- 1Department of Biology and Environmental Engineering, University of Craiova, Craiova, Romania
- 2Department of Plant Resources and Environment, Jeju National University, Jeju-si, Republic of Korea
- 3Division of Soil Science and Agricultural Chemistry, Sher-e-Kashmir University of Agricultural Sciences and Technology, Sopore, Jammu and Kashmir, India
- 4Division of Soil Science and Agricultural Chemistry, Faculty of Agriculture, Sher-e-Kashmir University of Agricultural Sciences and Technology of Jammu, Chatha, Jammu and Kashmir, India
- 5Department of Crop, Soil and Environmental Sciences, Auburn University, Auburn, AL, United States
- 6Department of Agronomy, Kansas State University, Manhattan, KS, United States
- 7Soil and Groundwater Division, School of Environment, Tsinghua University Beijing, China
- 8UWA School of Agriculture and Environment, Institute of Agriculture, Perth, WA, Australia
Detecting hazardous substances in the environment is crucial for protecting human wellbeing and ecosystems. As technology continues to advance, artificial intelligence (AI) has emerged as a promising tool for creating sensors that can effectively detect and analyze these hazardous substances. The increasing advancements in information technology have led to a growing interest in utilizing this technology for environmental pollution detection. AI-driven sensor systems, AI and Internet of Things (IoT) can be efficiently used for environmental monitoring, such as those for detecting air pollutants, water contaminants, and soil toxins. With the increasing concerns about the detrimental impact of legacy and emerging hazardous substances on ecosystems and human health, it is necessary to develop advanced monitoring systems that can efficiently detect, analyze, and respond to potential risks. Therefore, this review aims to explore recent advancements in using AI, sensors and IOTs for environmental pollution monitoring, taking into account the complexities of predicting and tracking pollution changes due to the dynamic nature of the environment. Integrating machine learning (ML) methods has the potential to revolutionize environmental science, but it also poses challenges. Important considerations include balancing model performance and interpretability, understanding ML model requirements, selecting appropriate models, and addressing concerns related to data sharing. Through examining these issues, this study seeks to highlight the latest trends in leveraging AI and IOT for environmental pollution monitoring.
1 Introduction
Hazardous substances in the environment are those that pose a threat to human health, plant, and animal life, or the environment. These substances include heavy metals, pesticides, herbicides, and persistent organic pollutants (POPs) that have been introduced into the environment through various means (Young et al., 2004). Sources of hazardous substances in soil include industrial activities, improper disposal of hazardous waste, agricultural practices, and natural processes such as erosion and weathering. These substances can persist in the environment for long periods and can have a negative impact on soil quality, plant growth, and human health (Bachmann, 2006; Baran et al., 2011; Bolan et al., 2021; Rani et al., 2021). The effects of hazardous substances in the environment can vary depending on the type and concentration of the substance, as well as the duration of exposure. Some hazardous substances can cause acute health effects, such as respiratory problems, skin irritation, poisoning, nausea, and vomiting, while others can lead to chronic health problems, including cancer, reproductive disorders, and developmental abnormalities (Baran et al., 2011; Li et al., 2022; Yang et al., 2022).
Effective management of hazardous substances in the environment requires monitoring, remediation, and prevention strategies. Monitoring involves regular testing of soil for the presence of hazardous substances, which allows for early detection and appropriate management strategies to be implemented. Remediation involves the removal or treatment of contaminated sites. Prevention strategies include reducing the use of hazardous materials (i.e., source control) and implementing best practices for waste disposal and land use. Effective management is essential for ensuring the long-term health and sustainability of soil and its ecosystems (Mansoor et al., 2022; Sharma et al., 2022; Sonne et al., 2023).
Real-time monitoring of hazardous materials in soil and plants is an important task that can help to ensure the safety of food crops, protect the environment, and prevent human exposure to harmful substances. The use of artificial intelligence (AI) powered sensors and devices can greatly enhance the accuracy and efficiency of this monitoring process. AI-powered sensors and devices can be used to detect and quantify the presence of various hazardous materials in soil and plants (Wilson, 2012; Yang et al., 2021). These sensors and devices can be designed to measure parameters such as pH, temperature, moisture, conductivity, and various chemical properties of the soil and plant tissue. Machine learning algorithms can be used to analyze the data collected by these sensors and devices, enabling the identification of specific hazardous materials in real-time (Wilson, 2012; Yang et al., 2021). These algorithms can also be used to predict the potential impact of these materials on human health and the environment. For example, E-nose (olfactory) algorithms are used to analyze data generated by sensors and identify the presence of hazardous chemicals based on their unique chemical signature (Jeong and Choi, 2022). These algorithms can use a variety of techniques, such as pattern recognition, artificial neural networks, and fuzzy logic. One of the key advantages of E-nose technologies is their ability to detect hazardous chemicals in real-time, allowing for immediate response to potential threats. E-nose technologies can be used for a variety of applications, such as monitoring air quality in urban areas, detecting leaks from industrial processes, and detecting explosives and other hazardous materials (Jeong and Choi, 2022).
The use of AI-powered sensors and devices for real-time monitoring of hazardous materials in soil and plants has several benefits (Singh and Kaur, 2022). Firstly, it allows for more accurate and reliable detection of these materials compared to traditional laboratory-based methods. Secondly, it provides real-time data, allowing for quick responses to any potential contamination events. Finally, it reduces the need for manual data collection and analysis, reducing the workload and increasing the efficiency of the monitoring process (Jeong and Choi, 2022). The use of AI-powered sensors and devices for real-time monitoring of hazardous materials in soil and plants is a promising approach that can help to ensure the safety of food crops, protect the environment, and prevent human exposure to harmful substances. Various approaches can be used for AI-based toxicity prediction, including machine learning methods, deep learning methods, and hybrid approaches that combine both methods. Integrating various sources of data, such as chemical structures, toxicological and physiological data, and environmental factors, to improve the accuracy and reliability of toxicity predictions, is important (Jeong and Choi, 2022; Chen et al., 2023).
One of the recent advances is the combination of AI and Internet of Things (IoT) technologies, for particulate matter (PM) monitoring, which uses low-cost sensors that can be easily deployed in various environments (Bhagat et al., 2020). These sensors can collect data on PM levels and send it to a centralized platform for analysis. AI algorithms can then process these data to provide real-time information on PM levels and predict future trends. Heavy metals can also be monitored using AI. Numerous studies have been conducted over the last 10 years to forecast the effectiveness of heavy metal removal from soil using machine learning (Zafar et al., 2017; Zhu et al., 2019). AI models for the optimization and prediction of heavy metal removal include black box, fuzzy logic, kernel, evolutionary, and hybrid models.
With the rapid changes that the environment is experiencing, data sharing and reuse with the help on AI algorithms and instruments (Shen, 2018), plays an important role in supporting researchers to safeguard the continuous threatened environment and ensure the implementation of sustainable environmental management practices (Aggestam and Mangalagiu, 2020). Scientists can make use of online data sharing tools and platforms that comprise vast and intricate Earth and environmental science data like climatic and atmospheric data, pedology, hidrology, ecology, and biodiversity data (Crystal-Ornelas et al., 2022; Basel et al., 2023) for testing, analyzing, interpretation of theories, prediction models and experimental data (Kostal et al., 2022) that lead to better undestanding environmental issues.
In this comprehensive review, we will examine the application of artificial intelligence (AI) in monitoring hazardous materials across different environments, namely, soil, air, and water. We will explore the latest breakthroughs and progress made in this field, which integrates machine learning algorithms with sensor technologies. We will also consider the benefits and drawbacks associated with AI-powered monitoring systems. This new field has revolutionized soil, air, and water monitoring, enhancing accuracy, efficiency, and timeliness in detecting and analyzing hazardous substances.
A literature search was conducted in Web of Science Core Collections with the following search terms (TS stands for Topic Searches): TS=(“artificial intelligence” or “AI” or “machine learning” or “deep learning” or “Internet of Things” or “IoT” or “computer vision” or “robotics” or “natural language process” or “real-time monitoring” or “e-nose”) AND TS=(“hazardous substance” or “hazardous chemical” or “hazardous material” or “pollutant” or “contamination” or “toxin” or “heavy metal” or “pesticide” or “herbicide” or “persistent organic pollutants” or “POPs” or “microplastic”) AND TS=(“environment” or “soil” or “terrestrial” or “aquatic” or “aqueous” or “freshwater” or “lake” or “river” or “sediment” or “marine” or “ocean” or “air” or “atmosphere”). A total of 2828 results were retrieved. The results were visualized using the VOS (visualization of similarities) viewer software (version 1.6.19). Figure 1 presents the systematic literature search results covering the number of publications and the keyword co-occurrence map on the topic of the review (AI-Driven Technologies for Hazardous Substance Monitoring).
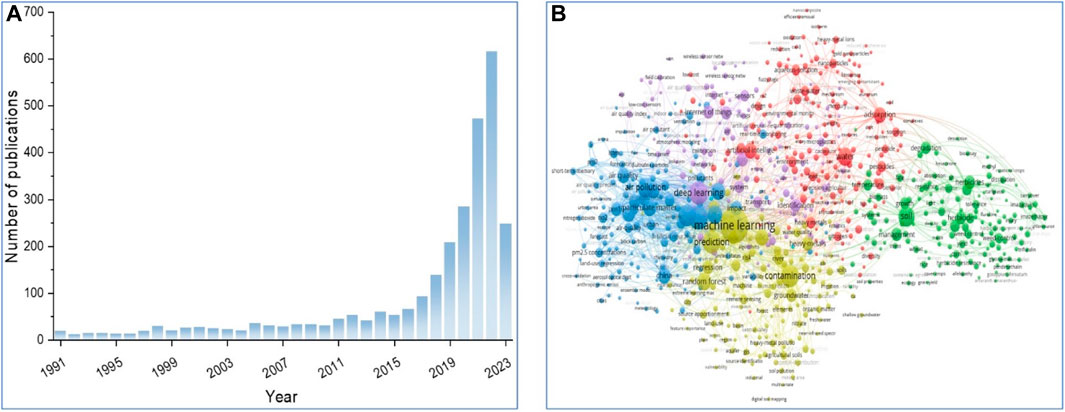
FIGURE 1. Systematic literature search results on AI-Driven Technologies for Hazardous Substance Monitoring topic. (A) The number of publications related to AI-Driven Technologies for Hazardous Substance Monitoring topic in each year following literature screening. (B) A network visualization map delineating keywords within the associated literature is depicted, revealing clusters indicative of distinct research themes. Nodes sharing analogous colors denote cohesive clusters comprising interrelated terms. This map was generated through the utilization of VOSviewer.
2 AI solutions for hazardous substance monitoring in different environments
In recent years, there has been an increase in interest for using AI to anticipate and predict environmental pollution. We can split AI solutions into three steps or phases that include inputs (data), models (AI algorithms), and outputs (monitoring or decision support) (Figure 2). Data forms the basis of any AI solution. These models work best when they have a high number of data points, especially the ones that are coming from environments that are continually being monitored and expending solutions for probable actions. Data sources today can be a variety of sensors, ranging from imaging to non-imaging types or remote to in-contact sensors that provide large volumes of data. Then there are the historic or legacy data. AI algorithms can analyse massive amounts of sensor readings, historical data, and other important information from monitoring systems. AI can detect hazardous material levels and contamination events by identifying patterns, trends, and anomalies in data using machine learning and data mining. Image analysis can detect hazardous material spills via satellite photos or drone images. Computer vision algorithms can recognise chemicals, vegetation changes, and pollution sources.
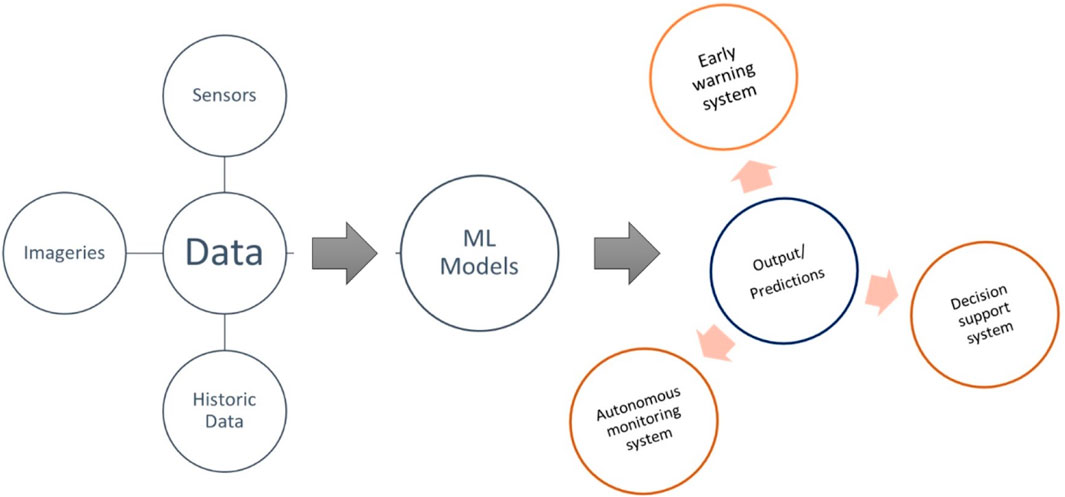
FIGURE 2. AI solutions: making use of Data and AI algorithms for hazardous substance monitoring and containment.
