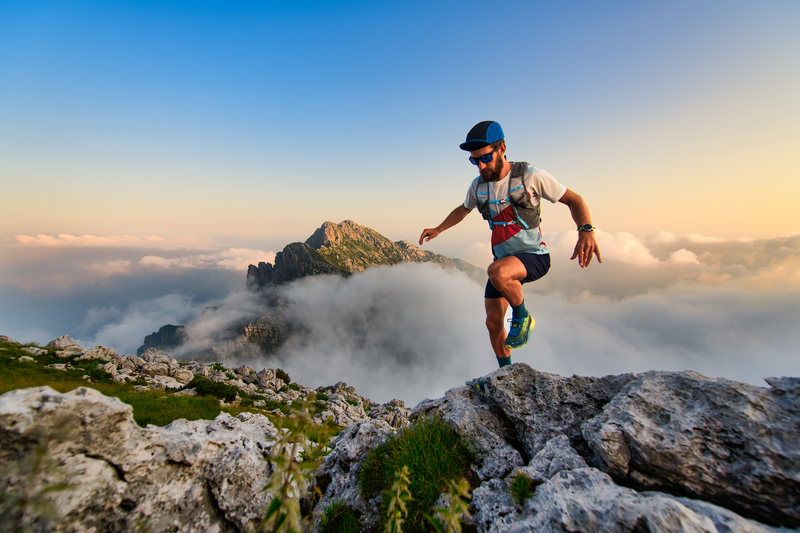
94% of researchers rate our articles as excellent or good
Learn more about the work of our research integrity team to safeguard the quality of each article we publish.
Find out more
ORIGINAL RESEARCH article
Front. Environ. Sci. , 09 January 2024
Sec. Environmental Informatics and Remote Sensing
Volume 11 - 2023 | https://doi.org/10.3389/fenvs.2023.1324742
Despite the research on the responses of grassland vegetation to climate change and topography has advance worldwide, the large-scale importance of these parameters to grassland vegetation greening in arid regions across environmental gradients is unclear. To address this, in this study, we applied MODIS Normalized Difference Vegetation Index (NDVI) data and trend analysis methods to measure the spatial–temporal variation in grassland vegetation greening in central Eurasia. Multiple regression models and hierarchical partitioning were used to quantify the importance of climate [annual precipitation (AP), annual mean temperature (AMT), relative humidity (RH)] and topography [elevation (ELE), aspect (ASP), topographic position index (TPI)] to the NDVI. The results showed that there was a significant increasing trend in the NDVI of meadows, but not other grassland types, from 2000 to 2021 (3.3 × 10−3/year, p < 0.05). Additionally, the responses of the NDVI to climate and topography in deserts were positively correlated with RH, AP, and ELE. Meanwhile, the dependence of NDVI on climate and topography decreased with increasing RH. Under conditions of escalating AMT and AP, RH and ELE independently contributed to explaining the NDVI. However, RH may be the key determinant of long-term NDVI stabilization in arid grassland. These findings underscore the significance of vegetation–climate–topography feedback and can inform the development of more comprehensive and effective climate mitigation and adaptation strategies.
Grasslands cover approximately 40% of the terrestrial biosphere (Hufkens et al., 2016) and provide abundant livestock products and diverse ecosystem services such as carbon cycling, climate regulation, and maintenance of biological diversity (Knapp and Smith, 2001; Maestre et al., 2022). Grasslands are commonly found in arid and semi-arid regions with an annual precipitation of at least 400 mm, and their existence is primarily attributed to climate control (Bardgett et al., 2021). Almost all grassland vegetation, which supports multiple ecosystem functions, has experienced large fluctuations due to frequent climatic and topographic changes over recent decades (Erdős et al., 2022).
Numerous studies have demonstrated that topography, an aggregate indicator of spatial and physical characteristics, can dramatically affect vegetation structure and functioning; elevation, in particular, is frequently associated with alterations in community composition (Fairbanks and McGwire, 2004; Hu and Xia, 2019). Since the last century, temperate grasslands have experienced warming and wetting, especially during extreme weather events, the effects of which are uncertain (Sloat et al., 2018; Styles et al., 2018). One fundamental way through which vegetation and variation in topography interact across all ecosystems is through their marked effect on local temperature and moisture availability, which can affect grassland vitality. However, their interactive effects are complex and unclear and have been largely ignored in these highly heterogeneous grasslands (Erdős et al., 2022).
The Normalized Difference Vegetation Index (NDVI), which is computed from the difference between the reflectance of the near-infrared (NIR) and red bands, is a commonly used measure for evaluating the presence of healthy green vegetation in an observed area (Dutta et al., 2021). It has been effectively used in vegetation dynamics monitoring (such as coverage, productivity, and phenology) and the study of vegetation responses to climatic changes at different scales during the past few years (Mao et al., 2012; Piao et al., 2014). Additionally, this index is the primary input for generating other geophysical products, such as the leaf area index, evapotranspiration, and land cover classification (Cihlar et al., 1991; Iiames et al., 2020).
On the other hand, topography plays a significant role in modulating the effect of climate on grassland vegetation. It affects local temperature and moisture availability, thereby influencing the distribution and characteristics of grassland ecosystems (Fang et al., 2005). Topographical factors, such as slope orientation and aspect, can determine whether a region supports forested areas or steppe-dominant landscapes (Lembrechts and Nijs, 2020). The presence of north-facing steep hills in the Inner Asian steppe region is often associated with forested areas, while south-facing hillsides promote the expansion of temperate steppe vegetation (Dengler et al., 2014). Soil moisture disparities resulting from topography further contribute to the distribution of grassland types (Andrade Diaz et al., 2023; Schrader Patton et al., 2023). Regions with better soil moisture, such as north-facing aspects, typically support mountain meadows or temperate meadow steppes, while south-facing hillsides with higher temperatures and decreased soil moisture favor temperate steppe expansion (Dunne et al., 2003). Additionally, specific topographic features like ravines, erosion gullies, and depressions can create microclimates with milder conditions and a greater supply of soil water, thereby facilitating the occurrence of meadows (Evans, 1960).
Abiotic factors can influence vegetation growth and then indirectly impact the NDVI (Joiner et al., 2018; Sanz et al., 2021). However, there is still no consensus on the relationship between climatic factors and topographic features (Callaghan et al., 2020). Large-scales studies covering a wide range of temperatures, moisture levels, and elevations are particularly scarce (Callaghan et al., 2020; Elsen et al., 2020). Typically, regions exhibiting high temperatures and moisture levels possess greater soil moisture inputs, thereby enhancing the NDVI. Paradoxically, NDVI values in the Mekong River Basin are lower in areas characterized by higher temperatures and moisture levels (Ouyang et al., 2020). This could primarily be attributed to the increased soil respiration rates and heightened soil moisture brought about by elevated temperatures, resulting in reduced oxygen levels with potential detrimental effects on the NDVI (Fu and Sun, 2022). Understanding vegetation changes in grasslands and their drivers is essential for comprehending the trade-offs between grassland function relating to food security and climate mitigation, as well as how grassland management could be improved to meet global climate targets.
