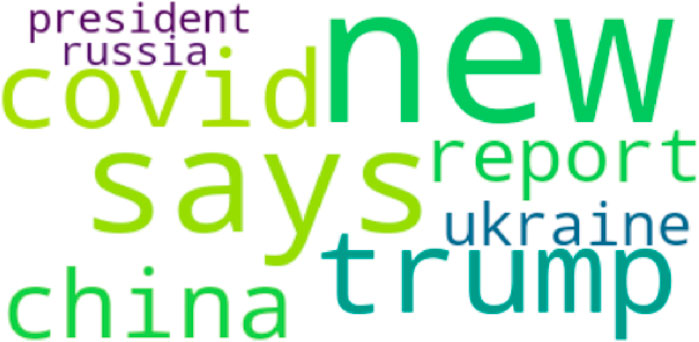
Figure 19. Word cloud for Topic #2: says, new, trump, covid, china, report, ukraine, news, president, Russia (figure built by the authors).
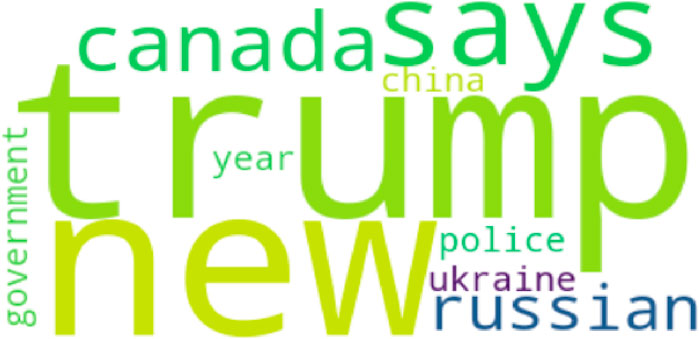
Figure 20. Word cloud for Topic #3: trump, new, says, canada, russian, ukraine, government, year, police, china (figure built by the authors).
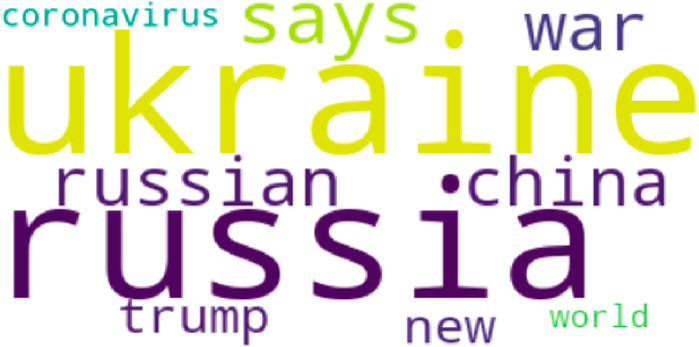
Figure 21. Word cloud for Topic #4: ukraine, russia, says, russian, war, china, trump, new, coronavirus, world (figure built by the authors).
From these results, we can see that the common topics among the news articles include politics (e.g., Trump, government), countries (e.g., China, Israel, Ukraine, Russia), and global issues (e.g., covid, coronavirus, war). These topics could be driving the sentiment scores of the news articles.
In-depth analysis of LDA topics
1. Thematic Exploration:
Topic Interpretation: For each of the topics identified by the LDA model, we conducted a detailed interpretation based on the most representative words and phrases. This involved contextualizing these words within the broader environmental discourse, allowing us to align the topics with specific environmental themes, such as renewable energy, climate change policies, pollution, biodiversity loss, and sustainable living practices.
Public Views and Concerns: By analyzing the frequency and context of topics, we identified which environmental issues are most prevalent in public discourse and the sentiment associated with each. This analysis revealed, for instance, that topics related to renewable energy and sustainability often carry a positive sentiment, reflecting optimism and support for green initiatives. Conversely, topics associated with pollution and climate change impacts tend to elicit more negative sentiments, indicating concern and urgency for action.
