- School of Business, Xinyang Normal University, Xinyang, China
Green finance (GF), new infrastructure (NI), and low-carbon green development play important roles in promoting high-quality economic development in China. To date, few studies have considered the relationships among GF, NI, and carbon emission performance (CEP) simultaneously. Using the panel data of 285 Chinese cities from 2011 to 2021, an improved vertical and horizontal pull-out gearing method was used to measure the GF and NI composite indices, and the CEP was measured using the slacks-based measure (SBM) non-expected output model. Using the panel data fixed-effects model and interactive effect model, it was found that both GF and NI can effectively enhance urban CEP and produce a superposition effect, promoting each other to deepen their ability to enhance the CEP. Heterogeneity analysis showed that the effects of GF and NI are more notable in eastern China, with a higher degree of marketization, policy support for the development of the digital economy, and resource-based cities. Green technological innovation, industrial structure upgrading, and resource allocation optimization are pathways for GF and NI to jointly enhance the CEP. Further analysis of the spatial system of the equation model using the generalized three-stage least squares (GS3SLS) methodology found that there were spatial interaction spillover effects among GF, NI, and CEP and that these effects were better for a local area than for the surrounding neighboring cities.
1 Introduction
The emission of greenhouse gases (GHGs), mainly carbon dioxide, has a profound impact on human living environments and sustainable socioeconomic development. The strategic goal of “striving to achieve carbon peaking by 2030 and carbon neutrality by 2060” is China’s solemn commitment to addressing global climate change. It not only reflects China’s image as a responsible and accountable big country but also fully demonstrates China’s courage and determination to actively participate in global climate governance.
The promotion of a “dual-carbon” strategy is a complex systematic project that requires a large amount of financial investment. The Chinese government estimates that achieving carbon neutrality will require an investment of over 100 trillion RMB over the next 40 years (Liu et al., 2022a). However, with its existing financial capacity, China’s contribution to the financial investment in carbon emission management is only approximately 15%. In the face of such a huge demand for carbon reduction funds and the pressure of economic transformation, the remaining financial gap can only be filled by financial means, and green finance (GF) has become an inevitable choice to comply with this trend. GF is an institutional arrangement and the financial services provided to improve the environment, conserve resources and cope with climate change, and promote the development of low-carbon economy, which is important for achieving the goal of “dual-carbon” (Zhang et al., 2022a). As a new type of financial model, GF promotes the research, development, and application of green technologies; promotes the optimization of the energy structure and upgrading of the industrial structure; optimizes the allocation of resources through the support and guidance of tools, such as green loans, green bonds, green funds, and green investments; and significantly reduces carbon emissions (Gu et al., 2021; Hu et al., 2021). However, some scholars have also shown that, owing to the influence of the performance appraisal mechanism, lack of local government environmental protection supervision, information asymmetry, and other factors, GF not only fails to inhibit carbon emissions but also promotes an increase in carbon emissions (Hasman et al., 2014; Mengze and Wei, 2015; Soundarrajan and Vivek, 2016). Therefore, there is a need for further research on the relationship between GF and carbon emissions.
New infrastructure (NI) is an infrastructure construction in the context of the development of digitalization, intelligence, and networking, oriented to adapt to the needs of the new round of technological revolution and change, led by the new development concept, driven by technological innovation, based on information networks, and oriented to the need for high-quality development to provide services, such as digital transformation, intelligent upgrading, convergence, and innovation (Ministry of Industry and Information Technology, 2021). NI is an important initiative for China to lead the new economic normal, support the digital transformation of the economy and society, improve the efficiency of resource allocation, improve people’s livelihoods, and enhance the people’s sense of wellbeing, and it is also the key to fostering new modes, new business models, and new industries, promoting the transformation of old and new kinetic energy and the green development of the economy (Zhang et al., 2023a). The outline of the 14th Five-Year Plan for the National Economic and Social Development of the People’s Republic of China and the Visionary Goals for the Year 2035 state that it is necessary to “focus on strengthening the support for digital transformation, intelligent upgrading, and convergence and innovation and to lay out and build new types of information infrastructures, convergence infrastructures, innovation infrastructures, and other new types of infrastructure.” The NI has the unique advantages of being “intelligent, green, and safe,” requiring “low energy consumption,” and producing “low emission” and “low pollution.” It is important to compensate for the shortcomings of China’s industrial development and promote carbon emission reduction (Du et al., 2022). Currently, the effects of NI on carbon emissions remain controversial. In addition to supporting the view that NI is favorable for carbon emission reduction, some studies suggest that NI promotes an increase in carbon emissions (Tang and Yang, 2023). The construction of NI promotes the rapid expansion of the digital economy, which significantly increases the energy consumption for data transmission, storage, computation, applications, and device connectivity (Hintemann, 2020). NI not only emits large amounts of carbon dioxide during construction but also stimulates the consumption behavior of the population, leading to increased energy consumption and exacerbating the current carbon emission situation (Tang and Yang, 2023).
Currently, China has the largest financial market and the world’s largest and most technologically advanced NI. However, it still faces major challenges in carbon emission reduction owing to its large population, the complexity of the financial market, the huge amount of capital required for NI construction, and its dependence on resources for living and production. Therefore, exploring the relationships among GF, NI, and carbon emission has become a key driver of modern economic development and an important factor in national competitiveness. As GF and NI are important elements of high-quality development, can their organic combination produce a “stacking effect” to further deepen the impact on carbon emission? What are the mechanisms underlying the relationships among GF, NI, and carbon emission? Considering the heterogeneity of regions and cities, are there any differences in the impacts of GF and NI on carbon emissions? Through which paths do they influence carbon emission?
In view of this, this study adopted panel data from 285 Chinese cities from 2011 to 2021 to explore the relationships among GF, NI, and carbon emission performance (CEP). First, this study placed GF, NI, and CEP into the same analytical framework and theoretically analyzed the mechanisms and synergistic paths of GF and NI on the CEP. The theoretical analysis showed that both GF and NI can not only directly affect CEP but also through green technology innovation, industrial structure upgrading, and resource allocation optimization. Simultaneously, they have synergistic effects that can promote each other to further deepen the effect on CEP through the “superposition effect.” Second, the GF and NI composite indexes were constructed, and improved vertical and horizontal pull-out gearing methods were adopted in order to measure China’s GF and NI composite indexes more comprehensively and accurately. To overcome the contradiction between the dual objectives of carbon emission reduction and economic growth, this study adopted the non-expected output SBM model to measure the CEP and indicate the level of carbon emissions. Third, we examined the general impact, synergistic effect, and transmission path of GF and NI on CEP. The results show that GF and NI can effectively improve CEP, and the conclusion still holds after the robustness test and endogeneity treatment. Their synergistic effects can also effectively improve the CEP. Green technology innovation, industrial structure upgrading, and resource allocation optimization are common paths for GF and NI to promote CEP. This study further explored the differences between GF and NI in terms of their impacts on urban CEP under heterogeneous factors, such as urban location, degree of marketization, policy support for digital economy development, and type of urban development. It was found that GF can effectively promote CEP in all regions of China with a high degree of marketization, policy support for digital economy development, and resource-based cities, whereas NI can more effectively promote CEP in eastern China with a higher degree of marketization, policy support for digital economy development, and resource-based cities. Finally, this study explored the spatial interactive spillover effects among GF, NI, and CEP. It was found that GF and NI have spatial interactive spillover effects on urban CEP; GF can effectively promote local CEP and NI construction, as well as the CEP of neighboring cities; NI can effectively promote the CEP and GF development of local and neighboring cities; and CEP can, in turn, promote the GF development and NI construction of local and neighboring cities, and the above effects are better on the local than on the surrounding neighboring cities.