AI algorithms can be trained on past data to predict hazardous material releases and environmental pollution. AI solutions for environmental monitoring, thus, would include early warning systems for hazardous material release, autonomous pollution monitoring systems as well as decision support systems. These models can help authorities and organisations prepare for and respond to emergencies. These models have proved useful for multiple environmental conditions, be it soil, air, or water. AI modellers, however, should offer sufficient details to explain and support the selection of model parameters, as well as their creation and assessment.
Many short- and long-term forecasting applications use ANNs (artificial neural networks). One has created an IoT-enabled environmental toxicology model to detect air pollution (Asha et al., 2022). The model uses artificial intelligence to report the status of the quality of air in real-time utilising a cloud server and broadcasts alarms when hazardous pollutants are present. The AAA (artificial algae algorithm)-based ENN (Elman neural network) model classifies and predicts air quality in future timestamps (a timestamp is the current time of an event that a computer records). WiFi gateways send the data collected by sensors to a cloud server. AAA optimises the ENN model parameters during data processing. For monitoring and enforcement, mobile electronic-nose (E-nose) devices and algorithms have been developed to quickly detect pollution from point sources. Due to their sensitivity, E-nose devices can detect carbon, GHG emissions, and particle pollutants emitted into air or water (Wilson, 2012).
Traditional soil mapping entails physically collecting soil samples and transferring them to a laboratory for additional analysis (Signes-Pastor et al., 2016; Sharma et al., 2017). The advent of technologies permitting high-resolution, quick, and inexpensive mapping of soil pollutants has favourable aspects over traditional techniques. Jia et al. (2021) created an unique modelling method that forecasts soil arsenic levels using high resolution aerial imagery (HRAI) photos. The method makes use of cameras that are installed on aircraft to take high-resolution (0.1–0.5 m) pictures of broad areas. The first layer of a model for displaying soil arsenic levels shows a thorough report on soil contamination and HRAI. The image is broken down into pixels in the second layer, and sample points are represented by pixel features. In order to forecast the risk levels of arsenic, four alternative machine learning algorithms were constructed. The Extreme Random Forest (ERF) algorithm had the best prediction and accuracy (Jia et al., 2021). Remote sensing and aerial imageries provide continuous spatial data which, coupled with machine learning models, are providing highly accurate maps of hazardous substances in the environment that were not possible with standard geostatistical techniques.
Microplastics in the environment have become a cause for concern, but their evaluation in soils is a laborious process. Hyperspectral imaging (HSI) and convolutional neural network (CNN) technology-based methods have been developed for identifying microplastic polymers (Ai et al., 2023). The technique developed by Ai et al. (2023) provides a non-destructive, rapid approach to detection of microplastics in soils. Microfluidic devices using machine learning and AI promise to be next-generation monitoring systems (Pouyanfar et al., 2022). Sensitive microfluidic devices produce high-quality pollutant data and reveal important environmental information. Artificial intelligence can categorize, characterize, and predict data from microfluidic systems. The two can be easily set up. Ahmadi et al. (2021) used ANN to predict the concentrations of organophosphorus pesticides in water. Their model worked best for prediction of Malathion (R2 = 0.887), followed by parathion (R2 = 0.711), and Diazinon (R2 = 0.714). However, the R-squared value was acceptable for all pesticide types (Sarkar and Pandey, 2015). Used flow discharge data from the Yamuna River in northern India, as well as biochemical oxygen demand, temperature, pH, and dissolved oxygen collected on a monthly basis in the vicinity of Mathura, India, and employed a feed forward, error back propagation algorithm to develop ANN models. The predictions from the ANN models were accurate, with correlation values being as high as 0.9, indicating that ANNs can be efficiently utilized to predict water quality.
3 AI-powered technologies for pollution monitoring
3.1 Spectroscopy
Spectroscopy, which involves measuring the functions of energy with matter, is of preeminent importance in remote sensing. It has been widely utilized in field of chemistry and astronomy to identify materials, and advancements in instrumentation have led to its increasing use in remote sensing studies (Slonecker et al., 2010). Visible and near-infrared reflectance spectroscopy is an environmentally friendly and cost-efficient technique that shows promise for estimating concentrations of various heavy metals in soil. Additionally, it offers a viable alternative for assessing heavy metal levels across large areas and for an extended period (Shi et al., 2022).
For example, Zhao et al. (2022) used visible and near-infrared spectroscopy with extreme gradient boosting (XGBoost) - as an effective machine learning technique to create an estimation model for heavy metal pollution in mangrove sediment sites. Vis-NIR spectroscopy coupled with partial least squares (PLS) and radial basis function neural network (RBFNN) prediction models, were engaged by Sanaeifar et al. (2022) in their study to investigate the effects of airborne Pb on tea plants. Elevated concentrations of Pb had deleterious effects on the plant and the results revealed PLS–RBFNN models to be more accurate and superior to conventional methods in terms of prediction, giving Vis-NIR spectral data traits like high speed and simplicity in monitoring heavy metal pollution.
Lately, there has been significant interest in the development and application of flexible, surface-enhanced Raman scattering (SERS) substrates for the detection of hazardous substances. Bharati and Soma (2021) conducted a comprehensive 4-year investigation into various flexible SERS substrates, such as paper or cellulose, polymer nanofibers, 3D sponges, and fabrics. They explored the potential of these substrates for on-site detection of explosives, pesticides, chemical warfare agents, and drugs, and the research was related to fields such as homeland security, food safety, and medicine. The results of their study highlight the considerable opportunities for SERS substrates in the detection and monitoring of hazardous waste.
3.2 Ground-based monitoring sensors
As reported by Pant et al. (Pant et al., 2019), air quality monitoring mainly employs two types of sensor networks: manual and automatic monitoring sensors (like wireless or community sensor networks). According to Kim et al. (Kim et al., 2008; Kumar et al., 2022), after combining air quality monitoring sensors with geo-sensor network technologies, the collection and interpretation of geospatial and pollution data were transformed. Levy et al. (2010) observed that the monitoring of pollutants, like particulate matter, nitrite, and sulphur dioxide, can be achieved using various sensor networks, including instruments like Met One Instrument BAM-1020 Beta Attenuation Monitor (Met one instruments ltd. 1,600 Washington Blvd. Grants Pass, Oregon), Alphasense OPC-N2 Particle Monitor (Halo labs 1828 Burlingame, CA, 94,010 USA) and Aeroqual Series 500 with NO2 Sensor Head (Aeroqual Limited, London). Ground-based aerosol optical measurements not only help characterize ambient aerosols, but also assist in validating satellite retrievals and numerical modeling algorithms (Levy et al., 2010; Mansoor et al., 2021). Amado and Cruz (Amado et al., 2018; Naz et al., 2023) used machine learning to calibrate a predictive model for monitoring and characterizing air quality. Their methodology involved creating a prototype with integrated sensors, including DHT 11 temperature and relative humidity sensors, as well as MQ2, MQ5, and MQ135 gas sensors (Zhengzhou Winsen Electronics Technology Co., Ltd.). The study developed five predictive models: k-nearest neighbors (KNN), support vector machine (SVM), naïve-Bayesian classifier, random forest, and neural network. The models demonstrated 99% accuracy.
Artificial intelligence has found its way into agricultural systems, incorporating various sensors for improved performance. Soil moisture sensors are used to ensure adequate irrigation, while temperature sensors monitor the ambient conditions of grain storage. Manickavasagan et al. (2006) utilized temperature and humidity monitoring technology to enhance quality control of stored products by indirectly measuring key parameters. This approach also enabled the prediction of quantitative and qualitative losses in stored grains, providing valuable insights for decision-making in agricultural businesses.
Under field conditions, Liu et al. (2019) explored the application of acoustic sensors, specifically piezoelectric sensors, for detecting insect infestation. Piezoelectric crystals, which transform mechanical stress into electrical charge, are the fundamental component of an acoustic sensor. By measuring sound waves or vibrations and transforming them into electronic signals, piezoelectric sensors can effectively indicate the presence of insects. To aid decision-making and improve management practices, Cruz et al. (2018) conducted a study on predicting flood levels in advance. They put in place a real-time monitoring system with numerous sensors that can gauge variables including rainfall volume and intensity, soil moisture, water level, and rate of water level rise. Using a multi-layered artificial neural network developed with MATLAB, they created a prediction model based on the gathered sensor data. The results showed a high level of accuracy, with goodness-of-fit values of 0.99889 for the training dataset, 0.99362 for the test dataset, and 0.99764 for the validation dataset.
3.3 Aerial imaging and unmanned aerial vehicles (UAVs)
Since the early 1990s, aerial imagery has been employed to monitor hazardous waste. Aerial photographs have proven useful in various applications for detecting and analyzing the presence of hazardous waste, waste-disposal sites, and landfills (Pope et al., 1996; Wani et al., 2023). Historical aerial photographs provide valuable documentation for compiling a record of changes occurring at hazardous sites and are considered reliable for monitoring changes over time. The U.S. Environmental Protection Agency (USEPA) has made use of an aerial imagery library that dates back to the 1930s to retrace the history of waste management and disposal at hazardous waste sites. Environmental clean-up programmes have generated more than 4,000 historical aerial photographic reports on hazardous waste activity and employed them in clean-up (Benger et al., 2004).
Aerial photography allows for the interpretation of various objects at hazardous waste sites, including evidence of discarded materials, barrels and drums, open dumps, spills, and disturbance. These features enable monitoring and analysis of potential impacts related to hazardous waste. Aerial imagery facilitates the identification of vegetation patterns, investigation of local groundwater movement to assess potential pollutant migration, determination of drainage routes, examination of hydrological conditions, and evaluation of subsequent land use on closed landfills (Slonecker et al., 2002; Garofalo, 2003).
Hyperspectral imagery-based models have proven effective in predicting heavy-metal distribution in soils. In a study conducted by Tan et al. (Tan et al., 2021), a competitive adaptive reweighted sampling (CARS) method was proposed for this purpose. The researchers compared the accuracy of various models and discovered that CARS in combination with a stacking method exhibited the highest accuracy and stability. This method utilized the spectrum in the range of 2–2.3 μm, which is a common characteristic band for heavy metals. Additionally, it effectively addressed challenges, such as overfitting due to imbalanced data and limited training sample sets. Importantly, even in the presence of spatial heterogeneity, the distribution of heavy-metal concentrations derived from the CARS-stacking method showed consistency in the verification analysis.
Increased population and industrialization have led to a rise in hazardous waste spillage or leakage incidents. Detecting and monitoring toxic, flammable, and inert gas leaks are a global priority and have been the subject of extensive research. The emergence of Unmanned Aerial Vehicles (UAVs) or drones has significantly enhanced the collection of aerial imagery. This technological advancement has provided a boost in obtaining high-resolution and up-to-date aerial data for various applications, including the monitoring and analysis of heavy-metal distribution in soils. Forward reconnaissance drones, equipped with sensors, are valuable tools for enhancing situational awareness in environments that are challenging for humans. Remote gas detection systems, utilizing mobile robotic platforms such as drones, have emerged as a promising approach in this field, especially in environments too hazardous for human exploration (Gerhardt et al., 2014).
By augmenting drones with sensors, hazardous materials or spills can be identified, and waste can be monitored to enable effective planning and management, thereby minimizing the exposure of first-response teams (Seiber et al., 2018). Also, integrating sensor-based particulate detection with autonomous drone flight control enables dynamic identification and real-time tracking of aerial plume boundaries. The findings of Seiber et al. (2018) demonstrate that UAVs can precisely recognize and track contaminant plumes over time, thus providing visual indicators and aid data collection that can be used to validate and advance the plume movement models. In synopsis, the utilization of sensor-equipped drones for forward reconnaissance purposes can significantly contribute to identifying and monitoring hazardous materials, spills, and gas leaks in environments where human access is unsafe. The integration of sensors and autonomous flight control enhances the capabilities of drones in real-time plume tracking and provides valuable data for improved modelling and management of hazardous incidents.
In incidents that involve hazardous materials, drone flights play a crucial role in promptly and accurately identifying the direction of spillage or gaseous material dispersion (Restas, 2015). Numerous studies have highlighted the ability of UAVs to be rapidly deployed to disaster sites and gather essential data about the extent and impact of spills. This information is vital for effective planning, management, and response efforts. Integrating UAVs into the toolkits of industry and government spill response teams can greatly enhance response capabilities, mitigation strategies, and overall accountability (Messinger and Silman, 2016). In summary, UAVs are indispensable in assessing and managing incidents involving hazardous materials. Their ability to be quickly deployed, gather data, and monitor critical areas provides valuable insights for response teams, enabling them to make informed decisions, mitigate risks, and safeguard both human lives and the environment.
3.4 Ground robotics
In recent years, ground robotics have emerged as a technology with gas-sensitive sensors that can be employed to assess situations in enclosed and unventilated spaces by detecting gas distributions, essentially acting as a “sense of smell.” A study conducted by Wandel et al. (Wandel et al., 2003) aimed to develop a unified algorithm for localizing the source of odors using ground robotics. They conducted experiments with ethanol as a test substance and successfully determined its concentration. However, a few irregularities occurred due to external factors that influenced the data. For instance, summertime natural convection caused heat transfer from the glass window in the enclosed space, increasing the concentration of ethanol. The researchers demonstrated the advantage of this tool in its ability to monitor suspected areas and detect the presence of hazardous substances.
