- School of Economics, Shandong University of Technology, Zibo, China
China's forestry industry's ecological, economic, and social benefits have improved significantly. However, practical problems remain, such as irrational industrial structure, serious resource consumption, and low environmental transformation efficiency. The green total factor productivity is an important index to measure the transformation of economic development mode, and improving the green total factor productivity in forestry is of great significance in guiding the transformation of forestry economy, improving the structure of agricultural economy, and realizing the green development of forestry economy. Based on the panel data of 30 provinces and cities in China from 2006 to 2021, this article adopts the entropy value method and the Global Malmquist–Luenberger index based on the SBM directional distance function to construct the index system of green finance and forestry green total factor productivity and empirically analyzes the impact and mechanism of green finance on forestry green total factor productivity. It was found that 1) green finance can improve local forestry green total factor productivity and influence the neighboring regions to improve forestry green total factor productivity through economic spillover effect, and the coefficient of spatial spillover effect was 0.1041. 2) There were regional differences in the impact of green finance on forestry green total factor productivity, which was explicitly presented as a gradual decrease from east to west “ladder-like”. 3) There was a 30.46% intermediary effect of forestry industry structure in the influence of green finance on forestry green total factor productivity. 4) There was a double-threshold effect of human capital in the influence of green finance on forestry green total factor productivity, and there was a non-linear effect of marginal increment in the influence of green finance on forestry green total factor productivity after the threshold of 5.87 human capital. Based on China's ecological and economic development background, it was significant to utilize green finance to solve forestry economic development problems and promote China's green and sustainable development, and therefore put forward policy recommendations.
1 Introduction
Forestry production is affected by natural factors and the growth cycle of tree species. There are problems such as the long cultivation cycle of forest species, the single structure of the forestry industry (Wang Yahui et al., 2022a), and obstacles to the development of scale and intensification of economic forest industry (Chen et al., 2023a). Farmers' part-time employment leads to the shortage of forestry labor; the externality of forestry itself leads to the limitation of forestry scale development; and the contracting of forest land is primarily a fine-grained operation, which makes it challenging to realize the scale effect. Problems such as the difficulty in handling forestry collateral and managing mortgage rights, the high threshold of forestry financing, and the unreliable foundation of forestry carbon sinks (Geng et al., 2023) are also important reasons for insufficient forestry capital stock and inefficient funding. Currently, China's natural resources are in severe shortage, and the fundamental problems of forest land fragmentation, the long production cycle of tree species, and lack of financial support are slowing down the economic development of forestry. Forestry, as an essential production sector that protects the balance of the ecological environment and cultivates and protects forest resources, is an integral part of China's modern economic and industrial development, and the promotion of forestry development is an inevitable requirement for realizing green development. In 2022, General Secretary Xi Jinping explicitly proposed to give full play to the fundamental and strategic position and role of forests in national ecological security and the sustainable economic and social development of people, which has provided an opportunity for forestry development to become more effective and efficient. Status and role point out a new direction for forestry development and promote forestry as a powerful support for China's development. Along with China's modernization and development stage, the green transformation of forestry development has become the trend of the times, and how to realize the synergistic development of forestry economic growth and ecological civilization construction has become the theme of the new era.
As an essential tool for regulating the financial market and ecological environment, green finance has become the glue for economic development and environmental protection (Oanh, 2023). Green finance aims to utilize green financial tools to solve the economic and ecological problems faced in forestry development, improve the forestry carbon model (Qin et al., 2023), continue to promote the innovation of forestry financial products (Zhang, 2023), improve the ecological environment of forestry, form a benign cycle of environmental protection and green development, and promote the green and sustainable development of forestry economy. Internationally, green finance and green trading tools aim at promoting environmental protection and sustainable development (Smol, 2022), but green finance involves a broader scope, uses more diversified tools, provides more comprehensive services, can provide sufficient financial support and risk protection for green development, can effectively couple with the development of the circular economy, and is conducive to realizing the developing countries' zero-carbon goal of developing countries (Kumar, 2023).
From the existing literature research, Chinese studies on green total factor productivity in forestry mainly focus on indicator measurement, evolution law, and influencing factors (Lv et al., 2022; Tan, 2022). Some foreign scholars have also studied the influence of law on forestry green total factor productivity from the aspects of digital economy, green urbanization (Wang et al., 2022b), and Internet technology (Wu et al., 2020). However, few scholars have studied the influence of the law of green finance on forestry economic development based on the financial perspective. Research on green finance, on one hand, has been that green finance can promote environmental protection and resource utilization and improve the ecological environment. Gao et al. (2021) and Lei et al. (2020) believed that green finance can significantly inhibit the development of polluting industries, promote the transformation and upgrading of China's industrial structure, promote the ecological development of industries, and drive high-quality development of the economy. On the other hand, green finance can improve financial services and regulatory tools and reduce economic risks. Zhouxiao et al. (2023) verified that based on the enterprise dynamic model, the institutional design of green finance affects enterprise innovation decision-making, promotes green technological innovation, and then improves the level of development. Yao et al. (2023) believed that implementing and promoting green financial policies will, to a certain extent, stimulate green consumption and then drive the development of the regional economy.
Green finance, as the bloodline of the national economy, injects living water into green development and becomes an indispensable driving force for economic development. Rodriguez (2017) argued that financial agglomeration can increase total factor productivity, while Ye et al. (2018) argued that green finance subsidies reduce the cost of industrial development, and Yagura (2020) suggested that financial services are a critical factor in the transformation of the agricultural industry. Ma Jun et al. (2021) indicated that the synergistic development of green finance and inclusive finance can promote the green development of agriculture. Kumar et al. (2022) demonstrated the vital role of green finance in promoting sustainable development through textile and leather SMEs in Pakistan. Shuhua et al. (2022) suggested that finance can influence technological innovation in forestry. However, some scholars believe that the influence of green bonds and green loans can cause the emergence of “green bleaching” and “green dyeing” behaviors (Marquis et al., 2016; Berrone et al., 2017) and that the influence of environmental regulations can produce a double-threshold effect (Ma Guoqun et al., 2021), hindering the high-quality development of forestry economy. The current development of green finance is a critical factor in realizing the green development of the forestry economy. Still, it is also necessary to reasonably utilize green financial tools to achieve low-carbon economic development while solving the problem of environmental pollution. Yin et al. (2021a) found that the impact of green finance on green total factor productivity shows a U-shaped relationship, while Xie et al. (2023) proposed that green finance has a spatial spillover effect on green total factor productivity in Chinese cities, and Zhou et al. (2022) proposed a mechanism that affects the growth of green total factor productivity in the forestry industry from the perspective of agricultural insurance development. Many scholars have studied the relationship between green finance and total factor productivity from different perspectives. Still, there is a lack of academic research on the relationship between green finance and green total factor productivity in forestry.
The sustainable development of the forestry industry is the key to promoting the sustainable development of the forestry economy. The rational allocation of the forestry industry can promote the transformation and upgrading of the industrial structure, increase the value of the forest industry chain, and promote the integrated development of the forestry industry (Chen et al., 2023b). Yan et al. (2022) found that the forestry industry can effectively promote the implementation of China's rural revitalization strategy and the coordinated development of regional agriculture. Ning Youliang et al. (2021), based on the traditional forestry industry development model, proposed promoting the high-quality development of the forestry industry, promoting the integration of basic plantation forestry, forest production industries and forestry services, and extending the industrial chain. Wang et al. (2022a) proposed that specialised clustering in the forestry industry can promote green total factor productivity in forestry; the existing literature for the forestry industry to carry out part of the study, but also some scholars for the relationship between the financial and forestry industry to carry out research. Zhao et al. (2022) argued that rural informal finance can promote the development of the forestry industry. Still, but the structure of the forestry industry has a negative impact on the development of the forestry industry. Lu et al. (2022) believed that green credit and technological innovation can promote the development of the forestry industry structure and that technological innovation and green credit are threshold variables for each other. Combined with the existing literature, there is no relevant research on green finance, forestry industry structure, and green total factor productivity in forestry.