The marginal contributions of this paper are mainly as follows: (1) on the basis of clarifying the connotation of GF, NI, and CEP, we focus on the mechanism of the “superposition effect” of the impact of GF development and NI construction on CEP, analyze the synergistic effect of China’s GF and NI on CEP and their complex influencing relationship, and enrich the new topic of GF and NI, leading to low-carbon development; (2) changing the characterization of previous studies using a single indicator, comprehensively measuring GF and NI levels, and adopting the non-expected output SBM model to measure CEP make the study indicators more accurate, comprehensive, and representative; and (3) a variety of econometric models were used to comprehensively analyze the general effect, synergistic effect, and spatial interactive spillover effect of GF and NI on CEP, test the common conduction paths of the two influences on CEP, and test the differences in the impacts of GF and NI on CEP in terms of regional heterogeneity, the level of marketization, the support of the digital economy development policy, the type of urban development, and other heterogeneity perspectives. These studies enrich the empirical evidence on this research topic and help promote the depth of related research.
The remainder of this paper is organized as follows: Section 2 presents the theoretical foundations and research hypotheses; Section 3 presents the research design; Section 4 presents the results of the empirical analyses, and Section 5 presents the conclusions and policy recommendations.
2 Research hypothesis
2.1 GF and CEP
The GF affects CEP mainly through the financial financing effect (Zhang et al., 2022d). Corporate environmental governance is usually characterized by a long cycle, high cost, high risk, and high uncertainty and is limited by the high innovation cost of green technology, which faces technical barriers and a shortage of funds in the process of promoting green low-carbon technology. GF, as an emerging financial model, can effectively solve the problem of matching the supply and demand of green project funds through the innovation of traditional financial instruments and provide sufficient financial guarantee for the green transformation of regional economy (Zhu et al., 2021). Therefore, this study proposes the following hypothesis:
Hypothesis 1. GF can improve CEP.
2.1.1 GF and green technological innovation
However, green technology innovation has high-risk, high-input, and long-cycle characteristics, which makes it difficult for enterprises to obtain effective support from traditional finance. Enterprises relying only on their own funds usually find it difficult to meet the needs of clean-tech production and green product development. GF can provide enterprises with long-term and stable financial support for green technological innovation through credit, funds, and other low-cost financing channels, continuously stimulate the willingness of enterprises' to invest in innovation and increase R&D, and strengthen the supervision of green technological innovation through post-credit management, thus enhancing the efficiency of green technological innovation and promoting CEP (Zhang et al., 2022c). Based on these observations, we propose the following hypothesis:
Hypothesis 1a. GF improves CEP through the green technology innovation effect.
2.1.2 GF and industrial structure upgrades
On one hand, GF can effectively cause a fund-orientation effect, provide low-cost credits through policy guidance, direct the flow of capital elements to energy-saving and environmental protection industries, promote the expansion of green industries, increase the production and consumption of clean energy, optimize the structure of energy consumption, and promote the upgrading of the industrial structure, thus achieving emission reduction effects (Wang and Wang, 2021). On the other hand, GF strictly controls the credit threshold of the main body of the enterprise, assesses the environmental risk and credit conditions of the enterprise, and, through the crowding-out effect, imposes financing constraints on high energy-consuming and high-polluting industries and enterprises, restricts the development of high-polluting enterprises, eliminates the pollution-intensive secondary industry, shifts the focus of enterprise development to the tertiary industry centered on high-tech and modern service industries, and promotes the transformation and upgrading of the regional industry so as to improve CEP (Gu et al., 2021). Based on these observations, we propose the following hypothesis:
Hypothesis 1b. GF improves CEP through the industrial structure upgrading effect.
2.1.3 GF and resource allocation optimization
GF can increase new channels of green investment in society, reduce the cost of financing, comprehensively assess the environmental standards and emission status of the financing subject through credit and post-lending links, reduce the degree of information asymmetry, use the information disclosure mechanism to have a financing penalty effect on high-pollution enterprises, and effectively exert a supervisory effect on carbon emission by the external public (Zhang et al., 2022d). Additionally, by playing the function of incentives and constraints to prompt green funds to knowledge, high-tech intensive and other green energy-saving and environmental protection industries, through the provision of diversified, multi-level, and flexible allocation of funds, optimize the allocation of resources and prompt financial institutions to participate directly in environmental governance so as to promote green and low-carbon development (Jiang et al., 2020). Therefore, this study proposes research hypothesis 1c:
Hypothesis 1c. GF improves CEP through the resource allocation optimization effect.
2.2 NI and CEP
NI breaks down the spatial and temporal restrictions and barriers between cities, which is conducive to strengthening the real-time acquisition, analysis, and judgment of urban ecological environmental data, such as CO2, enhancing the digital control capability of carbon emissions, and reshaping the pattern of urban environmental governance (Zhang et al., 2023a). NI provides spatial attraction and impetus for socioeconomic activities and related factors, enhances the spatial expansion of urban space and internal value-adding, promotes the polycentricity of the urban spatial structure, alleviates the problem of urban diseases caused by the expansion of the urban scale and overconcentration of the population, and effectively improves CEP (Zhang et al., 2023b). NI will ultimately improve CEP by significantly increasing productivity; improving energy efficiency; developing new and cleaner resources; fostering low-energy production; promoting e-mobility, smart cities, teleworking, and distance learning; encouraging individuals to use digital devices to shape their consumption patterns and behaviors; and changing their production and lifestyles (Zhang et al., 2023a; Tang and Yang, 2023). In general, the increase in carbon emissions due to NI construction is temporary, and the impact on carbon reduction is long-lasting. With the increasing maturity of digital technology and its operation, its own carbon emissions become limited, and overall, NI can effectively improve CEP (Yang et al., 2022). Therefore, this study proposes hypothesis 2:
Hypothesis 2. NI can improve CEP.
2.2.1 NI and green technological innovation
NI supported by digital technology can significantly reduce the cost of information search, transmission cost, and knowledge dissemination cost; accelerate the diffusion of technology and knowledge overflow; accelerate the integration of technology and industry; deepen regional cooperation; enhance the level and efficiency of regional innovation; innovatively stimulate enterprises to increase R&D investment; and promote enterprise independent innovation and industrial collaborative innovation (Xu et al., 2019). NI provides the visual instant communication environment and provides conditions for full spillover of technological knowledge (Xie et al., 2021). Based on this, we propose the following hypothesis:
Hypothesis 2a. NI improves CEP through the green technological innovation effect.
2.2.2 NI and industrial structural upgrades
NI endows machines and equipment with powerful learning and computing capabilities; makes capital present digital and intelligent trend construction; drives industrial digital development and digital industrialization transformation; promotes the synergistic development of software and hardware of AI, data center, cloud computing, and other application scenarios; and assists enterprises in their digital transformation by stimulating technological innovation, expanding knowledge spillover, and optimizing the market environment (Ren et al., 2021). NI promotes the development of industrial structures toward high-precision industries and the elimination of backward industries by improving technology, substituting labor with capital, promoting the dissemination of regional knowledge and technology, improving core competitiveness, improving the efficiency of production factors, and accelerating the high-end processes of industries, thus effectively improving the level of CEP (Wang et al., 2023). Accordingly, we propose the following hypothesis:
Hypothesis 2b. NI improves CEP through the industrial structure upgrading effect.
