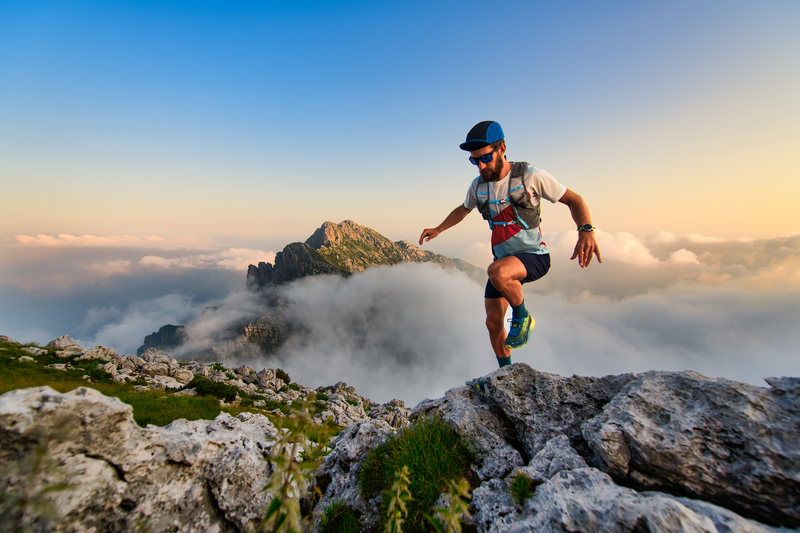
95% of researchers rate our articles as excellent or good
Learn more about the work of our research integrity team to safeguard the quality of each article we publish.
Find out more
ORIGINAL RESEARCH article
Front. Environ. Sci. , 01 February 2024
Sec. Land Use Dynamics
Volume 12 - 2024 | https://doi.org/10.3389/fenvs.2024.1338512
Water resource management requires a thorough examination of how land use and climate change affect streamflow; however, the potential impacts of land-use changes are frequently ignored. Therefore, the principal goal of this study is to isolate the effects of anticipated climate and land-use changes on streamflow at the Indus River, Besham, Pakistan, using the Soil and Water Assessment Tool (SWAT). The multimodal ensemble (MME) of 11 general circulation models (GCMs) under two shared socioeconomic pathways (SSPs) 245 and 585 was computed using the Taylor skill score (TSS) and rating metric (RM). Future land use was predicted using the cellular automata artificial neural network (CA-ANN). The impacts of climate change and land-use change were assessed on streamflow under various SSPs and land-use scenarios. To calibrate and validate the SWAT model, the historical record (1991–2013) was divided into the following two parts: calibration (1991–2006) and validation (2007–2013). The SWAT model performed well in simulating streamflow with NSE, R2, and RSR values during the calibration and validation phases, which are 0.77, 0.79, and 0.48 and 0.76, 0.78, and 0.49, respectively. The results show that climate change (97.47%) has a greater effect on river runoff than land-use change (2.53%). Moreover, the impact of SSP585 (5.84%–19.42%) is higher than that of SSP245 (1.58%–4%). The computed impacts of climate and land-use changes are recommended to be incorporated into water policies to bring sustainability to the water environment.
Global warming has significant impacts on meteorological parameters, which have changed the river flow in south Asian countries (Khan et al., 2022a; Khan M. et al., 2022; Singh et al., 2023). Climate change has profound impacts on the water resources of Pakistan (Kiran et al., 2023). The study conducted by Khan et al. (2022c) found that a 10% change in meteorological parameters can alter 10%–35% of river flow in 75% watersheds of Pakistan. Furthermore, Rizwan et al. (2023a) found that future streamflow will increase in Pakistan’s Kabul and the Upper Indus Basin under several climate change scenarios, including RCP2.6, RCP4.5, and RCP8.5. According to projections made by Almazroui et al. (2020), under various shared socioeconomic pathway (SSP) scenarios, the annual mean precipitation and temperature over Pakistan are expected to increase by 1.4°C–4.9°C and 26.4 (6.4–159.7)%, respectively, by the end of the 21st century. The aforesaid alteration in meteorological parameters might change streamflow and make water management more difficult. In order to formulate a water policy in the context of climate change, it is vital to investigate the underlying hydrological dynamics occurring in the basin under various SSP scenarios.
According to the Sixth Intergovernmental Panel on Climate Change (IPCC) Assessment Report, the climate change impacts will become severe in the future due to the combined impacts of global warming and socioeconomic changes. According to Amiri et al. (2023), climate change appears to be more dangerous to the sustainability of water supplies. Pakistan’s agriculture-based economy depends on surface water (Haleem et al., 2022). The Indus River system irrigates Pakistan’s most fertile region (Khan M. et al., 2022). Water is essential for both the country’s food security and economic development, both of which rely on an acceptable and appropriate water supply (Wisal et al., 2020). Water resources are under tremendous stress because of their continually expanding population. Pakistan’s per capita water availability has declined over time, owing to the combined effects of the growing population and declining river runoff and reservoir storage capacity (Haleem et al., 2023a). Therefore, it is important to investigate the impacts of climate and land-use changes on streamflow to bring sustainability to the water environment.
The Upper Indus Basin (UIB) is the largest irrigation system in Pakistan. For several decades, the region has faced severe threats from climate and land-use changes. The river flow in the UIB is affected by a variety of factors including seasonal snowpack melting, glacier melting, and precipitation (Khan et al., 2020). The erratic summertime and particularly wintertime variations in maximum and minimum temperatures have accelerated glacier melting. As a result, streamflow has increased, posing problems for the residents of the UIB (Bilal et al., 2021). Mountainous ecosystems are vulnerable to the influence of land use and climate change. The risks linked with increasing temperature in mountainous regions, particularly in areas like Gilgit Baltistan, which is a glacier-covered territory, will amplify in the future (Shahid et al., 2021). Therefore, it is necessary to assess the impacts of climate change on river flow to bring sustainability to the water environment.