Forestry is mainly a labor-intensive industry relying on natural capital (Smith et al., 2021), with a single industrial structure limited by geographic and biological factors, a cyclical growth pattern, and a significant reliance on artificial seeding, pest control, and irrigation. Human capital plays an irreplaceable and vital role in forestry production. It is found that improving human capital can effectively shorten forest species' growth cycle and strengthen the forestry labor force's structure by influencing laborers' willingness to return to their hometowns (Autor et al., 2001). Jin et al. (2023) argued that human capital can increase the desire of farmers to participate in constructing economic forests, improve endogenous motivation, and promote forestry development. Yang et al. (2019) proposed that human capital can increase green total factor productivity in forestry. Cai et al. (2022) proved that human capital has spatial spillover effects on green total factor productivity. However, few scholars believe that the impact of human capital on forestry green development is not apparent (Thomas et al., 2001), and Li et al. (2023) and Jiang et al. (2023a) discussed that human capital inhibits the improvement of green total factor productivity in forestry from the perspectives of regional development imbalance and population aging, respectively. Therefore, the effect of human capital on forestry economic development requires further in-depth research.
To summarize, concerning scholars' research on green finance and forestry economy, the research focus of this paper is as follows: first, the impact of green finance on green total factor productivity in forestry is discussed from the theoretical perspective. Second, based on the spatial perspective, we studied the spatial effect of green finance on green total factor productivity in forestry. Third, based on the forestry industry structure and human capital effect, the role path of green finance affecting forestry green total factor productivity is further studied. Fourth, the above analysis and policy recommendations on green finance and forestry development for developing countries such as China are proposed.
The marginal academic contributions of this paper are as follows: first, there is no qualitative research and quantitative analysis of the impact relationship between green finance and forestry green total factor productivity in the current academic world, and this paper enriches the research content to a certain extent. Second, this paper is based on the spatial perspective to study the relationship between the impact of green finance on forestry green total factor productivity, to test the regional development effect of green finance affecting forestry green total factor productivity, to analyze the spatial spillover effect of green finance affecting forestry green total factor productivity, and to enrich the scope of research. Third, we analyze the critical role of forestry industry structure and human capital in forestry development, reveal the role path of green finance's influence on forestry green total factor productivity, and improve the influence path.
2 Research context
2.1 Theoretical analysis framework
2.1.1 Direct effect of green finance on green total factor productivity in forestry
Green finance can lower the threshold of financial access, improve the rationing of green resources, improve the information disclosure environment, alleviate information asymmetry, reduce transaction costs, and satisfy the financial needs required for the growth of long-cycle forest species. First, green finance carries out financial activities for environmental protection, and its funds are mainly used to carry out green projects. For forest areas with different tree species, tree ages, and growth patterns, it formulates targeted investment and financing tools to meet the financial needs required for the growth of forest species in different cycles. Green finance can meet the financial needs of environmentally poor areas, build a forest ecosystem that prevents wind and fixes sand, optimize the environment, and drive the development of the forestry economy. The “Three-North” shelterbelt construction project supported by the Agricultural Development Bank of China is a vivid demonstration of support for the green development of forestry. Second, green finance can directly improve the output elasticity of tree species, forest species, forest age, and other factors (Yue et al., 2020); ensure the stability of production factor inputs; shorten the forestry production cycle; improve the efficiency of forestry production; and directly improve the green total factor productivity of forestry. Green finance based on environmental benefits can provide sufficient production security for economic forests, thereby increasing the ecological value. Sanming city, Fujian province, China, using the “Fu Lin loan,” to develop collective forestry to inject financial water, opens up the channels of financing forest rights and promotes the capitalization process of forestry resources. Third, green finance relies on digital technology to build an information service network, solve the problem of information disparity in smallholder and fragmented forestry farming, reduce information costs, optimize resource allocation according to demand, promote large-scale production by foresters, increase the speed of integration of different industries in forestry, and provide elemental conditions and mechanism guarantee for the organic convergence of foresters and modern forestry. Zhangzhou city, Fujian province, China, relies on the Industrial Bank to build a forestry digital platform, issue forest tickets and forestry land tickets, and provide other tools to establish a forestry financial ecology of the whole industry chain and the whole scene to promote the impact of digital financial development on forestry and then improve the capital market, optimize the new form of the forestry industry, improve the efficiency of resource allocation and operation, promote the development of the forestry economy, and improve the green total factor productivity of forestry. Accordingly, hypothesis H1 is proposed.
H1:. Green finance can improve the level of green total factor productivity in forestry.
2.1.2 Indirect effect of green finance on green total factor productivity in forestry
Green finance can macroscopically regulate financial resources and production factors, invest large amounts of capital and resources in forestry production, break the limitations of geographic location, information interaction, and time costs (An and Chen, 2020), and break through the spatial and temporal barriers of forestry production, alleviate the financial pressure of long-cycle production, and shorten the growth cycle of the original species of forests by superimposing resources and technological breakthroughs and improving the technology of forestry breeding, which will promote the improvement of green total factor productivity in forestry. Green finance can macroscopically regulate the reasonable flow of financial resources, realize cross-regional resource allocation, provide support for the economic development of forestry in the surrounding areas, and then drive the enthusiasm of forest farmers to promote forestry development. Green finance can innovate forestry cultivation tools, cultivate forestry technicians, and improve forest protection and forest species cultivation technology, which is conducive to the formation of an open and complementary learning environment, thus driving the progression of forestry technology in the surrounding areas, promoting regional technology exchanges, and improving the green total factor productivity of forestry in the surrounding areas. Faster development of green finance in the regions promotes the scale of forestry production and makes it easier to attract imitation learning in the surrounding areas, learn from the breeding experience, expand the scale of forestry production, reduce the risk of forestry production caused by natural disasters, improve production efficiency, and thus promote the green total factor productivity of forestry. Accordingly, hypothesis H2 is proposed.
H2:. There is a spatial spillover effect of the impact of green finance on the green total factor productivity of forestry in the surrounding areas.
2.1.3 The mediating effect of green finance on green total factor productivity in forestry
Industrial structure is an essential foundation for sustainable economic development and is mainly limited by factors such as factor input and management level (Jiang et al., 2023b), and forestry industrial structure is more dependent on capital input and resource allocation. Forestry development requires a large amount of capital investment to optimize the forestry production structure, promote the different industries in forestry, and realize the upgrading of the forestry industry structure. Numerous scholars have found that industrial agglomeration affects the level of regional total factor productivity (Ottaviano et al., 2006; Lu et al., 2017), and Xiaojie et al. (2019) found that forestry industry agglomeration can enhance the level of green total factor productivity in forestry. Green finance can solve the problem of insufficient funds and capital shortage for the development of the forestry industry (Xiao, 2018), broaden forestry financing channels, build a green financing platform, ensure the development of the forestry industry while providing the forestry industry with the opportunity of green transformation, and promote the green development of forestry. Accompanied by the continuous development of green finance, traditional production will inevitably be replaced by green production, deriving a variety of mixed industrial structures and new industrial chains, guiding the green development of the forestry industry, leading to structural change of the industry, and then injecting new momentum into forestry development. Accordingly, hypothesis H3 is proposed.
H3:. Forestry industry structure plays an intermediary role in the process of green finance affecting forestry green total factor productivity.