2.2.3 NI and resource allocation optimization
NI, with big data, artificial intelligence, industrial Internet, and other technological advantages, fundamentally breaks through the spatial obstacles to the flow of factors in the arithmetic and algorithms to promote the various types of data elements that can be fully circulated in the market, to overcome the drawbacks caused by the asymmetric information, and to improve the efficiency of resource allocation through the investment multiplier effect, the industrial optimization effect, the network demonstration effect, and the spatial spillover effect (Bakken, 2019). In addition, the integration of NI and traditional industries can improve the combination of inputs of different production factors in the real economy, reduce the probability of factor mismatch, achieve efficient allocation and use of factor resources, and thus, effectively improve the level of CEP (Martínez et al., 2022). Accordingly, we propose the following hypothesis:
Hypothesis 2c. NI improves CEP through the resource allocation optimization effect.
2.3 GF, NI, and CEP
The effective integration of NI centered on big data, cloud computing, blockchain, 5G, and other digital technologies with the financial system can effectively support the operational efficiency of the financial system, reduce the operating costs of financial institutions, improve the asymmetry of information and the matching of supply and demand in the financial market, expand the market’s supply potential, improve the efficiency of capital allocation, and promote the agglomeration of financial resources (Zhang et al., 2022d). The development of digital technology promoted by NI can regulate and ensure the targeted input of funds, effectively optimize the review process and supervision mechanism of various investment projects, reduce the systematic risk of financial institutions and the risk of investment across time, form an effective and stable financial system, and promote the green development of the local economy (Yang et al., 2022).
NI is an urban infrastructure representing a new round of technological and industrial revolution and is an important embodiment of capital. NI construction is characterized by high capital demand, a long investment cycle, high fixed cost and low marginal cost, and high degree of market operation, which require diversified subjects to participate in the investment and construction (Zhang et al., 2023a). In addition to government investments, most funds must be obtained through market-oriented approaches. As a new financial model, through policy and market mechanism design, it can have a social capital mobilization effect, guide the social capital layout of the green industry, and alleviate NI construction financing problems. Only when supported by sufficient funds can NI construction gradually improve to play the advantage of zero-cost diffusion, which can effectively play a low-carbon green role (Raheem et al., 2020).
The aforementioned analyses show that GF and NI not only contribute to CEP but also interact with each other to improve CEP. Therefore, the following research hypothesis is proposed:
Hypothesis 3. GF and NI interact with each other and synergistically improve CEP.
2.4 Spatial interaction spillover effects
Notably, GF and NI can transcend spatial limitations, not only affecting local cities but also extending their impacts to neighboring cities as well (Zhang et al., 2022d; Zhang et al., 2023a). The development of GF and NI in local cities not only improves the CEP of local cities but also shows potential to affect the CEP level of surrounding neighboring cities (Zhang et al., 2024). In addition, gases are mobile, and GHGs are no exception; CO2 can flow between different regions with clear spatial dependence (Li and Li, 2020). Cities imitate each other in the process of development and learn from each other’s advanced urban management experiences, and cities in the same region show the same development characteristics (Liu et al., 2022b). In addition, Section 2.2 shows that GF and NI can interact with and mutually reinforce each other, thereby improving CEP through the synergistic stacking effect. It is worthwhile considering whether the change in the level of urban carbon emissions may, in turn, influence the formulation of urban development policies, in particular the GF development policy and the speed of NI construction. In turn, CEP affects GF and NI. Therefore, hypothesis 4 is proposed.
Hypothesis 4. GF, NI, and CEP have spatial interaction spillover effects.
The specific mechanism of action is shown in Figure 1.
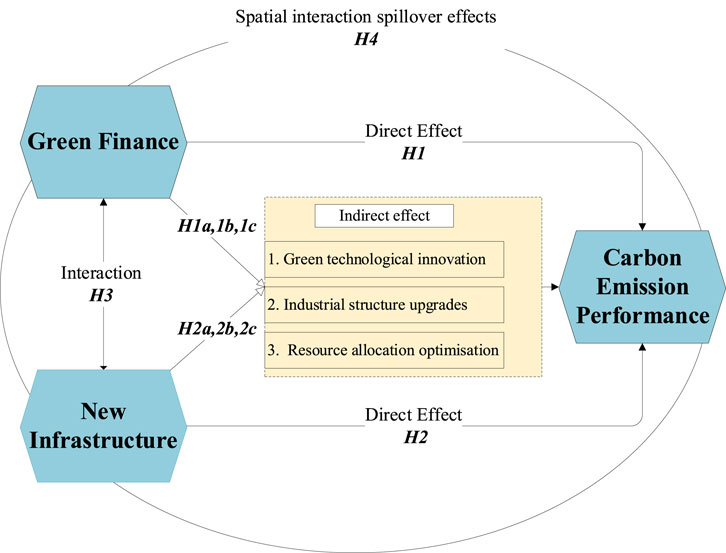
FIGURE 1. Mechanism analysis of green finance (GF) and new infrastructure (NI) affecting carbon emission performance (CEP).
3 Research design
3.1 Model
Based on the extended STIRPAT model, this study adopted city–year fixed effects to study the impacts of GF and NI on CEP and constructed the following model:
where
In this study, model 2 was constructed to examine the synergistic effects of GF and NI on CEP.
If
In this study, we also constructed the following spatial system of the simultaneous equation model to consider the spatial interaction spillover effects among GF, NI, and CEP:
where
3.2 Variables
3.2.1 CEP
In the study of carbon emission indicators, total carbon emissions, carbon emission intensity, and CEP are typically used. However, with the transformation of China’s economic development paradigm and the tightening of energy and environmental constraints, relying solely on a single-factor carbon productivity indicator (i.e., GDP per unit of carbon emissions) fails to capture the intricate reality of current economic and social progress. Compared with the total carbon emission indicators, CEP integrates various input factors and is a more comprehensive measure that includes the factors of economic development, which is the result of the combined effect of the energy structure and factor substitution, and can be interpreted as the total factor CEP (Zhang et al., 2022d). Therefore, CEP was selected as a measure of carbon emission in this study. CEP was calculated using the SBM non-expected output model proposed by Tone (2020), where the input variables were labor, capital, and energy; the expected output was GDP; and the non-expected output was CO2 emissions.
3.2.2 GF
According to the Guiding Opinions on Constructing the GF System (2016, No. 228), GF mainly comprises green credit, securities, insurance, and investments (Zhang et al., 2022d). Referring to existing studies and considering the actual development of GF in China and data availability, four dimensions of green credit, green securities, green insurance, and green investment, and six basic indicators were selected to construct the evaluation index system of the GF development level (Zhang et al., 2022b; Zhang et al., 2022d). The details are presented in Table 1.
To eliminate inconsistencies in the order of magnitude and scale, the raw data were first standardized using the extreme variance method. Then, an improved vertical and horizontal pull-out gearing method was used to calculate the GF development index for each region during the sample period.
3.2.3 NI
The 2020 China Development and Reform Commission pointed out that NI primarily includes three aspects: information infrastructure, convergence infrastructure, and innovation infrastructure. Compared to single indicators, such as the number of Internet accesses, composite indicators can integrate multiple indicator characteristics that are more representative and theoretically significant. Starting from the connotation of NI, basic indicators, such as broadband Internet base, mobile Internet base, telecommunication base, and information and software scale, were selected to construct the index system for measuring new infrastructure (Zhao and Sun, 2022; Zhang et al., 2023a). The same vertical and horizontal slotting methods were used to assign weights to calculate the composite index (Table 2).