Currently, there is insufficient knowledge about the empirical connections between climate, plants, soil, microbial physiological traits, and NDVI. Here, we investigated the relative contribution of climate [annual precipitation (AP), annual mean temperature (AMT), and relative humidity (RH)] and topography [aspect (ASP), elevation (ELE), and topography position index (TP)] to grassland vegetation greening. We collected meteorologic, topographic, and remote sensing data for different environmental gradients in grasslands from 2000 to 2021 in Xinjiang, a central part of the Eurasian and most arid region of China. We investigated whether increased hydrothermal conditions have a positive effect on grassland across environmental gradients and sought to identify the important predictors of NDVI variation. Understanding the changes in grassland vegetation greening is critical for addressing climate change-induced fluctuations in grassland vegetation.
Xinjiang is an autonomous region in northwestern China, situated at the intersection of Central and East Asia. It is the largest province-level division in China, encompasses an expansive area of over 1.6 million km2 and is home to approximately 25 million inhabitants. Geographically, Xinjiang shares borders with a multitude of countries, including Russia, Mongolia, Kyrgyzstan, Kazakhstan, Tajikistan, Pakistan, Afghanistan, and India. The majestic Karakoram, Kunlun, and Tian Shan Mountain ranges dominate substantial sections of Xinjiang’s borders, particularly in the western and southern regions. The study area focuses on the southern desert and northern marsh of Xinjiang (34°25′–48°10′N, 73°40′–96°18′E; altitude: 173–8,052 m; Figure 1). Xinjiang is subject to a semiarid or desert climate, as classified by the Köppen BSk or BWk climate types, respectively (Rubel and Kottek, 2011). The region is characterized by significant seasonal temperature fluctuations, with harsh winters. In the summer, the Turpan Depression frequently experiences some of the highest temperatures in the country, surpassing 40°C. In the northernmost regions, where the mountains reach their peak, winter temperatures routinely plummet below −20°C. The dominant soils are desert soils, brown calcic soils, chernozem, kastanozem, and saline–alkaline soils (Xie et al., 2007). Natural grassland covers 3.95 × 107 ha, or 23.85% of the entire area of Xinjiang (Sciences, 2001). The vegetation in this region is primarily composed of perennial plants such as tussock grasses (Agropyron cristatum, Festuca valesiaca, and Stipa spp.) and Seriphidium (Seriphidium transiliense and S. borotalense). According to China’s grassland classification standards, grassland in Xinjiang is divided into 11 types-alpine desert, temperate steppe desert, temperate desert, alpine steppe, temperate meadow steppe, temperate steppe, temperate desert steppe, lowland meadow, mountain meadow, alpine meadow, and marsh (Supplementary Figure S8). The study area encompasses three primary grassland types: meadow, steppe, and desert, as per the classification standards outlined by China’s grassland classification system (Su, 1996). Owing to the changeable climate and complex topography, the three types of grasslands are staggered with each other from north to south. The specific region of grassland is given by the Xinjiang Uygur Autonomous Region Forestry and Grassland Administration (Figure 1).
FIGURE 1. Overview of the location of the study area. The impacts of climate change and topography on the vegetation of grasslands in Xinjiang in northwest China were investigated; white on the map indicates non-grassland.
The topographic data with a spatial resolution of 30-m were obtained from the Geospatial Data Cloud of the Chinese Academy of Sciences (https://www.gscloud.cn). SuperMap GIS 9D was used to mosaic and mask, as well as to extract the elevation (ELE) and calculate the aspect (ASP) and topographic position index (TPI). The contribution of three climatic variables, namely, AP (mm), RH (%), and AMT (°C), to grassland variability was quantified. The study obtained these variables from 66 meteorological stations across the study region, which were acquired from the China Meteorological Data Service Center website (http://data.cma.cn/en). First, a spatial interpolation technique was used to estimate climate variables for the period 2000–2021. The ANUSPLIN software package was used to interpolated the site-level at a resolution of 250-m (Parra and Monahan, 2008). The package utilizes thin plate smoothing splines, a widely used for the interpolation of climatic variables (McKenney et al., 2006). The accuracy and reliability of the interpolated meteorological dataset have been rigorously evaluated and demonstrated to be of high quality (Mahony et al., 2017). Second, to obtain climate variables in grassland sampling sites from 2000 to 2021, image data were extracted using SuperMap GIS 9D.
The study utilized vegetation greening datasets (NDVI) produced by the MODIS instrument (MOD13Q1 product). The datasets utilized in this study were obtained from the MODIS Terra satellite sensor, providing a spatial resolution of 250 m. These datasets were composited over a 16-day period. Pre-processed MODIS NDVI images were downloaded from the NASA database available at https://ladsweb.modaps.eosdis.nasa.gov. The acquired NASA NDVI time series data spanning from 2000 to 2021 served as the fundamental dataset for analyzing the spatial and temporal patterns of NDVI within the study areas. To mitigate atmospheric effects and cloud contamination present in MODIS biweekly datasets, maximum value compositing (MVC) of daily data was employed (Gutman, 1991). The resultant biweekly NDVI datasets were in a band sequential (BSQ) image format. Image processing software, namely, SuperMap GIS 9D and GEOWAY, was employed to convert the images into raster grid format and extract the specific study areas.
Pearson’s rank correlation coefficient was employed to investigate relationship among interannual variability in AP, RH, AMT, ASP, ELE, TPI, and NDVI for grassland. After log transformation, Pearson’s rank correlation coefficients were calculated for non-normally distributed variables. Correlations with p-values < 0.05 were considered significant. A pixel-based analysis was performed to estimate vegetation trends on an annual time series using the Mann-Kendall test (Mann, 1945). Mann-Kendall statistics were computed to assess temporal trends for all possible combinations of values at each pixel over time (MauriceKendall, 1949). Subsequently, the NDVI time series data were analyzed to estimate the temporal variations within the three distinct grassland types, namely, meadow, steppe, and desert. We used MATLAB 2020b software to perform the Mann-Kendall. Random Forest classification was applied to determine pairwise proximities and measure the similarities between data points relation to the supervised task (Rhodes et al., 2023). “Random Forest classification” is a method used to analyze data and make predictions based on patterns. It works by creating a group of decision trees that work together to classify or categorize data. In this case, the Random Forest classification was used to find out how similar or close different data points are to each other in relation to a specific task that involves supervision or guidance (Speiser et al., 2019). The pairwise proximities refer to comparing each pair of data points to see how alike or different they are. This helps measure the similarities between the data points based on the supervised task. Overall, the Random Forest classification was used to find similarities between data points by comparing them to each other using a grouping of decision trees (Karabadji et al., 2023).