A previous theoretical study found that green finance can enhance the green total factor productivity of forestry, but this did not take into account the situation of the differences in resource endowment between regions in China and also ignored the differences in the development of human capital in rural areas as the main body of the supply and demand of green financial products and services (Li et al., 2023). The endogenous economic growth theory considers human capital as the primary source of economic growth in forestry (Xiuhua et al., 2019), and the development of green finance in rural areas cannot be separated from the promotion of human capital. However, the reality in China is that there are apparent differences in the level of human capital between different regions, which will also lead to differences in the effect of the role of green finance on the green total factor productivity of forestry. Human capital, as a necessary labor input for forestry production, plays a role in all aspects of forestry production, providing labor support for forestry development. As an essential driving force for green finance development in rural areas, human capital can innovate financial instruments and guide the allocation and application of financial resources (Sun et al., 2018). A high level of human capital will accelerate the spread of green finance in agriculture, improve the level of financial services, and build a more stable technology system, production system, and renewal system (Zhang et al., 2020), which is conducive to shortening the forestry production cycle, promoting the transformation and upgrading of the forestry economic structure, and improving the level of economic development. When farmers' understanding and acceptance of green finance knowledge increases, it will not only directly improve the level of green financial development but also improve the influence of green finance through the phenomenon of people-to-people. Accordingly, hypothesis H4 is proposed.
H4:. There is a threshold effect of human capital in the process of green finance affecting green total factor productivity in forestry.
2.2 Research methodology
2.2.1 GML index construction for SBM-DDF modeling
The traditional data envelopment analysis model is prone to bias when expanding outputs or reducing inputs year over year. However, the non-radial, non-angular directional distance function (SBM-DDF model) based on relaxation value measurement can effectively solve the variable relaxation problem and ensure the accuracy of experimental results. The traditional Malmquist index is based on the premise that the scale reward is unchanged to develop the measurement. However, the Global Malmquist–Luenberger (GML) has the advantages of multiplicability and transferability, which can make up for the fact that the traditional method cannot reflect the temporal trend of the total factor productivity of forestry and reflect the dynamic time–order change of forestry production. Therefore, this article refers to Shaopeng (2022) to select the GML index of the SBM-DDF model to measure the dynamic change of green total factor productivity in forestry, to make up for the short board of the static model, and reflect the characteristics of the time–order change. The formula is as follows:
In Equation 1, (
2.2.2 Modeling: the impact of green finance on green total factor productivity in forestry
(1) Spatial autocorrelation test. Construct Moran's I to test the spatial correlation between green finance and green total factor productivity in forestry. The formula of Moran's index is
In Equation 2,
(2) Spatial weight matrix design. The economic weight matrix is used to accurately measure the characteristics of the economic, spatial distribution between green finance and forestry green total factor productivity to ensure the robustness of the results in the empirical testing process.
In formula (3),
(3) Spatial Durbin model. To study the spatial relationship between green finance and green total factor productivity in forestry, this paper refers to Fan et al. (2021) for an empirical study using the spatial Durbin model.
In Eq. 4, the essence of the spatial Durbin model is to simultaneously introduce the explained variables and explanatory variables into the model with the spatial matrix, respectively.
2.2.3 Mediating effect test: mediating effect of the forestry industry structure
(4) Mediation model. This paper adopts the Bootstrap method, which is more prevalent at this stage, to carry out the mediation effect test. The mediation effect model can illustrate the mechanism by which the explanatory variables affect the explained variables through the mediating variables. The basic idea is first to examine the impact of green finance on mechanism variables and then test the impact of mechanism variables on agricultural green total factor productivity. According to the above explanation, this article takes the forestry industry structure as a mediating variable to test the corresponding mediating effect. Referring to Wen et al.'s (2014) research, we analyze the process and mechanism of influencing forestry green total factor productivity between green finance and forestry industry structure.
In equations (5) to (7), GTFPF is the explained variable; GF is the explanatory variable; FIS is the mediator variable; Control is the control variable; and i and t, respectively, stand for each province/city and year; and ε represents the error term.
2.2.4 Threshold effect test: the threshold effect of human capital
(5) Threshold model. There may be regional differentiation in the development of green finance in Chinese cities and municipalities, and there may be non-linear effects of green finance on green total factor productivity in forestry at different stages of human capital development. Therefore, in order to verify whether there is a threshold value, this article further constructs a threshold effect model to test the threshold effect of human capital and constructs the following model:
In Eq. 8, GTFPF is the explained variable and GF is the explanatory variable; fin is the threshold variable; μ is the threshold value that is to be estimated; I() represents the indicator function, which takes the value of 1 when the conditions in the indicator function are met and of 0 if otherwise, and is the random disturbance term.
3 Variable selection and data sources
3.1 Selection of variables
This article takes the green financial index and the green total factor productivity of forestry index as the main variables to ensure the accuracy of the experiment, exclude the influence of other factors, and increase the control variables to ensure the stability of the empirical results.
(1) Explained variable: The green total factor productivity of forestry (GTFPF) index refers to the efficiency of forestry production in the case of constant input factors to obtain the maximum output while reducing production pollution. Based on the fact that forestry inputs depend on the characteristics of tree species and forest age, while considering the environmental and economic benefits of forestry outputs, this article selects indicators as follows: Input indicators—capital inputs: the amount of investment in forestry fixed assets in various regions is selected. Referring to the study of Song et al. (2012), the perpetual inventory method is used to calculate the capital stock in the base period by dividing the amount of investment in forestry fixed assets in 2005 by 10%; labor input: the number of employees in forestry is selected; land input: the area of land used for forestry is selected; energy input: the energy consumption of forestry = (the total output value of the forestry industry in each province/gross regional product) × regional energy consumption; mechanical power input: selected forestry machinery power input, i.e., total forestry machinery power = (total forestry output value/gross value of agriculture in each province) × total agricultural machinery power. Output indicators—desired output: total forestry output value for economic benefits and afforestation area for ecological benefits; non-desired output: carbon dioxide emissions from forestry. The green total factor productivity indicator system of forestry is given in Table 1.
(2) Explanatory variables: The green finance (GF) index refers to investment and financing activities that can generate environmental benefits to support sustainable development. This paper combines the existing literature and finds that most scholars’ measurement of the level of green finance development is limited to substituting six high-energy–consuming industry indexes, which cannot represent the development of green finance well. Therefore, this paper refers to Ma (2015) when selecting green financial development indicators and to Yin et al. (2021b) studies and chooses the entropy value method that can scientifically and reasonably reflect the degree of indicators. The index system of green financial development is mainly constructed from five dimensions: green credit, green securities, green investment, green insurance, and carbon finance. The corresponding specific indicators are selected, as given in Table 2.
(3) Mediating variable—forestry industry structure (FIS): Forestry industry structure is in the position of wind vane in economic development, and its transformation is an integral part of forestry and forestry economic development. Optimization of the forestry industry structure can broaden the forestry industry chain, extend a variety of economic systems, innovate forestry production technology, increase forestry production links, reduce cyclical risks due to forest species problems, improve the income of forest households, drive forestry employment and forestry economic development, and determine the direction of forestry development. Forestry development direction: Green finance can not only use the capital effect to change the structure of the forestry industry but also promote the self-regulation of the forestry industry structure and then promote the development of the forestry economy. Therefore, this paper selects the proportion of the output value of the primary forestry industry in the total forestry output value to measure.
(4) Threshold variable—human capital (HG): Human resources are essential for developing green finance in rural areas. In construction, human capital can provide sufficient labor for rural areas, and the senior technical personnel can innovate forestry production technology and improve forestry production efficiency, whose relationship with green total factor productivity in forestry is self-evident. Human capital is a prerequisite for developing green finance; once there is no adequate talent supply, the development of green finance can only stagnate. Therefore, this article selects human capital as the threshold variable and adopts the average years of education of the rural population to measure the level of regional human capital development as regards Deng et al. (2023), who used the education method to calculate it, i.e., multiplying the proportion of rural residents in different levels of education by the corresponding number of years of education.
(5) Control variables: To exclude the influence of other factors and ensure the accuracy of the experiments, the forestry resource endowment (FRE) was selected to measure the area of forest cover. Government support for agriculture (GSA) was selected to measure the proportion of government financial expenditure on agriculture and forestry. Forestry policy reform (FPR) was selected to measure the structural change of forestry industry policy. This article refers to the research of Tang et al. (2017), taking 2006 as the boundary; according to the institutional reform process in recent years, the reform of the forest rights system is quantified, and the value of the forestry system reform variable is set as 0 in 2006, 1 in 2007–2011, and 2 in 2012–2021. The level of economic development (LED) is selected as the ratio of rural forestry expenditures in to the government fiscal expenditure. Economic development (LED) is measured by the rural per capita gross domestic product (GDP). The urbanization level (UL) is measured by the proportion of the urban population in the total population.