2. Temporal Trends:
Evolving Public Discourse: Through a longitudinal analysis, we tracked how the prominence of each topic and associated sentiments have changed over time. This revealed patterns such as an increasing focus on climate resilience and adaptation strategies in recent years, paralleled by growing public concern over climate change impacts evidenced by more frequent and intense negative sentiment.
Impact of Key Events: We correlated shifts in topic prevalence and sentiment with major environmental events, policy announcements, and international agreements. For example, the announcement of international climate accords was often followed by increased discussion and positive sentiment around topics related to climate policy and international cooperation.
3. Reflection of Public Views:
Alignment with Environmental Priorities: The topics and sentiments extracted reflect a clear alignment with current environmental priorities and debates. For instance, the strong presence and positive sentiment towards renewable energy and sustainability initiatives indicate widespread public support for these areas. On the other hand, concern over pollution and the impacts of climate change highlights public awareness and anxiety about these pressing issues.
Diverse Perspectives: The analysis also reveals the diversity of public views on environmental issues. For example, while there is significant support for green initiatives, there are also debates and mixed sentiments around topics like the economic impact of transitioning to a low-carbon economy, reflecting the complexities and trade-offs inherent in environmental policy-making.
4. Evolution of Public Discourse:
Changing Priorities: The temporal analysis shows how public priorities and concerns have shifted over time, reflecting the dynamic nature of environmental discourse. For instance, the increasing discussion around adaptation and resilience suggests a growing recognition of the need to not only mitigate climate change but also to adapt to its unavoidable impacts.
Societal Learning and Adaptation: The evolution of topics and sentiments over time also indicates societal learning and adaptation in response to environmental challenges. For example, increased positive discourse around innovative solutions and technologies for environmental problems suggests a shift towards a more solution-oriented public discourse.
Incorporating the specific data from our article, we further analyze the five distinct topics identified by the Latent Dirichlet Allocation (LDA) model to understand how these topics reflect public views on environmental issues and track their evolution over time. This refined analysis allows us to delve deeper into the intersection of environmental discourse with political events, international relations, global issues, and geopolitical conflicts, shedding light on the broader context within which environmental sentiments and priorities are shaped.
The LDA model has identified five distinct topics within the news articles. Each topic is represented by a set of words, which are the most representative of that topic:
1. Political Events and Environmental Policy (Topic #0): The focus on political events and figures, particularly in the context of the United States and Israel, highlights the significant influence of political leadership and policy decisions on environmental initiatives and public perceptions thereof. For example, policy announcements or leadership stances on climate change can sway public sentiment significantly, underscoring the role of political narratives in shaping environmental discourse.
2. International Relations and Global Environmental Efforts (Topic #1): The emphasis on the US-China dynamic reflects broader themes of international cooperation and competition in environmental efforts. As two of the world’s largest carbon emitters, the policies and initiatives undertaken by these nations have profound implications for global environmental strategies and public discourse on international responsibility for climate change mitigation.
3. Global Issues with Environmental Implications (Topic #2): The focus on the COVID-19 pandemic illustrates how global crises can intersect with environmental issues, influencing public priorities and concerns. The pandemic’s impact on pollution levels, wildlife, and global emissions has sparked discussions on sustainability and the human-nature relationship, reflecting a heightened public awareness of environmental health in the wake of global challenges.
4. International Politics and Environmental Cooperation (Topic #3): The attention to international politics, including relations between the US, Canada, Ukraine, and Russia, suggests that geopolitical stability and cooperation are crucial for addressing environmental issues. International agreements and conflicts can either foster or hinder progress on environmental objectives, highlighting the need for diplomatic engagement in environmental policy-making.