A series of studies conducted by various researchers assessed the impact of climate change on streamflow. Shahid et al. (2021) evaluated the impacts of climate change on surface runoff and found that the runoff will significantly increase in contrast to the base period. Mahmood and Jia (2016) assessed the effects of climate change on streamflow and found a 10%–15% increase in the mean annual flow in the transboundary Jhelum River Basin of Pakistan and India. Usman et al. (2021) assessed the impacts of climate change on streamflow under various RCP scenarios, and Shahid et al. (2018) found that streamflow will decrease in the Soan River Basin, Pakistan. Anjum et al. (2019) found that climate change will enhance the annual average flow under various RCP scenarios. Mahmood et al. (2016) assessed the impacts of climate change on streamflow in the Kunhar River Basin, Pakistan, and found an overall increase in the mean annual flow. Moreover, Ullah et al. (2023) and Khan M. et al. (2023) assessed the impacts of climate change on the streamflow under various SSP scenarios in the UIB using machine learning techniques and found an increase in futuristic streamflow. Chathuranika et al. (2022) and Karunanayake et al. (2020) evaluated the impacts of climate change on streamflow and found that streamflow will increase under various RCP scenarios in the future. The above discussion shows that researchers only investigated the impacts of climate change on streamflow. Therefore, it is necessary to estimate the impacts of climate and land-use changes on surface runoff under various SSPs and land-use scenarios. Within the larger context of climate change and its effects on river flow, particularly in Pakistan, this study tries to fill a significant knowledge gap by examining the comparative impact of climate change and land-use change on river runoff under various SSPs and land-use scenarios.
The main objectives of this study were to project streamflow in the upper regions of the Indus Basin using CMIP6-based general circulation models (GCMs) and investigate the effects of climate change and land-use change on the streamflow. This will help policymakers in formulating a water policy in the understudy area.
This study is carried out to assess the impacts of climate change and land-use change on streamflow of the Indus River, which passes through Pakistan, India, China, and Afghanistan. Originating on the Tibetan Plateau and falling into the Arabian Sea, the Indus River is the longest river in Asia. Pakistan encompasses a significant portion of the Indus River Basin, whereas Afghanistan has the smallest segment. Geographically, the river lies between latitudes 32.48 and 37.07 N and longitudes 67.33 and 81.83 E. The intended extent of this research is the upper section of the Indus River at Besham, Pakistan, as illustrated in Figure 1.
FIGURE 1. Study area, DEM, land use, and soil map. (A) shows monitoring stations (B) subbasins and river (C) land use classes (D) soil classes.
The digital elevation model (DEM) was sourced from the National Aeronautics and Space Administration (NASA) for the designated study area, accessible at https://earthdata.nasa.gov/. The DEM data, having a resolution of 30 m × 30 m, were used for watershed delineation. The land-use data were sourced from the United States Geographical Survey (USGS) using the moderate resolution imaging spectroradiometer (MODIS), available at http://www.earthexplorer.usgs.gov/. The soil data were acquired from the Food and Agriculture Organization (FAO) website, accessible at http://www.fao.org/. The details of DEM, land use, and soil data are shown in Figure 1.
Meteorological and streamflow data, spanning from 1990 to 2013, were obtained from the Pakistan Meteorological Department and the Water and Power Development Authority, respectively. The meteorological parameter data on 11 GCMs, namely, INM-CM4-8 (Russia), INM-CM5-0 (Russia), NESM3 (China), EC-Earth3-Veg-LR (Europe), GFDL-ESM4 (United States), CMCC-ESM2 (Italy), CNRM-CM6-1 (France), CNRM-ESM2-1 (France), MIROC6 (Japan), MRI-ESM2-0 (Japan), and MPI-ESM1-2-LR for SSP245 and SSP585 were downloaded from the online CMIP6 repository.
The detailed methodology of the present study is demonstrated by Figure 2.
Initially, data were prepared for the Soil and Water Assessment Tool (SWAT) model, which includes bias correction, multimodal ensemble (MME) estimation, and land-use prediction. The aforementioned data are utilized in isolating climate change impacts from land use on streamflow.
Bias correction is an important step in climate modeling, especially when there are large differences between simulated and observed data. Bias correction is used to bridge the gap between GCMs and observed data via transformation algorithms. This step is necessary in improving future GCM estimates (Nguyen et al., 2020). There are many bias correction techniques, namely, delta change correction (additive and multiplicative), linear scaling (additive and multiplicative), power transformation of precipitation, precipitation local intensity scaling, distribution mapping of precipitation and temperature, and variance scaling of temperature (Nguyen et al., 2020; Haleem et al., 2022). In a specific case study, Worku et al. (2020) noted that bias correction effectively aligns the GCM data with the observed data, further underscoring the significance of bias correction in enhancing the reliability of climate projections. In this study, the linear scaling technique was used for bias correction. The linear scaling method has notable advantages in terms of simplicity and efficiency compared with statistical or dynamic scaling techniques (Fang et al., 2015).