3.2 Data sources
In this article, the relevant data from 30 provinces in China (Tibet, Hong Kong, Macao, and Taiwan are not counted due to missing data) from 2006 to 2021 are selected for descriptive statistical analysis. The data on green finance indicators come from authoritative organizations such as the China Bureau of Statistics, the People's Bank of China, and provincial and municipal statistical yearbooks, such as the China Statistical Yearbook, the China Insurance Yearbook, the China Science and Technology Statistical Yearbook, and the China Energy Statistical Yearbook; the data on green total factor productivity indicators of forestry come from Statistical Yearbook of China Forestry, Statistical Yearbook of China Environment; the rest of the data come from the above statistical yearbooks, and the data sources are accurate and authoritative. The descriptive statistics of the variables are given in Table 3, which shows that there is a significant difference between the maximum value of 1.8570 and the minimum value of 0.25709 of China's forestry green total factor productivity index, indicating that there is a significant gap in the development level of China's forestry green total factor productivity in all provinces and cities and that there are pronounced regional differences in the development of the forestry industry and the forestry economy.
4 Results
4.1 Spatial autocorrelation test
It is necessary to test whether the green total factor productivity of green finance and forestry has a spatial correlation before the model analysis, and this article utilizes formula (1) to test Moran's I of green total factor productivity of green finance and forestry based on the economic distance matrix. According to the spatial autocorrelation test results given in Table 4, it can be seen that under the economic weight matrix, except for a few years, the Moran's index of green financial and forestry green total factor productivity in 2006–2021 passes the 10% significance level test, which indicates that there is significant spatial autocorrelation in both green financial and forestry green total factor productivity with the distribution characteristics of spatial agglomeration.
4.2 Spatial panel model selection
Moran's test was conducted for the initial test, followed by the logistic regression test (LM test), as given in Table 5. The LM test coefficients all passed the test at the 10% significance level, proving that the spatial Durbin model can be used. The Hausman test was performed, and the coefficients were positive; the test was at the 5% significance level, proving that fixed effects could be used. Finally, the likelihood ratio test (LR test) and Wald test were conducted, and the coefficients passed the test at the 1% significance level, proving that the spatial Durbin model can be degraded to the spatial lag model and the spatial error model.
4.3 Spatial Durbin model regression analysis
Based on the spatial economic distance matrix, this paper utilizes the spatial Durbin model to study the spatial impact effect of green finance on green total factor productivity in forestry, and the results are given in Table 6. In order to test the results more robustly, the results of the spatial lag model and the spatial error model test are listed at the same time.
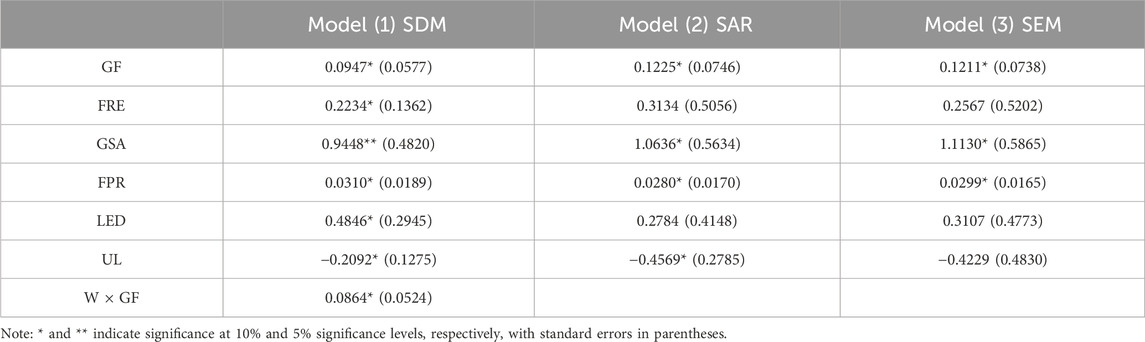
TABLE 6. Spatial regression results of green finance on green total factor productivity in forestry.
The results given in Table 6 show that the coefficients of green finance under different spatial econometric models are all positive and pass the 10% significance level test. This indicates that the development of green finance can improve the local forestry level of green total factor productivity of local forestry, according to which hypothesis H1 can be proved. At the same time, the spatial lag term W × GF of green finance is positive at a 10% significance level, which indicates that the improvement of the local green finance development level can lead to the enhancement of the green total factor productivity level of forestry in the neighboring areas, according to which hypothesis H2 can be proved to be true. Green finance can directly solve financial problems such as complex forestry financing and slow payback, alleviate the national financial pressure, provide financial security for forestry economic development, solve the problem of cash flow and imbalance between supply and demand, provide financial services for small- and medium-sized forestry enterprises, and promote the forestry economic cycle. Green finance can expand the scale of financial service subjects, improve the participation of private finance, and then enrich the financial supply subject and capital financing channels and expand the choice of forestry business subjects. Green finance reduces the cost of information flow by building a forestry information platform, promotes regional information sharing, solves the problem of asymmetric market information, alleviates the waste of resources caused by poor information, alleviates the problem of financial constraints in forestry, and solves the problem of misalignment of forestry deadlines. The green effect of green finance can effectively promote the green innovation of forestry production technology, guide and supervise the green production of business entities, reduce energy consumption, and reduce carbon emissions.
From the point of view of control variables, the forestry resource endowment, government support for agriculture, forestry policy reform, and economic development level have a significant positive effect on the enhancement of the forestry green total factor productivity level. Specifically, higher forestry resource endowment means more savings of forestry resources such as forests and wetlands, which can nourish water, purify the air, prevent wind and fix sand, effectively improve the rural environment, create a natural oxygen bar, and improve the living environment of residents. The higher the government's support for agriculture, the more the government allocates toward forestry development, indicating that the government attaches importance to the development of the forestry industry, provides financial and policy support for forestry development, alleviates the uncertainty brought by the long forestry production cycles, and guarantees the longevity of forestry development. The higher the forestry policy reform, the more importance the state attaches to forestry from the economic and legal systems. In recent years, the national collective forest rights system has been reformed continuously, and the state has been promoting the economic development of forestry at the institutional level. A higher level of economic development means more income for farmers, and a better economic infrastructure determines the level of essential equipment, scientific and technological services, and other high-quality conditions to ensure the economic development of forestry. The level of urbanization hurts the green total factor productivity of forestry, and the higher the level of urbanization, the more serious the loss of rural labor force to cities and towns; therefore, the shortage of rural labor force directly hinders the process of forestry production, which is not conducive to the development of forestry.
4.4 Decomposition of spatial spillovers
The effect of W × GF on forestry green total factor productivity in the spatial Durbin model is positive, indicating that the spatial spillover effect of the spatial Durbin model is global, and in order to study the spatial spillover effect of forestry green total factor productivity, the spatial Durbin model is decomposed into effects using partial differentiation as regards the study by Elhorst (2014).