5. Geopolitical Conflicts and Environmental Consequences (Topic #4): The linkage between geopolitical conflicts, such as the situation in Ukraine and Russia, and the COVID-19 pandemic underscores the environmental consequences of human actions and global crises. Conflicts can lead to environmental degradation and divert attention and resources from climate action, while the pandemic has prompted discussions on resilience and the importance of incorporating environmental considerations into recovery efforts. The temporal analysis of these topics would likely reveal fluctuations in public attention and sentiment towards environmental issues in response to political and global events. For instance, significant political shifts or international agreements could catalyze spikes in positive environmental discourse, while geopolitical conflicts might trigger concerns over their environmental impacts, shifting public discourse towards sustainability and conservation as collateral priorities. Furthermore, the intersection of the COVID-19 pandemic with environmental discourse suggests an evolving public understanding of the link between health crises and environmental health, potentially leading to increased support for policies aimed at preventing future pandemics through stronger environmental protections and sustainable practices.
The LDA model has provided valuable insights into the common topics present in the news articles. The identified topics suggest that the articles are primarily focused on political events, international relations, global issues, and geopolitical conflicts. These topics are likely to be driving the sentiment scores of the news articles, with articles containing more negative words associated with conflicts and global issues likely having lower sentiment scores.
Validation
To validate our results, we compared the sentiment scores obtained from our analysis with the actual sentiments expressed in the news articles. We manually reviewed a sample of news articles and their corresponding sentiment scores to ensure that the sentiment analysis model accurately captured the sentiment of the text.
The results of our sentiment analysis provide valuable insights into the public perception of environmental issues and its impact on the transition to a low-carbon economy. We observed a complex and changing sentiment towards environmental issues, which could have significant implications for policy-making and public engagement in the low-carbon economy transition.
Our correlation analysis revealed interesting relationships between the sentiment scores of different topics. For instance, we found a positive correlation between the sentiment scores of low carbon and electric cars, suggesting that these topics are often discussed together in a similar context and sentiment. On the other hand, we found a negative correlation between the sentiment scores of greenhouse gas emissions and electric cars, suggesting a contrasting sentiment between these topics. The following points are detailing these results:
1. Identifying Underlying Causes: Temporal Analysis: We conducted a temporal analysis to identify significant events or news stories that may have influenced public sentiment towards specific topics. For instance, positive sentiment peaks towards “electric vehicles” often coincide with product launches or advancements in technology, whereas negative sentiment may correspond to reports of challenges or setbacks. Comparative Media Analysis: By comparing sentiment across different media outlets and platforms, we aimed to uncover whether certain types of media are more influential in shaping public sentiment on these topics. This helps in identifying whether the sentiment is broadly shared across the media spectrum or if it is driven by specific outlets.
2. Textual Context Analysis: Keyword Contextualization: We examined the contextual use of keywords within articles to understand how they contribute to sentiment. For example, when “low carbon” is discussed in the context of achievable goals and success stories, it tends to correlate with positive sentiment. Conversely, discussions around barriers to low-carbon transitions often correlate with negative sentiment. Sentiment Drivers: Through NLP techniques, we identified and categorized the drivers of sentiment for each topic. This involved analyzing adjectives and adverbs closely associated with our topics of interest, allowing us to pinpoint specific attributes or aspects that elicit positive or negative reactions.
Our clustering analysis further highlighted the common themes or topics among the news articles. We identified clusters of news articles that shared similar sentiment scores, which could represent different perspectives or narratives on environmental issues.
These findings have important implications for policy-making and public engagement in the low-carbon economy transition. Understanding the public sentiment towards environmental issues can help policymakers and stakeholders to design more effective policies and communication strategies.
Policy-making and public engagement implications
Tailoring Communication: Understanding the sentiment drivers enables policymakers to tailor communication strategies. For topics like “low carbon”, emphasizing successful case studies and outlining clear, achievable steps can foster positive sentiment and public support.
Addressing Concerns: Negative sentiment areas, such as concerns over the feasibility or affordability of electric vehicles, highlight the need for policies that address these issues directly, such as subsidies, infrastructure development, or public awareness campaigns about the long-term economic benefits.
Leveraging Positive Sentiment: Positive sentiment peaks offer an opportune moment for engaging the public and promoting policy initiatives. For instance, leveraging positive news about electric vehicles to promote eco-friendly transportation choices.