3.2.4 Remaining key control variables
Referring to Zhang et al. (2022d), Zhang et al. (2023a), and Tang and Yang (2023), this study controlled other potential factors that may affect CEP. They mainly included economic development, energy consumption, population density, industrial structure, technological inputs, openness, and urbanization level. Economic development was measured using regional GDP per capita. The total carbon emissions were estimated based on the consumption of 14 fossil energy sources in the Energy Balance Sheet of the China Energy Statistics Yearbook, using the reference methodology and default parameters provided by the 2006 IPCC Guidelines for National Greenhouse Gas Inventories and combining them with the relevant parameters officially announced by China. The technology level was measured using per capita financial expenditure on science and technology. The forest coverage rate was measured as the ratio of the forest area to the urban land area. The urbanization rate was measured as the ratio of the urban population to the total population of the region. The degree of regional openness was measured using the ratio of the overall regional imports and exports to the regional GDP. The degree of government intervention was measured using the ratio of the fiscal expenditure of the regional government to the regional GDP.
3.3 Data
The implementation effect of policies often depends on strict constraints and the effective implementation of policies in prefectural cities; therefore, a study using a large sample of prefectural cities can objectively and effectively evaluate the implementation effect of the relevant “dual-carbon” policies formulated by the central government, as well as the provinces and municipalities. In this study, a panel of 285 cities in China from 2011 to 2021 was selected for empirical analysis (excluding regions with missing data). The data were mainly collected from all relevant statistical yearbooks and local statistical bureaus, as well as from the EPS, CSMAR, WIND, relevant websites, and the Internet. Missing data for cities in individual years were filled using linear interpolation. Descriptive statistics for each variable are shown in Table 3.
4 Empirical results and analyses
4.1 Benchmark regression results
In this study, the mean variance inflation factor (mean VIF) was used to test the model for multicollinearity, and the test results showed that none of the values exceeded the critical value of 10; therefore, it can be judged that the model variables do not have strict multicollinearity problems. Using the F-test and Hausman test, this study selected the panel data fixed-effects model for testing. The results of the benchmark regression are shown in Table 4.
In Table 4, regression result (1) shows the effect of considering only GF on CEP. The regression coefficient of GF and CEP was 0.3757 and significant at the 1% significance level, indicating that GF can effectively enhance CEP. The test results supported H1. The reason for this is that the most basic function of the GF is to raise and allocate funds so that financial resources can enter the field of green and low-carbon development in a more effectively way by optimizing the allocation of resources, encouraging green innovation, guiding the shift of enterprise production patterns to low-carbon and environmentally friendly patterns, and improving energy efficiency, thus enabling a significant increase in the CEP (Song et al., 2021; Zhang et al., 2022d).
The regression result (2) showed the effect of considering only the effect of NI on CEP. The regression results showed that NI construction is also effective in improving CEP, which is in line with research hypothesis 2. NI construction enables the government to accurately and efficiently obtain data related to carbon emissions to formulate appropriate carbon emission reduction measures (Zhang et al., 2022a). Moreover, NI benefits enterprises by optimizing resource allocation and upgrading production processes, thereby improving CEP (Martínez et al., 2022). In addition, NI reshapes consumers’ green awareness through the network and changes consumption patterns, thereby promoting low carbon consumption (Nudurupati et al., 2022). Therefore, NI can effectively influence the behavior of governments, enterprises, and residents, thus promoting the level of urban CEP.
Regression result (3) showed the effects of both GF and NI on CEP. The regression results showed that both GF and NI could effectively increase CEP levels. From the perspective of the control variables, the elasticity coefficient of GDP per capita was positive but not significant, indicating no notable relationship between economic growth and carbon emissions. The regression coefficient between the energy consumption structure and CEP was significantly negative because coal-based fossil fuel combustion remained one of the main sources of carbon emissions in China. The industrial structure also significantly reduced CEP, suggesting that an industrial structure dominated by secondary industries will exacerbate carbon emissions and reduce CEP; therefore, transformation of the industrial structure is urgent. The regression coefficient between technological innovation and CEP was significantly positive. Green technological innovation, represented by green invention, effectively reduces energy consumption through technological transformation and clean production mode, which, in turn, increases CEP (Xie et al., 2021). The regression coefficient between regional openness and CEP was significantly positively correlated. Regions with a high degree of openness were more inclined to absorb green investment and obtain advanced management experience and green innovation technology, which are conducive to CEP enhancement.
In regression result (4), we constructed the cross-term between GF and NI to test the effect of the interaction between GF and NI on CEP. The significant positive correlation between the cross-terms and regression coefficients of CEP show that the two can coordinate with each other, produce a superposition effect, and enhance the ability to improve CEP. On one hand, NI can promote GF to better play its role in capital financing and information disclosure and then promote the urban CEP; on the other hand, GF can provide sufficient funds to support the construction of NI and improve the urban NI and then play its role in information transmission, green innovation, resource saving, and efficiency enhancement, and thus, enhance the CEP (Zhang et al., 2022d; Zhang et al., 2023a). This finding is also in line with research hypothesis H3.
Because the core explanatory variables of this study, urban GF and NI construction levels, use a synthetic composite indicator, the regression estimation using only the panel data fixed-effects model may be biased. Regression result (4) is the result of the regression analysis using the systematic method of moment estimation to consider the robustness of the regression results and avoid the effect of endogeneity. The generalized method of moment (GMM) estimation is a commonly used method for solving the model endogeneity problem and is divided into differential GMM (DIF-GMM) and systematic GMM (SYS-GMM). Among them, SYS-GMM combines the DIF-GMM model and the horizontal GMM model, which can improve the estimation efficiency compared with DIF-GMM estimation (Liu and He, 2019). The system generalized moment estimation regression results show that the GF and NI constructions can still effectively improve the CEP.
4.2 Robustness tests
In this study, three main methods were used: the entropy weight method was used to re-measure GF and NI, the regression of GF and NI with a one-period lag was used, the core explanatory variables were replaced, and CO2 emission intensity was used instead of CEP. The robustness test results are presented in Table 5.
Regression results (1)–(3) show the regression results of the remeasured GF and NI indices using the entropy method. Column (1) shows the results of the regression of the newly measured GF, column (2) shows the results of the regression of the newly measured NI, and column (3) shows the results of the regression analyses of both using the newly measured indicators. As shown in Table 5, the regression results using the new indicators indicate that both GF and NI were effective in increasing CEP levels.
Regression results (4)–(6) show the regression results of the lagging GF, NI, and both for one period. The regression results showed that GF and NI can still effectively improve CEP after adopting the lagged one-period variables, and the size of the regression coefficients show that the regression results of the lagged variables are larger, which indicates that the effect of GF and NI on CEP has a certain lag, and the green and low-carbon efficacy of both needs a certain amount of time to transform (Raheem et al., 2020).
Regression result (7) showed the regression result after replacing CEP with carbon emission intensity. The results showed that GF and NI can effectively contribute to a reduction in carbon emission intensity, thus verifying the robustness of the results.
4.3 Endogeneity treatment
Although this study attempted to control the factors affecting CEP, the empirical results may still be affected by a number of unobservable factors. To mitigate endogeneity problems due to omitted variables, measurement errors, and reverse causality, this study used instrumental variable estimation methods for endogeneity (Table 6).