The ensemble computation of GCMs is a common technique used to improve the accuracy of climate predictions. In the present project, the performance of the GCM ensembles was computed using the Taylor skill score (TSS) and rating metric (RM) techniques (Dey et al., 2022; Shetty et al., 2023). First, the TSS was computed using meteorological parameters observed and GCM data via Equation (1):
The equation is based on the ratio of the standard deviation of a GCM to the standard deviation of observed data, as well as the highest correlation coefficient value (which is equal to 1), expressed as R0. The TSS value ranges between 0 and 1. A TSS score of 1 implies that a particular GCM excels in replicating the observed data.
Second, the RM is estimated for each GCM meteorological parameter based on their TSS rankings. The GCMs are then ranked collectively by averaging the RM values for each meteorological parameter. The RM value varies between 0 and 1, with a value closer to 1 indicating superior GCM performance and a value closer to 0 indicating worse performance.
where i is the rank of the particular GCM based on the TSS and n is the total number of CMIP6 GCMs.
Land use was predicted via the Model of Land Use Change and its Effects (MOLUSCE), a QGIS plugin, using the cellular automata artificial neural network (CA-ANN) algorithm. First, the model was trained to predict specific years of land use. Second, the predicted land use was compared with NASA MODIS land use. The model performance was assessed via statistical performance indicators, namely, kappa value and accuracy. After satisfactory performance, future land use for 2030, 2040, and 2050 was predicted. The predicted land use was used to assess land-use impacts on streamflow.
This section describes the SWAT model, calibration and validation procedures, and model performance assessment via statistical performance indicators.
The SWAT is a semi-distributed hydrological model that evaluates the complex interactions among terrestrial and meteorological parameters at the watershed scale (Yi and Sophocleous, 2011). This tool has gained increasing recognition in recent years, particularly for its effectiveness in predicting the effects of meteorological parameters, various land-use patterns, and soil conditions on streamflow (Gassman et al., 2014). This model necessitates a minimum number of input variables, including terrestrial data (including DEM, land use, and soil) and daily meteorological data (precipitation, temperature, wind speed, and solar radiation) (Ficklin and Barnhart, 2014; Wisal et al., 2020). The SWAT model is based on the principle of the water cycle, as demonstrated by Equation 3:
where SWt represents the final soil moisture content, mm; SW0 represents the initial soil moisture content of the ith day, mm; t represents the time, d; Rday represents the precipitation of the ith day, mm; Qsurf represents the surface runoff of day i, mm; Ea represents the amount of evapotranspiration on day i, mm; Wseep represents the amount of water entering the vale zone from the soil profile on day i, mm; and Qgw represents the return flow amount on day i, mm.
During the calibration process, model parameters were adjusted to ensure that the observed streamflow matched the simulated streamflow data. Fine-tuning was carried out in SWAT calibration and uncertainty procedures (CUPs) using optimization algorithms, namely, sequential uncertainty fitting (SUFI-2) (Shang et al., 2019). The SUFI-2 algorithm is based on 11 objective functions. In the current study, the Nash–Sutcliffe efficiency (NSE) was used as the objective function as it captures temporal dynamics, which is a crucial aspect of hydrologic model calibration (Khan S. et al., 2023). The model performance was assessed using statistical performance indicators, namely, the NSE, coefficient of determination (R2), the root mean squared error (RSR), and percentage error (PBIAS). The ideal number for RSR and PBIAS is zero, but for R2 and NSE, it is one. The 2/3 data are used for model calibration and 1/3 for model validation. The SWAT-CUP model was calibrated using monthly data from 1991 to 2006. After successful calibration, the model was validated using monthly data from 2007 to 2013. The model performance was assessed as per the criteria presented in Table 1.
The SWAT model was used to isolate the land use and climate change impacts on streamflow. The intercomparison of the observed and simulated streamflow data was carried out using preset land use and climate scenarios. In this study, four scenarios were prepared; scenarios 1 and 3 are related to land-use change impacts on river runoff, while scenarios 2 and 4 are related to the climate change impacts on river runoff, as shown in Table 8. The influence of climate change and land use was estimated via Equations (8) and (9), respectively (Shang et al., 2019).
where Q1, Q2, Q3, and Q4 represent the average streamflow.
Hypothetically, ∆Q is equal to ∆QM.
ηC represents the impacts of climate change on streamflow.
ηL represents the impacts of land use on streamflow.
In this study, we used a linear scaling correction method, encompassing both addition and multiplication, using the CMhyd tool (Worku et al., 2020). The model performance was assessed via statistical performance indicators, namely, R2, NSE, and RSR (Haleem et al., 2022). The R2, NSE, and RSR values for temperature and precipitation are 0.81, 0.85, and 0.35 and 0.66, 0.74, and 0.48 for SSP245, and 0.87, 0.82, and 0.31 and 0.76, 0.68, and 0.45 for SSP585, respectively, as presented in Table 2.
The MME was used to improve the performance of GCM simulations. The best combination of GCMs (SSP245 and SSP585) for the computation of MMEs was found via the TSS and RM, as shown in Figure 3. For SSP245, six GCMs, namely, NESM3 (China), CNRM-CM6-1 (France), CNRM-ESM2-1 (France), GFDL-ESM4 (United States), MIROC6 (Japan), and MRI-ESM2-0 (Japan) exceeded the target value which highlights their similarity with the observed data. These models were used for MME computation. Similarly, for SSP585, seven GCMs, namely, NESM3 (China), CNRM-CM6-1 (France), CNRM-ESM2-1 (France), GFDL-ESM4 (United States), MIROC6 (Japan), CMCC-ESM2, and MRI-ESM2-0 (Japan), crossed the target value. These models were later used for MME estimation. A simple arithmetic mean approach was used for MME estimation. The MME estimation reduces the biases and uncertainties of individual models and uses the collective predictive power of the selected models. The complete detail of GCMs is presented in Table 3.