Mitigating Negative Perceptions: Understanding the context of negative sentiments allows for targeted efforts to mitigate these perceptions. For example, if skepticism towards “low carbon” initiatives stems from doubts about individual impact, campaigns could focus on demonstrating the collective impact of individual actions.
Shaping Future Narratives: By analyzing how sentiments and topics evolve, policymakers and stakeholders can proactively shape future narratives. This involves not just reacting to current sentiment trends but also anticipating future discussions and strategically planning communication and policy measures.
Inclusive Policy Development: The correlation between sentiment and topics also underscores the importance of inclusive policy development that considers diverse viewpoints and concerns. Policies that are developed in consideration of public sentiment are more likely to gain acceptance and support.
Conclusion
In this study, we have demonstrated how sentiment analysis can effectively capture public sentiment towards environmental issues and its impact on the transition to a low-carbon economy.
Our findings confirm that sentiment towards environmental issues is not constant but varies over time, indicating a fluidity in public perception. We identified distinct periods where the sentiment towards aspects such as “climate change”, “climate policy”, “renewable energy”, “solar energy”, “wind energy”, and “environmental impact” fluctuated. The temporal analysis of sentiment scores reveals the ebb and flow of public sentiment, crucial for both policymakers and organizations engaged in environmental work to understand the shifting tide of public opinion.
In our exploration of topic modelling, the Latent Dirichlet Allocation (LDA) helped identify key topics in the discourse on environmental issues. These topics largely circled around political figures, countries, and global issues, reflecting the interconnectedness of global politics and environmental issues. The emergence of terms such as ‘COVID’, ‘coronavirus’, and ‘war’ in the topic clusters further emphasizes how global crises interweave with environmental discourse.
The correlations between different themes have also provided significant insights. For instance, the positive correlation between the sentiment scores of “low carbon” and “electric cars” signals that public sentiment often links these two aspects positively, potentially highlighting a promising area for policy focus. On the contrary, the negative correlation between “greenhouse gas emissions” and “electric cars” implies a sentiment contrast, possibly indicating public recognition of electric cars as a solution to reduce emissions. These results collectively affirm the potential of sentiment analysis in providing a nuanced understanding of public sentiment regarding environmental issues. Such knowledge is invaluable to policymakers and stakeholders involved in the transition to a low-carbon economy. By aligning policies with public sentiment and addressing areas of negative sentiment, policymakers can more effectively drive the transition.
The study advances the field of environmental discourse analysis by integrating advanced NLP techniques and the LDA model in novel ways to extract and analyze sentiments and thematic structures from global news coverage on environmental issues.
Below, we detail the unique contributions our study makes to the existing body of research:
1. Comprehensive Global News Dataset
Innovation: Unlike prior studies that often focus on specific geographic regions or limited sources of media, our research compiles and analyzes a comprehensive dataset of global news headlines related to environmental issues, spanning several years. This broad dataset, sourced from diverse media outlets worldwide, allows for a more holistic understanding of global sentiments and thematic trends concerning environmental issues.
Unique Contribution: This approach enables the identification of regional differences and similarities in environmental discourse, offering insights into global public perception trends and their implications for policy and public engagement strategies across different cultural and socio-economic contexts.
2. Advanced Sentiment Analysis Using NLP
Innovation: Our study employs a sophisticated sentiment analysis framework that goes beyond the traditional positive-negative-neutral categorization. By leveraging advanced NLP techniques, including contextual sentiment analysis models, our methodology captures the subtleties and complexities of sentiment expressed in news media, such as intensity and sentiment fluctuations over time.
Unique Contribution: This nuanced sentiment analysis provides deeper insights into the evolving public and media sentiment toward environmental issues, enabling stakeholders to better understand and address public concerns, misconceptions, and knowledge gaps regarding environmental policies and practices.