This study utilizes a machine-learning algorithm to enhance the conventional classification and regression tree methods. The algorithm was applied to identify the key factors that significantly influence NDVI and multiple ecosystem functions. The importance of environmental factors was assessed through 1,000 iterations of 10-fold cross-validation, implemented using the “rfcv” function from the R package “randomForest,” with five repetitions (Breiman, 2001). To conduct a comprehensive multi-model comparison (MUM), we employed the “dredge” function from the MuMIn package (Polley et al., 2012). This function systematically generates all possible sub-models and ranks them based on the corrected Akaike Information Criterion (AICc) values. Model averaging was performed for the best models with a ΔAICc < 2 threshold, utilizing the “model.avg” function from the MuMIn package. This process allowed us to obtain the most influential predictor variables and their corresponding coefficients. To determine the relative importance of predictors in driving NDVI, we calculated the relative effect of parameter estimates for each predictor compared to all parameter estimates in the model. Hierarchical partitioning (HP) was performed in R using rdacca.hp (Lai et al., 2022). To assess the direct and indirect effects of climate change and livestock grazing on grassland and evaluate their interaction, we employed piecewise structural equation modeling (SEM) with the piecewiseSEM package (Lefcheck, 2016). The flow chart for the methodology used is shown in Figure 2.
Grassland NDVI values displayed high spatial heterogeneity in Xinjiang. Areas with a high NDVI (>0.6) constituting 17.2% of the entire land area, were predominantly found in the mountainous regions located in the northern and central parts. In the central regions, 34.9% of the grassland NDVI was in the 0.3–0.6 range, while 47.9% of the north basin had a low (<0.3) NDVI (Figures 3A–C).
FIGURE 3. Spatial and temporal variation of climatic factors and vegetation greening in different grassland types between 2000 and 2021 in Xinjiang. (A–C) The spatial distribution of the Normalized Difference Vegetation Index (NDVI). (D–F) The NDVI trend of meadow, steppe, and desert, respectively. The white areas were excluded from the assessment because the focus of this study was limited to natural grassland. (G–J) Interannual climate factors and NDVI variation in different grassland types from 2000 to 2021.
The NDVI of grasslands displayed an overall increasing trend in Xinjiang (Figure 3J). By the Mann-Kendall trend test, regions exhibiting upward trends comprised more than 20% of the overall area, while those with a significantly increased NDVI (9.5%) were distributed in the central mountainous region (Tian Shan Mountains). Moreover, it is noteworthy that a considerable portion, 10.7% of the studied area exhibited a declining trend. Intriguingly, only a minuscule 1.1% of the region demonstrated an immensely significant increased trend in NDVI. This noteworthy trend was primarily observed in the northern sector, with sporadic occurrences also noted in the southern portion of the grassland (Figures 3D–F). We found a spatially and temporally distinct relationship between environmental factors and the NDVI in Xinjiang grassland from 2000 to 2021 (Figures 3A–F, J). During the designated study period, diverse distributions of NDVI were observed across various grassland types (Figures 3D–F). Notably, the NDVI of the meadow exhibited a substantial and statistically significant upward trend (3.3 × 10−3/year, R2 = 0.56, p < 0.05), while those of steppe (3.0 × 10−3/year, R2 = 0.04) and desert (2.8 × 10−4/year, R2 = 0.06) showed no significant trend (Figures 3A–C).
Moreover, spatial variation in climate and topography was found in both the horizontal and vertical dimensions. Horizontally, the AP in meadow, steppe, and desert was significantly higher toward the north than in the south (p < 0.05), and was also higher in the west than in the east (Supplementary Figure S1; Supplementary Table S8). On a vertical scale, there was a significant difference in AP between mountains and plains (p < 0.05), with the central portion of the Tian Shan Mountain range being considerably wetter than the meadow and steppe plains (Supplementary Figures S1A, B). RH exhibited comparable spatial variability and displayed a significant increase in both the mountainous and flat regions to the north compared with that in the south (Supplementary Figure S2). Meadows had a higher RH (78.04%) than deserts and steppes, and the higher RH meadows occur in the central Tian Shan Mountains (Supplementary Figure S2).
The AMT exhibited a spatial pattern opposite to that of AP and RH, with the greatest increase being observed in the southern plains, while a slight reduction was detected in the meadows of the center of the Tian Shan Mountain range (Supplementary Figure S3A). In deserts, however, warming was more pronounced in the northern basin (Supplementary Figure S3C). AP, AMT, and RH also varied from 2000 to 2021, exhibiting an upward trend in grasslands (Figure 3G). Over the past 21 years, the AP increased by an average of 2.06 mm, with the maximum (247.60 mm) occurring in 2016, exceeding the 21-year average precipitation of 105.52 mm (Supplementary Table S1). Although RH is positively correlated with AP, its variation was negligible, and the highest value was recorded in 2017 (60.74%). Additionally, there was a slight increase in the AMT, amounting to 0.12°C, from 2000 to 2021 (Supplementary Table S1).
In Xinjiang, the ELE of grasslands is generally higher in the south than in the north. In this study, the grassland distribution ranged from 6,323 m at its highest point in steppe on the west side of the Kunlun Mountains to −121 m at its lowest point, which was in the Turpan Basin (Supplementary Figure S4; Supplementary Table S2). The grassland area was divided into nine ASPs from south to north according to the orientation of the hillside (Supplementary Figure S5). North was the most common ASP in the three grassland types (meadow: 22.82%, steppe: 21.75%, desert: 22.27%), followed by south (meadow: 17.83%, steppe: 16.64%, desert: 17.10%); flat aspect accounted for <2% of the total grassland area (Supplementary Table S3). The TPI showed marked variation in mountains. The TPI value ranged from 1,127 in the Kunlun Mountains to −748 in the Tian Shan Mountains (Supplementary Figure S6; Supplementary Table S2).
The three grassland types exhibited a highly heterogeneous spatial distribution in our study areas, as illustrated by the radar plots in Figure 4. The highest ELE and NDVI values were found in the wettest meadows. Meanwhile, the driest deserts were distributed in areas with the highest AMT and more complex and variable topography (both ASP and TPI exhibit their maximum values in desert), while steppes were distributed in areas lying between deserts and meadows (Figure 4). Notably, our data suggested that different vegetation types across environmental gradients exhibit varying sensitivities to climatic and topographic factors. Through Pearson’s correlations, we observed a positive correlation between NDVI and AP, RH, and ELE, while AMT on NDVI varied depending on the specific vegetation type and topographical characteristics, resulting in both positive and negative effects (Supplementary Figure S7). To assess the impact of different environmental factors on the different grasslands, we applied ten-fold cross-validation and random forest classification in our study regions to determine the important predictors of changes in the NDVI. Based on the outcomes of the ten-fold cross-validation (Supplementary Table S4), it has been determined that only 4 out of the 6 variables are required to elucidate the variations observed in NDVI throughout this study. Consequently, through the implementation of random forests, the 4 explanatory variables possessing the highest levels of significance were diligently chosen for further investigation and analysis (RH, ELE, AP, and AMT) (Figure 4; Supplementary Table S4). The most important variables were found to be RH and ELE, especially in steppe and meadow. In these two grassland types, the importance of different climatic and topographic factors differed across our datasets. In addition to ELE and RH, AP and AMT were the major environmental factors affecting the NDVI in meadows and steppes (Figures 4A, B, E). In deserts, partial dependency plot analysis indicated that a higher NDVI was associated with higher AMT, RH, and AP, while ELE had a greater effect on the other grassland types (Figures 4C, F). Accordingly, the analysis results from the random forest model indicated that both RH and ELE emerged as the most influential predictors (Figure 4), and climate (AP and AMT) was the most significant driver of NDVI variation (Figure 4; Supplementary Table S4).