Vincent et al. (2019) conducted an experiment in a laboratory wind tunnel and a real-world environment to study the formation of gas plumes. Typically, sensors used for gas detection are characterized using data from controlled gas rigs, where precise step changes in gas mixtures are produced. However, in real-world scenarios, such as mobile robot exploration, gas sources do not emit steady concentrations. Instead, they generate plumes, resulting in areas of high and low gas concentrations in the surrounding environment.
An algorithm method to control mobile robots - iRobot Create was designed by Ahmed et al. (2016). The robotized mobile nodes are integrated in a WSN structure, have built-in sensors, equipped with a microprocessor Gumstix Verdex Pro™ XL6P. The real-time experiments demonstrated the suitability of this wireless sensor network robotized nodes that enables two robots to receive information from each other for detecting a pollutea area, based on pollution searching, reorientation and surveillance parameters.
Haldorai et al. (2024) developed a robot for polluted water surface cleaning. The autonomus robot is equiped with two Arduino microcontrollers, powered by a 6 V lead-acid battery, implemented with SSD real-time object detection deep learning model for the precise detection of wastes from water surface. The robot reaches maximum potential, can avoid obstacles and colect by itself the wastes from the water surface when it gets to 45 and 75 cm from the floating debris making it an effective tool for waste removal from water bodies.
As highlighted by Tsitsimpelis et al. (2019), robotic systems offer an ideal solution for identifying and monitoring extreme radiation exposure levels, as well as toxic and combustible atmospheres. These systems address several challenges by eliminating the need for humans to physically access hazardous locations. Furthermore, they can provide valuable data on the conditions of these places that would otherwise remain inaccessible. Ground robotics equipped with gas-sensitive sensors offer a valuable means of monitoring and detecting hazardous materials. Despite certain challenges and external influences on data accuracy, this technology holds promise in identifying and localizing odour sources in enclosed spaces, contributing to the overall effort of managing and minimizing the presence of dangerous substances.
3.5 Satellite remote sensing
Remote sensing is a scientific and technological approach that enables the identification and assessment of various characteristics and properties of Earth’s targets from a distance. On both a global and local level, it has offered systematic and repeated observations of numerous features of the Earth’s surface, including the atmosphere, water, land, living things, vegetation, pollution, and climate. The utilization of remote sensing has played a crucial role in detecting and quantifying pollution rates, mapping, monitoring, and mitigating pollution (Mertikas et al., 2021).
One of many applications of remote sensing is monitoring and managing hazardous waste and sites. Multispectral sensors (MSS) mounted on remote sensing platforms are capable of digitally collecting energy levels of reflectance in specific bands of the electromagnetic spectrum. These systems offer advantages such as statistical analysis of data and the ability to extend observations beyond the capabilities of aerial photography. Land use, regional risk assessment, and hazardous waste site spectral characteristics and pollution profiles have been monitored using multispectral imaging systems mounted on satellites and various aircraft-based systems. For instance, Bølviken et al. (Bølviken et al., 1977) demonstrated that MSS data could be employed to identify heavy metal contamination based on fundamental spectral characteristics.
Furthermore, multispectral imagery has been used by several researchers to identify and locate previously unknown as well as illegal hazardous waste sites. Errico et al. (Errico et al., 2014) proposed a methodology that combines synthetic aperture radar (SAR), multispectral data, and GIS-based processing for detecting environmental hazards. This system yielded satisfactory results and contributed to countering and controlling environmental crimes. Additionally, hyperspectral imagery can be employed to identify and map the spatial distribution of various heavy metals. For instance, Kemper and Sommer (Kemper et al., 2004) used a HyMap sensor and utilized airborne hyperspectral images to map lead and arsenic contamination in the Guadiamar flood plain, Andalusia. Similarly, Wu et al. (2011) reported satisfactory outcomes in heavy metal mapping in Nanjing City of China, using simulated HyMap data. They reported that direct predictions based on hyperspectral images often require signals from bare soils, which can be achieved during winter or early spring or when there is low vegetation coverage due to crop rotation in agricultural areas. Therefore, remote sensing has been instrumental in monitoring and managing hazardous waste and sites. Multispectral sensors and airborne hyperspectral images have provided valuable data for assessing pollution, identifying contamination, and mapping the distribution of heavy metals. These technologies offer a non-intrusive and comprehensive approach to understanding and addressing environmental concerns related to hazardous waste.
The incorporation of diverse advanced technologies has significantly improved the field of environmental monitoring, especially in the identification and control of hazardous waste. Spectroscopy, rooted in chemistry and astronomy, has been widely applied in remote sensing. Visible and near-infrared reflectance spectroscopy is presented as an environmentally friendly and cost-effective technique for approximating concentrations of heavy metals in soil. Machine learning methods, such as extreme gradient boosting and neural networks, enhance the accuracy of predictions regarding heavy metal pollution when combined with spectroscopic data. Ground-based monitoring sensors, encompassing both manual and automatic networks and utilizing machine learning models, play a role in robust air quality monitoring. Aerial imaging, covering historical images and hyperspectral imagery, provides a comprehensive perspective on hazardous waste sites, aiding in the evaluation of pollution. Unmanned Aerial Vehicles (UAVs) equipped with sensors are essential for real-time identification and tracking of contaminant plumes, thereby improving response capabilities. Ground robotics, equipped with gas-sensitive sensors, are valuable in enclosed spaces for the detection of hazardous substances. Ultimately, remote sensing, facilitated by multispectral and hyperspectral sensors, serves as a potent tool for systematic Earth observation, particularly in the identification of heavy metal contamination and mapping the distribution of pollutants. This holistic approach underscores the varied strategies employed to tackle environmental issues and emphasizes the pivotal role of advanced technologies in preserving ecosystems.
4 Maximizing safety in hazardous environments with AI-Driven monitoring
Nowadays awareness of the safety of employees working in the production and manufacturing operations in different industrial environments that involve hazardous substances and materials has become of utmost interest (Farrokhi-Asl et al., 2020). Thus, monitoring the concentration and leakage of pollutants (Fung et al., 2019) and development of safe and efficient methods (Fung et al., 2019) to reduce the exposure of humans and the detrimental risks for the environment, with fewer accidents provoked by hazardous substances (Wong et al., 2018; Wang et al., 2020), are becoming a priority in the implementation of safety plan management in many sectors of the economy (Binajjaj et al., 2018). In pollutant discharge events, immediate, precise, and intelligent intervention is needed to alarm, prevent, and control hazardous leakage (Mendil et al., 2022; Wang B. et al., 2023). Due to the fact that humans cannot identify in time possible threats, because the majority of gases are odorless, colorless, and tasteless (Visvanathan et al., 2018), implementation in environmental monitoring and detection of artificial intelligence based devices is getting more popular (Daam et al., 2019; Emaminejad and Akhavian, 2022). The popularity of AI and unmanned machines arises from the effective, real-time, automated solutions that these technologies provide when placed in hazardous environments. They can detect and isolate possible threats before they cause harm, with less or no human involvement (Palacín et al., 2019; Das et al., 2020; Jiang et al., 2022). Recent advances have been developed for the combination of autonomous robots with sensors that are flexible and easily and remotely deployed in unsafe and toxic environments (Ristic et al., 2017). They can improve the safety of workers and neighborhood areas without the risk of involving human life (Fan et al., 2019). These devices are embedded with systems capable of exploring, monitoring, detecting, and alarming, in case hazardous events occur (Maedche et al., 2019). For example, Joshna et al. (Joshna et al., 2019) developed an independent robot using Arduino UNO (R3), equipped with sensors that can detect and identify toxic gases. It can also perform degasification making use of oxidizing agents that are sprayed on the gases, reducing their deleterious effects. Fan et al. (Fan et al., 2019) developed a mobile robotic system equipped with an E-nose that is highly accurate in detecting and identifying different toxic gases like CO and NO2 present in the environment. It can be used in fire and emergency response departments. Gallego et al. (2015) developed an unmanned aerial vehicle (UAV) equipped with metal oxide semiconductor (MOX) gas sensors, capable of detection of toxic gas leaks, to monitor gas pipes in outdoor areas. Novelty traits of the system include improved velocity and reduced energy consumption and investment costs.
A wireless microcontroller (MCU) offers acquisitions of real-time data, and a GPS/GSM (Global Positioning System/Global System for Mobile Communication) modem offers accurate registration and communication of the location and route in addition to measuring the gas concentration. With the intention of maximizing safety for staff workers in factories, Shi et al. (2016) Burgués et al. (2019) proposed a Crazyflie 2.0 nano-drone assembled with a MOX gas sensor. This nano-air vehicle (NAV) can accurately localize the gas source with instantaneous gas distribution mapping, which requires less time for concentration measurements than other previous devices. Similarly, Das et al. (Das et al., 2020) proposed a robot that can be deployed in an unknown and uneven environment that can recognize hazardous gases (carbon dioxide, vaporized alcohol, and liquefied petroleum gas) with an average accuracy of 98%. The robot can be operated to avoid collision obstacles and detect the presence of humans, while mapping in real-time the locations of the gases detected through a GPS module. A mobile robot combined with MOX gas sensors to detect early gas leaks in hazardous events was also developed by Palacín et al. (2019). The innovative system has 16 E-nose gas sensors that are low-cost. They can detect two chemicals at low concentrations that are located at broad distances from the source. In case two gas sources are present simultaneously, the mobile robot will detect the chemical that has the highest concentration. To detect and identify possible threats before damage takes place, Chen et al. (2023) proposed an AI-based monitoring system using processing parts that were designed to be simple. It had a 96.1% efficiency of detecting and localizing in real time external vibrational disturbance of a buried pipeline. Table 1 summarizes some important research studies that have used AI applications for monitoring and detection of hazardous substances.
Monitoring of hazardous environments to increase safety and to protect humans while they are operating in them was the goal of the work of Cheung et al. (2018). These authors developed a wireless sensor network (WSN) monitoring system with sensor nodes that can gather information regarding hazardous gases, temperature and humidity. They are embedded into a building information model (BIM) that displays the safety status in real-time with colors and shows the precise location of a possible dangerous event in advance. When high concentrations of gases are detected, the system is activated automatically using a flashing alarm that can warn the staff and workers. A ventilator and other safety devices are enabled to reduce toxic and flammable gas flow, which will prevent hazardous accidents. In a similar way, Jualayba et al. (2018) designed a monitoring and warning system that can display the safety status with colors when different levels of gases are detected. An exhaust fan is triggered when a medium level is displayed. At dangerous levels, an alarm buzzer is activated to inform people of a gas leakage and the need to decrease the concentration of the detected gas. The system has sensors for hydrogen, liquefied petroleum gas, and methane.
Wilson (2012) suggested a multifunctional gas detection system utilizing an Arduino Uno R3 and an Internet of Things module equipped with an MQ-6 gas sensor, capable of identifying methane, propane, and butane. The primary objective is to avert potential dangers in industrial settings. Upon surpassing predetermined gas threshold levels, the system activates a combination of a light-emitting diode (LED), buzzer, and notification message. There have been additional AI-powered devices devised for identifying hazardous gases in factories and industrial environments, all geared towards enhancing employee safety. They can collect data in real-time and identify the source of the gas leak and map the location (Manes et al., 2016). They are built with climatic sensors in addition to the gas sensors. They offer accurate, continuous monitoring of gas concentrations and are cost-effective (Thomas et al., 2018). Wearable smart sensing devices attached to workers cloths can be used for continuous gas monitoring in different industrial and other toxic areas. They provide accurate and real-time data acquisition that allows users to take rapid safety measures (Antolín et al., 2017). For instance, Binajjaj et al. (2018) proposed a wearable gas sensor network provided with an MQ-7 sensor that can detect both CO and CH4, accurately and rapidly, using an accelerometer and an on-demand on/off switch that reduces energy consumption. It has a GPS and communication module that can transmit messages to other employees.
Wu et al. (2011) developed „WE-Safe”, a wearable IoT sensor node capable of promptly warning workers in hazardous environments. This device is based on LoRa wireless technology. It has one microcontroller unit (MCU) and sensors for detection of CO, CO2, as well as other sensors for environmental conditions (temperature, humidity, and UV light). It gives real time data and has a low energy exhaustion. Other researchers have designed devices with accurate and real time detection using MQ2, MQ5 gas sensors, which are capable of detecting hazardous gases in people’s houses. The devices alarm the users through a buzzer and a short message service (SMS) notification is sent to tell them that the gas leakage needs to be controlled (Karthika et al., 2019; Panganiban, 2019).
Another application based on AI technology, as a strategy to control indoor ventilation by rapidly getting rid of PM, was developed by Kim et al. (2021). The ventilation system has a high removal rate of the hazardous airborne particles, which is done in a short time with low power consumption. Mendil et al. (2022) proposed a machine learning (ML)-based surrogate model for transport and dispersion of air pollutants that can predict, fast and accurately, the concentration and dose of pollutants in urban areas. Asha et al. (2022) designed an IoT and ML based model (ETAPM-AIT) for air quality monitoring that uses a sensor array for eight pollutants, such as NH3, CO, NO2, CH4, CO2, and PM2.5. It also measures temperature and humidity. In this model an AAA-based ENN is used to classify air pollutants, and the model provides real-time detection of the pollutants. It has an alarm option that can alert people when hazardous pollutants are detected in the air. For safety purposes, Seo and Lee (2022) used an algorithm based on a CNN to accurately classify hazardous compounds to avoid hazardous chemicals being improperly used in laboratory environments. An intelligent system equipped with different sensors for CO, CO2, NO2, CH4, and H2S measurements using an Arduino Uno card and IoTs module monitors landfill biogas to prevent potential hazardous emissions (Mabrouki et al., 2021). Oduah and Ogunye (2023) developed a low-cost, smart, remote-sensing septic tank that can be used onsite to prevent sewage overflow. It uses an ultrasonic sensor for detection and monitoring the wastewater level in the septic tank and a GSM module to send SMS alerts to the users, avoiding spills of contaminants that can cause health problems.