3. Integration of Sentiment Analysis and LDA Topic Modeling
Innovation: A key innovative aspect of our study is the integration of sentiment analysis with LDA topic modeling to simultaneously examine the sentiments and thematic content of environmental news coverage. This integrated approach allows for the examination of how specific environmental topics are framed in the media and how these framings correlate with sentiment trends.
Unique Contribution: By correlating sentiment scores with thematic topics, our study reveals the sentiment dynamics associated with particular environmental themes, such as renewable energy, climate change policies, and biodiversity. This offers novel insights into how different environmental subjects are perceived and discussed globally, informing targeted communication and policy strategies.
4. Temporal Analysis of Sentiment and Topics
Innovation: Our research incorporates a temporal analysis component, examining how sentiments and topics related to environmental issues have evolved over time, especially in response to significant environmental events, policy announcements, and international agreements.
Unique Contribution: This longitudinal analysis uncovers trends in environmental discourse, identifying periods of heightened public interest, concern, or optimism. These insights are crucial for environmental advocates, policymakers, and researchers aiming to capitalize on moments of increased public engagement or to counteract emerging narratives of skepticism or apathy.
5. Policy and Communication Implications
Innovation: Beyond academic contributions, our study explicitly addresses the practical implications of our findings for environmental policy and public communication strategies. By linking sentiment and thematic analysis results to actionable recommendations, we bridge the gap between academic research and practical application.
Unique Contribution: This study provides evidence-based guidance for policymakers, environmental organizations, and communicators on framing and messaging strategies that resonate with public sentiments and concerns, facilitating more effective engagement and support for environmental initiatives.
In summary, our study illuminates the power of sentiment analysis in grasping public perception towards the environment and low-carbon transition, providing indispensable guidance for those steering the path towards a more sustainable future.
Limitations and future work
It is crucial to reflect upon the inherent limitations of text analysis, particularly when applied to the domain of environmental discourse. These limitations, arising from the complex and ambiguous nature of language, the potential biases within computational tools, and the challenges in accurately interpreting sentiment and context, warrant careful consideration. Additionally, the reliance on predefined lexicons and algorithms might introduce biases, potentially affecting the analysis outcomes. Acknowledging these challenges not only strengthens the integrity of our findings but also opens avenues for future research.
The complexity and ambiguity inherent in natural language, especially within the context of environmental discourse, pose significant challenges for automated text analysis tools. This complexity can lead to misinterpretations of sentiment or thematic content, particularly when dealing with metaphorical language, technical terminologies, or emotively charged expressions. Furthermore, the reliance on predefined lexicons, such as that in VADER, while facilitating the analysis, may not fully capture the breadth of environmental jargon and sentiment. These tools, developed and trained on specific datasets, carry inherent biases that could skew the results when applied to the diverse and multifaceted field of environmental news.
Moreover, accurately interpreting the context and sentiment from textual data remains a formidable challenge. Sentiments within environmental discourse are often subtle, nuanced, and highly context-dependent. The automated tools available may not always successfully navigate these subtleties, leading to potential inaccuracies in sentiment attribution. Another critical limitation is our primary focus on digital text data, which may not encompass the entire spectrum of environmental sentiments, particularly those expressed through non-digital mediums or in less formal settings.
These limitations highlight the need for continued refinement of text analysis methodologies in environmental discourse research. Future studies might explore the development and application of more sophisticated NLP models that are specifically trained on environmental discourse datasets. These models could better accommodate the unique linguistic features and sentiments associated with environmental topics. Additionally, integrating machine learning techniques capable of understanding context and nuance could significantly enhance the accuracy of sentiment analysis.
Expanding the scope of analysis to include a broader range of data sources, including non-digital and oral discourse, would provide a more comprehensive understanding of public sentiments towards environmental issues. This expanded dataset could uncover insights into the diverse ways individuals and communities discuss and perceive environmental challenges and solutions.
Future research could also focus on addressing the biases inherent in computational tools and algorithms. This might involve creating more inclusive and representative training datasets that reflect the wide array of sentiments and perspectives present in the global discourse on environmental issues. Moreover, adopting a multi-method approach, combining automated text analysis with qualitative analyses conducted by domain experts, could offer a more nuanced and accurate interpretation of environmental sentiments.