(1) GF endogeneity. The degree of financial market sophistication is closely related to GF development, while the number of historical banking institutions can reflect the basis of current financial development, which, in turn, is related to the level of GF development (Zhang et al., 2022d). Considering that a time-varying trend was observed in the development of GF and that the number of banking institutions in history was cross-sectional data, the interaction term of the number of branches of banking institutions in 1935 multiplied by the green coverage rate of each city lagged by three periods was used as an instrumental variable, and the two-stage instrumental variable method was chosen to deal with endogeneity. The selection of instrumental variables must fulfill the two conditions of correlation and exogeneity. The more business outlets banking financial institutions have in each region, the more convenient it is for enterprises to obtain credit. In addition, cities with more business outlets of financial institutions are generally located in economically developed regions, where the government pays more attention to environmental quality, which helps guide credit funds to green and low-carbon development industries and reduces credit fund support for high energy-consuming and high-emission enterprises (Zhang et al., 2022b). Therefore, it is highly correlated with carbon emissions. In addition, the greening coverage of built-up areas reflects the local green awareness; the stronger the green awareness, the smoother the GF development, which satisfies the correlation hypothesis. Moreover, greening coverage is not directly related to pollutants and carbon dioxide emissions, which is also exogenous. Therefore, it satisfies the strict exogeneity of the instrumental variables. The instrumental variable regression results show that GF has a significantly positive effect on CEP, which is consistent with the baseline results.
(2) NI endogeneity. Referring to the study by Zhang et al. (2023a), who adopted traditional infrastructure variables as instrumental variables of NI, this study selected the interaction term between the number of letters sent per capita and the number of Internet broadband users in 2000 as the instrumental variable. The “new” is relative to traditional infrastructure, and its essence is still infrastructure. The number of letters sent represents several elements in the context of traditional infrastructure, such as the level of logistics in each region, the number of staff in the relevant industries, and postal revenue, representing the predecessor of digital infrastructure. The instrumental variable data selected in this paper were 2,000, which is far from the sample data of this study, while the number of letters sent has almost no time effect (Zhang et al., 2023a). Therefore, it had no significant effect on CO2 and satisfied the exogenous condition. The instrumental variable regression results showed that NI had a significant positive effect on CEP, which is consistent with the benchmark results.
In addition, with reference to the study by Hu et al. (2023), this study also selected one-cycle lag GF and NI as instrumental variables. Using data lagged by one cycle, a direct association with the current cycle was ensured while avoiding a direct link with the current cycle variable. This approach is consistent with exogeneity. The regression results in columns (5) and (6) of Table 6 are consistent with those in the previous section.
4.4 Heterogeneity analysis
The impacts of GF and NI on CEP vary across Chinese cities owing to great differences in resource endowment, city size, and geographical location (Zhang et al., 2023b). In this study, we tested the heterogeneous effects of GF and NI on the impact of CEP according to heterogeneous factors, such as geographic location, market maturity, policy support for digital economy development, and type of urban development (Table 7).
(1) Geographic location of cities. Based on the criteria of the National Bureau of Statistics of China for dividing China into three major regions, the sample was divided into eastern, central, and western cities. The regression results are shown in columns (1) and (2) in Table 7. Among these, GF effectively promoted CEP in the eastern, central, and western cities of China. However, by analyzing the size of the regression coefficients, GF is more effective in promoting the CEP of eastern cities. Simultaneously, NI can effectively promote the CEP of the eastern cities, but its effect on the CEP of the central and western cities is not significant. The significance test of the difference in coefficients revealed that the p-values of the regression coefficients of GF and NI among the eastern, central, and western regions were 0.031 and 0.098, respectively, and significant at the 10% and 1% significance levels, respectively. This indicates a significant difference in the regression coefficients among the eastern, central, and western regions. Currently, cities in eastern China have a more complete digital infrastructure and stricter environmental protection measures, better human capital, a strong awareness of low-carbon and environmental protection among residents, and a greater demand for low-carbon products, forcing GF and NI to support the development of low-carbon projects and product production (Zhang et al., 2023b). However, the economic structure of the central and western regions lags behind that of the eastern regions, and traditional industries account for a high proportion; therefore, the influence of GF and NI on urban CEP is still in the primary stage. Moreover, the pressure of economic development is greater in the central and western regions, which are more willing to focus on economic construction than on reducing carbon emissions (Zhang et al., 2023a).
(2) Marketization level. Referring to the marketization index compiled by Fan et al. (2010), based on the median of the index, the sample is divided into cities with a “high level of market intermediary organization development” and cities with a “low level of market intermediary organization development.” GF and NI have a significant positive effect on CEP at a high level of market intermediary organizational development, whereas GF exerts a significant negative effect on CEP at a low level. The effect of the NI on CEP in low markets is not significant. The test of significance of the difference in coefficients showed a significant difference in the regression coefficients between the two groups. The industrial structure and green technology innovation effects of GF and NI are stronger in markets where GF and NI have a more robust market intermediary organization development and are more effective on CEP (Hu et al., 2021). The above study shows that the difference in the development of market intermediary organizations significantly influences the effects of GF and NI on CEP.
(3) Policy support for urban digital economy development. According to China’s smart city “white paper” and big data comprehensive pilot zone division standards, pilot cities are mostly cities with higher administrative levels in each province. Therefore, according to the list of China’s smart city pilot units and the national administrative level, this study defined pilot cities, municipalities, provincial capitals, and sub-provincial cities as cities with policy support for the development of the digital economy and the rest of the cities as ordinary cities. Generally, high-level cities are more active in market economy and infrastructure development than general cities (Zhang et al., 2023b). The results of the grouped regression are shown in columns (4) and (5) of Table 7. Among them, both GF and NI in high-level cities enhanced urban CEP at the 1% significance level, whereas in ordinary cities, GF only enhanced urban CEP at the 10% significance level, and NI did not have a significant effect on urban CEP. The test of significance of the difference in coefficients showed a significant difference in the regression coefficients between the two groups. This is because high-level cities can enjoy more policy favors from the state and are more sensitive to the development of the digital economy, and the green effects of GF and NI are more effective (Zhou et al., 2021).
(4) Urban development types. The economic development of resource-based cities is dominated by industries involved in the extraction and processing of natural resources. According to China’s National Sustainable Development Plan for Resource-Based Cities (2013–2022), the city sample was divided into resource-based and non-resource-based cities. The regression results in columns (8) and (9) show that GF and NI can effectively increase CEP in resource-based cities, whereas in non-resource-based cities, the effects of GF and NI on CEP are not significant. The test of significance of the difference in coefficients showed a significant difference in the regression coefficients between the two groups. This indicates that GF and NI are more effective in enhancing CEP in traditional resource-based cities. Traditional resource-based cities rely on energy-intensive and highly polluting industries related to traditional resources, resulting in high carbon emissions (Che and Wang, 2022). GF and NI play key roles in helping resource-based cities upgrade the existing industrial model, promote transformation and upgrading, and reduce carbon emissions (Bakken, 2019).
4.5 Underlying mechanisms
4.5.1 Technological innovation
The technological innovation (TI) effect refers to the use of digital technologies, such as Internet technology, big data analysis, and artificial intelligence, to innovate and optimize the industrial development model (Zhao et al., 2023). This effect can be used in production and life to promote the target subject to carry out green-based technological innovation, which, in turn, improves the resource utilization rate to reduce the pollution emission in production and life or improves the pollutant treatment technology and efficiency, which, in turn, improves CEP (Xie et al., 2021). In this study, the level of green technological innovation was expressed and specifically measured by the ratio of the number of granted green patents to the total number of patent applications in the year (Zhang et al., 2023a). The regression results are presented in Table 8.