Seker (2022) developed a real-time smart, cost-effective waste collection system that employs IoT with technologies like radio frequency identification (RFID), GIS, and ground penetrating radar systems (GPRS). It is effective for municipal waste collection and transportation and has the goal of reducing environmental pollution. Transportation and storage of hazardous materials can cause considerable risk to human life and the environment, due to their toxicity, corrosiveness, explosiveness, and radioactive characteristics (Mabrouk et al., 2017; Paredes-Belmar et al., 2017). In this respect, to design of a remote monitoring system based on a wireless sensor network that can offer real-time information on the vehicle that is transporting the hazardous materials. It can report the status of the vehicle (its attitude and tire pressure) and its location. In addition, the temperature of the transported hazardous chemicals is reported. This remote monitoring of hazardous materials during transportation provides safety measures for the workers and for citizens, minimizing the occurrence of accidents.
Hosseini and Verma (2021) proposed an analytical method that can determine the best route for transportation of hazardous materials, to assure the safety of inhabitants. It can exclude route zones that have high population density. Over the years, pollution of water resources has increased, making uncontaminated water bodies even more scarce all over the globe. The pollution has destroyed aquatic ecosystems and resulted in health and safety issues for humanity (Zhai et al., 2021; Yusuf et al., 2023). Protecting water resources and preventing the release of different pollutants represent the first steps in ensuring safety for the environment and people (Berman et al., 2020). Various approaches for monitoring the physico-chemical parameters of water resources have led to the implementation of novel smart technologies (Geetha and Gouthami, 2016; Huang et al., 2018). Water-quality smart monitoring systems using the Internet of Things (IoT) has gained popularity by engaging WSNs that offer continuous, remote and real-time monitoring and measurement of different water parameters. They can identify pollution sources and give the opportunity of early warnings, when possible threats or hazards are detected, and they can provide proper safety measures (Pule et al., 2017; Lakshmikantha et al., 2021).
A low cost system making use of the IoT for detection of water parameters in real time, like temperature, turbidity, conductivity, pH, and dissolved oxygen, which uses raspberry PI B+ as a core controller, has been designed (Vijayakumar and Ramya, 2015). Subsequently, Pasika and Gandla (Pasika and Gandla, 2020) proposed a low cost, efficient system based on the IoT using ThinkSpeak and various sensors to monitor drinking water quality in real time. In a similar way, Kumer et al. (Kumer et al., 2021) used ThinkSpeak and an Arduino Mega 2560 microcontroller in a device to analyze in real-time water parameters to detect levels of contamination when it happens and to warn inhabitants of hazardous health risks. It is very accurate and inexpensive.
Khan et al. (2020) developed a system that can monitor water quality for an industrial effluent treatment plant. It is composed of wireless sensor networks, a GSM module for notifications in case of emergency, an Arduino Uno R3 microcontroller, and an IoT-based cloud server. This system proved to be efficient and cost-effective. It gives continuous, real-time water quality monitoring, which allows the local authorities the benefit of supervising and checking to see if the released water from a specific industry is polluted or not. To tackle the problem of unreachable water bodies that are in secluded areas, Vasudevan and Baskaran (2021) proposed an innovative unmanned surface vehicle (USV) based on the IoT and sensors that provided control of these waters and diminished water pollution. They confirmed its efficiency, low investment, reduced energy consumption, and real-time data acquisition for surface water quality monitoring. Similarly, Ryu (2022) proposed an unmanned aircraft system (UAS) for water sampling and monitoring, which can be used in potentially hazardous environments, minimizing the involvement of humans. Its UASWQP platform can take water samples from various points and is effective in presenting real-time data regarding measurements of water parameters.
Adeleke et al. (2023) developed a highly accurate water quality monitoring system interfaced with sensors and machine learning algorithms to predict the level of water pollution. The remote system gathers water quality data in real-time and is built with an automated control system that can apply water treatment when the level of pollutants are above the standard limits, which reduces the expansion of diseases from contaminated water (Adeleke et al., 2023). A smart water monitoring system that collects real-time data for water quality and quantity was designed by Jha et al. (2018). The system uses sensors connected to an Arduino UNOTM and a Raspberry PiTM(RPi) microprocessor. It can avoid hazardous events caused by contaminated water seepage into drinking water sources by sending SMS and e-mail notifications when the monitored water is contaminated, which gives the opportunity for authorities and consumers to take rapid safety measures.
5 Advancements and challenges
5.1 BeeTox AI and other models
In a recent study (Moreira-Filho et al., 2021), a novel web application called BeeToxAI was introduced. It utilizes AI to assess the acute toxicity of chemicals to Apis mellifera, commonly known as the honey bee. BeeToxAI offers users a comprehensive set of features, including the classification as toxic or non-toxic of acute contact toxicity and acute oral toxicity endpoints. In addition, confidence scores for the prediction are given along with a visual representation of the results through maps. These color-coded maps illustrate the relative contribution of chemical fragments to toxicity. Since pesticides contain toxic substances that are injurious to health (Demirel and Kumral, 2021), AI algorithms are needed for controlling and tracking them, to reduce their toxicity. Because nitrogen based chemical fertilizers increase the amount of nitrate in groundwater, AI might be used to control excessive nitrate in soil.
The analysis of water quality as a source of irrigation can be done using AI-ANN models (Ostad-Ali-Askari et al., 2017). Over the past decade, a multitude of studies have been conducted to forecast the efficacy of machine learning in removing heavy metals from soil (Table 2). These investigations have focused on predicting the effectiveness of various techniques for soil remediation and heavy metal removal utilizing machine learning methodologies (Zafar et al., 2017; Zhu et al., 2019; Bhagat et al., 2020). Models used for optimizing and predicting heavy metal removal have encompassed black box, fuzzy logic, kernel, evolutionary, and hybrid models, and each offers distinct advantages.
In a study conducted by Talebkeikhah et al. (2022), which focussed on the adsorption of Pb (II) on biochar, eleven models were employed, which included group-data handling methods such as support vector machines (SVM), radiofrequency (RF), adaptive neuro-fuzzy interference systems (ANFIS), multilayer perception (MLP), and Decision Tree. Additionally, to evaluate the significance of coupling in creating predictive models for estimating adsorption efficiency, the researchers developed four coupled predictive models using the grasshopper optimization algorithm (GOA) and the bat algorithm, and they combined ANFIS and MLP.
Chen et al. (2020) developed a CNN architecture specifically designed for deep calibration using near-infrared (NIR) spectroscopy data. Their study focused on assessing the level of water pollution originating from domestic and industrial sources, with the aim of enabling suitable agricultural irrigation practices. The researchers successfully established intelligent spectroscopic models using the CNN architecture, which could be instrumental in addressing water recycling and conservation issues in agricultural cultivation.
The field of medicine is experiencing gradual transformations due to the development of AI. Various medical disciplines, including clinical, diagnostic, rehabilitative, surgical, and predictive practices, are being influenced by AI applications (Secinaro et al., 2021). AI technologies have the capacity to process and analyze large volumes of data from diverse modalities to aid disease detection and guide clinical decision-making (Cho et al., 2020).
5.2 Medical waste
Recently, global healthcare advancements have led to an increase in the generation of medical waste, thereby contributing to a rising trend in waste production (Bazrafshan and Kord Mostafapoor, 2011). Various types of hazardous waste, such as hospital waste, dental waste from medical laboratories, blood wastage, and clinical waste, pose potential risks to both human health and the environment (Bazrafshan and Kord Mostafapoor, 2011). Medical waste (MW) encompasses infectious waste, sharp waste, toxic waste, chemical waste, and pharmaceutical waste. Inadequate management of medical waste can lead to the transmission of infectious diseases, including AIDS, hepatitis, typhoid, and many others (Askarian et al., 2010; Aghapour et al., 2013). These wastes have the potential to pollute the environment and spread acute and latent viral infections. They contain significant amounts of bacteria and viruses. If they are not properly controlled, they have the potential to contaminate the environment, which includes air, water, plants, animals, and land (Rajan et al., 2019). This can lead to the spread of disease. The quality of life, physical and emotional health, and overall health of medical personnel and patients are all seriously threatened by MW (Nwachukwu et al., 2013).
In a recent study (Zhou et al., 2022), a novel image recognition system was introduced called Deep MW for the purpose of sorting medical waste. Deep MW utilizes a CNN as its underlying architecture. The system aims to enhance the ease, accuracy, and efficiency of medical waste sorting and recycling processes, while also reducing the risk of occupational exposure for workers in medical waste facilities. Other similar systems exist, like iWaste (Chen et al., 2020), Deep MW excels in recognizing and sorting diverse categories of medical waste, showcasing remarkable accuracy and practicality in classification. Its proficiency makes it adaptable for expanding its use to other object classifications. Amidst the ongoing COVID-19 pandemic and other outbreaks, artificial intelligence has proven pivotal in creating surveillance tools aimed at identifying individuals not adhering to quarantine regulations. Monitoring bracelets exemplify one such tool. Furthermore, AI-enhanced technologies, such as smartphones and thermal cameras, are utilized for detecting fever and signs of illness. (Whitelaw et al., 2020).
5.3 Other applications
These advancements in AI have significantly contributed to improving surveillance and monitoring capabilities in public health and safety. A country like Taiwan treats coronavirus patients based on their travel history and symptoms by integrating data from the immigration and customs database with the national medical insurance database (Wang J. et al., 2023). Based on research conducted by Taiwan’s Occupational Safety and Health Administration (OSHA), a significant number of surgical staff, approximately 50,000 individuals, face exposure to smoke hazards during procedures involving the use of lasers and electrocautery (Wang et al., 2020). This exposure poses potential risks to the health and safety of surgical personnel. AI can be used for assessing the characterization of the smoke emitted and also for carrying out an AI-powered air quality assessment (Kaginalkar et al., 2021).
Industry 4.0 is revolutionizing the way companies manufacture, improve, and distribute their products, and AI is widely recognized as a crucial technology for it (Mao et al., 2019). Its incorporation in these domains has significantly transformed and enhanced various aspects of industrial processes, enabling advanced automation, data-driven decision-making, predictive maintenance, and optimization of manufacturing operations (Figure 3). AI’s capabilities have played a pivotal role in driving efficiency, productivity, and innovation in the manufacturing sector, making it a key enabler of the Industry 4.0 paradigm. Industry 4.0 has brought about a number of cutting-edge technologies, and this new phase of AI development is known as AI 2.0 (Pan, 2016). The utilization of AI in smart factories and its application in modern industrial sectors have been acknowledged as transformative (Mao et al., 2019). It enables efficient decision-making processes based on real-time and historical data, minimizing the need for human intervention.
Liu et al. (2019) proposed the integration of intelligent manufacturing in-shop service modules with Big Data analytics, to enhance productivity in various industries. For the facial mask industry, Zhang (2019) suggested a remote design system utilizing AI for image processing and pattern recognition, coupled with a client wireless communication. Yan et al. (2018) highlighted the significance of AI algorithms in forecasting the lifespan of industrial equipment, particularly in the context of Industry 4.0.
AI, machine learning, and autonomous technologies have significantly impacted the mining industry by detecting hazards and reducing risks [136, 137]. The introduction of autonomous trucks and the utilization of AI and machine learning have brought cost savings, increased productivity, improved worker safety, and continuous production (Hyder et al., 2018). AI systems analyzing geological, topographical, mineralogical, and mapping data can identify anomalies, locate potential areas of interest, and facilitate autonomous drilling operations (Hyder et al., 2018).
In the architecture, engineering, and construction (AEC) sector, AI has been increasingly employed to enhance existing procedures and address challenges. Applications and algorithms of AI in the AEC industry have been studied. Virtual reality and augmented reality have been used for hazard identification and risk assessment in construction environments (Von Meding et al., 2009; Perlman et al., 2014; Abioye et al., 2021).
Advancements in artificial intelligence (AI) and machine learning (ML) have significantly improved efforts in mitigating pollution (Chen et al., 2023). State-of-the-art technologies are being utilized to improve air quality modeling, integrating a variety of data sources such as satellite imagery and meteorological data for more accurate predictions. The amalgamation of AI with satellite technology allows for comprehensive monitoring of environmental shifts, assisting in the identification of pollution sources. The introduction of edge computing enables real-time analysis of environmental data at its origin, facilitating swift responses to pollution incidents. Hybrid models, which merge physics-based simulations with ML, enhance the precision of identifying pollution sources (Anthony et al., 2023). ML-driven predictive analytics forecast pollution levels, enabling proactive measures to prevent environmental deterioration. In the energy sector, AI is optimizing smart grids and energy distribution, reducing the environmental impact (Gautam et al., 2023). AI-guided robotics are deployed for pollution clean-up, autonomously recognizing and addressing contaminants. The combination of AI and block chain technology ensures transparency and traceability in environmental data (Zhao et al., 2023). ML-powered adaptive monitoring networks intelligently allocate resources for effective pollution monitoring. Mobile applications, leveraging AI, empower citizens to contribute to environmental monitoring, promoting a decentralized approach to data collection (Sahil et al., 2023). These recent strides underscore the transformative potential of AI and ML in reshaping pollution mitigation strategies, steering towards a more sustainable and resilient future (Zahed et al., 2022).