Table 7 shows the direct effect of green finance is 0.1011, which passes the 10% significance level test, indicating that green finance can directly improve the level of green total factor productivity in forestry, i.e., for every unit of green finance enhancement, the green total factor productivity in forestry in the region will be enhanced by 0.1011 units, which once again verifies that hypothesis H1 is valid. Green finance can directly optimize the allocation of resources, reduce the cost of information exchange, reduce the risk of financial investment through the capital effect, guide the flow of funds to the green field, promote the green innovation of forestry enterprises, and then shorten the production cycle, promote the efficiency of forestry production, and promote the development of the local forestry economy. The spatial spillover effect coefficient of green finance is 0.1041, and it passes the 10% significance level test, indicating that the impact of green finance on forestry green total factor productivity has a spatial spillover effect, i.e., for every unit of green finance enhancement, the forestry green total factor productivity in the neighboring areas will be enhanced by 0.1041 units. However, the indirect impact coefficient of green finance is slightly larger than the direct impact coefficient, indicating that the impact of green finance on forestry green total factor productivity is dominated by the spatial spillover effect, according to which hypothesis H2 is verified again. Funds themselves have mobility, can be cross-regional resource allocation, provide financing channels for forestry green projects in the surrounding areas, drive resource elements under the spatial scope of resource allocation, improve the surrounding areas of forestry financial support and accelerate resource sharing, and drive the green development of forestry. Green finance can rely on the digital network to collect forestry information around, build a green information network service platform, which is conducive to the surrounding areas to carry out the credit pledge of forest land, effectively alleviate the credit risk of the surrounding areas, and drive the forestry economic development of the surrounding areas while promoting the flow of information in the region. The surrounding areas will also learn the region's advanced financial knowledge and forestry technology, improve their own infrastructure and industrial structure, form an exemplary role in driving forestry development in the surrounding areas, and promote the formation of a virtuous cycle to improve regional competitiveness.
4.5 Robustness tests
In order to ensure the accuracy of the experimental results and make the results more convincing, we carried out the robustness test by replacing the explained variable and the matrix, and the results are given in Table 8. In this article, green total factor productivity in forestry is split into technical efficiency and technical progress, respectively, and used as explained variables for the robustness test. After replacing the explained variables, green finance on technical efficiency and technical progress passes the 10% significance level test except for the coefficient fluctuation, and the test results are similar to the test results above. After replacing the spatial matrix, the impact of green finance on green total factor productivity in forestry is positive at a 10% significance level except for the coefficient fluctuation, which is similar to the previous test results, indicating that the regression results are more robust. At the same time, in order to exclude possible endogenous influence of variables, the systematic GMM test lagged one period of the explained variable; according to the results of model (9) and model (10), the coefficients of green finance are positive and pass the test at the significance level of 10%, which proves that the variable selection in this article is reliable and scientific.
4.6 Tests for regional heterogeneity
In order to further test the regional differences in the impact of green finance on green total factor productivity in forestry, this paper divides China into the eastern, central, and western regions according to the distribution of China's geographic location and researches the impact of green finance on green total factor productivity in forestry in different regions based on the spatial Durbin model. The results are given in Table 9. The coefficients of direct and indirect effects of green finance in the eastern and central regions are positive and pass the 10% significance level test. However, green finance's direct and indirect effects on forestry green total factor productivity are positive but not significant. From the total effect, it is not difficult to see that green finance's impact on forestry green total factor productivity exists from the east to west, gradually decreasing development law. The reason for the regional heterogeneity may be that the eastern region has mainly economically developed cities, with many forestry universities and research institutes, and the foundation of financial development is solid. The capital effect of green finance can effectively help eastern forestry enterprises carry out strategic upgrading and expansion, improve the environment for the development of the forestry industry, and improve the efficiency of forestry production. The spillover effect of the capital can lead to the development of the forestry industry in the surrounding areas, which can improve the green total factor productivity of the forestry industry. The central region that has China's richest forest and forestry resources has a good foundation for the forestry industry; forestry has the effect of large-scale production; green finance funds to drive the role of the central enterprises can influence the green transformation and then cultivate and plant new types of forests and tree species, shorten the production cycle, and improve the ability to withstand natural disasters; the learning effect of the surrounding areas can lead to the progress of the forestry industry in the region. It is conducive to improve the green total factor productivity of forestry. The western region is mostly pasture, nomadic, and sandy areas; the environment is more severe and not conducive to the growth of common tree species and forest species; the forestry foundation is weak and economically backward; it is challenging to realize the forestry scale operation and the development of green finance in the western region requires much money to solve the environmental problems, cultivate new windbreak forests to resist wind and sand invasion, to a certain extent, and the impact of the forest industry and related industries is relatively weak, while the green total factor productivity of forestry is slow to improve.
4.7 Tests for mediating effects
In order to further test the actual proportion of the forestry industry structure in the green financial impact on forestry green total factor productivity, we constructed a mediation effect model to analyze, and the regression results are given in Table 10. The coefficient in model (15) is 0.1982, which passes the 10% significance level test, which indicates that green finance can optimize the forestry industry structure and the impact of green finance and forestry industry structure on forestry green total factor productivity in model (16) is positive and passes the 10% significance level test, which indicates that there is an intermediary effect of forestry industry structure in green finance affecting the forestry green total factor productivity. The mediating effect can be calculated as 30.46%, proving that hypothesis H3 is valid. Green finance can integrate market resources, provide differentiated services for forestry enterprises, improve the spatial layout of the forestry industry structure in the region, guide the synergistic agglomeration of the forestry industry, promote the change of industrial structure, drive the development of the regional forestry economy, and improve the green total factor productivity of forestry.
4.8 Analysis of threshold effects
In order to further study the role mechanism of green finance affecting green total factor productivity in forestry, this paper continues to construct a threshold model test to verify the role mechanism of human capital in green finance affecting green total factor productivity in forestry.
In this paper, the Bootstrap sampling method is used to test the threshold effect, and if there is a threshold effect, the threshold value and interval are further tested, as given in Table 11. Approximately 500 times of sample self-sampling were carried out; the human capital was taken as the threshold variable; green finance was the core explanatory variable; and single-threshold, double-threshold, and triple-threshold tests were carried out. The F-value and p-value of the single threshold of human capital are 8.62 and 0.0961, respectively, and the F-value and p-value of the double threshold are 15.4700 and 0.0973, respectively, which have passed the 10% significance level test. In construction, the triple threshold is not significant, which indicates that there is a non-linear effect of green finance on green total factor productivity in agriculture, and there is a double threshold. Accordingly, hypothesis H5 can be proved to be valid.
According to the analysis of the results given in Table 12, there is a positive threshold effect of human capital in the process of green finance affecting forestry green total factor productivity. When the level of human capital is lower than 5.87, the development of green finance is not conducive to the level of green total factor productivity in forestry, and the effect is insignificant. When the level of human capital is better than 5.8700 but less than 5.900, for every unit of green finance level, the level of green total factor productivity in forestry increases by 0.0921 units. When the level of human capital is more significant than 5.99, for every unit of green finance level, the level of green total factor productivity in forestry is increased by 1.0266 units. It can be seen that green finance has a marginal incremental effect on forestry green total factor productivity under the regulation of the human capital level. The reason is that when the level of human capital is low, the forestry industry lacks sufficient labor and financial service personnel to meet resources such as water, fertilizer, and capital that are required for the growth of forest species and tree species, and the forest species grow slowly according to the cycle. When the level of human capital increases, it can attract labor and high-quality personnel, improve forestry production efficiency, financial resources, and green production technology so that green finance can avoid forestry production risks, improve the efficiency of capital allocation and service level, and then shorten the forestry growth cycle, reduce production risks, and promote forestry and forestry economic progress. Improving the level of human capital is also conducive to improving the green financial literacy of the operation's main body, reducing the market credit risk, thus reducing the financial risk, improving the financial environment, and promoting the green development of forestry and ecological protection. Accordingly, hypothesis H5 can be proved to be valid.
5 Discussion
According to the conclusion of the study, it can be seen that green finance can significantly enhance the green total factor productivity of local forestry, which is similar to the results obtained by Yin et al. (2021a), who verified that green finance has a spatial spillover effect on the green total factor productivity of forestry. However, considering the spatial factor, this paper verifies that green finance has a spatial spillover effect on forestry green total factor productivity and can increase the level of forestry green total factor productivity in neighboring areas, which proves that green finance not only affects the development of the local forestry economy but also drives the development of forestry in the surrounding areas through capital spillover. This conclusion argues that green finance plays a vital role in forestry and complements the relationship of green finance's impact on green total factor productivity in forestry.