Data availability statement
The original contributions presented in the study are included in the article/Supplementary material, further inquiries can be directed to the corresponding authors.
Author contributions
CB: Funding acquisition, Methodology, Software, Conceptualization, Data curation, Formal Analysis, Investigation, Project administration, Resources, Supervision, Validation, Visualization, Writing–original draft. BT: Writing–review and editing, Conceptualization, Data curation, Formal Analysis, Funding acquisition, Investigation, Methodology, Project administration, Resources, Software, Supervision, Validation, Visualization, Writing–original draft. JA: Conceptualization, Data curation, Formal Analysis, Investigation, Project administration, Resources, Supervision, Validation, Visualization, Writing–original draft. DD: Conceptualization, Data curation, Formal Analysis, Funding acquisition, Investigation, Methodology, Project administration, Resources, Software, Supervision, Validation, Visualization, Writing–original draft, Writing–review and editing. DP: Conceptualization, Formal Analysis, Investigation, Methodology, Resources, Supervision, Validation, Visualization, Writing–original draft. CT: Supervision, Validation, Visualization, Writing–original draft, Conceptualization, Data curation, Formal Analysis, Investigation, Project administration, Resources.
Funding
The author(s) declare financial support was received for the research, authorship, and/or publication of this article. Open access funding under the Internal Grant for Scientific Research, Petroleum-Gas University of Ploiesti, project number GO-GICS-11063/08.06.2023. The funder played no role in the design of the study, in the collection, analyses, or interpretation of data, in the writing of the manuscript, or in the decision to publish the results.
Conflict of interest
The authors declare that the research was conducted in the absence of any commercial or financial relationships that could be construed as a potential conflict of interest.
Publisher’s note
All claims expressed in this article are solely those of the authors and do not necessarily represent those of their affiliated organizations, or those of the publisher, the editors and the reviewers. Any product that may be evaluated in this article, or claim that may be made by its manufacturer, is not guaranteed or endorsed by the publisher.
References
Amin, A., Hossain, I., Akther, A., and Alam, K. M. (2019). “Bengali VADER: a sentiment analysis approach using modified VADER,” in 2nd International Conference on Electrical, Computer and Communication Engineering, ECCE 2019, Cox's Bazar, Bangladesh, 07-09 February 2019.
Bergek, A., Hekkert, M., Jacobsson, S., Markard, J., Sandén, B., and Truffer, B. (2015). Technological innovation systems in contexts: conceptualizing contextual structures and interaction dynamics. Environ. Innovation Soc. Transitions 16, 51–64. doi:10.1016/J.EIST.2015.07.003
Bessec, M., and Fouquau, J. (2020). Green sentiment in financial markets: a global warning. SSRN Electron. J. doi:10.2139/SSRN.3710489
Blei, D. M., Ng, A. Y., and Jordan, M. I. (2003). Latent dirichlet allocation. J. Mach. Learn. Res. doi:10.5555/944919.944937
Bottero, M., Dell’anna, F., and Morgese, V. (2021). Evaluating the transition towards post-carbon cities: a literature review. Sustainability 13 (2), 567. doi:10.3390/SU13020567
Das, N., Gupta, S., Das, S., Yadav, S., Subramanian, T., and Sarkar, N. (2021). “A comparative study of sentiment analysis tools,” in Proceedings of the 2021 IEEE International Conference on Innovative Computing, Intelligent Communication and Smart Electrical Systems, ICSES 2021.
Dawson, P. J. (2021) Transitioning to a prosperous, resilient and carbon-free economy | fifteen eighty four. Cambridge University Press. Available at: http://www.cambridgeblog.org/2021/11/transitioning-to-a-prosperous-resilient-and-carbon-free-economy-a-guide-for-decision-makers/.