6 Limitations
In recent years, there has been a notable surge in the utilization of AI as an emerging technology across various sectors, such as agriculture, medicine and healthcare, mining and manufacturing, environmental conservation, and numerous other domains (Trasande et al., 2011; Perez Santin et al., 2021). While AI brings forth numerous benefits, it is not extempted from challenges and limitations that require efficient and notable solutions. These issues encompass the effective execution of tasks and management and mitigation of risks, as well as the assessment of hazardous materials. The requirement for significant processing power raises concerns about environmental impact and energy consumption (Singh and Kaur, 2022). Developing countries may face infrastructure limitations that hinder the efficient deployment of AI (Singh and Kaur, 2022). Data acquisition costs in data-driven agriculture remain high, limiting the widespread impact of AI on agricultural productivity (Linaza et al., 2021). Furthermore, issues of data ownership, privacy, and cybersecurity arise, necessitating standardized protocols and integration with technologies like block chain to ensure data integrity and security (Abedjan et al., 2019; Misra et al., 2020). Block chain is a system in which a record of transactions is maintained across computers that are linked.
In the manufacturing field, there is a need for further research on the application of AI in various sub-components, such as design, machinery used, assembly, and production (Zeba et al., 2021). Ethical considerations also arise, including concerns about genetic engineering, moral decision-making by AI systems, and the impact on jobs, economy, and society (Zeba et al., 2021), including privacy under surveillance. The AEC industry faces challenges related to funding, data, ethics, privacy, trust, scalability, and expertise (Motawa, 2017; Hagendorff, 2020). Resistance to technology adoption stems from concerns about job displacement, uncertainty, wealth distribution, and the complex relationship between humans and technology (Kappal, 2017). Additionally, there are challenges in understanding how AI can effectively support individual and group decision-making (Maedche et al., 2019). Overall, the application of AI in various industries brings about significant opportunities and challenges, necessitating further research, infrastructure development, and ethical considerations.
7 Conclusion and future perspectives
The development of artificial intelligence-driven sensors for environmental monitoring of hazardous substances has the potential to revolutionize how we detect and respond to environmental threats. With their ability to process large amounts of data in real-time and identify patterns and anomalies that may indicate the presence of hazardous substances, these sensors can greatly improve our ability to protect public health and the environment.
However, there are still many challenges that must be addressed, such as ensuring the accuracy and reliability of these sensors and determining the best ways to integrate them into existing monitoring systems. Despite these challenges, the potential benefits of AI-driven sensors make them a promising avenue for future research and development in the field of environmental monitoring. In the near future, AI-powered hazardous substance monitoring has the potential for enhanced automation, the creation of more sophisticated models, and the fusion of AI with novel technologies like the IoT.
AI could deliver promises about prediction, optimization, and decision-making in the near future to help the traditional construction industry keep up with the rapid speed of automation and digitalization in a variety of risky and dangerous circumstances (Pan, 2016). It can offer a wide range of risk identification factors as well as assess and deliver for prioritization, because it can monitor, recognize, analyze, and anticipate possible risk in terms of safety, quality, efficiency, and cost across teams and work areas even in the presence of significant uncertainty (Afzal et al., 2021). AI-powered monitoring systems can provide real-time, on-ground detection of potentially dangerous substances and the location of the incident sites. They may prevent any serious accidents from happening that would be harmful to human health. These systems are applicable in the fields of agriculture, health, mining, manufacturing, and other industries (Ghayvat et al., 2021; Kim et al., 2021).
AI has the potential to remodel the monitoring and detection of hazardous substances in various environments by offering real-time analysis. This can be achieved through the integration of sensors, data processing capabilities, and machine learning algorithms. By leveraging these technologies, AI systems can swiftly and accurately analyse data streams to identify potential hazards. This application is particularly valuable in industrial settings or areas susceptible to chemical spills or leaks (Leppert et al., 2012). Furthermore, AI can contribute to predictive modelling and risk assessment of hazardous substances. By examining historical data and considering various environmental factors, AI algorithms can forecast the probability and severity of incidents involving hazardous substances. This allows for proactive mitigation measures to be implemented. AI can also integrate data from multiple sources, such as sensors, satellite imagery, and public health records. By combining diverse datasets, AI algorithms provide a holistic understanding of the distribution, movement, and impacts of hazardous substances (Wu et al., 2011; Hurlbert et al., 2019; Shafique et al., 2022; Shi et al., 2022). In addition, AI can offer decision support tools for human operators engaged in hazardous substance monitoring. Through user-friendly interfaces and natural language processing, AI systems can aid in data interpretation, anomaly detection, and timely decision-making. These features assist operators in effectively managing hazardous substance situations.
Author contributions
SP: Investigation, Visualization, Writing–original draft. SM: Conceptualization, Visualization, Writing–original draft, Writing–review and editing. OW: Writing–original draft, Writing–review and editing. SK: Writing–original draft. VS: Investigation, Visualization, Writing–original draft. AS: Writing–original draft. VA: Writing–original draft. MK: Data curation, Formal Analysis, Investigation, Writing–original draft, Writing–review and editing. DH: Formal Analysis, Validation, Visualization, Writing–original draft, Writing–review and editing. NB: Formal Analysis, Investigation, Supervision, Visualization, Writing–original draft, Writing–review and editing. YC: Formal Analysis, Funding acquisition, Investigation, Resources, Supervision, Writing–original draft, Writing–review and editing.
Funding
The author(s) declare financial support was received for the research, authorship, and/or publication of this article. The Basic Science Research Program supported this research through the National Research Foundation of Korea (NRF), funded by the Ministry of Education (2019R1A6A1A11052070).
Conflict of interest
The authors declare that the research was conducted in the absence of any commercial or financial relationships that could be construed as a potential conflict of interest.
Publisher’s note
All claims expressed in this article are solely those of the authors and do not necessarily represent those of their affiliated organizations, or those of the publisher, the editors and the reviewers. Any product that may be evaluated in this article, or claim that may be made by its manufacturer, is not guaranteed or endorsed by the publisher.
References
Abedjan, Z., Boujemaa, N., Campbell, S., Casla, P., Chatterjea, S., Consoli, S., et al. (2019). Data science in healthcare: benefits, challenges and opportunities. Springer.
Abioye, S. O., Oyedele, L. O., Akanbi, L., Ajayi, A., Delgado, J. M. D., Bilal, M., et al. (2021). Artificial intelligence in the construction industry: a review of present status, opportunities and future challenges. J. Build. Eng. 44, 103299. doi:10.1016/j.jobe.2021.103299
Adeleke, I. A., Nwulu, N. I., and Ogbolumani, O. A. (2023). A hybrid machine learning and embedded IoT-based water quality monitoring system. Internet Things 22, 100774. doi:10.1016/j.iot.2023.100774
Afzal, F., Yunfei, S., Nazir, M., and Bhatti, S. M. (2021). A review of artificial intelligence based risk assessment methods for capturing complexity-risk interdependencies: cost overrun in construction projects. Int. J. Manag. Proj. Bus. 14 (2), 300–328. doi:10.1108/ijmpb-02-2019-0047
Aggestam, F., and Mangalagiu, D. (2020). Is sharing truly caring? Environmental data value chains and policymaking in Europe and Central Asia. Environ. Sci. Policy 114, 152–161. doi:10.1016/j.envsci.2020.07.012
Aghapour, P., Nabizadeh, R., Nouri, J., Monavari, M., and Yaghmaeian, K. (2013). Analysis of the health and environmental status of sterilizers in hospital waste management: a case study of hospitals in Tehran. Waste Manag. Res. 31 (3), 333–337. doi:10.1177/0734242x12472706
Agrawal, A., Kumar, L., Kumar, P., and Jha, V. K. (2017). IOT based hazardous gas detection system using AVR microcontroller. Int. Res. J. Eng. Technol. 4 (3), 1–5.
Ahmadi, M., Jorfi, S., Birgani, Y. T., and Ghaedrahmat, Z. (2021). Monitoring and application of artificial neural network model for prediction of organophosphorus pesticides residue in Ahvaz water treatment plants. Biointerface Res. Appl. Chem. 11 (6), 14032–14043.
Ahmed, S. A., Popov, V. L., Topalov, A. V., and Shakev, N. G. (2016). Perimeter detection and surveillance of polluted areas by robotized agents in a hybrid wireless sensor network. IFAC-PapersOnLine 49 (29), 247–252. doi:10.1016/j.ifacol.2016.11.061
Ai, W., Chen, G., Yue, X., and Wang, J. (2023). Application of hyperspectral and deep learning in farmland soil microplastic detection. J. Hazard. Mater. 445, 130568. doi:10.1016/j.jhazmat.2022.130568
Amado, T. M., and Cruz, J. C. D. (2018). “Development of machine learning-based predictive models for air quality monitoring and characterization,” in TENCON 2018-2018 IEEE Region 10 Conference.
Anthony, D., Siriwardana, H., Ashvini, S., Pallewatta, S., Samarasekara, S. M., Edirisinghe, S., et al. (2023). Trends in marine pollution mitigation technologies: scientometric analysis of published literature (1990-2022). Regional Stud. Mar. Sci. 66, 103156. doi:10.1016/j.rsma.2023.103156
Antolín, D., Medrano, N., Calvo, B., and Pérez, F. (2017). A wearable wireless sensor network for indoor smart environment monitoring in safety applications. Sensors 17 (2), 365. doi:10.3390/s17020365
Asha, P., Natrayan, L., Geetha, B., Beulah, J. R., Sumathy, R., Varalakshmi, G., et al. (2022). IoT enabled environmental toxicology for air pollution monitoring using AI techniques. Environ. Res. 205, 112574. doi:10.1016/j.envres.2021.112574
Askarian, M., Heidarpoor, P., and Assadian, O. (2010). A total quality management approach to healthcare waste management in Namazi Hospital, Iran. Waste Manag. 30 (11), 2321–2326. doi:10.1016/j.wasman.2010.06.020
Bachmann, T. M. (2006). Hazardous substances and human health: exposure, impact and external cost assessment at the European scale. Elsevier.
Baran, W., Adamek, E., Ziemiańska, J., and Sobczak, A. (2011). Effects of the presence of sulfonamides in the environment and their influence on human health. J. Hazard. Mater. 196, 1–15. doi:10.1016/j.jhazmat.2011.08.082
Basel, A. M., Nguyen, K. T., Arnaud, E., and Craparo, A. C. (2023). The foundations of big data sharing: a CGIAR international research organization perspective. Front. Environ. Sci. 11, 1107393. doi:10.3389/fenvs.2023.1107393
Bazoobandi, A., Emamgholizadeh, S., and Ghorbani, H. (2022). Estimating the amount of cadmium and lead in the polluted soil using artificial intelligence models. Eur. J. Environ. Civ. Eng. 26 (3), 933–951. doi:10.1080/19648189.2019.1686429
Bazrafshan, E., and Kord Mostafapoor, F. (2011). Survey of medical waste characterization and management in Iran: a case study of Sistan and Baluchestan Province. Waste Manag. Res. 29 (4), 442–450. doi:10.1177/0734242x10374901
Benger, M. J. (2004). The EPA remote sensing archive,” in The Landscape Science Seminar Series. Reston, VA: Video Teleconference.
Berman, I., Zereik, E., Kapitonov, A., Bonsignorio, F., Khassanov, A., Oripova, A., et al. (2020). Trustable environmental monitoring by means of sensors networks on swarming autonomous marine vessels and distributed ledger technology. Front. Robotics AI 7, 70. doi:10.3389/frobt.2020.00070
Bhagat, S. K., Tung, T. M., and Yaseen, Z. M. (2020). Development of artificial intelligence for modeling wastewater heavy metal removal: state of the art, application assessment and possible future research. J. Clean. Prod. 250, 119473. doi:10.1016/j.jclepro.2019.119473
Bharati, M. S. S., and Soma, V. R. (2021). Flexible SERS substrates for hazardous materials detection: recent advances. Opto-Electronic Adv. 4 (11), 210048. doi:10.29026/oea.2021.210048
Binajjaj, A., Sheltami, T., Aliyu, F., and Kaosar, M. (2018). Design and implementation of a wearable gas sensor network for oil and gas industry workers. J. Comput. 13 (3), 300–308. doi:10.17706/jcp.13.3.300-308
Bolan, N., Sarkar, B., Yan, Y., Li, Q., Wijesekara, H., Kannan, K., et al. (2021). Remediation of poly-and perfluoroalkyl substances (PFAS) contaminated soils–To mobilize or to immobilize or to degrade? J. Hazard. Mater. 401, 123892. doi:10.1016/j.jhazmat.2020.123892
Bølviken, B., Honey, F., Levine, S., Lyon, R., and Prelat, A. (1977). Detection of naturally heavy-metal-poisoned areas by Landsat-1 digital data. Dev. Econ. Geol. 9, 457–471. Elsevier.