Column (1) shows that green technological innovation had a significantly positive effect on CEP. Column (2) shows that the GF and green technological innovation cross-multiplier term were significant at the 1% level, indicating that GF can strengthen the enhancement effect of green technological innovation on CEP, which is consistent with the theoretical analysis in the previous section. Column (3) shows that the estimated coefficient of NI and the green technological innovation cross-multiplier term were significantly positive at the 5% significance level, which indicates that NI is an important moderating variable of green technological innovation affecting CEP. The regression coefficient of GF × NI × TI in column (4) was also significantly positive at the 5% significance level, and combined with the positive regression results of green technological innovation and CEP in column (1), it can be observed that the construction of GF and NI can jointly promote CEP enhancement through the green technological innovation channel. In addition, comparing the size of the regression coefficients of the TI term in column (1) and the GF × NI × TI term in column (4), we found that the regression coefficients of the latter term were large, indicating that the synergistic effects of GF and NI significantly enhanced CEP promotion through green technological innovation. These results are consistent with hypotheses 1a and 1b.
4.5.2 Industrial structure upgrading
Industrial upgrading (IU) implies that highly polluting and energy-consuming industries will be eliminated, strategic emerging industries and modern service industries will be accelerated, pollutant emissions such as carbon dioxide and soot in production will be reduced, and production and consumption patterns will become more sustainable (Gu et al., 2021). Specifically, the industrial upgrading index is calculated by assigning weights of 1, 2, and 3 to primary, secondary, and tertiary industries, respectively. Higher values indicate more significant industrial upgrades (Zhang et al., 2023a). Table 9, result (1), shows that upgrading the industrial structure can effectively promote CEP. Result (2) shows that GF is an important moderating variable in industrial structure upgrades that affect the CEP enhancement. Regression result (3) shows that NI is an important moderating variable in industrial structure upgrading to enhance CEP. Regression result (4) shows the regression results of the cross-multiplier terms GF, NI, and industrial structure upgrading, showing that the three cross-multiplier terms are significantly positively related to CEP. Combined with the positive regression results of industrial structure upgrading and CEP in column (1), it can be observed that GF and NI can synergistically promote industrial structure upgrading and then promote CEP. Comparing the significance level and size of the regression coefficients of the IU term in column (1) and the GF × NI × IU term in column (4) showed that the regression coefficients of the cross-multiplier term are large, indicating that the synergistic effect of the construction of GF and NI significantly strengthens the promotion effect of industrial structure upgrading on CEP. These results are consistent with hypotheses 2a and 2b.
4.5.3 Resource allocation
The reallocation of resource factors, such as labor, capital, and technology, can effectively improve the efficiency of resource use and the economic cycle, reduce the production of pollutant emissions, and enhance CEP (Jiang et al., 2020). Zhang et al. (2019) reported that the optimization of resource allocation (RA) is characterized using the total factor productivity calculated from urban employment and fixed asset investment. Column (1) of Table 10 shows that resource allocation effectively enhanced CEP. Result (2) shows that the GF is an important moderating variable for resource allocation optimization that influences CEP enhancement. Similarly, result (3) shows that NI was a significant moderating variable for resource allocation optimization to enhance CEP. Result (4) is the regression result of the cross-multiplier terms of GF, NI, and RA, which shows that the cross-multiplier terms of the three were significantly positively related to CEP. Combined with the positive regression results of resource allocation optimization and CEP in column (1), it can be observed that GF and NI can synergistically promote the optimization of resource allocation and then promote the improvement of CEP. Comparing the significance level and size of the regression coefficients of the RA term in column (1) and the GF × NI × RA term in column (4) showed that the regression coefficients of the cross-multiplication term were large, indicating that the synergistic effects of GF and NI significantly enhance the promotion of resource allocation optimization on CEP. These results are consistent with hypotheses 1c and 2c.
4.6 Spatial interaction spillover effects of GF, NI, and CEP
In this study, we used the spatial system of simultaneous equation model (3) and investigated the spatial interaction spillover effects among the three using the generalized three-stage least squares (GS3SLS) method, which is used to estimate the overall spatial and considers the spatial correlation and possible correlation between the stochastic perturbation terms of the endogenous variable potential equations, which improves the validity of the estimation results (Rey and Boarnet, 2004).
A spatial correlation test was conducted using the Moran index with a spatial geoeconomic matrix, and the test results confirmed the spatial correlations among GF, NI, and CEP. As shown in Table 11, GF, NI, and CEP had significant spatial interaction spillover effects. The specific results are as follows: regression result (1) shows that local GF and NI can effectively enhance the CEP of local and neighboring cities. However, the effect of both on local CEP was larger than that on neighboring cities. Successful GF programmers and convenient and low-cost NI facilitate the exchange of environmental governance experience and technical cooperation between neighboring cities (Rissman et al., 2020). GF and NI expand the geographical flow boundaries of the factors of production materials, improve the efficiency of resource utilization by market-based financial regulation and remote monitoring, effectively promote the dissemination of green, low-carbon, and environmental protection awareness, and increase CEP through joint regional environmental prevention and control (Zhang et al., 2022d; Zhang et al., 2023a; Zhang et al., 2024). Local CEP is equally effective in enhancing neighboring CEP.
Regression results (2) show that both local CEP and NI can effectively promote the development of local GF and neighboring GF and that the effect of both on local GF is equal to that on neighboring GF. The enhancement of CEP is in line with the concept of green development in China and, thus, can further promote the development of GF, effectively supporting green and low-carbon development. The NI provides information about digital infrastructure support and services for GF development and, therefore, can also effectively promote the development of GF. Forced by the competitive pressure of governmental environmental performance assessments, local governments actively develop GF, which can also promote its development in the surrounding neighboring cities (Zhang et al., 2022a). Simultaneously, the local development of NI and the spread of the digital economy to neighboring cities cause a spillover of green development resources and environmental protection technologies, which can likewise promote the development of GF in neighboring cities. Local GF also significantly promotes GF development in neighboring cities.
Regression result (3) shows that the local CEP can effectively promote local and neighboring NI construction, and the effect on local NI is greater than that on neighboring land. Local GF significantly promotes local NI but inhibits neighboring NI construction. GF provides a large amount of funds for NI construction, but in the case of a limited total amount of funds, more green investment received locally will affect the scale of green funds invested in neighboring areas through the crowding-out effect (Liu et al., 2022a). Benefiting from the benefits of NI, neighboring governments will develop NI vigorously by mimicking a competitive effect (Zhang et al., 2023a). The above findings are also in line with previous research hypothesis H4.
4.7 Discussion
Previous studies have mainly focused on examining the impact of GF and NI on carbon emission separately (Zhang et al., 2022d; Zhang et al., 2023a). With the advent of the digital economy, the development of GF cannot be separated from the support of digital technology, although it can provide for the construction of NI. The construction of the NI provides a hardware foundation for the progress of digital technology, which, in turn, provides technical support for GF development. Therefore, it is important to explore whether there is a superimposed effect on the carbon emission impacts of GF and NI. If this effect exists, what is their common mechanism for effacing carbon emission? Is there a heterogeneous character in regard to the impact of both on carbon emission? However, these questions remain unanswered in previous studies.
Based on existing studies, this study characterized carbon emission levels by CEP and comprehensively measured the levels of GF and NI. Using the panel data fixed effects model, it was found that GF and NI can not only enhance CEP separately but also have a superimposed effect on each other, which further enhances CEP. Green technology innovation, industrial structure upgrading, and resource allocation optimization are common paths for both GF and NI to promote CEP. The impacts of GF and NI on CEP are heterogeneous in terms of the geographic location, level of marketization, policy support for the development of the digital economy, and type of urban development. Further research found that there are also spatial interaction spillover effects among GF, NI and CEP.