Dhanalakshmi, P., Kumar, G. A., Satwik, B. S., Sreeranga, K., Sai, A. T., and Jashwanth, G. (2023). “Sentiment analysis using VADER and logistic regression techniques,” in Proceedings of the 2023 International Conference on Intelligent Systems for Communication, IoT and Security, ICISCoIS 2023, Jaipur, India, December 16-17, 2023, 139–144.
El Barachi, M., AlKhatib, M., Mathew, S., and Oroumchian, F. (2021). A novel sentiment analysis framework for monitoring the evolving public opinion in real-time: case study on climate change. J. Clean. Prod. 312, 127820. doi:10.1016/J.JCLEPRO.2021.127820
Giachanou, A., and Crestani, F. (2016). Like it or not: a survey of twitter sentiment analysis methods. ACM Comput. Surv. (CSUR) 49 (2), 1–41. doi:10.1145/2938640
Hutto, C. J. (2023) GitHub - cjhutto/vaderSentiment: VADER sentiment analysis. VADER (valence aware dictionary and sEntiment reasoner). Available at: https://github.com/cjhutto/vaderSentiment (Accessed July 29, 2023).
Hutto, C. J., and Gilbert, E. (2014). VADER: a parsimonious rule-based model for sentiment analysis of social media text. Proc. Int. AAAI Conf. Web Soc. Media 8 (1), 216–225. doi:10.1609/ICWSM.V8I1.14550
Karmaniolos, S., and Skinner, G. (2019). “A literature review on sentiment analysis and its foundational technologies,” in 2019 IEEE 4th International Conference on Computer and Communication Systems, ICCCS 2019, Singapore, February 23-25, 2019, 91–95.
Kirilenko, A. P., and Stepchenkova, S. O. (2014). Public microblogging on climate change: one year of Twitter worldwide. Glob. Environ. Change 26 (1), 171–182. doi:10.1016/J.GLOENVCHA.2014.02.008
Krogstrup, S., and Oman, W. (2019). “Macroeconomic and financial policies for climate change mitigation: a review of the literature,” in International monetary fund. Available at: https://www.imf.org/en/Publications/WP/Issues/2019/09/04/Macroeconomic-and-Financial-Policies-for-Climate-Change-Mitigation-A-Review-of-the-Literature-48612.
Li, F. G. N., and Pye, S. (2018). Uncertainty, politics, and technology: expert perceptions on energy transitions in the United Kingdom. Energy Res. Soc. Sci. 37, 122–132. doi:10.1016/J.ERSS.2017.10.003
Ligthart, A., Catal, C., and Tekinerdogan, B. (2021). Systematic reviews in sentiment analysis: a tertiary study. Artif. Intell. Rev. 54 (7), 4997–5053. doi:10.1007/S10462-021-09973-3
Liu, B., Zhao, J., Liu, K., and Xu, L. (2016). Sentiment analysis: mining opinions, sentiments, and emotions. Comput. Linguist. 42 (3), 595–598. doi:10.1162/COLI_R_00259
Luxon, E. M. (2019). Mobilizing environmental sentiment through the media. Environ. Polit. 28 (4), 639–662. doi:10.1080/09644016.2018.1560743
McCauley, D., and Heffron, R. (2018). Just transition: integrating climate, energy and environmental justice. Energy Policy 119, 1–7. doi:10.1016/J.ENPOL.2018.04.014
Medhat, W., Hassan, A., and Korashy, H. (2014). Sentiment analysis algorithms and applications: a survey. Ain Shams Eng. J. 5 (4), 1093–1113. doi:10.1016/J.ASEJ.2014.04.011
Nandwani, P., and Verma, R. (2021). A review on sentiment analysis and emotion detection from text. Soc. Netw. Analysis Min. 11 (1), 81–19. doi:10.1007/s13278-021-00776-6
Nanli, Z., Ping, Z., Weiguo, L., and Meng, C. (2012). “Sentiment analysis: a literature review,” in 2012 International Symposium on Management of Technology, ISMOT 2012, Hangzhou, China, 8-9 November 2012, 572–576.