Burgués, J., Hernández, V., Lilienthal, A. J., and Marco, S. (2019). Smelling nano aerial vehicle for gas source localization and mapping. Sensors 19 (3), 478. doi:10.3390/s19030478
Chen, H., Chen, A., Xu, L., Xie, H., Qiao, H., Lin, Q., et al. (2020). A deep learning CNN architecture applied in smart near-infrared analysis of water pollution for agricultural irrigation resources. Agric. Water Manag. 240, 106303. doi:10.1016/j.agwat.2020.106303
Chen, H., Wong, R. C.-K., Park, S., and Hugo, R. (2023). An AI-based monitoring system for external disturbance detection and classification near a buried pipeline. Mech. Syst. Signal Process. 196, 110346. doi:10.1016/j.ymssp.2023.110346
Cheung, W.-F., Lin, T.-H., and Lin, Y.-C. (2018). A real-time construction safety monitoring system for hazardous gas integrating wireless sensor network and building information modeling technologies. Sensors 18 (2), 436. doi:10.3390/s18020436
Cho, B.-J., Choi, Y. J., Lee, M.-J., Kim, J. H., Son, G.-H., Park, S.-H., et al. (2020). Classification of cervical neoplasms on colposcopic photography using deep learning. Sci. Rep. 10 (1), 13652–13710. doi:10.1038/s41598-020-70490-4
Choubin, B., Abdolshahnejad, M., Moradi, E., Querol, X., Mosavi, A., Shamshirband, S., et al. (2020). Spatial hazard assessment of the PM10 using machine learning models in Barcelona, Spain. Sci. Total Environ. 701, 134474. doi:10.1016/j.scitotenv.2019.134474
Cruz, F. R. G., Binag, M. G., Ga, M. R. G., and Uy, F. A. A. (2018). “Flood prediction using multi-layer artificial neural network in monitoring system with rain gauge, water level, soil moisture sensors,” in TENCON 2018-2018 IEEE Region 10 Conference.
Crystal-Ornelas, R., Varadharajan, C., O’Ryan, D., Beilsmith, K., Bond-Lamberty, B., Boye, K., et al. (2022). Enabling FAIR data in Earth and environmental science with community-centric (meta) data reporting formats. Sci. data 9 (1), 700. doi:10.1038/s41597-022-01606-w
Daam, M. A., Chelinho, S., Niemeyer, J. C., Owojori, O. J., De Silva, P. M. C., Sousa, J. P., et al. (2019). Environmental risk assessment of pesticides in tropical terrestrial ecosystems: test procedures, current status and future perspectives. Ecotoxicol. Environ. Saf. 181, 534–547. doi:10.1016/j.ecoenv.2019.06.038
Das, T., Sut, D. J., Gupta, V., Gohain, L., Kakoty, P., and Kakoty, N. M. (2020). “A mobile robot for hazardous gas sensing,” in 2020 International Conference on Computational Performance Evaluation (ComPE), Shillong, India, 02-04 July 2020 (IEEE).
Demirel, M., and Kumral, N. A. (2021). “Artificial intelligence in integrated pest management,” in Artificial intelligence and IoT-based technologies for sustainable farming and smart agriculture (Hershey, Pennsylvania, United States: IGI Global), 289–313.
Emaminejad, N., and Akhavian, R. (2022). Trustworthy AI and robotics: implications for the AEC industry. Automation Constr. 139, 104298. doi:10.1016/j.autcon.2022.104298
Errico, A., Angelino, C. V., Cicala, L., Podobinski, D. P., Persechino, G., Ferrara, C., et al. (2014). SAR/multispectral image fusion for the detection of environmental hazards with a GIS. Earth Resour. Environ. Remote Sensing/GIS Appl. V. doi:10.1117/12.2066476
Fan, H., Hernandez Bennetts, V., Schaffernicht, E., and Lilienthal, A. J. (2019). Towards gas discrimination and mapping in emergency response scenarios using a mobile robot with an electronic nose. Sensors 19 (3), 685. doi:10.3390/s19030685
Farrokhi-Asl, H., Makui, A., Ghousi, R., and Rabbani, M. (2020). Developing a hazardous waste management system with consideration of health, safety, and environment. Comput. Electr. Eng. 82, 106553. doi:10.1016/j.compeleceng.2020.106553
Fung, A. G., Rajapakse, M. Y., McCartney, M. M., Falcon, A. K., Fabia, F. M., Kenyon, N. J., et al. (2019). Wearable environmental monitor to quantify personal ambient volatile organic compound exposures. ACS sensors 4 (5), 1358–1364. doi:10.1021/acssensors.9b00304
Galán-Freyle, N. J., Ospina-Castro, M. L., Medina-González, A. R., Villarreal-González, R., Hernández-Rivera, S. P., and Pacheco-Londoño, L. C. (2020). Artificial intelligence assisted mid-infrared laser spectroscopy in situ detection of petroleum in soils. Appl. Sci. 10 (4), 1319. doi:10.3390/app10041319
Gallego, V., Rossi, M., and Brunelli, D. (2015). “Unmanned aerial gas leakage localization and mapping using microdrones,” in 2015 IEEE Sensors Applications Symposium (SAS), Zadar, Croatia, 13-15 April 2015 (IEEE).
Garofalo, D. (2003). Aerial photointerpretation of hazardous waste sites: an overview. Boca Raton, FL, USA: CRC Press LLC.
Gautam, K., Sharma, P., Dwivedi, S., Singh, A., Gaur, V. K., Varjani, S., et al. (2023). A review on control and abatement of soil pollution by heavy metals: emphasis on artificial intelligence in recovery of contaminated soil. Environ. Res. 225, 115592. doi:10.1016/j.envres.2023.115592
Geetha, S., and Gouthami, S. (2016). Internet of things enabled real time water quality monitoring system. Smart Water 2 (1), 1–19. doi:10.1186/s40713-017-0005-y
Gerhardt, N., Clothier, R., and Wild, G. (2014). “Investigating the practicality of hazardous material detection using unmanned aerial systems,” in 2014 IEEE Metrology for Aerospace (MetroAeroSpace), Benevento, Italy, 29-30 May 2014 (IEEE).
Ghayvat, H., Awais, M., Gope, P., Pandya, S., and Majumdar, S, (2021). Recognizing suspect and predicting the spread of contagion based on mobile phone location data (counteract): a system of identifying covid-19 infectious and hazardous sites, detecting disease outbreaks based on the internet of things, edge computing, and artificial intelligence. Sustain. Cities Soc. 69, 102798. doi:10.1016/j.scs.2021.102798
Hagendorff, T. (2020). The ethics of AI ethics: an evaluation of guidelines. Minds Mach. 30 (1), 99–120. doi:10.1007/s11023-020-09517-8
Haldorai, A., Suriya, M., and Balakrishnan, M. (2024). An improved single short detection method for smart vision-based water garbage cleaning robot. Cogn. Robot. 4, 19–29. doi:10.1016/j.cogr.2023.11.002
Hosseini, S. D., and Verma, M. (2021). Equitable routing of rail hazardous materials shipments using CVaR methodology. Comput. Operations Res. 129, 105222. doi:10.1016/j.cor.2021.105222
Huang, C., Chen, Y., Zhang, S., and Wu, J. (2018). Detecting, extracting, and monitoring surface water from space using optical sensors: a review. Rev. Geophys. 56 (2), 333–360. doi:10.1029/2018rg000598
Hurlbert, M., Krishnaswamy, J., Johnson, F. X., Rodríguez-Morales, J. E., and Zommers, Z. (2019). Risk management and decision making in relation to sustainable development.
Hyder, Z., Siau, K., and Nah, F. F.-H. (2018). Use of artificial intelligence, machine learning, and autonomous technologies in the mining industry.
Jeong, J., and Choi, J. (2022). Artificial intelligence-based toxicity prediction of environmental chemicals: future directions for chemical management applications. Environ. Sci. Technol. 56 (12), 7532–7543. doi:10.1021/acs.est.1c07413
Jha, M. K., Sah, R. K., Rashmitha, M., Sinha, R., Sujatha, B., and Suma, a. (2018). “Smart water monitoring system for real-time water quality and usage monitoring,” in 2018 International Conference on Inventive Research in Computing Applications (ICIRCA).
Jia, X., Cao, Y., O Connor, D., Zhu, J., Tsang, D. C., Zou, B., et al. (2021). Mapping soil pollution by using drone image recognition and machine learning at an arsenic-contaminated agricultural field. Environ. Pollut. 270, 116281. doi:10.1016/j.envpol.2020.116281
Jiang, J., Lin, C., Han, G., Abu-Mahfouz, A. M., Shah, S. B. H., and Martínez-García, M. (2022). How AI-enabled SDN technologies improve the security and functionality of industrial IoT network: architectures, enabling technologies, and opportunities. Digital Commun. Netw. 9, 1351–1362. doi:10.1016/j.dcan.2022.07.001
Joshna, V., Kashyap, M., Ananya, V., and Manitha, P. (2019). “Fully autonomous robot to detect and degasify hazardous gas after flood disaster,” in 2019 2nd International Conference on Power and Embedded Drive Control (ICPEDC).
Jualayba, M., Regio, K., Quiozon, H., and Destreza, A. (2018). “Hazardous gas detection and notification system,” in 2018 IEEE 10th International Conference on Humanoid, Nanotechnology, Information Technology, Communication and Control, Environment and Management (HNICEM).
Kaginalkar, A., Kumar, S., Gargava, P., and Niyogi, D. (2021). Review of urban computing in air quality management as smart city service: an integrated IoT, AI, and cloud technology perspective. Urban Clim. 39, 100972. doi:10.1016/j.uclim.2021.100972
Karthika, S., Vanitha, U., Rejina, P., Supraja, A., Sampritha, R., and Srinithi, K. (2019). Toxic gas detection using low power controller with alert system. Int. J. Innov. Technol. Explor. Eng. 8, 1074–1079. doi:10.35940/ijitee.i7655.0881019
Kemper, T., and Sommer, S. (2004). Use of airborne hyperspectral data to estimate residual heavy metal contamination and acidification potential in the Guadiamar floodplain Andalusia, Spain after the Aznacollar mining accident. Maspalomas, Canary Islands, Spain: Remote sensing for environmental monitoring, GIS applications, and geology IV.
Khan, A., Gupta, S., and Gupta, S. K. (2020). Multi-hazard disaster studies: monitoring, detection, recovery, and management, based on emerging technologies and optimal techniques. Int. J. disaster risk Reduct. 47, 101642. doi:10.1016/j.ijdrr.2020.101642
Kim, N. K., Kang, D. H., Lee, W., and Kang, H. W. (2021). Airflow pattern control using artificial intelligence for effective removal of indoor airborne hazardous materials. Build. Environ. 204, 108148. doi:10.1016/j.buildenv.2021.108148
Kim, Y., Evans, R. G., and Iversen, W. M. (2008). Remote sensing and control of an irrigation system using a distributed wireless sensor network. IEEE Trans. Instrum. Meas. 57 (7), 1379–1387. doi:10.1109/tim.2008.917198
Kostal, J., Brooks, B. W., Smith, C. A., and Devineni, G. (2022). O data, where art thou? Revolutionizing data sharing to advance our sustainability goals through smart chemical innovation. Iscience 25 (11), 105256. doi:10.1016/j.isci.2022.105256
Kumar, S. S., Mir, S. A., Wani, O. A., Babu, S., Yeasin, M., Bhat, M., et al. (2022). Land-use systems regulate carbon geochemistry in the temperate Himalayas, India. J. Environ. Manag. 320, 115811. doi:10.1016/j.jenvman.2022.115811
Kumer, S. A., Kanakaraja, P., Mounika, V., Abhishek, D., and Reddy, B. P. (2021). Environment water quality monitoring system. Mater. Today Proc. 46, 4137–4141. doi:10.1016/j.matpr.2021.02.674
Lakshmikantha, V., Hiriyannagowda, A., Manjunath, A., Patted, A., Basavaiah, J., and Anthony, A. A. (2021). IoT based smart water quality monitoring system. Glob. Transitions Proc. 2 (2), 181–186. doi:10.1016/j.gltp.2021.08.062
Leppert, J., Horner, G., Rietz, F., Ringer, J., Lammers, P. S., and Boeker, P. (2012). Near real time detection of hazardous airborne substances. Talanta 101, 440–446. doi:10.1016/j.talanta.2012.09.056
Levy, R., Remer, L., Kleidman, R., Mattoo, S., Ichoku, C., Kahn, R., et al. (2010). Global evaluation of the Collection 5 MODIS dark-target aerosol products over land. Atmos. Chem. Phys. 10 (21), 10399–10420. doi:10.5194/acp-10-10399-2010
Li, X., Peng, L., Hu, Y., Shao, J., and Chi, T. (2016). Deep learning architecture for air quality predictions. Environ. Sci. Pollut. Res. 23, 22408–22417. doi:10.1007/s11356-016-7812-9
Li, Z., Yang, Y., Chen, X., He, Y., Bolan, N., Rinklebe, J., et al. (2022). A discussion of microplastics in soil and risks for ecosystems and food chains. Chemosphere 313, 137637. doi:10.1016/j.chemosphere.2022.137637
Linaza, M. T., Posada, J., Bund, J., Eisert, P., Quartulli, M., Döllner, J., et al. (2021). Data-driven artificial intelligence applications for sustainable precision agriculture. Agronomy 11 (6), 1227. doi:10.3390/agronomy11061227
Liu, C., Zhou, Y., Cen, Y., and Lin, D. (2019). Integrated application in intelligent production and logistics management: technical architectures concepts and business model analyses for the customised facial masks manufacturing. Int. J. Comput. Integr. Manuf. 32 (4-5), 522–532. doi:10.1080/0951192x.2019.1599434
Mabrouk, A., Boulmakoul, A., Karim, L., and Lbath, A. (2017). Safest and shortest itineraries for transporting hazardous materials using split points of Voronoï spatial diagrams based on spatial modeling of vulnerable zones. Procedia Comput. Sci. 109, 156–163. doi:10.1016/j.procs.2017.05.311
Mabrouki, J., Azrour, M., Fattah, G., Dhiba, D., and El Hajjaji, S. (2021). Intelligent monitoring system for biogas detection based on the Internet of Things: mohammedia, Morocco city landfill case. Big Data Min. Anal. 4 (1), 10–17. doi:10.26599/bdma.2020.9020017
Maedche, A., Legner, C., Benlian, A., Berger, B., Gimpel, H., Hess, T., et al. (2019). AI-based digital assistants: opportunities, threats, and research perspectives. Bus. Inf. Syst. Eng. 61, 535–544. doi:10.1007/s12599-019-00600-8
Mahmoud, A. S., Mostafa, M. K., and Abdel-Gawad, S. A. (2018). Artificial intelligence for the removal of benzene, toluene, ethyl benzene and xylene (BTEX) from aqueous solutions using iron nanoparticles. Water Supply 18 (5), 1650–1663. doi:10.2166/ws.2017.225
Manes, G., Collodi, G., Gelpi, L., Fusco, R., Ricci, G., Manes, A., et al. (2016). Realtime gas emission monitoring at hazardous sites using a distributed point-source sensing infrastructure. Sensors 16 (1), 121. doi:10.3390/s16010121
Manickavasagan, A., Jayas, D., White, N., and Jian, F. (2006). Thermal imaging of a stored grain silo to detect a hot spot. Appl. Eng. Agric. 22 (6), 891–897. doi:10.13031/2013.22243
Mansoor, S., Khan, N. F., Farooq, I., Kaur, N., Manhas, S., Raina, S., et al. (2022). “Phytoremediation at molecular level,” in Phytoremediation (Elsevier), 65–90.