FIGURE 4. (A) Radar plots representing the relationship between grassland vegetation greening and environmental factors. (B–D) Pearson correlations between climate, topography and vegetation greening. Red and blue color indicate a positive and negative relationship. (E–G) The importance ranking of features obtained from random forest analysis across various grasslands. (B,E) Meadow, (C,F) steppe, and (D,G) desert. Climatic factors include annual mean precipitation (AP, mm), relative humidity (RH, %), and annual mean temperature (AMT, °C); topography includes elevation (ELE, m), aspect (ASP), and topography position index (TPI).
Based on the four most important environmental factors (RH, ELE, AP, and AMT) predicted by the random forest model, we further quantitatively analyzed the impact of those environmental factors on the NDVI. Analysis based on multivariable stepwise regression and the analytic hierarchy process revealed significant relationships between environmental factors and changes in grassland vegetation (Figure 5; Supplementary Table S5). The model that best explained the NDVI included climate, topography, and interaction predictors (Supplementary Table S5). Within the selected AIC threshold (ΔAICc ≤ 2), only one model, namely, Model 1(Supplementary Table S5) demonstrated a substantial degree of explanatory power in accounting for the majority of the variance observed in NDVI (meadow: R2 = 0.688, steppe: R2 = 0.648, desert: R2 = 0.648; Figure 5). Variation in the NDVI among grassland types was most significantly linked with environmental factors. Model-averaged coefficients were estimated from the six best-candidate models (Supplementary Tables S5, S6). Meanwhile, we also considered the interaction between climate and topography because the NDVIs of the various grasslands are always associated with different topographical and climatic contexts (Supplementary Table S4). The results of MUM, HP, and SEM collectively showed that the dominant drivers of grassland NDVI differed among environmental gradients (Figures 4, 5). For the moistest meadows, the MUM and HP results revealed that interaction between ELE and RH played an important role in regulating the NDVI across the study area. RH × ELE explained 42.45% of the spatial variability observed in the NDVI (Figure 5A). SEM analysis also demonstrated that the NDVI was primarily and directly affected by ELE and RH, with a standardized direct effect of 0.57 and 0.23, respectively (Figure 6A).
FIGURE 5. The importance of climate and topography on Normalized Difference Vegetation Index (NDVI) variation. Multiple rank regression revealed the relative importance of the most important predictors of NDVI variation in (A) meadow, (B) steppe, and (C) desert. The standardized regression coefficients of the models along with their associated 95% confidence intervals are shown for each predictor. *p < 0.05, **p < 0.01, ***p < 0.001. The bar graphs show the relative importance of each group of predictors, expressed as the percentage of the explained variance. Climatic factors include annual precipitation (AP, mm), relative humidity (RH, %), and annual mean temperature (AMT, °C); topography includes elevation (ELE, m), aspect (ASP), and topography position index (TPI).
FIGURE 6. Path diagram for structural equation models (SEM) relating to the influence of climate and grazing variables on vegetation greening in the three grassland types [(A) Meadow, (B) Steppe, (C) Desert]. Climatic factors include annual precipitation (AP, mm), relative humidity (RH, %), and annual mean temperature (AMT, °C); topography includes elevation (ELE, m).
Like meadow, HP results showed that RH × ELE explained a much higher proportion (23.36%) of the variation in the NDVI than ELE (10.41%) or RH (14.68%) alone in steppe (Figure 5B). SEM analysis also confirmed the important role of climatic and topographical factors in NDVI variation, showing that with increasing ELE, greater amounts of AP exert a negative effect on the NDVI (−0.24) (Figure 6B). AP and ELE jointly explained 13.77% of the spatial variation in the NDVI (Figures 4B, 6B). In contrast, in arid deserts, an increase in the AMT generally had a direct negative effect on the NDVI (Figure 6C). Furthermore, AP and RH both significantly increased the NDVI (AP: 14.42% and RH: 15.49%) (Figure 5C); with increasing ELE, greater AP levels decreased the NDVI (−0.35), whereas RH × ELE increased NDVI (Figure 6C).
Through the utilization of bibliography, long-term observations, and various statistical methodologies, we conducted a comprehensive assessment of the temporal and spatial patterns as well as the primary factors influencing the greening of grassland vegetation across environmental gradients in Xinjiang. We found increased temperature and precipitation have resulted in an increase in NDVI within the grasslands of Xinjiang, specifically in the mountain meadows (Figure 3). Besides, there is a relatively close relationship of climate in arid grasslands compared to wet grasslands (desert: 47.24% vs. meadow: 28.09%; Figures 5A, C). This phenomenon might be caused by rise in atmospheric CO2; CO2 has been identified as a significant factor contributing to various environmental changes. One notable effect is the reduction in precipitation sensitivity, which is observed globally due to the decreased leaf-level transpiration caused by elevated CO2 levels, especially in regions with high moisture content. However, it is important to note that in arid regions, this reduction in leaf-level transpiration is counterbalanced at the canopy scale by a substantial proportional increase in leaf area. This phenomenon underscores the complexity of CO2-induced impacts on transpiration and highlights the need for further investigation in dryland ecosystems (Zhang et al., 2022). We also observed that the main drivers changed with environmental gradients: AMT and AP dominantly regulated the NDVI of arid grassland, while interaction between RH and ELE played a more important role in all grasslands. Despite the significant relationships with ASP and TPI, they did not enter the final SEM. These results indicate that microclimate due to changes in topography is not an important factor for vegetation characteristics as previous studies reported (Burnett and Anderson, 2019; Ferreira and Duarte, 2019). Instead, it is possible that other biotic and abiotic factors, such as RH and ELE, may play a more significant role in influencing the development and persistence of vegetation greening across the grasslands of Xinjiang.