Table 4 demonstrate an increase in average precipitation for all scenarios and time periods, with SSP245 witnessing a 5.43% increase from 2015 to 2037 and a significant 9.7% increase from 2038 to 2054. SSP 585, on the other hand, shows a lesser 3.26% growth from 2015 to 2037 but a considerable 10.71% increase from 2038 to 2054. These patterns indicate that both scenarios predict a considerable increase in precipitation, which might have serious consequences for regional water resource management and ecosystems.
Similarly, Table 4 shows variations in minimum and maximum temperatures. Temperature is clearly increasing in both scenarios and time periods. The maximum temperature increases from 20.6% in the base period to 21.53% in 2038–2054 for SSP245, whereas the minimum temperature increases from 8.44% to 9.22%. The temperature fluctuations are more dramatic for SSP 585, with maximum and minimum temperatures increasing significantly. These changes point to a significant warming effect, which might have far-reaching implications for ecosystems, agriculture, and water resource management.
It is worth noting that the observed average precipitation and temperatures from 1991 to 2013 are significantly lower than the expected changes in future scenarios. These findings highlight the severity of the expected temperature and precipitation changes, as well as the necessity of proactive arrangements for water resource management in the region.
Model calibration (1991–2006) and validation (2007–2013) were carried out using the observed streamflow data at Besham Qila, Indus River. A 2-year warm-up period (1989–1990) was used to initiate the calibration process. In this study, NSE was used as the objective function during the model calibration. After the model calibration process, a sensitivity analysis was performed to select the parameters that governed the streamflow. The 28 most sensitive parameters were used to calibrate the model, as shown in Table 5. The simulated streamflow during the calibration and validation phases is presented in Figure 4.
Statistical performance indicators including NSE, R2, and RSR were used to assess the model’s effectiveness in predicting the observed streamflow. The NSE, R2, and RSR values during the calibration and validation phases are 0.77, 0.79, and 0.48, and 0.76, 0.78, and 0.49, respectively, as presented in Table 6.
The selected MMEs were used to predict future streamflow for the next 31 years under SSP245 and SSP585 scenarios, as shown in Figure 5. In contrast to the reference period (1991–2013) shown in Table 7, future streamflow estimates show a divergent pattern under SSP245 and SSP585 scenarios, for the time spans 2015–2037 and 2038–2054, respectively. SSP585 showed a 19.42% increase in streamflow for the time span 2015–2037, followed by a 5.84% decline for the time span 2038–2054. In contrast, under SSP245, the increase in streamflow is relatively modest, with a 4% increase from 2015 to 2037, and continues to increase by 1.58% from 2038 to 2054. These findings suggest that streamflow will vary in the near future, which could have consequences for future water resource management in the study region.
FIGURE 5. SWAT model monthly futuristic streamflow results at the Besham station for the selected MMEs of six GCMs under SSP245 and SSP585 scenarios.
As depicted in Figure 6, the frequency of occurrence of the highest flow spikes of the best-simulated model is lower than the observed data. Conversely, the frequency of occurrence of the general and lowest flow values is consistent between the best-simulated model data and observed data. The same pattern is also found in the projected future streamflow for both SSP scenarios, with the main difference being that the peak flow spikes in both SSPs surpass those found in the observed data. Moreover, the peak flow spikes of SSP585 are higher than those of SSP245.
MOLUSCE, a QGIS plugin, was used to predict the land use of the study area. First, the CA-ANN model was trained via 2001 and 2010 land use to predict the 2018 land use. Second, the 2018 predicted land-use map was compared to NASA MODIS land use for accuracy and kappa value, which were determined to be 84.94% and 0.80, respectively. Following validation, 2001 and 2018 land-use maps were used to predict 2030, 2040, and 2050 land uses. Figure 7 depicts the predicted land use in 2030, 2040, and 2050 and the percentage composition of various land use classes.
FIGURE 7. Schematic representation of the predicted land use and percentage composition of various land-use classes.
The impact of 2050's predicted land use on surface runoff was assessed using the SWAT model. The river flow alters in the study area by 2.53% due to land use and 97.47% due to climate change, as shown in Table 8. The results clearly depict that climate change has severe impacts on streamflow in the study area.
Climate change and land use are the major determinants that alter streamflow. Haleem et al. (2022) conducted a study in the Upper Indus Basin, Pakistan, and found that climate change overweighs land use in altering streamflow. In another study, Haleem et al. (2023b) found that streamflow will increase by 19%–30% under SSP245 scenarios. Haider et al. (2023) found that the mean monthly flow will increase by 18%–33.4% in the Kunhar River Basin, Pakistan, under various land use and climate change (SSP2 and SSP5) scenarios. Masood et al. (2023) found that the mean annual flow will increase in the Mohmand Dam catchment, Pakistan, ranging from 13.7% to 34.8% due to the combined effects of land use and climate change (SSP2 and SSP5). Rizwan et al. (2023b) found that the mean monthly streamflow will decrease in the Jhelum River and will increase in the Kabul River and Upper Indus River, Pakistan, under various RCP scenarios. Ismail et al. (2022) found that the average annual stream flow will decrease in the future under various RCP scenarios in the Soan River, Pakistan. Hassan et al. (2023) found that streamflow will increase in the future under various SSPs and land use scenarios in the Rawal Dam catchment, Pakistan. Lutz et al. (2014) found that the water level will rise in the Swat and Indus rivers. Akbar and Gheewala (2021) found that the average annual streamflow will increase due to land use but is not significant in the Kunhar River Basin, Pakistan. It shows that both climate change and land use are dominant determinants that alter streamflow. It is suggested that watershed managers frame water policies based on the climate change and land use impacts on streamflow because these determinants play a vital role in shaping sustainable water resource management strategies.