Mansoor, S., Kour, N., Manhas, S., Zahid, S., Wani, O. A., Sharma, V., et al. (2021). Biochar as a tool for effective management of drought and heavy metal toxicity. Chemosphere 271, 129458. doi:10.1016/j.chemosphere.2020.129458
Mao, S., Wang, B., Tang, Y., and Qian, F. (2019). Opportunities and challenges of artificial intelligence for green manufacturing in the process industry. Engineering 5 (6), 995–1002. doi:10.1016/j.eng.2019.08.013
Mendil, M., Leirens, S., Armand, P., and Duchenne, C. (2022). Hazardous atmospheric dispersion in urban areas: a Deep Learning approach for emergency pollution forecast. Environ. Model. Softw. 152, 105387. doi:10.1016/j.envsoft.2022.105387
Mertikas, S. P., Partsinevelos, P., Mavrocordatos, C., and Maximenko, N. A. (2021). “Environmental applications of remote sensing,” in Pollution assessment for sustainable practices in applied sciences and engineering (Elsevier), 107–163.
Messinger, M., and Silman, M. (2016). Unmanned aerial vehicles for the assessment and monitoring of environmental contamination: an example from coal ash spills. Environ. Pollut. 218, 889–894. doi:10.1016/j.envpol.2016.08.019
Misra, N., Dixit, Y., Al-Mallahi, A., Bhullar, M. S., Upadhyay, R., and Martynenko, A. (2020). IoT, big data, and artificial intelligence in agriculture and food industry. IEEE Internet things J. 9 (9), 6305–6324. doi:10.1109/jiot.2020.2998584
Moreira-Filho, J. T., Braga, R. C., Lemos, J. M., Alves, V. M., Borba, J. V., Costa, W. S., et al. (2021). BeetoxAI: an artificial intelligence-based web app to assess acute toxicity of chemicals to honey bees. Artif. Intell. Life Sci. 1, 100013. doi:10.1016/j.ailsci.2021.100013
Motawa, I. (2017). Spoken dialogue BIM systems–an application of big data in construction. Facilities 35 (13/14), 787–800. doi:10.1108/f-01-2016-0001
Naz, H., Sayyed, R., Khan, R. U., Naz, A., Wani, O. A., Maqsood, A., et al. (2023). Mesorhizobium improves chickpea growth under chromium stress and alleviates chromium contamination of soil. J. Environ. Manag. 338, 117779. doi:10.1016/j.jenvman.2023.117779
Nwachukwu, N. C., Orji, F. A., and Ugbogu, O. C. (2013). Health care waste management–public health benefits, and the need for effective environmental regulatory surveillance in federal Republic of Nigeria. Curr. Top. public health 2, 149–178. doi:10.5772/53196
Oduah, U. I., and Ogunye, E. B. (2023). A smart solution for preventing environmental pollution caused by overflowing onsite sewage septic tank. Heliyon 9 (4), e14925. doi:10.1016/j.heliyon.2023.e14925
Ostad-Ali-Askari, K., Shayannejad, M., and Ghorbanizadeh-Kharazi, H. (2017). Artificial neural network for modeling nitrate pollution of groundwater in marginal area of Zayandeh-rood River, Isfahan, Iran. KSCE J. Civ. Eng. 21, 134–140. doi:10.1007/s12205-016-0572-8
Palacín, J., Martínez, D., Clotet, E., Pallejà, T., Burgués, J., Fonollosa, J., et al. (2019). Application of an array of metal-oxide semiconductor gas sensors in an assistant personal robot for early gas leak detection. Sensors 19 (9), 1957. doi:10.3390/s19091957
Pan, Y. (2016). Heading toward artificial intelligence 2.0. Engineering 2 (4), 409–413. doi:10.1016/j.eng.2016.04.018
Panganiban, E. (2019). Automated hazardous gas detecting robot using wireless sensor networks with GSM-SMS alert and fire control system for households. Int. J. Adv. Trends Comput. Sci. Eng. 8 (3), 804–809. doi:10.30534/ijatcse/2019/72832019
Pant, P., Lal, R. M., Guttikunda, S. K., Russell, A. G., Nagpure, A. S., Ramaswami, A., et al. (2019). Monitoring particulate matter in India: recent trends and future outlook. Air Qual. Atmos. Health 12, 45–58. doi:10.1007/s11869-018-0629-6
Paredes-Belmar, G., Bronfman, A., Marianov, V., and Latorre-Núñez, G. (2017). Hazardous materials collection with multiple-product loading. J. Clean. Prod. 141, 909–919. doi:10.1016/j.jclepro.2016.09.163
Pasika, S., and Gandla, S. T. (2020). Smart water quality monitoring system with cost-effective using IoT. Heliyon 6 (7), e04096. doi:10.1016/j.heliyon.2020.e04096
Perez Santin, E., Rodríguez Solana, R., González García, M., García Suárez, M. D. M., Blanco Díaz, G. D., Cima Cabal, M. D., et al. (2021). Toxicity prediction based on artificial intelligence: a multidisciplinary overview. Wiley Interdiscip. Rev. Comput. Mol. Sci. 11 (5), e1516. doi:10.1002/wcms.1516
Perlman, A., Sacks, R., and Barak, R. (2014). Hazard recognition and risk perception in construction. Saf. Sci. 64, 22–31. doi:10.1016/j.ssci.2013.11.019
Pope, P., Van Eeckhout, E., Rofer, C., Baldridge, S., Ferguson, J., Jiracek, G., et al. (1996). Environmental waste site characterization utilizing aerial photographs. remote Sens. Surf. Geophys.
Pouyanfar, N., Harofte, S. Z., Soltani, M., Siavashy, S., Asadian, E., Ghorbani-Bidkorbeh, F., et al. (2022). Artificial intelligence-based microfluidic platforms for the sensitive detection of environmental pollutants: recent advances and prospects. Trends Environ. Anal. Chem. 34, e00160. doi:10.1016/j.teac.2022.e00160
Praveenchandar, J., Vetrithangam, D., Kaliappan, S., Karthick, M., Pegada, N. K., Patil, P. P., et al. (2022). IoT-based harmful toxic gases monitoring and fault detection on the sensor dataset using deep learning techniques. Sci. Program. 2022, 1–11. doi:10.1155/2022/7516328
Pule, M., Yahya, A., and Chuma, J. (2017). Wireless sensor networks: a survey on monitoring water quality. J. Appl. Res. Technol. 15 (6), 562–570. doi:10.1016/j.jart.2017.07.004
Rajan, R., Robin, D. T., and Vandanarani, M. (2019). Biomedical waste management in Ayurveda hospitals–current practices and future prospectives. J. Ayurveda Integr. Med. 10 (3), 214–221. doi:10.1016/j.jaim.2017.07.011
Rani, L., Thapa, K., Kanojia, N., Sharma, N., Singh, S., Grewal, A. S., et al. (2021). An extensive review on the consequences of chemical pesticides on human health and environment. J. Clean. Prod. 283, 124657. doi:10.1016/j.jclepro.2020.124657
Ravi, N., and Johnson, D. P. (2021). Artificial intelligence based monitoring system for onsite septic systems failure. Process Saf. Environ. Prot. 148, 1090–1097. doi:10.1016/j.psep.2021.01.049
Restas, A. (2015). Drone applications for supporting disaster management. World J. Eng. Technol. 3 (03), 316–321. doi:10.4236/wjet.2015.33c047
Ristic, B., Angley, D., Moran, B., and Palmer, J. L. (2017). Autonomous multi-robot search for a hazardous source in a turbulent environment. Sensors 17 (4), 918. doi:10.3390/s17040918
Ryu, J. H. (2022). UAS-based real-time water quality monitoring, sampling, and visualization platform (UASWQP). HardwareX 11, e00277. doi:10.1016/j.ohx.2022.e00277
Sahil, K., Mehta, P., Bhardwaj, S. K., and Dhaliwal, L. K. (2023). “Development of mitigation strategies for the climate change using artificial intelligence to attain sustainability,” in Visualization techniques for climate change with machine learning and artificial intelligence (Elsevier), 421–448.
Sanaeifar, A., Zhang, W., Chen, H., Zhang, D., Li, X., and He, Y. (2022). Study on effects of airborne Pb pollution on quality indicators and accumulation in tea plants using Vis-NIR spectroscopy coupled with radial basis function neural network. Ecotoxicol. Environ. Saf. 229, 113056. doi:10.1016/j.ecoenv.2021.113056
Sari, M., Cosgun, T., Yalcin, I. E., Taner, M., and Ozyigit, I. I. (2022). Deciding heavy metal levels in soil based on various ecological information through artificial intelligence modeling. Appl. Artif. Intell. 36 (1), 2014189. doi:10.1080/08839514.2021.2014189
Sarkar, A., and Pandey, P. (2015). River water quality modelling using artificial neural network technique. Aquat. Procedia 4, 1070–1077. doi:10.1016/j.aqpro.2015.02.135
Secinaro, S., Calandra, D., Secinaro, A., Muthurangu, V., and Biancone, P. (2021). The role of artificial intelligence in healthcare: a structured literature review. BMC Med. Inf. Decis. Mak. 21, 125–223. doi:10.1186/s12911-021-01488-9
Seiber, C., Nowlin, D., Landowski, B., and Tolentino, M. E. (2018). “Tracking hazardous aerial plumes using IoT-enabled drone swarms,” in 2018 IEEE 4th World Forum on Internet of Things (WF-IoT).
Seker, S. (2022). IoT based sustainable smart waste management system evaluation using MCDM model under interval-valued q-rung orthopair fuzzy environment. Technol. Soc. 71, 102100. doi:10.1016/j.techsoc.2022.102100
Seo, M., and Lee, S. W. (2022). Methodology to classify hazardous compounds via deep learning based on convolutional neural networks. Curr. Appl. Phys. 41, 59–65. doi:10.1016/j.cap.2022.06.003
Shafique, A., Cao, G., Khan, Z., Asad, M., and Aslam, M. (2022). Deep learning-based change detection in remote sensing images: a review. Remote Sens. 14 (4), 871. doi:10.3390/rs14040871
Sharma, P., Bano, A., Singh, S. P., Sharma, S., Xia, C. L., Nadda, A. K., et al. (2022). Engineered microbes as effective tools for the remediation of polyaromatic aromatic hydrocarbons and heavy metals. Chemosphere 306, 135538. doi:10.1016/j.chemosphere.2022.135538
Sharma, V., Arya, V. M., and Sharma, K. (2017). Spatial variability of soil pH and electrical conductivity in the Jammu district of Jammu and Kashmir. J. Soil Water Conservation 16 (4), 320–324. doi:10.5958/2455-7145.2017.00047.9
Shen, Y. (2018). Data sharing practices, information exchange behaviors, and knowledge discovery dynamics: a study of natural resources and environmental scientists. Environ. Syst. Res. 6 (1), 9–14. doi:10.1186/s40068-017-0086-5
Shi, L., Wang, X., Zhang, T., Hu, C., Luo, K., and Bai, B. (2016). Hazardous gas detection four-rotor UAV system development. 2016 IEEE International Conference on Mechatronics and Automation.