Meadow are considered azonal grasslands, and the natural conditions of the environmental gradients where they are located favor vegetation growth to a certain extent (Janisova et al., 2011). In our study period, in meadow located in the moistest area, the NDVI was more closely associated with RH and ELE (p < 0.05), with RH × ELE explaining 42.45% of the spatial variance in NDVI values (Figures 3A, 4A); additionally, as the RH and ELE increased, the meadow NDVI also increased, and this effect was significant (3.3 × 10−3/year, R2 = 0.56, p < 0.05; Figure 3A). This can be explained by the fact that water use efficiency is enhanced with increasing RH, which can lead to higher rates of leaf-level photosynthesis and a decrease in transpiration, resulting in higher NDVI values (Cernusak and Cheesman, 2015). Boosting leaf-level water use efficiency promotes ecosystem health, driving vegetation growth and soil moisture, particularly in water-scarce areas. It paves the way for sustainable ecosystem management and increases the ecosystem NDVI as well as soil moisture availability (Wolf et al., 2016). Previous modeling studies have suggested that indirect effects play a much more important role in arid regions than in humid ones because they can be amplified due to differences in vegetation (Wang et al., 2014; Fatichi et al., 2016).
Furthermore, aside from hydrothermal conditions, other environmental factors also significantly influence the NDVI. Limited vegetation greening in these regions is due to constraints like light, nutrient availability, sink strength, and plant carbon allocation (Zhang et al., 2022). Generally, higher elevations benefit from warming and increased humidity, supporting herbaceous plant growth such as Carex breviculmis and C. alatauensis. However, excessive rainfall decouples transpiration and precipitation, reducing vegetation’s reliance on precipitation and increasing resilience to climate variations (Zhang et al., 2023). The varying strengths of indirect environmental gradients, particularly hydrothermal conditions, contribute to differences in vegetation’s dependence on precipitation along dryness gradients (Alkama et al., 2022).
The minimalistic model employed in this study provides a hydrological perspective to explain the observed phenomena, namely, for wetter meadows, where energy availability is the primary limiting factor, increasing the RH essentially decreases transpiration and the degree of NDVI dependency on other environmental factors. This response is also supported by the similar trends observed in global wetter grasslands for drylands and non-drylands (Higgins et al., 2023). Furthermore, it is important to consider additional factors such as soil texture, rooting depth, water table depth, precipitation seasonality, and stomatal sensitivity to RH. These factors influence ecosystem water availability and precipitation sensitivity, and their interaction with ELE requires further investigation. Additionally, although the trends in RH and ELE may not fully elucidate the contrasting global-scale NDVI trends, they do play a significant role in regulating local water availability. Therefore, studying the interplay between RH, ELE, and NDVI variation at local scales contributes to a more comprehensive understanding of ecosystem dynamics and water availability dynamics.
Despite plant growth is affected by both water availability and temperature, in temperate grasslands, our results indicated that RH had a stronger regulatory influence on the spatiotemporal pattern of NDVI (Figures 5B, C). The fact that the major water sources of the inland steppes and deserts in Xinjiang are brought in by the moist westerly Atlantic air masses explains why the NDVI of both relatively arid grasslands generally decreased from the northwest to the southeast in Central Eurasia, except for the eastern edge that experiences the influenced of the western North Pacific monsoon. This effect of topography was reported to result in higher precipitation in the windward slope of the Tian Shan mountain range (Schiemann et al., 2008), which supported the relatively high NDVI in steppes in the west/northwest of the mountains observed in our study (Figure 3B). Since the late 1990s, the temperature and precipitation in Xinjiang have increased significantly, possibly due to protracted El Niño episodes (He et al., 2020; Zhang et al., 2021). This change in climate explains why steppe and desert NDVIs in the arid areas of our study grasslands increased, but not significantly (steppe: 3.0 × 10−3/year, R2 = 0.04; desert: 2.8 × 10−4/year, R2 = 0.06) in the 2000s (Figure 3G). This phenomenon was also found in five Central Asian grasslands; approximately 14% of the region exhibited a notable and substantial decline in NDVI, with only 5% seeing an increase in the NDVI increase due to drought, which can be identified as the primary factor causing vegetation degradation in this area during the 2000s (Zhang et al., 2016).
Future convection activities in the equatorial Pacific could intensify El Niño events, leading to droughts in central Eurasia and threatening its steppe and desert ecosystems (Ding et al., 2022). Meanwhile, IPCC climate simulations predict a 10%–30% increase in warm nights and precipitation in Eurasia throughout the 21st century (IPCC, 2023). Although quantifying the exact effects of climate change is challenging, future precipitation changes could significantly impact the dynamics and heterogeneity of Xinjiang’s desert NDVI. Grasslands across different environments exhibit distinct NDVI patterns and sensitivities to precipitation. Consequently, future climate change may induce vegetation succession in the grasslands studied, leading to substantial uncertainties in NDVI dynamics within the steppe and desert regions (Figures 4, 5).
Xinjiang has experienced one of the most rapid temperatures increases globally since the 1980s, with a rate ranging from 0.36°C to 0.42°C per decade (He et al., 2020). The warming trend observed in recent years is projected to persist throughout the 21st century, potentially surpassing the global average rate (Huber and Knutti, 2014). In our study, higher NDVIs in deserts were found to be positively correlated with AMT (Figure 4G), the main reason being that temperature changes can affect the length of the growing season (Möhl et al., 2022). Furthermore, it is important to note that with the increase in evapotranspiration, the aridity of the steppes and deserts may be further exacerbated by the warming effects. Additionally, the rising temperatures pose a significant threat to the sustainability of the mountain glaciers found in the Pamir and Tian Shan regions. These glaciers serve as crucial water sources during the summer months for numerous grassland ecosystems in Central Eurasia. Specifically, the riparian shrubs, which exhibit relatively high coverage, are of particular concern as they are predominantly located at the western and north-western foot of the Tian Shan Mountains. It is imperative to address these environmental changes to ensure the long-term viability of these ecosystems (Sorg et al., 2012).
Although we have convincingly demonstrated the pivotal role play by climate and topography in regulating grassland vegetation greening across environmental gradients, there were some uncertainties associated with the auxiliary variables employed to elucidate the spatial pattern of the NDVI. First, meteorological data after 2000 was selected for analysis, which might not reflect actual long-term climate change across the study area. However, based on continuous measurements obtained from the China Meteorological Administration, some studies have shown abrupt changes in temperature and precipitation series after 2000 (He et al., 2020). Additionally, the quality of remote sensing data has improved significantly since 2000. Accordingly, to ensure accuracy in our analysis, we chose data from the 21st century. Moreover, although the aridity index is often used to analyze vegetation changes, the RH data used in this study can represent the comprehensive effects of AP and AMT. And because they are derived from actual monitoring data, they may more accurately reflect the microclimates associated with topography complexity (Waterhouse, 1950).