Climate change is consistently acknowledged as a prominent factor impacting streamflow in various river basins of Pakistan, with several studies forecasting an increase in streamflow under various climate change scenarios. However, there are doubts about the influence of land-use changes since results range from considerable increases in streamflow to non-significant changes in certain basins. The alterations in river flow suggest that the influence of land use and climate change is context-dependent and multifaceted. This complexity may be attributed to diverse methodologies and regional differences. Regional differences in streamflow responses show the importance of conducting regional studies. The variations in futuristic streamflow are attributed to various climate change scenarios adopted by various researchers, highlighting the complex interplay of determinants affecting the regional hydrological systems. Overall, while climate change continues to be a major driver, addressing uncertainty requires a more detailed understanding of regional conditions as well as improved modeling techniques to represent the complicated relationships between climate change and land use across various river basins of Pakistan.
This study pointed out that land-use changes have less influence on streamflow regime in comparison to climate change. Due to global warming, climate change will increase water availability in the short term, followed by a decrease in the long run. Summer flow will be enhanced in the early 21st century due to excessive snowmelt and decline in the later decades due to melted glaciers although this decline will be reduced by rainfall. To improve the condition, current water management practices need to be reviewed. It is essential to implement water management strategies that necessitate water storage to lessen the effects of extreme conditions. The stored water will be used for various purposes in the study area. Plantation is suggested in the region to control the glacier melting and bring sustainability to the water environment (Ahmad et al., 2020).
Pakistan is ranked among the top 10 most susceptible countries to climate change, with 10,000 deaths and $4 billion in financial losses due to climate-related disasters. The country is facing climatic threats, owing to melting glaciers, changing monsoon rainfall patterns, rising temperatures, and recurrence of droughts and floods. The country has already faced 2010 and 2022 floods which have incurred financial and human costs (Ahmed et al., 2018).
The Indus River is important for Pakistan’s food production and economic growth, providing approximately 25% gross domestic product and 90% water for irrigation purposes. Increasing air temperature has an influence on agriculture as it increases crop water requirements. In Pakistan, 2010 and 2020 floods damaged million hectares of cropland, created food shortage, and increased wheat prices by 50% above pre-flood levels (Saeed et al., 2015). The urban poor dwellers spent more money on food items. Furthermore, global warming will increase net agricultural water requirements, putting additional stress on water resources. A 6% decrease in rainfall increases net irrigation water requirements by 29% in the country (Arif et al., 2019). According to the World Bank’s 2020–2021 projection, the water shortfall will expand to 32% by 2025, resulting in a food shortage of over 70 million tons. Recent projections indicate that siltation and climate change will diminish the water storage capacity by over 30% by 2025. Pakistan has a per capita water storage capacity of roughly 150 m3, which is fairly low in contrast to other countries. The condition will be further exacerbated by climate change. In this regard, water management is necessary at the country level (Janjua et al., 2021).
The findings that climate change has a greater impact on river runoff than land-use change emphasize the critical need for adaptive water management and water diplomacy. A multimodal strategy is required to solve this, including investments in climate-resilient water infrastructure capable of handling changing runoff patterns, namely, climate-resilient reservoirs and small dams at the district level. Integrated planning that takes into account both land-use and climate change considerations is critical, highlighting the necessity of collaboration between urban planners and environmental authorities. Collaboration among these stakeholders may promote the creation of sustainable land-use plans that improve adaptive methods to deal with the effects of climate change, namely, in situ and ex situ rainwater harvesting systems at the city level. Water allocation strategies should be created for various sectors that are flexible enough to adjust to shifting river runoff patterns. This might include reviewing water distribution quotas for various sectors on a regular basis in light of revised climate forecasts. To deal with transient fluctuations in runoff, we can consider putting in place systems that enable real-time modifications to water allocations for various sectors. Community involvement and education efforts should empower local communities to contribute to water conservation such as sustainable agricultural practices and rainwater harvesting, while the implementation of early warning systems backed by modern technology can improve the responsiveness of water management techniques. Policy incentives for sustainable land-use practices, as well as international collaboration on shared water resources, strengthen water management systems’ resilience in the face of increasing environmental concerns (Haleem et al., 2023b; Ishaque et al., 2023).
This study was carried out to isolate the effects of anticipated climate and land-use changes on streamflow at the Indus River, Besham, Pakistan, using the Soil and Water Assessment Tool (SWAT). Future climate change and land-use projections were created using an ensemble of general circulation models and CA-ANN, respectively. The historical record (1991–2013) was separated into two parts: calibration (1991–2006) and validation (2007–2013). The SWAT model produced statistically significant results during calibration and validation. The NSE, R2, and RSR values during the calibration and validation phases are 0.77, 0.79, and 0.48, and 0.76, 0.78, and 0.49, respectively. Climate change (97.47%) has a greater effect on river runoff than land-use change (2.53%). Moreover, the impact of SSP585 (5.84%–19.42%) is higher than that of SSP245 (1.58%–4%). The computed impacts of climate and land-use changes on streamflow are recommended to be incorporated into water policies to bring sustainability to the water environment.
The findings of this study are significant as it is based on the MME of 11 GCMs for SSP245 and SSP585 scenarios. However, the results can be improved by using deep learning techniques for bias correction and MME computation, which are sophisticated in comparison to linear scaling and arithmetic mean techniques, respectively. This acknowledgment underscores the need for further improvement in streamflow forecasting for better water resource management.