The results of regional heterogeneity show that there are regional differences in the impact of green finance on green total factor productivity in forestry in China at this stage, which is similar to the findings of Li (2021). However, this paper is based on the perspective of green finance and forestry economy, and the content of the study is more targeted. This conclusion proves the vital role of green finance in driving the coordinated development of the region and promotes the development process of green finance in the forestry field.
Analyzing the mediating effect, it can be seen that the structure of the forestry industry is an essential driving force for green finance to influence the green total factor productivity in forestry, which is similar to the results obtained by Wei Xiuhua et al. (2019) and Zhang et al. (2020). However, what is different is that this paper is based on the background of the impact of green finance to explore the influence of the law of green finance on the forestry economy.
Analyzing the threshold effect shows that the impact of human capital on green finance impact on forestry green total factor productivity has a marginal increasing trend, which is similar to the results of Zhang et al. (2022) and Yang et al. (2015), who studied that human capital can affect forestry economic development. However, this paper takes human capital as the threshold variable to study the effect of human capital in green finance on the forestry economy to broaden the research perspective.
This paper focuses on the theoretical mechanism and functioning mechanism of green finance affecting green total factor productivity in forestry, explores the influencing law of green finance and green total factor productivity in forestry from theoretical and empirical evidence, and researches the functioning mechanism of green finance on green total factor productivity in forestry based on the structure of the forestry industry and human capital, which is of great significance for accelerating the green development of the forestry economy and realizing the modernization of agriculture.
6 Conclusion
Based on the panel data of 30 provinces in China from 2006 to 2021, this paper utilizes the spatial Durbin model, the mediation model, and the threshold model to conduct an empirical analysis and then draws the following research conclusions:
(1) Green finance can increase the green total factor productivity in forestry. The direct and indirect effect coefficients of green finance on forestry green total factor productivity are 0.1011 and 0.1041, respectively, which pass the 10% significance level test. At the same time, the indirect effect coefficient is higher than the direct effect coefficient, indicating that the promotion effect of green finance on the green total factor productivity of forestry in neighboring areas is more significant than that on the green total factor productivity of forestry in this region.
(2) The impact of green finance on green total factor productivity in forestry has regional differences, manifested explicitly in decreasing effect from east to west. This shows that the development of green finance is more advantageous in economically developed regions green finance in the eastern region, and central forestry green total factor productivity level enhancement effect is significantly better than that of the western region.
(3) The forestry industry structure has a mediating effect of 30.46% in the green total factor productivity of forestry affected by green finance. This shows that green finance improves forestry green total factor productivity by optimizing forestry industry structure.
(4) There is a double-threshold effect of human capital in the influence of green finance on green total factor productivity in forestry, and it shows the trend of marginal increment. When the level of human capital exceeds the threshold of 5.87, the effect of green finance on forestry green total factor productivity gradually increases.
Although the article has ensured scientificity and rationality of the theoretical explanation and indicator selection in the research process, the article still has many shortcomings that require further improvement and research.
(1) Regarding the measurement of forestry green total factor productivity indicators, considering the difficulty of obtaining non-desired outputs such as forestry pollution, there may be limitations in replacing them with forestry carbon dioxide emissions. Considering the wide range of sources of forestry CO2 emissions and data availability, it is more difficult to measure them, and there may be some errors in the measured results. Second, forestry's green total factor productivity may be biased based on different measurement methods and inputs and outputs. In future research, it is essential to consider incorporating more forestry pollution emission indicators and adopting more accurate measurement methods to compare the results.
(2) Regarding the measurement of green financial development, due to the availability of data on green credit and green bonds, the coverage of the measurement indicators is incomplete, and it is impossible to obtain accurate and complete data, which leads to the consideration of the development situation only from the macro level. Future research should focus more on the precision of green financial data and the improvement of the construction system, collect and organize rural green financial data, incorporate them into the evaluation system, and construct a more comprehensive and complete evaluation system for green financial development.
(3) The article utilizes the macro data on 30 provinces and cities in China to carry out static research and does not consider the development situation of specific cities and municipalities in China from a micro perspective. In the future, we can utilize the microdata to examine the dynamic impact of green finance on the green total factor productivity from a dynamic perspective.
7 Policy recommendations
In today's society, forest protection and forestry reform have become a social hotspot, and the need to realize the green development of forestry requires green finance to provide continuous power. Therefore, this paper presents four policy insights from green finance, forestry industry structure, human capital, and forestry green development.
(1) Scientific innovation of green financial tools to accelerate the development of green finance. To accelerate the innovation and application of forest offset-based development of carbon financial derivatives, enrich the types of carbon finance, and utilize its pricing function to lay the foundation for promoting carbon financial development. To clarify the property rights of forest resources on which carbon emission offsets are based; innovate, research, and develop forest carbon sinks marketable trading technologies; increase investment in carbon financing tools; build a scientific and reasonable trading system for forest carbon sinks; and improve international influence by benchmarking against international standards. To innovate green bonds, green funds, and other financial tools to provide financial security for forest green development, environmental pollution control, clean energy construction, and other projects, focusing on the spatial spillover effect of financial resources, improving spatial distribution, focusing on the spatial balance of urban and rural development, and perfecting China's green financial development.
(2) To rationalize the distribution of forestry resources and accelerate technological progress. To sincerely promote the construction of a forestry-led three-product integrated forestry development system, highlight the leading position of forestry, safeguard the legitimate rights and interests of forestry business subjects, guide the construction of forest parks, ecological wetlands, and other scenic spots, and enhance the added-value of forestry while effectively benefiting farmers. To promote the transfer of forest land, promote large-scale operations of forest land, reduce the degree of forest land fragmentation, improve the market system of forest rights trading, and actively integrate into the public resources trading platform to achieve standardized trading. To strengthen the concept of management according to local conditions and classified implementation, implement differentiated planting and development strategies for collective forests and economic forests in different regions, further improve the development of digital economy–enabled forestry, and give full play to the functions of the digital economy in the confirmation of rights and digital finance, to improve the level of economic development of forestry.
(3) To optimize the structure of the forestry industry and improve the efficiency of forestry production. To strengthen forestry policy support and infrastructure construction for the development of excellent forestry industry, improve laws and regulations related to the protection of forestry property rights, and increase the production enthusiasm of forest owners. In the context of the reform of the collective forest area system and the reform strategy of state-owned forest farms, it is necessary to continue to promote the transformation of the forestry structure led by the forestry industry, to promote the accelerated integration of the primary, secondary, and tertiary industries of forestry, and to improve the level of production of forest farmers. To promote the rights below the local, according to local conditions, to develop the economy of the forestry industry, guide regional innovation and precision, and effectively use the advantages of forest resources agglomeration. The market rationally regulates price fluctuations in the forestry industry and clarifies the reward and punishment mechanism. To actively develop a modern forestry production system, build a green forestry industry chain, and utilize the spillover effect of green finance to drive the development of the surrounding forestry industry. To strengthen resource exchange and information sharing with neighboring forest areas, universities, and research institutes to form industrial linkages, support the weak with the strong, complement each other's advantages, and drive the synergistic development of the forestry industry.
(4) To encourage talents to return to their hometowns to start their businesses and improve the level of the rural labor force. To strengthen the cultivation of forestry talents, improve the knowledge level of farmers, vigorously publicize digital technology and green technology, actively guide farmers to make green technological innovations, and cultivate farmers' low-carbon and environmentally friendly business philosophy. To encourage leading enterprises to set up factories in rural areas, cultivate specialized forestry talents, encourage technicians from research institutes and universities to start businesses in rural areas, and provide supportive policies for specialized technical talents in terms of tax exemption, interest subsidies, and other aspects, to retain talents for rural areas. To strengthen and improve the agricultural vocational and technical education system, encourage farmers to enroll in schools to learn professional technology, cultivate professional and technical personnel to meet the green development of forestry, and improve the level of education in rural areas.
Data availability statement
The raw data supporting the conclusion of this article will be made available by the authors, without undue reservation.
Author contributions
HG: manuscript writing-original draft, review, and editing. ZW: manuscript writing-review and editing.