Second, nomadic pastoralism is a form of pastoralism in which livestock are herded to search for new pastures. In Xinjiang, a traditionally nomadic area, vertical transhumant pastoralism is one of the most enduring and successful prosperous ecological strategies, suggesting that vegetation can adapt to the original grazing intensity even without grazing exclusion practices (Frachetti et al., 2017). Consequently, the impact of livestock grazing on grassland vegetation was not considered in this study.
Third, China’s grassland classification in Xinjiang consists of 11 types (Supplementary Figure S8). For this study, we categorized them into three main types based on environmental gradients to analyze grassland responses to climate change. Future studies should prioritize assessing hydrothermal conditions across different grassland types in Xinjiang.
In conclusion, our findings, derived from a comprehensive analysis encompassing large-scale investigation and model simulation, we observed that the contribution of the interaction between climate and topography to the NDVI in different environmental gradients reached up to 60% across Xinjiang grasslands, highlighting the critical role it plays in representing vegetation greening stabilization. We also found that the dominant factors regulating NDVI were grassland type-dependent, namely, the effects of AP weakened with increased ELE in wetter meadows, whereas the influence of RH strengthened with increasing ELE in arid deserts. Future studies should incorporate the differential regulatory effects of ELE and RH on the NDVI to reduce uncertainties in forecasting vegetation greening dynamics. Our findings also suggest that RH may be the crucial factor in regulating long-term stabilization of NDVI in arid grassland (steppe and desert), with the potential to mitigate the putative positive NDVI-climate feedback under a changing climate.
The original contributions presented in the study are included in the article/Supplementary Material, further inquiries can be directed to the corresponding author.
QX: Conceptualization, Formal Analysis, Methodology, Software, Visualization, Writing–original draft, Writing–review and editing. SA: Data curation, Investigation, Methodology, Writing–review and editing. ML: Funding acquisition, Project administration, Software, Supervision, Visualization, Writing–review and editing.
The authors declare financial support was received for the research, authorship, and/or publication of this article. This research was funded by National Key Research and Development Program of China (2022YFF1300905).
The authors wish to thank the Key Laboratory of Vegetation Ecology of Ministry of Education, Northeast Normal University and College of Grassland Sciences, Xinjiang Agricultural University for support of this project, as well as the reviewers whose inputs greatly improved the quality of this manuscript. We also thank the handling editor and two reviewers for their contributions to improving this manuscript.
The authors declare that the research was conducted in the absence of any commercial or financial relationships that could be construed as a potential conflict of interest.
All claims expressed in this article are solely those of the authors and do not necessarily represent those of their affiliated organizations, or those of the publisher, the editors and the reviewers. Any product that may be evaluated in this article, or claim that may be made by its manufacturer, is not guaranteed or endorsed by the publisher.
The Supplementary Material for this article can be found online at: https://www.frontiersin.org/articles/10.3389/fenvs.2023.1324742/full#supplementary-material
Alkama, R., Forzieri, G., Duveiller, G., Grassi, G., Liang, S., and Cescatti, A. (2022). Vegetation-based climate mitigation in a warmer and greener World. Nat. Commun. 13 (1), 606. doi:10.1038/s41467-022-28305-9
Andrade Diaz, M. S., Piquer Rodriguez, M., and Baldi, G. (2023). Conservation opportunities for threatened paleochannel grasslands in the South American Dry Chaco. J. Nat. Conserv. 71, 126306. doi:10.1016/j.jnc.2022.126306
Bardgett, R. D., Bullock, J. M., Lavorel, S., Manning, P., Schaffner, U., Ostle, N., et al. (2021). Combatting global grassland degradation. Nat. Rev. Earth Environ. 2 (10), 720–735. doi:10.1038/s43017-021-00207-2
Burnett, J. D., and Anderson, P. D. (2019). Using generalized additive models for interpolating microclimate in dry-site ponderosa pine forests. Agric. For. Meteorol. 279, 107668. doi:10.1016/j.agrformet.2019.107668
Callaghan, M. W., Minx, J. C., and Forster, P. M. (2020). A topography of climate change research. Nat. Clim. Change 10 (2), 118–123. doi:10.1038/s41558-019-0684-5
Cernusak, L. A., and Cheesman, A. W. (2015). The benefits of recycling: how photosynthetic bark can increase drought tolerance. New Phytol. 208 (4), 995–997. doi:10.1111/nph.13723
Cihlar, J., St.-Laurent, L., and Dyer, J. A. (1991). Relation between the normalized difference vegetation index and ecological variables. Remote Sens. Environ. 35 (2), 279–298. doi:10.1016/0034-4257(91)90018-2
Dengler, J., Janišová, M., Török, P., and Wellstein, C. (2014). Biodiversity of Palaearctic grasslands: a synthesis. Agric. Ecosyst. Environ. 182, 1–14. doi:10.1016/j.agee.2013.12.015
Ding, R., Tseng, Y. H., Di Lorenzo, E., Shi, L., Li, J., Yu, J. Y., et al. (2022). Multi-year El Niño events tied to the north Pacific oscillation. Nat. Commun. 13 (1), 3871. doi:10.1038/s41467-022-31516-9
Dunne, J. A., Harte, J., and Taylor, K. J. (2003). Subalpine meadow flowering phenology responses to climate change: integrating experimental and gradient methods. Ecol. Monogr. 73 (1), 69–86. doi:10.1890/0012-9615(2003)073[0069:SMFPRT]2.0.CO;2
Dutta, S., Rehman, S., Chatterjee, S., and Sajjad, H. (2021). “Chapter 3 - analyzing seasonal variation in the vegetation cover using NDVI and rainfall in the dry deciduous forest region of Eastern India,” in Forest resources resilience and conflicts. Page. Editors P. Kumar Shit, H. R. Pourghasemi, P. P. Adhikary, G. S. Bhunia, and V. P. Sati (Elsevier).