The original contributions presented in the study are included in the article/Supplementary Material; further inquiries can be directed to the corresponding authors.
SM: data curation, formal analysis, software, validation, visualization, and writing–original draft. AUK: conceptualization, formal analysis, investigation, methodology, software, validation, supervision, and writing–original draft. MB: formal analysis, investigation, methodology, and writing–review and editing. AG: conceptualization, formal analysis, funding acquisition, investigation, resources, supervision, and writing–review and editing. AMA-A: formal analysis, investigation, and writing–review and editing. DK: funding acquisition, investigation, project administration, resources, supervision, and writing–review and editing. TN: funding acquisition, investigation, methodology, project administration, resources, supervision, and writing–review and editing. YG: formal analysis, funding acquisition, resources, supervision, and writing–review and editing.
The author(s) declare financial support was received for the research, authorship, and/or publication of this article. This research was funded by the Institutional Funding Committee at Najran University, the Kingdom of Saudi Arabia.
The authors would like to acknowledge the support of this research by the Deputy for Research and Innovation Ministry of Education, the Kingdom of Saudi Arabia, through grant number (NU/IFC/2//SERC/-/2) under the Institutional Funding Committee at Najran University, the Kingdom of Saudi Arabia. The authors also would like to express their gratitude to the supporting staff and management of WAPDA, PMD, and the Irrigation Department for their invaluable assistance in facilitating and supplying the necessary data. Their contributions were instrumental in successfully completing this study.
The authors declare that the research was conducted in the absence of any commercial or financial relationships that could be construed as a potential conflict of interest.
All claims expressed in this article are solely those of the authors and do not necessarily represent those of their affiliated organizations, or those of the publisher, the editors, and the reviewers. Any product that may be evaluated in this article, or claim that may be made by its manufacturer, is not guaranteed or endorsed by the publisher.
Ahmad, W., Fayaz Ahmad Khan, M. F., Baig, A. A., Shah, L. A., and Khan, J. (2020). How vegetation spatially alters the response of precipitation and air temperature? Evidence from Pakistan. Asian J. Atmos. Environ. 14 (2), 133–145. doi:10.5572/ajae.2020.14.2.133
Ahmed, K., Shahid, S., and Nawaz, N. J. A. r. (2018). Impacts of climate variability and change on seasonal drought characteristics of Pakistan. Atmos. Res. 214, 364–374. doi:10.1016/j.atmosres.2018.08.020
Akbar, H., and Gheewala, S. H. J. A. J. o.G. (2021). Impact of climate and land use changes on flowrate in the Kunhar River Basin. Arabian J. Geosciences 14, 1–11. doi:10.1007/s12517-021-07058-7
Almazroui, M., Saeed, S., Saeed, F., Islam, M. N., and Ismail, M. (2020). Projections of precipitation and temperature over the South Asian countries in CMIP6. Earth Syst. 4, 297–320. doi:10.1007/s41748-020-00157-7
Amiri, S. N., Khoshravesh, M., and Valashedi, R. N. J. A. W. S. (2023). Assessing the effect of climate and land use changes on the hydrologic regimes in the upstream of Tajan river basin using SWAT model. Appl. Water Sci. 13 (6), 130. doi:10.1007/s13201-023-01932-3
Anjum, M. N., Ding, Y., and Shangguan, D. J. A. R. (2019). Simulation of the projected climate change impacts on the river flow regimes under CMIP5 RCP scenarios in the westerlies dominated belt, northern Pakistan. Atmos. Res. 227, 233–248. doi:10.1016/j.atmosres.2019.05.017
Arif, G. M., Riaz, M., Faisal, N., Khattak, M. J. K., Sathar, Z., Khalil, M., et al. (2019). Climate, population, and vulnerability in Pakistan: exploring evidence of linkages for adaptation. Islamabad Popul. Counc. 50 (5).
Bilal, H., Chamhuri, S., Mazlin, B. M., Kasturi, D. K., Rajesh, G., and Tareq, A.-A. (2021). Assessment of land use and land cover changes in association with hydrometeorological parameters in the Upper Indus Basin. J. Himal. Earth Sci. 54 (2).
Chathuranika, I. M., Gunathilake, M. B., Azamathulla, H. M., and Rathnayake, U. (2022). Evaluation of future streamflow in the upper part of the nilwala river basin (Sri Lanka) under climate change. Hydrology 9 (3), 48. doi:10.3390/hydrology9030048
Dey, A., Sahoo, D. P., Kumar, R., and Remesan, R. (2022). A multimodel ensemble machine learning approach for CMIP6 climate model projections in an Indian River basin. Int. J. Climatol. 42 (16), 9215–9236. doi:10.1002/joc.7813
Fang, G., Yang, J., Chen, Y. N., and Zammit, C. (2015). Comparing bias correction methods in downscaling meteorological variables for a hydrologic impact study in an arid area in China. Hydrology Earth Syst. Sci. 19 (6), 2547–2559. doi:10.5194/hess-19-2547-2015
Ficklin, D. L., and Barnhart, B. L. J. J. o.h. (2014). SWAT hydrologic model parameter uncertainty and its implications for hydroclimatic projections in snowmelt-dependent watersheds. J. Hydrology 519, 2081–2090. doi:10.1016/j.jhydrol.2014.09.082
Gassman, P. W., Sadeghi, A. M., and Srinivasan, R. J. J. o.E. Q. (2014). Applications of the SWAT model special section: overview and insights. J. Environ. Qual. 43 (1), 1–8. doi:10.2134/jeq2013.11.0466
Haider, S., Masood, M. U., Rashid, M., Alshehri, F., Pande, C. B., Katipoğlu, O. M., et al. (2023). Simulation of the potential impacts of projected climate and land use change on runoff under CMIP6 scenarios. Water 15 (19), 3421. doi:10.3390/w15193421
Haleem, K., Khan, A. U., Khan, F. A., Zada, U., Khan, J., and Khan, M. (2023a). Futuristic hydroclimatic projections under CMIP6 GCMs: implications for water resources management. https://assets.researchsquare.com/files/rs-3222779/v1/efe6a512-a80d-4db4-89fd-49168dc5a04a.pdf?c=1696195332.