Funding
The authors declare that financial support was received for the research, authorship, and/or publication of this article. This research was funded by the Social Science Planning Research Programme of Shandong Province, grant number 19CJJJ24.
Acknowledgments
Shandong Province Social Science Planning Research Project (19CJJJ24):Research on the Path Choice of Modern Finance to Support the Development of Real Economy in Shandong Province.
Conflict of interest
The authors declare that the research was conducted in the absence of any commercial or financial relationships that could be construed as a potential conflict of interest.
Publisher’s note
All claims expressed in this article are solely those of the authors and do not necessarily represent those of their affiliated organizations, or those of the publisher, the editors, and the reviewers. Any product that may be evaluated in this article, or claim that may be made by its manufacturer, is not guaranteed or endorsed by the publisher.
References
An, T., and Chen, Y. (2020). Internet reshaping China’s economic geography:micro mechanisms and macro effects. Econ. Res. 55 (02), 4–19.
Autor, D. H., Levy, F., and Murnane, R. J. (2001). The skill content of recent technological change: an empirical exploration. NBER Work. Pap. 118 (04), 1279–1333. doi:10.1162/003355303322552801
Berrone, P., Fosfuri, A., and Gelabert, L. (2017). Does greenwashing pay off? Understanding the relationship between environmental actions and environmental legitimacy. J. Bus. Ethics 144 (02), 363–379. doi:10.1007/s10551-015-2816-9
Cai, D., Dong, Q., Zhu, Y., and Kang, Y. (2022). Industrial agglomeration, demographic structure and green economic efficiency. Statistics Decis. Mak. 38 (07), 112–116. doi:10.13546/j.cnki.tjyjc.2022.07.022
Chen, J., Dai, J., and Zhou, W. (2023b). The impact of heterogeneous human capital on farmers’ economic forest management behavior - an analysis based on the moderating effect of the policy of returning farmland to forest. For. Econ. 45 (02), 20–41. doi:10.13843/j.cnki.lyjj.20230414.001
Chen, J., Song, Li, Ding, S., Wu, M., Zhao, Q., et al. (2023a). Analysis of economic forest industry agglomeration in China based on spatial perspective. J. Nanjing For. Univ. Nat. Sci. Ed. 47 (02), 159–166.
Deng, Y., and Jiang, W. (2023). Impact of urban-rural education resource imbalance on rural industrial revitalization. J. Manag. 36 (01), 33–42. doi:10.19808/j.cnki.41-1408/F.2023.0003
Fan, S., Li, Y., Ma, X., and Liu, H. (2021). An empirical study on the impact of digitization level on the green development of agriculture - based on panel data from 30 provinces in China. World Agric. (12), 4–16. doi:10.13856/j.cn11-1097/s.2021.12.001
Gao, J., and Zhang, W. (2021). The impact of green finance on the ecologization of China’s industrial structure--an empirical test based on a systematic GMM model. Econ. J. (02), 105–115. doi:10.16528/j.cnki.22-1054/f.202102105
Geng, Z., and Wu, S. (2023). Increasing financial support for the development of forestry carbon sinks. China Finance (12), 102.
Jiang, J., Tang, X., and Li, S. (2023a). Research on the impact of rural population aging on agricultural total factor productivity. Chin. J. Agric. Mech. Chem. 44 (02), 230–240. doi:10.13733/j.jcam.issn.2095-5553.2023.02.032
Jiang, X., Niu, Z., Wang, H., and Wang, W. (2023b). Impact of investment in forestry ecological construction and protection on the development of forestry industry. Ecol. Econ. 39 (08), 112–121.
Jin, M., Chen, N., Wang, S., et al. (2023). Does forestry industry integration promote total factor productivity of forestry industry? Evidence from China. J. Clean. Prod. 137767.
Kumar, B., Kumar, L., Kumar, A., Kumari, R., Tagar, U., and Sassanelli, C. (2023). Green finance in circular economy: a literature review. Environ. Dev. Sustain, 1–41. doi:10.1007/s10668-023-03361-3
Kumar, L., Nadeem, F., Sloan, M., Restle-Steinert, J., Deitch, M. J., Ali Naqvi, S., et al. (2022). Fostering green finance for sustainable development: a focus on textile and leather small medium enterprises in Pakistan. sustainability 14, 11908. doi:10.3390/su141911908
Lei, H., and Wang, X. (2020). Environmental pollution, green finance and high quality economic development. Statistics Decis. Mak. 36 (15), 18–22.
Li, J. (2021). Research on rural financial development and forestry green total factor productivity enhancement. Manag. Rev. 33 (03), 84–95. doi:10.14120/j.cnki.cn11-5057/f.20201223.008
Li, X., Cai, Y., and Yuan, S. (2023). A study on the impact of green finance development on agricultural eco-efficiency-based on the perspective of rural human capital differentiation. Finance Theory Pract. (02), 78–86.
Lu, P. (2017). Can industrial agglomeration increase regional total factor productivity? --An empirical analysis based on spatial measurement. Shanghai Econ. Res. (07), 60–68. doi:10.19626/j.cnki.cn31-1163/f.2017.07.007
Lu, Y., and Zhang, C. (2022). Interactive effects of green credit and technological innovation on the structure of China’s forestry industry. For. Econ. Issues 42 (05), 524–531. doi:10.16832/j.cnki.1005-9709.20220170
Lv, J., Sun, J., and Cai, X. (2022). Analysis of the spatial and temporal evolution of green total factor productivity in China’s forestry industry. J. Agric. For. Econ. Manag. 21 (03), 320–330. doi:10.16195/j.cnki.cn36-1328/f.2022.03.34
Ma, G., and Tan, Y. (2021). A study on the impact of environmental regulation on green total factor productivity in forestry--an analysis based on panel threshold model. Agric. Technol. Econ. 313 (05), 77–92. doi:10.13246/j.cnki.jae.2021.05.006
Ma, J. (2015). On building China’s green financial system. Financ. Forum 20 (05), 18–27. doi:10.16529/j.cnki.11-4613/f.2015.05.002
Ma, J., and Li, J. (2021). Research on the impact of inclusive financial development on rural revitalization in China. Regional Financial Res. (02), 5–10.
Marquis, C., Toffel, M. W., and Zhou, Y. H. (2016). Scrutiny, norms, and selective disclosure: a global study of greenwashing. Organ. Sci. 27 (02), 483–504. doi:10.1287/orsc.2015.1039
Ning, Y., Shen, W., Song, C., and Zhao, R. (2021). Research on the strategy of promoting high-quality development of forestry industry. Agric. Econ. Issues (02), 117–122. doi:10.13246/j.cnki.iae.2021.02.011
Oanh, T. T. K. (2023). Sustainable development: driving force from the relationship between finance inclusion, green finance and green growth. Sustain. Dev. doi:10.1002/sd.2808
Ottaviano, G. I. P., and Pinell, I. D. (2006). Market potential and productivity: evidence from Finnish regions. Regional Sci. Urban Econ. 36 (5), 636–657. doi:10.1016/j.regsciurbeco.2006.06.005
Qin, T., Du, Y., Chen, Y., and Wang, S. (2023). Comparison of forestry carbon sink pledge loan financing modes, realistic dilemma and breakthrough direction. Agric. Econ. Issues 517 (01), 120–130.
Rodriguez, C. M. (2017). The growth effects of financial openness and ex⁃change rates. Int. Rev. Econ. Finance 48, 492–512. doi:10.1016/j.iref.2016.12.015
Shaopeng, T. (2022). Measurement of green total factor productivity in China’s forestry industry and its influencing factors. J. Central South For. Univ. Sci. Technol. 42 (08), 202–210.
Shuhua, B., Wang, Y., and Shen, J. (2022). Research on the impact of financial support on forestry technology innovation--an empirical study based on the spatial Durbin model in 31 provinces from 2011 to 2019. For. Econ. 44 (03), 66–77.