Elsen, P. R., Monahan, W. B., and Merenlender, A. M. (2020). Topography and human pressure in mountain ranges alter expected species responses to climate change. Nat. Commun., 11, 1974. doi:10.1038/s41467-020-15881-x
Erdős, L., Török, P., Veldman, J. W., Bátori, Z., Bede-Fazekas, Á., Magnes, M., et al. (2022). How climate, topography, soils, herbivores, and fire control forest–grassland coexistence in the Eurasian forest-steppe. Biol. Rev. Camb. Philos. Soc. 97 (6), 2195–2208. doi:10.1111/brv.12889
Evans, R. A. (1960). Differential responses of three species of the annual grassland type to plant competition and mineral nutrition. Ecology 41, 305–310. doi:10.2307/1930220
Fairbanks, D. H. K., and Mcgwire, K. C. (2004). Patterns of floristic richness in vegetation communities of California: regional scale analysis with multi-temporal NDVI. Glob. Ecol. Biogeogr. 13 (3), 221–235. doi:10.1111/j.1466-822X.2004.00092.x
Fang, J., Piao, S., Zhou, L., He, J., Wei, F., Myneni, R. B., et al. (2005). Precipitation patterns alter growth of temperate vegetation. Geophys. Res. Lett. 32 (21), L21411. doi:10.1029/2005GL024231
Fatichi, S., Leuzinger, S., Paschalis, A., Langley, J. A., Donnellan Barraclough, A., and Hovenden, M. J. (2016). Partitioning direct and indirect effects reveals the response of water-limited ecosystems to elevated CO2. Proc. Natl. Acad. Sci. U. S. A. 113 (45), 12757–12762. doi:10.1073/pnas.1605036113
Ferreira, L. S., and Duarte, D. H. S. (2019). Exploring the relationship between urban form, land surface temperature and vegetation indices in a subtropical megacity. Urban Clim. 27, 105–123. doi:10.1016/j.uclim.2018.11.002
Frachetti, M. D., Smith, C. E., Traub, C. M., and Williams, T. (2017). Nomadic ecology shaped the highland geography of Asia’s silk roads. Nature 543 (7644), 193–198. doi:10.1038/nature21696
Fu, G., and Sun, W. (2022). Temperature sensitivities of vegetation indices and aboveground biomass are primarily linked with warming magnitude in high-cold grasslands. Sci. Total Environ. 843, 157002. doi:10.1016/j.scitotenv.2022.157002
Gutman, G. G. (1991). Vegetation indices from AVHRR: an update and future prospects. Remote Sens. Environ. 35 (2-3), 121–136. doi:10.1016/0034-4257(91)90005-q
He, B., Sheng, Y., Cao, W., and Wu, J. (2020). Characteristics of climate change in northern Xinjiang in 1961–2017, China. China. Chin. Geogr. Sci. 30 (2), 249–265. doi:10.1007/s11769-020-1104-5
Higgins, S. I., Conradi, T., and Muhoko, E. (2023). Shifts in vegetation activity of terrestrial ecosystems attributable to climate trends. Nat. Geosci. 16 (2), 147–153. doi:10.1038/s41561-022-01114-x
Hu, M., and Xia, B. (2019). A significant increase in the normalized difference vegetation index during the rapid economic development in the Pearl River Delta of China. Land Degrad. Dev. 30 (4), 359–370. doi:10.1002/ldr.3221
Huber, M., and Knutti, R. (2014). Natural variability, radiative forcing and climate response in the recent hiatus reconciled. Nat. Geosci. 7 (9), 651–656. doi:10.1038/ngeo2228
Hufkens, K., Keenan, T. F., Flanagan, L. B., Scott, R. L., Bernacchi, C. J., Joo, E., et al. (2016). Productivity of North American grasslands is increased under future climate scenarios despite rising aridity. Nat. Clim. change 6 (7), 710–714. doi:10.1038/NCLIMATE2942
Iiames, J. S., Cooter, E., Pilant, A. N., and Shao, Y. (2020). Comparison of EPIC-simulated and MODIS-derived leaf area index (LAI) across multiple spatial scales. Remote Sens. 12 (17), 2764. doi:10.3390/rs12172764
Ipcc (2023). Climate change 2021 – the physical science basis: working group I contribution to the sixth assessment report of the Intergovernmental panel on climate change. Cambridge: Cambridge University Press.
Janisova, M., Bartha, S., Kiehl, K., and Dengler, J. (2011). Advances in the conservation of dry grasslands: introduction to contributions from the seventh European Dry Grassland Meeting. Plant Biosyst. 145 (3), 507–513. doi:10.1080/11263504.2011.603895
Joiner, J., Yoshida, Y., Anderson, M., Holmes, T., Hain, C., Reichle, R., et al. (2018). Global relationships among traditional reflectance vegetation indices (NDVI and NDII), evapotranspiration (ET), and soil moisture variability on weekly timescales. Remote Sens. Environ. 219, 339–352. doi:10.1016/j.rse.2018.10.020
Karabadji, N. E. I., Amara Korba, A., Assi, A., Seridi, H., Aridhi, S., and Dhifli, W. (2023). Accuracy and diversity-aware multi-objective approach for random forest construction. Expert Syst. Appl. 225, 120138. doi:10.1016/j.eswa.2023.120138
Knapp, A. K., and Smith, M. D. (2001). Variation among biomes in temporal dynamics of aboveground primary production. Science, 291(5503), 481–484. doi:10.1126/science.291.5503.481
Lai, J., Zou, Y., Zhang, J., and Peres-Neto, P. R. (2022). Generalizing hierarchical and variation partitioning in multiple regression and canonical analyses using the rdacca.hp R package. Methods Ecol. Evol. 13 (4), 782–788. doi:10.1111/2041-210X.13800
Lefcheck, J. S. (2016). PiecewiseSEM: piecewise structural equation modelling in R for ecology, evolution, and systematics. Methods Ecol. Evol. 7 (5), 573–579. doi:10.1111/2041-210X.12512
Lembrechts, J. J., and Nijs, I. (2020). Microclimate shifts in a dynamic world. Science 368 (6492), 711–712. doi:10.1126/science.abc1245
Maestre, F. T., Le Bagousse-Pinguet, Y., Delgado-Baquerizo, M., Eldridge, D. J., Saiz, H., Berdugo, M., et al. (2022). Grazing and ecosystem service delivery in global drylands. Science 378 (6622), 915–920. doi:10.1126/science.abq4062
Mahony, C. R., Cannon, A. J., Wang, T., and Aitken, S. N. (2017). A closer look at novel climates: new methods and insights at continental to landscape scales. Glob. Change Biol. 23 (9), 3934–3955. doi:10.1111/gcb.13645
Mann, H. B. (1945). Nonparametric tests against trend. Econometrica 13 (3), 245–259. doi:10.2307/1907187
Mao, D., Wang, Z., Luo, L., and Ren, C. (2012). Integrating AVHRR and MODIS data to monitor NDVI changes and their relationships with climatic parameters in Northeast China. Int. J. Appl. Earth Observation Geoinformation 18, 528–536. doi:10.1016/j.jag.2011.10.007
Maurice, G., and Kendall, M. A. (1949). Rank correlation methods. J. Inst. Actuar. 75 (1), 140–141. doi:10.1017/S0020268100013019
Mckenney, D. W., Pedlar, J. H., Papadopol, P., and Hutchinson, M. F. (2006). The development of 1901–2000 historical monthly climate models for Canada and the United States. Agric. For. Meteorol. 138 (1), 69–81. doi:10.1016/j.agrformet.2006.03.012