Although this study quantitatively analyzed how GF and NI influence CEP and provided novel insights, it is important to acknowledge the inherent limitations of our study. For example, we used a range of approaches to avoid the effects of endogeneity, and the instrumental variable approach to endogeneity testing selected separate instrumental variables for GF and NI. If common instrumental variables of GF and NI can be identified in future research, this will make the conclusion of the synergistic impact of the two more reliable. In addition, this study first used a panel data fixed-effects model and then considered the spatial spillover effect. In future research, we will further discuss the spatial effects of the synergy between GF and NI and include factors such as urban distance. Finally, it is imperative to recognize that the generalizability of our results may be constrained by specific country-level features within the sample. Further research is necessary to evaluate the applicability of these findings in a diverse range of contexts.
Despite these limitations, our study has important implications for promoting low-carbon, green development in Chinese economy. The insights provided are valuable for policymakers and researchers. In addition, this study is of theoretical and demonstrative significance, given that energy transition requires substantial financial and technical support. This not only re-validates the green effect of carbon emission reduction of GF and NI but also demonstrates the synergistic impact between them. This study showed that the GF and NI can promote each other to further deepen their ability to improve CEP. In addition, the study further finds that an improvement in urban CEP has an impact on urban GF and NI, which provides theoretical guidance and policy support for urban green development, financial policy formulation, and NI construction.
5 Conclusion and policy recommendations
5.1 Main conclusion
In this study, the intrinsic relationships among GF, NI, and CEP were investigated using panel data from 285 Chinese cities from 2011 to 2021. The following main conclusions were drawn: (1) GF and NI can not only effectively promote the level of CEP but also synergistically enhance CEP through the superposition effect and mutual reinforcement; (2) heterogeneity analysis shows that GF can effectively promote CEP in all regions of China, high marketization levels, high administrative levels, and resource-based cities, while NI can effectively promote CEP in the east, high marketization levels, high administrative levels, and resource-based cities; (3) green technological innovation, industrial structure upgrading, and resource allocation optimization are important common paths for GF and NI to enhance CEP; (4) local GF can effectively promote local CEP and NI and neighboring CEP and inhibit the surrounding neighboring NI, while NI construction can effectively promote local and neighboring CEP and GF. Simultaneously, CEP can promote local GF and NI and the GF and NI of surrounding neighboring cities. However, the effect of the above objects on the local area is greater than the effect on the surrounding neighboring cities.
5.2 Policy recommendations
The development of GF markets should be continued. We should continue to improve the GF market, establish a sound legal and regulatory system for GF, establish effective incentive mechanisms, and guide financial resources toward green and low-carbon projects. The use of digital and Internet technologies should be promoted to actively innovate carbon finance products.
This further strengthens the construction of new infrastructure, increasing investment in new infrastructure, accelerating the deep integration of new infrastructure with various industries, and fully utilizing policy guidance to ensure the stable development of new infrastructure. We should pay attention to regional development differences, strengthen interregional connections, actively cooperate in infrastructure construction with neighboring regions, and promote the sharing of cross-regional infrastructure equipment and resources.
Continue to deepen cooperation and development between GF and NI, with a focus on regional cooperation and promoting technological innovation, upgrading industrial structure, and optimizing resource allocation of enterprises through the synergy effect between the two. Strengthen the cultivation of consumer awareness of green consumption, promoting green and low-carbon lifestyles.
Data availability statement
The raw data supporting the conclusion of this article will be made available by the authors, without undue reservation.
Author contributions
HL: writing–original draft and writing–review and editing. ZY: data curation and writing–original draft. JA: data curation and writing–original draft.
Funding
The author(s) declare financial support was received for the research, authorship, and/or publication of this article. This research was funded by the Soft Science Research Program of Henan Province (20231466); the Decision Research Bidding Project of Henan Province (2023JC011); the Key Research Projects for Higher Education Institutions of Henan Province (24B790023); the 2023 Training Program for Young Backbone Teachers of Xinyang Normal University; the Research Topics of Henan Provincial Federation of Social Sciences (SKL-2023-567); and the Postgraduate Education Reform and Quality Improvement Project of Henan Province (YJS2022JD30).
Conflict of interest
The authors declare that the research was conducted in the absence of any commercial or financial relationships that could be construed as a potential conflict of interest.
Publisher’s note
All claims expressed in this article are solely those of the authors and do not necessarily represent those of their affiliated organizations, or those of the publisher, the editors, and the reviewers. Any product that may be evaluated in this article, or claim that may be made by its manufacturer, is not guaranteed or endorsed by the publisher.
References
Bakken, B. E. (2019). Energy transition dynamics: does participatory modelling contribute to alignment among differing future world views? Syst. Res. Behav. Sci. 36 (2), 184–196. doi:10.1002/sres.2578
Che, S., and Wang, J. (2022). Can environmental regulation solve the carbon curse of natural resource dependence: evidence from China. Resour. Policy 79, 103100. doi:10.1016/j.resourpol.2022.103100
Du, X., Zhang, H., and Han, Y. (2022). How does new infrastructure investment affect economic growth quality? Empirical evidence from China. Sustainability 14 (6), 3511. doi:10.3390/su14063511
Gu, B., Chen, F., and Zhang, K. (2021). The policy effect of green finance in promoting industrial transformation and upgrading efficiency in China: analysis from the perspective of government regulation and public environmental demands. Environ. Sci. Pollut. Res. Int. 28, 47474–47491. doi:10.1007/s11356-021-13944-0
Hasman, A., Samartín, M., and van Bommel, J. (2014). Financial intermediation in an overlapping generations model with transaction costs. J. Econ. Dyn. Control 45, 111–125. doi:10.1016/j.jedc.2014.05.012
Hintemann, R. (2020). Efficiency gains are not enough: data center energy consumption continues to rise significantly. Borderstep Inst. für Innov. Nachhalt. gGmbH.