Shi, S., Hou, M., Gu, Z., Jiang, C., Zhang, W., Hou, M., et al. (2022). Estimation of heavy metal content in soil based on machine learning models. Land 11 (7), 1037. doi:10.3390/land11071037
Shirkoohi, M. G., Tyagi, R. D., Vanrolleghem, P. A., and Drogui, P. (2022). Artificial intelligence techniques in electrochemical processes for water and wastewater treatment: a review. J. Environ. Health Sci. Eng. 20 (2), 1089–1109. doi:10.1007/s40201-022-00835-w
Signes-Pastor, A. J., Carey, M., Carbonell-Barrachina, A. A., Moreno-Jiménez, E., Green, A. J., and Meharg, A. A. (2016). Geographical variation in inorganic arsenic in paddy field samples and commercial rice from the Iberian Peninsula. Food Chem. 202, 356–363. doi:10.1016/j.foodchem.2016.01.117
Singh, P., and Kaur, A. (2022). A systematic review of artificial intelligence in agriculture. Deep Learn. Sustain. Agric., 57–80. doi:10.1016/b978-0-323-85214-2.00011-2
Slonecker, E., Lacerte, M., and Garofalo, D. (2002). The value of historic imagery. Earth Obs. Mag. 8, 39–41.
Slonecker, T., Fisher, G. B., Aiello, D. P., and Haack, B. (2010). Visible and infrared remote imaging of hazardous waste: a review. Remote Sens. 2 (11), 2474–2508. doi:10.3390/rs2112474
Sonne, C., Jenssen, B. M., Rinklebe, J., Lam, S. S., Hansen, M., Bossi, R., et al. (2023). EU need to protect its environment from toxic per-and polyfluoroalkyl substances. Sci. Total Environ. 876, 162770. doi:10.1016/j.scitotenv.2023.162770
Talebkeikhah, F., Rasam, S., Talebkeikhah, M., Torkashvand, M., Salimi, A., and Moraveji, M. K. (2022). Investigation of effective processes parameters on lead (II) adsorption from wastewater by biochar in mild air oxidation pyrolysis process. Int. J. Environ. Anal. Chem. 102 (16), 3975–3995. doi:10.1080/03067319.2020.1777291
Tan, K., Ma, W., Chen, L., Wang, H., Du, Q., Du, P., et al. (2021). Estimating the distribution trend of soil heavy metals in mining area from HyMap airborne hyperspectral imagery based on ensemble learning. J. Hazard. Mater. 401, 123288. doi:10.1016/j.jhazmat.2020.123288
Tawabini, B., Yassin, M. A., Benaafi, M., Adetoro, J. A., Al-Shaibani, A., and Abba, S. (2022). Spatiotemporal variability assessment of trace metals based on subsurface water quality impact integrated with artificial intelligence-based modeling. Sustainability 14 (4), 2192. doi:10.3390/su14042192
Thomas, G. W., Sousan, S., Tatum, M., Liu, X., Zuidema, C., Fitzpatrick, M., et al. (2018). Low-cost, distributed environmental monitors for factory worker health. Sensors 18 (5), 1411. doi:10.3390/s18051411
Trasande, L., Massey, R. I., DiGangi, J., Geiser, K., Olanipekun, A. I., and Gallagher, L. (2011). How developing nations can protect children from hazardous chemical exposures while sustaining economic growth. Health Aff. 30 (12), 2400–2409. doi:10.1377/hlthaff.2010.1217
Tsitsimpelis, I., Taylor, C. J., Lennox, B., and Joyce, M. J. (2019). A review of ground-based robotic systems for the characterization of nuclear environments. Prog. Nucl. energy 111, 109–124. doi:10.1016/j.pnucene.2018.10.023
Vasudevan, S. K., and Baskaran, B. (2021). An improved real-time water quality monitoring embedded system with IoT on unmanned surface vehicle. Ecol. Inf. 65, 101421. doi:10.1016/j.ecoinf.2021.101421
Vijayakumar, N., and Ramya, (2015). “The real time monitoring of water quality in IoT environment,” in 2015 International Conference on Innovations in Information, Embedded and Communication Systems (ICIIECS).
Vincent, T. A., Xing, Y., Cole, M., and Gardner, J. W. (2019). Investigation of the response of high-bandwidth MOX sensors to gas plumes for application on a mobile robot in hazardous environments. Sensors Actuators B Chem. 279, 351–360. doi:10.1016/j.snb.2018.08.125
Visvanathan, R., Kamarudin, K., Mamduh, S., Yeon, A., Zakaria, A., Kamarudin, L., et al. (2018). Gas sensing mobile robot: a review. J. Telecommun. Electron. Comput. Eng. (JTEC) 10 (1-15), 101–105.
Von Meding, J., Oyedele, L., and Cleland, D. (2009). Developing NGO competencies in post-disaster reconstruction: a theoretical framework. Disaster Adv. 2 (3), 36–45.
Wandel, M., Lilienthal, A. J., Duckett, T., Weimar, U., and Zell, A. (2003). “Gas distribution in unventilated indoor environments inspected by a mobile robot,” in IEEE international conference on advanced robotics, ICAR 2003, Coimbra, Portugal, June 30-July 3, 2003.
Wang, B., Yun, M., Liu, Q., and Wang, Y. (2023a). Precision safety management (PSM): a novel and promising approach to safety management in the precision era. Saf. Sci. 157, 105931. doi:10.1016/j.ssci.2022.105931
Wang, C. J., Ng, C. Y., and Brook, R. H. (2020). Response to COVID-19 in Taiwan: big data analytics, new technology, and proactive testing. Jama 323 (14), 1341–1342. doi:10.1001/jama.2020.3151
Wang, J., Huang, Y., Zhai, W., Li, J., Ouyang, S., Gao, H., et al. (2023b). Research on coal mine safety management based on digital twin. Heliyon 9 (3), e13608. doi:10.1016/j.heliyon.2023.e13608
Wani, O. A., Akhter, F., Kumar, S. S., Babu, S., Kanth, R. H., Mir, S. A., et al. (2023). Mitigating soil erosion through biomass-derived biochar: exploring the influence of feedstock types and pyrolysis temperature. Land 12 (12), 2111. doi:10.3390/land12122111
Whitelaw, S., Mamas, M. A., Topol, E., and Van Spall, H. G. (2020). Applications of digital technology in COVID-19 pandemic planning and response. Lancet Digital Health 2 (8), e435–e440. doi:10.1016/s2589-7500(20)30142-4
Wilson, A. D. (2012). Review of electronic-nose technologies and algorithms to detect hazardous chemicals in the environment. Procedia Technol. 1, 453–463. doi:10.1016/j.protcy.2012.02.101
Wong, C., Yang, E., Yan, X.-T., and Gu, D. (2018). Autonomous robots for harsh environments: a holistic overview of current solutions and ongoing challenges. Syst. Sci. Control Eng. 6 (1), 213–219. doi:10.1080/21642583.2018.1477634
Wu, Y., Zhang, X., Liao, Q., and Ji, J. (2011). Can contaminant elements in soils be assessed by remote sensing technology: a case study with simulated data. Soil Sci. 176 (4), 196–205. doi:10.1097/ss.0b013e3182114717
Yan, H., Wan, J., Zhang, C., Tang, S., Hua, Q., and Wang, Z. (2018). Industrial big data analytics for prediction of remaining useful life based on deep learning. IEEE access 6, 17190–17197. doi:10.1109/access.2018.2809681
Yang, C.-T., Chen, H.-W., Chang, E.-J., Kristiani, E., Nguyen, K. L. P., and Chang, J.-S. (2021). Current advances and future challenges of AIoT applications in particulate matters (PM) monitoring and control. J. Hazard. Mater. 419, 126442. doi:10.1016/j.jhazmat.2021.126442
Yang, H., Yang, X., Ning, Z., Kwon, S. Y., Li, M.-L., Tack, F. M., et al. (2022). The beneficial and hazardous effects of selenium on the health of the soil-plant-human system: an overview. J. Hazard. Mater. 422, 126876. doi:10.1016/j.jhazmat.2021.126876
Yetilmezsoy, K., and Demirel, S. (2008). Artificial neural network (ANN) approach for modeling of Pb (II) adsorption from aqueous solution by Antep pistachio (Pistacia Vera L.) shells. J. Hazard. Mater. 153 (3), 1288–1300. doi:10.1016/j.jhazmat.2007.09.092
Young, S., Balluz, L., and Malilay, J. (2004). Natural and technologic hazardous material releases during and after natural disasters: a review. Sci. Total Environ. 322 (1-3), 3–20. doi:10.1016/s0048-9697(03)00446-7
Yusuf, A., Amusa, H. K., Eniola, J. O., Giwa, A., Pikuda, O., Dindi, A., et al. (2023). Hazardous and emerging contaminants removal from water by plasma-based treatment: a review of recent advances. Chem. Eng. J. Adv. 14, 100443. doi:10.1016/j.ceja.2023.100443
Zafar, M., Van Vinh, N., Behera, S. K., and Park, H.-S. (2017). Ethanol mediated as (III) adsorption onto Zn-loaded pinecone biochar: experimental investigation, modeling, and optimization using hybrid artificial neural network-genetic algorithm approach. J. Environ. Sci. 54, 114–125. doi:10.1016/j.jes.2016.06.008
Zahed, Z., Mufti, S., Kumar, S. S., Wani, O. A., Mushtaq, F., Rasool, R., et al. (2022). Organic and inorganic mulches combination improves the productivity, quality and profitability of rainfed potato in the temperate himalayan region. Gesunde Pflanz. 74 (4), 1109–1122. doi:10.1007/s10343-022-00650-1
Zeba, G., Dabić, M., Čičak, M., Daim, T., and Yalcin, H. (2021). Technology mining: artificial intelligence in manufacturing. Technol. Forecast. Soc. Change 171, 120971. doi:10.1016/j.techfore.2021.120971
Zhai, A., Hou, B., Ding, X., and Huang, G. (2021). Hazardous chemical accident prediction for drinking water sources in Three Gorges Reservoir. J. Clean. Prod. 296, 126529. doi:10.1016/j.jclepro.2021.126529
Zhang, Y. (2019). Research on key technologies of remote design of mechanical products based on artificial intelligence. J. Vis. Commun. Image Represent. 60, 250–257. doi:10.1016/j.jvcir.2019.02.010
Zhao, D., Wang, J., Jiang, X., Zhen, J., Miao, J., Wang, J., et al. (2022). Reflectance spectroscopy for assessing heavy metal pollution indices in mangrove sediments using XGBoost method and physicochemical properties. Catena 211, 105967. doi:10.1016/j.catena.2021.105967
Zhao, P., Gao, Y., and Sun, X. (2023). The impact of artificial intelligence on pollution emission intensity—evidence from China. Environ. Sci. Pollut. Res. 30 (39), 91173–91188. doi:10.1007/s11356-023-28866-2
Zhou, H., Yu, X., Alhaskawi, A., Dong, Y., Wang, Z., Jin, Q., et al. (2022). A deep learning approach for medical waste classification. Sci. Rep. 12 (1), 2159. doi:10.1038/s41598-022-06146-2
Zhu, X., Wang, X., and Ok, Y. S. (2019). The application of machine learning methods for prediction of metal sorption onto biochars. J. Hazard. Mater. 378, 120727. doi:10.1016/j.jhazmat.2019.06.004
Zinnuraain, S., Hasan, M., Hakque, M. A., and Arefin, M. M. N. (2019). “Smart gas leakage detection with monitoring and automatic safety system,” in 2019 International Conference on Wireless Communications Signal Processing and Networking (WiSPNET).
Glossary
Keywords: environmental pollution, monitoring, safety, advance technologies, machine learning, IoT
Citation: Popescu SM, Mansoor S, Wani OA, Kumar SS, Sharma V, Sharma A, Arya VM, Kirkham MB, Hou D, Bolan N and Chung YS (2024) Artificial intelligence and IoT driven technologies for environmental pollution monitoring and management. Front. Environ. Sci. 12:1336088. doi: 10.3389/fenvs.2024.1336088
Received: 10 November 2023; Accepted: 06 February 2024;
Published: 20 February 2024.
Edited by:
Chengye Zhang, China University of Mining and Technology, ChinaReviewed by:
Shashi Kant Gupta, Eudoxia Research University, United StatesIoannis G. Tsoulos, University of Ioannina, Greece
Wu Ling, China University of Geosciences, China
Jun Yue, Central South University, China
Copyright © 2024 Popescu, Mansoor, Wani, Kumar, Sharma, Sharma, Arya, Kirkham, Hou, Bolan and Chung. This is an open-access article distributed under the terms of the Creative Commons Attribution License (CC BY). The use, distribution or reproduction in other forums is permitted, provided the original author(s) and the copyright owner(s) are credited and that the original publication in this journal is cited, in accordance with accepted academic practice. No use, distribution or reproduction is permitted which does not comply with these terms.
*Correspondence: Yong Suk Chung, eXNjaHVuZ0BqZWp1bnUuYWMua3I=; Sheikh Mansoor, bWFuc29vcnNoYWZpMjFAZ21haWwuY29t