Möhl, P., Von Büren, R. S., and Hiltbrunner, E. (2022). Growth of alpine grassland will start and stop earlier under climate warming. Nat. Commun. 13 (1), 7398. doi:10.1038/s41467-022-35194-5
Ouyang, W., Wan, X., Xu, Y., Wang, X., and Lin, C. (2020). Vertical difference of climate change impacts on vegetation at temporal-spatial scales in the upper stream of the Mekong River Basin. Sci. Total Environ. 701, 134782. doi:10.1016/j.scitotenv.2019.134782
Parra, J. L., and Monahan, W. B. (2008). Variability in 20th century climate change reconstructions and its consequences for predicting geographic responses of California mammals. Glob. Change Biol. 14 (10), 2215–2231. doi:10.1111/j.1365-2486.2008.01649.x
Piao, S., Nan, H., Huntingford, C., Ciais, P., Friedlingstein, P., Sitch, S., et al. (2014). Evidence for a weakening relationship between interannual temperature variability and northern vegetation activity. Nat. Commun. 5 (1), 5018. doi:10.1038/ncomms6018
Polley, H. W., Jin, V. L., and Fay, P. A. (2012). Feedback from plant species change amplifies CO2 enhancement of grassland productivity. Glob. Change Biol. 18 (9), 2813–2823. doi:10.1111/j.1365-2486.2012.02735.x
Rhodes, J. S., Cutler, A., and Moon, K. R. (2023). Geometry- and accuracy-preserving random forest proximities. IEEE Trans. Pattern Anal. Mach. Intell. 45 (9), 10947–10959. doi:10.1109/tpami.2023.3263774
Rubel, F., and Kottek, M. (2011). Comments on: the thermal zones of the earth by Wladimir Köppen (1884). Meteorol. Z. 20, 361–365. doi:10.1127/0941-2948/2011/0285
Sanz, E., Saa-Requejo, A., Díaz-Ambrona, C. H., Ruiz-Ramos, M., Rodríguez, A., Iglesias, E., et al. (2021). Normalized difference vegetation index temporal responses to temperature and precipitation in arid rangelands. Remote Sens. 13 (5), 840. doi:10.3390/rs13050840
Schiemann, R., Lüthi, D., Vidale, P. L., and Schär, C. (2008). The precipitation climate of Central Asia—intercomparison of observational and numerical data sources in a remote semiarid region. Int. J. Climatol. 28 (3), 295–314. doi:10.1002/joc.1532
Schrader Patton, C. C., Underwood, E. C., and Sorenson, Q. M. (2023). Annual biomass spatial data for southern California (2001–2021): above- and belowground, standing dead, and litter. Ecology 104 (5), e4031. doi:10.1002/ecy.4031
Sloat, L. L., Gerber, J. S., Samberg, L. H., Smith, W. K., Herrero, M., Ferreira, L. G., et al. (2018). Increasing importance of precipitation variability on global livestock grazing lands. Nat. Clim. Change 8 (3), 214–218. doi:10.1038/s41558-018-0081-5
Sorg, A., Bolch, T., Stoffel, M., Solomina, O., and Beniston, M. (2012). Climate change impacts on glaciers and runoff in Tien Shan (Central Asia). Nat. Clim. Change 2 (10), 725–731. doi:10.1038/nclimate1592
Speiser, J. L., Miller, M. E., Tooze, J., and Ip, E. (2019). A comparison of random forest variable selection methods for classification prediction modeling. Expert Syst. Appl. 134, 93–101. doi:10.1016/j.eswa.2019.05.028
Styles, D., Gonzalez-Mejia, A., Moorby, J., Foskolos, A., and Gibbons, J. (2018). Climate mitigation by dairy intensification depends on intensive use of spared grassland. Glob. Change Biol. 24 (2), 681–693. doi:10.1111/gcb.13868
Su, D. (1996). Grassland resources map of China (1:4000 000). Beijing, China: China Science Publishing & Media Ltd.(CSPM).
Wang, X., Liu, L., Piao, S., Janssens, I. A., Tang, J., Liu, W., et al. (2014). Soil respiration under climate warming: differential response of heterotrophic and autotrophic respiration. Glob. Change Biol. 20 (10), 3229–3237. doi:10.1111/gcb.12620
Waterhouse, F. L. (1950). Humidity and temperature in grass microclimates with reference to insolation. Nature 166 (4214), 232–233. doi:10.1038/166232b0
Wolf, A., Anderegg, W. R. L., and Pacala, S. W. (2016). Optimal stomatal behavior with competition for water and risk of hydraulic impairment. Proc. Natl. Acad. Sci. U. S. A. 113 (46), E7222–E7230. doi:10.1073/pnas.1615144113
Xie, Z., Zhu, J., Liu, G., Cadisch, G., Hasegawa, T., Chen, C., et al. (2007). Soil organic carbon stocks in China and changes from 1980s to 2000s. Glob. Change Biol. 13 (9), 1989–2007. doi:10.1111/j.1365-2486.2007.01409.x
Zhang, C., Lu, D., Chen, X., Zhang, Y., Maisupova, B., and Tao, Y. (2016). The spatiotemporal patterns of vegetation coverage and biomass of the temperate deserts in Central Asia and their relationships with climate controls. Remote Sens. Environ. 175, 271–281. doi:10.1016/j.rse.2016.01.002
Zhang, L., Liu, Y., Zhan, H., Jin, M., and Liang, X. (2021). Influence of solar activity and EI Niño-Southern Oscillation on precipitation extremes, streamflow variability and flooding events in an arid-semiarid region of China. J. Hydrol. 601, 126630. doi:10.1016/j.jhydrol.2021.126630
Zhang, Y., Gentine, P., Luo, X., Lian, X., Liu, Y., Zhou, S., et al. (2022). Increasing sensitivity of dryland vegetation greenness to precipitation due to rising atmospheric CO2. Nat. Commun. 13 (1), 4875. doi:10.1038/s41467-022-32631-3
Keywords: climate, topography, NDVI, Xinjiang, grassland
Citation: Xun Q, An S and Lu M (2024) Climate change and topographic differences influence grassland vegetation greening across environmental gradients. Front. Environ. Sci. 11:1324742. doi: 10.3389/fenvs.2023.1324742
Received: 27 October 2023; Accepted: 26 December 2023;
Published: 09 January 2024.
Edited by:
Ana Maria Tarquis, Polytechnic University of Madrid, SpainReviewed by:
Ernesto Sanz Sancho, Polytechnic University of Madrid, SpainCopyright © 2024 Xun, An and Lu. This is an open-access article distributed under the terms of the Creative Commons Attribution License (CC BY). The use, distribution or reproduction in other forums is permitted, provided the original author(s) and the copyright owner(s) are credited and that the original publication in this journal is cited, in accordance with accepted academic practice. No use, distribution or reproduction is permitted which does not comply with these terms.
*Correspondence: Mingzhi Lu, bHVtejIzMUBuZW51LmVkdS5jbg==
Disclaimer: All claims expressed in this article are solely those of the authors and do not necessarily represent those of their affiliated organizations, or those of the publisher, the editors and the reviewers. Any product that may be evaluated in this article or claim that may be made by its manufacturer is not guaranteed or endorsed by the publisher.
Research integrity at Frontiers
Learn more about the work of our research integrity team to safeguard the quality of each article we publish.