Hu, G., Wang, X., and Wang, Y. (2021). Can the green credit policy stimulate green innovation in heavily polluting enterprises? Evidence from a quasi-natural experiment in China. Energy Econ. 98, 105134. doi:10.1016/j.eneco.2021.105134
Hu, J., Zhang, H., and Irfan, M. (2023). How does digital infrastructure construction affect low-carbon development? A multidimensional interpretation of evidence from China. Clean. Prod. 396, 136467. doi:10.1016/j.jclepro.2023.136467
Jiang, H., Wang, W., Wang, L., and Wu, J. (2020). Research on the carbon emission reduction effect of green financial development in China—Taking green credit and green venture capital as examples. Financ. Forum (11), 39–48+80. doi:10.16529/j.cnki.11-4613/f.2020.11.006
Li, J., and Li, S. (2020). Energy investment, economic growth and carbon emissions in China-Empirical analysis based on spatial Durbin model. energy policy 140, 111425. doi:10.1016/j.enpol.2020.111425
Liu, H., and He, Q. (2019). The effect of basic public service on urban-rural income inequality: a sys-GMM approach. Econ. research-Ekonomska istraživanja 32 (1), 3211–3229. doi:10.1080/1331677x.2019.1661005
Liu, J., Yu, Q., Chen, Y., and Liu, J. (2022a). The impact of digital technology development on carbon emissions: a spatial effect analysis for China. Resour. Conservation Recycl. 185, 106445. doi:10.1016/j.resconrec.2022.106445
Liu, Z., Deng, Z., He, G., Wang, H., Zhang, X., Lin, J., et al. (2022b). Challenges and opportunities for carbon neutrality in China. Nat. Rev. Earth Environ. 3 (2), 141–155. doi:10.1038/s43017-021-00244-x
Martínez, J. M. G., Puertas, R., Martín, J. M. M., and Ribeiro-Soriano, D. (2022). Digitalization, innovation and environmental policies aimed at achieving sustainable production. Sustain. Prod. Consum. 32, 92–100. doi:10.1016/j.spc.2022.03.035
Mengze, H., and Wei, L. (2015). A comparative study on environment credit risk management of commercial banks in the Asia-Pacific region. Bus. Strategy Environ. 24 (3), 159–174. doi:10.1002/bse.1810
Nudurupati, S. S., Budhwar, P., Pappu, R. P., Chowdhury, S., Kondala, M., Chakraborty, A., et al. (2022). Transforming sustainability of Indian small and medium-sized enterprises through circular economy adoption. J. Bus. Res. 149, 250–269. doi:10.1016/j.jbusres.2022.05.036
Raheem, I. D., Tiwari, A. K., and Balsalobre-Lorente, D. (2020). The role of ICT and financial development in CO2 emissions and economic growth. Environ. Sci. Pollut. Res. 27 (2), 1912–1922. doi:10.1007/s11356-019-06590-0
Ren, S., Hao, Y., Xu, L., Wu, H., and Ba, N. (2021). Digitalization and energy: how does internet development affect China's energy consumption? Energy Econ. 98, 105220. doi:10.1016/j.eneco.2021.105220
Rey, S. J., and Boarnet, M. G. (2004). “A Taxonomy of Spatial Econometric Models for Simultaneous Equations Systems,” in Advances in Spatial Econometrics. Advances in Spatial Science. Editors L. Anselin, R. J. G. M. Florax, and S. J. Rey (Springer, Berlin: Heidelberg). doi:10.1007/978-3-662-05617-2_5
Rissman, J., Bataille, C., Masanet, E., Aden, N., Morrow, W. R., Zhou, N., et al. (2020). Technologies and policies to decarbonize global industry: review and assessment of mitigation drivers through 2070. Appl. energy 266, 114848. doi:10.1016/j.apenergy.2020.114848
Song, M., Xie, Q., and Shen, Z. (2021). Impact of green credit on high-efficiency utilization of energy in China considering environmental constraints. Energy Policy 153, 112267. doi:10.1016/j.enpol.2021.112267
Soundarrajan, P., and Vivek, N. (2016). Green finance for sustainable green economic growth in India. Agric. Econ. 62 (1), 35–44. doi:10.17221/174/2014-agricecon
Tang, K., and Yang, G. (2023). Does digital infrastructure cut carbon emissions in Chinese cities? Sustain. Prod. Consum. 35, 431–443. doi:10.1016/j.spc.2022.11.022
Tone, K., Toloo, M., and Izadikhah, M. (2020). A modified slacks-based measure of efficiency in data envelopment analysis. Eur. J. Operational Res. 287 (2), 560–571. doi:10.1016/j.ejor.2020.04.019
Wang, H., Li, Y., Lin, W., and Wei, W. (2023). How does digital technology promote carbon emission reduction? Empirical evidence based on e-commerce pilot city policy in China. Environ. Manag. 325, 116524. doi:10.1016/j.jenvman.2022.116524
Wang, X., and Wang, Q. (2021). Research on the impact of green finance on the upgrading of China's regional industrial structure from the perspective of sustainable development. Resour. Policy 74, 102436. doi:10.1016/j.resourpol.2021.102436
Xie, Z., Wu, R., and Wang, S. (2021). How technological progress affects the carbon emission efficiency? Evidence from national panel quantile regression. J. Clean. Prod. 307, 127133. doi:10.1016/j.jclepro.2021.127133
Xu, X., Watts, A., and Reed, M. (2019). Does access to internet promote innovation? A look at the US broadband industry. growth change 50 (4), 1423–1440. doi:10.1111/grow.12334
Yang, Z., Gao, W., Han, Q., Qi, L., Cui, Y., and Chen, Y. (2022). Digitalisation and carbon emissions: how does digital city construction affect China's carbon emission reduction? Sustain. cities Soc. 87, 104201. doi:10.1016/j.scs.2022.104201
Zhang, A., Deng, R., and Wu, Y. (2022a). Does the green credit policy reduce the carbon emission intensity of heavily polluting industries? Evidence from China’s industrial sectors. J. Environ. Manag. 311, 4815, 114815. doi:10.1016/j.jenvman.2022.114815
Zhang, J., Wu, Q., and Zhou, Z. (2019). A two-stage DEA model for resource allocation in industrial pollution treatment and its application in China. J. Clean. Prod. 228, 29–39. doi:10.1016/j.jclepro.2019.04.141
Zhang, W., Fan, H., and Zhao, Q. (2023a). Seeing green: how does digital infrastructure affect carbon emission intensity? Energy Econ. 127, 107085. doi:10.1016/j.eneco.2023.107085
Zhang, W., Liu, X., Liu, J., and Zhou, Y. (2022b). Endogenous development of green finance and cultivation mechanism of green bankers. Environ. Sci. Pollut. Res. 29, 15816–15826. doi:10.1007/s11356-021-16933-5
Zhang, W., Liu, X., Wang, D., and Zhou, J. (2022c). Digital economy and carbon emission performance: evidence at China's city level. Energy Policy 165, 112927. doi:10.1016/j.enpol.2022.112927
Zhang, W., Liu, X., Zhao, S., and Tang, T. (2024). Does green finance agglomeration improve carbon emission performance in China? A perspective of spatial spillover. Appl. Energy 358, 122561. doi:10.1016/j.apenergy.2023.122561
Zhang, W., Zeng, M., Zhang, Y., and Su, C. W. (2023b). Reducing carbon emissions: can high-speed railway contribute? J. Clean. Prod. 413, 137524. doi:10.1016/j.jclepro.2023.137524
Zhang, W., Zhu, Z., Liu, X., and Cheng, J. (2022d). Can green finance improve carbon emission efficiency? Environ. Sci. Pollut. Res. 29 (45), 68976–68989. doi:10.1007/s11356-022-20670-8
Zhao, M., and Sun, T. (2022). Dynamic spatial spillover effect of new energy vehicle industry policies on carbon emission of transportation sector in China. Energy Policy 165, 112991. doi:10.1016/j.enpol.2022.112991
Zhao, Y., Zhao, Z., Qian, Z., Zheng, L., Fan, S., and Zuo, S. (2023). Is cooperative green innovation better for carbon reduction? Evidence from China. J. Clean. Prod. 394, 136400. doi:10.1016/j.jclepro.2023.136400
Zhou, X., Cai, Z., Tan, K. H., Zhang, L., Du, J., and Song, M. (2021). Technological innovation and structural change for economic development in China as an emerging market. Technol. Forecast. Soc. Change 167, 120671. doi:10.1016/j.techfore.2021.120671
Keywords: green finance, new infrastructure, carbon emission performance, synergistic effect, spatial interaction spillovers
Citation: Li H, Yang Z and An J (2024) Green finance, new infrastructure, and carbon emission performance in Chinese cities. Front. Environ. Sci. 12:1352869. doi: 10.3389/fenvs.2024.1352869
Received: 09 December 2023; Accepted: 13 February 2024;
Published: 28 February 2024.
Edited by:
Wei Zhang, China University of Geosciences Wuhan, ChinaReviewed by:
Jinkai Zhao, Shandong University of Science and Technology, ChinaMingbo Zheng, Chang’an University, China
Copyright © 2024 Li, Yang and An. This is an open-access article distributed under the terms of the Creative Commons Attribution License (CC BY). The use, distribution or reproduction in other forums is permitted, provided the original author(s) and the copyright owner(s) are credited and that the original publication in this journal is cited, in accordance with accepted academic practice. No use, distribution or reproduction is permitted which does not comply with these terms.
*Correspondence: Hao Li, eHlsaWhhb0B4eW51LmVkdS5jbg==