Smith, G. S., Ascui, F., O’Grady, A. P., and Pinkard, E. (2021). Materiality assessment of natural capital risks in Australian forestry. Curr. For. Rep. 7, 282–304. doi:10.1007/s40725-021-00147-6
Smol, M. (2022). Is the green deal a global strategy? Revision of the green deal definitions, strategies and importance in post-COVID recovery plans in various regions of the world. Energy Policy 169, 113152.
Song, C., and Xiang, Y. (2012). Research on forestry technical efficiency and its influencing factors--Based on stochastic frontier production function. For. Econ. (02), 66–70.
Sun, H. P., Sun, W. F., Geng, Y., and Kong, Y. S. (2018). Natural resource dependence, public education investment, and humancapital accumulation. Petroleum Sci. 15 (03), 657–665. doi:10.1007/s12182-018-0235-0
Tan, S. (2022). Measurement of green total factor productivity in China’s forestry industry and its influencing factors. J. Central South For. Univ. Sci. Technol. 42 (08), 202–210. doi:10.14067/j.cnki.1673-923x.2022.08.020
Tang, Z., and Li, H. (2017). Empirical analysis of the impact of urbanization on the optimization of forestry industry structure. Res. Agric. Mod. 38 (02), 226–233. doi:10.13872/j.1000-0275.2017.0007
Thomas, V., Wang, Y., and Fan, X. (2001). Measuring education inequality:gini coefficients of education. Washington: World Bank Publications.
Wang, F., Wang, H., Liu, C., Xiong, L., and Qian, Z. (2022b). The effect of green urbanization on forestry green total factor productivity in China: analysis from a carbon neutral perspective. Land 11, 1900. doi:10.3390/land11111900
Wang, Y., Wang, Y., Yang, J., Zhao, T.-Ye, and Zhang, D. (2022a). Impact of specialization and diversification agglomeration in forestry industry on total factor productivity in forestry. For. Econ. Issues 42 (02), 142–150. doi:10.16832/j.cnki.1005-9709.20210256
Wen, Z., and Ye, B. (2014). Analyses of mediating effects: the development of methods and models. Adv. Psychol. Sci. 22 (05), 731–745. doi:10.3724/sp.j.1042.2014.00731
Wu, L., and Zhang, Z. (2020). Impact and threshold effect of Internet technology upgrade on forestry green total factor productivity: evidence from China. J. Clean. Prod. 271, 122657. doi:10.1016/j.jclepro.2020.122657
Xiao, L. (2018). Research on forestry investment and financing platform construction problems. For. Econ. 40 (08), 104–107. doi:10.13843/j.cnki.lyjj.2018.08.019
Xiaojie, W., and Zhang, M. (2019). Mechanism of the role of forestry industry agglomeration on total factor productivity of China’s forestry industry--an empirical analysis based on dynamic spatial econometric model. World For. Res. 32 (03), 67–72. doi:10.13348/j.cnki.sjlyyj.2019.0037.y
Xie, D., Hu, S., and Bao, Y. (2023). Can green finance increase green total factor productivity in Chinese cities - evidence based on 285 Chinese cities. J. China Univ. Geosciences Soc. Sci. Ed. 23 (04), 122–137. doi:10.16493/j.cnki.42-1627/c.20230613.002
Xiuhua, W., Yang, J., and Cao, W. (2019). Interaction effects of human capital, industrial structure and forestry economic growth-an empirical analysis based on collective forest areas in the south. Fujian Forum Humanities Soc. Sci. Ed. (12), 144–153.
Yagura, K. (2020). Rapid diffusion of combine harvesters in Cambodian rice farming: a business analysis. Asian J. Agric. Dev. 17, 71–88. doi:10.37801/ajad2020.17.1.5
Yan, J., and Qi, L. (2022). Realistic dilemmas and practical paths of forestry industry for rural revitalization. For. Ind. 59 (03), 66–68. doi:10.19531/j.issn1001-5299.202203012
Yang, G., Zhang, Y., and Mao, Y. (2015). The impact of human capital on the ecological economic growth of forestry--Based on the systematic cluster analysis of 31 provinces and regions in China. J. Shaanxi Normal Univ. Philosophy Soc. Sci. Ed. 44 (05), 167–176.
Yang, J., Li, J., and Luo, N. (2019). Rural infrastructure, human capital investment and agricultural total factor productivity-an empirical study based on spatial Durbin model. J. Henan Normal Univ. Philosophy Soc. Sci. Ed. 46 (04), 46–52. doi:10.16366/j.cnki.1000-2359.2019.04.007
Yao, Z., and Xia, J. (2023). Green financial policy and green consumption development - based on green financial reform and innovation pilot zone and synthetic control method. China Circ. Econ., 1–15. doi:10.14089/j.cnki.cn11-3664/f.2023.08.009
Ye, C., Sun, C., and Chen, L. (2018). New evidence for the impact of financial agglomeration on urbanization from a spatial econometrics analysis. J. Clean. Prod. 200 (07), 65–73. doi:10.1016/j.jclepro.2018.07.253
Yin, Z., Sun, X., and Xing, M. (2021a). Research on the impact of green finance development on green total factor productivity. Statistics Decis. Mak. 37 (03), 139–144. doi:10.13546/j.cnki.tjyjc.2021.03.030
Yin, Z., Sun, X., and Xing, M. (2021b). Research on the impact of green financial development on green total factor productivity. Statistics Decis. Mak. 37 (03), 139–144. doi:10.13546/j.cnki.tjyjc.2021.03.030
Yue, M., and Zhang, B. (2020). Research on the estimation of capital stock and its allocation efficiency in China’s forestry industry. Financ. Econ. (10), 20–28. doi:10.14057/j.cnki.cn43-1156/f.2020.10.003
Zhang, B., and Lu, J. (2020). Analysis of spatial effects of factor input driving, industrial structure upgrading and forestry economic growth--Taking key state-owned forest areas in Heilongjiang Province as an example. For. Sci. 56 (06), 142–151.
Zhang, J. (2023). The necessity and path analysis of forestry financial innovation--review of case study on innovation and application of forestry financial instruments. For. Econ. 45 (02), 102. doi:10.13843/j.cnki.lyjj.2023.02.001
Zhang, M., and Hu, Y. (2020). The impact of innovative human capital on green total factor productivity in the Yangtze River Delta region-an empirical analysis based on the spatial Durbin model. China Population-Resources Environ. 30 (09), 106–120.
Zhang, Y., Zhou, W., and Li, X. (2022). Analysis of the spatial effect of heterogeneous rural human capital on forestry economic growth--Based on the perspective of new economic geography. For. Econ. 44 (10), 25–43. doi:10.13843/j.cnki.lyjj.20221212.001
Zhao, H., and Huang, A. (2022). Impact of rural informal finance on forestry industry development--an empirical analysis based on panel data from 30 provinces in China. For. Econ. 44 (09), 50–60. doi:10.13843/j.cnki.lyjj.20221109.001
Zhou, F.Fa, Zheng, Yi, and Li, J. (2022). Agricultural insurance development and green total factor productivity in forestry: intrinsic mechanism and empirical test. World Agric. 522 (10), 70–82. doi:10.13856/j.cn11-1097/s.2022.10.007
Keywords: green finance, green total factor productivity in forestry, spatial spillover effects, forestry industry structure, human capital
Citation: Gong H and Wang Z (2024) Study on the impact of green finance on green total factor productivity in forestry—evidence from China. Front. Environ. Sci. 12:1335210. doi: 10.3389/fenvs.2024.1335210
Received: 08 November 2023; Accepted: 19 February 2024;
Published: 11 March 2024.
Edited by:
Claudio Sassanelli, Politecnico di Bari, ItalyCopyright © 2024 Gong and Wang. This is an open-access article distributed under the terms of the Creative Commons Attribution License (CC BY). The use, distribution or reproduction in other forums is permitted, provided the original author(s) and the copyright owner(s) are credited and that the original publication in this journal is cited, in accordance with accepted academic practice. No use, distribution or reproduction is permitted which does not comply with these terms.
*Correspondence: Hao Gong, 18653281397@163.com