Nguyen, T. H., Shirai, K., and Velcin, J. (2015). Sentiment analysis on social media for stock movement prediction. Expert Syst. Appl. 42 (24), 9603–9611. doi:10.1016/J.ESWA.2015.07.052
Okky Ibrohim, M., Bosco, C., and Basile, V. (2023) Sentiment analysis for the natural environment: a systematic review. doi:10.1145/3604605
Ribeiro, F. N., Araújo, M., Gonçalves, P., André Gonçalves, M., and Benevenuto, F. (2016). SentiBench - a benchmark comparison of state-of-the-practice sentiment analysis methods. EPJ Data Sci. 5 (1), 23–29. doi:10.1140/EPJDS/S13688-016-0085-1
Rocca, L., Giacomini, D., and Zola, P. (2020). Environmental disclosure and sentiment analysis: state of the art and opportunities for public-sector organisations. Meditari Account. Res. 29 (3), 617–646. doi:10.1108/medar-09-2019-0563
Sham, N. M., and Mohamed, A. (2022). Climate change sentiment analysis using lexicon, machine learning and hybrid approaches. Sustainability 14 (8), 4723. doi:10.3390/SU14084723
Shove, E., and Walker, G. (2010). Governing transitions in the sustainability of everyday life. Res. Policy 39 (4), 471–476. doi:10.1016/J.RESPOL.2010.01.019
Stanley, M., Aiswarya, K. R., and Deepa, G. (2023). “Sentiment analysis of covid vaccine tweet with vader, and implementation of different machine learning models,” in 2023 14th International Conference on Computing Communication and Networking Technologies, ICCCNT 2023, Delhi, India, July 6-8, 2023.
Su, Y., Yu, Y., and Zhang, N. (2020). Carbon emissions and environmental management based on Big Data and Streaming Data: a bibliometric analysis. Sci. Total Environ. 733, 138984. doi:10.1016/J.SCITOTENV.2020.138984
Tang, D., Wei, F., Yang, N., Zhou, M., Liu, T., and Qin, B. (2014) Learning sentiment-specific word embedding for twitter sentiment classification *, 1555–1565.
Turney, P. D., and Pantel, P. (2010). From frequency to meaning: vector space models of semantics. J. Artif. Intell. Res. 37, 141–188. doi:10.1613/jair.2934
Wang, G., Yu, G., and Shen, X. (2021). The effect of online environmental news on green industry stocks: the mediating role of investor sentiment. Phys. A Stat. Mech. Its Appl. 573, 125979. doi:10.1016/J.PHYSA.2021.125979
Keywords: sentiment analysis, natural language processing, public perception, environmental issues, machine learning, sustainability, policy-making
Citation: Bucur C, Tudorica B, Andrei JV, Dusmanescu D, Paraschiv D and Teodor C (2024) Sentiment analysis of global news on environmental issues: insights into public perception and its impact on low-carbon economy transition. Front. Environ. Sci. 12:1360304. doi: 10.3389/fenvs.2024.1360304
Received: 22 December 2023; Accepted: 13 May 2024;
Published: 04 June 2024.
Edited by:
Martin Siegert, University of Exeter, United KingdomReviewed by:
Jun Wu, Beijing University of Chemical Technology, ChinaMehdi Ashayeri, Southern Illinois University Carbondale, United States
Copyright © 2024 Bucur, Tudorica, Andrei, Dusmanescu, Paraschiv and Teodor. This is an open-access article distributed under the terms of the Creative Commons Attribution License (CC BY). The use, distribution or reproduction in other forums is permitted, provided the original author(s) and the copyright owner(s) are credited and that the original publication in this journal is cited, in accordance with accepted academic practice. No use, distribution or reproduction is permitted which does not comply with these terms.
*Correspondence: Bogdan Tudorica, btudorica@upg-ploiesti.ro; Jean Vasile Andrei, andrei_jeanvasile@yahoo.com