Haleem, K., Khan, A. U., Ahmad, S., Khan, M., Khan, F. A., Khan, W., et al. (2022). Hydrological impacts of climate and land-use change on flow regime variations in upper Indus basin. J. Water Clim. Change 13 (2), 758–770. doi:10.2166/wcc.2021.238
Haleem, K., Khan, A. U., Khan, J., Ghanim, A. A. J., and Al-Areeq, A. M. (2023b). Evaluating future streamflow patterns under SSP245 scenarios: insights from CMIP6. Sustainability 15 (22), 16117. doi:10.3390/su152216117
Hassan, S., Masood, M. U., Haider, S., Anjum, M. N., Hussain, F., Ding, Y., et al. (2023). Investigating the effects of climate and land use changes on rawal dam reservoir operations and hydrological behavior. Water 15 (12), 2246. doi:10.3390/w15122246
Ishaque, W., Mukhtar, M., and Tanvir, R. J. F. i.E. S. (2023). Pakistan’s water resource management: ensuring water security for sustainable development. Front. Environ. Sci. 11, 1096747. doi:10.3389/fenvs.2023.1096747
Ismail, M., Ahmed, E., Peng, G., Xu, R., Sultan, M., Khan, F. U., et al. (2022). Evaluating the impact of climate change on the stream flow in Soan River Basin (Pakistan). Water 14 (22), 3695. doi:10.3390/w14223695
Janjua, S., Hassan, I., Muhammad, S., Ahmed, S., and Ahmed, A. (2021). Water management in Pakistan’s Indus Basin: challenges and opportunities. Water Policy 23 (6), 1329–1343. doi:10.2166/wp.2021.068
Karunanayake, C., Gunathilake, M. B., and Rathnayake, U. (2020). Inflow forecast of iranamadu reservoir, Sri Lanka, under projected climate scenarios using artificial neural networks. Appl. Comput. Intell. Soft Comput. 2020, 1–11. doi:10.1155/2020/8821627
Khan, A. J., Koch, M., and Tahir, A. A. J. S. (2020). Impacts of climate change on the water availability, seasonality and extremes in the Upper Indus Basin (UIB). Sustainability 12 (4), 1283. doi:10.3390/su12041283
Khan, M., Khan, A. U., Khan, S., and Khan, F. A. (2023a). Assessing the impacts of climate change on streamflow dynamics: a machine learning perspective. Water Sci. Technol. 88 (9), 2309–2331. doi:10.2166/wst.2023.340
Khan, M., Khan, S., Ullah Khan, A., Noman, M., Usama, M., Ahmad Khan, F., et al. (2022b). Effect of land use change on climate elasticity of water quality at multiple spatial scales. Water Pract. Technol. 17 (11), 2334–2350. doi:10.2166/wpt.2022.131
Khan, S., Khan, A. U., Khan, M., Khan, F. A., Khan, S., and Khan, J. (2023b). Intercomparison of SWAT and ANN techniques in simulating streamflows in the astore Basin of the upper Indus. Water Sci. Technol., wst2023299.
Khan, Z., Khan, F. A., Khan, A. U., Hussain, I., Khan, A., Shah, L. A., et al. (2022a). Climate-streamflow relationship and consequences of its instability in large rivers of Pakistan: an elasticity perspective. Water 14 (13), 2033. doi:10.3390/w14132033
Khan, Z., Khan, F. A., Khan, A. U., Hussain, I., Khan, A., Shah, L. A., et al. (2022c). Climate-streamflow relationship and consequences of its instability in large rivers of Pakistan: an elasticity perspective. Water 14 (13), 2033. doi:10.3390/w14132033
Kiran, S. N., Iqbal, M. F., and Mahmood, I. (2023). Assessing the impacts of climate change on flooding under coupled model intercomparison project phase 6 scenarios in the river chenab, Pakistan. Nat. Hazards 117 (1), 1005–1033. doi:10.1007/s11069-023-05892-4
Lutz, A., Immerzeel, W. W., Shrestha, A. B., and Bierkens, M. F. P. (2014). Consistent increase in High Asia’s runoff due to increasing glacier melt and precipitation. Nat. Clim. Change 4 (7), 587–592. doi:10.1038/nclimate2237
Mahmood, R., Jia, S., and Babel, M. S. J. W. (2016). Potential impacts of climate change on water resources in the Kunhar River Basin, Pakistan. Water 8 (1), 23. doi:10.3390/w8010023
Mahmood, R., and Jia, S. J. W. (2016). Assessment of impacts of climate change on the water resources of the transboundary Jhelum River basin of Pakistan and India. Water 8 (6), 246. doi:10.3390/w8060246
Masood, M. U., Khan, N. M., Haider, S., Anjum, M. N., Chen, X., Gulakhmadov, A., et al. (2023). Appraisal of land cover and climate change impacts on water resources: a case study of Mohmand dam catchment, Pakistan. Water 15 (7), 1313. doi:10.3390/w15071313
Nguyen, H., Mehrotra, R., and Sharma, A. J. W. r.r. (2020). Assessment of climate change impacts on reservoir storage reliability, resilience, and vulnerability using a multivariate frequency bias correction approach. Water Resour. Res. 56 (2), e2019WR026022. doi:10.1029/2019wr026022
Rizwan, M., Li, X., Chen, Y., Anjum, L., Hamid, S., Yamin, M., et al. (2023a). Simulating future flood risks under climate change in the source region of the Indus River. J. Flood Risk Manag. 16 (1), e12857. doi:10.1111/jfr3.12857
Rizwan, M., Li, X., Chen, Y., Anjum, L., Hamid, S., Yamin, M., et al. (2023b). Simulating future flood risks under climate change in the source region of the Indus River. J. Flood Risk Manag. 16 (1), e12857. doi:10.1111/jfr3.12857
Saeed, F., Salik, K., and Ishfaq, S. (2015). Climate induced rural-to-urban migration in Pakistan’pathways to resilience in semi-arid economies (PRISE). https://sdpi.org/sdpiweb/publications/files/Climate-induced-rural-to-urban-migration-in-Pakistan.pdf.
Shahid, M., Cong, Z., and Zhang, D. (2018). Understanding the impacts of climate change and human activities on streamflow: a case study of the Soan River basin, Pakistan. Theor. Appl. Climatol. 134, 205–219. doi:10.1007/s00704-017-2269-4
Shahid, M., Rahman, K. U., Haider, S., Gabriel, H. F., Khan, A. J., Pham, Q. B., et al. (2021). Quantitative assessment of regional land use and climate change impact on runoff across Gilgit watershed. Environ. Earth Sci. 80, 743–818. doi:10.1007/s12665-021-10032-x
Shang, X., Jiang, X., Jia, R., and Wei, C. (2019). Land use and climate change effects on surface runoff variations in the upper Heihe River basin. Water 11 (2), 344. doi:10.3390/w11020344
Shetty, S., Umesh, P., and Shetty, A. J. I. J. o.C. (2023). The effectiveness of machine learning-based multi-model ensemble predictions of CMIP6 in Western Ghats of India. Int. J. Climatol. 43, 5029–5054. doi:10.1002/joc.8131
Singh, D., Vardhan, M., Sahu, R., Chatterjee, D., Chauhan, P., and Liu, S. (2023). Machine-learning-and deep-learning-based streamflow prediction in a hilly catchment for future scenarios using CMIP6 GCM data. Hydrology Earth Syst. Sci. 27 (5), 1047–1075. doi:10.5194/hess-27-1047-2023
Ullah, B., Fawad, M., Khan, A. U., Mohamand, S. K., Khan, M., Iqbal, M. J., et al. (2023). Futuristic streamflow prediction based on CMIP6 scenarios using machine learning models. Water Resour. Manag. 37, 6089–6106. doi:10.1007/s11269-023-03645-3
Usman, M., Ndehedehe, C. E., Manzanas, R., Ahmad, B., and Adeyeri, O. E. (2021). Impacts of climate change on the hydrometeorological characteristics of the soan river basin, Pakistan. Atmosphere 12 (6), 792. doi:10.3390/atmos12060792
Wisal, K., Asif, K., Afed Ullah, K., and Mujahid, K. (2020). Evaluation of hydrological modeling using climatic station and gridded precipitation dataset. Mausam 71 (4), 717–728. doi:10.54302/mausam.v71i4.63
Worku, G., Teferi, E., Bantider, A., and Dile, Y. T. (2020). Statistical bias correction of regional climate model simulations for climate change projection in the Jemma sub-basin, upper Blue Nile Basin of Ethiopia. Theor. Appl. Climatol. 139, 1569–1588. doi:10.1007/s00704-019-03053-x
Keywords: streamflow, climate change, land-use change, CMIP6, prediction
Citation: Mahmood S, Khan AU, Babur M, Ghanim AAJ, Al-Areeq AM, Khan D, Najeh T and Gamil Y (2024) Divergent path: isolating land use and climate change impact on river runoff. Front. Environ. Sci. 12:1338512. doi: 10.3389/fenvs.2024.1338512
Received: 14 November 2023; Accepted: 15 January 2024;
Published: 01 February 2024.
Edited by:
Christopher Lant, Utah State University, United StatesReviewed by:
Muhammad Shahid, Brunel University London, United KingdomCopyright © 2024 Mahmood, Khan, Babur, Ghanim, Al-Areeq, Khan, Najeh and Gamil. This is an open-access article distributed under the terms of the Creative Commons Attribution License (CC BY). The use, distribution or reproduction in other forums is permitted, provided the original author(s) and the copyright owner(s) are credited and that the original publication in this journal is cited, in accordance with accepted academic practice. No use, distribution or reproduction is permitted which does not comply with these terms.
*Correspondence: Afed Ullah Khan, YWZlZHVsbGFoQHVldHBlc2hhd2FyLmVkdS5waw==; Taoufik Najeh, dGFvdWZpay5uYWplaEBsdHUuc2U=
Disclaimer: All claims expressed in this article are solely those of the authors and do not necessarily represent those of their affiliated organizations, or those of the publisher, the editors and the reviewers. Any product that may be evaluated in this article or claim that may be made by its manufacturer is not guaranteed or endorsed by the publisher.
Research integrity at Frontiers
Learn more about the work of our research integrity team to safeguard the quality of each article we publish.